DOI:
10.1039/D2CS00894G
(Viewpoint)
Chem. Soc. Rev., 2023,
52, 1983-1994
Nanopores: synergy from DNA sequencing to industrial filtration – small holes with big impact
Received
29th October 2022
First published on 16th February 2023
Abstract
Nanopores in thin membranes play important roles in science and industry. Single nanopores have provided a step-change in portable DNA sequencing and understanding nanoscale transport while multipore membranes facilitate food processing and purification of water and medicine. Despite the unifying use of nanopores, the fields of single nanopores and multipore membranes differ – to varying degrees – in terms of materials, fabrication, analysis, and applications. Such a partial disconnect hinders scientific progress as important challenges are best resolved together. This Viewpoint suggests how synergistic crosstalk between the two fields can provide considerable mutual benefits in fundamental understanding and the development of advanced membranes. We first describe the main differences including the atomistic definition of single pores compared to the less defined conduits in multipore membranes. We then outline steps to improve communication between the two fields such as harmonizing measurements and modelling of transport and selectivity. The resulting insight is expected to improve the rational design of porous membranes. The Viewpoint concludes with an outlook of other developments that can be best achieved by collaboration across the two fields to advance the understanding of transport in nanopores and create next-generation porous membranes tailored for sensing, filtration, and other applications.
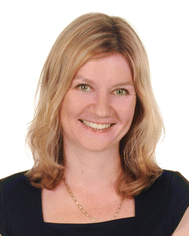
Zuzanna S. Siwy
| Dr Zuzanna S. Siwy received her PhD in 1997 from the Silesian University of Technology, Poland. In 2005, she joined the Department of Physics and Astronomy at the University of California, Irvine. She was a Fellow of the Alfred von Sloan Foundation and received the Presidential Early Career Award for Scientists and Engineers, and the Bessel Award. She is a Fellow of the American Physical Society, and AAAS. Her current research interests focus on using synthetic nanopores as templates for biomimetic channels, preparation of ionic diodes and ionic transistors as well as model systems to discover new physics under nanoconfinement. |
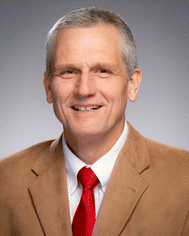
Merlin L. Bruening
| Merlin Bruening is the Donald and Susan Rice Professor of Engineering in the Department of Chemical and Biomolecular Engineering at the University of Notre Dame. He obtained his PhD from the Weizmann Institute of Science in 1995 and served as an NIH postdoctoral fellow at Texas A&M University. Merlin was a faculty member at Michigan State University from 1997 until 2016, when he moved to Notre Dame. His research interests include membranes for ion separations and for protein isolation and quantitation. He has authored or coauthored more than 150 research papers and developed commercial membranes for protein purification and digestion. |
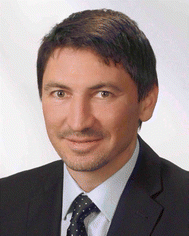
Stefan Howorka
| Stefan Howorka is Professor of Chemical Biology at the Department of Chemistry at University College London (UCL). He obtained his PhD from the University of Vienna in 1999 and performed his postdoctoral research at Texas A&M University. After a 3 year stint at a biotech incubator, he joined UCL and was promoted to Professor in 2016. His research interests include the characterization of biological protein and peptide pores, the design of synthetic membrane nanopores for biosensing, and the development of research tools for membrane biophysics (https://www.howorkalab.com). He is co-inventor and secured a patent on a protein pore technology licensed for widely used portable and low-cost DNA sequencing. |
Introduction
Membranes with single holes have achieved a step-change in portable label-free DNA and RNA sequencing, including the sequencing of COVID samples.1 Single nanopores are also a powerful research tool for examining individual molecules, particles and cells.2,3 In the sensing principle, electrically induced passage of individual analytes through a small electrolyte-filled pore (Fig. 1, left panel) leads to transient detectable increases in electrical resistance due to blockage of electrolyte ions, which in DNA sequencing can distinguish among the very similar nucleobases.4 Research with single nanopores is often inspired by biological channels and pores that feature exceedingly high ion selectivities and fluxes, and additionally function as switches, amplifiers and relay systems for ionic signals.5 Nanopores are therefore used to prepare systems that mimic properties of biological channels and control over ionic transport in solution.6–9 Furthermore, single nanopores provide a model system to unravel new physical and chemical phenomena, transport properties and transport modes that are induced by the nanoconfinement.10–12 Transport is studied for ions, small organic molecules, folded proteins, DNA and RNA, as well as extended organic polymers and biopolymers. Due to their applications in biosensing and biomimetics, single nanopores have been primarily probed in aqueous and well-defined solutions. Depending on the application, single nanopores can have opening diameters of ∼0.3 to hundreds of nm, and lengths spanning from a single atomic layer to the micrometer scale.
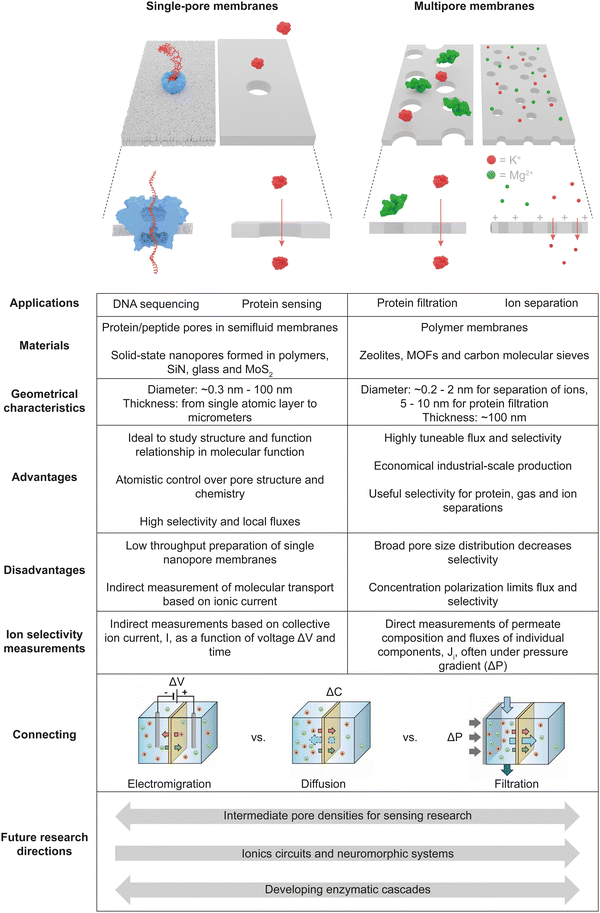 |
| Fig. 1 Dialogue between single nanopore and membrane fields and proposed steps to improve it. The scheme in the Connecting row shows measurements that are most prevalent in the fields of single nanopores and membranes, adapted with permission from ref. 13, copyright 2022 American Chemical Society. The figure of the blue protein pore was drawn using the pdb file published in ref. 14. | |
Multipore membranes are technologically very different from single-pore systems. Applications of multipore membranes may require 1000's of square meters of membranes. The multipore membranes create a 10 billion dollar annual market and are essential in water-based and non-aqueous filtration, gas separations, fuel cells and batteries, and purification of biological materials including small molecules and folded proteins for food processing, biotechnology, and biomedicine.15–18 Within these applications, the membranes function as selective barriers that allow passage of one or more molecular species, while predominantly retaining others on the membranes’ feed side or within the membrane (Fig. 1, right panel). The generic principle of filtration spans from the molecular to the micrometer scale and typically aims for selective separation and high flux across the porous ∼100 nm thick barrier containing sponge-like interconnected voids. In ionic separations, the average size of these voids is between 0.2 to 2 nm.
Despite the unifying principle of nanopores, the fields of single- and multipore membranes can be different in terms of materials, fabrication, and type of measurements. In their very essence, studies of single pores emphasize their defined atomistic structure and its influence on transport, whereas research on multipore membranes aims toward fabricating materials for high-volume separations. Furthermore, transport properties across single nanopores and multipore membranes are probed differently. Single nanopores are probed by ion current, which is a cumulative measure of fluxes of all ions. Consequently, the transport of molecules can be measured only in an indirect fashion by tracking the change in ion current caused when individual molecules pass through the single pore (Fig. 1). By comparison, multipore membranes generate high transport fluxes such that the change in concentrations of transported ions and molecules can be directly measured on both sides of the membrane (Fig. 1). The different approaches also influence how selectivity for transport is measured in both fields. Despite these differences, there is overlap in some topics such as the fundamental physical chemistry underpinning nanoscale transport. Nevertheless, the communication between the two fields is limited, with the exception of some successful cases.19–22
This Viewpoint aims to show that synergistic crosstalk among communities studying single- and multipore membranes can provide considerable benefits. Joint efforts of single-nanopore and membrane researchers could facilitate development of next-generation materials with enhanced functional properties to improve existing or facilitate new applications (Fig. 1).15,19,23 For example, single pores need to expand from aqueous biosensing of a narrow analyte range towards wider environmental, biomedical, or biosafety screening in various solvents, thereby demonstrating the broader value of nanopore sensing. Similarly, new multipore membranes should enable highly selective yet low-energy industrial separations ranging from purification of specific salts and solutes to pharmaceutical production (Fig. 1).23 Establishment of effective communication between the two fields will require advances along the following directions. (i) There is a need to find correspondence between selectivity measurements performed with single nanopores and membranes. This will include the development of new experimental tools to probe selectivity of single nanopores directly, as done in multipore membranes. (ii) New modeling methods are necessary to link existing and new data on transport and selectivity in both single nanopores and multipore membranes.13 (iii) Single nanopore studies have to learn from multipore membranes on using non-aqueous and complex media in order to expand the sensing range. In the following, we first highlight the main advantages and key differences among the single and multipore membranes, then outline the steps to improve communication between the two fields, and finally describe new research directions to develop new next-generation membranes.
Single-pore studies focus on structure-function relationships
Research on single nanopores emphasizes the tunability of pore geometry and chemistry, which in turn controls the interactions of the passing ions and other analytes with the pore walls (Fig. 2A–G). Fig. 2B–G shows examples of single nanopores used in nanopore analytics and fundamental studies of ionic transport in nanoconfinement.24,25 All these structures have well-defined geometrical and electrochemical properties, in some cases with atomistic precision. The protein pore CsgG (Fig. 2B) is used in commercial DNA and RNA sequencing.14 Another biological nanopore, MspA, is also explored for DNA sequencing26 and in the emerging field of peptide and protein sequencing,27 similar to the aerolysin pore.28 Carbon nanotubes29 and pores in 2D materials such as MoS230 also contain structures known with atomistic precision as well as control over openings at the Å level (Fig. 2C and D). Other types of nanopores prepared by top-down approaches with nanoscale precision include silicon nitride nanopores drilled by focused ion31 or electron beams,32 polymer and silicon nitride nanopores fabricated by track-etching,33 and glass nanopipettes34,35 (Fig. 2E–G). Single nanopores of tunable diameter down to the nanometer scale can even be prepared without expensive large-scale facilities by a process of dielectric breakdown.36,37 All of these nanopores are used for analytical applications, in fundamental studies to help understand highly selective transport of small ions, and to uncover the physico-chemical principles underpinning transport across defined nanoscale space.38 Below we describe the vital role of size control and tuning of pore-wall properties in applications of single nanopores.
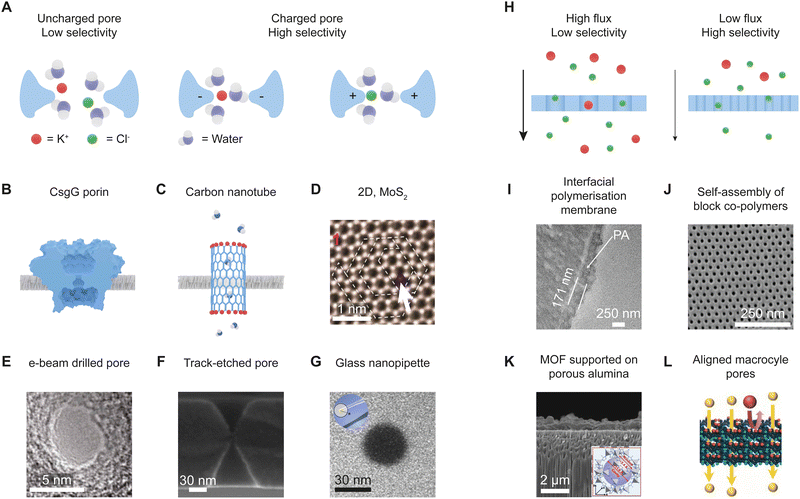 |
| Fig. 2 Examples of single nanopores and porous membranes. Studies of single nanopores (A–G) focus on tuning the pore diameter, charge, and chemistry to understand how these variables affect analyte fluxes and selectivities. The nanopore opening and electrochemical characteristics tune ion selectivity. (A) A wide, uncharged pore is not ion selective, whereas narrower negatively or positively charged nanopores transport counterions and inhibit transport of co-ions. (B) Bacterial porin CsgG. The figure was drawn using pdb file published in ref. 14. (C) Carbon nanotube with an opening of <1 nm.39 (D) A single layer of MoS2 with electrochemically removed individual atoms. Adapted with permission from ref. 30. Copyright 2015 American Chemical Society. (E) A nanopore in silicon nitride drilled by an electron beam in TEM. Adapted with permission from ref. 32. Copyright 2003 Springer Nature. (F) A nanopore in silicon nitride fabricated using the track-etching technique. Adapted with permission from ref. 40. (G) Glass nanopipette whose opening was controlled by e-beam irradiation. Adapted with permission from ref. 41. Copyright 2013 American Chemical Society. (H) The focus in multipore membranes is to tune the size but also optimize larger-scale separation performance. (I) A membrane obtained by interfacial polymerisation. Adapted with permission from ref. 42. Copyright 2021 American Chemical Society. (J) A membrane obtained by self-assembly of block copolymers; SEM image courtesy of Prof. William Phillip, University of Notre Dame.43,44 (K) Metal Organic Framework (MOF) deposited on a porous support. The inset shows the crystal structure of ZIF-8. Adapted with permission from ref. 45 (L) Membranes with oriented macrocyle nanopores whose opening can be tuned with Å precision. Reprinted with permission from ref. 46. https://creativecommons.org/licenses/by/4.0/. | |
The importance of atomistically tight interactions between passing molecules and chemically defined pore walls is perhaps most clear in the remarkable ionic and molecular selectivities of <1 nm-narrow biological channels. For example, potassium channels transport K+ ions 10
000 times more rapidly than Na+ ions across cellular bilayer membranes,47 whereas aquaporin channels transport more than 109 water molecules per second with exclusion of ions.29,48 Ion selectivity controlled by pore diameter was also observed with pores in synthetic 2D materials49–51 as well as in carbon nanotubes,52 where the energetic penalty of ionic desolvation governs transport selectivity. Pores used for DNA analysis allow transport of single-stranded DNA but are too narrow to accommodate double-stranded DNA molecules.
The desired interactions of pores with target molecules and ions can be engineered by attaching molecular recognition sites at the pore walls.9,12,53,54 Chemically specific interactions can lead to selectivities even when the pore radius is larger than the target molecule. For example, placing a ring-shaped adaptor inside the lumen of protein pores can help to recognise organic analytes that without the ring would not be detected.55 Pores decorated with double-stranded DNA can detect even a single-base mismatch of DNA molecules present in solution.56 Attachment of crown ethers, antibodies, DNA strands, or aptamers9,12,53,57,58 leads to nanopores that either selectively transport or detect specific analytes.
Controlling the surface charge on pore walls gives rise to electrostatic filters that transport only counterions. The pores are often significantly wider than the diameters of the ions. Nanopores with controllable surface charge have become a template to fabricate biomimetic channels.7,8,59 Such nanopores can function as ionic switches and transport ions most efficiently in one direction.8 Transport through charged nanopores depends strongly on the pore shape. For example, cylindrical, conical and hour-glass shaped nanopores give very different current–voltage curves.8,60 Even bending nanopores, as done with carbon nanotubes embedded in elastomeric membranes affects current–voltage curves such that curved carbon nanotubes exhibit ion current rectification.11,61
For multipore membranes the focus is on maximizing flux and selectivity through an ensemble of pores
In contrast to the focus on structural and electrochemical details of individual nanopores, separation membranes contain a distribution of effective pore sizes. Yet, effective membrane separations require tuning the size distribution of the pore opening, considering the trade-off between maximizing flux and selectivity (Fig. 2H).15 The majority of commercial membranes are prepared by interfacial polymerisation62 (Fig. 2I) and phase inversion.63 These membranes are often highly porous with tortuous and interconnected pores, which makes pore-diameter characterization challenging. The transport mechanism in interfacially polymerised nanofiltration membranes may include some combination of transport through nm-sized pores and solution-diffusion. Phase-segregation methods, where block copolymers self-assemble into thin films, can lead to nearly monodisperse pore diameters (Fig. 2J).43,44 Pores in these membranes can be tuned from 10 to 100 nm, and reach values as low as 1.0 to 1.5 nm with copolymers featuring polyelectrolytes. Membranes with well-defined pores but lower porosity are prepared using heavy-ion irradiation and etching.33 Other nearly monodispere pores are accessible using zeolites,64 metal organic frameworks (MOFs)65 (Fig. 2K), aligned macrocycle pores (Fig. 2L),46 and carbon molecular sieves66 as well as graphene oxide67,68 and channels obtained using graphene sheets as spacers.69 Advantages of membranes with monodisperse nanopores in separation of solutes with similar sizes have recently been analyzed in detail.70
However, membranes with well-defined and monodisperse pores are currently much too expensive for most practical separations. Research into forming membranes containing well-defined free-volume-based channels, such as those in polymers of intrinsic microporosity, may provide one means to more readily create very small pores in polymer membranes.71,72 In these membranes, inefficient packing of polymer chains creates porosity, i.e. unoccupied free volume through which analytes may traverse the membrane. Methods to increase free volume and create selective diffusion pathways are common in gas-separations.73,74 However, transport channels need to connect and have diameters of >1 nm in solution-based separations. More research into polymer assembly through the use of small removable porogens could lead to selective transport pathways. Polymer imprinting provides some selectivity, but the highly cross-linked films may not prove highly permeable unless it is possible to imprint channels. Use of imprinted nanofibers may help in this regard.75
Despite issues with heterogeneously sized pores, selectivity can be improved by taking advantage of the steric and electrostatic interactions at the nanoscale, as done in single nanopores with tunable pore size and chemical properties of the pore walls.15,23 However, most ion-exchange membranes contain pores with diameters significantly larger than the ions so selectivity among counterions is modest. Depositing additional layers on the membrane surface can significantly enhance selectivity. For example, adding a layer with positive charges enhanced membrane selectivity between potassium and magnesium ions through increased rejection of magnesium ions.76 Alternating adsorption of polycations and polyanions further improves selectivity and can yield >99% pure Li+ in separations of Li+ and Mg2+.76,77
Separation of ions is, however, highly complex when the pores have sub-nanometer diameter such as in cellulose acetate membranes that are uncharged. To resolve the complexity, machine learning was employed to understand how up to 126 variables influence selectivity and the thermodynamics of transport of 18 different anions.78 Anion selectivity was heavily influenced by interactions of passing ions with the membrane, underpinning the key entropy–enthalpy compensation. Furthermore, the free energy barriers for transport of the anions were mostly determined by the electrostatic interactions between the charge-neutral pore and the polyatomic anions with non-uniform charge distribution. The study emphasizes that tuning ionic selectivity under extreme nanoconfinement, such as by introducing charged walls, requires careful consideration of the thermodynamics of ionic transport.
An alternative route to control selectivity in porous membranes is liquid-gating technology. The approach is used for separation of fluids and gases. It is based on infiltrating porous membranes with a functional liquid that can repeatedly open and close the membrane pores via pressure change in response to various external stimuli.79–81 Porous confinement stabilized the infiltrating liquid through capillary and van de Waals forces. The fluid to be transported can enter the pores at a threshold pressure value that is fluid dependent, rendering the liquid-gating membranes selective. Liquid-gating membranes are also protected from fouling due to the defect free and lubricating liquid interface.79,82
Bringing single-nanopore and membrane fields together relies on progress in experiments and theory
Enhancing a dialogue between single-nanopore and membrane communities is very important, because it would lead to new developments in both fields (Fig. 1). As an example, single nanopores could be used as a testbed for novel solute/solute selectivity mechanisms that could be introduced into membrane systems. New mechanisms may enable design of membranes that offer high selectivity between ions of the same charge or between uncharged molecules, which is a major challenge for the membrane field.23 Additionally, dialogue between these fields may help to extend single-nanopore applications to non-aqueous and complex media, where membrane separations already occur. If nanopores functioned in a wide range of media, nanopore analytics could be extended to analytes that are not soluble in water. Probing transport of ions and molecules under nanoconfinement in non-aqueous media would also help elucidate the structure of the solid/liquid interface, which is crucial for detection and separation. A constructive communication will, however, have to address the difference in experimental and modeling techniques used for single nanopores and mulipore membranes. Achieving this requires (i) to find the relationship between selectivity measurements performed with single nanopores and with membranes,13 (ii) to integrate new measurement tools for both systems, and (iii) to develop modeling methods that link existing data from both fields to describe transport in both single nanopores and membranes.
Unifying experimental measurements in single nanopores and membranes
One of the biggest differences between single nanopores and membranes is the way transport is characterized. Single nanopores are probed electrochemically by recording ionic current passing through a pore.7,8 In sensing, transient blocking of current can denote the presence of a specific analyte.3 Determination of the transport selectivity between two different ions often relies on comparing the magnitudes of ion currents in solutions of the two different salts. Hindered transport of a specific ion leads to a lower current.58 Ionic selectivity can also be quantified using the potential difference developed across an ion-selective membrane in contact with different salt solutions on the two sides of the membrane.5 Elucidation of ion selectivity based on ion currents recorded in mixtures of salts is difficult because the ion current is a sum of fluxes of all ions in the solution.
By comparison, experiments with separation membranes frequently use mixtures as well as a pressure gradient to drive transport. Thus, the membrane separates a complex feed solution from a permeate. Analysis of the permeate yields the concentration of each species and selectivity in the mixture. This approach is not applicable to single nanopores due to the miniscule flow through an individual pore which is in the range of femto to attoliters per second. As pointed out recently,13 extrapolation of transport properties of individual nanopores to membranes containing many nanopores may not be straightforward. The same membrane can exhibit very different selectivities when probed using the two different measurement approaches. In a striking example, a reverse osmosis membrane that highly rejects all ions in pressure-driven transport conducts ionic current once a transmembrane potential is applied.83
An increased understanding of the interrelation between electrically and pressure-driven transport could result from single-nanopore studies with a viscosity gradient that leads to small pressure differences,35,84,85 streaming-current measurements38 or experiments where electrokinetic flow is counter-acted with pressure-driven flow.35,86,87 Establishment of standardized protocols for measuring selectivity, including flow rate, pressure and voltage range as well as ionic concentrations, could also link data collected for single nanopores and membranes.13 Finally, new experimental approaches may enable performing the same selectivity measurements with both single nanopores and membranes. For example, modified mass-spectrometry measurements, where ions are delivered from a nanopore directly into high vacuum and focused, could potentially detect ions in the permeate of individual nanopores.88 This approach already showed promise in detecting ions from aqueous solutions passing through individual pores with ∼100 nm diameters.
Bridging modeling of single nanopores and membranes
Modeling approaches applied to single nanopores and membranes are different as well. Due to the atomistic knowledge of the structure of single nanopores, the distribution of all molecules in the nanopore, including solvent, are available. This allows linking of the structure with measurable quantities such as ion current and current–voltage curves.89 First principles approaches with fundamental quantum mechanical laws describe solvation of ions under confinement, the distribution of ions, interactions at a solid/liquid interface and their consequences for ionic selectivity.52 Molecular dynamics (MD) simulations provide an all-atomistic picture of transport through nanopores and are crucial in understanding DNA and protein sequencing with nanopores as well as the selectivity of biological and solid-state nanopores.27,90,91 Single nanopores are therefore a perfect testbed for probing new selectivity mechanisms.
In contrast to atomistic simulations of single pores, modeling for multipore membranes often focuses on steric exclusion that predicts selectivity when the size of the solute, r, approaches the pore radius, R. The partition coefficient, K, can be approximated as:
where
cmi and
csi are the concentrations of species
i in the membrane pore and solution, respectively.
R is the average pore opening determined using adsorption–desorption, gas permeability or other techniques.
92 Ion selectivity is often described through steric, electrostatic, and dielectric interactions.
93,94 Further efforts aim to develop atomistic and coarse-grained modeling tools that apply to membranes and membrane processes. For example, atomistic simulations were already applied to describe anion selectivity and the structure of ion-exchange membranes,
95 as well as the structure and transport through polyamide reverse osmosis membranes.
96–100 However, determining the representative structure in amorphous, heterogeneous membranes is still a challenge.
Simulations will play a crucial role in bridging the two fields, such as by finding the relationship between electrochemical and pressure-driven measurements in single nanopores and membranes, respectively. Modeling will also enable scaling of the physical mechanisms that underlie selectivity in single nanopores into large-scale membranes. This scaling up process will, however, require careful consideration of phenomena that occur only in membranes due to the array of nanopores. Concentration polarization is one of the most important effects that limit the performance of membranes and is less acute in single pores.101 In this phenomenon, concentration gradients develop in boundary regions outside of a pore so that ions or molecules rejected by the membrane accumulate near the pore entrance (Fig. 3B). The phenomenon results from a combination of diffusion, convection, and electromigration but depends on the pore densities (Fig. 3B). If the pore density is low, the extent of concentration polarization is relatively small because diffusion occurs 3-dimensionally away from the pore to maintain steady state. In contrast, at high pore densities one-dimensional diffusion of species away from the pore leads to relatively large molecular accumulations. For charged pores, high ionic strengths near the pore surface may decrease ion exclusion from the pore. The effect of concentration polarization may be partly mitigated by designing new selectivity mechanisms that are independent of salt-concentration. Alternatively, one could design membranes with a lower porosity to mitigate the effect of concentration polarization through hemispherical diffusion.101 However, this will require pores characterized by high transport rates, such as carbon nanotubes, and the density of nanopores needed for practical fluxes may still be too high to avoid significant concentration polarization.101 In yet another approach, larger pores filled with polyelectrolytes may help to rectify concentration polarization in electrically driven transport.102 Modelling is important to optimize such designs and determine what is possible.
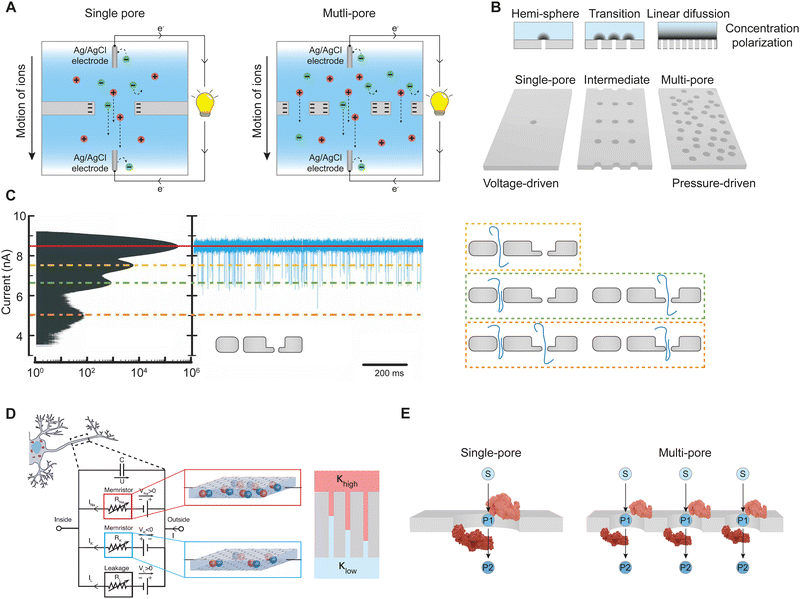 |
| Fig. 3 New directions of research facilitated by a dialogue between the fields of single nanopores and multipore membranes. (A) Combined ideas from the fields of single nanopores and membranes led to nanoporous osmotic power generators that utilize salinity gradients and ion-selective membranes. Adapted with permission from ref. 103. (B) Low-density arrays of nanopores could enable establishment of a relationship between selectivities measured through conductance and in a pressure driven mode and104 mitigate concentration polarization. Performance of single nanopores, low-density arrays and membranes with intermediate porosity will be influenced by concentration polarization to a different extent. Schemes of diffusion and concentration polarization shown on top have different profiles during flow through membranes with different pore densities.101 Darker areas in the solution boundary layer represent higher concentrations of the rejected solute. The concentration gradient is much larger in linear diffusion. (C) A sensing system composed of two nanopores with different thicknesses creates multiple blockage levels corresponding to different configurations of DNA molecules (unfolded and folded) passing through one or both nanopores. Each nanopore creates a distinct current signal enabling making correspondence of each current blockage to the number and configuration of the molecules. Adapted with permission from ref. 105. Copyright 2021 AIP Publishing. (D) (left) Neuromorphic systems are created with memristors i.e. components whose resistance depends on the past states of the system. From ref. 106 Adapted with permission from AAAS. (right) Robust ionic memristors can be created based on nanopores placed in contact with two immiscible liquids of different conductivities, K.107 (E) Enzymatic cascades based on nanopores enable placing two or more enzymes in close proximity to each other decreasing diffusion distances between the enzymes. The substrate (S) of the first enzyme yields a product (P1) that becomes a substrate for the second enzyme. | |
Moving single nanopores into non-aqueous and complex media
Applications of single nanopores have thus far focused on well-controlled solutions prepared with pur water. The emphasis on aqueous solutions stems from applications of single nanopores in biological sensing and biomimetics. A dialogue between single-nanopore researchers and membrane specialists could enhance the translation of single nanopores to new operating conditions, non-aqueous solvents and analytes. There are already reports on unusual transport properties of nanopores in contact with aprotic organic solvents such as acetonitrile and propylene carbonate.108,109 When in contact with silica pores, these solvents form a highly organized structure that persists over a few nanometers. A multipronged experimental and modeling approach revealed that the organized solvent structure, not the solid properties, determines the distribution of ions and electric potential at the interface.108 There has also been interest in understanding solid/liquid interfaces in ionic liquids together with ion transport through these liquids in nanoconfinement,110 and using them as media that facilitate sensing.111
Introducing complex solutions to single nanopores can, however, be difficult because of material instabilities and nonspecific adsorption, or fouling. Fortunately, studies of membrane separations provide a wealth of methods for combatting biological fouling, including pretreatment, sparging or vibrating of membranes, periodic backflushing, addition of antifouling agents, controlled crossflow, restricting transmembrane solution flux, and modifying surfaces to limit adsorption.112 Nanopore sensing in complex biological fluids will likely require a combination of antifouling methods such as surface modification, pretreatment, and pulsed backflushing with electric fields as well as machine-learning aided analysis of the recorded data.113 Pore walls can be passivated with polyethylene glycol chains114 or lipid bilayers115 to prevent nonspecific adsorption. Fouling can also be mitigated using the liquid-gating technology mentioned above, where a porous membrane is infused with a functional liquid that creates a smooth lubricating liquid layer on the pore walls.80,82
New research directions
Increased communication between the single-nanopore and membrane fields will undoubtedly enhance research in both areas. As described in the previous section, this dialogue would challenge the scientific community to develop new experimental techniques applicable to both fields, engage in multiscale modeling of membranes, use single nanopores as a model system for investigating new selectivity mechanisms, and encourage single-nanopore groups to experiment with applications in non-aqueous media (Fig. 1). The dialogue could, however, also lead to new research directions and next-generation materials, as described below. We start (i) with a successful example of collaboration between single and multipore fields. Next, we discuss the following challenging questions that are best resolved by scientists from both fields. (ii) Can next-generation nanoporous materials with intermediate pore densities be built to determine selectivity via electric and pressure gradients to correlate data from single-nanopore and membrane fields? (iii) Can membranes with intermediate pore densities create biomimetic ionic circuits for new sensing applications? (iv) How can single and multipore systems be harnessed to couple pore transport with multi-step chemical transformation?
Successful dialogue led to osmotic energy conversion systems
The joint effort for a new reserach direction can follow a successful example. Combining expertise in single nanopores and membranes has previously led to osmotic energy conversion which achieves a very high density, at least in single-nanopore systems.19,116 This blue energy takes advantage of natural salinity gradients, such as those between sea water and river water, to create electrical energy using nanoporous structures that transport only cations or anions (Fig. 3A). The feasibility of this self-powered nanosystem was demonstrated by powering a MoS2 transistor with osmotically driven current densities.20 To achieve practical levels of osmotic energy harvesting, the single-nanopore prototype20,117 was later scaled up to membranes.21 Developing the concept of osmotic energy conversion also benefited from biomimetic single nanopores that were designed to function as ionic diodes.8 When scaled up into membrane systems, diode-like membranes exhibited improved performance compared to membranes that contained only one polarity of surface charge.22 However, predictions of osmotic power based on single nanopores are likely overoptimistic and unattainable in membrane systems due to concentration polarization and the need for water pretreatment to limit fouling.118 Still, osmotic energy conversion remains an active research topic aiming to utilize this renewable energy source.
Intermediate pore densities for new sensing and addressing concentration polarization
As other research direction, dialogue between the single and multipore fields can lead to new hybrid membrane formats that feature intermediate pore numbers from ten to thousands (Fig. 3B). Investigating the unexplored pore densities between single-pore devices and separation membranes can yield measurable fluxes in uniform pores. This should help to bridge gaps in experimental and theoretical methods to describe transport through single and multipores. Low-density arrays with well-defined nanopores will allow both conductance and pressure-driven experiments to relate the selectivities under the two conditions to nanopore structure and to each other.
As other advantage, low pore densities reduce concentration polarization (Fig. 3B). The effectiveness of these low-density arrays will depend on the polydispersity of their pore diameters, but arrays with desired uniform pores can be made with biological channels or fabricated with helium-ion microscope milling.104 Low-density arrays with tunable positioning of nanopores118 can help understand the role of concentration polarization in transport through individual nanopores and small arrays.101 Methods to mitigate transport limitations posed by concentration polarization can then be explored. For example, placement of a surface electrode was predicted to change local concentrations and increase ionic transport.119 Introduction of gates will also induce different transport properties in otherwise identical pores due to inhomogeneities in the local concentrations.
Low-density arrays of nanopores are further expected to enable new sensing mechanisms using, for example, channels with different openings and/or geometries. A two-nanopore system containing nanopores of the same diameter but different thicknesses provided a proof of principle of this idea.105 When applied in sensing, signals from each pore can be easily distinguished from one another (Fig. 3C). Thinking forward, such nanopore arrays can offer a more comprehensive characterization of an analyte than a single pore system, because the same analyte will be probed with different nanopores in one experiment. For example, if one nanopore is much narrower than the other, one can observe different degrees of unfolding of molecules such as DNA and proteins.
Ionic circuits and neuromorphic systems
In extended approaches, membranes containing a few different types of nanopores could be connected to create new functional materials. For example, the membranes could be connected in parallel or in series to form reconfigurable ionic circuits.120 It would be of interest to embed nanopores with different chemistries and transport properties in the same membrane. This should achieve propagation of signals in space and time, similar to action potentials in biology.5,106 To propagate signals over the micrometer scale and larger distances, one could pattern multipore membranes with patches that differ in chemical characteristics. The first ionic circuits based on nanopores were already reported, including circuits based on ionic diodes that function as bridge rectifiers to turn alternating into direct current.121 Other circuits with diodes and load capacitors are possible.122 Recently, an ionic neuromorphic engineering system was designed with ionic memristors as key components (Fig. 3D, left panel).106 The memory effects stemmed from monolayer electrolytes that facilitated formation of transient ion clusters. Formation and dissolution of the clusters assured generation of voltage-spikes indicative of neuromorphic activity. Nanopore memristors were reported as well, such as those with single pores placed between two immiscible liquids of different conductivities.107 We predict that nanopore memristors embedded in intermediate porosity membranes (Fig. 3D, right panel) could lead to more versatile neuromorphic systems. Integrated ionic circuits are likely to become an inspiration to both experimentalists and modelers to create more sensitive sensors and biomimetic systems.
Enzymatic cascades
Dialogue between single-nanopore and membrane communities could finally lead to systems that transcend simple transport and couple it with chemical transformation (Fig. 3E). Membrane reactors that combine catalysis with separations123 already inspired single-pore analysis of chemical reactions.124 The key question is how nanoconfinement and pore dimensions influence catalytic activity. Single nanopore and multipore membranes would be key to study different aspects of the enzymatic-transport systems. Single pores could help determine enzyme alignment and docking within the pore, but also detailed kinetics.125,126 By comparison, multipore membranes could help determine throughput on the enzymatic conversion. Equally exciting is the development of biomimetic enzymatic cascades of high-turnover.127 In the cascades, nanoconfinement shortens diffusion distances between the enzymatic substrates and products.128 Stacking of porous membranes could extend reaction cascades to a large scale.129
In conclusion, this Viewpoint invites increased collaboration between researchers working with porous membranes and single nanopores to enhance both fields. To increase collaboration, the Viewpoint delineates possible research directions that will advance both fundamental knowledge and applications.
Conflicts of interest
SH is co-inventor of a patent on the CsgG protein pore licensed to Oxford Nanopore Technologies plc.
Acknowledgements
ZS acknowledges support from the National Science Foundation CHE, MPS 2200524, and the Center for Enhanced Nanofluidic Transport, an Energy Frontier Research Center funded by the U.S. Department of Energy, Office of Science, Basic Energy Sciences at the University of California, Irvine, under Award No. DE-SC0019112. MLB acknowledges funding from the Division of Chemical Sciences, Geosciences, and Biosciences, Office of Basic Energy Sciences of the U.S. Department of energy through Grant DE-SC0023262. We are very grateful to Dr. Adam Dorey for help in preparing figures.
References
- R. A. Bull, T. N. Adikari, J. M. Ferguson, J. M. Hammond, I. Stevanovski, A. G. Beukers, Z. Naing, M. Yeang, A. Verich, H. Gamaarachchi, K. W. Kim, F. Luciani, S. Stelzer-Braid, J.-S. Eden, W. D. Rawlinson, S. J. van Hal and I. W. Deveson, Nat. Commun., 2020, 11, 6272 CrossRef CAS PubMed.
- S. Howorka and Z. Siwy, Chem. Soc. Rev., 2009, 38, 2360–2384 RSC.
- M. Wanunu, Phys. Life Rev., 2012, 9, 125–158 CrossRef PubMed.
- R. Bowden, R. W. Davies, A. Heger, A. T. Pagnamenta, M. de Cesare, L. E. Oikkonen, D. Parkes, C. Freeman, F. Dhalla, S. Y. Patel, N. Popitsch, C. L. C. Ip, H. E. Roberts, S. Salatino, H. Lockstone, G. Lunter, J. C. Taylor, D. Buck, M. A. Simpson and P. Donnelly, Nat. Commun., 2019, 10, 1869 CrossRef PubMed.
-
B. Hille, Ion Channels of Excitable Membranes, Sinauer, Sunderland, Mass, 2001 Search PubMed.
- X. Hou, W. Guo and L. Jiang, Chem. Soc. Rev., 2011, 40, 2385–2401 RSC.
- S. W. Kowalczyk, T. R. Blosser and C. Dekker, Trends Biotechnol., 2011, 29, 607–614 CrossRef CAS PubMed.
- Z. S. Siwy and S. Howorka, Chem. Soc. Rev., 2010, 39, 1115 RSC.
- M. Lepoitevin, T. J. Ma, M. Bechelany, J. M. Janot and S. Balme, Adv. Colloid Interface Sci., 2017, 250, 195–213 CrossRef CAS PubMed.
- S. Faucher, N. Aluru, M. Z. Bazant, D. Blankschtein, A. H. Brozena, J. Cumings, J. Pedro de Souza, M. Elimelech, R. Epsztein, J. T. Fourkas, A. G. Rajan, H. J. Kulik, A. Levy, A. Majumdar, C. Martin, M. McEldrew, R. P. Misra, A. Noy, T. A. Pham, M. Reed, E. Schwegler, Z. Siwy, Y. Wang and M. Strano, J. Phys. Chem. C, 2019, 123, 21309–21326 CrossRef CAS.
- M. Wang, Y. Hou, L. Yu and X. Hou, Nano Lett., 2020, 20, 6937–6946 CrossRef CAS PubMed.
- Z. Zhu, D. Wang, Y. Tian and L. Jiang, J. Am. Chem. Soc., 2019, 141, 8658–8669 CrossRef CAS PubMed.
- R. Wang, J. Zhang, C. Y. Tang and S. Lin, Environ. Sci. Technol., 2022, 56, 2605–2616 CrossRef CAS PubMed.
- P. Goyal, P. V. Krasteva, N. Van Gerven, F. Gubellini, I. Van den Broeck, A. Troupiotis-Tsaïlaki, W. Jonckheere, G. Péhau-Arnaudet, J. S. Pinkner, M. R. Chapman, S. J. Hultgren, S. Howorka, R. Fronzes and H. Remaut, Nature, 2014, 516, 250–253 CrossRef CAS PubMed.
- H. B. Park, J. Kamcev, L. M. Robeson, M. Elimelech and B. D. Freeman, Science, 2017, 356, eaab0530 CrossRef PubMed.
- R. P. Lively and D. S. Sholl, Nat. Mater., 2017, 16, 276–279 CrossRef CAS PubMed.
- R. W. Baker and B. T. Low, Macromolecules, 2014, 47, 6999–7013 CrossRef CAS.
- P. Arora and Z. M. Zhang, Chem. Rev., 2004, 104, 4419–4462 CrossRef CAS PubMed.
- Z. Zhang, X. Huang, Y. Qian, W. Chen, L. Wen and L. Jiang, Adv. Mater., 2020, 32, 1904351 CrossRef CAS PubMed.
- J. D. Feng, M. Graf, K. Liu, D. Ovchinnikov, D. Dumcenco, M. Heiranian, V. Nandigana, N. R. Aluru, A. Kis and A. Radenovic, Nature, 2016, 536, 197–200 CrossRef CAS PubMed.
- Z. Zhang, X. Sui, P. Li, G. Xie, X.-Y. Kong, K. Xiao, L. Gao, L. Wen and L. Jiang, J. Am. Chem. Soc., 2017, 139, 8905–8914 CrossRef CAS PubMed.
- X. Zhu, J. Hao, B. Bao, Y. Zhou, H. Zhang, J. Pang, Z. Jiang and L. Jiang, Sci. Adv., 2018, 4, eaau1665 CrossRef CAS PubMed.
- R. Epsztein, R. M. DuChanois, C. L. Ritt, A. Noy and M. Elimelech, Nat. Nanotechnol., 2020, 15, 426–436 CrossRef CAS PubMed.
- Y. Wu and J. J. Gooding, Chem. Soc. Rev., 2022, 51, 3862–3885 RSC.
- L. Xue, H. Yamazaki, R. Ren, M. Wanunu, A. P. Ivanov and J. B. Edel, Nat. Rev. Mater., 2020, 5, 931–951 CrossRef CAS.
- E. A. Manrao, I. M. Derrington, A. H. Laszlo, K. W. Langford, M. K. Hopper, N. Gillgren, M. Pavlenok, M. Niederweis and J. H. Gundlach, Nat. Biotechnol., 2012, 30, 349–353 CrossRef CAS PubMed.
- H. Brinkerhoff, A. S. W. Kang, J. Liu, A. Aksimentiev and C. Dekker, Science, 2021, 374, 1509–1513 CrossRef CAS PubMed.
- H. Ouldali, K. Sarthak, T. Ensslen, F. Piguet, P. Manivet, J. Pelta, J. C. Behrends, A. Aksimentiev and A. Oukhaled, Nat. Biotechnol., 2020, 38, 176–181 CrossRef CAS PubMed.
- R. H. Tunuguntla, R. Y. Henley, Y. C. Yao, T. A. Pham, M. Wanunu and A. Noy, Science, 2017, 357, 792–796 CrossRef CAS PubMed.
- J. Feng, K. Liu, M. Graf, M. Lihter, R. D. Bulushev, D. Dumcenco, D. T. L. Alexander, D. Krasnozhon, T. Vuletic, A. Kis and A. Radenovic, Nano Lett., 2015, 15, 3431–3438 CrossRef CAS PubMed.
- J. Li, D. Stein, C. McMullan, D. Branton, M. J. Aziz and J. A. Golovchenko, Nature, 2001, 412, 166–169 CrossRef CAS PubMed.
- A. J. Storm, J. H. Chen, X. S. Ling, H. W. Zandbergen and C. Dekker, Nat. Mater., 2003, 2, 537–540 CrossRef CAS PubMed.
-
R. L. Fleischer, P. B. Price, R. M. Walker and R. M. Walker, Nuclear Tracks in Solids: Principles and Applications, University of California Press, 1975 Search PubMed.
- W. Li, N. A. W. Bell, S. Hernández-Ainsa, V. V. Thacker, A. M. Thackray, R. Bujdoso and U. F. Keyser, ACS Nano, 2013, 7, 4129–4134 CrossRef CAS PubMed.
- L. Luo, S. R. German, W.-J. Lan, D. A. Holden, T. L. Mega and H. S. White, Annu. Rev. Anal. Chem., 2014, 7, 513–535 CrossRef CAS PubMed.
- H. Kwok, K. Briggs and V. Tabard-Cossa, PLoS One, 2014, 9, e92880 CrossRef PubMed.
- Y. M. N. D. Y. Bandara, B. I. Karawdeniya and J. R. Dwyer, ACS Omega, 2019, 4, 226–230 CrossRef CAS PubMed.
- R. B. Schoch, J. Y. Han and P. Renaud, Rev. Mod. Phys., 2008, 80, 839–883 CrossRef CAS.
- Z. Siwy and F. Fornasiero, Science, 2017, 357, 753 CrossRef CAS PubMed.
- I. Vlassiouk, P. Y. Apel, S. N. Dmitriev, K. Healy and Z. S. Siwy, Proc. Natl. Acad. Sci. U. S. A., 2009, 106, 21039–21044 CrossRef CAS PubMed.
- L. J. Steinbock, J. F. Steinbock and A. Radenovic, Nano Lett., 2013, 13, 1717–1723 CrossRef CAS PubMed.
- M. Q. Seah, Y. S. Khoo, W. J. Lau, P. S. Goh and A. F. Ismail, Ind. Eng. Chem. Res., 2021, 60, 9167–9178 CrossRef CAS.
- H. Yu, X. Qiu, N. Moreno, Z. Ma, V. M. Calo, S. P. Nunes and K.-V. Peinemann, Angew. Chem., Int. Ed., 2015, 54, 13937–13941 CrossRef CAS PubMed.
- Y. Zhang, R. A. Mulvenna, S. Qu, B. W. Boudouris and W. A. Phillip, ACS Macro Lett., 2017, 6, 726–732 CrossRef CAS PubMed.
- H. Zhang, J. Hou, Y. Hu, P. Wang, R. Ou, L. Jiang, J. Z. Liu, B. D. Freeman, A. J. Hill and H. Wang, Sci. Adv., 2018, 4, eaaq0066 CrossRef PubMed.
- Z. Jiang, R. Dong, A. M. Evans, N. Biere, M. A. Ebrahim, S. Li, D. Anselmetti, W. R. Dichtel and A. G. Livingston, Nature, 2022, 609, 58–64 CrossRef CAS PubMed.
- E. Gouaux and R. MacKinnon, Science, 2005, 310, 1461–1465 CrossRef CAS PubMed.
- P. Agre, Angew. Chem., Int. Ed., 2004, 43, 4278–4290 CrossRef CAS PubMed.
- S. Sahu and M. Zwolak, Nanoscale, 2017, 9, 11424–11428 RSC.
- J. D. Feng, K. Liu, M. Graf, D. Dumcenco, A. Kis, M. Di Ventra and A. Radenovic, Nat. Mater., 2016, 15, 850–855 CrossRef CAS PubMed.
- Y. Fu, S. Su, N. Zhang, Y. Wang, X. Guo and J. Xue, ACS Appl. Mater. Interfaces, 2020, 12, 24281–24288 CrossRef CAS PubMed.
- Z. Li, Y. Li, Y.-C. Yao, F. Aydin, C. Zhan, Y. Chen, M. Elimelech, T. A. Pham and A. Noy, ACS Nano, 2020, 14, 6269–6275 CrossRef CAS PubMed.
- I. Duznovic, A. Gräwe, W. Weber, L. K. Müller, M. Ali, W. Ensinger, A. Tietze and V. Stein, Small, 2021, 17, 2101066 CrossRef CAS PubMed.
- M. Ali, S. Nasir and W. Ensinger, Chem. Commun., 2015, 51, 3454–3457 RSC.
- L. Q. Gu, O. Braha, S. Conlan, S. Cheley and H. Bayley, Nature, 1999, 398, 686–690 CrossRef CAS PubMed.
- S. Howorka, S. Cheley and H. Bayley, Nat. Biotechnol., 2001, 19, 636–639 CrossRef CAS PubMed.
- Q. Liu, K. Xiao, L. P. Wen, H. Lu, Y. H. Liu, X. Y. Kong, G. H. Xie, Z. Zhang, Z. S. Bo and L. Jiang, J. Am. Chem. Soc., 2015, 137, 11976–11983 CrossRef CAS PubMed.
- E. T. Acar, S. F. Buchsbaum, C. Combs, F. Fornasiero and Z. S. Siwy, Sci. Adv., 2019, 5, eaav2568 CrossRef CAS PubMed.
- M. Ali, P. Ramirez, S. Mafé, R. Neumann and W. Ensinger, ACS Nano, 2009, 3, 603–608 CrossRef CAS PubMed.
- E. Kalman, I. Vlassiouk and Z. S. Siwy, Adv. Mater., 2008, 20, 293–297 CrossRef CAS.
- M. Wang, H. Meng, D. Wang, Y. Yin, P. Stroeve, Y. Zhang, Z. Sheng, B. Chen, K. Zhan and X. Hou, Adv. Mater., 2019, 31, 1805130 CrossRef PubMed.
- W. Choi, S. Jeon, S. J. Kwon, H. Park, Y.-I. Park, S.-E. Nam, P. S. Lee, J. S. Lee, J. Choi, S. Hong, E. P. Chan and J.-H. Lee, J. Membr. Sci., 2017, 527, 121–128 CrossRef CAS.
- A. K. Hołda and I. F. J. Vankelecom, J. Appl. Polym. Sci., 2015, 132, 42130 CrossRef.
- N. Rangnekar, N. Mittal, B. Elyassi, J. Caro and M. Tsapatsis, Chem. Soc. Rev., 2015, 44, 7128–7154 RSC.
- X. Zhao, Y. Wang, D.-S. Li, X. Bu and P. Feng, Adv. Mater., 2018, 30, 1705189 CrossRef PubMed.
- C. Zhang and W. J. Koros, Adv. Mater., 2017, 29, 1701631 CrossRef PubMed.
- J. Abraham, K. S. Vasu, C. D. Williams, K. Gopinadhan, Y. Su, C. T. Cherian, J. Dix, E. Prestat, S. J. Haigh, I. V. Grigorieva, P. Carbone, A. K. Geim and R. R. Nair, Nat. Nanotechnol., 2017, 12, 546–550 CrossRef CAS PubMed.
- S. Hong, C. Constans, M. V. S. Martins, Y. C. Seow, J. A. G. Carrio and S. Garaj, Nano Lett., 2017, 17, 728–732 CrossRef CAS PubMed.
- K. Gopinadhan, S. Hu, A. Esfandiar, M. Lozada-Hidalgo, F. C. Wang, Q. Yang, A. V. Tyurnina, A. Keerthi, B. Radha and A. K. Geim, Science, 2019, 363, 145–148 CrossRef CAS PubMed.
- R. Z. Waldman, F. Gao, W. A. Phillip and S. B. Darling, J. Membr. Sci., 2021, 633, 119389 CrossRef CAS.
- N. B. McKeown and P. M. Budd, Chem. Soc. Rev., 2006, 35, 675–683 RSC.
- T. L. Frey, K. R. Fruehauf, R. A. Lucas, J. W. Polster, K. J. Shea and Z. S. Siwy, J. Electrochem. Soc., 2022, 169, 020566 CrossRef.
- H. W. H. Lai, F. M. Benedetti, J. M. Ahn, A. M. Robinson, Y. Wang, I. Pinnau, Z. P. Smith and Y. Xia, Science, 2022, 375, 1390–1392 CrossRef CAS PubMed.
- T. J. Corrado, Z. Huang, D. Huang, N. Wamble, T. Luo and R. Guo, Proc. Natl. Acad. Sci. U. S. A., 2021, 118, e2022204118 CrossRef CAS PubMed.
- M. Yoshikawa, K. Tharpa and Ş.-O. Dima, Chem. Rev., 2016, 116, 11500–11528 CrossRef CAS PubMed.
- J. A. Armstrong, E. E. L. Bernal, A. Yaroshchuk and M. L. Bruening, Langmuir, 2013, 29, 10287–10296 CrossRef CAS PubMed.
- N. White, M. Misovich, A. Yaroshchuk and M. L. Bruening, ACS Appl. Mater. Interfaces, 2015, 7, 6620–6628 CrossRef CAS PubMed.
- C. L. Ritt, M. Liu, T. A. Pham, R. Epsztein, H. J. Kulik and M. Elimelech, Sci. Adv., 2022, 8, eabl5771 CrossRef CAS PubMed.
- Z. Sheng, J. Zhang, J. Liu, Y. Zhang, X. Chen and X. Hou, Chem. Soc. Rev., 2020, 49, 7907–7928 RSC.
- S. Wang, Y. Zhang, Y. Han, Y. Hou, Y. Fan and X. Hou, Acc. Mater. Res., 2021, 2, 407–419 CrossRef CAS.
- X. Hou, Adv. Mater., 2016, 28, 7049–7064 CrossRef CAS PubMed.
- X. Hou, Y. Hu, A. Grinthal, M. Khan and J. Aizenberg, Nature, 2015, 519, 70–73 CrossRef CAS PubMed.
- X. Zhou, Z. Wang, R. Epsztein, C. Zhan, W. Li, J. D. Fortner, T. A. Pham, J.-H. Kim and M. Elimelech, Sci. Adv., 2020, 6, eabd9045 CrossRef CAS PubMed.
- S. R. German, L. Luo, H. S. White and T. L. Mega, J. Phys. Chem. C, 2013, 117, 703–711 CrossRef CAS.
- L. M. Innes, C.-H. Chen, M. Schiel, M. Pevarnik, F. Haurais, M. E. Toimil-Molares, I. Vlassiouk, L. Theogarajan and Z. S. Siwy, Anal. Chem., 2014, 86, 10445–10453 CrossRef CAS PubMed.
- J. D. Feng, K. Liu, R. D. Bulushev, S. Khlybov, D. Dumcenco, A. Kis and A. Radenovic, Nat. Nanotechnol., 2015, 10, 1070–1073 CrossRef CAS PubMed.
- Y. H. Qiu, R. A. Lucas and Z. S. Siwy, J. Phys. Chem. Lett., 2017, 8, 3846–3852 CrossRef CAS PubMed.
- J. Bush, W. Maulbetsch, M. Lepoitevin, B. Wiener, M. M. Skanata, W. Moon, C. Pruitt and D. Stein, Rev. Sci. Instrum., 2017, 88, 113307 CrossRef PubMed.
- C. Maffeo, S. Bhattacharya, J. Yoo, D. Wells and A. Aksimentiev, Chem. Rev., 2012, 112, 6250–6284 CrossRef CAS PubMed.
- S. Y. Noskov, S. Bernèche and B. Roux, Nature, 2004, 431, 830–834 CrossRef CAS PubMed.
- J. Mathe, A. Aksimentiev, D. R. Nelson, K. Schulten and A. Meller, Proc. Natl. Acad. Sci. U. S. A., 2005, 102, 12377–12382 CrossRef CAS PubMed.
- M. B. Tanis-Kanbur, R. I. Peinador, J. I. Calvo, A. Hernández and J. W. Chew, J. Membr. Sci., 2021, 619, 118750 CrossRef CAS.
- A. Yaroshchuk, M. L. Bruening and E. Zholkovskiy, Adv. Colloid Interface Sci., 2019, 268, 39–63 CrossRef CAS PubMed.
- S. Sengupta and A. V. Lyulin, J. Phys. Chem. B, 2019, 123, 6882–6891 CrossRef CAS PubMed.
- J. Lu, L. C. Jacobson, Y. A. Perez Sirkin and V. Molinero, J. Chem. Theory Comput., 2017, 13, 245–264 CrossRef CAS PubMed.
- T. Kawakami, M. Nakada, H. Shimura, K. Okada and M. Kimura, Polym. J., 2018, 50, 327–336 CrossRef CAS.
- V. Kolev and V. Freger, Polymer, 2014, 55, 1420–1426 CrossRef CAS.
- L. Wang, R. S. Dumont and J. M. Dickson, RSC Adv., 2016, 6, 63586–63596 RSC.
- S. Liu, S. Ganti-Agrawal, S. Keten and R. M. Lueptow, J. Membr. Sci., 2022, 644, 120057 CrossRef CAS.
- H. Zhang, M. S. Wu, K. Zhou and A. W.-K. Law, Environ. Sci. Technol., 2019, 53, 6374–6382 CrossRef CAS PubMed.
- V. Freger, Faraday Discuss., 2018, 209, 371–388 RSC.
- C.-Y. Lin, C. Combs, Y.-S. Su, L.-H. Yeh and Z. S. Siwy, J. Am. Chem. Soc., 2019, 141, 3691–3698 CrossRef CAS PubMed.
- Y.-C. Liu, L.-H. Yeh, M.-J. Zheng and K. C.-W. Wu, Sci. Adv., 2021, 7, eabe9924 CrossRef CAS PubMed.
- D. Emmrich, A. Beyer, A. Nadzeyka, S. Bauerdick, J. C. Meyer, J. Kotakoski and A. Gölzhäuser, Appl. Phys. Lett., 2016, 108, 163103 CrossRef.
- Y. C. Chou, J. Chen, C. Y. Lin and M. Drndić, J. Chem. Phys., 2021, 154, 105102 CrossRef CAS PubMed.
- P. Robin, N. Kavokine and L. Bocquet, Science, 2021, 373, 687–691 CrossRef CAS PubMed.
- P. Zhang, M. Xia, F. Zhuge, Y. Zhou, Z. Wang, B. Dong, Y. Fu, K. Yang, Y. Li, Y. He, R. H. Scheicher and X. S. Miao, Nano Lett., 2019, 19, 4279–4286 CrossRef CAS PubMed.
- J. W. Polster, A. J. Souna, M. H. Motevaselian, R. A. Lucas, J. D. Tran, Z. S. Siwy, N. R. Aluru and J. T. Fourkas, Nat. Sci., 2022, 2, e20210099 CAS.
- S. Nasir, M. Ali, P. Ramirez, K. Froehlich, J. Cervera, S. Mafe and W. Ensinger, J. Membr. Sci., 2021, 635, 119505 CrossRef CAS.
- M. Z. Bazant, B. D. Storey and A. A. Kornyshev, Phys. Rev. Lett., 2011, 106, 046102 CrossRef PubMed.
- S. Marion, N. Vučemilović-Alagić, M. Špadina, A. Radenović and A.-S. Smith, Small, 2021, 17, 2100777 CrossRef CAS PubMed.
- M. Bagheri and S. A. Mirbagheri, Bioresour. Technol., 2018, 258, 318–334 CrossRef CAS PubMed.
- M. Taniguchi, S. Minami, C. Ono, R. Hamajima, A. Morimura, S. Hamaguchi, Y. Akeda, Y. Kanai, T. Kobayashi, W. Kamitani, Y. Terada, K. Suzuki, N. Hatori, Y. Yamagishi, N. Washizu, H. Takei, O. Sakamoto, N. Naono, K. Tatematsu, T. Washio, Y. Matsuura and K. Tomono, Nat. Commun., 2021, 12, 3726 CrossRef CAS PubMed.
- R. Wei, V. Gatterdam, R. Wieneke, R. Tampé and U. Rant, Nat. Nanotechnol., 2012, 7, 257–263 CrossRef CAS PubMed.
- E. C. Yusko, J. M. Johnson, S. Majd, P. Prangkio, R. C. Rollings, J. L. Li, J. Yang and M. Mayer, Nat. Nanotechnol., 2011, 6, 253–260 CrossRef CAS PubMed.
- A. Siria, M.-L. Bocquet and L. Bocquet, Nat. Rev. Chem., 2017, 1, 0091 CrossRef CAS.
- A. Siria, P. Poncharal, A. L. Biance, R. Fulcrand, X. Blase, S. T. Purcell and L. Bocquet, Nature, 2013, 494, 455–458 CrossRef CAS PubMed.
- L. Wang, Z. Wang, S. K. Patel, S. Lin and M. Elimelech, ACS Nano, 2021, 15, 4093–4107 CrossRef CAS PubMed.
- R. A. Lucas and Z. S. Siwy, ACS Appl. Mater. Interfaces, 2020, 12, 56622–56631 CrossRef CAS PubMed.
- G. Pérez-Mitta, A. G. Albesa, C. Trautmann, M. E. Toimil-Molares and O. Azzaroni, Chem. Sci., 2017, 8, 890–913 RSC.
- G. Maglia, A. J. Heron, W. L. Hwang, M. A. Holden, E. Mikhailova, Q. Li, S. Cheley and H. Bayley, Nat. Nanotechnol., 2009, 4, 437–440 CrossRef CAS PubMed.
- P. Ramirez, V. Garcia-Morales, V. Gomez, M. Ali, S. Nasir, W. Ensinger and S. Mafe, Phys. Rev. Appl., 2017, 7, 064035 CrossRef.
- Y. M. Sun and S. J. Khang, Ind. Eng. Chem. Res., 1988, 27, 1136–1142 CrossRef CAS.
- M. Ali, M. N. Tahir, Z. Siwy, R. Neumann, W. Tremel and W. Ensinger, Anal. Chem., 2011, 83, 1673–1680 CrossRef CAS PubMed.
- H.-C. Wu and H. Bayley, J. Am. Chem. Soc., 2008, 130, 6813–6819 CrossRef CAS PubMed.
- N. S. Galenkamp, A. Biesemans and G. Maglia, Nat. Chem., 2020, 12, 481–488 CrossRef CAS PubMed.
- H. Wang, Z. Zhao, Y. Liu, C. Shao, F. Bian and Y. Zhao, Sci. Adv., 2018, 4, eaat2816 CrossRef PubMed.
- L. Sun, Y. Gao, Y. Xu, J. Chao, H. Liu, L. Wang, D. Li and C. Fan, J. Am. Chem. Soc., 2017, 139, 17525–17532 CrossRef CAS PubMed.
- S. R. Lewis, S. Datta, M. Gui, E. L. Coker, F. E. Huggins, S. Daunert, L. Bachas and D. Bhattacharyya, Proc. Natl. Acad. Sci. U. S. A., 2011, 108, 8577–8582 CrossRef PubMed.
|
This journal is © The Royal Society of Chemistry 2023 |
Click here to see how this site uses Cookies. View our privacy policy here.