A quantum chemical study on the anti-SARS-CoV-2 activity of TMPRSS2 inhibitors†
Received
16th April 2023
, Accepted 15th July 2023
First published on 18th July 2023
Abstract
Nafamostat and camostat are known to inhibit the spike protein-mediated fusion of severe acute respiratory syndrome coronavirus 2 (SARS-CoV-2) by forming a covalent bond with the human transmembrane serine protease 2 (TMPRSS2) enzyme. Previous experiments revealed that the TMPRSS2 inhibitory activity of nafamostat surpasses that of camostat, despite their structural similarities; however, the molecular mechanism of TMPRSS2 inhibition remains elusive. Herein, we report the energy profiles of the acylation reactions of nafamostat, camostat, and a nafamostat derivative by quantum chemical calculations using a combined molecular cluster and polarizable continuum model (PCM) approach. We further discuss the physicochemical relevance of their inhibitory activity in terms of thermodynamics and kinetics. Our analysis attributes the strong inhibitory activity of nafamostat to the formation of a stable acyl intermediate and its low activation energy during acylation with TMPRSS2. The proposed approach is also promising for elucidating the molecular mechanisms of other covalent drugs.
1. Introduction
The outbreak of the new coronavirus (severe acute respiratory syndrome coronavirus 2; SARS-CoV-21) infection (COVID-19) has caused severe public health problems and its impact has also changed our way of life, including our economy and culture.2,3 Numerous COVID-19 treatments have been studied, resulting in the successful development of COVID-19 vaccines and anti-SARS-CoV-2 agents4,5 that are gradually returning our lives to pre-pandemic conditions.6 Meanwhile, many studies have reported effective COVID-19 treatment methods at the basic research level,7,8 that are expected to be crucial in preparing for unknown viral diseases.
SARS-CoV-2 is transmitted by the binding of a spike protein (S protein) in the envelope to the angiotensin-converting enzyme 2 (ACE2) receptor on the human cell membrane.9,10 The S protein is then cleaved by the human transmembrane serine protease 2 (TMPRSS2) enzyme,11,12 resulting in membrane fusion between the virus and the human cell.13 This membrane fusion has been reported to be prevented by the synthesized TMPRSS2 inhibitors nafamostat and camostat (Fig. 1a), which are approved in Japan for the treatment of acute/chronic pancreatitis.10,14 Nafamostat and camostat have similar basic structures, and both inhibit viral entry into human cells by binding to TMPRSS2.
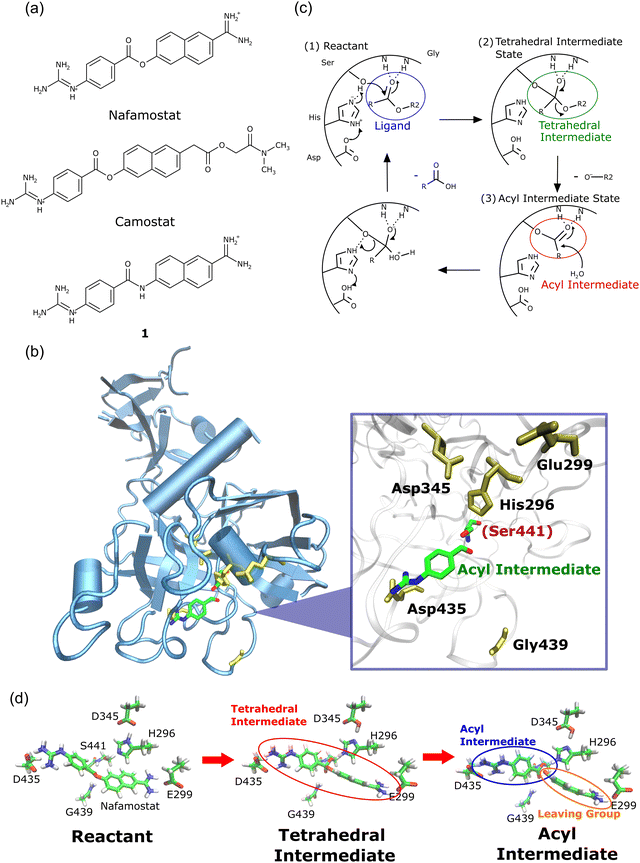 |
| Fig. 1 (a) Chemical structures of nafamostat, camostat, and compound 1. (b) Crystal structure of TMPRSS2 forming an acyl intermediate with nafamostat (Protein Data Bank (PDB) ID: 7MEQ15). (c) Proposed reaction mechanism of serine protease.21,22 Nafamostat and camostat inhibit TMPRSS2 by covalently binding to Ser441.16 (d) Structures of the reactant, tetrahedral intermediate, and acyl intermediate states optimized by the cluster + PCM model. | |
A crystallographic study revealed that the ester group of nafamostat reacts with the Ser441 side chain in TMPRSS2 to form a covalently bound acyl intermediate15 (Fig. 1b). The proposed mechanism of the TMPRSS2 enzymatic reaction is shown in Fig. 1c.16 Camostat also forms a chemically identical acyl intermediate, as evidenced by the crystal structures of other serine proteases, such as prostasin17 and enteropeptidase.18 These findings imply that nafamostat and camostat can be classified as so-called covalent drugs that bind to their target proteins through covalent bond formation.16
Several previous studies have demonstrated the inhibitory activity of nafamostat and camostat against TMPRSS2.10,14,19 Yamamoto et al. measured the half maximum effective concentration (EC50) of nafamostat and camostat using SARS-CoV-2-infected Calu-3 cells. The results showed that the EC50 of nafamostat (1–10 nM) was approximately 1/10 that of camostat,14 indicating that nafamostat can inhibit TMPRSS2 more potently than camostat.
Despite forming chemically identical acyl intermediates, nafamostat and camostat exhibit different TMPRSS2 inhibitory activity. This fact raises the question: how does nafamostat work more effectively than camostat? These two compounds have similar basic structures but differ on one side (Fig. 1a): nafamostat consists of an amidino group, while camostat comprises ester and amide moieties. This structural difference causes significant difference in their inhibitory effect.
To investigate the differences in the potency of TMPRSS2 inhibitors, we previously calculated the binding affinity of nafamostat, camostat, and five nafamostat derivatives for TMPRSS2.20 Notably, our calculations characterized the binding between TMPRSS2 and the compounds as a non-covalent interaction because drug potency is generally discussed in terms of binding affinity to the target protein via the classical treatment. The calculations indicated the nafamostat derivative where the ester bond of nafamostat was replaced with an amide bond (compound 1) as having the highest binding affinity. Based on this result, we expected compound 1 exhibiting the strongest inhibitory activity;20 however, in vitro assays using SARS-CoV-2-infected cells demonstrated that compound 1 had the weakest inhibitory activity.20 Therefore, it was inferred that the antiviral activity of these agents cannot be explained in terms of binding affinity.
The difference in TMPRSS2 inhibitory activity of these compounds may be attributed to the process of their covalent bond formation with TMPRSS2. In other words, the ease of covalent bond formation with TMPRSS2 may contribute to the inhibitory activity. The aforementioned binding affinity factors in the interaction energy between the compound and TMPRSS2 when forming a non-covalent complex.20 In contrast, the formation of covalent bonds corresponds to the occurrence of a chemical reaction and strongly depends on the relative energy of the product to the reactant and the activation energy in the chemical reaction.23 Therefore, quantitative analysis of the free energy profile during acylation with TMPRSS2 is necessary to examine the covalent bond formation of TMPRSS2 inhibitors.
Classical methods, such as docking simulation and molecular dynamics simulations, are widely used in drug discovery;24,25 nevertheless, these techniques are not suitable for analyzing the process of covalent bond formation.26 In contrast, quantum chemical calculations are effective for investigating reaction pathways and are suitable for this task.27 However, the quantum mechanical (QM) treatment of the protein environment, which is composed of thousands of atoms, is impractical due to the enormous computational cost.28,29 Hybrid quantum mechanics/molecular mechanics (QM/MM)30 calculations are an effective approach to this problem, but even with this method, it is not easy to search for transition states (TSs) considering the huge number of atoms.
To circumvent this problem, this study adopts a combined approach including the molecular cluster model31 and the polarizable continuum model (PCM),32 and applies it to the analysis of acylation reactions for TMPRSS2 inhibition. In this approach, molecules in the active site are treated quantum mechanically, and the remaining protein environment is approximated as a polarizable continuum.33 This method is hereafter referred to as the cluster + PCM model.
The aim of this study is to elucidate the molecular mechanism underlying the inhibitory activity of TMPRSS2 inhibitors in terms of the ease of covalent bond formation by means of the cluster + PCM model. To this end, the free energies of the reactants, products, and TSs of the three TMPRSS2 inhibitors were calculated along the reaction coordinates, focusing on the differences in the effects of (i) nafamostat and camostat and (ii) nafamostat and compound 1. The calculations allowed us to identify the most prominent differences in their inhibitory activities both from a thermodynamic and reaction kinetics aspect.
2. Methods
2.1. Energy profile calculations using the cluster + PCM model
The atomic coordinates of the TMPRSS2-nafamostat and TMPRSS2-camostat complexes are obtained from the trajectories of molecular dynamics (MD) simulations performed by Hempel et al.16 Snapshot structures at 136.8 μs for nafamostat and 50.0 μs for camostat were extracted from the MD trajectories, and these were used as the initial computational models.16 In these structures, nafamostat (or camostat) was located near Ser441, Asp435, and Glu299, and His296 and Ser441 were in close proximity, making it suitable as a reactant. For compound 1, the ester moiety of nafamostat was replaced by an amide moiety in the nafamostat snapshot structure. The energy profiles including reactant, TS1, tetrahedral intermediate, TS2, and acyl intermediate were generated from these structures using the cluster + PCM32 model. Here, the TMPRSS2 inhibitor (nafamostat, camostat, or compound 1) plus the six surrounding amino acids (Asp345, His296, Ser441, Asp435, Glu299, and Gly439) were treated explicitly as the QM atoms, and the remaining environmental effects were approximated by PCM with dielectric constant of 2.0.34 The α-carbon of each amino acid was substituted with a methyl group. To maintain the overall protein backbone, the coordinates of the methyl groups were fixed during the geometry optimization. For Ser441 and Gly439, the peptide moiety of the main chain was further incorporated into the QM atoms. The guanidino and amidino groups present in nafamostat, camostat, and compound 1 were treated as cations because the molecules containing these functional groups, such as arginine, have a pKa value of ∼13.8.35
In the cluster + PCM model, density functional theory (DFT) at the B3LYP-GD3BJ36/6-31G(d) level was used to optimize the QM atoms in the five states (reactant, TS1, tetrahedral intermediate, TS2, and acyl intermediate). The obtained TSs were verified using the intrinsic reaction coordinate (IRC) calculations.37 The free energies at 298.15 K were calculated using the results of the normal mode analysis. All these calculations were performed using the Gaussian16 program package.38
2.2. Geometry optimization using the ONIOM method
TMPRSS2-inhibitor complexes were optimized by the “our own n-layered integrated molecular orbital and molecular mechanics” (ONIOM) method,39 in which DFT with the B3LYP-GD3BJ functional36 and the Amber99 force field40 were employed for the QM and MM regions, respectively. The 6-31G(d) basis set was used throughout the QM calculations. The general AMBER force field (GAFF)41 was used for TMPRSS2 inhibitors (nafamostat, camostat, and compound 1). Similar to the handling in the cluster + PCM model, TMPRSS2 inhibitors (nafamostat, camostat, compound 1) and the side chains and α-carbons at six amino acids in TMPRSS2 (Asp345, His296, Ser441, Asp435, Glu299, and Gly439) were incorporated as the QM regions. The peptide moieties in the main chains of Ser441 and Gly439 were also incorporated into QM region, and the rest was treated as the MM region. The ONIOM geometry optimization was also performed using the Gaussian16 program package.38
3. Results and discussion
We first performed geometry optimization42 using the cluster + PCM model on the reactants, tetrahedral intermediates, acyl intermediates, and the two TSs between them. The resulting structures are presented in Fig. 1d. While recognizing the significance of calculating the deacylation process subsequent to acylation, we omitted the consideration of deacylation within the context of this study. This omission arises from the formidable challenge associated with accurately accounting for the intricate arrangement of leaving groups and water molecules involved in the process. The detailed structures are shown in Fig. S1 (ESI†). Nafamostat and compound 1 did not show significant conformational changes between the five states (reactant, TS1, tetrahedral intermediate, TS2, acyl intermediate), whereas camostat exhibited large orientational changes in the ester and amide moieties. These structural differences probably resulted from the lack of a cationic amidino group on one side of camostat.
The energy profiles corresponding to these structures are displayed in Fig. 2a. The energies of the acyl intermediates of nafamostat and camostat were 6.63 and 0.66 kcal mol−1 lower than their reactants, respectively, implying that they both produced stable acyl intermediates. In contrast, compound 1 produced an unstable acyl intermediate that was 16.00 kcal mol−1 higher in energy than the reactant. These results showed that the stability of the acyl intermediates varies widely among the three compounds.
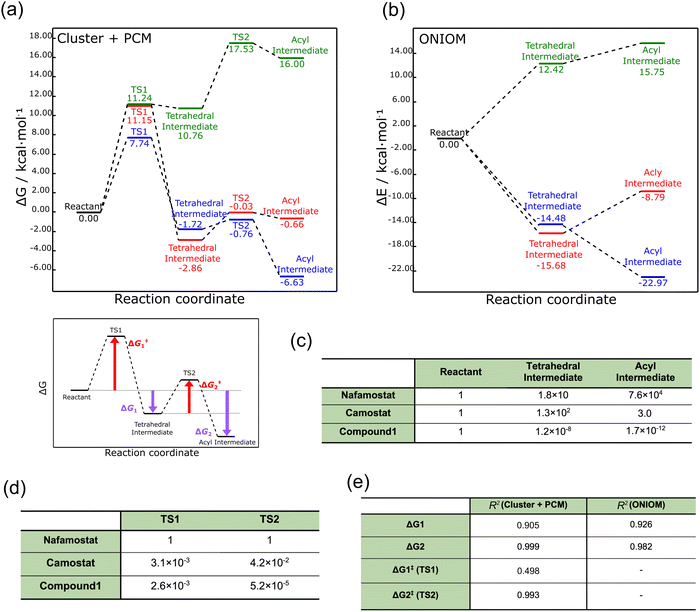 |
| Fig. 2 (a) Free energy profiles of TMPRSS2 inhibitors obtained by the cluster + PCM model. Nafamostat, camostat, and compound 1 are indicated by blue, red, and green, respectively. (b) Electronic energy profiles of TMPRSS2 inhibitors obtained by the ONIOM method. (c) Boltzmann distribution ratio of tetrahedral and acyl intermediates to reactants at 298 K. (d) Rate constant ratio of camostat and compound 1 to nafamostat at 298 K. (e) Coefficient of determination (R2) between pEC50 and each energy difference (zG1, ΔG2, ΔG‡1, and ΔG‡2). The coefficient of determination values were calculated based on the results obtained from nafamostat, camostat, and compound 1. Detailed information is provided in Fig. S2 (ESI†). | |
To further examine these results, we used the ONIOM method39 to describe the protein environment of TMPRSS2 in more detail. Here the ONIOM calculations were performed only for the reactant, tetrahedral intermediate, and product, and not for the TS1 and TS2 states. As shown in Fig. 2b, the ONIOM optimization yielded relative trends in the energy profiles similar to those produced by the cluster + PCM model, although the absolute values relatively differed. These results confirm that the cluster + PCM model adequately represents the protein environment.
The activation energy ΔG‡1 was also calculated using the cluster + PCM model with the M06-2X43 and APFD44 functionals. As summarized in Table S1 (ESI†), these calculations yielded activation energies with relatively similar trends to the B3LYP-GD3BJ result. Therefore, the energy profiles calculated from the cluster + PCM model at the B3LYP-GD3BJ/6-31G(d) level were employed in the subsequent analyses. To examine the influence of basis function dependence, the gas-phase electronic energy was computed using the 6-311G(d) basis function for the structure optimized at the B3LYP-GD3BJ/6-31G(d) level. Remarkably, extending the basis function from 6-31G(d) to 6-311G(d) resulted in a mere 0.07 kcal mol−1 variation in electronic energy. This finding indicates the suitability of the selected basis function for the cluster + PCM model.
The calculated free energies were used to estimate the ratio of the Boltzmann distribution of the acyl intermediate to the reactant (ρA/ρR) at 298 K, according to the following equation:
| 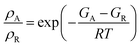 | (1) |
where
R and
T are the gas constant and temperature, respectively, while
GA and
GR are the free energies for the acyl intermediate and reactant, respectively. The results are summarized in
Fig. 2c. The ratios were calculated to be 7.59 × 10
4, 3.04, and 1.65 × 10
−12 for nafamostat, camostat, and compound
1, respectively, indicating that the acyl intermediate is more likely to be formed with nafamostat and camostat, and less likely to be formed with compound
1. These thermodynamic analyses successfully reproduced a trend in the inhibitory activities of the three compounds which was obtained from
in vitro studies.
20
Next, the TMPRSS2 inhibitory activity of nafamostat and camostat was investigated in terms of reaction kinetics. As shown in Fig. 2a, the activation energies of nafamostat in TS1 and TS2 were 7.74 and 0.96 kcal mol−1, respectively, while those of camostat were 11.15 and 2.83 kcal mol−1, respectively. Thus, the activation energies of nafamostat were found to be 3.41 and 1.87 kcal mol−1 lower than those of camostat in TS1 and TS2, respectively. These results indicate that nafamostat is more reactive than camostat.
To gain further insight into the reactivities of these compounds, we estimated the ratio of the rate constants of camostat and compound 1 to nafamostat at 298 K. Assuming that the transmission factor in the Eyring equation for all three compounds is the same,45,46 the relative reaction rate ki/kNaf (for i = camostat or compound 1) is determined as follows:
| 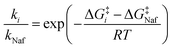 | (2) |
where Δ
G‡i and Δ
G‡Naf are the activation energies of molecule
i (for
i = camostat or compound
1) and nafamostat, respectively. The results of the relative rates are summarized in
Fig. 2d. In TS1, they were calculated to be 3.07 × 10
−3 and 2.62 × 10
−3 for camostat and compound
1, respectively. These results indicate that nafamostat is 3.25 × 10
2 and 3.82 × 10
2 times more reactive than camostat and compound
1, respectively. In TS2, the calculated relative rates were 4.22 × 10
−2 and 5.21 × 10
−5 for camostat and compound
1, respectively, indicating that the reactivity of nafamostat is 2.37 × 10 and 1.92 × 10
4 times higher than those of camostat and compound
1, respectively. Thus, the kinetic analysis of
eqn (2) demonstrated that nafamostat is more likely to produce an acyl intermediate than camostat and compound
1.
We next attempted to compare the results of the energy profile analysis with the results of the in vitro assay.20 To identify the most significant contribution to the anti-SARS-CoV-2 effect, we estimated the correlations of ΔG1, ΔG2, ΔG‡1, and ΔG‡2 to pEC50 (−log10[EC50]).20 Here, ΔG1 and ΔG2 represent the free energy differences from the reactant to the tetrahedral and acyl intermediate states, respectively, and ΔG‡1 and ΔG‡2 are the activation energies of TS1 and TS2, respectively. The definition of each energy difference is shown in Fig. 2a.
Fig. 2e summarizes the coefficient of determination (R2) between pEC50 and each energy difference (see Fig. S2 for details, ESI†). The R2 values were calculated to be 0.905, 0.999, 0.498, and 0.993 for ΔG1, ΔG2, ΔG‡1, and ΔG‡2, respectively. Thus, although the number of data points is small (three), all three energy differences except ΔG‡1 were highly correlated with pEC50; the value for ΔG2 showed the largest correlation. This correlation may warrant further analysis of the relationship between the stability of the acyl intermediate state and the TMPRSS2 inhibitory activity.
We further investigated the cause of the stability in the acyl intermediates of nafamostat. In the calculated structures presented in Fig. S1 (ESI†), both nafamostat and compound 1 produce acyl intermediates with the same structure. The structure of this acyl intermediate is identical to the crystal structure of nafamostat (PDB ID: 7MEQ) depicted in Fig. 1b. As a result, we can infer the structure of the leaving group in this system. However, the formation of the acyl intermediate results in the production of different types of leaving groups for nafamostat and compound 1. In nafamostat, the leaving group consists of a naphthoxide moiety, while in compound 1, it consists of a naphthylamide moiety (Fig. 3a). To analyze the effect of these leaving groups, we calculated the interaction energies between the leaving groups and the acyl intermediates as follows:
| GInt = GAll − (Gi + GLG) | (3) |
where
Gi and
GLG denote the free energies of the acyl intermediate (Ser441) and the leaving group, respectively, and
GAll is the free energy of their complex. The interaction energies for nafamostat and compound
1 were calculated to be −4.33 and −0.52 kcal mol
−1, respectively, confirming that the leaving group of nafamostat produces a more stable structure. We further extended the interaction analysis of the leaving group to the amino acids in the active site (
i.e., Asp345, His296, Asp435, Glu299, and Gly439). Here, the free energy of amino acid
i (for
i = Asp345, His296, Asp435, Glu299, and Gly439) was employed for
Gi in
eqn (3). The structures of these amino acids are shown in
Fig. 3a. The results are summarized in
Fig. 3b. The strongest interaction was obtained from Glu299, with interaction energies of −33.89 and −30.78 kcal mol
−1 for nafamostat and compound
1, respectively. The total interaction energies were calculated to be −2.53 and 3.38 kcal mol
−1 for nafamostat and compound
1, respectively. The difference between their total interaction energies was 5.91 kcal mol
−1, which accounts for as much as a quarter of the energy difference between the acyl intermediates of nafamostat and compound
1 in the energy profiles (22.63 kcal mol
−1). Thus, the stability of the acyl intermediate of nafamostat is attributed to the strong interaction of its leaving group with the acyl intermediate and surrounding protein. This result is more clearly understood by the electrostatic potential (ESP) maps of the acyl intermediate state shown in
Fig. 3c. The naphthoxide moiety of nafamostat generates a larger negative ESP than the naphthylamide moiety of compound
1. This fact indicates that the leaving group of nafamostat attracts the acyl intermediate more strongly than that of compound
1, stemming from the presence of the positive charge derived from the guanidino group of the acyl intermediate. These analyses successfully demonstrate that the more negative ESP generated by the nafamostat leaving group directly contributes to the stability of its acyl intermediate. The Mulliken population analyses also support these results (see Fig. S3 for details, ESI
†).
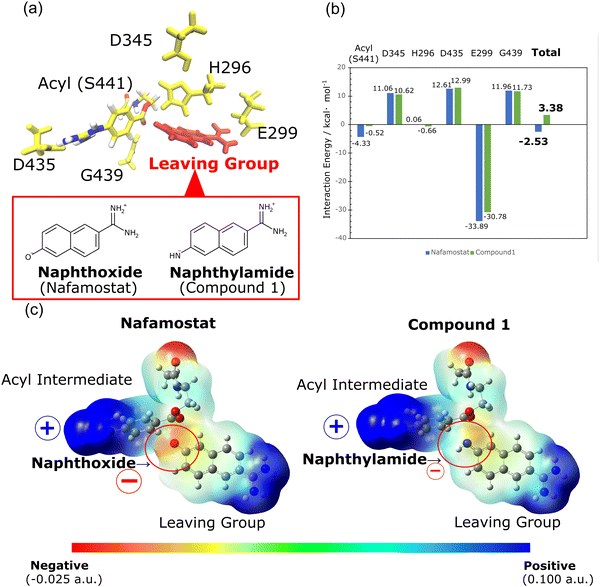 |
| Fig. 3 (a) Structures of the amino acids in TMPRSS2 used to calculate the interaction energies with the leaving group. (b) Interaction energies of the leaving groups with the acyl intermediates and amino acids for nafamostat and compound 1. (c) Electrostatic potential (ESP) map generated by the leaving groups and acyl intermediates of nafamostat and compound 1. | |
The origin of the stability of the tetrahedral intermediate was also investigated. The formation of the tetrahedral intermediate requires the oxygen atom of the Ser441 side chain to attack the carbonyl carbon of the TMPRSS2 inhibitor. To examine this effect, the orbital interaction between the TMPRSS2 inhibitor and Ser441 were estimated. Here, we focused on the molecular orbitals (MOs) distributed on Ser441 and the TMPRSS2 inhibitors before and after the orbital interaction. The shapes of reactive orbitals are displayed in Fig. 4a. The orbitals after interaction are shown in Fig. S4 (ESI†). Notably, in the pre-interaction calculations, only the catalytic triad (Asp435, His296, Ser441) and the TMPRSS2 inhibitor were calculated as QM atoms, while the other amino acids were incorporated as point charges. The post-interaction calculations are performed for the complex comprising the catalytic triad and the covalently bound inhibitor, with the other molecules once again treated as point charges. Fig. 4b illustrates the orbital interactions between the occupied orbitals distributed on Ser441 (HOMO−6 (HOMO−6), HOMO−4, and HOMO−5 for nafamostat, camostat, and compound 1, respectively) and the lowest unoccupied MOs (LUMOs) of the TMPRSS2 inhibitors. The occupied orbital involved in the interaction was selected from the highest-energy orbital that exhibited a substantial lobe extending over the oxygen atom of Ser441. The magnitudes of the orbital interactions (orbital energy gaps) were calculated to be 4.98, 4.40 and 4.15 eV for camostat, nafamostat, and compound 1, respectively. As already shown in Fig. 2, the analysis of the energy profiles revealed that the stability of the tetrahedral intermediates was in the order of camostat, nafamostat, and compound 1. Thus, the magnitude of the orbital interactions was in good agreement with the trend of the energy profiles. Further MO analysis revealed that the ester moiety of camostat and the OH group of Ser441 faced each other, resulting in a larger orbital overlap between them (Fig. 4a). By conjointly considering the above, the stability of the tetrahedral intermediate of camostat was inferred to result from the larger orbital interaction between camostat and Ser441.
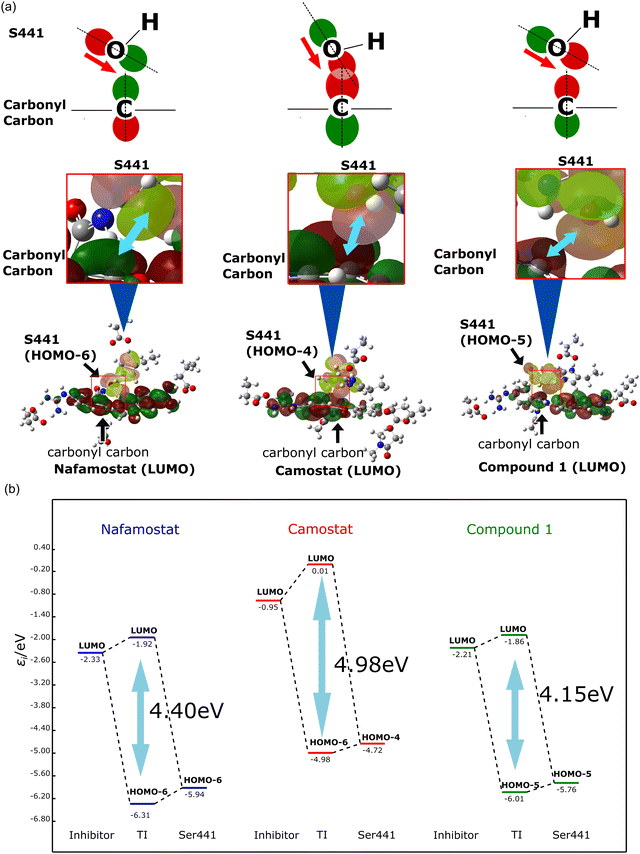 |
| Fig. 4 (a) Shape of molecular orbitals (MOs) involved in the formation of tetrahedral intermediates. (b) Orbital interactions during the formation of tetrahedral intermediates. | |
4. Conclusions
In this study, the TMPRSS2 inhibitory activity of nafamostat, camostat, and compound 1 was investigated using the cluster + PCM model and the ONIOM method. The calculations of the energy profiles including the reactants, tetrahedral intermediates, acyl intermediates, and their two TS states clearly indicated that nafamostat proceeds the acylation reaction more efficiently than camostat. The calculations also showed that despite its structural similarity to nafamostat, compound 1 is not a suitable TMPRSS2 inhibitor. Since these results could not be derived from conventional binding affinity calculations, this study highlights for the first time the significance of energy profile analysis in the investigation of TMPRSS2 inhibitory activity.
The present study demonstrates the potent TMPRSS2 inhibitory activity of nafamostat both from a thermodynamic and kinetic point of view, which is attributed to the formation of a stable acyl intermediate and its low activation energy.
The cluster + PCM model employed in this study offers simplicity and broad prospects for combination with other methods. By considering various molecular conformations using the cluster + PCM model, more accurate and detailed analysis will be conducted. In our future work, this approach will be used to elucidate the molecular mechanisms of other covalent drugs with the aim of developing new effective antiviral agents.
Conflicts of interest
There are no conflicts to declare.
Acknowledgements
This study was supported by a grant from the Japan Society for the Promotion of Science KAKENHI program (grant no. 20K05430 to K. J. F. and 21H01881 and JP21K18931 to T. Y.). A. K. thanks the Graduate Program of Transformative Chem-Bio Research at Nagoya University, supported by MEXT (WISE Program).
References
- A. E. Gorbalenya, S. C. Baker, R. S. Baric, R. J. de Groot, C. Drosten, A. A. Gulyaeva, B. L. Haagmans, C. Lauber, A. M. Leontovich, B. W. Neuman, D. Penzar, S. Perlman, L. L. M. Poon, D. V. Samborskiy, I. A. Sidorov, I. Sola, J. Ziebuhr and Coronaviridae Study Group of the International Committee on Taxonomy of Viruses, Nat. Microbiol., 2020, 5, 536–544 CrossRef PubMed.
-
H. Wang, K. R. Paulson, S. A. Pease, S. Watson, H. Comfort, P. Zheng, A. Y. Aravkin, C. Bisignano, R. M. Barber, T. Alam, J. E. Fuller, E. A. May, D. P. Jones, M. E. Frisch, C. Abbafati, C. Adolph, A. Allorant, J. O. Amlag, B. Bang-Jensen, G. J. Bertolacci, S. S. Bloom, A. Carter, E. Castro, S. Chakrabarti, J. Chattopadhyay, R. M. Cogen, J. K. Collins, K. Cooperrider, X. Dai, W. J. Dangel, F. Daoud, C. Dapper, A. Deen, B. B. Duncan, M. Erickson, S. B. Ewald, T. Fedosseeva, A. J. Ferrari, J. J. Frostad, N. Fullman, J. Gallagher, A. Gamkrelidze, G. Guo, J. He, M. Helak, N. J. Henry, E. N. Hulland, B. M. Huntley, M. Kereselidze, A. Lazzar-Atwood, K. E. LeGrand, A. Lindstrom, E. Linebarger, P. A. Lotufo, R. Lozano, B. Magistro, D. C. Malta, J. Månsson, A. M. Mantilla Herrera, F. Marinho, A. H. Mirkuzie, A. T. Misganaw, L. Monasta, P. Naik, S. Nomura, E. G. O'Brien, J. K. O'Halloran, L. T. Olana, S. M. Ostroff, L. Penberthy, R. C. Reiner Jr, G. Reinke, A. L. P. Ribeiro, D. F. Santomauro, M. I. Schmidt, D. H. Shaw, B. S. Sheena, A. Sholokhov, N. Skhvitaridze, R. J. D. Sorensen, E. E. Spurlock, R. Syailendrawati, R. Topor-Madry, C. E. Troeger, R. Walcott, A. Walker, C. S. Wiysonge, N. A. Worku, B. Zigler, D. M. Pigott, M. Naghavi, A. H. Mokdad, S. S. Lim, S. I. Hay, E. Gakidou and C. J. L. Murray, Lancet, 2022, 399, 1513–1536 Search PubMed.
- J. E. Pekar, A. Magee, E. Parker, N. Moshiri, K. Izhikevich, J. L. Havens, K. Gangavarapu, L. M. Malpica Serrano, A. Crits-Christoph, N. L. Matteson, M. Zeller, J. I. Levy, J. C. Wang, S. Hughes, J. Lee, H. Park, M.-S. Park, K. Ching Zi Yan, R. T. P. Lin, M. N. Mat Isa, Y. M. Noor, T. I. Vasylyeva, R. F. Garry, E. C. Holmes, A. Rambaut, M. A. Suchard, K. G. Andersen, M. Worobey and J. O. Wertheim, Science, 2022, 377, 960–966 CrossRef CAS PubMed.
- Z. Zhang, Q. Shen and H. Chang, Front. Immunol., 2022, 13, 843928 CrossRef CAS PubMed.
- O. J. Watson, G. Barnsley, J. Toor, A. B. Hogan, P. Winskill and A. C. Ghani, Lancet Infect. Dis., 2022, 22, 1293–1302 CrossRef CAS PubMed.
- M. Biancolella, V. L. Colona, R. Mehrian-Shai, J. L. Watt, L. Luzzatto, G. Novelli and J. K. V. Reichardt, Hum. Genomics, 2022, 16, 19 CrossRef CAS PubMed.
- S. Pandamooz, B. Jurek, C.-P. Meinung, Z. Baharvand, A. S. Shahem-abadi, S. Haerteis, J. A. Miyan, J. Downing, M. Dianatpour, A. Borhani-Haghighi and M. S. Salehi, Annu. Rev. Pharmacol. Toxicol., 2022, 62, 25–53 CrossRef PubMed.
- C. Macraild, M. Rehman, F. Faheem, S. Murugesan, I. Styles, A. Peterson, C. Kirkpatrick, M. Cooper, E. Palombo, M. Simpson, H. Jain, V. Agarwal, A. McAuley, A. Kumar, D. Creek, N. Trevaskis and S. Vasan, Int. J. Mol. Sci., 2022, 23, 11851 CrossRef CAS PubMed.
- P. Zhao, J. L. Praissman, O. C. Grant, Y. Cai, T. Xiao, K. E. Rosenbalm, K. Aoki, B. P. Kellman, R. Bridger, D. H. Barouch, M. A. Brindley, N. E. Lewis, M. Tiemeyer, B. Chen, R. J. Woods and L. Wells, Cell Host Microbe, 2020, 28, 586–601.e586 CrossRef CAS PubMed.
- M. Hoffmann, H. Kleine-Weber, S. Schroeder, N. Krüger, T. Herrler, S. Erichsen, T. S. Schiergens, G. Herrler, N.-H. Wu, A. Nitsche, M. A. Müller, C. Drosten and S. Pöhlmann, Cell, 2020, 181, 271–280.e278 CrossRef CAS PubMed.
- I. Glowacka, S. Bertram, M. A. Müller, P. Allen, E. Soilleux, S. Pfefferle, I. Steffen, T. S. Tsegaye, Y. He, K. Gnirss, D. Niemeyer, H. Schneider, C. Drosten and S. Pöhlmann, J. Virol., 2011, 85, 4122–4134 CrossRef PubMed.
- S. Matsuyama, N. Nagata, K. Shirato, M. Kawase, M. Takeda and F. Taguchi, J. Virol., 2010, 84, 12658–12664 CrossRef CAS PubMed.
- D. Bestle, M. R. Heindl, H. Limburg, T. V. L. van, O. Pilgram, H. Moulton, D. A. Stein, K. Hardes, M. Eickmann, O. Dolnik, C. Rohde, H.-D. Klenk, W. Garten, T. Steinmetzer and E. Böttcher-Friebertshäuser, Life Sci. Alliance, 2020, 3, e202000786 CrossRef PubMed.
- M. Yamamoto, M. Kiso, Y. Sakai-Tagawa, K. Iwatsuki-Horimoto, M. Imai, M. Takeda, N. Kinoshita, N. Ohmagari, J. Gohda, K. Semba, Z. Matsuda, Y. Kawaguchi, Y. Kawaoka and J.-I. Inoue, Viruses, 2020, 12, 629 CrossRef CAS PubMed.
- B. J. Fraser, S. Beldar, A. Seitova, A. Hutchinson, D. Mannar, Y. Li, D. Kwon, R. Tan, R. P. Wilson, K. Leopold, S. Subramaniam, L. Halabelian, C. H. Arrowsmith and F. Bénard, Nat. Chem. Biol., 2022, 18, 963–971 CrossRef CAS PubMed.
- T. Hempel, L. Raich, S. Olsson, N. P. Azouz, A. M. Klingler, M. Hoffmann, S. Pöhlmann, M. E. Rothenberg and F. Noé, Chem. Sci., 2021, 12, 983–992 RSC.
- G. Spraggon, M. Hornsby, A. Shipway, D. C. Tully, B. Bursulaya, H. Danahay, J. L. Harris and S. A. Lesley, Protein Sci., 2009, 18, 1081–1094 CrossRef CAS PubMed.
- W. Sun, X. Zhang, M. Cummings, K. Albarazanji, J. Wu, M. Wang, R. Alexander, B. Zhu, Y. Zhang, J. Leonard, J. Lanter and J. Lenhard, J. Pharmacol. Exp. Ther., 2020, 375, 510–521 CrossRef CAS PubMed.
- R. Hashimoto, A. Sakamoto, S. Deguchi, R. Yi, E. Sano, A. Hotta, K. Takahashi, S. Yamanaka and K. Takayama, Mol. Ther. Nucleic. Acids, 2021, 26, 1107–1114 CrossRef CAS PubMed.
- K. J. Fujimoto, D. C. F. Hobbs, M. Umeda, A. Nagata, R. Yamaguchi, Y. Sato, A. Sato, K. Ohmatsu, T. Ooi, T. Yanai, H. Kimura and T. Murata, Viruses, 2022, 14, 389 CrossRef CAS PubMed.
- P. Carter and J. A. Wells, Nature, 1988, 332, 564–568 CrossRef CAS PubMed.
- D. M. Blow, J. J. Birktoft and B. S. Hartley, Nature, 1969, 221, 337–340 CrossRef CAS PubMed.
- S. De Cesco, J. Kurian, C. Dufresne, A. K. Mittermaier and N. Moitessier, Eur. J. Med. Chem., 2017, 138, 96–114 CrossRef CAS PubMed.
- V. Salmaso and S. Moro, Front. Pharmacol., 2018, 9, 923 CrossRef PubMed.
- I. Halperin, B. Ma, H. Wolfson and R. Nussinov, Proteins, 2002, 47, 409–443 CrossRef CAS PubMed.
- M. De Vivo, M. Masetti, G. Bottegoni and A. Cavalli, J. Med. Chem., 2016, 59, 4035–4061 CrossRef CAS PubMed.
- R. Lonsdale and R. A. Ward, Chem. Soc. Rev., 2018, 47, 3816–3830 RSC.
- Y. Wang, S. Murlidaran and D. Pearlman, J. Comput. Aided Mol. Des., 2021, 35, 963–971 CrossRef CAS PubMed.
- C. H. S. da Costa, V. Bonatto, A. M. dos Santos, J. Lameira, A. Leitão and C. A. Montanari, J. Chem. Inf. Model., 2020, 60, 880–889 CrossRef CAS PubMed.
- A. Warshel and M. Levitt, J. Mol. Biol., 1976, 103, 227–249 CrossRef CAS PubMed.
- U. Ryde and P. Söderhjelm, Chem. Rev., 2016, 116, 5520–5566 CrossRef CAS PubMed.
- J. Tomasi, B. Mennucci and R. Cammi, Chem. Rev., 2005, 105, 2999–3094 CrossRef CAS PubMed.
- L. M. Mihalovits, G. G. Ferenczy and G. M. Keserű, Int. J. Quantum Chem., 2022, 122, e26768 CrossRef CAS.
- M. K. Gilson, A. Rashin, R. Fine and B. Honig, J. Mol. Biol., 1985, 184, 503–516 CrossRef CAS PubMed.
- C. A. Fitch, G. Platzer, M. Okon, B. E. Garcia-Moreno and L. P. McIntosh, Protein Sci., 2015, 24, 752–761 CrossRef CAS PubMed.
- J. Antony and S. Grimme, J. Comput. Chem., 2012, 33, 1730–1739 CrossRef CAS PubMed.
- K. Fukui, Acc. Chem. Res., 1981, 14, 363–368 CrossRef CAS.
-
M. J. Frisch, G. W. Trucks, H. B. Schlegel, G. E. Scuseria, M. A. Robb, J. R. Cheeseman, G. Scalmani, V. Barone, G. A. Petersson, H. Nakatsuji, X. Li, M. Caricato, A. V. Marenich, J. Bloino, B. G. Janesko, R. Gomperts, B. Mennucci, H. P. Hratchian, J. V. Ortiz, A. F. Izmaylov, J. L. Sonnenberg, D. Williams, F. Ding, F. Lipparini, F. Egidi, J. Goings, B. Peng, A. Petrone, T. Henderson, D. Ranasinghe, V. G. Zakrzewski, J. Gao, N. Rega, G. Zheng, W. Liang, M. Hada, M. Ehara, K. Toyota, R. Fukuda, J. Hasegawa, M. Ishida, T. Nakajima, Y. Honda, O. Kitao, H. Nakai, T. Vreven, K. Throssell, J. A. Montgomery Jr., J. E. Peralta, F. Ogliaro, M. J. Bearpark, J. J. Heyd, E. N. Brothers, K. N. Kudin, V. N. Staroverov, T. A. Keith, R. Kobayashi, J. Normand, K. Raghavachari, A. P. Rendell, J. C. Burant, S. S. Iyengar, J. Tomasi, M. Cossi, J. M. Millam, M. Klene, C. Adamo, R. Cammi, J. W. Ochterski, R. L. Martin, K. Morokuma, O. Farkas, J. B. Foresman and D. J. Fox,Gaussian 16, 2016 Search PubMed.
- L. W. Chung, W. M. C. Sameera, R. Ramozzi, A. J. Page, M. Hatanaka, G. P. Petrova, T. V. Harris, X. Li, Z. Ke, F. Liu, H.-B. Li, L. Ding and K. Morokuma, Chem. Rev., 2015, 115, 5678–5796 CrossRef CAS PubMed.
- J. Wang, P. Cieplak and P. A. Kollman, J. Comput. Chem., 2000, 21, 1049–1074 CrossRef CAS.
- J. Wang, R. M. Wolf, J. W. Caldwell, P. A. Kollman and D. A. Case, J. Comput. Chem., 2004, 25, 1157–1174 CrossRef CAS PubMed.
- C. Peng, P. Y. Ayala, H. B. Schlegel and M. J. Frisch, J. Comput. Chem., 1996, 17, 49–56 CrossRef CAS.
- Y. Zhao and D. G. Truhlar, Theor. Chem. Acc., 2008, 120, 215–241 Search PubMed.
- A. Austin, G. A. Petersson, M. J. Frisch, F. J. Dobek, G. Scalmani and K. Throssell, J. Chem. Theory Comput., 2012, 8, 4989–5007 CrossRef CAS PubMed.
- H. Eyring, J. Chem. Phys., 1935, 3, 107–115 CrossRef CAS.
- K. J. Laidler, Pure Appl. Chem., 1996, 68, 149–192 CrossRef CAS.
|
This journal is © the Owner Societies 2023 |