An experimental, computational, and uncertainty analysis study of the rates of iodoalkane trapping by DABCO in solution phase organic media†
Received
11th November 2022
, Accepted 3rd February 2023
First published on 7th February 2023
Abstract
NMR spectroscopy was used to measure the rates of the first and second substitution reactions between iodoalkane (R = Me, 1-butyl) and DABCO in methanol, acetonitrile and DMSO. Most of the reactions were recorded at three different temperatures, which permitted calculation of the activation parameters from Eyring and Arrhenius plots. Additionally, the reaction rate and heat of reaction for 1-iodobutane + DABCO in acetonitrile and DMSO were also measured using calorimetry. To help interpret experimental results, ab initio calculations were performed on the reactant, product, and transition state entities to understand structures, reaction enthalpies and activation parameters. Markov chain Monte Carlo statistical sampling was used to determine a distribution of kinetic rates with respect to the uncertainties in measured concentrations and correlations between parameters imposed by a kinetics model. The reactions with 1-iodobutane are found to be slower in all cases compared to reactions under similar conditions for iodomethane. This is due to steric crowding around the reaction centre for the larger butyl group compared to methyl which results in a larger activation energy for the reaction.
Introduction
Nuclear magnetic resonance (NMR) spectroscopy is an important tool in structural biology, for example, where it can be used to study protein folding and dynamics.1 NMR spectroscopy is also an invaluable quantitative technique that is well-established in analytical and physical chemistry research.2,3 Here, we have employed this technique to follow the time dependent behaviour of the reactants, intermediates and products3–5 of the single and double SN2 substitution of 1,4-diazabicyclo[2.2.2]octane (DABCO) reaction with iodoalkanes in solution.6–8 DABCO is a tertiary amine and strong nucleophile. This Menshutkin reaction is of interest, not only from the fundamental science perspective,6–25 but also for nuclear safety applications, since DABCO-saturated materials are used in reprocessing plant ductwork filters,26–30 and to clean up iodine spills from nuclear reactors.31–36 During nuclear accidents, volatile iodine radioisotopes can be released as inorganic iodines (I2, IxOy), and as organic iodides that are created via reactions with hydrocarbons and other volatile organic chemicals.37–46 These compounds react in the environment in different ways: homogeneous and heterogeneous reactions in the atmosphere,47–50 and substitution reactions with aquatic, soil, and plant materials in terrestrial ecosystems.51–55 The deposition rate of organic iodides is approximately 200 times smaller than that of molecular iodide50 and, consequently, these spread farther in the environment. One form of organic iodide of particular interest is iodomethane, which is the most abundant (as CH3127I) iodo-organic compound in the atmosphere,56,57 where it can influence the chemistry of ozone.47,49 An especially important radioisotope is 131I (t1/2 = 8.05 days), which poses a danger to human health,58 and needs to be captured immediately after release, since it accumulates in the thyroid gland and influences metabolic processes and presents a considerable cancer risk.58–60 The iodoalkane-DABCO reaction serves as a simple chemical model for the interaction of iodoalkanes with a wide variety of organic materials that may be found in the environment.
Here we present a multifaceted approach to determining kinetics and thermodynamic parameters for iodoalkane-DABCO SN2 reactions. Unlike previously reported DABCO reaction investigations,6–8 we consider both first and second substitutions with iodoalkanes. We report reaction enthalpies, activation energies and kinetics for the first and second substitution of DABCO with iodomethane and 1-iodobutane in the perdeuterated solvents acetonitrile-d3 (AcN), dimethyl sulfoxide-d6 (DMSO), and methanol-d4 (CD3OD). Ab initio calculations have also been performed to determine transition state and product thermodynamic parameters, and transition state and product structures. The polarizable continuum model was added to the calculation to account for solvent shell effects which are known to have a considerable effect on stabilizing molecular species compared to the gas phase.61
Our approach illustrates how mechanistic kinetic modelling can be used to predict product and reactant concentrations forward in time,62 so that concentration levels at longer reaction times may, for example, serve to inform public health decisions. Also, this modelling can be run ‘backward’ in time to predict the concentration of materials at a potential origin of a reactant in time and location. We have used 1H NMR to illustrate how in situ methods available to track the concentrations of multiple species simultaneously increases the certainty of the kinetic modelling and provides an opportunity to increase and quantify uncertainty predictions.
A Markov chain Monte Carlo (MCMC) sampling method63–65 was used to determine the distribution of rate constants and initial concentrations for a kinetic model. This uncertainty quantification (UQ) method was used to propagate the uncertainty in the integrated concentration measurements onto the kinetics parameters and allows us to interpolate and extrapolate reactant and product concentrations and their uncertainties forward and backward in time. In environmental studies, this UQ method is an invaluable tool for tracing effluent sources and final deposition in time and location.66–69
Methodology
Experimental
General.
Perdeuterated solvents were purchased as anhydrous and sealed in ampoules from either Sigma-Aldrich or Cambridge Isotope Laboratories. Iodomethane was purchased from Acros Organics, deoxygenated during three freeze–pump–thaw cycles, and stored over copper chips under inert atmosphere at −33 °C. 1-Iodobutane was purchased from Sigma-Aldrich, deoxygenated during three freeze–pump–thaw cycles, and stored in the dark in an inert atmosphere. 1,4-Diazabicyclo[2.2.2]octane (DABCO) was purchased from ACROS Organics and stored under an inert atmosphere at −33 °C. 1-Methyl-1,4-diazabicyclo[2.2.2]octyl-iodide (Me-DABCO-I) was synthesized according to the literature.70 The 1-butyl-1,4-diazabicyclo[2.2.2]octyl-iodide (Bu-DABCO-I) starting material was made in a similar manner. 1H NMR Spectra were recorded on a Varian INOVA 500 MHz spectrometer and referenced to residual proton signal of the appropriate deuterated solvent (i) CD2HCN, (ii) S(O)CD3CD2H, and (iii) CHD2OD.
Variable temperature NMR experiments
In a glove box, 1,4-diazabicyclo[2.2.2]octane (DABCO) was dissolved in the appropriate perdeuterated solvent. Next, 1.0 mL of this solution was drawn and put in an NMR tube and closed with a septum. The tube contained a reference capillary of known concentration (standardization of the reference capillary is outlined below). The tube was then inserted into the NMR instrument and equilibrated at the appropriate temperature for 30 min. Then, the first spectrum was recorded and the number of moles of DABCO was calculated. Based on this calculation, the volume of iodoalkane required for a given DABCO-to-iodoalkane molar ratio was calculated using the density of the iodoalkane reagent given in Table S1 in ESI.† Addition was accomplished by ejecting the NMR sample tubes from the magnet, injecting the iodoalkane using a microliter syringe, shaking the tube for ∼10 seconds, and then dropping the sample tube back into the magnet followed by immediate recording of a time series of spectra.
Standardization of the reference capillary (C6H3(CF3)3)
A flame-sealed capillary containing 1,3,5-tris(trifluoromethyl) benzene (C6H3(CF3)3) was put in an NMR tube containing perdeuterated AcN and 5 μL of CH2Cl2. The number of moles of the reference standard in the capillary was calculated using the following equation:2,3
Next, another 5 μL of CH2Cl2 was added and the standardization procedure was repeated. The results of the standardization procedure showed that there were 7.5 × 10−5 moles of (C6H3(CF3)3) in the reference capillary.
Temperature calibration of the NMR instrument was performed by setting the desired temperature and measuring the difference in the chemical shift of ethylene glycol signals: T = (4.637 − Δ)/0.009967 (instrument operator's manual), where Δ is the shift difference in ppm between the CH2 and OH signals.
NMR data workup
All NMR spectra were processed using Mestrenova (Mestrelabs version 14.2.0-26256, Mestrelabs Research S.L.) as follows: 1 Hz line broadening, manual phase correction, and automatic baseline correction (splines) were applied to each spectrum. These sets of data were exported for analytical analysis as .csv and .mnova files for further analysis. Concentration versus time curves from the MNova processed NMR spectra were fit to a kinetic model using the Berkeley Madonna (version 10.2.8) general purpose differential equation solver. Kinetic rate constants and starting reactant concentrations were fit as unbounded parameters.
Calorimetry
A Setaram C80 Calvet calorimeter was used to measure the heat of reaction for iodoalkane addition to DABCO in the protonated solvents AcN (CH3CN) and DMSO (S(O)CH3CH3). In a typical experiment, the two compartments of a C80 vessel were charged with stock solutions of 1.0 mL stock solution of DABCO in AcN or DMSO and 1.0 mL stock solution of iodoalkanes in the same, corresponding solvent. The reference vessel was charged with 2.0 mL of AcN or DMSO solvent. All samples were prepared in a glove box, sealed, and placed in the calorimeter at 30 °C. Once this temperature was reached and the heat flow had stabilized, the reaction was initiated by reversal mixing and data points were collected until heat flow returned to the baseline. Two experiments were conducted in AcN: 250 mM DABCO + 50 mM 1-iodobutane, and 125 mM DABCO + 50 mM 1-iodobutane. One experiment was conducted in DMSO: 250 mM DABCO + 50 mM 1-iodobutane. An additional measurement was performed using iodomethane in AcN reacting with DABCO, but the high volatility of the iodomethane called into question the results of this measurement. The DMSO stock solutions were used immediately after preparation to minimize the amount of 1-iodobutane-DMSO adduct formed slowly over time at ambient temperatures. The heat flow data was analysed using the Berkeley Madonna (version 10.2.8) general purpose differential equation solver to provide both kinetic and thermodynamic data. The heat flow rate is equal to the product of the reaction volume, the reaction enthalpy and reaction rate. The equation solver software takes into account the mixing time constant (13.7 s) and the instrument time constant (282 s) when calculating the reaction enthalpy and rate. A detailed description of this fitting procedure is given in ref. 71.
Uncertainty quantification
The uncertainty in kinetics parameters was measured by assuming their values are described by probability distributions. A statistical sampling method called Markov chain Monte Carlo (MCMC)63–65 was used to investigate the likelihood of a variety of possible values for the kinetics parameters with respect to the uncertainties in the measured concentrations and the correlations between parameters imposed by the kinetics model. These parameter distributions were then used to generate confidence intervals on the concentration profiles predicted by the model, and then the measured concentration uncertainties were calibrated until the confidence intervals explained as much of the variance in the measured data as possible. For each chemical reaction analysed, the time interval of data used to calculate the parameters was adjusted to avoid potentially inaccurate data near time 0, opting instead to allow later datapoints to constrain the initial concentrations. There are existing chemical dynamics analysis packages, such as Berkeley Madonna, which provide uncertainties on reaction rates given probability distributions on model parameters. These uncertainties are assumed and not derived from the data itself. One reason for the custom statistical approach developed herein is the added ability to quantify the impact of data error estimates on the model parameters. An assumption of perfect data can lead not only to poor fits but also underestimates of rate uncertainties.
To compare measured concentrations to the model, the time delays in the collection of NMR spectra were considered. The concentrations were estimated from peak integrals of the mean spectra, and the mean spectra averaged a set of Ne observed NMR spectra taken over a sequence of times 0 ≤ t0, t1, t2,…, tNe−1 < T, where T is the collection interval. Due to rapidly changing concentrations due to the reaction kinetics, the mean of the peak integrals of individual spectra is not equal to the integral of the mean peaks. A model of the NMR-to-concentration measurement process was created to relate the measured concentrations to the kinetics model parameters. The kinetics model (a system of nonlinear ordinary differential equations) is integrated numerically using Python or MATLAB and the second order Heun's method to discretize the model solution, a set of time-dependent functions cj(t), 0 ≤ t for the concentration of the jth species in the reaction model.72 Then, a measurement of the model analogous to averaging the species concentrations obtained from NMR peak integrals is given by
which is the arithmetic average of the concentrations predicted by the model at the times when NMR spectra were observed.
Integrated spectral peaks often introduce uncertainties on the order of 2% of the peak area. To account for the impact of concentration uncertainties, each concentration measurement cj,d is assumed to have a zero-mean Gaussian error, εj,d. The error standard deviation τj,d is initially taken to be the nominal 0.02 times the concentration magnitude, and then it is calibrated to match the predicted concentration uncertainty to the observed variation in the concentration measurements, which is described below and with key results in a following section. Due to the measurement errors, the relationship between the measured model and the experimental measurement is
. Then, the log-likelihood which models the probability of observing the measurements c1,d,…,cNs,d from each species involved in the reaction is
Concentration measurements at more than one time are required to estimate kinetics rates, and the log-likelihood of the full time series is the product of the log-likelihood at each individual time. Markov chain Monte Carlo is used to generate a sequence of predictions of kinetics parameters from correlated samples of the log-likelihood distribution. The statistics of these sequences provide uncertainties for the kinetics parameters, in particular, the mean and covariance. Notably, MCMC analysis allows the correlations between kinetics parameters to be estimated, such as coupling between reaction rates and initial concentrations.63
The uncertainty in concentration measurements is not known a priori; the nominal relative error standard deviation of τj,d = 0.02 is only a starting point. These species-dependent parameters influence the confidence intervals on the model parameters as well as the concentration profiles simulated by the model. If the model is accurate and the error parameters τj,d are correct for each species, then the confidence intervals for a given confidence level (e.g., 95%) on the concentration profiles should contain the same percentage of the concentration measurements. An estimate of the true τj,d values can be made by iteratively adjusting them until the concentration profile confidence intervals contain the same percentage of concentration measurements as the level of significance of the confidence interval.
Quantum chemistry calculations
Calculations on discrete molecular models of the SN2 reaction between DABCO and both iodomethane and 1-iodobutane were carried out using the NWChem software version 6.8.173 using the PBE0 hybrid functional74 with Grimme's DFT-D3 corrections to represent dispersive interactions75 in order to help interpret experimental observations. The augmented correlation-consistent polarized valence double zeta (aug-ccpvdz) basis set was used for all elements with the associated effective core potential for iodine.76,77 The conductor-like screening model (COSMO) of Klamt and Schüürmann was used to describe dielectric screening effects on the reactive species in a solvent environment.78,79
The enthalpies of the following chemical reactions corresponding to the first and second alkylations of the DABCO molecule (R = Me, Bu):
RI + DABCO → [DABCO–R]+ + I− |
[DABCO–R]+ + RI → [R–DABCO–R]2+ + I− |
were determined by performing full geometry optimizations of the reactants and products, followed by a frequency calculation to verify the minimum energy configuration and to calculate the enthalpy. The transition state for each reaction was determined by performing a constrained potential energy scan along the DABCO(N)–R(C) reaction coordinate followed by a saddle point search conducted at the maximum energy on the curve. A frequency calculation was performed at the determined saddle point to obtain the enthalpy and entropy of activation for comparison with experimental data.
Results
The advantage of using time-resolved 1H NMR spectroscopy to obtain kinetic rate information is the ability to perform the experiment in situ at a set temperature and the ability to track starting material, intermediates, and products over a time interval.80 This allows for a multi-component analysis to fit a single rate constant; for example, the rate of decrease in DABCO and iodomethane and the rate of increase in the formation of the product, 1-methyl-1,4-diazabicyclo[2.2.2]octyl iodide (Me-DABCO+ + I−) must fit a single rate expression.
d(MeI)/dt = −k1[MeI][DABCO] |
d(DABCO)/dt = −k1[MeI][DABCO] |
d(MeDABCOI)/dt = k1[MeI][DABCO] |
The challenge with using 1H NMR spectroscopy for kinetic rate data is the limited time resolution on short time scales (<15 seconds). The detectable concentration range is limited by the number of equivalent protons that can be integrated to provide a concentration and the need to signal average a sufficient number of transient pulses to obtain a S/N ratio that provides a minimum of 4 distinct time points to follow kinetics over the first half-life. In our experiments, DABCO solution without added iodoalkane was equilibrated inside of the magnet at the desired temperature, followed by the tuning and shimming of the instrument. Next, the sample was quickly ejected, a measured quantity of iodoalkane was injected into the 5 mm NMR tube. After shaking tube manually to rapidly mix its contents, the NMR tube containing iodoalkane and DABCO was reinserted into the magnet. The whole process required about 15 seconds before accumulation of the first data point. For the NMR measurements, eight transients were coadded, setting the average time resolution for the NMR data sets at 23 seconds per time point. From these limitations, the ability to record 4 data points over the first half-life, and a minimum starting concentration of 0.01 M DABCO, we were limited to measuring rate constants <1 M−1 s−1 for an expected half-life of ca. 100 seconds for a second order reaction.
t1/2 ≈ 1/k[DABCO] = 1/(1 M−1 s−1 × [0.01 M]) |
Based on the rate constants reported in the literature,7 the first substitution reaction of MeI + DABCO in AcN (k1ca. 2.05 M−1 s−1 at 30 °C) was expected to be too fast to be reliably followed by NMR spectroscopy; however, kinetics data could be recorded for the reaction run in methanol (k1ca. 0.014 M−1 s−1 at 30 °C). We were unable to record kinetic data for the second substitution of DABCO with MeI, since the di-substituted (Me2DABCOI2) product precipitates out of AcN solution after reaching saturation. Disubstituted species always precipitate out of solution when acetonitrile is the solvent, no matter the temperature. Therefore, we measure the second substitution rates, k2, by starting from the well-defined monosubstituted species so that there is a species whose disappearance can be monitored by NMR with a high degree of confidence. The rate constant for the first substitution of DABCO with BuI was measured in AcN and DMSO at three temperatures (see Table 1). With methanol as the solvent, only the rate at 55 °C was measured and was k1 = 0.41 × 10−3 M−1 s−1. Based on this result, we did not attempt to measure the reaction at lower temperatures, hypothesizing that the degree of observable change (via the NMR method) would be too small to provide high-confidence results. The second substitution of DABCO with BuI progresses too slowly to measure it confidently in AcN or methanol; it could be measured, however, in DMSO. The rates, for both the mono-substitution and di-substitution reactions, increase as DMSO > AcN > CH3OH. Fig. 1 shows an example NMR data set collected for the first substitution of DABCO with BuI in methanol at 55 °C. Fig. 2 shows an example of a Berkeley Madonna fit of reactants and product concentrations versus time for DABCO + 1.5 BuI in AcN at 51 °C.
Table 1 Reaction rates (M−1 s−1) (with ±1σ uncertainties) for DABCO and iodoalkane reactions in various solvents
|
AcN-d3 |
DMSO-d6 |
Methanol-d4 |
Diff. eq. |
MCMC |
Diff. eq. |
MCMC |
Diff. eq. |
MCMC |
DABCO + MeI |
Too fast |
|
Too fast |
|
|
|
k
1
|
|
|
9.33 × 10−3 [30 °C] |
9.65(25) × 10−3 |
k
1
|
|
|
2.081 × 10−2 [45 °C] |
2.156(79) × 10−2 |
k
1
|
|
|
3.309 × 10−2 [55 °C] |
3.400(253) × 10−2 |
|
DABCO + BuI |
|
|
Too fast |
|
|
|
k
1
|
1.468 × 10−2 [30 °C] |
1.389(96) × 10−2 |
|
|
|
k
1
|
3.073 × 10−2 [51 °C] |
2.856(38) × 10−2 |
|
4.1 × 10−4 [55 °C] |
3.0(1.2) × 10−4 |
k
1
|
7.181 × 10−2 [78 °C] |
6.856(477) × 10−2 |
|
|
|
|
MeDABCOI + MeI |
|
|
|
|
|
|
k
2
|
1.00 × 10−3 [30 °C] |
1.02(7) × 10−3 |
5.098 × 10−2 [30 °C] |
4.981(235) × 10−2 |
5.20 × 10−5 [30 °C] |
5.26(37) × 10−5 |
k
2
|
1.93 × 10−3 [51 °C] |
1.84(9) × 10−3 |
1.094 × 10−1 [50 °C] |
9.351(525) × 10−2 |
1.31 × 10−4 [45 °C] |
1.251(89) × 10−4 |
k
2
|
4.28 × 10−3 [78 °C] |
3.55(30) × 10−3 |
1.996 × 10−1 [70 °C] |
1.7804(1127) × 10−1 |
2.37 × 10−4 [55 °C] |
2.152(64) × 10−4 |
|
BuDABCOI + BuI |
Too slow |
|
|
|
Too slow |
|
k
2
|
|
2.4 × 10−4 [30 °C] |
2.0(1) × 10−4 |
|
k
2
|
|
8.4 × 10−4 [50 °C] |
7.4(6) × 10−4 |
|
k
2
|
|
2.16 × 10−3 [70 °C] |
2.04(5) × 10−3 |
|
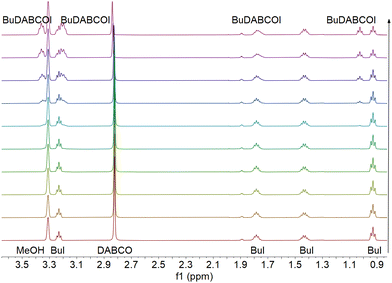 |
| Fig. 1 An example of the NMR data collected during first substitution of DABCO with BuI in methanol-d4 at 55 °C. The progression of spectra moving up is increasing with time. Overall, 102 spectra were recorded over 66 h and only select spectra are shown above. | |
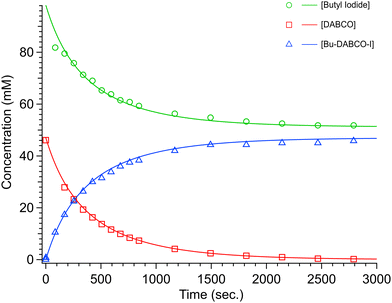 |
| Fig. 2 Concentration (mM) vs. time (s) plot for DABCO + 1.5 BuI in AcN at 51 °C. The open circles, triangles and squares represent collected experimental data. The solid traces are the Berkeley Madonna fit to the data. | |
The raw NMR data were processed with MNova as described above to determine concentrations and then analysed using the Berkeley Madonna software and MCMC methods to determine the reaction rate constants and initial concentrations. The temperature-dependent data were used to create Eyring and Arrhenius plots to determine reaction enthalpies and reaction activation parameters, respectively. The rate constants are presented in Table 1, the thermodynamic parameters in Table 2, and the initial reactant concentrations as determined by NMR in Table 3. See the ESI† documents for this article for further results details.
Table 2 Activation energies, Gibbs energy parameters, and reaction enthalpies, (with ±1σ uncertainties) for DABCO and iodoalkane reactions in various solvents. See the ESI for a discussion of the uncertainties. Quantities marked with a * were measured using calorimetry
|
AcN-d3 |
DMSO-d6 |
Methanol-d4 |
Vacuum |
Exp. |
Calc. |
Exp. |
Calc. |
Exp. |
Calc. |
Calc. |
DABCO + MeI |
Too fast |
|
Too fast |
|
|
|
|
E
a (kJ mol−1) |
|
24.9 |
|
23.8 |
42(4) |
30.3 |
68.7 |
ΔH‡ (kJ mol−1) |
|
26.7 |
|
22.6 |
39(4) |
29.1 |
70.1 |
ΔS‡ (J mol−1 K−1) |
|
−17.0 |
|
−24.3 |
−154(11) |
−35.0 |
−64.7 |
ΔHrxn (kJ mol−1) |
|
−147 |
|
−140 |
|
−141 |
+31 |
|
DABCO + BuI |
|
|
|
|
Too slow |
|
|
E
a (kJ mol−1) |
29(2) |
35.2 |
|
34.2 |
|
38.4 |
83.2 |
ΔH‡ (kJ mol−1) |
26(4) |
35.9 |
|
37.1 |
|
38.7 |
86.8 |
ΔS‡ (J mol−1 K−1) |
−193(6) |
−53.0 |
|
−34.0 |
|
−40.4 |
−28.8 |
ΔHrxn (kJ mol−1) |
−126*/−122* |
−128 |
−123* |
−127 |
|
−125 |
−18 |
|
MeDABCOI + MeI |
|
|
|
|
|
|
|
E
a (kJ mol−1) |
26(2) |
35.6 |
30(2) |
|
50(4) |
36.5 |
121 |
ΔH‡ (kJ mol−1) |
24(2) |
24.6 |
27(2) |
|
47(4) |
24.6 |
110 |
ΔS‡ (J mol−1 K−1) |
−224(7) |
−127 |
−181(6) |
|
−171(11) |
−147 |
−92.5 |
ΔHrxn (kJ mol−1) |
|
−110 |
|
|
|
−102 |
+102 |
|
BuDABCOI + BuI |
Too slow |
|
|
|
Too slow |
|
|
E
a (kJ mol−1) |
|
|
48(2) |
|
|
|
+210 |
ΔH‡ (kJ mol−1) |
|
|
45(2) |
|
|
|
|
ΔS‡ (J mol−1 K−1) |
|
|
−166(7) |
|
|
|
|
ΔHrxn (kJ mol−1) |
|
|
|
|
|
|
|
Table 3 Initial concentrations (mM) of DABCO and monosubstituted DABCO, as determined by NMR method; concentrations of iodoalkane were calculated as outlined in the experimental section. MCMC initial concentration uncertainties are ± 1σ
|
NMR |
MCMC |
BuI concentration could not be tracked for this reaction.
|
|
AcN-d3 |
DABCO + 1.5BuI |
DABCO |
BuI |
DABCO |
BuI |
BuDABCOI |
30 °C |
35.3 |
53.0 |
39.0(2) |
68.8(1.7) |
1(7) |
51 °C |
46.1 |
69.2 |
42.5(9) |
94.4(9) |
3.1(7) |
78 °C |
57.3 |
86.0 |
42.7(2.2) |
115.5(2.3) |
3.7(2.2) |
|
MeDABCOI + MeI |
MeDABCOI |
MeI |
MeDABCOI |
MeI |
Me2DABCOI2 |
30 °C |
57.8 |
57.8 |
61.6(3.6) |
79.1(2.9) |
2.2(1.4) |
51 °C |
64.5 |
64.5 |
63.1(3.0) |
92.2(4.4) |
3.2(2.5) |
78 °C |
68.3 |
68.3 |
63.8(7) |
96(17) |
2.0(1.1) |
|
|
DMSO-d6 |
DABCO + 2BuI |
DABCO |
BuI |
DABCO |
BuI |
BuDABCOI |
30 °C |
50.1 |
100.2 |
52.0(2.4) |
|
0.8(1.2) |
50 °C |
47.6 |
95.2 |
47.9(1.9) |
|
0.4(6) |
70 °C |
50.4 |
100.8 |
48.2(2.1) |
|
1.1(9) |
|
MeDABCOI + MeI |
MeDABCOI |
MeI |
MeDABCOI |
MeI |
Me2DABCOI2 |
30 °C |
9.38 |
15.8 |
8.7(3) |
26.4(4) |
0.7(2) |
50 °C |
8.75 |
16.5 |
8.8(3) |
19.6(5) |
1.8(3) |
70 °C |
9.75 |
13.5 |
10.5(3) |
17.0(3) |
0.3(2) |
|
|
Methanol-d4 |
DABCO + MeI |
DABCO |
MeI |
DABCO |
MeI |
MeDABCOI |
30 °C |
20.4 |
20.4 |
17.5(2) |
27.7(2) |
0.05(4) |
45 °C |
22.3 |
22.3 |
19.1(3) |
32.6(3) |
0.11(9) |
55 °C |
22.3 |
22.3 |
19.3(8) |
29.8(5) |
0.08(7) |
|
DABCO + BuI |
DABCO |
BuI |
DABCO |
BuI |
BuDABCOI |
55 °C |
21.0 |
21.0 |
21.0(5) |
28.5(8) |
0.9(6) |
|
MeDABCOI + 2MeI |
MeDABCOI |
MeI |
MeDABCOI |
MeI |
Me2DABCOI2 |
30 °C |
12.8 |
25.6 |
14.1(2.1) |
36.0(2.0) |
0.2(4) |
45 °C |
13.9 |
27.8 |
13.7(1.9) |
44.4(1.7) |
0.1(3) |
55 °C |
13.9 |
27.8 |
14.7(2.0) |
38.1(1.2) |
0.7(4) |
For Berkeley Madonna simulations, reactions were assumed to be second order and both reactants and products were fit to experimental data. Starting concentration of the product was set to 0 and concentrations of both reactants were fit without constraints.
The uncertainty quantification (UQ) method detailed in the Methodology section was used to propagate the uncertainty in the integrated concentration measurements onto the kinetics parameters (rate coefficients and initial concentrations). The kinetics parameters sampled by the MCMC procedure provided estimates and confidence intervals when analysed as a distribution. When these samples were used to generate confidence intervals on the concentration profiles, these bounds were used to scale the assumed concentration measurement uncertainty for each species until the percentage coverage of the measurements approximately matched the confidence level of the confidence interval. The joint probability distribution of the kinetics and initial concentration parameters were evaluated by estimating the histograms of the sampled parameters in Fig. 3. For each pair of parameters, the 1σ and 2σ confidence regions of the distribution were plotted and contain approximately 68% and 95% of the samples, respectively. Qualitatively, thin confidence regions slanted to the upper-right suggest the two parameters are positively correlated, while vertical or horizontal orientations suggest the two parameters are not correlated. Along each axis are the marginal probability distributions of each parameter, from which confidence intervals on those parameters are defined (see Tables 1 and 3).
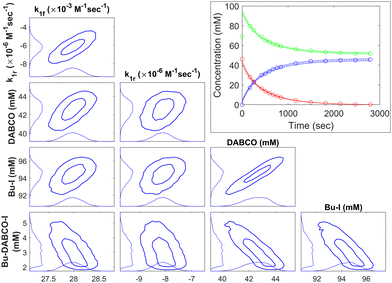 |
| Fig. 3 Distribution of kinetics parameters and concentration profiles for BuI + DABCO (first substitution) in AcN at 50 °C due to uncertainty in concentration measurements: (left) covariances between kinetics parameters in each panel, and marginal distributions for each parameter along each axis, and (right) concentration data (circles) and concentration mean (dashed) and 95%-confidence intervals (solid). | |
For example, for BuI + DABCO (first substitution) in AcN at 50 °C, the kinetics rate and concentration confidence intervals are plotted in Fig. 3. The rate coefficients and initial conditions showed at least some correlation. Correlation was most significant among the initial conditions. This is consistent with the coupling of these parameters by the kinetics model and conservation of mass and can be predicted by a perturbation sensitivity analysis of the model. The initial concentration of the product, BuDABCOI, shows significant positive skewness, which is to be expected for a positive-definite parameter with sufficient variance. The confidence bounds on the concentration show coverage over 90–95% of the data (with the notable exception of the initial concentration for the product). This consistency is lost if the first three data points (including the initial concentration) are included in the dataset.
The 30 °C calorimetry results for the 250 mM DABCO + 50 mM 1-iodobutane measurements in AcN and DMSO are shown in Fig. 4. The results for the 125 mM DABCO + 50 mM 1-iodobutane are given in the ESI† for this article. The solid trace in these figures is from the Berkeley Madonna fit (see the Methodology section) and the markers are the measured data points. A datum was collected every second, but for clarity on the plots, markers are shown for every sixteenth data point. The 250 mM DABCO + 50 mM 1-iodobutane and 125 mM DABCO + 50 mM 1-iodobutane calorimetry measurements gave enthalpies and reaction rates of ΔH = −126 kJ mol−1, k1 = 0.015 M−1 s−1 and ΔH = −122 kJ mol−1, k1 = 0.016 M−1 s−1, respectively, showing good agreement between the fit constants for the reaction in AcN. For the 250 mM DABCO + 50 mM 1-iodobutane in DMSO reaction, the calorimetry data give ΔH = −123 kJ mol−1 and k1 = 0.067 M−1 s−1. These reaction enthalpies are reported in Table 2. The reactant vessels in the C80 are open to one another in the reaction chamber, and when we tried to measure the reaction rates and enthalpies of iodomethane with DABCO we got results that are far from those of Kondo and co-workers.7 We suspect that the volatile iodomethane was pre-reacting with DABCO before the measurement started.
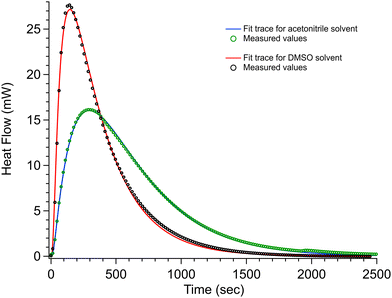 |
| Fig. 4 Calorimetry data for the reaction 250 mM DABCO + 50 mM 1-iodobutane using AcN and then DMSO as solvents. The data were recorded using a Setaram C80 Calvet calorimeter operating at an equilibrium temperature of 30 °C. The markers in the plot are the measured data points and the solid lines are from the Berkeley Madonna fit of the data. For clarity, only 1/16 of the total number of data points are shown on the plot. In AcN, k1 = 0.015 M−1 s−1, ΔH = −126 kJ mol−1, and in DMSO k1 = 0.067 M−1 s−1, ΔH = −123 kJ mol−1. | |
The computational chemistry results provide several important insights into the reaction mechanism at the molecular level. The mechanism is thought to proceed via the reaction of two neutral molecules (iodoalkane and DABCO) reacting through an SN2-type mechanism to produce two charged species (a quaternary ammonium cation and the iodide anion). The classical view of the SN2 mechanism involves the attack of a nucleophile (in this case the amine nitrogen) at the backside of the reaction centre followed by the expulsion of an appropriate leaving group (iodide). Our calculations suggest that this is a good model for the process and show reasonable agreement with our calculated thermodynamic and kinetic parameters with available experimental data. The structure of the calculated transition state shows the expected approximate sp2-hybridized planar geometry at the reaction centre (see Fig. 5(a) for iodomethane). Given the significant change in charge during the reaction it might be expected that the polarity of the solvent would play a role in the mechanism, and our calculations predict that is the case. Calculations in the gas phase predict a high barrier and positive or thermoneutral reaction free energies, whereas with the introduction of a continuum solvent model, the computed activation energies are reduced, and the reactions become more exothermic. This is likely due to the stabilization of the transition state and the reaction products, which have significant polar character. The change in activation energies can be related to the dielectric constant of the solvent, with dimethyl sulfoxide showing the largest effect; however, the dependence for the enthalpy of the reaction is less clear. There are some notable differences between the calculated mechanisms for 1-iodobutane compared to iodomethane. The addition of a butyl group to the reaction centre requires considerable conformational reorganization to accommodate nucleophilic attack (Fig. 5(b)) with the attacking amine centre approaching at a different angle. The presence of the additional alkyl chain also introduces increased opportunities for dispersive interactions between the components that can stabilize other geometries. For example, once the reaction is complete, iodide can shift away from the ammonium cation to exploit increased binding interactions with the side of the alkylated species.
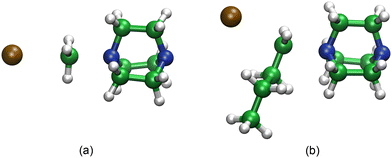 |
| Fig. 5 Computed transition states for (a) MeI and DABCO, and (b) BuI and DABCO. | |
Discussion
This study details a method for integrating experimental measurements of the kinetics of a relatively simple chemical reaction with computational methods and uncertainty quantification tools to develop predictive models that can be used to extrapolate chemical change both forward and backward in time as a function of temperature when only a limited set of experimental data is available. We also considered the effect of different solvent environments on the reaction rate, which has been known for a considerable time to significantly change kinetics.81,82
Our incorporation of the techniques of uncertainty quantification in the analysis provide considerable flexibility and value to our method. For example, complications arising from an inability of the NMR measurement to capture the initial data points in the time series at the beginning of the reaction, and also solubility limits causing the possible precipitation of reaction products can be included in the kinetic analysis in a systematic way and used to estimate errors. This approach allowed us to put an approximate uncertainty on the initial concentration of a reactant to ascertain how precise the measurement was for our experimental technique. Usually, the uncertainty would be obtained by performing multiple measurements under the same conditions, which is not always possible, for example, when performing studies to inform emergency response for a radioactive iodine release.
Computed activation energies, in general, follow the experimentally observed trend, with reactions in DMSO being the fastest and in methanol the slowest. Calculations for reactions performed in vacuum appear to have the most unfavourable enthalpy changes, suggesting that in the absence of solvent the activated complex cannot be sufficiently stabilized. The measured and calculated negative values of the entropy of activation in each solvent support the assumption that the formation of the transition state is via an associative mechanism consistent with the SN2 mechanism.
This study is also consistent with previous studies that have shown the difficulty of using computational methods to obtain accurate entropies of activation (and by extension the Gibbs free energy of activation).83 For example, East and co-workers illustrate the problem using a different SN2 reaction and show that errors of 8.4 kJ mol−1 lead to a change in the rate constants of a factor of 30. The rates depend on the exponential of the thermodynamic parameters (Eyring equation), so small changes in parameters can result in large changes in predicted rate constants.84
The monosubstitution of DABCO with iodomethane at 30 °C was studied by Kondo and co-workers,7 in the 1980s. The focus of that paper was the influence of the solvent medium on the reaction rates between iodomethane and a base in the Menschutkin reaction. The authors compared single solvents as well as the binary mixtures of protonated AcN and protonated MeOH and concluded that the monosubstitution is about 150 times faster in AcN, even though the dielectric constants of these two solvents are very similar. We observe a similar trend, with good agreement with Kondo and co-workers for MeI reaction with DABCO in methanol-d4 at room temperature and the rate in AcN is too fast for us to measure by NMR. However, we observe a different temperature dependence with our measured rates, slower at higher temperatures than Kondo reports resulting in different activation parameters, ΔH‡ = 39.2(9) kJ mol−1 (Kondo ΔH‡ = 59.8 kJ mol−1) and ΔS‡ = −154(3) J K−1 mol−1 (Kondo ΔS‡ = −83 J K−1 mol−1). The resulting difference in rates for MeI addition to DABCO in methanol at 60 °C, as calculated from the activation parameters, has the Kondo reaction rate greater than our rate by a factor of 2.7, which is outside our uncertainty limits obtained from simultaneously fitting the disappearance of DABCO and MeI in addition to the appearance of MeDABCOI. In our case we know that it is difficult to measure the initial concentration of iodomethane due to its high volatility. However, our UQ analysis permits us to obtain the initial concentration of MeI by fitting a series of reactions tied to the observed final concentration of the product, MeDABCOI. Specifically, we assume that all the MeI present in the experiment is trapped by DABCO and thus the starting concentration of MeI should be equal to the final concentration of MeDABCOI. Furthermore, NMR analysis allows us to rule out the formation of other products since no new unidentified peaks are observed in the spectrum.
Another factor to consider when comparing our measured rates in deuterated solvents and Kondo's rates measured in the corresponding protonated solvents are kinetic isotope effects (KIE) on the reaction rate. We observe k1 rates of 0.015 and 0.016 M−1 s−1 for two independent BuI + DABCO C80 experiments in CH3CN and it agrees well with our NMR experiment k1 = 0.014 in CD3CN. So, there is no KIE in AcN, but there could very well be a KIE in a protic solvent CD3OD vs. CH3OH. To follow the evolution of multiple species by 1H NMR, that is, DABCO, MeI and MeDABCOI, we used deuterated solvents. However, to test the possibility of a solvent KIE as the observed difference in our rates compared to Kondo, we repeated the experiment at 55 °C in CH3OH, where we observe the greatest difference in rates with Kondo. In this case the protons in the solvent CH3OH ‘swamp’ the proton chemical shifts of MeI and MeDABCOI thus we can only follow the reaction with one parameter, the decay of DABCO. The data for the reaction is shown in the ESI† file SUPPLEMENTARY_INFORMATION_KINETICS_PLOTS_v3, and see the DABCO_MeI_CH3OH_55C worksheet. Also, see the MCMC analysis starting on page S3 of the ESI.† The Berkeley Madonna analysis of this data gave a reaction rate value of k1 = 6.0 × 10−2 M−1 s−1 at 55 °C. The MCMC analysis of the same data set gave a rate value of 5.39(1.01) × 10−2 M−1 s−1 at 55 °C. While we do observe a faster rate for disappearance of DABCO in CH3OH, k1 = 5.39(1.01) × 10−2 M−1 s−1 at 55 °C compared to the observed rate in CD3OD, k1 = 3.400(253) × 10−2 M−1 s−1 at 55 °C, the rate is still about half the rate we calculate from Kondo's activation parameters, ca. 1.2 × 10−1 M−1 s−1 at 55 °C. Even though the data from a single parameter in CH3OH has a higher degree of uncertainty, it is faster than the rate we measure in CD3OD, suggesting a KIE; however, it is still slower than the rate measured by Kondo, so this control was not able to explain the discrepancy in observed rates. However, the focus of this paper is on using multiple parameters to obtain measured rates to enhance the UQ to make predictions both forward and backward in time to understand the initial concentrations and when concentrations are sufficiently low to provide a possible prediction of iodine compound concentrations in the environment.
The rates of reaction of BuI with DABCO are significantly slower as expected for an SN2 reaction between a bulkier butyl group of 1-iodobutane compared to iodomethane. For example, the reaction of BuI with DABCO in AcN-d3k1 (30 °C) ca. 1.389(96) × 10−2 M−1 s−1 (MCMC value) is 2 orders of magnitude slower than the rate of reaction of MeI with DABCO in AcN reported by Kondo (2.2 M−1 s−1).7
The time-resolved calorimetry experiments used in this study are slightly different than the approach used by Kondo. Kondo had the iodoalkane (MeI) in a sealed ampule in the calorimeter with a solution of DABCO. After the instrument baseline stabilized, they were able to break the seal of the ampule to mix the two reactants. For the calorimeter used in this study, the iodoalkane and the DABCO were separated into two different chambers, but these chambers were open to one another in the calorimeter. Due to the volatile nature of MeI, the reaction started before the baseline was able to stabilize and we were unable to measure the ΔHrxn for MeI to compare directly. However, 1-iodobutane is less volatile and a stable baseline was established before any significant reaction could take place. Thus, when the C80 instrument was changed into a mixing mode, the experiment provides a heat of reaction, ΔHrxnca. −123 kJ mol−1, for BuI with DABCO that is in good agreement with the heat of reaction for MeI reacting with DABCO in AcN reported by Kondo.7 The C80 approach further provides an alternate measurement of the reaction rate that is in good agreement with the NMR measured rate.
Looking in more detail at our data sets, we can see that comparison of the experimental and calculated kinetic parameters for the first substitution reaction of iodomethane and DABCO in methanol shows the best agreement between theory and experiment, and also shows a good comparison with previously reported values in the literature. For example, the reported value of k1 by Kondo and co-workers is 1.37 × 10−2 M−1 s−1,7 while the k1 obtained from our NMR measurement is 9.65(25) x 10−3 M−1 s−1, although there appears to be some ambiguity in the treatment of units in the previous study.7 Our work also uses in situ measurements of concentrations of reactants and products as a function of time rather than the ex situ quenching method employed by Kondo and co-workers.7
In the case of 1-iodobutane, examination of the calculated MCMC values of k1 and k2 rate constants (Table 1) show close correlation to the values obtained by experimental 1H NMR methods.
In our approach, we included not only protic and aprotic solvents of differing dipole moments (for example, methanol and acetonitrile, Table S1 in ESI†) but also two aprotic solvents of similar dipole moments (acetonitrile and dimethyl sulphoxide). The range of solvent effects on the reactions can make data collection challenging in some cases for our experimental method. For example, in dimethyl sulphoxide, the first substitution of DABCO molecule is too fast to be observed by NMR spectroscopy, while in methanol, the same reaction occurs at a much slower rate. We attribute this result to the ability of DMSO to stabilize both the activated complex and charged products. Other complications can arise from the nature of the solvent. For example, in the case of methanol, the hydroxyl group can hydrogen bond to the basic nitrogen of the DABCO, potentially blocking approach of the iodoalkane required to enable the SN2 reaction. In addition, dimethyl sulphoxide is known to react with iodomethane forming alkoxysulfonium salts via a sulphur ylide.85 While the presence of this side product diminishes the concentration of available iodoalkane, it doesn’t seem to have a significant influence on the overall kinetics of the reaction. This is probably because iodoalkane is always added in slight excess.
To support the idea that the iodoalkanes react with DMSO, we performed additional, “blank” reactions without the presence of DABCO. In this reaction, a drop of 1-iodobutane was added to DMSO-d6 and a spectrum was recorded. An additional spectrum was taken after ∼48 h with the NMR tube standing at room temperature. In addition to the signals from the 1-iodobutane, a new set of 1H NMR signals appeared (Fig. S1 in ESI†). No attempt was made to assign these or ascertain a structure for the reaction product. For iodomethane reacting with DABCO in DMSO solvent, some signals in our 1H NMR spectra were consistent with those in the literature where a trimethyl-oxosulfonium iodide ylide was formed when CH3I was reacted with DMSO at room temperature.85
Conclusions
Considering one example of simple substitution reactions, we have illustrated the utility of NMR spectroscopy as a powerful tool to obtain kinetic data.80 Using the first and second substitution of DABCO with iodoalkanes in three different solvents and at three different temperatures, we have obtained raw data which was then refined in secondary software and used in kinetic modelling and uncertainty analysis. We have shown that while it is possible to obtain kinetic parameters using programs like Berkeley Madonna, it is not straightforward to ascertain uncertainty of the model using the same set of software. It is possible however, to analyse data using MCMC sampling and create a more accurate reaction profile that accounts for uncertainties of the experiment. Taken together, it is compelling to imagine that such a robust approach could be a useful tool to provide a degree of uncertainty analysis to predict the concentrations of reactants, intermediates, and products in both the forward and reverse directions when a limited amount of time-dependent data are available.
Author contributions
TA conceptualized the study and secured the funding. KG performed the NMR measurements. TA and KK performed the calorimetry measurements and TA analysed this data using Berkeley Madonna. TA and KG performed the initial kinetics analysis of the NMR data using Berkeley Madonna. NJH performed the ab initio calculations. WSR performed the uncertainty quantification and kinetics modelling. KG, SF and WSR performed the NMR data reduction. KG and TAB made the Eyring and Arrhenius plots and the uncertainty analysis for these. KG and TAB wrote the initial draft of the paper. KG, WSR, TA, NJH and TAB reviewed and edited the paper. All authors were responsible for data interpretation.
Conflicts of interest
There are no conflicts to declare.
Acknowledgements
The Pacific Northwest National Laboratory is a multiprogram national laboratory operated for the U.S. Department of Energy by the Battelle Memorial Institute under Contract DE-AC05-76RL01830. This research was supported by PNNL's Laboratory Directed Research and Development program, Chemical Dynamics Initiative. The authors would like to thank Drs Marvin Warner and Wendy Shaw for their support and guidance of this research. The authors would also like to thank Drs Yongsoon Shin and Abhi Karkamkar for sample preparation and preliminary measurements, and Dr Nancy M. Washton for help with NMR data analysis.
References
-
J. Cavanagh, N. Skelton, W. Fairbrother, M. Rance and A. Palmer, Protein NMR Spectroscopy: Principles and Practice, Academic Press, New York, NY, 2nd edn, 2006 Search PubMed.
-
H. Gunther, NMR Spectroscopy: Basic Principles, Concepts and Applications in Chemistry, Wiley-VCH, New York, NY, 3rd edn, 2013 Search PubMed.
- S. K. Bharti and R. Roy, Quantitative 1H NMR Spectroscopy, Trends Anal. Chem., 2012, 35, 5–26, DOI:10.1016/j.trac.2012.02.007.
- F. Susanne, D. S. Smith and A. Codina, Kinetic Understanding Using NMR Reaction Profiling, Org. Process Res. Dev., 2012, 16, 61–64, DOI:10.1021/op200202k.
- D. A. Foley, A. L. Dunn and M. T. Zell, Reaction Monitoring Using Online vs. Tube NMR Spectroscopy: Seriously Different Results, Magn. Reson. Chem., 2016, 54, 451–456, DOI:10.1002/mrc.4259.
- J.-Y. Kazok, M. Taggougui, B. Carre, P. Willmann and D. Lemordant, Simple and Efficient Synthesis of N-Quaternary Salts of Quinuclidinium Derivatives, Synthesis, 2007, 3776–3778, DOI:10.1055/s-2007-990908.
- Y. Kondo, R. Uematsu, Y. Nakamura and S. Kusabayashi, Menshutkin Reactions of Bicyclic Aliphatic Amines and of Pyridine Derivatives with Methyl Iodide. Extended Brønsted Treatments and Isokinetic Relationships in Acetonitrile-Methanol, J. Chem. Soc., Perkin Trans. 2, 1988, 1219–1224, 10.1039/P29880001219.
- M. Hou, K. Jin, Q. Li and S. Liu, Systematic Study of the Substitution Effect on the Tetrel Bond Between 1,4-Diazabicyclo[2.2.2]octane and TH3X, RSC Adv., 2019, 9, 18459–18466, 10.1039/C9RA03351C.
- E. M. Arnett, W. G. Bentrude, J. J. Burke and P. McC. Duggleby, Solvent Effects in Organic Chemistry. V. Molecules, Ions, and Transition States in Aqueous Ethanol, J. Am. Chem. Soc., 1965, 87, 1541–1553, DOI:10.1021/ja01085a024.
- A. J. Parker, Protic-Dipolar Aprotic Solvent Effects on Rates of Bimolecular Reactions, Chem. Rev., 1969, 69, 1–32, DOI:10.1021/cr60257a001.
- C. G. Swain and N. D. Hershey, Effect of Steric Hinderance on the Structure of Transition States, J. Am. Chem. Soc., 1972, 94, 1901–1905, DOI:10.1021/ja00761a019.
- Y. Kondo, M. Ohnishi and N. Tokura, Solvent Effects Under High Pressures. IV. A Procedure for the Estimation of Solvation in the Transition State, Bull. Chem. Soc. Jpn., 1972, 45, 3579–3583, DOI:10.1246/bcsj.45.3579.
- M. H. Abraham, Solvent Effects on Transition States and Reaction Rates, Prog. Phys. Org. Chem., 1974, 11, 1–87, DOI:10.1002/9780470171905.ch1.
- M. H. Abraham and P. L. Grellier, Substitution at Saturated Carbon. Part XX. The Effect of 39 Solvents on the Free Energy of Et3N, EtI, and the Et3N−EtI Transition State. Comparison with Solvent Effects on the Equilibria Et3N + EtI ↔ Et4N + I− and Et3N + EtI ↔ Et4N+ + I−, J. Chem. Soc., Perkin Trans. 2, 1976, 1735–1741, 10.1039/P29760001735.
- Y. Kondo, M. Shinzawa and N. Tokura, The Reaction of 4-Thiazoline-2-thione with Methyl Iodide. Solvent Effects and Pressure Effects, Bull. Chem. Soc. Jpn., 1977, 50, 713–717, DOI:10.1246/bcsj.50.713.
- M. H. Abraham and A. Nasehzadeh, Early or Late Transition States in the Menschutkin Reaction, A Resolution of the Entropy Problem, J. Chem. Soc., Chem. Commun., 1981, 905–906, 10.1039/C39810000905.
- Y. Kondo, M. Ittoh and S. Kusabayashi, Reaction of Ethyl Iodide with Bromide Ion: Kinetics, Transfer Enthalpies of Anions and Extended Brønsted Relationships in Acetonitrile + Methanol and N,N-Dimethylacetamide + Methanol Mixtures, J. Chem. Soc., Faraday Trans. 1, 1982, 78, 2793–2806, 10.1039/F19827802793.
- Y. Kondo, M. Ogasa and S. Kusabayashi, Menshutkin Reaction of Triethylamine and of Pyridine with Methyl Iodide. Activation Enthalpy versus Activation Entropy Correlations and Extended Brønsted Treatments in Acetonitrile-Methanol Mixtures, J. Chem. Soc., Perkin Trans. 2, 1984, 2093–2097, 10.1039/P29840002093.
- Y. Kondo, A. Zanka and S. Kusabayashi, Reaction of Triphenylphosphine with Methyl Iodide. Transfer Thermodynamic Quantities and Various Extended Brønsted Treatments, J. Chem. Soc., Perkin Trans. 2, 1985, 827–832, 10.1039/P29850000827.
- Y. Kondo, H. Kambe and S. Kusabayashi, Menshutkin Reactions of Aliphatic Diamine with Methyl Iodide in Acetonitrile-Methanol Mixtures. Evaluation of the Role of the Non-Reacting Nitrogen Atom, J. Chem. Soc., Perkin Trans. 2, 1990, 915–919, 10.1039/P29900000915.
-
E. V. Anslyn and D. A. Dougherty, Modern Physical Organic Chemistry, University Science Books, Mill Valley, CA, 2006 Search PubMed.
- A. Melo, A. J. I. Alfaia, J. C. R. Reis and A. R. T. Calado, Unusual Solvent Effect on a SN2 Reaction. A Quantum Mechanical and Kinetic Study of the Menshutkin Reaction between 2-Amino-1-Methylbenzimidazole and Iodomethane in the Gas Phase and In Acetonitrile, J. Phys. Chem. B, 2006, 110, 1877–1888, DOI:10.1021/jp055660a.
- K. J. Stanger, J.-J. Lee and B. D. Smith, Dramatic Acceleration of the Menschutkin Reaction and Distortion of Halide Leaving-Group Order, J. Org. Chem., 2007, 72, 9663–9668, DOI:10.1021/jo702090p.
- O. Acevedo and W. L. Jorgensen, Exploring Solvent Effects upon the Menshutkin Reaction Using a Polarizable Force Field, J. Phys. Chem. B, 2010, 114, 8425–8430, DOI:10.1021/jp100765v.
- S. Giri, R. Inostroza-Rivera, B. Herrera, A. S. Nunez, F. Lund and A. Toro-Labbe, The Mechanism of Menshutkin Reaction in Gas and Solvent Phases from the Perspective of Reaction Electronic Flux, J. Mol. Model., 2014, 20, 2353, DOI:10.1007/s00894-014-2353-y.
- G.-I. Park, I.-T. Kim, J. K. Lee, S. K. Ryu and J. H. Kim, Effect of Temperature on the Adsorption and Desorption Characteristics of Methyl Iodide over TEDA-Impregnated Activated Carbon, Carbon Lett., 2001, 2, 9–14 Search PubMed.
- A. Peuronen, A. Valkonen, M. Kortelainen, K. Rissanen and M. Lahtinen, Halogen Bonding-Based “Catch and Release”: Reversible Solid-State Entrapment of Elemental Iodine with Monoalkylated DABCO Salts, Cryst. Growth Des., 2012, 12, 4157–4169, DOI:10.1021/cg300669t.
- C. Herdes, C. Prosenjak, S. Roman and E. A. Muller, Fundamental Studies of Methyl Iodide Adsorption in DABCO Impregnated Activated Carbons, Langmuir, 2013, 29, 6849–6855, DOI:10.1021/la401334d.
- J. Huve, A. Ryzhikov, H. Nouali, V. Lalia, G. Auge and T. J. Daou, Porous Sorbents for the Capture of Radioactive Iodine Compounds: A Review, RSC Adv., 2018, 8, 29248–29273, 10.1039/C8RA04775H.
- E. Aneheim, D. Bernin and M. R. St. John Foreman, Affinity of Charcoals for Different Forms of Radioactive Organic Iodine, Nucl. Eng. Des., 2018, 328, 228–240, DOI:10.1016/j.nucengdes.2018.01.007.
- J. Mancusco and R. J. McEachern, Applications of the PM3 Semi-Empirical Method to the Study of Triethyldiamine, J. Mol. Graphics Modell., 1997, 15, 82–90, DOI:10.1016/S1093-3263(97)00025-9.
-
A. Karhu, Methods to Prevent the Source Term of Methyl Iodide During Core Melt Accident; Technical Report NKS-13, Nordic Nuclear Safety Research, Roskilde, Denmark, 1999, https://inis.iaea.org/search/search.aspx?orig_q=source:%22ISBN%2087-7893-063-4%22 Search PubMed.
- S. Guentay, R. C. Cripps, B. Jackel and H. Bruchertseifer, Iodine Behavior During a Severe Accident in a Nuclear Power Plant, Chimia, 2005, 59, 957–965, DOI:10.2533/000942905777675453.
- C. M. Gonzalez-Garcia, J. F. Gonzalez and S. Roman, Removal Efficiency of Radioactive Methyl Iodide on TEDA-Impregnated Activated Carbons, Fuel Process. Technol., 2011, 92, 247–252, DOI:10.1016/j.fuproc.2010.04.014.
- B. J. Riley, J. D. Vienna, D. M. Strachan, J. S. McCloy and J. L. Jerden Jr., Materials and Processes for the Effective Capture and Immobilization of Radioiodine: A Review, J. Nucl. Mater., 2016, 470, 307–326, DOI:10.1016/j.jnucmat.2015.11.038.
- B. Li, X. Dong, H. Wang, D. Ma, K. Tan, S. Jensen, B. J. Deibert, J. Butler, J. Cure, Z. Shi, T. Thonhauser, Y. J. Chabal, Y. Han and J. Li, Capture of Organic Iodides from Nuclear Waste by Metal–Organic Framework-Based Molecular Traps, Nat. Commun., 2017, 8, 485, DOI:10.1038/s41467-017-00526-3.
- F. Taghipour and G. J. Evans, Iodine Behavior Under Conditions Relating to Nuclear Reactor Accidents, Nucl. Technol., 2002, 137, 181–193, DOI:10.13182/NT02-A3267.
- F. Taghipour and G. J. Evans, Modeling of Iodine Radiation Chemistry in the Presence of Organic Compounds, Radiat. Phys. Chem., 2002, 64, 203–213, DOI:10.1016/S0969-806X(01)00495-9.
- L. Bosland, F. Funke, N. Girault and G. Langrock, PARIS Project: Radiolytic Oxidation of Molecular Iodine in Containment during a Nuclear Reactor Severe Accident. Part 1. Formation and Destruction of Air Radiolysis Products – Experimental Results and Modelling, Nucl. Eng. Des., 2008, 238, 3542–3550, DOI:10.1016/j.nucengdes.2008.06.023.
- L. Bosland, F. Funke, G. Langrock and N. Girault, PARIS Project: Radiolytic Oxidation of Molecular Iodine in Containment During a Nuclear Reactor Severe Accident. Part 2. Formation and Destruction of Iodine Compounds Under Irradiation – Experimental Results Modelling, Nucl. Eng. Des., 2011, 241, 4026–4044, DOI:10.1016/j.nucengdes.2011.06.015.
-
J. Holm, T. Kärkelä, A. Auvinen, H. Glanneskog and C. Ekberg, Experimental Study on Iodine Chemistry (EXIS) – Containment Experiments with Methyl Iodide, Technical Report NKS-245, Nordic Nuclear Safety Research, Roskilde, Denmark, 2011 Search PubMed.
- B. Clement and R. Zeyen, The Objectives of the Phébus FP Experimental Programme and Main Findings, Ann. Nucl. Energy, 2013, 61, 4–10, DOI:10.1016/j.anucene.2013.03.037.
- S. Tietz, M. R. St. J. Foreman and C. H. Ekberg, Formation of Organic Iodides from Containment Paint Ingredients Caused by Gamma Irradiation, J. Nucl. Sci. Technol., 2013, 50, 689–694, DOI:10.1080/00223131.2013.799400.
- S. Dickinson, A. Auvinen, Y. Ammar, L. Bosland, B. Clément, F. Funke, G. Glowa, T. Kärkelä, D. A. Powers, S. Tietze, G. Weber and S. Zhang, Experimental and Modelling Studies of Iodine Oxide Formation and Aerosol Behaviour Relevant to Nuclear Reactor Accidents, Ann. Nucl. Energy, 2014, 74, 200–207, DOI:10.1016/j.anucene.2014.05.012.
- M. R. St John Foreman, An Introduction to Serious Nuclear Accident Chemistry, Cogent Chem., 2015, 1, 1049111, DOI:10.1080/23312009.2015.1049111.
- T. Haste, M. Di Giuli, G. Weber and S. Weber, Iodine Benchmarks in the SARNET Network of Excellence, J. Nucl. Eng. Radiat. Sci., 2016, 2, 021022, DOI:10.1115/1.4031652.
- A. Saiz-Lopez, J. M. C. Plane, A. R. Baker, L. J. Carpenter, R. von Glasow, J. C. Gomez Martin, G. McFiggans and R. W. Saunders, Atmospheric Chemistry of Iodine, Chem. Rev., 2012, 112, 1773–1804, DOI:10.1021/cr200029u.
- T. Jabbar, G. Wallner and P. A. Steier, A Review on 129I Analysis in Air, J. Environ. Radioact., 2013, 126, 45–54, DOI:10.1016/j.jenvrad.2013.07.013.
- A. Saiz-Lopez, R. P. Fernandez, C. Ordonez, D. E. Kinnison, J. C. Gomez Martin, J.-F. Lamarque and S. Tilmes, Iodine Chemistry in the Troposphere and its Effect on Ozone, Atmos. Chem. Phys., 2014, 14, 13119–13143, DOI:10.5194/acp-14-13119-2014.
- L. S. Lebel, R. S. Dickson and G. A. Glowa, Radioiodine in the Atmosphere after the Fukushima Dai-ichi Nuclear Accident, J. Environ. Radioact., 2016, 151, 82–93, DOI:10.1016/j.jenvrad.2015.06.001.
- X. Hou, V. Hansen, A. Aldahan, G. Possnert, O. C. Lind and G. A. Lujaniene, A Review on Speciation of Iodine-129 in the Environmental and Biological Samples, Anal. Chim. Acta, 2009, 632, 181–196, DOI:10.1016/j.aca.2008.11.013.
- A. Moreda-Pinerio, V. Romaris-Hortas and P. A. Bermejo-Barrera, A Review on Iodine Speciation for Environmental, Biological
and Nutrition Fields, J. Anal. At. Spectrom., 2011, 26, 2107–2152, 10.1039/C0JA00272K.
- W. H. Shetaya, S. D. Young, M. J. Watts, E. L. Ander and E. H. Bailey, Iodine Dynamics in Soils, Geochim. Cosmochim. Acta, 2012, 77, 457–473, DOI:10.1016/j.gca.2011.10.034.
- D. I. Kaplan, M. E. Denham, S. Zhang, C. Yeager, C. Xu, K. A. Schwehr, H. P. Li, Y. F. Ho, D. Wellman and P. H. Santschi, Radioiodine Biogeochemistry and Prevalence in Groundwater, Crit. Rev. Environ. Sci. Technol., 2014, 44, 2287–2335, DOI:10.1080/10643389.2013.828273.
- H. Fujiwara, Observation of Radioactive Iodine (131I, 129I) in Cropland Soil after the Fukushima Nuclear Accident, Sci. Total Environ., 2016, 566–567, 1432–1439, DOI:10.1016/j.scitotenv.2016.06.004.
- B. C. Sive, R. K. Varner, H. Mao, D. R. Blake, O. W. Wingenter and R. A. Talbot, A Large Terrestrial Source of Methyl Iodide, Geophys. Res. Lett., 2007, 34, L17808, DOI:10.1029/2007GL030528.
- N. Bell, L. Hsu, D. J. Jacob, M. G. Schultz, D. R. Blake, J. H. Butler, D. B. King, J. M. Lobert and E. Maier-Reimer, Methyl Iodide: Atmospheric Budget and Use as a Tracer of Marine Convection in Global Models, J. Geophys. Res., 2002, 107(D17), 4340, DOI:10.1029/2001JD001151.
-
National Research Council, Exposure of the American People to Iodine-131 from Nevada Nuclear Bomb Tests, The National Academies Press, Washington, D.C., 1999 DOI:10.17226/6283.
- S. Venturi, Evolutionary Significance of Iodine, Curr. Chem. Biol., 2011, 5, 155–162, DOI:10.2174/2212796811105030155.
- B. E. Mileson, L. M. Sweeney, M. L. Gargas and J. Kinzell, Iodomethane Human Health Risk Characterization, Inhalation Toxicol., 2009, 21, 583–605, DOI:10.1080/08958370802601627.
- M. Capurso, R. Gette, G. Radivoy and V. Dorn, The SN2 Reaction: A Theoretical-Computational Analysis of a Simple and Very Interesting Mechanism, Proceedings, 2019, 41, 81, DOI:10.3390/ecsoc-23-06514.
- S. Patel and K. K. Pant, Experimental Study and Mechanistic Kinetic Modeling for Selective Production of Hydrogen via Catalytic Steam Reforming of Methanol, Chem. Eng. Sci., 2007, 62, 5425–5433, DOI:10.1016/j.ces.2007.01.044.
-
C. P. Robert and G. Casella, Monte Carlo Statistical Methods, Springer-Verlag, New York, N.Y., 2nd edn, 2004 Search PubMed.
- H. Haario, M. Laine, A. Mira and E. Saksman, DRAM: Efficient Adaptive MCMC, Stat. Comput., 2006, 16, 339–354, DOI:10.1007/s11222-006-9438-0.
-
C. P. Robert, The Metropolis-Hastings Algorithm. Wiley StatsRef: Statistics Reference Online, John Wiley & Sons, Ltd., New York, N. Y., 2016 DOI:10.1002/9781118445112.stat07834.
- Y. Liu, J.-M. Haussaire, M. Bocquet, Y. Roustan, O. Saunier and A. Mathieu, Uncertainty Quantification of Pollutant Source Retrieval: Comparison of Bayesian Methods with Application to the Chernobyl and Fukushima Daiichi Accidental Releases of Radionuclides, Q. J. R. Meteorol. Soc., 2017, 143, 2886–2901, DOI:10.1002/qj.3138.
- J. D. Le Brazidec, M. Bocquet, O. Saunier and Y. Roustan, Quantification of Uncertainties in the Assessment of an Atmospheric Release Source Applied to the Autumn 2017 106Ru Event, Atmos. Chem. Phys., 2021, 21, 13247–13267, DOI:10.5194/acp-21-13247-2021.
- L. E. Herranz, S. Beck, V. H. Sanchez-Espinoza, F. Mascari, S. Brumm, O. Coindreau and S. Paci, The EC MUSA Project on Management and Uncertainty of Severe Accidents: Main Pillars and Status, Energies, 2021, 14, 4473, DOI:10.3390/en14154473.
- J. Kalilainen and T. Lind, Uncertainty Quantification Study on Gas Phase Iodine Release from Fukushima Daiichi Accident in Unit 3, Ann. Nucl. Energy, 2022, 166, 108740, DOI:10.1016/j.anucene.2021.108740.
- T. Takewaki, L. W. Beck and M. E. Davis, Zeolite Synthesis Using 1,4-Diazabicyclo[2,2,2]octane (DABCO) Derivatives as Structure-Directing Agents, Microporous Mesoporous Mater., 1999, 33, 197–207, DOI:10.1016/S1387-1811(99)00138-9.
- A. Y. Houghton and T. Autrey, Calorimetric Study of the Activation of Hydrogen by Tris(pentafluorophenyl)borane and Trimesitylphosphine, J. Phys. Chem. A, 2017, 121, 8785–8790, DOI:10.1021/acs.jpca.7b08582.
-
A. Iserles, A First Course in the Numerical Analysis of Differential Equations, Cambridge University Press, Cambridge, U. K., 2nd edn, 2008 DOI:10.1017/CBO9780511995569.
- E. Aprà, E. J. Bylaska, W. A. de Jong, N. Govind, K. Kowalski, T. P. Straatsma, M. Valiev, H. J. J. van Dam, Y. Alexeev, J. Anchell, V. Anisimov, F. W. Aquino, R. Atta-Fynn, J. Autschbach, N. P. Bauman, J. C. Becca, D. E. Bernholdt, K. Bhaskaran-Nair, S. Bogatko, P. Borowski, J. Boschen, J. Brabec, A. Bruner, E. Cauët, Y. Chen, G. N. Chuev, C. J. Cramer, J. Daily, M. J. O. Deegan, T. H. Dunning Jr., M. Dupuis, K. G. Dyall, G. I. Fann, S. A. Fischer, A. Fonari, H. Früchtl, L. Gagliardi, J. Garza, N. Gawande, S. Ghosh, K. Glaesemann, A. W. Götz, J. Hammond, V. Helms, E. D. Hermes, K. Hirao, S. Hirata, M. Jacquelin, L. Jensen, B. G. Johnson, H. Jónsson, R. A. Kendall, M. Klemm, R. Kobayashi, V. Konkov, S. Krishnamoorthy, M. Krishnan, Z. Lin, R. D. Lins, R. J. Littlefield, A. J. Logsdail, K. Lopata, W. Ma, A. V. Marenich, J. Martin del Campo, D. Mejia-Rodriguez, J. E. Moore, J. M. Mullin, T. Nakajima, D. R. Nascimento, J. A. Nichols, P. J. Nichols, J. Nieplocha, A. Otero-de-la-Roza, B. Palmer, A. Panyala, T. Pirojsirikul, B. Peng, R. Peverati, J. Pittner, L. Pollack, R. M. Richard, P. Sadayappan, G. C. Schatz, W. A. Shelton, D. W. Silverstein, D. M. A. Smith, T. A. Soares, D. Song, M. Swart, H. L. Taylor, G. S. Thomas, V. Tipparaju, D. G. Truhlar, K. Tsemekhman, T. Van Voorhis, Á. Vázquez-Mayagoitia, P. Verma, O. Villa, A. Vishnu, K. D. Vogiatzis, D. Wang, J. H. Weare, M. J. Williamson, T. L. Windus, K. Woliński, A. T. Wong, Q. Wu, C. Yang, Q. Yu, M. Zacharias, Z. Zhang, Y. Zhao and R. J. Harrison, NWChem: Past, Present, and Future, J. Chem. Phys., 2020, 152, 184102, DOI:10.1063/5.0004997.
- C. Adamo and V. Barone, Toward Reliable Density Functional Methods without Adjustable Parameters: The PBE0 Model, J. Chem. Phys., 1999, 110, 6158–6170, DOI:10.1063/1.478522.
- S. Grimme, J. Antony, S. Ehrlich and H. Krieg, A Consistent and Accurate ab initio Parametrization of Density Functional Dispersion Correction (DFT-D) for the 94 Elements H-Pu, J. Chem. Phys., 2010, 132, 154104, DOI:10.1063/1.3382344.
- K. A. Peterson, D. Figgen, E. Goll, H. Stoll and M. Dolg, Systematically Convergent Basis Sets with Relativistic Pseudopotentials. II. Small-Core Pseudopotentials and Correlation Consistent Basis Sets for the Post-d Group 16–18 Elements, J. Chem. Phys., 2003, 119, 11113–11123, DOI:10.1063/1.1622924.
- K. A. Peterson, B. C. Shepler, D. Figgen and H. Stoll, On the Spectroscopic and Thermochemical Properties of ClO, BrO, IO, and their Anions, J. Phys. Chem. A, 2006, 110, 13877–13883, DOI:10.1021/jp065887l.
- A. Klamt and G. Schüürmann, COSMO: A New Approach to Dielectric Screening in Solvents with Explicit Expressions for the Screening Energy and its Gradient, J. Chem. Soc., Perkin Trans. 2, 1993, 799–805, 10.1039/p29930000799.
- D. M. York and M. Karplus, A Smooth Solvation Potential Based on the Conductor-Like Screening Model, J. Phys. Chem. A, 1999, 103, 11060–11079, DOI:10.1021/jp992097l.
- Y. Ben-Tal, P. J. Boaler, H. J. A. Dale, R. E. Dooley, N. A. Fohn, Y. Gao, A. Garcia-Dominguez, K. M. Grant, A. M. R. Hall, H. L. D. Hayes, M. M. Kucharski, R. Wei and G. C. Lloyd-Jones, Mechanistic Analysis by NMR Spectroscopy: A Users Guide, Prog. Nucl. Magn. Reason. Spectrosc., 2022, 129, 28–106, DOI:10.1016/j.pnmrs.2022.01.001.
- H. Castejon and K. B. Wiberg, Solvent Effects on Methyl Transfer Reactions. 1. The Menshutkin Reaction, J. Am. Chem. Soc., 1999, 121, 2139–2146, DOI:10.1021/ja983736t.
- N. Menshutkin, Beiträgen zur Kenntnis der Affinitätskoeffizienten der Alkylhaloide und der Organischen Amine, Z. Phys. Chem., 1890, 5, 589–600, DOI:10.1515/zpch-1890-0546.
- C. Canepa, M. Mosso, A. Maranzana and G. Tonachini, Entropy of Activation for Reactions in the Condensed Phase: A Theoretical Study of the SN2 Alkylation of Amines, Eur. J. Org. Chem., 2005, 3342–3347, DOI:10.1002/ejoc.200500063.
- D. J. S. Sandbeck, C. M. Kuntz, C. Luu, R. A. Mondor, J. G. Ottaviano, A. V. Rayer, K. Z. Sumon and A. L. L. East, Challenges in Predicting ΔG‡ in Solution: The Mechanism of Ether-Catalyzed Hydroboration of Alkenes, J. Phys. Chem. A, 2014, 118, 11768–11779, DOI:10.1021/jp507793v.
- E. Avella-Moreno, N. Nuñez-Dallos, L. Garzón-Tovar and A. Duarte-Ruiz, Reactions and Products Revealed by NMR Spectra of Deuterated Dimethylsulfoxide with Iodomethane in Neutral and Basic Media, J. Sulfur Chem., 2015, 36, 535–543, DOI:10.1080/17415993.2015.1066376.
|
This journal is © the Owner Societies 2023 |