DOI:
10.1039/D3CB00114H
(Review Article)
RSC Chem. Biol., 2023,
4, 850-864
Protein conformational ensembles in function: roles and mechanisms
Received
30th June 2023
, Accepted 2nd September 2023
First published on 5th September 2023
Abstract
The sequence-structure-function paradigm has dominated twentieth century molecular biology. The paradigm tacitly stipulated that for each sequence there exists a single, well-organized protein structure. Yet, to sustain cell life, function requires (i) that there be more than a single structure, (ii) that there be switching between the structures, and (iii) that the structures be incompletely organized. These fundamental tenets called for an updated sequence-conformational ensemble-function paradigm. The powerful energy landscape idea, which is the foundation of modernized molecular biology, imported the conformational ensemble framework from physics and chemistry. This framework embraces the recognition that proteins are dynamic and are always interconverting between conformational states with varying energies. The more stable the conformation the more populated it is. The changes in the populations of the states are required for cell life. As an example, in vivo, under physiological conditions, wild type kinases commonly populate their more stable “closed”, inactive, conformations. However, there are minor populations of the “open”, ligand-free states. Upon their stabilization, e.g., by high affinity interactions or mutations, their ensembles shift to occupy the active states. Here we discuss the role of conformational propensities in function. We provide multiple examples of diverse systems, including protein kinases, lipid kinases, and Ras GTPases, discuss diverse conformational mechanisms, and provide a broad outlook on protein ensembles in the cell. We propose that the number of molecules in the active state (inactive for repressors), determine protein function, and that the dynamic, relative conformational propensities, rather than the rigid structures, are the hallmark of cell life.
Introduction
The energy landscape concept1–4 inspired experimental and computational approaches to characterize biomolecular ensembles that are now bearing functional insight.5–8 The landscape concept underscored the importance of proper physicochemical description of biological molecules—not as single structures but as ensembles with dynamic distributions that change with changes in the environment.9–24 While the classical sequence-single structure-function dogma has been enormously useful and influential in driving the relevance of structure in biology over multiple decades, it did not portray the non-linear propagation of information which is responsible for life.5 Different from the free energy landscape outlook, the single structure paradigm that prevailed for scores of years failed in embracing multiple states, thus in capturing biological actions, since its underlying premise has been unable to describe biological macromolecular dynamics. This is important since X-ray structures capture only snapshots, which are unable to explain exactly how function is executed. Adhering to a single shape leads to (i) attempting to explain molecular mechanisms by arguing that binding of incompatible shapes forces “pushing” molecules to change their shapes, rather than recognizing that binding involves selection of compatible shapes among the many available with subsequent minor conformational changes for optimization, which relieve incurring energetic conflicts.25,26 It also fails in (ii) identifying the preferred activation pathway in e.g., kinases, which requires not only a catalogue of the conformational states but their occupancies.27–33 And importantly, consequently it is unable to (iii) capture allosteric mechanisms.34–42 In enzymatic reactions,23,41,43–45 conformational dynamic amplifies the heterogeneity of the transition state.46 This suggests that the classical view of catalysis as a single tight optimized transition-state structure lowering the activation energy may also need to be updated to a transition state surface. In this population landscape view, rather than a single well-defined transition-state structure, enzymes can bind efficiently with a transition-state ensemble.
Physical and chemical principles underlie the fundamental functional processes harnessed by living systems in their diverse environments. In proteins and RNA, these include not only the conformational ensembles, but the kinetic barriers separating them, which function may need to overcome.47–50 Under normal physiological conditions, proteins largely populate their inactive conformations with the ensembles harboring only a minor population of the active state. Binding of effectors, or the membrane, can stabilize the active states, increasing the probabilities of barrier crossing. Oncogenic mutations similarly increase the relative stability of the active versus inactive conformations, mimicking this physicochemical principle.38,51–54 Thus, binding, whether noncovalent by effectors or covalent as in the case of mutations, posttranslational modifications, and protein family members with similar, albeit not identical sequences, modify the free energy landscape, driving the ensemble over the barriers, tipping the stability scales, and accomplishing a bistable switch.
Unquestionably, structural biology is essential for understanding biological processes. Equally so is the premise that conformational ensembles underlie all protein functions.23,55–58 The challenging aim facing the community is to elucidate the functions, especially how they are executed at the basic conformational level, and the mechanistic underpinnings of their signaling pathways. A conformational view is essential for in-depth comprehension of how proteins work and how they are regulated in the cell. Often single structures are harnessed to depict proteins and their interactions to explain observations, and the databases collect them. Undoubtedly, the mammoth task of assembling cellular, biochemical, protein–protein interaction, clinical, and high-resolution structural data results in an extremely useful overall picture; however, it still falls short. On their own these databases still cannot decipher the relationship between distinct isoforms and their preferred signaling pathways; nor are they able to explain mutational, or isoform, consequences, or the root causes of oncogenic signaling trends which correlate with specific cancers. They are critical; but insufficient. By harnessing dynamic populations, a conformational view of the data can bridge structural and cellular data and make these connections. Their foundation is the notion that biomolecules are not static sculptures, and their relative populations determine their functional states. Within this framework, our pioneering dynamic free energy landscape and redistribution of the population concepts, which were validated by numerous experiments, serve as guiding principles for deciphering function and dysfunction.59–62
The protein sequence-conformational ensemble-function paradigm was inspired by the realization that even living things must conform to the laws of physics, including the laws of motion and structural chemistry. At the same time, conformational principles are insufficient to explain protein actions in vivo. The cell is complex.63,64 Molecular concentrations are influenced by cell types and states, thus chromatin accessibility. Ensembles are also influenced by feedback loops. Through the actions of negative feedback loops, which often wield phosphatases, such as phosphatase and tensin homolog (PTEN) or dual specificity phosphatase 6 (DUSP6), tumor suppressors which can stymie active conformations and curb signaling.65–69 The ensembles are also impacted by the inherent liquefied droplets in the dynamic spatial structure of the cell, which extends from small complexes and assemblies to micrometer scales and coordinates cellular behavior.70–74
Below, we first discuss allostery,40,75–83 a hallmark of the functional role of conformational ensembles, followed by examples drawn from kinases and small GTPases. The references above provide more. These examples fit into the classical description of conformational ensembles, i.e., those generated by (almost) the same sequence, including single point mutations. We then expand our discussion to ensembles generated by isoforms and homologs belonging to the same family. In our outlook, isoforms and homologs with similar sequences occupy the same states, albeit with different propensities, thus favoring different ligands. Examples include cyclin-dependent kinase (CDK) family homologs CDK1, CDK2 and CDK4,84 and Ras. K-Ras4A favors Raf-1, K-Ras4B favors B-Raf.85 Along these lines, Rap1A, a GTPase highly homologous to K-Ras4B (Fig. 1), activates B-Raf, but not Raf-1 due to the lower affinity of the Raf-1 Ras binding domain (RBD) to the Rap1A catalytic domain, and the consequent failure of Raf-1 activation, which is not the case for B-Raf.86
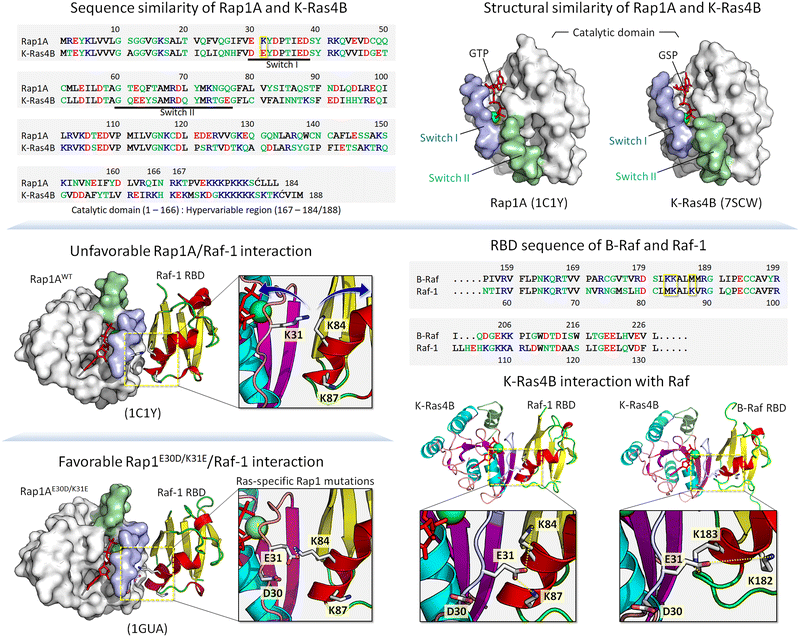 |
| Fig. 1 Sequence alignment and side-by-side comparison of the catalytic domain structures of Rap1A and K-Ras4B showing similarity (top panels). In the sequence, hydrophobic, polar/glycine, positively charged, and negatively charged residues are colored black, green, blue, and red, respectively. The yellow box at position 31 in the sequence indicates a key residue involved in the Raf RBD interaction. The crystal structure of the Rap1A catalytic domain interacting with the Raf-1 RBD shows an unfavorable interaction due to electrostatic repulsion between Lys31 of Rap1A and Lys84 of the Raf-1 RBD (middle left panel). Ras-specific mutation E30D/K31E of Rap1A restores interaction with Raf-1 RBD (bottom left panel). RBD sequences of B-Raf and Raf-1 and highlighting key residues in the yellow boxes that are involved in the GTPase interaction (middle right panel). Small GTPases interact with Raf through their catalytic domains, forming a strong backbone β-sheet interface with the Raf RBD. In addition to the backbone interaction, a strong salt bridge interaction contributes to the stability of the complex, as shown by the model structures of K-Ras4B interacting with the Raf-1 and B-Raf RBDs (bottom right panels). The example shows that Glu31 of K-Ras4B can form salt bridges with Lys84 and Lys87 of the Raf-1 RBD and Lys182 and Lys183 of the B-Raf RBD. | |
Allostery is a hallmark of function. If no protein conformations, no allostery, and no function
The free energy landscape is forceful since it can capture the mapping of all possible conformations that the molecule can populate. The lower the energy the higher the population. Mapping on a Cartesian coordinate system is both simple and powerful. With only small differences in energies among the states, the ensemble of (sub)states tend to lie within the native, lowest energy basin (Fig. 2A). A minor change in the stabilities of these near-energy states can easily flip an inactive state to an active one. The more stabilized is the active state versus the inactive state the more potent will be the bistable switch of the energy scales toward it and the stronger the resulting emitted signal. The rate of the switch is a function of the relative occupancies of the states on the energy mapping. The switch from the inactive to the active (and vice versa) is driven by allostery.59,87,88 Allostery can be triggered by noncovalent or covalent binding events,88–112 leading to conformational and dynamic changes,36,41,94,95,113–120 by inciting local energetic frustration, or conflicts.25,121–123 Conformational changes relieving the frustration propagate in the structure, shifting the ensemble from the inactive toward the stable, minimally frustrated active state (Fig. 2B). Thus, rather than the classical two active/inactive states captured by crystallography, there are multiple conformations in the ensemble with multiple possible propagation pathways. The favored route proceeds through the one involving lower kinetic barriers.89,124–128 Allosteric propagation pathways pre-exist in the ensemble, with preferred pathways required for functional population shift. In two (or multi) domains proteins joined by long, disordered linkers, the probability of propagation via the linker is relatively low. The disordered state harbors multiple conformations.129–135 It is characterized by its remarkable conformational flexibility and structural plasticity136 and its prevalence in the proteome,137–140 testifying to its usefulness. In the absence of specific stable interactions, there are likely no preferred propagation pathways. Raf's activation can serve as an example. Even though Ras binding initiates allosteric propagation in Raf's RBD, with a long, disordered linker, the signal is unlikely to be responsible for activation of Raf's kinase domain. Instead, it is more likely that the high affinity interactions shift the Raf ensemble from the inactive to the active state, liberating its autoinhibition.141
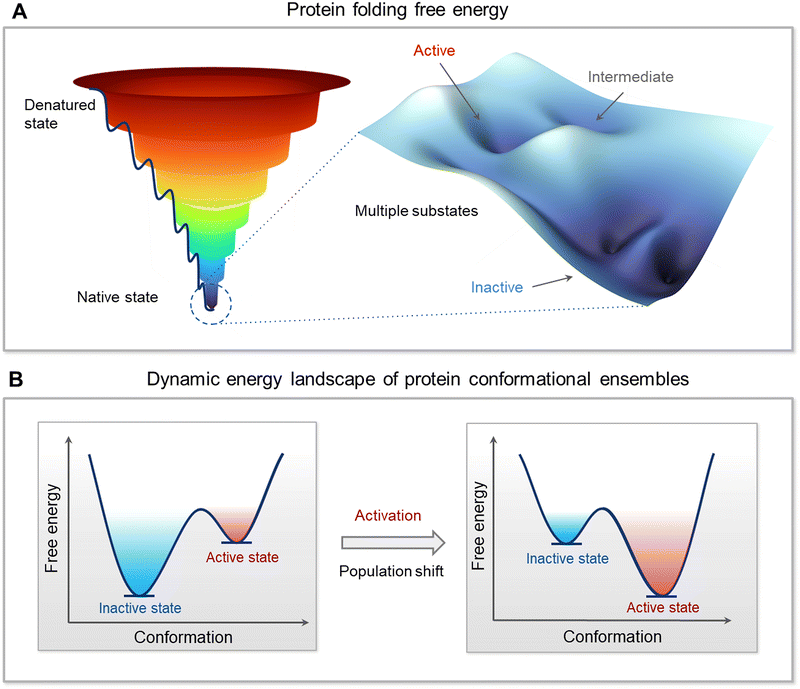 |
| Fig. 2 Multidimensional free energy landscape and conformational dynamics of protein. (A) Protein folding free energy landscape on a Cartesian coordinate system using a funnel-like shape with local valleys and peaks representing the vast ensembles of protein conformations. The depth or height of a point within the funnel corresponds to the free energy of a conformation, with deeper valleys indicating lower energy states and higher likelihood of occupancy. The native state is characterized by the lowest-energy valley at the bottom of the funnel, where the protein's functional conformations are concentrated. Within this native state, the landscape is populated with multiple substates, such as inactive states typically having the lowest energy, active states with slightly elevated energy, and intermediate states that can act as transition configurations. Subtle changes in the energy landscape at the bottom of the funnel, around the native state, can have profound effects on a protein's function and ability to interact with other molecules. The color gradient, ranging from deep blue at the bottom (indicative of lower energy) to red at the top (indicative of higher energy), visually encodes the probability of state occupancy and reflects the stability of conformations. (B) Dynamic energy landscape and how external factors such as genetic mutations, post-translational modifications like phosphorylation, and interactions with ligands or other proteins can alter the landscape. These alterations induce shifts in the population distribution of protein conformations, effectively transitioning proteins from a predominantly inactive state to an active state or vice versa. The figure emphasizes the dynamic nature of proteins and how their function is intrinsically tied to their conformational flexibility and adaptability within the cellular environment. | |
Allostery is how proteins are regulated. Autoinhibition and activation are allosteric events. Allosteric events can be impacted by concentration (since higher concentration implies higher chances for intermolecular contacts), can take place via coordination by ions, by interactions with signaling lipids, small molecule ligands, proteins, nucleic acids, and water molecules. Because allosteric propagation involves alterations of interactions at the atomic/residue level, a short-range allosteric signal is expected to be stronger. Through the occupancy of the relevant states, statistical mechanics relates the free-energy landscapes to the atomic level properties of molecules, and the macroscopic behavior of a population of molecules,2,9,21,142,143 where the occupancy of the states is a function of their relative energies. For enzymes, the catalytic reaction rate relates to the population of each state on a multidimensional energy landscape and the probability of reacting from that state.23 Below we provide a range of examples of the roles and mechanisms played by protein conformational ensembles in function.
The conformational ensemble of c-Abl autoinhibition and activation is controlled by a myristoyl lipid moiety, a posttranslational modification
Abl, the key kinase in leukemia, is an allosterically regulated non-receptor tyrosine kinase.144–153 There are two alternatively spliced Abl isoforms,147 1a and 1b (Fig. 3A). The N-terminus of the 19-residue longer 1b, but not of 1a, harbors a covalently connected myristoyl. Apart from this distinction, the isoforms have the same structural elements, the SH3, SH2, and kinase domains. In the autoinhibited conformation, the SH2 and SH3 domains latch onto the back of the kinase domain (Fig. 3B), with the N-terminal disordered region further contributing to Abl autoinhibition147,154via its proline-rich (CapPxxP) and C-terminal caps (CapC).146 Sites to which posttranslational modification moieties are attached are typically disordered. The myristoyl moiety acts as the switch between the autoinhibited and activated Abl. Binding of the myristoyl to the C-lobe pocket stabilizes Abl's autoinhibited conformation; its release triggers a conformational change which promotes the release of the SH2–SH3 domains. The released SH2 domain undergoes reorientation and translocation, inducing a transition toward a catalysis-favored conformation. The αC-helix shifts from the OUT to the IN conformation stabilizing the interacting P-loop/αC,146 the IN αC-helix, the R-spine, and the compact ATP-binding space.
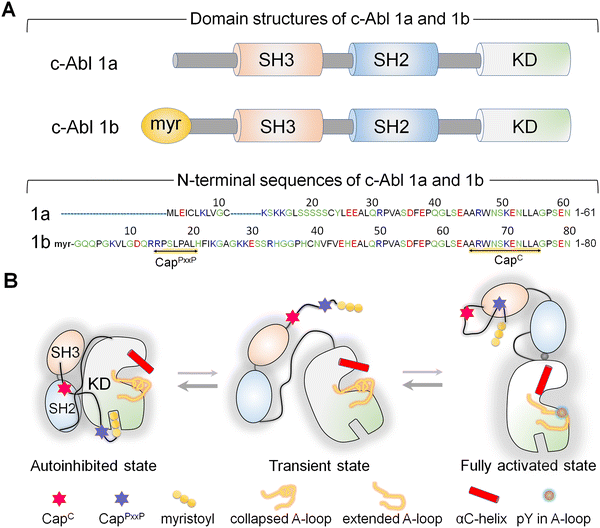 |
| Fig. 3 (A) The c-Abl protein exists in two isoforms: 1a and 1b. Both isoforms share three common domains: SH3, SH2, and kinase domains. A myristoyl group connects the N-terminus of the 1b isoform, which is not present in 1a. The N-terminal region of the 1b isoform is 19 residues longer compared to the 1a isoform. Both isoforms possess a CapC region, while only the 1b isoform contains a CapPxxP motif. (B) In the autoinhibited state of c-Abl 1b, the SH3 and SH2 domains dock onto the back of the kinase domain, and the myristoyl group inserts into a pocket within the C-lobe of the kinase domain. The CapC region interacts with the SH2 domain, lashing the SH2–SH3 module to the kinase domain. The myristoyl group acts as a switch for Abl's autoinhibition and activation. Upon release of the myristoyl group, the SH2–SH3 module dissociates from the kinase domain. The SH2 domain undergoes reorientation and translocation, relocating to interact with the top region of the N-lobe of the kinase domain, fully activating Abl. When autoinhibition is released, the canonical polyproline type II (PPII)-binding site of the SH3 domain of the c-Abl 1b isoform becomes exposed, potentially enabling optimal interaction with the CapPxxP motif. Abbreviation: KD, kinase domain. | |
Abl provides an example of how, through optimized positioning and atomistic contacts, a posttranslational modification toggles a conformational ensemble of a kinase driving its functional transition.
The conformations of the stronger K-Ras4B G12V oncogenic mutation visit the active state more frequently than those of the weaker G12D mutation
The conformational ensemble of a protein is heterogeneous, and the distributions of the same conformations by different mutants differ. That is, the mutations bias the ensemble differently, depending on how stabilizing (or destabilizing) the mutation is. Features of conformations that are stabilized by strong activating mutations resemble those of the active state. A strong mutation more forcefully biases the conformational ensemble toward the catalytic state, which is connected to the clinical phenotypes, by creating more favorable interactions. K-Ras is a good example.
Active K-Ras GTPase activates its effectors which then activate major signaling cascades in the cell, including MAPK and PI3K/AKT/PDK1/mTOR (Fig. 4). Both pathways are key actors in aggressively stimulating cell proliferation, survival, and invasion in cancer. The mutations point to variable tumorigenic outcome, including response to chemotherapy.155–159 K-Ras4B strong activating mutations act by blocking GTP hydrolysis. The strongest involve substitutions of G12, G13, and Q61. Weaker mutations disable nucleotide exchange, GDP by GTP.160,161 Among the G12 mutations, G12D is the most common, especially in pancreatic cancer, where it is present in approximately 35% of people diagnosed with the disease, and G12V is the most aggressive and chemotherapy resistant. NMR, crystallography, and computations were used to explore why G12V mutations are more aggressive than G12D.162
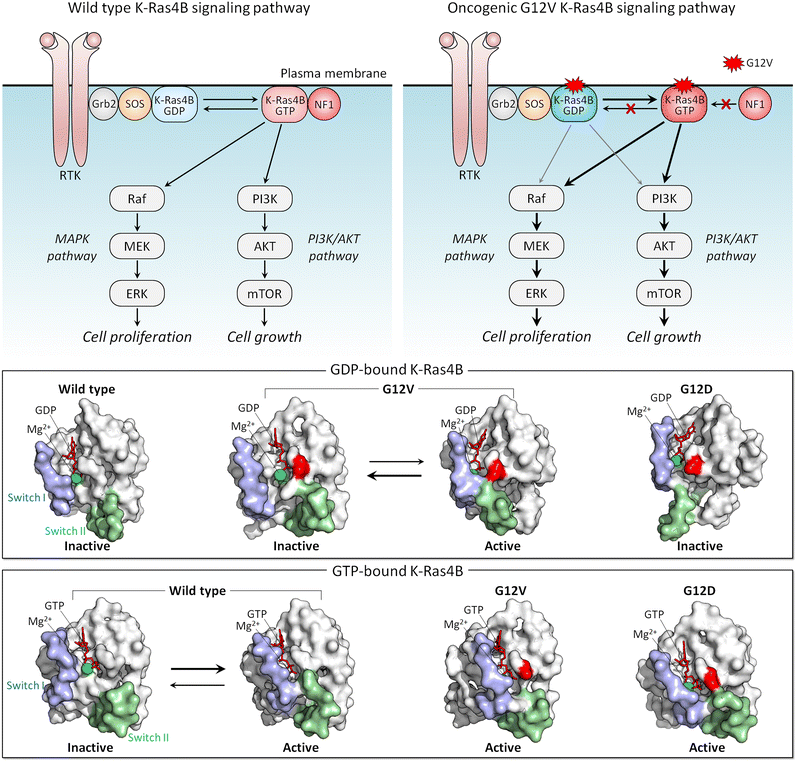 |
| Fig. 4 K-Ras4B signaling pathway. K-Ras4B is activated by SOS, a nucleotide exchange factor (GEF), via the GDP-to-GTP exchange and deactivated by NF1, a GTPase-activating protein (GAP) via the GTP-to-GDP hydrolysis (top left panel). Oncogenic driver mutations at the position 12, such as G12D, G12C, and G12V, impair the GAP-mediated hydrolysis and maintain Ras in a constitutively active GTP-bound state (top right panel). In the MAPK pathway, active GTP-bound K-Ras4B proteins recruit Raf to the plasma membrane and lead to Raf activation through dimerization of kinase domains. Active Raf dimers lead to the activation of a series of the MAPK kinases, resulting in cell proliferation. In the PI3K/AKT pathway, K-Ras4B recruits PI3K to the plasma membrane, leading to the production of the signaling lipid PIP3, which recruits AKT to the membrane, followed by activation by PDK1 and mTORC2. Active AKT is involved in the activation of mTORC1, leading to cell growth. Active-like, GDP-bound K-Ras4B with oncogenic G12V mutation can activate MAPK and PI3K/AKT pathways. The GDP-bound K-Ras4B (middle panel) and GTP-bound K-Ras4B (bottom panel) structures. In surface representation, two distinct conformations correspond to the inactive and active-like structures for K-Ras4BG12V-GDP, and the inactive-like and active structures for K-Ras4BWT-GTP. Both K-Ras4BG12V-GDP and K-Ras4BWT-GTP exhibit the open and closed Switch I and Switch II conformations. The open and closed switch regions represent K-Ras4B in the inactive and active states, respectively. Abbreviation: RTK, receptor tyrosine kinase; Grb2, growth factor receptor-bound protein 2; SOS, Son of sevenless; NF1, neurofibromin 1, MAPK, mitogen-activated protein kinase; MEK, mitogen-activated protein kinase kinase; ERK, extracellular signal-regulated kinase; PI3K, phosphoinositide 3-kinase; AKT, protein kinase B; mTOR, mammalian target of rapamycin. | |
The conformational behavior of K-Ras4B oncogenic mutants G12D and G12V differs34 (Fig. 4). While both favor an active-like state resembling GTP-bound K-Ras, the extent varies, due to the distinct atomic interactions formed by valine and the Switch II region in the GDP-bound K-Ras4B as compared to aspartic acid. These have not been captured in the crystal structures, where crystal contacts favor alternate stabilized Switch I region, but were observed by NMR and molecular dynamics simulations. We expect that this mechanism applies broadly, clarifying the differential strengths of mutations: stronger activating mutations favor more strongly the active state.163,164 To put it in terms of dynamic fluctuating ensembles, conformations favored by strong mutations visit the active state more frequently than weaker mutations do. In the case of K-Ras4B, which is not an enzyme, the conformation of the active state is the one that preferentially binds its effectors, such as Raf and PI3K.165,166 Visiting the active state more frequently is important for function since this results in the protein spending more time in the active, here oncogenic, state.
PI3K: conformational transitions for phosphorylation at the membrane
PI3Kα (PI3K below) lipid kinase phosphorylates signaling lipid PIP2 to PIP3 (Fig. 5A). The ensuing signaling cascade proceeds through the major PI3K/AKT/mTOR pathway.167 AKT and mTOR are protein kinases that phosphorylate innumerable substrates, thereby regulating cell growth, proliferation, differentiation, migration, mobility, and apoptosis.168,169 Their activating mutations drive cancer and neurodevelopmental disorders.170–172 PI3K's action is opposed by PTEN tumor suppressor which dephosphorylates PIP3 to PIP2. PI3K is a functional heterodimer consisting of the catalytic p110α and the regulatory p85α (Fig. 5B), which under physiological conditions is involved in inhibition of the p110α catalytic activity.173 The landmark crystal structure with the soaked PIP2 captured its binding site.174 Since it is away from ATP, and the nSH2 domain attached to the p110α subunit, it portrayed PI3K in its inactive state. In vivo, activation involves binding of the p85 nSH2 domain to phosphorylated C-terminal of activated receptor tyrosine kinases (RTKs), such as the insulin receptor, and active K-Ras. Calmodulin can also activate PI3K.175–177 The nSH2–RTK interaction disrupts the interactions of the nSH2 with the helical domain of the catalytic subunit, and of the iSH2 domain of p85 with the C2 domain of p110. These conformational changes result in relocation of the ABD of the catalytic subunit, and in interaction of the kinase domain with the membrane, which is promoted by Ras.53 Ras binding increases the PI3K residence time at the membrane, fostering PIP2 binding at the active site. Thus, RTKs allosterically activate PI3Kα; however, merging their action with Ras accomplishes full activation.141 At the same time, exactly how these actions, which are far away from the catalytic site, relate to PI3K activation has been unclear. It was also unclear how nSH2 interaction with the RTKs and the conformational changes that it stimulates lead to these actions and activation.
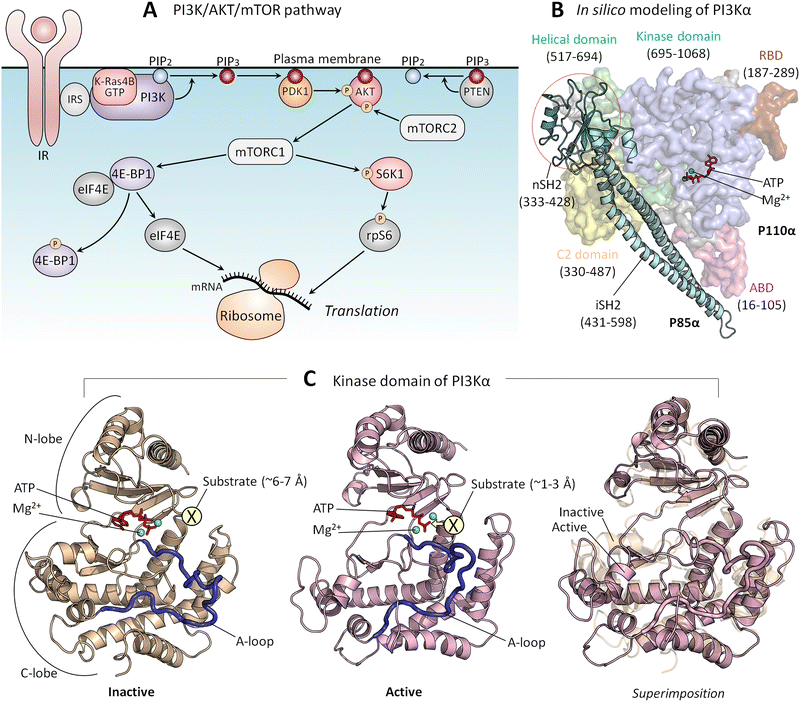 |
| Fig. 5 (A) In the PI3K/AKT/mTOR pathway, the lipid kinase, PI3K is activated by IR and IRS. PI3K phosphorylates PIP2 to PIP3, and PTEN dephosphorylates PIP3 back to PIP2. PDK1 and mTORC2 phosphorylate and activate PIP3-bound AKT, leading to mTORC1 activation. Active mTORC1 phosphorylates 4E-BP1 and S6K1. Phosphorylated 4E-BP1 releases eIF4B, which initiates mRNA translation at the ribosome. Phosphorylated S6K1 phosphorylates rpS6, also resulting in the initiation of mRNA translation. (B) An in silico model structure shows PI3Kα in an inactive state derived from the crystal structure (PDB: 4OVV). (C) The kinase domain structure of PI3Kα in the inactive (left panel) and active (middle panel) states and the superimposition of both (right panel). The active kinase domain structure was obtained from our previous studies.167 In the inactive state, the substrate, the inositol head of PIP2, is located ∼6–7 Å from the γ-phosphate of the ATP. In the active state, the substrate is located ∼1–3 Å from the γ-phosphate of the ATP. Abbreviation: IR, insulin receptor; IRS, insulin receptor substrate; PI3K, phosphoinositide 3-kinase; PIP2, phosphatidylinositol 4,5-bisphosphate; PIP3, phosphatidylinositol 3,4,5-trisphosphate; PDK1, phosphoinositide-dependent protein kinase 1; AKT, protein kinase B; mTORC1/2, mammalian target of rapamycin complex 1/2; 4E-BP1, eukaryotic translation initiation factor 4E (eIF4E)-binding protein 1: S6K1, ribosomal S6 kinase 1; rpS6, ribosomal protein S6. | |
Transfer of a phosphoryl group from a donor to a receptor requires a distance of 1–3 Å174 (Fig. 5C). The crystal structure of the inactive PI3K points to ∼6–7 Å between the γ-phosphate of the ATP and the PIP2. This conundrum has been resolved by data showing that the interaction of the phosphorylated, activated RTK allosterically triggers conformational transitions, culminating in reduced distance and in a reoriented, exposed kinase domain on the membrane.167 nSH2 release removes conformational constraints and leads to formation of new stabilizing interactions. The C-lobe of the kinase domain, where the PIP2 binds to a positively charged residue patch, moves away from the C2 domain, the C2/helical/C-lobe angle increases, and the distance between the C2 domain and the C-lobe of the kinase domain increases as well. Thus, through multiple conformational transitions emerging from allosteric energetic conflicts incurred by the binding of the nSH2 domain with the C-terminal of the insulin receptor, these conformational changes result in the kinase domain surface becoming fully accessible for interaction with the membrane.
Affinity and shift of the ensemble are a key mechanism for conformational transition in Raf's activation by Ras at the membrane
In the cell, the ensemble of Raf monomers can populate several states. These include (i) Ras-free open conformations, (ii) autoinhibited closed Raf conformations, which are inactive, and (iii) active Ras-bound conformations.141 In the absence of active Ras, the most highly populated conformation is the closed autoinhibited state (Fig. 6). It is the most stable.178 In this conformation, the surface of the kinase domain which serves for dimerization is occluded. There is only a minor population of the unstable open, Ras-free state. In the presence of active, GTP-bound Ras at the membrane, the RBD of Raf interacts with Ras with high affinity (measured in solution to be in the nanomolar range),179–181 as does Raf's cysteine-rich domain (CRD) with the membrane.182–184 CRD binding is stabilized by its positively charged membrane insertion loop.182,185 The Ras—RBD-CRD organization at the membrane tamps down the Ras—RBD fluctuations, increasing Raf's cooperative stabilization at the membrane. In the absence of active Ras molecules, Raf mostly populates a closed autoinhibited state, where the access to the kinase domain is hindered by other Raf domains/segments.
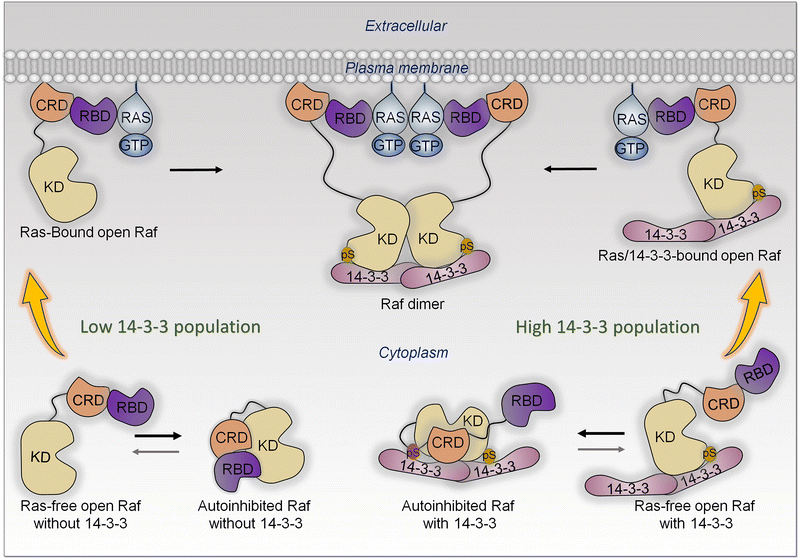 |
| Fig. 6 All Raf kinases share three conserved regions (CRs); CR1 involves the tandem Ras-binding domain (RBD) and cysteine-rich domain (CRD), CR2 contains the Ser/Thr rich region at the flexible linker, and CR3 is the kinase domain (KD). In the cytosol, Raf can exist in two autoinhibited states in the presence and absence of 14-3-3. When 14-3-3 concentration is low, Raf populates the closed, autoinhibited state without 14-3-3. In this state, the CRD and RBD dock onto the kinase domain. This closed state is most stable when active Ras is absent. Upon release of the RBD and CRD, Raf undergoes an open conformation, exposing the dimerization surface of the kinase domain. However, in the absence of active Ras, this open conformation is not sustained. The binding of Raf to membrane-bound active Ras stabilizes the open conformation and facilitates Raf's localization to the membrane, ultimately promoting dimerization through side-to-side interaction between two kinase domains. With a high 14-3-3 population, Raf interacts with the 14-3-3 dimer, resulting in autoinhibited Raf with 14-3-3. Phosphorylation of Ser residues in the linker (Ser365 for B-Raf and Ser259 for Raf-1) and C-terminal tail (Ser729 for B-Raf and Ser621 for Raf-1) enhances the interaction between Raf and 14-3-3 proteins. In this state, phosphorylated Ser residues serve as anchor points for the association of the kinase domain with the 14-3-3 dimer. This interaction protects the dimerization surface. For Ras-free open Raf with 14-3-3, the released RBD can engage with GTP-bound Ras, promoting Raf dimerization. Phosphorylation of the Ser residue in the linker is required only for autoinhibition, whereas phosphorylation of the Ser residue in the C-terminal tail has a dual function in both kinase domain dimerization and autoinhibition. | |
The high affinity Ras—RBDs and CRD—membrane interactions stabilize the open, Ras-bound state, leading to a population shift toward this conformation largely from the less stable, open Ras-free ensemble, which is likely separated from the Ras-bound state by low kinetic barriers. The shift impacts the relative populations, thus equilibrium between the Ras-free open state and the closed state, which is restored mostly by a shift of the autoinhibited state (Fig. 6). The resulting high population of the open, stabilized Ras-bound state, with the exposed surface of the kinase domain, enables its dimerization. Depending on the location in different Raf isoforms, with 14-3-3 involvement, phosphorylation may weaken or promote the autoinhibition.186–194
Thus, data and theory suggest that allostery, via a Ras-promoted conformational change, does not play a role in neither PI3Kα nor Raf activation.195 Significantly, in agreement with this, no activating mutations that can replace Ras are located in the RBDs, of neither Raf nor PI3K.
In the literature it is often noted that active Ras anchored at the plasma membrane “recruits” Raf. Such “recruitment” terminology is used quite frequently. We clarify that recruitment invariably implies a high affinity interaction that stabilize the conformation, thus a shift of the population toward the recruited state.
Splicing, and different gene isoforms, may share ensembles, although with different propensities
The Ras superfamily is diverse, with multiple members. Among them, three major genes encode Ras, HRAS, NRAS, and KRAS.196 Their sequences are highly similar, but not identical. Their mutational frequencies differ, with KRAS most frequently mutated, and HRAS and NRAS expression appearing insufficient to promote oncogenesis when mutated.197 Their protein products, K-Ras, H-Ras and N-Ras, differ primarily in their C-terminals, and in the attached combination of lipid posttranslational modifications. There are also minor differences in their catalytic domains. Their structures are highly similar as well. Their differences lead to their differential membrane anchorage preferences, and altered interactions with varied stabilities with the same effectors.198–201 Analysis of their dimer preferences demonstrates distinct behavior as well.202 These point to differential distributions (propensities) of their conformational ensembles, which correlate with their altered preferred functions in different cell types. The Ras ensembles portray all states,22,198,203 including the GTP and GDP bound conformations, effector bound states, membrane anchored active and inactive conformations, farnesylated, palmitoylated, phosphorylated, and ubiquitylated states, conformations harboring mutations, and transition states. Some conformations carry functions, others may not. All are allosterically modulated. Although all Ras isoforms have overlapping functions and upon inhibition of one isoform can carry its tasks, they are optimized for specific cell types, suggesting distinct binding preferences by their respective conformations, with different isoforms exhibiting different signaling and biological functions. They may recruit different partners implicating different affinities or availabilities in the specific cell types that they populate or localize in. The interactions may also be GDP/GTP protein state dependent, although as we discussed above, GDP-bound conformations of strong driver mutations may trend toward the GTP-bound state. Within this framework, K-Ras splice isoforms K-Ras4A and K-Ras4B are of particular interest as they populate two states with lipid posttranslational modifications in their C-terminal hypervariable tails that mimic K-Ras4B, with only a farnesyl, and N-Ras, with a palmitoyl and farnesyl.198,203 The C-terminal of K-Ras4A is positively charged, in between the more highly positively charged K-Ras4B and the lesser charged N-Ras. This suggest that in its K-Ras4B-like conformation, K-Ras4A can be linked with K-Ras4B cancers, such as pancreatic ductal adenocarcinoma, colorectal, and lung cancers. In its N-Ras-like state it may be associated with melanoma, an N-Ras cancer. Recently, stable isotope labeling with amino acids in cell culture and affinity-purification mass spectrometry observed differences between K-Ras4A and K-Ras4B, which may explain some of the functional differences,85 with v-ATPase a2 and eIF2Bδ interacting with only K-Ras4B. Especially of interest, K-Ras4AG12D binds Raf-1 stronger than K-Ras4B, reminiscent of the Rap1 inclination discussed above. We expect differential conformational trends of K-Ras4AG12D and K-Ras4BG12D.
In another example, CDKs in the cell cycle are also similar and expected to share ensembles albeit with different propensities and distributions204–206 (Fig. 7A). Especially, even though there are multiple CDKs, a single CDK is responsible for both G1/S and G2/M transitions.207 Cyclin-E—CDK2 is the primary complex responsible for leading the cells through the G1/S transition stage (Fig. 7B), although recently, this “fixed point” estimate, which assumes that further cell cycle commitment ceases as soon as the mitogen signaling stimulus is removed, has been challenged.208 However, when unavailable, CDK1 can undertake this role. Similarly, if its level is too low, CDK1 can be substituted by cyclin-B—CDK2 complexes in the G2/M checkpoint transition allowing cell cycle progression. Finally, sufficiently high hurdles in physiological time scales can constitute translational barriers with kinetically trapped states in synonymous single-nucleotide polymorphism. The translational pausing observed in the MDR1 (multidrug resistance 1) gene can lead to alternative folded structures which are functionally distinct.209–211 Eventually, the time scale of the altered conformation depends on the barrier height and relative stability. Recent examples concern type III chloramphenicol acetyltransferase, D-alanine-D-alanine ligase B and dihydrofolate reductase.212
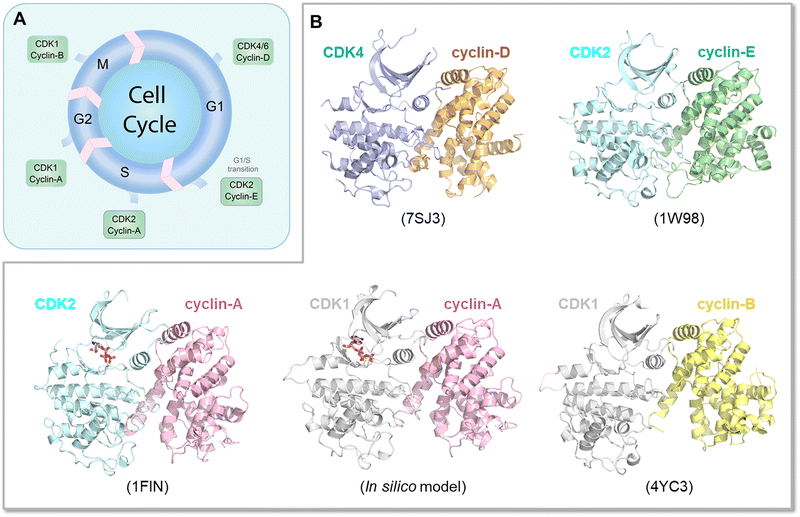 |
| Fig. 7 Conformational diversity and regulation of CDKs in the cell cycle. (A) A schematic representation of the cell cycle, highlighting the involvement of various cyclin—CDK complexes at different phases (G1, S, G2, M). (B) Active conformations of the cyclin—CDK complexes participating in each stage of the cell cycle, with a focus on the structural similarities and subtle differences among them. These structural differences are crucial as they dictate the specific roles and regulatory functions of the complexes. Annotations include PDB codes for the crystal structures of the CDK complexes shown. In the absence of a crystal structure for the cyclin-A—CDK1 complex, an in silico model was constructed based on the active conformation of CDK1 and cyclin-A derived from the CDK2 complex. The figure also suggests the adaptability of CDKs; for example, when cyclin-E—CDK2 is unavailable for the G1/S transition, CDK1 can take over this role. Similarly, cyclin-B—CDK2 can substitute for CDK1 during the G2/M transition under certain conditions. The figure underscores the importance of structural variations and dynamic interactions in the fine-tuning of cell cycle regulation through cyclin—CDK complexes. | |
Conclusions
Dynamic, interconverting, protein conformational ensembles sustain life. Rigid protein crystal structure snapshots do not. Despite the overwhelming support for the need for conformational ensembles to understand function, still not all in the community accede to its veracity. The view that the change of a protein structure is induced by steric clashes, and electrostatic repulsion, which physically dislodge an impeding protein component is incompatible with a physics-based outlook. The concept underscored here is of a relationship between molecular structure and potential energy, where structures of conformers are related to their energy, and therefore to their relative stabilities.213 This view explains that protein ensembles consist of all possible conformations with their populations depending on their relative energies. The partner protein selects the conformer whose shape and chemistry fit it best. Binding can still result in certain energetic frustration of local interactions.121 Minimal local frustration indicates stable folded molecule; higher local frustration can point to functionally relevant regions where binding can promote allosteric signal that relieves it. Relieving the energetic frustration optimizes the interaction. Evolution exploited the ensemble by using the conformational ensemble for function. In our examples above, evolution has toyed with myristoyl to flip Abl conformations; in the case of K-Ras oncogenic mutations, the most aggressive K-RasG12V shifts the ensemble to the active state even when GDP-bound; in PI3K, an activated receptor binding shifts the ensemble to the active state, relieving the autoinhibition. And splicing isoforms preferentially bind partners to optimize function as in the case of K-Ras4A which favors Raf-1 whereas K-Ras4B prefers B-Raf, interestingly mimicking Rap1A, which favors B-Raf, over Raf-1. The C-terminal of Rap1A is strongly positively charged, resembling K-Ras4B. Rap1B's is neutral, resembling H-Ras and N-Ras.86
Here, we underscore conformational propensities. These determine protein function and cell activity. The relative number of active conformations, or molecules, decides the protein functional state: active or inactive. Together with expression level, they also determine signaling strength. Signaling strength decides cell activity. Exactly how to experimentally measure signal strength to establish cell activity is still enigmatic. Yet, this is vital as conformational propensities and the ensuing signaling strength can establish cell proliferation and forecast the emergence of drug resistance.
Author contributions
R. Nussinov, H. Jang: concept and original version. R. Nussinov: writing – original draft and supervision. Y. Liu, W. Zhang, H. Jang: visualization. R. Nussinov, Y. Liu, W. Zhang, H. Jang; writing – review and editing.
Conflicts of interest
There are no conflicts to declare.
Acknowledgements
This project has been funded in whole or in part with federal funds from the National Cancer Institute, National Institutes of Health, under contract HHSN261201500003I. The content of this publication does not necessarily reflect the views or policies of the Department of Health and Human Services, nor does mention of trade names, commercial products, or organizations imply endorsement by the U.S. Government. This Research was supported [in part] by the Intramural Research Program of the NIH, National Cancer Institute, Center for Cancer Research.
Notes and references
- J. N. Onuchic, Z. Luthey-Schulten and P. G. Wolynes, Annu. Rev. Phys. Chem., 1997, 48, 545–600 CrossRef CAS PubMed.
- H. Frauenfelder, S. G. Sligar and P. G. Wolynes, Science, 1991, 254, 1598–1603 CrossRef CAS PubMed.
- F. Mallamace, C. Corsaro, D. Mallamace, S. Vasi, C. Vasi, P. Baglioni, S. V. Buldyrev, S. H. Chen and H. E. Stanley, Proc. Natl. Acad. Sci. U. S. A., 2016, 113, 3159–3163 CrossRef CAS PubMed.
- K. Roder and D. J. Wales, Front. Mol. Biosci., 2022, 9, 820792 CrossRef PubMed.
- R. Nussinov and P. G. Wolynes, Phys. Chem. Chem. Phys., 2014, 16, 6321–6322 RSC.
- K. Roder, J. A. Joseph, B. E. Husic and D. J. Wales, Adv. Theor. Simul., 2019, 2, 1800175 CrossRef.
- S. Neelamraju, D. J. Wales and S. Gosavi, Curr. Opin. Struct. Biol., 2020, 64, 145–151 CrossRef CAS PubMed.
- S. H. Chen and R. Elber, Phys. Chem. Chem. Phys., 2014, 16, 6407–6421 RSC.
- G. Wei, W. Xi, R. Nussinov and B. Ma, Chem. Rev., 2016, 116, 6516–6551 CrossRef CAS PubMed.
- P. Biswas, J. Zou and J. G. Saven, J. Chem. Phys., 2005, 123, 154908 CrossRef PubMed.
- H. Nymeyer, N. D. Socci and J. N. Onuchic, Proc. Natl. Acad. Sci. U. S. A., 2000, 97, 634–639 CrossRef CAS PubMed.
- R. Nussinov, Chem. Rev., 2016, 116, 6263–6266 CrossRef PubMed.
- A. M. Ruvinsky, T. Kirys, A. V. Tuzikov and I. A. Vakser, Protein Sci., 2013, 22, 734–744 CrossRef CAS PubMed.
- M. N. Sanches, K. Knapp, A. B. Oliveira, Jr., P. G. Wolynes, J. N. Onuchic and V. B. P. Leite, J. Phys. Chem. B, 2022, 126, 93–99 CrossRef CAS PubMed.
- A. J. Wand, Struct. Dyn., 2023, 10, 020901 CrossRef CAS PubMed.
- J. Juarez-Jimenez, A. A. Gupta, G. Karunanithy, A. Mey, C. Georgiou, H. Ioannidis, A. De Simone, P. N. Barlow, A. N. Hulme, M. D. Walkinshaw, A. J. Baldwin and J. Michel, Chem. Sci., 2020, 11, 2670–2680 RSC.
- J. Ding, Y. T. Lee, Y. Bhandari, C. D. Schwieters, L. Fan, P. Yu, S. G. Tarosov, J. R. Stagno, B. Ma, R. Nussinov, A. Rein, J. Zhang and Y. X. Wang, Nat. Commun., 2023, 14, 714 CrossRef CAS PubMed.
- R. Nussinov, M. Zhang, Y. Liu and H. Jang, J. Phys. Chem. B, 2022, 126, 6372–6383 CrossRef CAS PubMed.
- B. Ma, G. Bai, R. Nussinov, J. Ding and Y. X. Wang, J. Phys. Chem. B, 2021, 125, 2589–2596 CrossRef CAS PubMed.
- H. Jang, A. Banerjee, K. Marcus, L. Makowski, C. Mattos, V. Gaponenko and R. Nussinov, Structure, 2019, 27, 1647–1659e1644 CrossRef CAS PubMed.
- R. Nussinov, C. J. Tsai and H. Jang, PLoS Comput. Biol., 2019, 15, e1006648 CrossRef CAS PubMed.
- S. Lu, H. Jang, S. Muratcioglu, A. Gursoy, O. Keskin, R. Nussinov and J. Zhang, Chem. Rev., 2016, 116, 6607–6665 CrossRef CAS PubMed.
- F. Yabukarski, T. Doukov, M. M. Pinney, J. T. Biel, J. S. Fraser and D. Herschlag, Sci. Adv., 2022, 8, eabn7738 CrossRef CAS PubMed.
- F. Yabukarski, J. T. Biel, M. M. Pinney, T. Doukov, A. S. Powers, J. S. Fraser and D. Herschlag, Proc. Natl. Acad. Sci. U. S. A., 2020, 117, 33204–33215 CrossRef CAS PubMed.
- M. I. Freiberger, P. G. Wolynes, D. U. Ferreiro and M. Fuxreiter, J. Phys. Chem. B, 2021, 125, 2513–2520 CrossRef CAS PubMed.
- E. Karlsson, E. Andersson, J. Dogan, S. Gianni, P. Jemth and C. Camilloni, J. Biol. Chem., 2019, 294, 1230–1239 CrossRef CAS PubMed.
- N. Teruel, O. Mailhot and R. J. Najmanovich, PLoS Comput. Biol., 2021, 17, e1009286 CrossRef CAS PubMed.
- A. Nicolai, P. Delarue and P. Senet, PLoS Comput. Biol., 2013, 9, e1003379 CrossRef PubMed.
- C. Draper-Joyce and S. G. B. Furness, ACS Pharmacol. Transl. Sci., 2019, 2, 285–290 CrossRef CAS PubMed.
- A. Pandini and A. Fornili, J. Chem. Theory Comput., 2016, 12, 1368–1379 CrossRef CAS PubMed.
- Y. Li and H. Gong, J. Chem. Theory Comput., 2022, 18, 4529–4543 CrossRef CAS PubMed.
- C. Kohler, G. Carlstrom, A. Gunnarsson, U. Weininger, S. Tangefjord, V. Ullah, M. Lepisto, U. Karlsson, T. Papavoine, K. Edman and M. Akke, Sci. Adv., 2020, 6, eabb5277 CrossRef CAS PubMed.
- Y. T. Lee, E. C. Glazer, R. F. Wilson, C. D. Stout and D. B. Goodin, Biochemistry, 2011, 50, 693–703 CrossRef CAS PubMed.
- P. Grudzien, H. Jang, N. Leschinsky, R. Nussinov and V. Gaponenko, J. Mol. Biol., 2022, 434, 167695 CrossRef CAS PubMed.
- R. C. Maloney, M. Zhang, Y. Liu, H. Jang and R. Nussinov, Cell. Mol. Life Sci., 2022, 79, 281 CrossRef CAS PubMed.
- L. Zhang, M. Li and Z. Liu, PLoS Comput. Biol., 2018, 14, e1006393 CrossRef PubMed.
- H. Abdelkarim, N. Leschinsky, H. Jang, A. Banerjee, R. Nussinov and V. Gaponenko, Curr. Opin. Struct. Biol., 2021, 71, 164–170 CrossRef CAS PubMed.
- M. Zhang, H. Jang and R. Nussinov, Cancer Res., 2021, 81, 237–247 CrossRef CAS PubMed.
- Q. Huang, P. Song, Y. Chen, Z. Liu and L. Lai, J. Phys. Chem. Lett., 2021, 12, 5404–5412 CrossRef CAS PubMed.
- J. Wang, A. Jain, L. R. McDonald, C. Gambogi, A. L. Lee and N. V. Dokholyan, Nat. Commun., 2020, 11, 3862 CrossRef CAS PubMed.
- R. Nussinov and C. J. Tsai, Cell, 2013, 153, 293–305 CrossRef CAS PubMed.
- T. Haliloglu, A. Hacisuleyman and B. Erman, Bioinformatics, 2022, 38, 3590–3599 CrossRef CAS PubMed.
- C. Stark, T. Bautista-Leung, J. Siegfried and D. Herschlag, eLife, 2022, 11, e72884 CrossRef CAS PubMed.
- M. M. Pinney, D. A. Mokhtari, E. Akiva, F. Yabukarski, D. M. Sanchez, R. Liang, T. Doukov, T. J. Martinez, P. C. Babbitt and D. Herschlag, Science, 2021, 371, eaay2784 CrossRef CAS PubMed.
- M. Zhang, R. Maloney, Y. Liu, H. Jang and R. Nussinov, Cancer Commun., 2023, 43, 405–408 CrossRef PubMed.
- B. Ma, S. Kumar, C. J. Tsai, Z. Hu and R. Nussinov, J. Theor. Biol., 2000, 203, 383–397 CrossRef CAS PubMed.
- V. Galstyan and R. Phillips, J. Phys. Chem. B, 2019, 123, 10990–11002 CrossRef CAS PubMed.
- T. R. Sosnick, Protein Sci., 2008, 17, 1308–1318 CrossRef CAS PubMed.
- S. J. Wodak, E. Paci, N. V. Dokholyan, I. N. Berezovsky, A. Horovitz, J. Li, V. J. Hilser, I. Bahar, J. Karanicolas, G. Stock, P. Hamm, R. H. Stote, J. Eberhardt, Y. Chebaro, A. Dejaegere, M. Cecchini, J. P. Changeux, P. G. Bolhuis, J. Vreede, P. Faccioli, S. Orioli, R. Ravasio, L. Yan, C. Brito, M. Wyart, P. Gkeka, I. Rivalta, G. Palermo, J. A. McCammon, J. Panecka-Hofman, R. C. Wade, A. Di Pizio, M. Y. Niv, R. Nussinov, C. J. Tsai, H. Jang, D. Padhorny, D. Kozakov and T. McLeish, Structure, 2019, 27, 566–578 CrossRef CAS PubMed.
- V. Munoz and M. Cerminara, Biochem. J., 2016, 473, 2545–2559 CrossRef CAS PubMed.
- S. Kang, A. G. Bader and P. K. Vogt, Proc. Natl. Acad. Sci. U. S. A., 2005, 102, 802–807 CrossRef CAS PubMed.
- M. Sun, P. Hillmann, B. T. Hofmann, J. R. Hart and P. K. Vogt, Proc. Natl. Acad. Sci. U. S. A., 2010, 107, 15547–15552 CrossRef CAS PubMed.
- J. E. Burke, O. Perisic, G. R. Masson, O. Vadas and R. L. Williams, Proc. Natl. Acad. Sci. U. S. A., 2012, 109, 15259–15264 CrossRef CAS PubMed.
- R. Nussinov, M. Zhang, R. Maloney and H. Jang, Expert Opin. Drug Discovery, 2021, 16, 823–828 CrossRef CAS PubMed.
-
S. Du, S. A. Wankowicz, F. Yabukarski, T. Doukov, D. Herschlag and J. S. Fraser, bioRxiv, 2023 DOI:10.1101/2023.05.05.539620.
- C. Di Pietrantonio, A. Pandey, J. Gould, A. Hasabnis and R. S. Prosser, Methods Enzymol., 2019, 615, 103–130 CAS.
- A. N. Naganathan, R. Dani, S. Gopi, A. Aranganathan and A. Narayan, J. Mol. Biol., 2021, 433, 167325 CrossRef CAS PubMed.
- K. Lindorff-Larsen and J. Ferkinghoff-Borg, PLoS One, 2009, 4, e4203 CrossRef PubMed.
- S. Kumar, B. Ma, C. J. Tsai, N. Sinha and R. Nussinov, Protein Sci., 2000, 9, 10–19 CrossRef CAS PubMed.
- C. J. Tsai, S. Kumar, B. Ma and R. Nussinov, Protein Sci., 1999, 8, 1181–1190 CrossRef CAS PubMed.
- C. J. Tsai, B. Ma and R. Nussinov, Proc. Natl. Acad. Sci. U. S. A., 1999, 96, 9970–9972 CrossRef CAS PubMed.
- B. Ma, S. Kumar, C. J. Tsai and R. Nussinov, Protein Eng., 1999, 12, 713–720 CrossRef CAS PubMed.
- O. Farc and V. Cristea, Exp. Ther. Med., 2021, 21, 96 CrossRef CAS PubMed.
- A. Ma’ayan, J. R. Soc., Interface, 2017, 14, 20170391 CrossRef PubMed.
- K. Ingram, S. C. Samson, R. Zewdu, R. G. Zitnay, E. L. Snyder and M. C. Mendoza, Oncogene, 2022, 41, 293–300 CrossRef CAS PubMed.
- R. Mukherjee, K. G. Vanaja, J. A. Boyer, S. Gadal, H. Solomon, S. Chandarlapaty, A. Levchenko and N. Rosen, Mol. Cell, 2021, 81, 708–723e705 CrossRef CAS PubMed.
- J. Stebbing, L. C. Lit, H. Zhang, R. S. Darrington, O. Melaiu, B. Rudraraju and G. Giamas, Oncogene, 2014, 33, 939–953 CrossRef CAS PubMed.
- W. S. Chen, Y. Liang, M. Zong, J. J. Liu, K. Kaneko, K. L. Hanley, K. Zhang and G. S. Feng, Cell Rep., 2021, 37, 109974 CrossRef CAS PubMed.
- L. Guo, K. Zhu, M. Pargett, A. Contreras, P. Tsai, Q. Qing, W. Losert, J. Albeck and M. Zhao, iScience, 2021, 24, 103240 CrossRef CAS PubMed.
- R. Nussinov, Phys. Biol., 2013, 10, 045004 CrossRef CAS PubMed.
- L. Wakefield, S. Agarwal and K. Tanner, Cell, 2023, 186, 1792–1813 CrossRef CAS PubMed.
- D. S. W. Lee, N. S. Wingreen and C. P. Brangwynne, Nat. Phys., 2021, 17, 531–538 Search PubMed.
- A. L. S. Cruz, N. Carrossini, L. K. Teixeira, L. F. Ribeiro-Pinto, P. T. Bozza and J. P. B. Viola, Mol. Cell. Biol., 2019, 39, e00374–18 CrossRef CAS PubMed.
- C. A. Weber and C. Zechner, Phys. Today, 2021, 74, 38–43 CrossRef CAS.
- J. Liu and R. Nussinov, PLoS Comput. Biol., 2016, 12, e1004966 CrossRef PubMed.
- M. Leander, Y. Yuan, A. Meger, Q. Cui and S. Raman, Proc. Natl. Acad. Sci. U. S. A., 2020, 117, 25445–25454 CrossRef CAS PubMed.
- P. Campitelli, T. Modi, S. Kumar and S. B. Ozkan, Annu. Rev. Biophys., 2020, 49, 267–288 CrossRef CAS PubMed.
- G. M. Verkhivker and L. Di Paola, J. Phys. Chem. B, 2021, 125, 850–873 CrossRef CAS PubMed.
- G. M. Verkhivker, Curr. Opin. Struct. Biol., 2021, 71, 71–78 CrossRef CAS PubMed.
- Y. Yuan, J. Deng and Q. Cui, J. Am. Chem. Soc., 2022, 144, 10870–10887 CrossRef CAS PubMed.
- M. Leander, Z. Liu, Q. Cui and S. Raman, eLife, 2022, 11, e79932 CrossRef PubMed.
- A. Dayananda, T. S. H. Dennison, H. Y. Y. Fonseka, M. S. Avestan, Q. Wang, R. Tehver and G. Stan, J. Chem. Phys., 2023, 158, 125101 CrossRef CAS PubMed.
- S. Dey and H. X. Zhou, J. Chem. Phys., 2023, 158, 091105 CrossRef CAS PubMed.
- R. Nussinov, H. Jang, G. Nir, C. J. Tsai and F. Cheng, Signal Transduction Targeted Ther., 2021, 6, 3 CrossRef PubMed.
- X. Zhang, J. Cao, S. P. Miller, H. Jing and H. Lin, ACS Cent. Sci., 2018, 4, 71–80 CrossRef CAS PubMed.
- R. Nussinov, H. Jang, M. Zhang, C. J. Tsai and A. A. Sablina, Trends Cancer, 2020, 6, 369–379 CrossRef CAS PubMed.
- R. Nussinov, C. J. Tsai and H. Jang, Adv. Exp. Med. Biol., 2019, 1163, 25–43 CrossRef CAS PubMed.
- K. Gunasekaran, B. Ma and R. Nussinov, Proteins, 2004, 57, 433–443 CrossRef CAS PubMed.
- R. Nussinov, M. Zhang, R. Maloney, Y. Liu, C. J. Tsai and H. Jang, J. Mol. Biol., 2022, 434, 167569 CrossRef CAS PubMed.
- C. J. Tsai and R. Nussinov, PLoS Comput. Biol., 2014, 10, e1003394 CrossRef PubMed.
- A. del Sol, C. J. Tsai, B. Ma and R. Nussinov, Structure, 2009, 17, 1042–1050 CrossRef CAS PubMed.
- Q. Cui and M. Karplus, Protein Sci., 2008, 17, 1295–1307 CrossRef CAS PubMed.
- J. P. Changeux and A. Christopoulos, Cell, 2016, 166, 1084–1102 CrossRef CAS PubMed.
- A. Chatzigoulas and Z. Cournia, Wiley Interdiscip. Rev.: Comput. Mol. Sci., 2021, 11, e1529 CAS.
- G. Kar, O. Keskin, A. Gursoy and R. Nussinov, Curr. Opin. Pharmacol., 2010, 10, 715–722 CrossRef CAS PubMed.
- V. J. Hilser, J. O. Wrabl and H. N. Motlagh, Annu. Rev. Biophys., 2012, 41, 585–609 CrossRef CAS PubMed.
- J. P. Changeux, Annu. Rev. Biophys., 2012, 41, 103–133 CrossRef CAS PubMed.
- S. Schann, S. Mayer, C. Franchet, M. Frauli, E. Steinberg, M. Thomas, L. Baron and P. Neuville, J. Med. Chem., 2010, 53, 8775–8779 CrossRef CAS PubMed.
- T. W. Schwartz and B. Holst, Trends Pharmacol. Sci., 2007, 28, 366–373 CrossRef CAS PubMed.
- C. J. Wenthur, P. R. Gentry, T. P. Mathews and C. W. Lindsley, Annu. Rev. Pharmacol. Toxicol., 2014, 54, 165–184 CrossRef CAS PubMed.
- Z. Fang, C. Grutter and D. Rauh, ACS Chem. Biol., 2013, 8, 58–70 CrossRef CAS PubMed.
- F. Quaglia, T. Lazar, A. Hatos, P. Tompa, D. Piovesan and S. C. E. Tosatto, Curr. Protoc., 2021, 1, e192 CrossRef CAS PubMed.
- A. Bah and J. D. Forman-Kay, J. Biol. Chem., 2016, 291, 6696–6705 CrossRef CAS PubMed.
- F. Jin and F. Grater, PLoS Comput. Biol., 2021, 17, e1008939 CrossRef CAS PubMed.
- R. Nussinov, C. J. Tsai, F. Xin and P. Radivojac, Trends Biochem. Sci., 2012, 37, 447–455 CrossRef CAS PubMed.
- G. Stetz, A. Tse and G. M. Verkhivker, Sci. Rep., 2018, 8, 6899 CrossRef PubMed.
- A. S. Venne, L. Kollipara and R. P. Zahedi, Proteomics, 2014, 14, 513–524 CrossRef CAS PubMed.
- B. C. Lechtenberg, M. P. Gehring, T. P. Light, C. R. Horne, M. W. Matsumoto, K. Hristova and E. B. Pasquale, Nat. Commun., 2021, 12, 7047 CrossRef CAS PubMed.
- L. Alaalm, J. L. Crunden, M. Butcher, U. Obst, R. Whealy, C. E. Williamson, H. E. O'Brien, C. Schaffitzel, G. Ramage, J. Spencer and S. Diezmann, Front. Cell. Infect. Microbiol., 2021, 11, 637836 CrossRef CAS PubMed.
- N. Sostaric and V. van Noort, PLoS Comput. Biol., 2021, 17, e1008988 CrossRef CAS PubMed.
- H. Zhang, J. He, G. Hu, F. Zhu, H. Jiang, J. Gao, H. Zhou, H. Lin, Y. Wang, K. Chen, F. Meng, M. Hao, K. Zhao, C. Luo and Z. Liang, J. Med. Chem., 2021, 64, 15111–15125 CrossRef CAS PubMed.
- H. F. Liu and R. Liu, Briefings Bioinf., 2020, 21, 609–620 CrossRef CAS PubMed.
- R. Nussinov and C. J. Tsai, Curr. Pharm. Des., 2012, 18, 1311–1316 CrossRef CAS PubMed.
- J. A. Byun, B. VanSchouwen, M. Akimoto and G. Melacini, Comput. Struct. Biotechnol. J., 2020, 18, 3803–3818 CrossRef CAS PubMed.
- J. R. Wagner, C. T. Lee, J. D. Durrant, R. D. Malmstrom, V. A. Feher and R. E. Amaro, Chem. Rev., 2016, 116, 6370–6390 CrossRef CAS PubMed.
- A. K. Gupta, X. Wang, C. V. Pagba, P. Prakash, S. Sarkar-Banerjee, J. Putkey and A. A. Gorfe, Chem. Biol. Drug Des., 2019, 94, 1441–1456 CAS.
- B. Musafia and H. Senderowitz, Expert Opin. Drug Discovery, 2010, 5, 943–959 CrossRef CAS PubMed.
- F. Marchetti, E. Moroni, A. Pandini and G. Colombo, J. Phys. Chem. Lett., 2021, 12, 3724–3732 CrossRef CAS PubMed.
- N. Greives and H. X. Zhou, Proc. Natl. Acad. Sci. U. S. A., 2014, 111, 10197–10202 CrossRef CAS PubMed.
- J. W. Biddle, R. Martinez-Corral, F. Wong and J. Gunawardena, eLife, 2021, 10, e65498 CrossRef CAS PubMed.
- A. B. Guzovsky, N. P. Schafer, P. G. Wolynes and D. U. Ferreiro, Methods Mol. Biol., 2022, 2376, 387–398 CrossRef CAS PubMed.
- R. G. Parra, N. P. Schafer, L. G. Radusky, M. Y. Tsai, A. B. Guzovsky, P. G. Wolynes and D. U. Ferreiro, Nucleic Acids Res., 2016, 44, W356–360 CrossRef CAS PubMed.
- S. Gianni, M. I. Freiberger, P. Jemth, D. U. Ferreiro, P. G. Wolynes and M. Fuxreiter, Acc. Chem. Res., 2021, 54, 1251–1259 CrossRef CAS PubMed.
- A. Olivera and J. M. Lopez-Novoa, Br. J. Pharmacol., 1992, 107, 341–346 CrossRef CAS PubMed.
- L. Ponzoni and I. Bahar, Proc. Natl. Acad. Sci. U. S. A., 2018, 115, 4164–4169 CrossRef CAS PubMed.
- P. Csermely, T. Korcsmaros, H. J. Kiss, G. London and R. Nussinov, Pharmacol. Ther., 2013, 138, 333–408 CrossRef CAS PubMed.
- X. Ma, H. Meng and L. Lai, J. Chem. Inf. Model., 2016, 56, 1725–1733 CrossRef CAS PubMed.
- L. Astl and G. M. Verkhivker, J. Chem. Inf. Model., 2020, 60, 1614–1631 CrossRef CAS PubMed.
- L. M. Pietrek, L. S. Stelzl and G. Hummer, Curr. Opin. Struct. Biol., 2023, 78, 102501 CrossRef CAS PubMed.
- P. E. Wright and H. J. Dyson, J. Mol. Biol., 1999, 293, 321–331 CrossRef CAS PubMed.
- G. W. Gomes, M. Krzeminski, A. Namini, E. W. Martin, T. Mittag, T. Head-Gordon, J. D. Forman-Kay and C. C. Gradinaru, J. Am. Chem. Soc., 2020, 142, 15697–15710 CrossRef CAS PubMed.
- P. Kulkarni, S. Bhattacharya, S. Achuthan, A. Behal, M. K. Jolly, S. Kotnala, A. Mohanty, G. Rangarajan, R. Salgia and V. Uversky, Chem. Rev., 2022, 122, 6614–6633 CrossRef CAS PubMed.
- C. Gao, C. Ma, H. Wang, H. Zhong, J. Zang, R. Zhong, F. He and D. Yang, Sci. Rep., 2021, 11, 2985 CrossRef CAS PubMed.
- N. Rangarajan, P. Kulkarni and S. Hannenhalli, PLoS One, 2015, 10, e0126729 CrossRef PubMed.
- M. K. Yoon, D. M. Mitrea, L. Ou and R. W. Kriwacki, Biochem. Soc. Trans., 2012, 40, 981–988 CrossRef CAS PubMed.
- V. N. Uversky, Front. Phys., 2019, 7, 10 CrossRef.
- Z. Peng, J. Yan, X. Fan, M. J. Mizianty, B. Xue, K. Wang, G. Hu, V. N. Uversky and L. Kurgan, Cell. Mol. Life Sci., 2015, 72, 137–151 CrossRef CAS PubMed.
- J. J. Ward, J. S. Sodhi, L. J. McGuffin, B. F. Buxton and D. T. Jones, J. Mol. Biol., 2004, 337, 635–645 CrossRef CAS PubMed.
- H. J. Dyson, Q. Rev. Biophys., 2011, 44, 467–518 CrossRef CAS PubMed.
- P. Radivojac, L. M. Iakoucheva, C. J. Oldfield, Z. Obradovic, V. N. Uversky and A. K. Dunker, Biophys. J., 2007, 92, 1439–1456 CrossRef CAS PubMed.
- R. Nussinov, C. J. Tsai and H. Jang, Front. Oncol., 2019, 9, 1231 CrossRef PubMed.
- D. D. Boehr, R. Nussinov and P. E. Wright, Nat. Chem. Biol., 2009, 5, 789–796 CrossRef CAS PubMed.
- H. N. Motlagh, J. O. Wrabl, J. Li and V. J. Hilser, Nature, 2014, 508, 331–339 CrossRef CAS PubMed.
- Y. Liu, M. Zhang, C. J. Tsai, H. Jang and R. Nussinov, Comput. Struct. Biotechnol. J., 2022, 20, 4257–4270 CrossRef CAS PubMed.
- B. Nagar, O. Hantschel, M. A. Young, K. Scheffzek, D. Veach, W. Bornmann, B. Clarkson, G. Superti-Furga and J. Kuriyan, Cell, 2003, 112, 859–871 CrossRef CAS PubMed.
- T. Saleh, P. Rossi and C. G. Kalodimos, Nat. Struct. Mol. Biol., 2017, 24, 893–901 CrossRef CAS PubMed.
- O. Hantschel, B. Nagar, S. Guettler, J. Kretzschmar, K. Dorey, J. Kuriyan and G. Superti-Furga, Cell, 2003, 112, 845–857 CrossRef CAS PubMed.
- T. Xie, T. Saleh, P. Rossi and C. G. Kalodimos, Science, 2020, 370, eabc2754 CrossRef CAS PubMed.
- K. M. Smith, R. Yacobi and R. A. Van Etten, Mol. Cell, 2003, 12, 27–37 CrossRef CAS PubMed.
- F. Grebien, O. Hantschel, J. Wojcik, I. Kaupe, B. Kovacic, A. M. Wyrzucki, G. D. Gish, S. Cerny-Reiterer, A. Koide, H. Beug, T. Pawson, P. Valent, S. Koide and G. Superti-Furga, Cell, 2011, 147, 306–319 CrossRef CAS PubMed.
- S. Panjarian, R. E. Iacob, S. Chen, T. E. Wales, J. R. Engen and T. E. Smithgall, J. Biol. Chem., 2013, 288, 6116–6129 CrossRef CAS PubMed.
- M. L. Martin-Fernandez, D. T. Clarke, S. K. Roberts, L. C. Zanetti-Domingues and F. L. Gervasio, Cells, 2019, 8, 316 CrossRef CAS PubMed.
- S. Chen, S. Brier, T. E. Smithgall and J. R. Engen, Protein Sci., 2007, 16, 572–581 CrossRef CAS PubMed.
- O. Hantschel and G. Superti-Furga, Nat. Rev. Mol. Cell Biol., 2004, 5, 33–44 CrossRef CAS PubMed.
- F. Al-Mulla, E. J. Milner-White, J. J. Going and G. D. Birnie, J. Pathol., 1999, 187, 433–438 CrossRef CAS PubMed.
- N. T. Ihle, L. A. Byers, E. S. Kim, P. Saintigny, J. J. Lee, G. R. Blumenschein, A. Tsao, S. Liu, J. E. Larsen, J. Wang, L. Diao, K. R. Coombes, L. Chen, S. Zhang, M. F. Abdelmelek, X. Tang, V. Papadimitrakopoulou, J. D. Minna, S. M. Lippman, W. K. Hong, R. S. Herbst, I. I. Wistuba, J. V. Heymach and G. Powis, J. Natl. Cancer Inst., 2012, 104, 228–239 CrossRef CAS PubMed.
- J. C. Hunter, A. Manandhar, M. A. Carrasco, D. Gurbani, S. Gondi and K. D. Westover, Mol. Cancer Res., 2015, 13, 1325–1335 CrossRef CAS PubMed.
- M. V. Cespedes, F. J. Sancho, S. Guerrero, M. Parreno, I. Casanova, M. A. Pavon, E. Marcuello, M. Trias, M. Cascante, G. Capella and R. Mangues, Carcinogenesis, 2006, 27, 2190–2200 CrossRef CAS PubMed.
- M. C. Garassino, M. Marabese, P. Rusconi, E. Rulli, O. Martelli, G. Farina, A. Scanni and M. Broggini, Ann. Oncol., 2011, 22, 235–237 CrossRef CAS PubMed.
- A. Wittinghofer, K. Scheffzek and M. R. Ahmadian, FEBS Lett., 1997, 410, 63–67 CrossRef CAS PubMed.
- Y. Wang, D. Ji, C. Lei, Y. Chen, Y. Qiu, X. Li, M. Li, D. Ni, J. Pu, J. Zhang, Q. Fu, Y. Liu and S. Lu, Comput. Struct. Biotechnol. J., 2021, 19, 1184–1199 CrossRef CAS PubMed.
- S. Lu, H. Jang, R. Nussinov and J. Zhang, Sci. Rep., 2016, 6, 21949 CrossRef CAS PubMed.
-
H. Jang, J. Chen, L. M. Iakoucheva and R. Nussinov, bioRxiv, 2023 DOI:10.1101/2023.01.26.525746.
- R. Nussinov, C. J. Tsai and H. Jang, Cancer Res., 2022, 82, 4114–4123 CrossRef CAS PubMed.
- M. Geyer and A. Wittinghofer, Curr. Opin. Struct. Biol., 1997, 7, 786–792 CrossRef CAS PubMed.
- E. M. Terrell and D. K. Morrison, Cold Spring Harbor Perspect. Med., 2019, 9, a033746 CrossRef CAS PubMed.
- M. Zhang, H. Jang and R. Nussinov, Chem. Sci., 2019, 10, 3671–3680 RSC.
- D. A. Fruman, H. Chiu, B. D. Hopkins, S. Bagrodia, L. C. Cantley and R. T. Abraham, Cell, 2017, 170, 605–635 CrossRef CAS PubMed.
- M. S. Lawrence, P. Stojanov, C. H. Mermel, J. T. Robinson, L. A. Garraway, T. R. Golub, M. Meyerson, S. B. Gabriel, E. S. Lander and G. Getz, Nature, 2014, 505, 495–501 CrossRef CAS PubMed.
- L. Stephens, R. Williams and P. Hawkins, Curr. Opin. Pharmacol., 2005, 5, 357–365 CrossRef CAS PubMed.
- L. M. Thorpe, H. Yuzugullu and J. J. Zhao, Nat. Rev. Cancer, 2015, 15, 7–24 CrossRef CAS PubMed.
- R. Williams, A. Berndt, S. Miller, W. C. Hon and X. Zhang, Biochem. Soc. Trans., 2009, 37, 615–626 CrossRef CAS PubMed.
- C. H. Huang, D. Mandelker, O. Schmidt-Kittler, Y. Samuels, V. E. Velculescu, K. W. Kinzler, B. Vogelstein, S. B. Gabelli and L. M. Amzel, Science, 2007, 318, 1744–1748 CrossRef CAS PubMed.
- M. S. Miller, O. Schmidt-Kittler, D. M. Bolduc, E. T. Brower, D. Chaves-Moreira, M. Allaire, K. W. Kinzler, I. G. Jennings, P. E. Thompson, P. A. Cole, L. M. Amzel, B. Vogelstein and S. B. Gabelli, Oncotarget, 2014, 5, 5198–5208 CrossRef PubMed.
- D. A. Fruman and C. Rommel, Nat. Rev. Drug Discovery, 2014, 13, 140–156 CrossRef CAS PubMed.
- R. Nussinov, G. Wang, C. J. Tsai, H. Jang, S. Lu, A. Banerjee, J. Zhang and V. Gaponenko, Trends Cancer, 2017, 3, 214–224 CrossRef CAS PubMed.
- M. Zhang, H. Jang, V. Gaponenko and R. Nussinov, Biophys. J., 2017, 113, 1956–1967 CrossRef CAS PubMed.
- M. Zhang, H. Jang, Z. Li, D. B. Sacks and R. Nussinov, Structure, 2021, 29, 768–777e762 CrossRef CAS PubMed.
- H. Chong and K. L. Guan, J. Biol. Chem., 2003, 278, 36269–36276 CrossRef CAS PubMed.
- C. Herrmann, G. Horn, M. Spaargaren and A. Wittinghofer, J. Biol. Chem., 1996, 271, 6794–6800 CrossRef CAS PubMed.
- H. Jang, M. Zhang and R. Nussinov, Comput. Struct. Biotechnol. J., 2020, 18, 737–748 CrossRef CAS PubMed.
- S. Li, H. Jang, J. Zhang and R. Nussinov, Structure, 2018, 26, 513–525e512 CrossRef CAS PubMed.
- Z. L. Li, P. Prakash and M. Buck, ACS Cent. Sci., 2018, 4, 298–305 CrossRef CAS PubMed.
- T. Travers, C. A. Lopez, Q. N. Van, C. Neale, M. Tonelli, A. G. Stephen and S. Gnanakaran, Sci. Rep., 2018, 8, 8461 CrossRef PubMed.
- T. Improta-Brears, S. Ghosh and R. M. Bell, Mol. Cell. Biochem., 1999, 198, 171–178 CrossRef CAS PubMed.
- N. R. Michaud, J. R. Fabian, K. D. Mathes and D. K. Morrison, Mol. Cell. Biol., 1995, 15, 3390–3397 CrossRef CAS PubMed.
- G. Tzivion, Z. Luo and J. Avruch, Nature, 1998, 394, 88–92 CrossRef CAS PubMed.
- A. J. Muslin, J. W. Tanner, P. M. Allen and A. S. Shaw, Cell, 1996, 84, 889–897 CrossRef CAS PubMed.
- C. Rommel, G. Radziwill, J. Lovric, J. Noeldeke, T. Heinicke, D. Jones, A. Aitken and K. Moelling, Oncogene, 1996, 12, 609–619 CAS.
- H. Lavoie and M. Therrien, Nat. Rev. Mol. Cell Biol., 2015, 16, 281–298 CrossRef CAS PubMed.
- A. S. Dhillon, S. Meikle, Z. Yazici, M. Eulitz and W. Kolch, EMBO J., 2002, 21, 64–71 CrossRef CAS PubMed.
- D. Abraham, K. Podar, M. Pacher, M. Kubicek, N. Welzel, B. A. Hemmings, S. M. Dilworth, H. Mischak, W. Kolch and M. Baccarini, J. Biol. Chem., 2000, 275, 22300–22304 CrossRef CAS PubMed.
- M. Jaumot and J. F. Hancock, Oncogene, 2001, 20, 3949–3958 CrossRef CAS PubMed.
- D. Matallanas, M. Birtwistle, D. Romano, A. Zebisch, J. Rauch, A. von Kriegsheim and W. Kolch, Genes Cancer, 2011, 2, 232–260 CrossRef CAS PubMed.
- M. Zhang, H. Jang and R. Nussinov, Phys. Chem. Chem. Phys., 2019, 21, 12021–12028 RSC.
- G. A. Hobbs, C. J. Der and K. L. Rossman, J. Cell Sci., 2016, 129, 1287–1292 CAS.
- F. E. Hood, Y. M. Sahraoui, R. E. Jenkins and I. A. Prior, Oncogene, 2023, 42, 1224–1232 CrossRef CAS PubMed.
- R. Nussinov, C. J. Tsai, M. Chakrabarti and H. Jang, Cancer Res., 2016, 76, 18–23 CrossRef CAS PubMed.
- A. Y. Volmar, H. Guterres, H. Zhou, D. Reid, S. Pavlopoulos, L. Makowski and C. Mattos, Biophys. J., 2022, 121, 3616–3629 CrossRef CAS PubMed.
- J. A. Parker, A. Y. Volmar, S. Pavlopoulos and C. Mattos, Structure, 2018, 26, 810–820e814 CrossRef CAS PubMed.
- J. A. Parker and C. Mattos, Cold Spring Harbor Perspect. Med., 2018, 8, a031427 CrossRef PubMed.
- H. Jang, S. Muratcioglu, A. Gursoy, O. Keskin and R. Nussinov, Biochem. J., 2016, 473, 1719–1732 CrossRef CAS PubMed.
- F. D. Tsai, M. S. Lopes, M. Zhou, H. Court, O. Ponce, J. J. Fiordalisi, J. J. Gierut, A. D. Cox, K. M. Haigis and M. R. Philips, Proc. Natl. Acad. Sci. U. S. A., 2015, 112, 779–784 CrossRef CAS PubMed.
- E. S. Knudsen, V. Kumarasamy, R. Nambiar, J. D. Pearson, P. Vail, H. Rosenheck, J. Wang, K. Eng, R. Bremner, D. Schramek, S. M. Rubin, A. L. Welm and A. K. Witkiewicz, Cell Rep., 2022, 38, 110448 CrossRef CAS PubMed.
- M. Barbiero, L. Cirillo, S. Veerapathiran, C. Coates, C. Ruffilli and J. Pines, Open Biol., 2022, 12, 220057 CrossRef CAS PubMed.
- M. Malumbres and M. Barbacid, Nat. Rev. Cancer, 2009, 9, 153–166 CrossRef CAS PubMed.
- H. W. Lau, H. T. Ma, T. K. Yeung, M. Y. Tam, D. Zheng, S. K. Chu and R. Y. C. Poon, Cell Rep., 2021, 37, 109808 CrossRef CAS PubMed.
- R. F. Brooks, Cell Div., 2023, 18, 2 CrossRef CAS PubMed.
- C. J. Tsai, Z. E. Sauna, C. Kimchi-Sarfaty, S. V. Ambudkar, M. M. Gottesman and R. Nussinov, J. Mol. Biol., 2008, 383, 281–291 CrossRef CAS PubMed.
- C. Kimchi-Sarfaty, J. M. Oh, I. W. Kim, Z. E. Sauna, A. M. Calcagno, S. V. Ambudkar and M. M. Gottesman, Science, 2007, 315, 525–528 CrossRef CAS PubMed.
- R. C. Hunt and C. Kimchi-Sarfaty, N. Engl. J. Med., 2022, 387, 753–756 CrossRef PubMed.
- Y. Jiang, S. S. Neti, I. Sitarik, P. Pradhan, P. To, Y. Xia, S. D. Fried, S. J. Booker and E. P. O'Brien, Nat. Chem., 2023, 15, 308–318 CrossRef CAS PubMed.
- T. R. Weikl and F. Paul, Protein Sci., 2014, 23, 1508–1518 CrossRef CAS PubMed.
|
This journal is © The Royal Society of Chemistry 2023 |
Click here to see how this site uses Cookies. View our privacy policy here.