DOI:
10.1039/D2AN01508K
(Paper)
Analyst, 2023,
148, 120-127
Fully integrated on-line strategy for highly sensitive proteome profiling of 10–500 mammalian cells†
Received
13th September 2022
, Accepted 16th November 2022
First published on 23rd November 2022
Abstract
Recent development in proteomic sample preparation using nanofluidic devices has made single-cell proteome profiling possible. However, these nanofluidic devices require special expertise and costly nanopipetting instruments. They are also specially designed for single cells, are not well-suited for profiling rare samples consisting of a few hundred mammalian cells, arguably a more common need that remains a great challenge. Herein, we developed an easy-to-use and scalable device for processing low-input samples, which combined the merits of previously reported rare cell proteomic reactor (RCPR) and mixed-mode simple and integrated spintip-based proteomics technology, as an alternative to nanofluidic devices. All steps of proteomics sample preparation, including protein preconcentration, impurity removal, reduction, alkylation, digestion, and desalting, were fully integrated in our workflow, and the device can be directly connected to online nanoLC-MS system after processing the rare samples. Using the developed 3-frit mixed-mode RCPR, we identified on average 946 ± 158, 2 998 ± 106, and 3 934 ± 85 protein groups in data-dependent acquisition (DDA) mode from 10, 100, and 500 fluorescence-activated cell sorting (FACS)-sorted 293T cells, respectively. As an illustrative application of this technology, we performed a label-free proteome comparison of 500 FACS-sorted mouse cochlear hair cells of two different ages. On average, 2 595 ± 230 and 2 042 ± 120 protein groups were quantified in the juvenile and the adult samples in DDA mode, respectively, achieving dynamic ranges of over 6 orders of magnitude for both.
Introduction
Although shotgun proteomics has become a well-established and widely used tool in biological research, typical experimental protocols require microgram-level of input samples. In many applications, the accessible samples cannot meet this input requirement, as is often the case in clinical proteomics studies or with primary cells that cannot be expanded in vitro. State-of-the-art sample preparation methods for standard proteomics, such as filter-aided sample preparation (FASP),1 “in-StageTip” (iST),2 single-pot solid-phase-enhanced sample preparation (SP3),3 and simple and integrated spintip-based proteomics technology (SISPROT),4,5 often fit for processing starting materials containing more than 200 ng of proteins or about 2000 human cells. All these strategies integrate multiple steps in a single device to avoid sample loss during liquid transfer. However, a considerable amount of sample may still be lost due to non-specific binding to the tube/tip inner surface, or to magnetic beads or desalting resins. On the other hand, the recently developed devices for single-cell proteomics, such as nanoliter-scale oil–air–droplet (OAD) chip,6 integrated device for single-cell analysis (iPAD-1),7 and nanodroplet processing in one-pot for trace samples (nanoPOTS),8–10 are sophisticatedly designed for single or a few cells and thus not suitable for samples consisting of a few hundreds of cells. In addition, these nanofluidic platforms require highly skilled expertise and costly robotic nanopipetting instruments, and may not be accessible to most life science researchers. Therefore, there is a need for better and easy-to-use sample preparation methods for low-input proteomics to bridge the gap between standard proteomics and single-cell proteomics.
Significant progress has been made towards sample preparation in low-input proteomics recently, the major results of which are summarized in Table S1.† Wu et al., quantitatively assessed sample loss during each step of a conventional in-solution sample preparation workflow and achieved substantial increase in protein identification by using commercial LoBind tubes and in-line desalting.11 After optimization, Wu et al., identified on average 829 proteins with 1000 U2OS osteosarcoma cells. Zhang et al., developed a miniaturized filter-aided sample preparation (micro-FASP) microreactor which dramatically reduced the volume and filter size of conventional FASP reactors.12 Thus, sample loss and contamination were decreased, and an average of 1895 and 3069 protein groups were identified from 100 and 1000 MCF-7 cells, respectively. Recently, Yuan et al., developed a hollow fibre membrane-aided fully automated sample treatment (FAST) device to be integrated online with nanoLC-MS.13 Protein denaturation, reduction, desalting, and digestion were integrated in FAST, and 644 ± 8 (n = 3) and 1 673 ± 4 (n = 3) protein groups were identified from 100 and 500 HeLa cells, respectively. Liang et al., scaled up nanoPOTS and developed a fully automated platform termed autoPOTS (automated preparation in one pot for trace samples) based on a modified autosampler and a robotic pipetting platform.14 Using 30 μm I.D. narrow-bore columns together with a low flow rate at ∼40 nL min−1 and a 100 min gradient, Liang et al., identified on average 1461, 2679, and 3347 protein groups from 10, ∼150, and ∼500 HeLa cells, respectively. Despite those advances, in-depth proteomic analysis for rare cells remains a major challenge for most laboratories.
Hearing and balance rely on rare mechanosensory receptor hair cells in the inner ear to convey information to the brain, a process that remains poorly understood.15 However, only a few proteomic studies were conducted in this area, mainly due to difficulties in developing ultrasensitive proteomic workflows and in acquiring pure hair cells. Hickox et al., identified 12 712 proteins in total from 200
000 hair cells collected from 132 sacrificed mice and found 38 hair cell-specific protein groups by comparing the hair cell proteome with other inner ear subproteomes.15 Zhu et al., identified a few tens and nearly 600 protein groups from 1 cell and 20 cells, respectively, isolated from a chick utricle using nanoPOTS.16
Herein we report a 3-frit mixed-mode rare cell proteomic reactor (RCPR) for processing 10–500 mammalian cells, which identified on average 946 ± 158, 2 998 ± 106, and 3 934 ± 85 protein groups from 10, 100, and 500 293T cells in data-dependent acquisition (DDA) mode. In view of the mechanism of mixed-mode SISPORT,5,17 we improved our previously reported RCPR18 by replacing the strong cation exchange (SCX) monolith column with mixed-mode SCX and strong anion exchange (SAX) beads, and adding C18 beads below them to avoid potential sample loss from ion exchange beads. Using this 3-frit mixed-mode RCPR, we compared the proteomes of inner ear hair cells between juvenile and adult mice, as a demonstration of this technology.
Experimental
Mouse model, cell culture and FACS sorting
All animals were maintained under pathogen-free conditions and cared with free access to water and food. All animal studies were reviewed and approved by the Institutional Animal Care and Use Committee at Southern University of Science and Technology, China. Juvenile and adult hair cells were collected from Atoh1-CreER+; Ai9 (Atoh1CreER+; Ai9/+; Atoh1CreER+; Ai9/Ai9) mice at postnatal days 10–11 (P10–11) and P30–45, respectively. The hair cells are genetically and permanently labelled with tdTomato and thus can be well sorted in fluorescence-assisted cell sorting (FACS) with high purity. Tamoxifen (3 mg per 40 g of body weight, Sigma) was given at P0 and P1. The cochlea of each mouse was dissected under microscopy and then digested with protease (Cat#: P5147, Sigma) at 25 °C for 30 min. Then 1× phosphate buffered saline (PBS) was added to stop the reaction. Subsequently, the cochlea was blown into single cells with a poly-(L-lysine)-graft-poly(ethylene glycol) (PLL-g-PEG) coated glass tube without adding fetal bovine serum (FBS). Human embryonic kidney (HEK) 293T cells were cultured as previously reported.4 Culture medium in 293T cells was removed by washing with PBS for four times. Five microliters of 7-amino-actinomycin D (7-AAD) per million cells were added into 293T cells before sorting.
Both hair cells and 293T cells were flow-sorted using a BD FACSAria SORP flow cytometer (BD Biosciences, San Jose, USA) with pure PBS as the sheath liquid. Forward scatter and side scatter were used to exclude cell debris and aggregated cells from the analysis. Viable 293T cells were gated based on the 7-AAD staining of dead cells. Hair cells were gated based on the expression tdTomato (DsRed+) signal. Desired numbers of 293T cells or 500 hair cells were sorted and counted in “single cell mode” to ensure purity. Protein-LoBind tubes (0.5 mL, Eppendorf) were cut to ca. 0.2 mL left due to height limit and put on a 96-well plate. To minimize sample loss, all cells were directly sorted into 20 μL of cell lysis buffer in those truncated protein-LoBind tubes. The final 1× lysis buffer was composed of 20 mM N-2-hydroxyethylpiperazine-N-2-ethane sulfonic acid (HEPES) (pH 7.4), 150 mM NaCl, 600 mM guanidine HCl, 1% (w/v) N-dodecyl β-D-maltoside (DDM), and protease inhibitor mixture [1 mM phenylmethylsulfonyl fluoride (PMSF), 1 μg mL−1 leupeptin, 1 μg mL−1 pepstatin, and 1 μg mL−1 aprotinin], and the lysis was assisted by non-contact intermittent sonication in an ice-water ultrasonic bath. Micro BCA Kit (Thermo scientific) was used to determine the protein concentration.
Preparation of 3-frit mixed-mode RCPR
Three-frit mixed-mode RCPR (3 μm C18) was fabricated as follows: Kasil#1 solution (PQ Corporation) was freshly mixed with formamide (v/v, 10/1) well and was allowed to migrate via capillary action to the middle part of an empty capillary column (ca. 20 cm length, 100 μm I.D., Polymicro Technologies). An approximately 1 mm section of the silicate solution located in the middle of the column was heated at 200 °C for ca. 15 s by contact with a customized soldering iron tip, and the capillary column was slowly rotated during heating. After heating, the residual silicate solution was removed immediately by back-flushing with ca. 50 μL of isopropanol under ca. 3 MPa using a pressure pump, and then the capillary column was dried under N2 for a few minutes. Subsequently, the prepared first frit was reheated at 200 °C for 30 s. Next, the capillary column with the first frit was packed with 2 mg mL−1 of 3 μm C18 slurry (120 Å ReproSil-Pur, Dr Maisch GmbH, Germany) to a designed length. After packing C18 beads, the capillary column was dried under N2 as well. Then the second frit was formed ca. 5 mm away from the C18 beads in a similar way as the first one, except that the silicate solution was slowly drawn by a 10 μL syringe and reheating was not applied. Subsequently, 3 mg mL−1 of 20 μm POROS SCX and SAX beads (Applied Biosystems, USA., 1
:
1 mixed in 80% MeOH and 20% 200 mM NaCl) were packed below the second frit to a designed length. After packing SCX and SAX beads, the capillary column was dried under N2 as well. Finally, the third frit was formed ca. 5 mm away from the SCX and SAX beads in the same way as the second frit. Both C18, SCX, and SAX slurries were sonicated in an ultra-sonication bath for 5 min before packing and the pressure was slowly released after packing. The designed length of C18 and SCX/SAX beads was ca. 1 mm for processing 10 293T cells, ca. 3 mm for processing 10 ng of 293T digested peptides or 100 293T cells, ca. 5 mm for processing 500 293T or hair cells, respectively.
Two-frit mixed-mode RCPR (3 μm C18) was prepared in the same way as 3-frit mixed-mode RCPR, except that SCX and SAX beads were directly packed under C18 beads without the second frit. Two-frit mixed-mode RCPR (10 μm C18) was prepared in the same way as 2-frit mixed-mode RCPR (3 μm C18) except that 10 μm C18 beads was replaced by 3 μm C18 beads.
Integrated proteomics sample preparation with mixed-mode RCPR
After cell lysis, the cell lysate was acidified and diluted by 0.1% formic acid (FA) in an Eppendorf Protein-LoBind tube. Before use, mixed-mode RCPR was equilibrated with methanol and then 100 mM and 10 mM potassium citrate buffer (pH 3), respectively. Acidified protein samples (pH 3) were loaded into the RCPR capillary slowly under low pressure, and the loading was repeated to minimize potential sample loss. After removing impurities with 20% (v/v) acetonitrile (ACN) in 8 mM potassium citrate buffer (pH 3) and then 100% ACN with 0.1% FA, proteins were reduced by 10 mM Tris (2-carboxyethyl) phosphine hydrochloride (TCEP) in 9 mM potassium citrate buffer (pH 3) at room temperature for 15 min. Subsequently, TCEP was removed by washing with ∼20 μL 0.1% FA and then the pH of the sample was adjusted with 20 mM Tris-HCl buffer (pH 8). Next, proteins were digested by mixed trypsin (Promega, USA), LysC (Wako, Germany), and 10 mM iodoacetamide in 50 mM Tris-HCl buffer (pH 8) for 2 h at 37 °C (in darkness). The ratio of protein:enzyme (w
:
w) was roughly 5
:
1, 2
:
1, and 1
:
1 for 500, 100, and 10 cells, respectively. Next, the peptides were then transferred from the ion exchange beads to C18 beads with ca. 50 μL of 500 mM NaCl (pH 10) slowly. After washing with ca. 60 μL of 1% FA thoroughly to desalt, the processed RCPR was directly connected to a home-made nanoLC analytical column (100 μm I.D. × 20 cm, 1.9 μm/120 Å ReproSil-Pur C18 resins, Dr Maisch GmbH) with a PicoClear™ zero-dead-volume union (New Objective). A new mixed-mode RCPR and fresh reagents were used for each sample to avoid carry-over.
Data analysis
RAW data from Q Exactive HF-X were processed using Proteome Discoverer (PD) 2.4 software with Sequest HT and MSPepSearch search engines as previously reported.19 RAW data from Orbitrap Fusion were processed using PD 2.4 the same as Q Exactive HF-X data, except that MSPepSearch search engine was not used and the precursor and fragment mass tolerances were set to be 10 ppm and 0.6 Da, respectively.
RAW data from timsTOF Pro were also processed using MSFragger with FragPipe.20 Protein identification in MSFragger used the ProteinProphet algorithm and the false discovery rate (FDR) was set at 0.01 for ion FDR, peptide FDR, and protein FDR Match between runs was enabled for label-free quantification (LFQ) and the other settings are default. RAW data from timsTOF Pro were also processed using Open-pFind software for deep analysis.21 FASTA, modification and enzyme settings etc. were the same as that used in PD 2.4.
Human UniProt FASTA database (74 811 entries, downloaded on March 20, 2020) and mouse UniProt FASTA database (50 306 entries, downloaded on February 12, 2020) were used for human and mouse samples, respectively.
The copy number values were calculated using the Proteomic ruler in Perseus. Volcano plot of two-sample t-tests (p value ≤ 0.05, S0 = 2), heat map and gene ontology categories of biological processes (GOBP) analysis were performed in Perseus as well.
Results and discussion
Design of online RCPR-nanoLC-MS workflow
We previously reported the SCX monolith-based RCPR in which protein preconcentration, reduction, alkylation, and digestion were integrated into a single capillary column.18 To further reduce the potential sample loss during buffer exchange and to avoid salt contamination of high-resolution MS during online desalting, we made the following improvements. First, we replaced the SCX monolith with mixed-mode SCX and SAX beads, since it is impractical to prepare mixed-mode SCX and SAX monolith together with C18 matrix. In addition, mixed-mode ion-exchange beads can reduce sample loss in comparison to SCX beads.5 Second, we added C18 beads below the ion-exchange beads for collecting potentially lost sample from the ion-exchange beads, as well as to integrate the desalting step after digestion (top panel of Fig. 1), to avoid a separate desalting step using an SPE column in other workflows. Accordingly, frits (ca. 1 mm length for each) were formed in situ to prevent the loss of beads and samples. Importantly, our device enables removing impurities including DDM etc. before reduction and digestion. It has been shown previously that having both enzymes and proteins adsorbed onto ion-exchange beads can lead to high digestion efficiency and low auto-digestion.5,22 In addition, to avoid carry-over, fresh RCPR devices and reagents were used for each sample. As depicted in the bottom panel of Fig. 1, the RCPR capillary column after processing samples is directly connected online to a C18 analytical column using a PicoClear zero-dead-volume union for nanoLC-MS analysis. Therefore, four procedures in conventional sample preparation, including peptide elution, drying, redissolution, and sample loading onto nanoLC-MS, were obviated, minimizing sample loss and saving time. In addition, the RCPR is readily scalable, since the packed length of the ion-exchange beads and C18 beads can be easily controlled according to the sample amount. Active non-specific adsorption sites on frits, ion-exchange and C18 beads could be saturated before use with peptides from nonmammalian species, like E. coli or yeast. However, small number of interference proteins need to be removed in bioinformatics analysis.
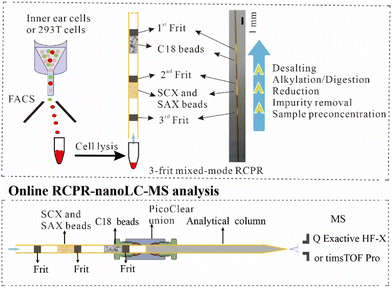 |
| Fig. 1 Workflow of online RCPR-nanoLC-MS analysis. Top panel: Inner ear cells or 293T cells were sorted by FACS and then processed by mixed-mode RCPR. Bottom panel: Processed mixed-mode RCPR was online connected to nanoLC-MS. | |
FACS sorting was used to collect the desired number of pure hair cells from juvenile and adult mice. To avoid contamination, the hair cells were dispersed with a PLL-g-PEG-coated glass tube without adding FBS and the hair cells were sorted in pure PBS. The resulting layer of grafted PEG in PLL-g-PEG coating imparts a high resistance to protein nonspecific adsorption, and thus cells should adhere minimally to the surface and remain suspended in the medium.23,24 In addition, hair cells (or HEK 293T cells) were directly sorted into lysis buffer in protein-LoBind tubes instead of traditional FACS tubes, to minimize sample loss. Before sonication-assisted cell lysis, most of the supernatant was carefully removed. After lysis, the cell lysate was directly loaded into the RCPR under pressure for integrated sample pretreatment (top panel of Fig. 1). In summary, our new design leveraged the benefits of an easy-to-use and miniaturized proteomic reactor and combined merits of previously reported RCPR and mixed-mode SISPROT.5,18
Development of the mixed-mode RCPR
For processing low-input samples, we compared three types of mixed-mode RCPRs: 2-frit RCPR (3 μm C18), 2-frit RCPR (10 μm C18), 3-frit RCPR (3 μm C18), as summarized in Fig. 2 and Fig. S1.† We could identify over 2400 protein groups from 10 ng of 293T total cell lysate processed by 2-frit RCPR (3 μm C18), but the variation was quite large. To improve repeatability, we first tested increasing the size of C18 beads from 3 μm to 10 μm, as the size of SCX and SAX beads is 20 μm. However, the variation did not improve. We then tested adding the third frit to prevent the mixing of ion-exchange beads and C18 beads, since proteins cannot be eluted from C18 beads efficiently. We then identified on average 2421 protein groups and 14 184 peptide groups from 10 ng of 293T total cell lysate, and the coefficient of variation (CV) remarkably reduced from 40% and 55% to 12% and 15% for protein groups and peptide groups, respectively (Fig. 2A and Fig. S1A†). The Venn diagrams of protein groups identified demonstrated the same trend, 55% of the detected protein groups were found in all three replicates using 3-frit RCPR (3 μm C18), whereas only 24% and 29% overlaps were obtained using those two types of 2-frit RCPR (Fig. 2B and C and Fig. S1B†). The higher variation and lower overlap using the 3-frit RCPR suggested that proteins were likely lost to non-specific binding to the C18 beads. This finding highlighted the importance of preventing undigested proteins from interacting the C18 resin in our design.
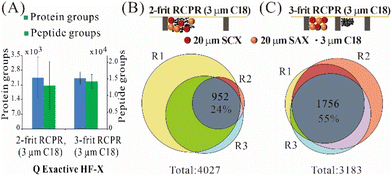 |
| Fig. 2 Method development of mixed-mode RCPR. (A) Identified protein groups and peptide groups from 10 ng of 293T total cell lysate processed by 2-frit (3 μm C18) and 3-frit (3 μm C18) mixed-mode RCPR, respectively. Data were collected on a Q Exactive HF-X. Error bars show standard deviations from three independent replicates. (B and C) Venn diagrams of protein groups identified by these two types of mixed-mode RCPR. R1–3 indicate three independent replicates. | |
Performance of 3-frit mixed-mode RCPR
Since 3-frit mixed-mode RCPR (3 μm C18) achieved much better repeatability than the other two types, we further tested its performance on different numbers of FACS-sorted viable 293T cells. Using 3-frit mixed-mode RCPR (3 μm C18), we identified on average 946, 2 998, and 3934 protein groups from 10, 100, and 500 FACS-sorted 293T cells (Fig. 3A), respectively, whereas 219 protein groups were found in the blank controls. In addition, an average of 4 037, 20 601, and 29 945 peptide groups were identified from 10, 100, and 500 FACS-sorted 293T cells, respectively (Fig. 3A). The numbers of identified protein groups and peptide groups increased remarkably when cell number increased by several folds. Those results indicated good applicability of our method for as few as 10 cells to hundreds of cells. The average percentages of identified peptides having fully tryptic cleavage sites were 74%, 84%, and 76% for 10, 100, and 500 293T cells, respectively. The overlap of identified proteins from samples within each group is shown in Fig. 3B–D. The ratios of identified proteins in all the three replicates were 28%, 58%, and 68% for 10, 100, and 500 293Tcells, respectively. Similar results and trends were observed for label-free quantified protein groups (Fig. S2†). As summarized in Table S1,† the average number of identified protein groups and peptide groups from 100/500 cells processed by 3-frit mixed-mode RCPR were higher than the reported ones, indicating the developed 3-frit mixed-mode RCPR are especially suitable for processing around a few hundred cells.
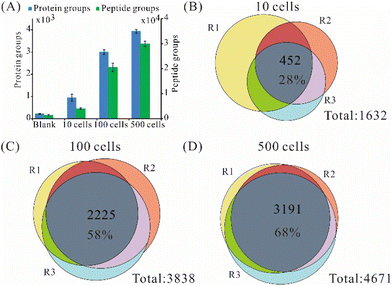 |
| Fig. 3 Performance of 3-frit mixed-mode RCPR for 0, 10, 100, and 500 viable FACS-sorted 293T cells on a Q Exactive HF-X. Error bars indicate standard deviations from three independent replicates. (A–D) Venn diagrams of protein groups identified from 10 (B), 100 (C), and 500 (D) 293T cells. R1–3 stand for three independent replicates. | |
Optimization of data-dependent acquisition parameters on timsTOF Pro
To get better results, we utilized a more sensitive MS, timsTOF Pro, for real sample analysis. We systematically optimized its DDA parameters to accumulate more peptide precursors and to improve MS/MS spectra quality especially for low-input proteomics. After optimization, we identified 3 963 ± 44 and 5 514 ± 42 protein groups from 10 and 50 ng of commercially sourced Pierce HeLa digest, which were improved by 39% and 31%, respectively, when compared with the default settings on timsTOF Pro for low amounts of samples (Fig. 4A). The average numbers of label-free quantified protein groups were 4152 and 5665 from 10 and 50 ng of HeLa digest, respectively (Fig. 4A). The numbers of label-free quantified protein groups were higher than identified ones, due to “match between runs” was enabled in LFQ. Also, we identified 17 273 ± 202 unique peptides and 51 126 ± 1626 peptide spectrum matches (PSMs) from 10 ng of HeLa digest (Fig. 4B); whereas from 50 ng of HeLa digest, we identified 30 520 ± 509 unique peptides and 95 473 ± 3500 PSMs (Fig. 4B). Those results are substantially better than what we achieved previously on a Thermo Q Exactive HF-X.19
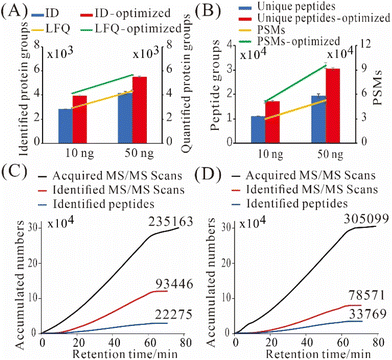 |
| Fig. 4 Proteomic analysis from 10 and 50 ng of HeLa digest on a timsTOF Pro before and after optimization of data-dependent acquisition parameters. (A) Identified and label-free quantified protein groups. (B) Unique peptides and PSMs. Error bars indicate standard deviations from three technical replicates. (C and D) Accumulated numbers of acquired MS/MS scans, identified MS/MS scans, and identified peptides of default method (C) and optimized method (D). | |
The major changes of DDA parameters during the optimization were listed in Table S2:† Intensity threshold for MS2 scans and mobilogram peak detection was decreased from 1000 and 5000 to 100 and 4000, respectively, to detect more low-abundance peaks; mass range, scan range of ion mobility (1/K0) and charge range were narrowed down from 100–1700 m/z, 0.60–1.60 i.e., V s cm−2 and 0–5 to 300–1500 m/z, 0.75–1.30 i.e., V s cm−2 and 2–3, respectively, to reduce singly charged impurities; ramp time was increased from 166 to 200 ms to accumulate more precursors; number of PASEF MS/MS scans was decreased from 10 to 4, and total cycle time was reduced from 1. 89 s to 1.03 s, as the available peptides were limited; target intensity was decreased from 20
000 to 5000 to save time for triggering more MS/MS scans for low-abundance peaks. Release time was decreased from 0.40 to 0.30 min, and current intensity/previous intensity was decreased from 4.00 to 3.00, to allow for more MS/MS repetitions on low-abundance peaks. As a result, more MS/MS scans were triggered, less MS/MS scans were identified, but more peptides were identified (Fig. 4C and D). Importantly, the ratio of identified MS/MS scans to peptide sequences (SPR) decreased from 4.2 to 2.3, which indicated that the sampling redundancy was reduced by 45%.
Proteome comparison of hair cells between juvenile and adult cochlea
We then applied the developed 3-frit mixed-mode RCPR workflow to the proteome comparison of highly pure cochlear hair cells between juvenile and adult mice collected from FACS sorting. Atoh1-CreER is a transgenic mouse in which tamoxifen-inducible Cre is efficient when tamoxifen is given at P0 and P1. Both inner hair cells and outer hair cells would be labelled with tdTomato in Atoh1-CreER+; Ai9 (Atoh1CreER+; Ai9/+; Atoh1CreER+; Ai9/Ai9) mice.25,26 Thus, tdTomato+ cochlear hair cells are subject to FACS. To enable LFQ, the timsTOF Pro data were analyzed using MSFragger with the “match between runs” feature. The number of hair cells collected from adult cochlea is much less than that in juvenile mice, allowing only hundreds of hair cells in each triplicate analysis from one mouse, indicating the necessity of low-input proteomics herein. The protein amount of 500 hair cells was estimated to be 32.5 ng using Micro BCA Kit. Despite similar cell sizes, nearly 600 more protein groups on average were detected from hair cells of juvenile mice: 2 654 ± 235 and 2 074 ± 115 protein groups were identified from 500 hair cells of juvenile and adult mice, respectively (Fig. 5A). Moreover, 2 595 ± 230 protein groups were label-free quantified (protein groups quantified using a LFQ algorithm) from 500 hair cells of juvenile mice, 27% higher than that from adult mice (Fig. 5A). Similar numbers of unique peptides but 14% more PSMs were found in hair cells of juvenile mice (Fig. 5B). Pearson correlation coefficients between hair cells from juvenile and juvenile mice ranged between 0.837–0.876 and 0.850–0.892, respectively (Fig. 5C and D), indicating acceptable repeatability for processing merely 500 hair cells with the 3-frit mixed-mode RCPR. Remarkably, the apparent dynamic ranges of protein expression were over 6 orders of magnitude for hair cells from both juvenile and adult mice (Fig. 5E and F). Interestingly, the median protein expression was 1.3 × 106 and 6.9 × 105 copies per cell for hair cells from juvenile and adult mice, respectively, suggesting much higher overall protein expression levels in juvenile mice.
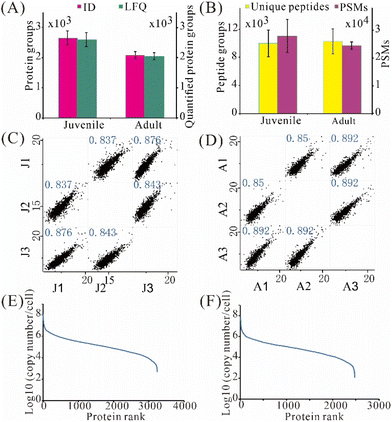 |
| Fig. 5 Proteomic analysis of 500 FACS-sorted inner ear hair cells from juvenile and adult mice. (A) Identified and label-free quantified protein groups. (B) Unique peptides and PSMs. Error bars stand for standard deviations from three independent replicates. (C and D) Pearson correlation of label-free quantified protein groups from juvenile (C) and adult (D) mice. J1–3 and A1–3 represent triplicates of juvenile and adult mice, respectively. (E and F) Dynamic range of protein expression from juvenile (E) and adult (F) mice. | |
Bioinformatic analysis of hair cells from juvenile and adult mice
The differentially expressed proteins (DEPs), defined as those having q-value ≤ 0.05 and fold change ≥ 2, were depicted in a volcano plot (Fig. 6A). Remarkably, 241 DEPs were up-regulated in juvenile mice, while only 59 DEPs were up-regulated in adult mice, suggesting that a more diverse set of genes were expressed in the juvenile period. The top DEPs were Stat1, Ctstm, Bdh2, Prkar2a, Nme2, Hspa12 etc. (Fig. 6A, Table S3†). Importantly, some well-known marker proteins for inner ear development were quantified in our data. Both calbindin and parvalbumin are cytosolic calcium-binding proteins; the immune reactivity of calbindin was reported to increase significantly with aging but no such age-related alterations in parvalbumin were observed.27 Myosin 6 plays an important role in maintaining the hair cell structure integrity and its mutation could cause deafness in humans.28,29 In our data, myosin 6 (Myo6) and parvalbumin (Pvalb) were well-quantified without any missing values, but no significant changes in their expression levels between the juvenile and adult mice were detected. Though not shown as a DEP in the volcano plot, calbindin, a known marker in hair cells, was quantified in two of the three replicates of juvenile mice with CV less than 10%, and was absent in all three replicates of adult mice. Due to the missing values, calbindin did not pass our conservative filter for differential expression, but the data suggests that calbindin may have different levels in juvenile and adult hair cells.
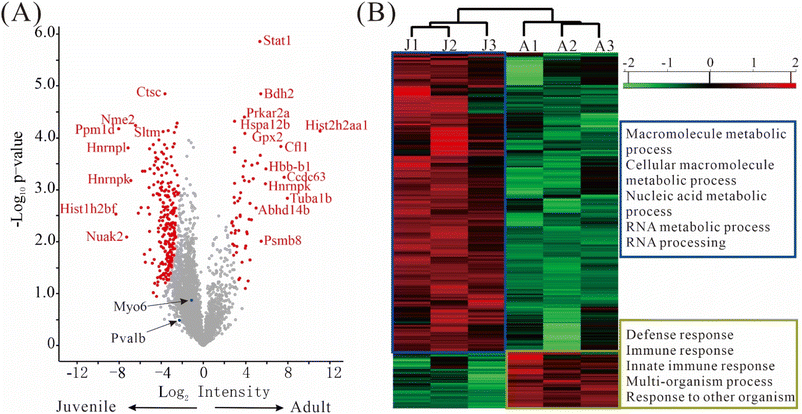 |
| Fig. 6 Bioinformatic analysis of 500 inner ear hair cells from juvenile and adult mice. (A) Volcano plot. (B) Heat map of protein expression. J1–3 and A1–3 stand for independent replicates 1–3 of hair cells from juvenile and adult mice, respectively. | |
Similarly, as shown in the heat map (Fig. 6B, Table S4†), much more protein groups are up-regulated in juvenile mice than in adult mice. According to gene ontology categories of biological processes (GOBP) analysis, the 481 up-regulated protein groups in juvenile mice were highly enriched in processes involved in macromolecule metabolic process, cellular macromolecule metabolic process, nucleic acid metabolic process, RNA metabolic process and RNA processing, whereas the 90 protein groups up-regulated in adult mice were highly enriched in processes involved in defense response, immune response, innate immune response, multi-organism process and response to other organism (Fig. 6B).
Conclusions
This work describes an easy-to-use and scalable device for processing low-input proteomic samples, in which protein preconcentration, reduction, alkylation, digestion, and desalting were fully integrated. The developed 3-frit mixed-mode RCPR can be directly connected online to nanoLC-MS, obviating peptide elution, drying, redissolution, and sample loading steps. Using this device, we could identify on average 2998 and 3934 protein groups, 20 601 and 29 945 peptide groups from 100 and 500 293T cells in DDA mode, respectively. This device was then applied to evaluate the proteomic differences in 500 hair cells between juvenile and adult mice, an example application that demands low-input proteomics. We identified on average 2654 and 2074 protein groups in DDA mode from 500 hair cells of juvenile and adult mice, respectively. These small number of cells can be collected from a single mouse, in contrast to 132 mice were sacrificed in another recent study.15 More than 200 protein groups were up-regulated in juvenile mice, while only 59 protein groups were up-regulated in adult mice. As a possible extension of this work, the sensitivity can be further improved by using narrow-bore nanoLC columns together with lower flow rates. The throughput of our workflow can also be improved by using a multiplexed pressure pump system30 or combining with automation devices like AutoProteome Chip System17 and AssayMAP31etc., since RCPR devices are capillary devices driven by low pressure. In addition, DDA mode can be replaced by data-independent acquisition (DIA) mode to further improve sensitivity and reproducibility.32,33
Author contributions
Yun Yang: methodology, investigation, formal analysis, writing manuscript. Suhong Sun: methodology, investigation. Shunji He: investigation. Chengmin Liu: investigation. Changying Fu: investigation. Min Tang: formal analysis. Chao Liu: supervision. Ying Sun: supervision. Henry Lam: supervision, writing – review & editing. Zhiyong Liu: supervision, writing – review & editing. Ruijun Tian: conceptualization, supervision, writing – review & editing.
Conflicts of interest
There are no conflicts to declare.
Acknowledgements
The authors acknowledge the assistance of Xibi Lu and Lin Lin from SUSTech Core Research Facilities, An He from SUSTech, and Long Wu from HKUST. This study was supported by grants from National Natural Science Foundation of China (31700088 and 81771012), China State Key Basic Research Program Grants (2020YFE0202200 and 2017YFA0103901), Guangdong Provincial Fund for Distinguished Young Scholars (2019B151502050), Shenzhen Innovation of Science and Technology Commission Grant (JCYJ20200109141212325 and JCYJ20170412154126026), SUSTech grant (G02216304/G02216404) and the Research Grants Council of the Hong Kong Special Administrative Region Government (Grant No. 16306417 and R5013-19).
References
- J. R. Wiśniewski, A. Zougman, N. Nagaraj and M. Mann, Nat. Methods, 2009, 6, 359–362 CrossRef.
- N. A. Kulak, G. Pichler, I. Paron, N. Nagaraj and M. Mann, Nat. Methods, 2014, 11, 319–324 CrossRef CAS PubMed.
- C. S. Hughes, S. Foehr, D. A. Garfield, E. E. Furlong, L. M. Steinmetz and J. Krijgsveld, Mol. Syst. Biol., 2014, 10, 1–14 CrossRef CAS PubMed.
- W. Chen, S. Wang, S. Adhikari, Z. Deng, L. Wang, L. Chen, M. Ke, P. Yang and R. Tian, Anal. Chem., 2016, 88, 4864–4871 CrossRef CAS PubMed.
- L. Xue, L. Lin, W. Zhou, W. Chen, J. Tang, X. Sun, P. Huang and R. Tian, J. Chromatogr. A, 2018, 1564, 76–84 CrossRef CAS PubMed.
- Z. Y. Li, M. Huang, X. K. Wang, Y. Zhu, J. S. Li, C. C. L. Wong and Q. Fang, Anal. Chem., 2018, 90, 5430–5438 CrossRef CAS.
- X. Shao, X. Wang, S. Guan, H. Lin, G. Yan, M. Gao, C. Deng and X. Zhang, Anal. Chem., 2018, 90, 14003–14010 CrossRef CAS PubMed.
- Y. Zhu, G. Clair, W. B. Chrisler, Y. Shen, R. Zhao, A. K. Shukla, R. J. Moore, R. S. Misra, G. S. Pryhuber, R. D. Smith, C. Ansong and R. T. Kelly, Angew. Chem., Int. Ed., 2018, 57, 12370–12374 CrossRef CAS.
- M. Dou, G. Clair, C. F. Tsai, K. Xu, W. B. Chrisler, R. L. Sontag, R. Zhao, R. J. Moore, T. Liu, L. Pasa-Tolic, R. D. Smith, T. Shi, J. N. Adkins, W. J. Qian, R. T. Kelly, C. Ansong and Y. Zhu, Anal. Chem., 2019, 91, 13119–13127 CrossRef CAS.
- Y. Cong, Y. Liang, K. Motamedchaboki, R. Huguet, T. Truong, R. Zhao, Y. Shen, D. Lopez-Ferrer, Y. Zhu and R. T. Kelly, Anal. Chem., 2020, 92, 2665–2671 CrossRef CAS.
- R. Wu, S. Xing, M. Badv, T. F. Didar and Y. Lu, Anal. Chem., 2019, 91, 10395–10400 CrossRef CAS PubMed.
- Z. Zhang, K. M. Dubiak, P. W. Huber and N. J. Dovichi, Anal. Chem., 2020, 92, 5554–5560 CrossRef CAS.
- H. Yuan, Z. Dai, X. Zhang, B. Zhao, H. Chu, L. Zhang and Y. Zhang, Sci. China: Chem., 2021, 64, 313–321 CrossRef CAS.
- Y. Liang, H. Acor, M. A. McCown, A. J. Nwosu, H. Boekweg, N. B. Axtell, T. Truong, Y. Cong, S. H. Payne and R. T. Kelly, Anal. Chem., 2021, 93, 1658–1666 CrossRef CAS PubMed.
- A. E. Hickox, A. C. Y. Wong, K. Pak, C. Strojny, M. Ramirez, J. R. Yates, A. F. Ryan and J. N. Savas, J. Neurosci., 2017, 37, 1320–1339 CrossRef CAS.
- Y. Zhu, M. Scheibinger, D. C. Ellwanger, J. F. Krey, D. Choi, R. T. Kelly, S. Heller and P. G. Barr-Gillespie, eLife, 2019, 8, 1–26 Search PubMed.
- X. Lu, Z. Wang, Y. Gao, W. Chen, L. Wang, P. Huang, W. Gao, M. Ke, A. He and R. Tian, Anal. Chem., 2020, 92, 8893–8900 CrossRef CAS PubMed.
- R. Tian, S. Wang, F. Elisma, L. Li, H. Zhou, L. Wang and D. Figeys, Mol. Cell. Proteomics, 2011, 10, 1–10 CrossRef.
- X. Ye, Y. Yang, J. Zhou, L. Xu, L. Wu, P. Huang, C. Feng, P. Ke, A. He, G. Li, Y. Li, Y. Li, H. Lam, X. Zhang and R. Tian, Anal. Chim. Acta, 2021, 1173, 338672–338682 CrossRef CAS PubMed.
- F. Yu, S. E. Haynes, G. C. Teo, D. M. Avtonomov, D. A. Polasky and A. I. Nesvizhskii, Mol. Cell. Proteomics, 2020, 19, 1575–1585 CrossRef CAS.
- H. Chi, C. Liu, H. Yang, W. F. Zeng, L. Wu, W. J. Zhou, R. M. Wang, X. N. Niu, Y. H. Ding, Y. Zhang, Z. W. Wang, Z. L. Chen, R. X. Sun, T. Liu, G. M. Tan, M. Q. Dong, P. Xu, P. H. Zhang and S. M. He, Nat. Biotechnol., 2018, 36, 1059–1066 CrossRef CAS.
- Y. Li, L. Yan, Y. Liu, K. Qian, B. Liu, P. Yang and B. Liu, RSC Adv., 2015, 5, 1331–1342 RSC.
- R. Marie, J. P. Beech, J. Vörös, J. O. Tegenfeldt and F. Höök, Langmuir, 2006, 22, 10103–10108 CrossRef CAS.
- S. F. M. Van Dongen, J. Janvore, S. S. Van Berkel, E. Marie, M. Piel and C. Tribet, Chem. Sci., 2012, 3, 3000–3007 RSC.
- L. M. L. Chow, Y. Tian, T. Weber, M. Corbett, J. Zuo and S. J. Baker, Dev. Dyn., 2006, 235, 2991–2998 CrossRef CAS PubMed.
- N. A. Bermingham, B. A. Hassan, S. D. Price, M. A. Vollrath, N. Ben-Arie, R. A. Eatock, H. J. Bellen, A. Lysakowski and H. Y. Zoghbi, Science, 1999, 284, 1837–1841 CrossRef CAS.
- N. Fischer, L. Johnson Chacko, A. Majerus, T. Potrusil, H. Riechelmann, J. Schmutzhard, A. Schrott-Fischer and R. Glueckert, ORL J Otorhinolaryngol Relat Spec., 2019, 81, 138–154 CrossRef CAS.
- M. J. RÈ©dowicz, J. Muscle Res. Cell Motil., 1999, 20, 241–248 CrossRef PubMed.
- K. B. Avraham, T. Hasson, K. P. Steel, D. M. Kingsley, L. B. Russell, M. S. Mooseker, N. G. Copeland and N. A. Jenkins, Nat. Genet., 1995, 11, 369–375 CrossRef CAS PubMed.
- J. B. Müller-Reif, F. M. Hansen, L. Schweizer, P. V. Treit, P. E. Geyer and M. Mann, Mol. Cell. Proteomics, 2021, 20, 1–9 CrossRef.
- M. Kuras, L. H. Betancourt, M. Rezeli, J. Rodriguez, M. Szasz, Q. Zhou, T. Miliotis, R. Andersson and G. Marko-Varga, J. Proteome Res., 2019, 18, 548–556 CAS.
- F. Meier, A. D. Brunner, M. Frank, A. Ha, I. Bludau, E. Voytik, S. Kaspar-Schoenefeld, M. Lubeck, O. Raether, N. Bache, R. Aebersold, B. C. Collins, H. L. Röst and M. Mann, Nat. Methods, 2020, 17, 1229–1236 CrossRef CAS PubMed.
- A. Brunner, M. Thielert, C. Vasilopoulou, C. Ammar, F. Coscia, A. Mund, O. B. Hoerning, N. Bache, A. Apalategui, M. Lubeck, S. Richter, D. S. Fischer, O. Raether, M. A. Park, F. Meier, F. J. Theis and M. Mann, Mol. Syst. Biol., 2022, 18, e10798 CrossRef CAS PubMed.
|
This journal is © The Royal Society of Chemistry 2023 |
Click here to see how this site uses Cookies. View our privacy policy here.