DOI:
10.1039/D1SM01456K
(Paper)
Soft Matter, 2022,
18, 1071-1081
Defect-controlled softness, diffusive permeability, and mesh-topology of metallo-supramolecular hydrogels†
Received
9th October 2021
, Accepted 5th December 2021
First published on 14th January 2022
Abstract
Hydrogels are polymer networks swollen in water; they are suitable materials for biomedical applications such as tissue engineering and drug delivery. In the latter, the controlled diffusion of small diffusants inside the network is essential, as it determines the release mechanism of the drug. In general, the diffusion inside a polymer network is controlled by its mesh-size. Here, we actively control the diffusivity and also the softness of metallo-supramolecular hydrogels via the network mesh-topology by introducing connectivity defects. A model polymer network is realized based on a 4-arm poly(ethylene glycol) (pEG) where each arm is capped with terpyridine moieties that are capable of forming metallo-supramolecular complexes with zinc ions. In this model network, we insert 8-arm pEG macromolecules that are functionalized with terpyridine at different ratios to create connectivity defects. With an increasing amount of 8-arm pEG, the polymer network forms more loops, as quantified by double quantum-NMR. This doped network shows an enhanced self-diffusivity of the building block molecules within the network, as examined by fluorescence recovery after photobleaching, and a higher softness, as investigated by oscillatory shear rheology. With these findings, we show that it is possible to tune the diffusivity and softness of hydrogels with defects in a rational fashion.
Introduction
Defect engineering in soft matter is a wide field of research that allows for tuning material properties on multiple levels.1 In one branch of that discipline, structural inhomogeneities affect the properties of polymer-network gels, specifically the local and global elasticity,2 fracture mechanism,3 and the diffusive permeability of guest substances,4 and since these properties lay the ground for the main applications of gels, the impact and ability of controlling such inhomogeneities is crucial. Prominent examples for inhomogeneities are found in hydrogels, which are three-dimensional polymer networks swollen in water.5–7 These polymer networks can be formed by connection of polymer strands either by supramolecular or covalent crosslinks. While covalent networks are stable in form and shape, supramolecular networks have the great advantage of being dynamic which means being responsive and recyclable. As a consequence, also the heterogeneities of covalent networks are frozen in time and space, whereas those of supramolecular networks follow spatiotemporal evolutions. The study and modelling of the extent, effect, and evolution of heterogeneities and defects in supramolecular networks require a model platform. One such platform is model transient networks formed by supramolecular crosslinking of multi-arm star-like polymer precursors. In such a network, the network strands denote the chain sections between branching points of two adjacent polymer precursors, and their length determines the network mesh-size ξ, as illustrated in Fig. 1. During network formation, different types of defects can form. Uneven distribution of the network crosslinks causes global spatial heterogeneities of some tens to hundreds of nanometers in space, and these defects are further pronounced when the polymer network is swollen.8
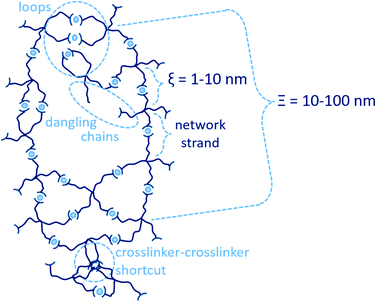 |
| Fig. 1 Defects in polymer networks created by interconnection of tetra-arm star supramolecular precursors on different length scales. Local connectivity defects on the order of few nanometers form due to misconnectivities in the network. Global defects on the order of 10–100 nm result due to the inhomogeneous distribution of crosslinking density. | |
Furthermore, irregularity in the local crosslinking-junction functionality results in local connectivity defects, which are in the magnitude of 1–10 nm, as shown in Fig. 1.7,9 These connectivity defects are loops, dangling chains, and crosslinker–crosslinker shortcuts. A loop is formed when several (usually two) arms of the same polymer form intra-molecular connections, or when two adjacent polymers form multiple inter-molecular connections, resulting in higher order loops.10 A dangling chain is an arm that does not form any connections with another arm. A crosslinker-crosslinker shortcut is formed when two crosslinks interact with each other in form of uncontrolled clusters with varying number of junction functionalities. In view of this picture, we define connectivity as the number of potential inter-molecular connections that a branched polymer macromolecule can form with its arms. Thus, the expected connectivity in a perfectly regular network consisting of only tetra-arm shaped telechelic polymers is four.
For rational material design, it is essential to understand how these different types of defects affect the properties of the hydrogel, such as its viscoelasticity and permeability, to optimize their use in applications. As hydrogels are constituted mainly by water, they are suitable for biomedical applications, such for example in tissue engineering or drug delivery.11–13 However, to be used in these applications, they need to meet further requirements. In tissue engineering, for example, they need to resemble the mechanical properties of original natural tissues, and this can be challenging,14,15 as the stiffness of living tissues can vary from a few Pascals to giga-Pascals, and it is even more challenging when considering the diverse functions of tissues.11 In drug delivery, by contrast, not only the stiffness or softness of the tissue is important, but also the diffusive permeability, which is governed by the mesh size of the hydrogel.16 If the drug is smaller, similar, or larger than the network mesh-size, then the diffusion would be fast, slow, or even hindered, respectively.16 Therefore, the control of the diffusion kinetics inside the hydrogel through engineering the mesh-size is crucial for its applications.
There are several approaches to engineer the mesh-size and its distribution in hydrogels. One way is to use precursors of different molecular weights, as suggested by Sakai et al.17 These workers have systematically varied the heterogeneous distribution of the mesh-size in covalent model-network hydrogels formed by tetra-poly(ethylene glycol) (tetra-pEG), by employing precursors of two different molecular weights.17 Despite they did not characterize the heterogeneities on the nanoscale, their macroscopic findings suggest that these bimodal networks have properties of a hydrogel having a strand size in between the arm length of the two precursors.17
Another approach to control the diffusion inside a hydrogel is to add dangling chains to the main network. With this strategy, Grunlan et al. controlled the mesh-size of a hydrogel with the introduction of thermoresponsive and charged dangling chains.18 Their findings show that with negatively charged chains, smaller meshes are possible.18 In an own previous work, we introduced poly(N-isopropylacrylamide) (pNIPAAm) dangling chains into a supramolecular polymer network and were able to similarly hinder the diffusion of small probes upon switching temperature above the lower critical solution temperature of pNIPAAm.5 Similarly, we investigated how the network dynamics of gels, constituted by chains carrying sticky side-groups, changes with the introduction of sticky tracers having the same or lower connectivity than the network itself, thereby creating local connectivity defects.4 Our findings show that the chain dynamics is enhanced in case of the network with connectivity defects, meaning that the probe diffuses faster than expected.4 The effect of defects on mechanical properties has also been studied by means of simulations. For example, Shibayama et al. have introduced random defects in tetra-pEG networks and investigated the influence of the structural inhomogeneity on the mechanical properties.19 Their findings show that with an increasing defect fraction, the Young modulus of the tetra-pEG networks decreased accordingly.19
Targeted mesh-size variations or intentional incorporation of defects in polymer networks allow for a systematic investigation of their impact on the final material properties such as permeability or viscoelasticity. A recent review on defect engineering in soft matter highlights the utmost importance of structure control to implement a novel type of rational material design based on these aforementioned minor structural deviations.1
With this work, we aim to contribute to this emerging field of soft matter material design by presenting how to tailor supramolecular hydrogels in a systematic way via connectivity mismatches. We achieve that by systematically introducing different percentages of connectivity defects in a model supramolecular network and investigating the resulting macroscopic elastic response by oscillatory shear rheology, the microscopic self-diffusivity by fluorescence recovery after photobleaching (FRAP), and characterizing and proving the specific type of defects by double-quantum NMR (DQ-NMR).
Our material basis is composed of a supramolecular pEG hydrogel, where each arm of a star-shaped precursor is functionalized with a terpyridine unit that is capable of forming transient bis-complexes with divalent zinc ions. Starting from a regular network that only consists of 4-arm pEG-terpyridine macro-precursors (left network in Fig. 2), we successively replace a certain percentage of 4-arm pEG building blocks by 8-arm pEG-terpyridine polymers. To ensure a constant total terpyridine concentration, coming along with a constant number of arms, we select the molar mass of the 8-arm pEG to be a twofold of that of a 4-arm pEG. In other words, replacement of two macromolecules with four arms by one macromolecule with eight arms yields the same number of overall terpyridine units but a different degree of connectivity, keeping the same number of network strands per total volume constant (Fig. 2). With increasing amount of connectivity defects, the hydrogel becomes softer and the self-diffusivity inside increases, as demonstrated by complementary oscillatory shear rheology and FRAP experiments. These findings are associated with the emergence of various defect types, which are quantified by DQ-NMR. Our results suggest a new paradigm for tuning properties of supramolecular hydrogels in a rational manner that might be transferable to other transient systems.
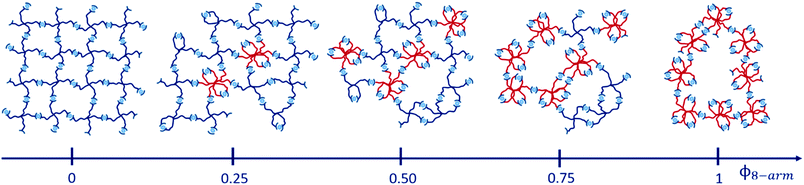 |
| Fig. 2 Concept of this work. Metallo-supramolecular polymer-network gels composed of 4-arm pEG-terpyridine (Mw = 20 kg mol−1) and 8-arm pEG-terpyridine (Mw = 40 kg mol−1), combined in different ratios, thereby increasing the amount of connectivity defects in a controlled way. The impact of connectivity defects on macroscopic elastic and microscopic permeability properties are then investigated. | |
Experimental
Sample syntheses
The list of raw materials used to prepare the polymer samples of this work and the synthesis procedure can be found in the ESI.†
In short, the hydroxyl termini of commercially available 4-arm 20 K and 8-arm 40 K pEG polymers were converted to terpyridine units by a Williamson type ether synthesis (Fig. SI1, ESI†).
Methods
Oscillatory shear rheology
To prove the effect of defects on the elastic properties of the supramolecular hydrogels, oscillatory shear rheology experiments were performed. For this purpose, stock solutions of 4-arm 20 K and 8-arm 40 K pEG-terpyridine with a concentration of 70 g L−1 were prepared by dissolving the appropriate amount of each polymer in Milli-Q water. This concentration was chosen to obtain gels in a regime well above the overlap concentration of a 4-arm 20 K pEG-terpyridine (c* = 35 g L−1).20 Another aqueous stock solution of zinc nitrate hexahydrate was prepared and a ratio of terpyridine to metal of 2
:
1 was ensured. In a glass vial, the polymer solution was inserted and then subsequently, the metal ion solution was added quickly. For hydrogels consisting of two precursor polymers (4-arm 20 K and 8-arm 40 K), both polymer stock solutions were mixed in a glass vial before adding the metal ion stock solution. All mixtures were then vigorously vortexed until complete gel formation. In total, five samples were prepared and characterized, with 8-arm pEG-terpyridine volume fractions Φ8-arm being respectively 0, 0.25, 0.5, 0.75 and 1. Finally, the gels were allowed to equilibrate overnight on a plate shaker at room temperature, to remove all air bubbles.
Oscillatory shear rheology experiments were carried out on an Anton Paar rheometer of the type MCR 302 (Anton Paar, Graz, Austria), equipped with a solvent trap to avoid dehydration and a parallel plate geometry PP-25. After equilibration, amplitude sweeps were recorded in order to determine the linear viscoelastic regime. These were done at a temperature of 25 °C, a constant shear frequency of ω = 1 rad s−1 and an increasing amplitude of γ = 0.1–100%. Afterwards, the sample was allowed to equilibrate again and then frequency sweeps were obtained at 25 °C, at a constant shear rate of γ = 0.1% and a frequency range of ω = 0.01–100 rad s−1.
Fluorescence recovery after photobleaching
Fluorescence recovery after photobleaching was chosen as a method to microscopically investigate the self-diffusivities of 4-arm pEG-terpyridine macromolecules within the hydrogels depending on the amount of added 8-arm pEG-terpyridine. For this purpose, 3 wt% of a dye-labelled pEG moiety that contains both required functional groups on each arm, a terpyridine unit and a fluorescent NBD group, was integrated into the gels. These polymer tracers were synthesized according to a previously described procedure21 and are visualized in Fig. SI4 (ESI†).
The FRAP experiments were carried out on a Leica TCS-SP8 AOBS SMD confocal laser scanning microscope, equipped with a 20× immersion objective (HC PL APO CS2 20×/0.75 IMM, numerical aperture: 0.75) and a PMT detector. Aqueous stock solutions of the 4-arm pEG-terpyridine and the 8-arm pEG-terpyridine (c = 118.5 g L−1, respectively) were prepared, as well as of the NBD-tagged fluorescent 4-arm pEG-terpyridine (c = 14.7 g L−1) and the zinc nitrate hexahydrate (c = 7.28 g L−1). Final gels were prepared by mixing the polymer stock solutions (40 μL total volume: 0%; 25%, 38%, 50%, 63%, 75%, and 100% 8-arm content), adding 10 μL of the dye-labelled polymer stock solution, followed by the addition of 20 μL zinc nitrate solution and subsequent vortexing. With this procedure, the desired stoichiometric ratio of terpyridine to zinc of 2
:
1 was obtained, as well as the overall polymer concentration of 70 g L−1. Due to the twofold molar mass of the 8-arm pEG-terpyridine compared to the 4-arm pEG-terpyridine, the number of terpyridine stickers remains constant in all networks while a sole variation in the overall connectivity is achieved. Samples were gently shaken over night at 39 °C to reach full equilibration. After cooling to room temperature for two hours, the gels were loaded into an eight-well plate (Ibidi®) and sealed to avoid dehydration during the scans. Prior to each measurement, a set of four pre-bleach images is recorded. Permanent irradiation of the fluorophores and therefore creation of a bleached point pattern was achieved by applying a full-power laser beam (10 mW) with a duration of 1 s. A weak reading beam with a wavelength of 488 nm (maximum excitation wavelength of the NBD-dye) was used to excite the dye molecules during the scan series. For the recovery process, it is important to use a remarkable attenuated laser beam to avoid re-bleaching of the region of interest. A series of 250 images was recorded with time intervals of 0.173 s between each scan (bidirectional scan frequency: 400 Hz) to capture the re-emerging of fluorescing macromolecules into the bleached area. Analogous to previous works,4,22 the FRAP data were analysed using a diffusion model that is based on fitting Gaussian shaped intensity profiles I(r, t) to the time-depending disappearance of the initial bleached spot.23,24 The bleached area vanishes due to the exchange and diffusive motion of the dye-labelled supramolecular polymers throughout the system until its pre-bleached fluorescence intensity is fully recovered.
1H DQ-NMR
Low-field NMR experiments were performed on a Bruker MiniSpec mq20 with a magnetic field of B0 = 0.47 T. The pulse lengths were between 1.5 and 1.7 μs for 90° pulses and between 3.5 and 4.0 μs for 180° pulses. A BVT3000 temperature unit was used to keep the samples at a stable temperature of 30 °C ± 1 °C. Samples were synthesized in 10 mm diameter glass tubes according to the synthesis protocol presented in the rheology section and flame-sealed at about 5 cm height to prevent solvent evaporation during the experiments. For the DQ experiments, the Baum–Pines sequence25 with incremented delays between the pulses and a constant number of two cycles was used. The recycle delay was set to 1.5 s, which is enough to filter out about 90% of the HDO signal (estimating an average longitudinal relaxation time of about T1,HDO = 12 s), while retaining >95% of the polymer signal (estimation T1,poly = 0.5 s). This was validated by the first DQ-measurement of the sample consisting of only 4-arm stars (Φ8-arm = 0) (see DQ-NMR results), where a combined fraction of defects and solvent signal of less than 1% was measured. Therefore, we refrain from the commonly used procedure of quantifying the leftover HDO component after the T1-filtering by exploiting the large difference in T1 relaxation times in a saturation recovery experiment, because such a small contribution will be shown to be negligible and the introduced uncertainty significantly larger than the obtained value.
In the following, a concise overview of the obtained data using the DQ experiment and a reasoning for the data treatment is provided. For further information on the derivation and meaning of the measured quantities, the reader is referred to ref. 10. After application of the Baum–Pines sequence, two phase cycle-controlled signals are obtained: the first one is the DQ build-up function IDQ(tDQ), which contains structural information about the sample in the form of residual dipolar coupling (RDC) values and its quantitative distribution. However, it is also modulated by transverse relaxation of the total NMR signal, so the RDC is not easily accessible using only a simple fitting procedure to the IDQ(tDQ) curve. Thus, a second signal function, sometimes also called reference function Iref(tDQ) is needed for independent quantification of the relaxational contribution to the signal. Using a specific phase cycle described in ref. 26, the shape of this signal will only depend on the transverse relaxation times being present in the sample. As shown and already applied on, e.g., the tetra-pEG system of Sakai et al.27 and another terpyridine-based tetra-pEG hydrogel,28 the following fitting functions (1)–(3) are used for the two signals:
| 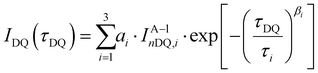 | (1) |
and
| 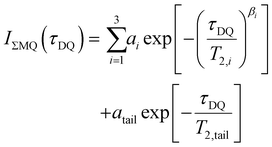 | (2) |
here
InDQ combines the Abragham–Like (A–l)-function that is defined as
|  | (3) |
that was firstly derived in ref.
29. Both functions are fitted simultaneously to the
IΣMQ and
IDQ data, with shared parameters for the relaxation part and the respective fractions
ai. In all known cases with tetra-pEG-based systems, including this work, a minimum of 3 A–I functions is needed to sufficiently fit the curves. Each extracted component
ai corresponds to a certain species with molecular mobility and therefore to a certain type of connectivity motif in the network. A fourth, purely exponential function that is exclusive to
Iref, is used for characterising sample fractions with unhindered, isotropic motion (
e.g., protonated solvent, sol, dangling chains), which reflects in the long tail of the relaxation function, while not contributing to the DQ function. In contrast to ref.
27 we refrain from explicitly assigning all components
ai to certain connectivities as, admittedly, the simply picture of three easily distinguishable types of connectivities is certainly a simplification in such a complex system. Instead, we only assign the component
a1 to the single link, as the single link can safely be assigned to the component with the highest RDC using the proportionality
Dres ∼
Mc−1 and the simple argument of an increased, apparent molecular weight of the crosslinks for higher-order connectivities. Analysis of the data shows that a two-component model is not sufficient, thus we still use a 3-component model as the model with the least possible parameters, and therefore, we present results in a 3-parted fashion, however, we suggest to only interpret the sum of the higher order connectivity defects
a2 +
a3 or the ratio between the ideal connectivities
a1 and the sum
a2 +
a3. This is especially important in the case of samples containing 8-arm stars, as here even more possible connections can be formed which, however, cannot be extracted with our approach due to the strongly increasing number of fitting parameters and the increasing fitting ambiguity.
Results and discussion
Our material basis for systematically studying the effects of connectivity defects on the elastic response and diffusive permeability of supramolecular model hydrogels is composed of 4-arm and 8-arm pEG where each arm was end-capped with terpyridine units that are capable of forming bis-complexes with zinc ions. To ensure the same number of terpyridine stickers in each sample, the 8-arm pEG was chosen with a twofold molar mass compared to the 4-arm pEG, therefore also ensuring the same arm length. To increase the amount of connectivity defects, 8-arm pEG-terpyridine was introduced into the 4-arm model pEG-terpyridine system in different volume fractions Φ8-arm. To investigate the influence of connectivity defects on the properties of supramolecular hydrogels, the macroscopic elastic response of the gels was characterized by oscillatory shear rheology whereas the microscopical diffusive permeability was characterized by FRAP measurements. Finally, the defects were quantified nanoscopically by DQ-NMR. The combination of these three characterization techniques allows us to obtain information not only on the relaxation dynamics, but also on the structural properties of the system at multiple length-scales.
Oscillatory shear rheology
The influence of connectivity defects on the macroscopic elastic response of a hydrogel was probed through oscillatory shear rheology experiments. Starting from a network purely composed of 4-arm pEG-terpyridine macromolecules, we successfully replaced different amounts of 4-arm pEG-terpyridine by 8-arm 40 K polymers, thereby keeping the number of sticky terpyridine units constant, and an overall polymer concentration of 70 g L−1. The volume fraction of 8-arm pEG-terpyridine Φ8-arm is then respectively 0, 0.25, 0.5, 0.75 and 1. Frequency-dependent dynamic moduli were investigated at 25 °C. The frequency-dependent dynamic moduli of all networks can be described by a single Maxwell element, as demonstrated in Fig. 3(A) by the dashed lines. However, with increasing Φ8-arm, a slight deviation in the moduli at high and low frequencies becomes visible. Such a deviation from the Maxwell model might be an indication of multiple relaxation times.30 These relaxation times can derive from the relaxation of higher order loops, where two polymer stars share more than one connection or a bigger loop results by connecting several stars in circle. The measured plateau moduli depending on the volume fraction of 8-arm 40 K polymers is shown in Fig. 3(B). The pure 4-arm 20 K sample exhibits the highest elastic modulus corresponding to GP,4-arm 20
K = 13 kPa, whereas the lowest elastic modulus is obtained in the pure 8-arm 40 K gel, corresponding to GP,8-arm 40 K = 1 kPa. The plateau storage moduli of the samples at intermediate compositions systematically decrease with increasing the volume fraction Φ8-arm. Based on the affine network model theory, we expected all samples to show the same plateau modulus, as the number of network strands per volume (corresponding to ν) is kept constant in all gels. In this model, the plateau modulus G0N is given bywhere ν is the number of network strands per volume, R is the universal gas constant, and T denotes the temperature. ν can be calculated from the molar concentration of pEG precursors and it corresponds to |  | (5) |
with f the functionality of the precursor and μ the density of network crosslinks.31 Hence, the theoretical plateau modulus for both pure 8-arm 40 K and 4-arm 20 K networks is calculated to be roughly G0N = 17 kPa. This value is valid for a fully percolated and defect-free theoretical polymer network. However, experimental polymer networks always show intrinsic topological defects that determine a deviation from the theoretical value.31 In this context, the measured plateau modulus of the pure 4-arm network (GP,4-arm 20
K = 13 kPa) is not significantly lower than the calculated theoretical one. Since this is the system with the highest modulus, it will be further taken as reference when compared to the other samples. If the plateau modulus is considered as a quantification of the elastically active network strands,5 the continuous decrease of Gp depending on an increasing amount of 8-arm in the networks, indicates a decreasing degree of network connectivity. As the matter of fact, with a higher density of arms per each molecule, the 8-arm is more likely to form intra-molecular instead of inter-molecular associations.32 Alternative to the affine model, one could use the phantom model, which would consider the functionality of the stars instead of the total number of strands. In this case, the plateau modulus of the 8-arm pEG is predicted to be higher than that of the 4-arm pEG. In addition, the phantom model would need to define a functionality for the mixed system, which has not been taken into account so far. Up to now, it is still a matter of debate which of both models is more suitable to describe the rheological spectrum of supramolecular polymer networks, whereas a concentration-dependent transition between the two has been found for chemically crosslinked tetra-arm pEG.33 The concentration used in this study (2c*), would fall at the boundary between these two models. However, the initial hypotheses of our studies are in line with the affine model, since we designed our study such to have the same number of network strands in each sample.
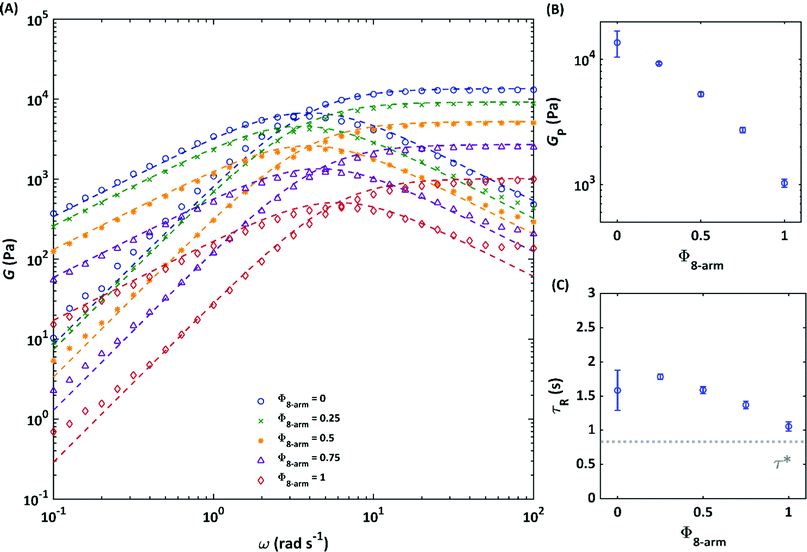 |
| Fig. 3 Frequency-dependent elastic and loss modulus of the hydrogels composed by different ratios of 4-arm pEG-terpyridine (Mw = 20 kg mol−1) and 8-arm pEG-terpyridine (Mw = 40 kg mol−1) with zinc in water at a total polymer concentration of 70 g L−1 and a temperature of 25 °C, assuring the same terpyridine concentration in all gels. Dashed lines represent fits to the Maxwell model (A). Influence of the 8-arm pEG-terpyridine proportion on the plateau modulus Gp (B) and on the relaxation time τR (cross-over frequency of G′ and G′′) (C). The lifetime τ* of an isolated zinc-terpyridine complex (taken from ref. 37) in dilute aqueous conditions is depicted as dotted grey line. The error bars depict the fit parameters of the Maxwell model within a confidence interval of 95%. The pure 4-arm sample is an outlier. | |
The fact that the 8-arm 40 K pEG network exhibits a lower plateau modulus can be explained by connectivity defects occurring due to a higher local density of arms. With such overcrowding of arms, it is more likely that two neighbouring arms of the same macromolecule find each other thereby creating loops of different orders. These loops cause a loss in modulus, because they do not actively store energy. Therefore, going from 100% 4-arm pEG-terpyridine (Φ8-arm = 0) to 100% 8-arm pEG-terpyridine (Φ8-arm = 1), the network becomes locally more heterogeneous.
In addition to the elastic modulus, the terminal relaxation times (inverse of the crossover frequency of G′ and G′′) of the networks slightly decrease with increasing Φ8-arm (Fig. 3(C)). This can be explained by the fact that the 8-arm pEG has a higher functionality and therefore each terpyridine exchanges faster because it is easier to find another free ligand of another 8-arm polymer in close proximity. Even though, for crowded systems there is usually a prolongation of the effective lifetime of the bonds due to the constraint of other chains, this usually occurs in the melt state or at high concentrated regime, while we are still in the semi-dilute regime. In addition, it was already observed in other pEG-terpyridine telechelic systems that the dynamic of the complex in the polymer in enhanced when compared to the one of the terpyridine and metal complex alone.34 This is in line with previous observations for associative networks with cluster forming end groups, for which it was shown that terminal flow is indeed mediated by the relaxation of single chains.35,36 Moreover, as it can be seen from Fig. 3(C), with increasing Φ8-arm, the dissociation times of the gels approaches the dissociation time τ* of an isolated zinc-terpyridine complex in dilute conditions (dotted grey line), taken from ref. 37 as 1/kdiss. The macroscopic network relaxation time measured by rheology is influenced by the complex dissociation time, as the applied shear stress is released by chain motion and subsequent breaking of the transient crosslink. However, a direct comparison between both times should be considered carefully. First, the lifetime of the isolated complex has been determined in aqueous dilute conditions whereas our network relaxation time is obtained in a semi-dilute regime of 2c*. An earlier work of Olsen et al.38 showed by forced Rayleigh experiments (FRS) that the complex dissociation time is also strongly influenced by the crowded environment of a network and can therefore deviate from the value obtained in dilute un-percolated conditions. Second, Wilkins et al.37 used pure terpyridine (2,6-bis(2-pyridyl)pyridine) for their metal exchange studies whereas in our case, the terpyridine unit is attached to a polymer chain via oxygen atom that influences the electronic properties of the aromatic pyridiyl-ring and therefore also the complex dissociation time. With increasing 8-arm (40 K) content, the macroscopic network relaxation time approaches the dissociation time in dilute conditions. In all other networks, the influence of the bond lifetime renormalization (meaning that the complex opens and closes several times before it actually releases the applied shear stress) becomes pronounced. This bond lifetime renormalization vanishes with increased defect fraction.
We further estimate the network mesh size ξ from the elastic modulus by
|  | (6) |
with
R the universal gas constant,
T the temperature,
Gp the plateau modulus, and
NA the Avogadro number.
39 According to these calculations, we find an increase in the mesh size with increasing
Φ8-arm in the network (
Fig. 4(A)). We suggest the 8-arm pEG molecules to significantly contribute to the formation of loops and dangling ends, leading to an increase of the average network mesh-size. The mesh-size in a pure 8-arm pEG-terpyridine network is found to be approximately three times larger than the one of the pure 4-arm pEG-terpyridine, suggesting that bigger meshes are formed as a combination of domains with a lower local density of polymers and domains with a higher local density of polymers. A schematic visualization of these higher order loops is shown in
Fig. 4, where
Fig. 4(B) represents the pure 4-arm 20 K network and
Fig. 4(C) depicts a network with included connectivity defects (pure 8-arm pEG). It is shown that with increasing number of intra-molecular connections (loop formation), the average mesh-size also increases. To prove our hypothesis on the micro- and nano-scale, we further study the diffusive permeability of the networks by FRAP, and the structural properties by DQ-NMR, as follows.
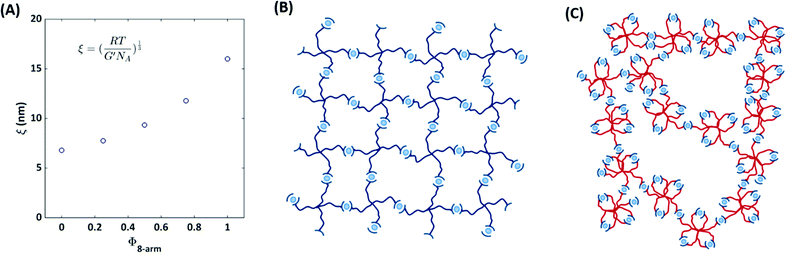 |
| Fig. 4 Mesh-size of the network in function of the fraction of 8-arm pEG in the system (A). Ideal 4-arm 20 K network with homogeneous mesh-size distribution (B) and pure 8-arm 40 K network that includes connectivity defects (C). The formation of intra-molecular loops has the consequence to increase the average mesh-size of the network by forming higher order loops. | |
Fluorescence recovery after photobleaching
To microscopically investigate the influence of connectivity defects on the network structure and the resulting diffusive permeability properties, FRAP measurements were conducted.
For this purpose, a small fraction of a 4-arm polymer 20 K (3 wt%) with fluorescing NBD groups attached to each arm in addition to the sticky terpyridine group was integrated into the 4-arm/8-arm matrix gels. Based on their structural similarity, it was possible to track the intrinsic motion of the 4-arm polymer building blocks within the networks without the application of any external shear forces such as those used during rheology experiments. The self-diffusion coefficients of the investigated gels versus Φ8-arm, are shown in Fig. 5.
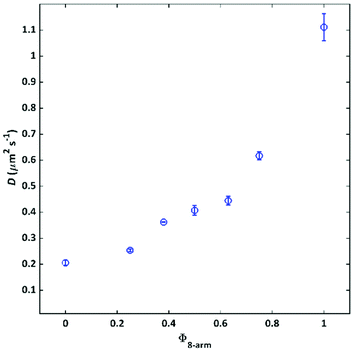 |
| Fig. 5 Self-diffusion coefficients of a 4-arm fluorescently dye labelled pEG-terpyridine within the networks consisting of different ratios of 4-arm and 8-arm pEG-terpyridine (ctotal = 70 g L−1), obtained by FRAP. Errorbars represent standard deviations of measurements at three randomly chosen gel positions. | |
As a general trend, the diffusion coefficient increases by a factor of 5 from 0.21 μm2 s−1 to 1.1 μm2 s−1 between the two composition extremes pure 4-arm 20 K and pure 8-arm 40 K. This observation is in line with the rheology results, where less elastically active strands were formed upon increasing Φ8-arm. Although all networks contain the same number of terpyridine stickers and therefore theoretically the same probability for a tracer molecule to attach to the network, the diffusivity in a pure 8-arm network is significantly enhanced.
The fact that the diffusion coefficient increases with Φ8-arm, could be rationalized considering that with misconnectivities, the diffusant travels faster without actually forming transient bonds, because the next available terpyridine is farther away. This vision is coherent also with the increasing mesh-sizes calculated through rheology. The trend of the diffusion coefficient follows also the trend of the relaxation time calculated through rheology. As, a faster exchange is strictly connected to a faster diffusion.
1H DQ-NMR
To directly evaluate the contribution of 8-arm stars to the formation of local defects and the overall connectivity of the polymer chains in the system, we apply static time-domain 1H DQ-NMR, as described in the experimental part, on a series of networks prepared with different ratios of 4-arm pEG-terpyridine stars and 8-arm pEG-terpyridine stars (see Fig. 6(A) for the normalised experimental data). Starting with a pure tetra-pEG system (4-arm 20 K) transiently linked by terpyridine-zinc complexes, two different fitting approaches for the analysis of the DQ data were tried: A two-component approach assuming that two main connectivities in the network are present, being the single link and one type of connectivity defect respectively, and a three-component approach, assuming one additional type of misconnectivity with non-vanishing anisotropic constraints. Although previous experiments on tetra-pEG systems have already shown the necessity of three distinct components in the fitting model, the two-component approach was still tried for the sake of completeness (see Fig. 6(B)). It was again shown that the two-component model (although suggested by a first glance at the data) was not sufficient and therefore one additional fit component was introduced. Again, we want to stress the ambiguity of the 3-component model that was already mentioned in the Experimental section, meaning that in the final discussion one should not treat a2 and a3 as different components, but rather as a combined fraction of higher-order connectivities. With this in mind, we extracted three different types of connectivities, namely the single link and at least two unassigned types of connectivity defects, as well as the fraction of isotropic defects.
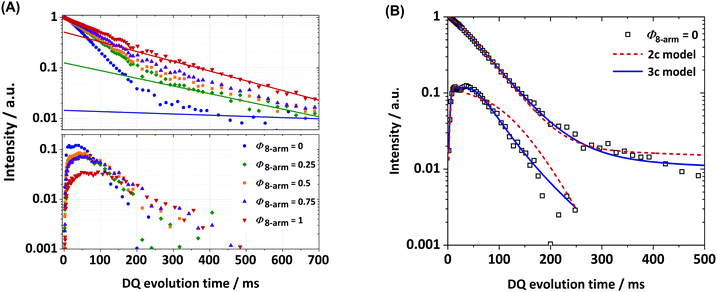 |
| Fig. 6 (A) Overview of the measured DQ data with a shared x-axis but different log10-y-axes. Top: IΣQM curves with exemplary tail fits (fit extrapolated to tDQ = 0) for Φ8-arm = 0, 0.5, 1, respectively. Bottom: Corresponding IDQ. The steadily decreasing intensity is caused by the increase in the defects (‘long tail’) in the IΣMQ data. (B) Exemplary data fit for Φ8-arm = 0. The fit model with 2-components (single links +1 connectivity defect) does not replicate the measured data, whereas the 3-component model does sufficiently fit all maxima. | |
Firstly, we analyse the amount of isotropic material being present in a sample by quantifying the tail fraction in the relaxation signal of the DQ experiment (see Fig. 6(A) for exemplary tail fits). As already mentioned, this is a quantitative sum of protonated solvent and actual isotropic polymer material (defects), where the former constitutes only a small contribution due to the choice of a rather short recycle delay of 1.5 s. Additionally, all samples were prepared by the same preparation protocol and have the same polymer concentration and roughly the same overall volume. Therefore, we reasonably assume a comparable fraction of protonated D2O for all samples, being at maximum 1%, as the total isotropic fraction (solvent + defects) in the DQ experiment for the pure 4-arm star system is only 1%. Across the sample series there is a trend of an increasing defect fraction from 1% to 51% with increasing number of 8-arm stars, which we mostly attribute to a steadily increasing number of loops in the 8-arm stars as it can be seen in Fig. 7(A).
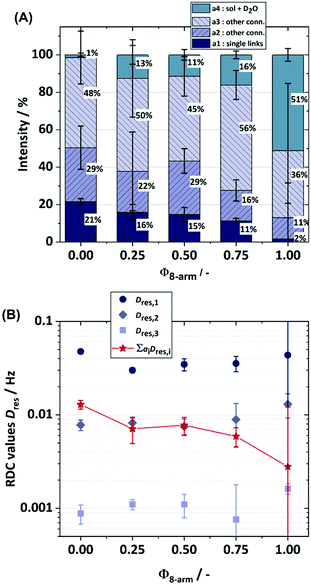 |
| Fig. 7 (A) The fractions for each type of connectivities a1–3 and atail as extracted from the fitting procedure are plotted vs. the respective samples. Here, Φ8-arm indicates the volume fraction of 40 K 8-arm pEG. A clear relationship between defects and content of 40 K 8-arm stars is found. (B) The corresponding residual dipolar coupling (RDC) values. Within the error bars, no significant change was observed, although the dilution effect induced by the 8-arm stars becomes visible when the weighted RDC is calculated (red star-shaped symbols; connection line as guide for the eye). | |
This conclusion is supported by the analysis of the corresponding RDC values. As described in the advised literature,10 the obtained RDC values are an abstract measure of the bulk-averaged anisotropy of motion of the backbone segments under the assumption of fast orientational averaging of the segmental motion (T ≫ TG). Therefore, one can assume that in a network with an increasing and equally distributed number of defects, the RDC values must decrease, as these increase the effective mesh size of the network. With the introduction of the 8-arm stars, as it can be seen in Fig. 7(B), a weak but significant drop of the RDC value of the a1 component (the single links) from 47 Hz (Φ8-arm = 0) to 30 Hz (Φ8-arm = 0.25) can be seen, even though in an ideal network the coupling must slightly increase given the fact that the RDCs are scaling with the phantom factor (f − 2)/f,40 where f is the star functionality, and we now introduced a component with f = 8. We attribute this effect to the increase in the defects from 1% to 13%, having, as mentioned above, a strong influence on the overall network elasticity.41 An interesting behaviour can be found by looking now at the rest of the sample series with even more 8-arm star content. With increasing Φ8-arm we now assume even further decreasing RDCs for the single links, while defects increase strongly up to 51%. One would expect a vanishing RDC close to 0, as the network is now theoretically transitioning into a liquid (assuming a liquid-gel transition at 50% successful connections between the precursors).
Instead, the RDC value is not changing within the error bars, thus we necessarily must assume that not all defects are equally distributed between the network chains. Given the strong influence of loops on the RDCs, we assume that a majority of the introduced 8-arm stars are creating intra-molecular associations, therefore not or only barely contributing to the network. Thus, with increasing content of 8-arm stars, mostly a dilution effect is observed, which is also reflected in the rheology measurements, especially in the decreasing plateau modulus and increasing network mesh size. A comparison of RDC values and plateau moduli can be made by calculating the averaged RDC of the sample (weighted by the respective fraction) without further normalisation by the respective defect fraction (because the modulus also accounts for the dilution effect of the defects), as it is proportional to the molar concentration of elastic chains. The comparison can be found in Fig. 8 and both curves show qualitatively the same behaviour, although the data did not overlap within the resulting error bars for the averaged RDC (under the assumption of Gaussian error propagation for ai and Dres,i). The quantitative mismatch can mainly be attributed to the complexity of the investigated system.
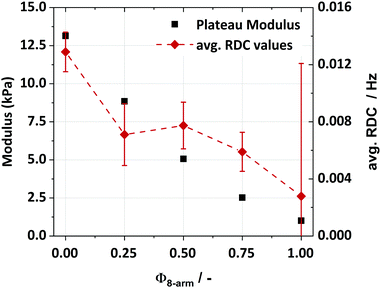 |
| Fig. 8 On the left axis, the plateau moduli from rheology are shown and plotted against the volume fraction of 8-arm stars. On the right y-axis, the averaged residual dipolar coupling constant (RDC) is plotted against the same molar fractions. Both curves follow the same trend – as expected – although they do not overlap within the error, presumably due to a fit model that does not sufficiently replicate this complex system. | |
The same 3-component approach was chosen for all samples, although especially samples with a mixture of 4-arm stars and 8-arm stars may show more types of connectivities and would therefore require a more complex model that distinguishes between them more precisely. However, an increase in the number of fit parameters would also dramatically increase the ambiguity involved in fitting, which we wanted to minimise with our decision of sticking with the model with the least possible parameters. As a final remark, it should be noted that this decision and the resulting mismatch does not influence the conclusions extracted from the experiments, as it can be seen visually, as well as from the fit optimisation, that the individual RDCs roughly stay the same, whereas the defect fraction strongly increases, therefore leading to the stated dilution effect.
A similar non-linear increase of defects is reflected also in the FRAP results, where a non-linear increase in the self-diffusion coefficients is found coming along with the incorporation of 8-arm precursors, and therefore increased defect fraction (compare Fig. 5 and 7). The trend of the curves is similar, but mirrored, since at high Φ8-arm, more defects translate in a higher diffusion coefficient.
The DQ-NMR experiments revealed a dilution effect induced by an increasing fraction of 8-arm polymers that have a higher likelihood to form intra-molecular loops. Since loops do generally not contribute to the overall network elasticity, a decrease in the elastic plateau modulus is found, accompanied by an increase in the softness of the hydrogel. At the same time, the mesh-size of the network increases with increasing Φ8-arm, as the number of inter-molecular connections in the network decreases, while the number of intra-molecular connections increases. In addition to that, also the self-diffusion coefficient of a 4-arm 20 K macromolecule increases with increasing Φ8-arm, as intra-molecular loops might retard the re-attachment of diffusing dye-labelled polymers.
Conclusions
Hydrogels are appealing materials that, due to their high content of water, can be used for biomedical applications such as in tissue engineering and drug delivery. However, due to the amorphous structures that naturally occur in such soft-matter systems, the presence of connectivity defects cannot be ignored. Their influence on the final properties of the hydrogel, such as its softness and diffusivity still remains to be further exploited. Therefore, for a rational material design, it is important to know how these defects affect the final gel properties. In this work, we have investigated the effect of connectivity defects on the softness and diffusive permeability of supramolecular pEG-terpyridine hydrogels, on multiple length-scales by systematically introducing 8-arm pEG-terpyridine into a 4-arm pEG-terpyridine model network and characterizing the hydrogels with DQ-NMR, oscillatory shear rheology, and FRAP. As unravelled by DQ-NMR, the pure 8-arm pEG network is more prone to form connectivity defects such as loops. The pronounced loop formation of 8-arm pEG-terpyridine macromolecules is caused by a higher likelihood of forming intra- instead of inter-molecular connections driven by metal–ligand complexation. By increasing the fraction Φ8-arm of 8-arm pEG-terpyridine, these defects lead to an apparent dilution effect resulting in an increase in the mesh-size of the hydrogels and consequently an increase of the self-diffusivity and the softness of the hydrogels, as probed by oscillatory shear rheology and FRAP. These results give a first insight on the effect of controlled connectivity defects on elastic properties of supramolecular hydrogels and bring awareness when tailoring the diffusive properties of the dynamic hydrogel is to be performed. Future perspectives might include the increase in overall polymer concentration to possibly damp the effect of the incorporated amount of 8-arm pEG (40 K), and the variation of the polymer strand size to include further types of defects, as well as taking into account polydisperse building block materials.
Conflicts of interest
The authors declare no conflicts of interest.
Acknowledgements
This work was funded from the H2020 Programme (Marie Skłodowska-Curie Actions) of the European Commission's Innovative Training Networks (H2020-MSCA-ITN-2017) under DoDyNet REA Grant Agreement No. 765811, as well as through the DFG Research Unit FOR 2811, SA982/14-1 (Project. No. 397384169). Parts of this work were further supported by the German Research Foundation (DFG) under Grant No. SE 1888/7-1 (Project No. 376900084). Microscopy data of this study were acquired with a confocal laser scanning microscope funded in part by the Major Research Instrumentation Program of the German Research Foundation under grant No. INST 247/878-1 FUGG. Paola Nicolella thanks Evelyne Van Ruymbeke and Charles-André Fustin for useful discussions, and Rowanne Lyons and Alena Kuzmina for support with the synthesis. Martha Franziska Koziol kindly thanks Holger Adam for helpful methodological advices concerning FRAP. The authors would like to thank the referees of the peer-review process for their valuable and helpful comments.
Notes and references
- A. Jangizehi, F. Schmid, P. Besenius, K. Kremer and S. Seiffert, Soft Matter, 2020, 16, 10809–10859 RSC.
- M. Zhong, R. Wang, K. Kawamoto, B. D. Olsen and J. A. Johnson, Science, 2016, 353, 1264–1268 CrossRef CAS PubMed.
- S. Lin and X. Zhao, Phys. Rev. E, 2020, 102, 052503 CrossRef CAS PubMed.
- A. Habicht, S. Czarnecki, T. Rossow and S. Seiffert, J. Polym. Sci., Part B: Polym. Phys., 2017, 55, 19–29 CrossRef CAS.
- P. Nicolella, D. Lauxen, M. Ahmadi and S. Seiffert, Macromol. Chem. Phys., 2021, 222, 2100076 CrossRef CAS.
- S. Seiffert, Polym. Chem., 2017, 8, 4472–4487 RSC.
- F. Di Lorenzo and S. Seiffert, Polym. Chem., 2015, 6, 5515–5528 RSC.
- S. Mallam, F. Horkay, A. Hecht and E. Geissler, Macromolecules, 1989, 22, 3356–3361 CrossRef CAS.
- Y. Gu, J. Zhao and J. A. Johnson, Trends Chem., 2019, 1, 318–334 CrossRef CAS.
-
K. Saalwächter, in Modern Magnetic Resonance, ed. G. A. Webb, Springer International Publishing, Cham, 2017, pp. 755–781 Search PubMed.
- N. Sood, A. Bhardwaj, S. Mehta and A. Mehta, Drug Delivery, 2016, 23, 748–770 CrossRef CAS PubMed.
- S. Mantha, S. Pillai, P. Khayambashi, A. Upadhyay, Y. Zhang, O. Tao, H. M. Pham and S. D. Tran, Materials, 2019, 12, 3323 CrossRef CAS PubMed.
- A. S. Hoffman, Adv. Drug Delivery Rev., 2012, 64, 18–23 CrossRef.
- C. F. Guimarães, L. Gasperini, A. P. Marques and R. L. Reis, Nat. Rev. Mater., 2020, 5, 351–370 CrossRef.
- M. A. Haque, T. Kurokawa and J. P. Gong, Polymer, 2012, 53, 1805–1822 CrossRef CAS.
- J. Li and D. J. Mooney, Nat. Rev. Mater., 2016, 1, 16071 CrossRef CAS PubMed.
- S. Kondo, H. Sakurai, U. Il Chung and T. Sakai, Macromolecules, 2013, 46, 7027–7033 CrossRef CAS.
- P. Dong, B. J. Schott, A. K. Means and M. A. Grunlan, ACS Appl. Polym. Mater., 2020, 2, 5269–5277 CrossRef CAS.
- A. Sugimura, M. Asai, T. Matsunaga, Y. Akagi, T. Sakai, H. Noguchi and M. Shibayama, Polym. J., 2013, 45, 300–306 CrossRef CAS.
- M. Koziol, K. Fischer and S. Seiffert, Soft Matter, 2019, 15, 2666–2676 RSC.
- S. Tang, A. Habicht, S. Li, S. Seiffert and B. D. Olsen, Macromolecules, 2016, 49, 5599–5608 CrossRef CAS.
- T. Rossow, A. Habicht and S. Seiffert, Macromolecules, 2014, 47, 6473–6482 CrossRef CAS.
- S. Seiffert and W. Oppermann, J. Microsc., 2005, 220, 20–30 CrossRef CAS PubMed.
- G. I. Hauser, S. Seiffert and W. Oppermann, J. Microsc., 2008, 230, 353–362 CrossRef CAS PubMed.
- J. Baum, M. Munowitz, A. N. Garroway and A. Pines, J. Chem. Phys., 1985, 83, 2015–2025 CrossRef CAS.
- K. Saalwächter, P. Ziegler, O. Spyckerelle, B. Haidar, A. Vidal and J. U. Sommer, J. Chem. Phys., 2003, 119, 3468–3482 CrossRef.
- F. Lange, K. Schwenke, M. Kurakazu, Y. Akagi, U. Chung, M. Lang, J.-U. Sommer, T. Sakai and K. Saalwächter, Macromolecules, 2011, 44, 9666–9674 CrossRef CAS.
- M. Ahmadi, L. Löser, K. Fischer, K. Saalwächter and S. Seiffert, Macromol. Chem. Phys., 2020, 221, 1900400 CrossRef CAS.
- W. Chassé, J. L. Valentín, G. D. Genesky, C. Cohen and K. Saalwächter, J. Chem. Phys., 2011, 134, 044907 CrossRef PubMed.
- A. Parker and W. Fieber, Soft Matter, 2013, 9, 1203–1213 RSC.
- M. Ahmadi and S. Seiffert, Soft Matter, 2020, 16, 2332–2341 RSC.
- R. Wang, M. Geven, P. J. Dijkstra, P. Martens and M. Karperien, Soft Matter, 2014, 10, 7328–7336 RSC.
- Y. Akagi, J. P. Gong, U. Chung and T. Sakai, Macromolecules, 2013, 46, 1035–1040 CrossRef CAS.
- W. Schmolke, M. Ahmadi and S. Seiffert, Phys. Chem. Chem. Phys., 2019, 21, 19623–19638 RSC.
- A. Mordvinkin, D. Döhler, W. H. Binder, R. H. Colby and K. Saalwächter, Phys. Rev. Lett., 2020, 125, 127801 CrossRef CAS PubMed.
- A. Mordvinkin, D. Döhler, W. H. Binder, R. H. Colby and K. Saalwächter, Macromolecules, 2021, 54, 5065–5076 CrossRef CAS.
- R. H. Holyer, C. D. Hubbard, S. F. A. Kettle and R. G. Wilkins, Inorg. Chem., 1966, 5, 622–625 CrossRef CAS.
- S. Tang and B. D. Olsen, Macromolecules, 2016, 49, 9163–9175 CrossRef CAS.
- J. Karvinen, T. O. Ihalainen, M. T. Calejo, I. Jönkkäri and M. Kellomäki, Mater. Sci. Eng., C, 2019, 94, 1056–1066 CrossRef CAS PubMed.
-
M. Rubinstein and R. H. Colby, Polymer Physics, Oxford University Press, Oxford, 2003 Search PubMed.
- M. Lang, ACS Macro Lett., 2018, 7, 536–539 CrossRef CAS.
Footnotes |
† Electronic supplementary information (ESI) available. See DOI: 10.1039/d1sm01456k |
‡ These authors contributed equally. |
|
This journal is © The Royal Society of Chemistry 2022 |
Click here to see how this site uses Cookies. View our privacy policy here.