A highly productive mixotrophic fed-batch strategy for enhanced microalgal cultivation†
Received
27th January 2022
, Accepted 10th April 2022
First published on 11th April 2022
Abstract
Microalgal biomass offers great opportunities for green energy generation within emerging biorefinery frameworks. However, the conventional cultivation of microalgae in phototrophic batch systems, which typically yield low biomass productivities, is unfit for large-scale applications. Fed-batch cultivation, on the other hand, represents a more reliable strategy for sustained biomass growth. This work presents a highly productive fed-batch cultivation strategy consisting of intermittent pulses of organic carbon that promotes microalgal growth in mixotrophic mode whilst favouring the formation of starch and lipid metabolites, which have various applications for fuel and high value-added chemicals. Using a combined experimental and modelling approach, the fed-batch pulse feeding regime was additionally optimised for maximal starch and lipid formation, resulting in a 3-pulse strategy which yielded substantial increases of 94% biomass, 676% starch, and 252% lipids with respect to a standard batch scenario. This fed-batch strategy represents a promising cultivation strategy fit for sustainable biofuel production.
Introduction
Transitioning into a sustainable and competitive bio-based economy is the target of various governmental frameworks and research efforts deployed across the globe,1,2 with special interest given to the search and successful utilisation of renewable biomass sources.1 In this regard, microalgae are a promising platform for generating bioenergy and for the production of high value-added products with multiple industrial applications. The versatility of microalgae is the result of a cellular composition rich in proteins, carbohydrates, and lipids, which are industrially important biomolecules.3
Microalgae have gained terrain in the market for high-value, low-demand commodities such as pharmaceuticals or nutraceuticals (e.g. astaxanthin, β-carotene, vitamins, and omega-3 fatty acids), but high-demand low-value chemicals such as advanced biofuels (e.g. biodiesel, bioethanol, and biobutanol) still face significant production challenges.4–6 The successful production of microalgal biofuels, in particular, is an important goal of recent bioeconomy frameworks which will play a key role in meeting the various climate and energy targets set by the EU, such as the 80–95% reduction in greenhouse gases, with respect to the 1990 level, by the year 2050.2 The environmental impacts (e.g. land use, water use, fertiliser use, and greenhouse gas emissions) associated with microalgal biofuel production are estimated to be much lower than those associated with traditional crop-based biofuels or those produced from lignocellulosic substrates.7,8 In addition, since microalgae are aquatic photosynthetic organisms which can grow in a variety of freshwater, marine, or even wastewater environments, the cultivation of microalgae for the purpose of biofuel production avoids one of the most controversial disadvantages of food-based bioenergy feedstocks: the competition for agricultural resources and arable land needed for human food security.6
The successful commercialisation of microalgal fuels and chemicals relies on establishing mass-scale cultivation systems for generating high-density biomass, but traditional phototrophic systems (i.e. where CO2 is fixed in the presence of light) typically underperform in terms of biomass productivity.9 Phototrophic growth is the most common approach for the large-scale cultivation of microalgal biomass, but sustaining high-density cultures is challenging due to CO2 availability and weather conditions restricting light supply.6,10 The need for optimal cultivation strategies for biomass production is leading towards the exploitation of microalgal species capable of assimilating carbon through multiple carbon fixation mechanisms. Heterotrophic organisms, which grow in the absence of light by using organic carbon sources as an energy source, have been shown to maintain higher growth rates than phototrophic cultures with the added advantage of avoiding light limitations. However, heterotrophic-based strategies can be limited by contamination events and higher operating costs.6,11 Mixotrophic species (capable of simultaneously growing phototrophically and heterotrophically) can offer greater resistance to photoinhibition whilst yielding higher biomass productivities than phototrophs or heterotrophs.11,12
Although the supplementation of organic carbon sources can pose economic challenges, optimised light and nutrient supply conditions can position mixotrophic strategies as a more suited approach for the cultivation of high-density fast-growing biomass. Mixotrophic growth combined with well-known nutrient-limited strategies, for example, nitrogen or phosphorus limitation, can improve carbohydrate and lipid formation, so long as nutrients are optimally provided to sustain biomass growth.13,14
In this regard, fed-batch systems are an industrially preferred operating mode leading to increased cell culture lifetime,15 and are proven to yield high microalgal biomass densities.16–19 Mixotrophic-based fed-batch strategies that were reported to increase microalgal growth when compared to typical phototrophic cultivation include supplementation of succinic acid in Haematococcus pluvialis cultures,20 glycerol in Scenedesmus incrassulatus cultures,21 acetic acid in Chlamydomonas reinhardtii cultures,17 and cattle wastewater in Chlorella thermophila cultures.22 The implementation of fed-batch cultivation relies on the difficult identification of adequate feeding regimes, but modelling and simulation techniques can enable fast identification and further optimisation of potential strategies for algal biomass and storage molecule formation, whilst diminishing the costs and time associated with experimental analysis.23,24
This work presents a highly productive fed-batch cultivation strategy consisting of intermittent pulses of organic carbon to promote mixotrophic growth, resulting in markedly increased biomass, starch, and lipid concentrations. In addition, an optimised pulse-assisted fed-batch cultivation strategy for maximal starch and lipid formation is identified via a combined experimental and modelling approach. The optimised pulse strategy, validated experimentally, yielded not only substantially improved biomass concentrations but also significantly higher production of starch and lipids compared to a typical batch scenario, which would allow commercially viable and sustainable biofuel production.
Results and discussion
Evaluation of pulse-assisted fed-batch cultivation on microalgal growth
Mixotrophic growth via acetic acid supply is proven to yield increased biomass densities in batch systems.25 To avoid the eventual depletion of organic carbon and promote further growth, a fed-batch strategy consisting of intermittent carbon pulses was experimentally evaluated at the laboratory scale with the model microalga Chlamydomonas reinhardtii, grown mixotrophically in standard tris–acetate–phosphate (TAP) growth medium with acetic acid as the carbon source.26 To understand the effect of carbon pulses on biomass, starch, and lipid production, cultures were subjected to 1 carbon pulse on the 4th day of cultivation, or with 2 pulses on the 4th and 9th days of cultivation. The cultures were then fully harvested when the cell density reached the late-stationary phase. Pulses were prepared to increase the residual medium concentration of acetic acid in a range of 0.25 g L−1 to 1.5 g L−1 (+0.1 gC L−1 to +0.6 gC L−1). For reference, the initial concentration of acetic acid in a standard TAP medium is 1.05 g L−1 (ref. 26) (0.42 gC L−1).
For comparison, an additional culture was grown in standard batch mode, reaching a biomass concentration of 0.579 g L−1 and accumulating 6.11% and 10.2% of its dry weight as starch and lipids, respectively (Fig. 1). When compared to this batch case, 1-pulse cultures attained biomass concentrations up to 0.863 g L−1 (+49% with respect to batch) when [P1] = +0.5 g L−1 of acetic acid. Nevertheless, as the pulse concentration increased further, the biomass dropped down to 0.495 g L−1 (−14% with respect to the batch) when [P1] = +1.5 g L−1, suggesting inhibition by high acetic acid concentrations. In the 2-pulse cultures, the first pulse was supplemented at the concentration that yielded the highest biomass in the 1-pulse cultures, i.e. [P1] = 0.5 g L−1 (Fig. 1a). The 2-pulse cultures attained significantly higher concentrations than the batch culture (p < 0.01, as per one-way ANOVA), reaching up to 1.107 g L−1 biomass when [P2] = 0.5 g L−1, corresponding to a 28% increase with respect to the maximum obtained by the 1-pulse cultures, and a 91% increase with respect to the batch. However, the biomass concentration dropped with [P2] = 0.75 g L−1, again indicating inhibitory effects.
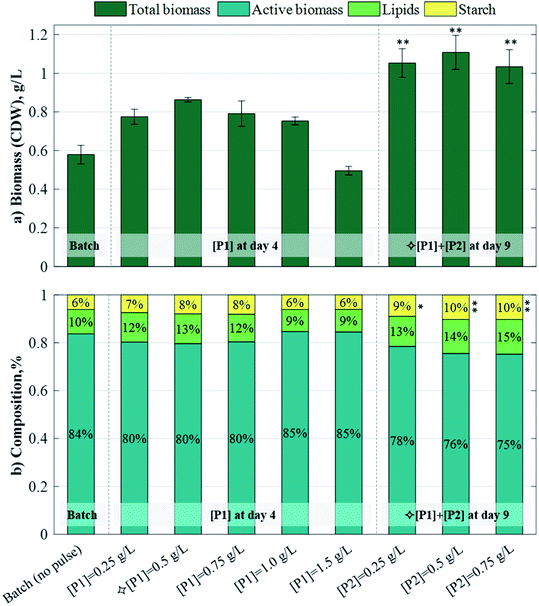 |
| Fig. 1 Effect of acetic acid pulses on: (a) biomass (CDW) and (b) biomass composition in C. reinhardtii. The batch and 1-pulse cultures were harvested on day 9; the 2-pulse cultures (subject to [P1] and various [P2]) were harvested on day 12. The results and SD. are the mean of the two biological replicates. Asterisks denote significant differences (p < 0.05*, 0.01**, 0.001***) with respect to the batch culture. | |
Biomass inhibition by high acetic acid concentrations has also been reported for Graciella sp. In the strain WBG-1, a maximal biomass concentration of 1.5 g L−1 was obtained at 59 mM (∼3.5 g L−1) of acetic acid, and higher concentrations of 73 mM (∼4.3 g L−1) and 147 mM (∼8.8 g L−1) caused a drop in biomass down to 1.21 g L−1.27 In C. reinhardtii, acetic acid concentrations higher than 3.75 g L−1 (∼1.5 gC L−1) have been found to be inhibitory for biomass growth.14 However, since the maximum pulse concentration explored here is only 1.5 g L−1, the inhibition may instead be explained by (i) the reduction of the natural buffering capacity of the medium as growth takes place, (ii) an inhibitory effect caused by the pulse buffering agent (i.e. KOH), which increased proportionately with the acetic acid concentration, or (iii) insufficient medium mixing during pulse addition since this protocol was carried out off-line. Overcoming pulse inhibition effects through an improved on-line pulse feeding system could thus potentially lead to even higher biomass densities. For example, a semi-continuous on-line feeding system of acetic acid with pH control (6.9–7.1) for C. reinhardtii CC-2937 has yielded a biomass density of 23.69 g L−1 (after 168 h), higher than the 2.33 g L−1 (after 123 h) obtained in the batch system.17 In a heterotrophic fed-batch controlled fermenter, a 1.8-fold increase in the C. reinhardtii CS-51 biomass concentration was attained by feeding acetic acid (in the late exponential phase) at a concentration 4-times higher than that used for batch growing conditions.28
Starch and lipid contents increased slightly following the addition of a single pulse, reaching up to 12.5% lipid with [P1] = 0.5 g L−1, and 8.1% starch with [P1] = 0.75 g L−1, respectively (Fig. 1b). In the 2-pulse cultures, starch and lipid contents increased further up to 10.24% and 14.5%, respectively, in [P2] = 0.75 g L−1. The starch contents attained by the 2-pulse cultures were significantly different to those attained in the batch case (p < 0.05 for [P2] = 0.25 g L−1; p < 0.01 for [P2] = 0.5g L−1 and [P2] = 0.75 g L−1), but the lipid contents were not, which is in line with a previous study showing starch as the dominant carbon sink in C. reinhardtii during both nitrogen-starved or excess-acetate culturing conditions.29 The increase in the storage molecule content, coupled with the improved biomass production resulting from pulse additions, led to significant increases in the starch and lipid volumetric concentrations (Fig. 2). The 1-pulse cultures yielded up to 0.679 g L−1 starch and 0.108 g L−1 lipids, corresponding to a 92.8% and 83.3% increase with respect to the batch, respectively. The 2-pulses culture attained up to 0.112 g L−1 starch and 0.158 g L−1 lipids, representing a 65.1% and a 46.2% increase with respect to the maximum concentrations obtained by 1-pulse cultures, and a 218.2% and 168% increase with respect to the batch, respectively.
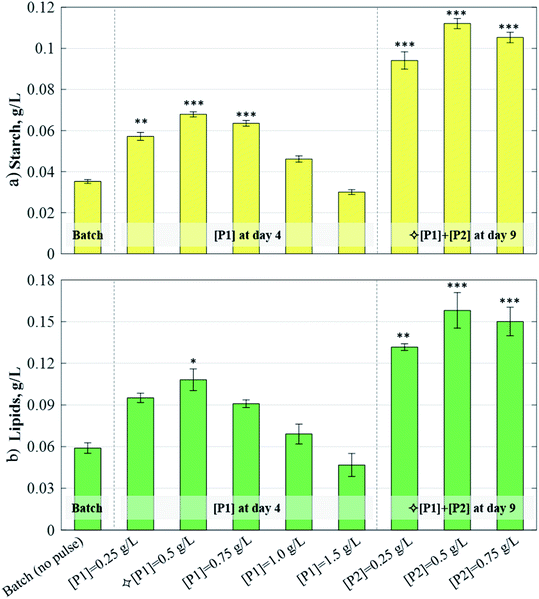 |
| Fig. 2 Effect of acetic acid pulses on: (a) starch and (b) lipid volumetric concentrations in C. reinhardtii cultures. The batch culture and cultures subjected to various [P1] were harvested on day 9. Cultures subjected to [P1] and various [P2] were harvested on day 12. The results and SD. are the mean of the two biological replicates. Asterisks denote significant differences (p < 0.05*, 0.01**, 0.001***) with respect to the batch culture. | |
The continued increase in starch and lipid contents following the addition of a second pulse is attributed to the gradual consumption of nutrients leading to nutrient limitation, which is well-known to induce storage molecule accumulation.30 Limitation by nitrogen or phosphorus, particularly, is shown to increase carbohydrate and lipid contents in green microalgae,31–34 including for C. reinhardtii, which stores carbohydrates mainly in the form of starch.35 However, storage products in C. reinhardtii have also been reported to increase under nutrient-replete, high-acetate conditions (in batch mode),36 which indicates that the addition of pulses may directly lead to an increase of assimilated acetate into starch and lipid metabolites. In particular, assimilated acetate is mainly converted into acetyl-CoA which feeds into the biosynthetic pathways responsible for starch and lipid production.37
Increased production of biomass under mixotrophic conditions is widely reported in the literature. For instance, C. vulgaris biomass has been reported to increase from 1.08 g L−1 when grown phototrophically, to 2.62 g L−1 when grown mixotrophically with molasses.38 For a strain of Scenedesmus sp., mixotrophic growth has led to a 3-fold increase in biomass when compared to phototrophic growth.39 The use of mixotrophic-based fed-batch operation for increased microalgae growth has been less explored, but results from this work along with other recent studies highlight the positive effects of such strategies for biomass and for storage molecule production. For example, the biomass concentration in Scenedesmus incrassulatus has increased from 1.43 g L−1 in phototrophic batch growth (with 23.24% lipid), to 2.83 g L−1 (44.64% lipid) and further up to 5.65 g L−1 (52% lipid) in mixotrophic growth with glycerol during batch and fed-batch operation, respectively.21 Similarly, biomass production of H. pluvialis cultivated with succinic acid was reported to increase from 1.7 g L−1 in the batch to 2.01 g L−1 in fed-batch mode, which in turn increased the concentration of the astaxanthin pigment from 47.89 mg L−1 to 64.61 mg L−1.20
Overall, the pulse-assisted fed-batch cultivation strategy presented here led to significantly enhanced biomass, starch, and lipid production. This strategy thus represents a highly productive cultivation system suited to overcome the low productivities generally attained by traditional phototrophic systems, particularly at open pond outdoor scales.40 Evaluating the outcome of pulse additions at different times and concentrations can therefore provide a means to establish optimal operating conditions. A systematic experimental and modelling approach was exploited here to facilitate this analysis.
Modelling fed-batch cultivation
A kinetic model for microalgal growth dynamics showing a high predictive capacity was recently developed in-house.14 This model considers that the total cell biomass is comprised of three carbon-based pools: starch, lipids, and active biomass. The intracellular carbon partitioning between these compartments is governed by the uptake of external carbon (i.e. the mixotrophic substrate) and regulated by the intracellular nitrogen and phosphorous quotas. The state variables of the model include: total biomass, X (gC L−1); total nitrogen, N (gN L−1); nitrogen quota, qN (gN gC−1); phosphorous, P (gPO4 L−1); phosphorus quota, qP (gPO4 gC−1); acetic acid, A (gC L−1); starch, S (gC L−1); lipids, L (gC L−1); and active biomass, x* (gC L−1).14 This model was shown to have great value as an optimisation tool, but as it was only formulated to portray batch conditions, an enhanced yet simpler formulation accounting for fed-batch dynamics is presented here.
Specific growth rate.
The specific growth rate, μ, considers mixotrophic microalgal growth to be limited by nitrogen, acetic acid, and light: | μ(A,I,qN) = M,max(A,Ī) × μN(qN) × f(APulse) | (1) |
Here,
max(A,Ī) is the mixotrophic growth rate, dependent on the weighed contributions of the heterotrophic growth rate, μH(A), and the phototrophic growth rate, μI(Ī), which account for substrate inhibition and photoinhibition effects, respectively: |  | (2) |
Here, μmax is the maximum specific growth rate; KS,A and KI,A are the half-saturation and inhibition constants, respectively; wH and wI are weighing functions that regulate the extent of heterotrophic and phototrophic growth, respectively;14 and KS,I and Ki,I are the half-saturation and inhibition constants, respectively, associated with the average light received by the culture, Ī: |  | (3) |
where Io is the incident light (at z = 0), L is the culture depth, and σ is the light attenuation coefficient. The nitrogen-limited growth rate, μN(qN) depends on the nitrogen quota, qN, i.e. the ratio of intracellular nitrogen to the carbon-biomass concentration:41 | 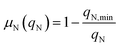 | (4) |
Here, qN,min, is the minimum quota necessary for growth, i.e. μN(qN) = 0 if qN ≤ qN,min. The specific growth rate is further regulated by a function dependent on the pulse concentration, accounting for the inhibition effects observed experimentally (Fig. 1a): | 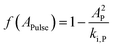 | (5) |
Here, AP is the increase in the acetic acid concentration caused by the pulse, and ki,P is an inhibition constant. As mentioned previously, the original model formulation was developed to portray batch dynamics and was thus unable to describe the outcome of pulse-assisted cultivation. Particularly, simulations using the batch model formulation yielded no further growth in biomass after 2 or more pulses. This halt in cellular growth, as simulated by the batch model, was observed to be a consequence of the nitrogen quota of cells falling below their minimum quota, qN,min. Therefore, to account for the continued growth of biomass due to the pulses, the model was enhanced by refining the dynamics of nitrogen uptake, which in turn drive the intracellular nitrogen quota levels.
Nutrient uptake rates.
The batch model considered that the rate of accumulation of the nitrogen quota was driven by the uptake of the total nitrogen in the medium. This assumption, however, did not account for the fact that not all of the sources of nitrogen are bioavailable to cells. In TAP medium, the bioavailable source of nitrogen is ammonium, but the remaining nitrogen source originates from a biochemical buffer, i.e. Tris-base, H2NC(CH2OH)3. Taking this into consideration, the model was refined to consider that ammonium is the driver for cellular growth. In line with this, the nitrogen uptake rate, ρN, is: |  | (6) |
Here, ρN,max, is the maximum nitrogen uptake rate, ks,N and ki,N are the half-saturation and inhibition constants associated with nitrogen; and ks,A:N and ki,A:N are the half-saturation and inhibition constants associated with acetic acid. In eqn (6), f(qP) is a Droop function of the phosphorus quota which regulates nitrogen uptake: | 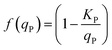 | (7) |
Here, KP is the minimum P quota below which nitrogen uptake stops, i.e. if qP < KP, ρN = 0. The expression for nitrogen uptake presented above is a more simple formulation that avoids the incorporation of more complex luxury uptake kinetics. The uptake rate of phosphorus is described by: | 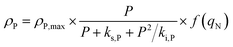 | (8) |
Here, ρP,max is the maximum phosphorus uptake rate, ks,P is a phosphorus-associated half-saturation constant, and ki,P is an inhibition constant. f(qN) is a function of the nitrogen quota which regulates phosphorus uptake during nitrogen-limited scenarios: | 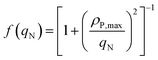 | (9) |
The uptake rate of acetic acid, ρA, is assumed to be proportional to the heterotrophic contribution to the microalgae's mixotrophic growth rate, so that:
| ρA = ρA,max × wH × μH | (10) |
Here,
ρA,max is the maximum uptake rate of acetic acid.
Starch and lipid formation rates.
The accumulation of starch and lipids is regulated by their synthetic rates, R1 and R2, and their degradation rates, R3 and R4: |  | (11) |
|  | (12) |
| 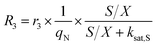 | (13) |
| 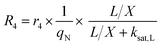 | (14) |
In eqn (11) and (12), Ni = qN × X is the intracellular nitrogen concentration; r1,1 and r1,2 are the starch synthetic rate constants; r2,1 and r2,2 are the lipid synthetic rate constants; ks,S and ks,L are the half-saturation constants for starch and lipids, respectively; nS and nL are shape-controlling parameters; kA,S and kA,L are half-saturation constants; and ki,S and ki,L are inhibition constants. In eqn (13) and (14) (degradation rates), r3 and r4 are the starch and lipid degradation rate constants, respectively, and ksat,S and ksat,L are the half-saturation constants that regulate the extent of starch and lipid degradation.
Time-dependent kinetic expressions.
The rate of accumulation of each model state variable is denoted by: | 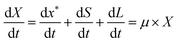 | (15) |
|  | (16) |
|  | (17) |
| 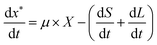 | (18) |
|  | (19) |
| 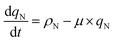 | (20) |
|  | (21) |
| 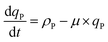 | (22) |
|  | (23) |
Assessing the model's performance
The updated model presented here comprises 9 state variables (eqn (15)–(23)) and 31 kinetic parameters (Table 1). To estimate the parameter values and assess the model's predictive capacity, a set of fed-batch cultivation experiments were performed with C. reinhardtii subjected to three consecutive pulses (i.e. [P1] + [P2] + [P3]) injected at different times: (i) short-interval mode, where tP,1 = 56 h, tP,2 = 124 h, and tP,3 = 221 h (days 2, 5, and 9), (ii) regular-interval mode, where tP,1 = 97 h, tP,2 = 241 h, and tP,3 = 354 h (days 4, 10, and 14), and (iii) long-interval mode, where tP,1 = 148 h, tP,2 = 291 h, and tP,3 = 408 h (days 6, 12, and 17). In the short-, regular-, and long-interval modes, the pulses raised the residual concentration of acetic acid by 0.5 g L−1 (i.e. +0.2 gC L−1). An additional experiment at regular intervals was conducted with the High-A concentration at 1.25 g L−1 (i.e. +0.5 gC L−1).
Table 1 List of parameter values and definitions, employed in the predictive model for fed-batch microalgal cultivation
Type |
Symbol |
Parameter description |
Value |
Units |
Associated with biomass growth |
μ
max
|
Maximum specific growth rate |
2.497 |
h−1 |
q
N,0
|
Minimum nitrogen quota |
0.125 |
gN gC−1 |
K
s,A
|
Acetate saturation constant |
0.144 |
gC L−1 |
k
i,A
|
Acetate inhibition constant |
0.132 |
gC L−1 |
K
s,I
|
Light saturation constant |
20.8 |
μmol m−2 s−1 |
k
i,I
|
Light inhibition constant |
0.000 |
μmol m−2 s−1 |
ρ
A,max
|
Maximum acetate uptake rate |
1.864 |
h−1 |
σ
|
Light attenuation coefficient |
34.75 |
L gC−1 m−1 |
Associated with N & P uptake |
ρ
N,max
|
Maximum N uptake rate |
22.46 |
gN gC−1 h−1 |
K
s,N
|
Uptake saturation constant, N |
0.240 |
gN L−1 |
k
i,N
|
Uptake inhibition constant, N |
0.085 |
gN L−1 |
K
s,A : N |
Uptake saturation constant, A : N |
16.026 |
gC L−1 |
k
i,A : N |
Uptake inhibition constant, A : N |
21.128 |
gC L−1 |
K
P
|
P Quota supporting N uptake |
0.145 |
gPO4 gC−1 |
ρ
P,max
|
Maximum P uptake rate |
1.02 |
gPO4 gC−1 h−1 |
K
s,P
|
Uptake saturation constant, P |
0.317 |
gPO4 L−1 |
k
i,P
|
Uptake inhibition constant, P |
1.445 |
gPO4 L−1 |
Associated with starch & lipid formation |
r
1,1
|
Starch formation rate (R1) |
3.135 |
gC gC−1 |
k
s,S
|
Saturation constant (R1) |
0.132 |
gN L−1 |
n
S
|
Shape parameter (R1) |
21.545 |
— |
r
1,2
|
Starch formation rate (R1) |
0.533 |
gC gC−1 |
k
A,S
|
Half-saturation constant (R1) |
6.968 |
gC L−1 |
k
i,S
|
Inhibition constant (R1) |
2.675 |
gC L−1 |
r
2
|
Starch degradation rate (R3) |
0.000 |
gC gC−1 |
k
sat,S
|
Starch saturation constant (R3) |
10.680 |
— |
r
2,1
|
Lipid formation rate (R2) |
14.854 |
gC gC−1 |
k
s,L
|
Saturation constant (R2) |
0.292 |
gN L−1 |
n
L
|
Shape parameter (R2) |
4.069 |
— |
r
2,2
|
Starch formation rate (R2) |
0.293 |
gC gC−1 |
k
A,L
|
Half-saturation constant (R2) |
6.968 |
gC L−1 |
k
i.L
|
Inhibition constant (R2) |
2.675 |
gC L−1 |
r
4
|
Starch degradation rate (R4) |
0.000 |
gC gC−1 |
k
sat,L
|
Starch saturation constant (R4) |
15.000 |
— |
The short-interval, regular-interval, and High-A datasets were used for model fitting. In line with the model formulation, the concentrations of all carbon-based molecules (i.e. biomass, active biomass, starch, lipids, and acetic acid) are expressed in terms of their carbon-equivalent concentration (conversion factors are available in the Experimental section). The fitting datasets yielded a good level of agreement with the corresponding experimental data (ESI†), and as observed in Fig. 3, the model predictions were additionally found to be in good agreement with the data obtained from the long-interval pulse scenario which was used for model validation. By enhancing model considerations for nitrogen uptake, the fed-batch model formulation was capable of describing the continued growth of biomass after pulse injection. However, it is worth mentioning that more complex metabolic processes, which the model does not account for, may also be responsible for the cells growing further following the addition of pulses.
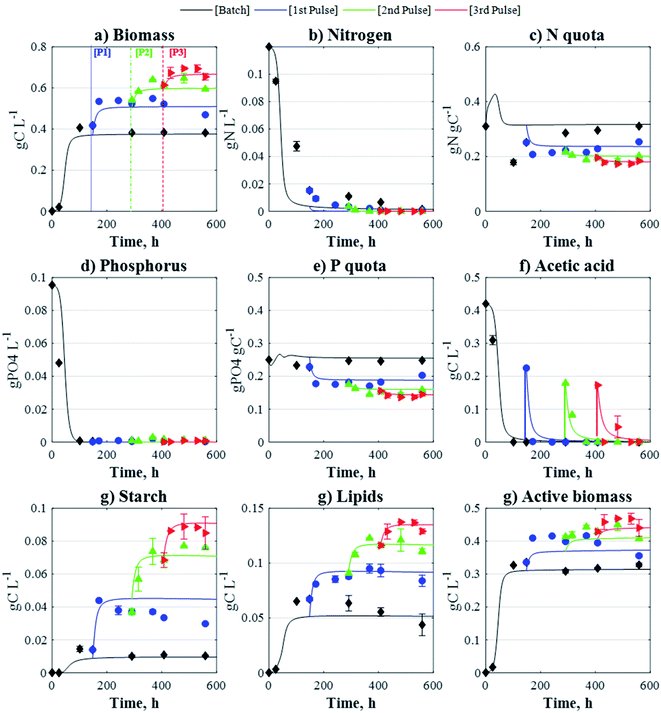 |
| Fig. 3 Comparison between model-derived concentration profiles (lines) and experimental data (points) obtained by the long-interval pulse strategy (model validation). Pulse injection times: tP,1 = 148 h, tP,2 = 291 h, and tP,3 = 408 h. Data and SD. are the mean of the two biological replicates. | |
In microalgae, proteins and enzymes associated with nutrient transport and assimilation may be either suppressed when the intracellular nutrients are sufficient, or activated when nutrient levels are low.42 For example, it has been reported that nutrient-stressed cells of C. reinhardtii increase the enzymes responsible for the recycling of nitrogen-containing (or phosphorous-containing) molecules, e.g. proteins and nucleic acids.37,43 These mechanisms may allow nutrient-limited cells to replenish their nutrient quotas, leading to the assimilation of additional carbon (such as that supplied through pulses) and ultimately increase biomass production. On the other hand, other studies have evidenced that high acetate conditions can inhibit photosynthetic activity.36,44 The addition of acetate pulses may thus switch cells into a heterotrophic mode, reducing the metabolic burdens associated with photosynthesis and allowing further growth during nutrient limitation.
A summarised view of the final biomass, starch, and lipid concentrations generated from the four different fed-batch strategies, against the corresponding model outputs, is presented in Fig. 4. Compared to the batch scenario (0.708 g L−1 biomass, 0.031 g L−1 starch, and 0.055 g L−1 lipids), the fed-batch strategies yielded substantial increases in biomass of up to 43% and 64% after 1 and 2 regular-interval pulses, respectively, and 75% after 3 short-interval pulses. The starch concentration increased up to 119%, 460%, and 524% after 1, 2, and 3 long-interval pulses, respectively. Meanwhile, lipids increased up to 111% after 1 short-interval pulse, 179% after 2 regular-interval pulses, and 206% after 3 short-interval pulses. These strategies showcase the potential of a pulse-assisted fed-batch strategy for enhanced microalgal production.
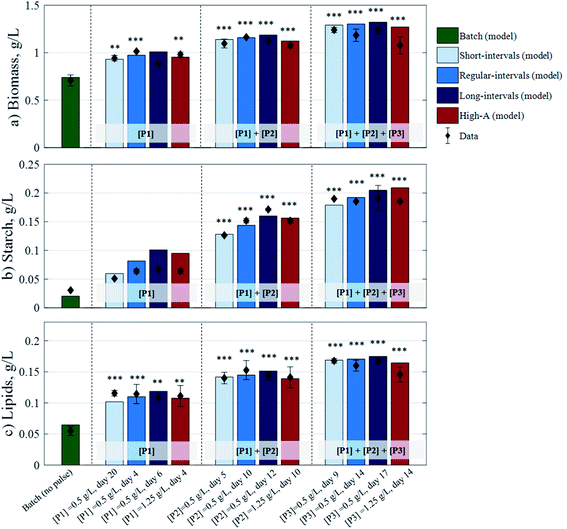 |
| Fig. 4 Experimental data for biomass, starch, and lipid concentrations against model-derived data (in g L−1), subjected to the different fed-batch strategies. Data and error bars are the average of the two biological replicates taken at the end of each cultivation strategy: 408 h (short-interval strategy), 503 h (batch, regular-interval, and High-A strategies), and 559 h (long-interval strategies). Asterisks denote significant differences (p < 0.05*, 0.01**, 0.001***) with respect to the batch culture. | |
An optimal pulse feeding strategy for maximised starch and lipid production
The fed-batch model presented here was exploited to identify an optimal 3-pulse strategy leading to maximised starch and lipid formation. An optimisation problem was set up to compute two pulse-related operating parameters (eqn (24)): (i) their optimal injection times and (ii) the required increase in the acetic acid concentration: | Objective = max[S(tPi,APi) + L(tPi,APi)]t=tfinal | (24) |
Here, S and L are the model-predicted starch and lipid medium concentrations at a time of tfinal = 600 h (25 days), following 3 pulses at times tPi = 1,2,3, with concentrations of APi = 1,2,3, subjected to the constraints: 0.025 g L−1 < APi < 1.5 g L−1 (i.e. +0.01 gC L−1 < APi < +0.6 gC L−1); 24 h < tP1 < 200 h; 201 h < tP2 < 360 h; and 361 h < tP3 < 540 h, selected so as to be within the experimental operational ranges used for model fitting and validation. The optimisation problem was solved via a sequential stochastic-deterministic optimisation algorithm (Experimental section).
The resulting optimal fed-batch strategy consisted of: [P1], AP,1 = 0.925 g L−1 (+0.37 gC L−1) at tP,1 = 199 h; [P2], AP,2 = 0.575 g L−1 (+0.23 gC L−1) at tP,2 = 330 h; and [P3], AP,3 = 0.475 g L−1 (+0.19 gC L−1) at tP,3 = 445 h. The model-based optimal strategy was further validated experimentally, and as shown in Fig. 5, the model predictions for biomass, starch, and lipids, as well as the predictions for biomass composition (e.g. active biomass, starch, and lipid contents), were in good agreement with the corresponding experimental data, highlighting the model's predictive power. Overall, and when compared to batch, the 3-pulse strategy yielded statistically significant increases in biomass, starch, and lipid concentrations of 94%, 676%, and 252%, respectively. As shown in Table 2, the bioprocess efficiency of the optimal fed-batch strategy subjected to three pulses was higher than the batch scenario, with starch and lipid contents increasing 4-fold and 2-fold following the addition of three pulses, respectively.
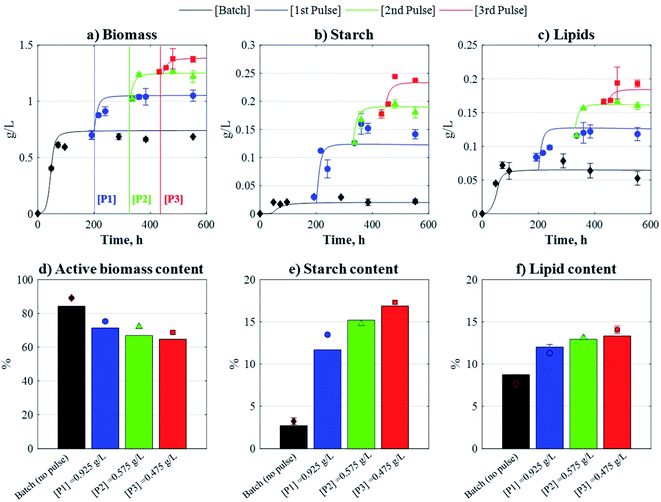 |
| Fig. 5 Model-derived time-profiles (solid lines) and biomass composition (solid bars) against experimental data (points), as obtained by the optimal 3-pulse fed-batch strategy. Pulse injection times: tP,1 = 199 h, tP,2 = 330 h, and tP,3 = 445 h. Biomass composition measured at 552 h. Data and SD. are the mean of the two biological replicates. | |
Table 2 Experimental data and efficiency parameters for the model-based optimal fed-batch (FB) strategy for maximal starch and lipid formation. Asterisks denote significant differences (p < 0.05*, 0.01**, 0.001***) with respect to the batch culture
|
Biomass g L−1 |
Starch g L−1 |
Lipids g L−1 |
Starch% |
Lipids% |
μ
x
d−1 |
P
x g L−1 d−1 |
P
S mg L−1 d−1 |
P
L mg L−1 d−1 |
Measured at t = 503 h.
Measured at t = 552 h.
|
Batcha (non-optimised) |
0.708 |
0.031 |
0.055 |
4.3% |
7.7% |
0.28 |
0.034 |
1.449 |
2.605 |
FB [P1]b |
1.051** |
0.142*** |
0.118** |
13.5% |
11.2% |
0.27 |
0.046 |
6.150 |
5.133 |
FB [P2]b |
1.221** |
0.180*** |
0.160*** |
14.8% |
13.1% |
0.28 |
0.053 |
7.842 |
6.954 |
FB [P3]b |
1.374*** |
0.238*** |
0.193*** |
17.3% |
14.0% |
0.28 |
0.060 |
10.324 |
8.383 |
The increase in starch and lipid by this optimal strategy was found to be additionally higher than that obtained with optimal batch scenarios for maximal starch and lipid formation, which reached an increase of 270% and 74%, respectively.14 It is noteworthy to mention that although the pulse strategies evaluated in this work have been validated with acetic acid, more sustainable alternative waste products (e.g. glycerol from bio-diesel waste) can potentially lead to more sustainable cultivation scenarios. Nevertheless, the substantial increases in storage metabolites represent a positive step towards successfully implementing microalgal biorefineries targeting the production of biofuels to achieve net zero energy transportation systems in the future.
Conclusions
A fed-batch cultivation strategy for increased microalgae biomass formation was evaluated with C. reinhardtii subjected to intermittent pulses of acetic acid. When compared to a batch case, the addition of consecutive pulses of acetic acid yielded significant increases in the concentrations of biomass, starch, and lipids. A model for fed-batch cultivation dynamics was additionally developed and exploited to identify an optimal 3-pulse strategy. The optimal strategy was validated experimentally, demonstrating a higher production efficiency than a typical batch cultivation, with 94% more biomass, 676% more starch, and 252% more lipids. The pulse feeding strategy explored in this work is thus a highly suited cultivation strategy with great industrial potential within emerging biorefinery frameworks.
Experimental section
Strain and fed-batch cultivation
All experiments were carried out with the wild-type strain Chlamydomonas reinhardtii CCAP 11/32C, grown in tris–acetate–phosphate (TAP) medium.45 Prior to the cultivation experiments, an active algal inoculum was prepared by growing the strain for 7 days (up to the late stationary phase) in 150 mL of sterile TAP medium. The algal inoculum was kept in a rotary shaker at a rotating speed of 150 rpm. Temperature was maintained at 25 °C, and light was supplied at an incident intensity of 125 μmol m−2 s−1 (one-side illumination, from above) in a photoperiod of 16 h light and 8 h dark.
Fed-batch cultivation
Fed-batch experiments were performed in duplicate in borosilicate glass bottles with 500 mL of sterile TAP medium under the environmental conditions described above. Growth was initiated by inoculating all culture vessels with 1 mL of algal inoculum. All cultures were started in a batch mode and then subjected to a feeding strategy involving intermittent pulses of acetic acid (i.e. the organic carbon source in a standard TAP medium) at different concentrations and cultivation times. The volume of the pulses was set to 20 mL, and their acetic acid concentration ranged from 6.5 to 39 g L−1 so that the corresponding increase in the medium concentration after pulse addition ranged from 0.25 to 1.5 g L−1, e.g. a 20 mL pulse with 6.5 g L−1 of acetic acid, supplied to 500 mL of a growing culture increases the residual medium concentration by 0.25 g L−1 (0.1 gC L−1), and so on. For reference, standard TAP medium contains an initial acetic acid concentration of 1.05 g L−1 (0.42 gC L−1).
The pulses were prepared by mixing the required concentration of acetic acid in nitrogen-free TAP salt solution (diluted 1
:
4 in distilled water) to maintain nitrogen-limited conditions. To avoid drastic reductions of pH in the culture medium after pulse injection, the pH of the pulse solution was set to 4.75 with potassium hydroxide (KOH) 3 M. All pulses were sterilised prior to use. At each sampling time, replicate cultures were fully harvested for analysis of the biomass (cell dry weight) and residual concentrations of metabolites. All data were statistically analysed by one-way ANOVA in Origin Pro 2022 (9.9.0.220).
Analytical methods
Cell dry weight.
The biomass cell dry weight (CDW) was measured by centrifuging microalgal cultures for 5 min at 8885 g in an Eppendorf centrifuge 5804. The pelleted cells were placed in pre-weighed tubes and left to dry at 90 °C for 24 h. The pellets were cooled down to room temperature in a desiccator and the cell dry weight was calculated gravimetrically using a M-Pact AX221 fine balance (Sartorius). The samples of the supernatant were kept in Falcon tubes and stored at −20 °C for further analysis. The medium pH was measured using a bench-type HI-2211 pH meter (Hanna Instruments).
Residual nutrient concentration.
The concentration of acetic acid was quantified via HPLC analysis using a HPX-87H column (300 × 7 mm) and a UV detector at a wavelength of 210 nm. The mobile phase (H2SO4, 0.005 M) was set at a flow rate of 0.6 mL min−1 and a temperature of 50 °C. The acetic acid concentration was measured from the area of the chromatographic peaks read against a calibration curve. The concentration of nitrogen was measured using a spectrophotometric Ammonia Assay Kit with a concentration range of 0.2–15 μg mL−1 (Sigma-Aldrich); the samples were diluted accordingly in Type 1 grated water. The intracellular concentration of nitrogen was assumed to be equivalent to the nitrogen consumed by cells (i.e. the difference between the initial and residual nitrogen concentration), and the nitrogen quota, qN (gN gC−1), was then estimated by dividing the intracellular nitrogen concentration by the biomass (CDW) concentration.46
Starch and lipid quantification.
Microalgal starch was measured using a Total Starch kit (Megazyme International, Ireland). Prior to analysis, the harvested cells were pre-treated as in (ref. 47) to remove chlorophyll pigments, break cells, and solubilise starch. The cells were then subjected to a two-stage enzymatic hydrolysis (as per the manufacturer's instructions) to release D-glucose, after which the concentration was measured colorimetrically (at 508 nm) against a standard calibration curve. The starch concentration was calculated by multiplying the D-glucose concentration by 0.9, a factor adjusting free D-glucose to anhydrous D-glucose. Microalgal lipids were measured by solvent extraction using an ST-243 SoxtecTM (FOSS). Prior to lipid extraction, the dried cell pellets (as obtained from CDW measurements) were manually pulverised using a mortar and pestle alternated with liquid nitrogen supply. The cells were weighed and placed in cellulose extraction thimbles (26 × 60 mm, 603, Whatman®), and the lipids were then extracted using a three-stage program as in (ref. 13). The extracted lipids were allowed to cool down to room temperature in a desiccator and the concentration was then calculated gravimetrically.
Estimation of model parameters
The kinetic model presented above contains 31 kinetic parameters (Table 1). The parameter values were estimated by minimising the squared relative error (eqn (25)) between the predicted data and experimental data (for the 9 cultivation variables). The objective function was solved via an optimisation-based routine combining stochastic (simulated annealing, SA) and deterministic (successive quadratic programming, SQP) algorithms, thus avoiding the chances of getting trapped in local minima.48 | 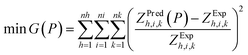 | (25) |
Here, G is the objective function (i.e. the squared relative error), P is a vector with the kinetic parameters to be estimated, and Z is a vector with the predicted data (subject to P) or experimental data corresponding to each model state variable. nh, ni, and nk denote the number of data points in time, the number of datasets used for fitting, and the number of state variables, respectively. The resulting parameter values obtained from the fitting protocol described here are included in Table 1 along with their definitions and corresponding units.
As mentioned before, the model developed here considers that the cell biomass is made up of three carbon-based compartments (starch, lipids, and active biomass). To represent the intracellular carbon partitioning between these molecules, experimental data for starch, lipids, active biomass, and the mixotrophic carbon source (acetic acid) were expressed in terms of their carbon-equivalent concentrations. First, the active biomass fraction was estimated by subtracting the starch and lipid concentrations from the total biomass dry weight. Then, carbon-equivalent concentrations were computed by means of the following conversion factors:46 0.44 gC g−1 starch (C6H10O5)n; 0.77 gC g−1 lipid (taken as C55H98O6); 0.4 gC g−1 acetic acid CH3COOH; 0.504 gC g−1C. reinhardtii biomass, taken as CH1.75O0.56N0.08.49
Author contributions
GFT: formal analysis, investigation, methodology, software, validation, visualization, writing – original draft. JKP: funding acquisition, project administration, supervision, writing – review & editing. CT: conceptualization, funding acquisition, project administration, resources, supervision, writing – review & editing.
Conflicts of interest
There are no conflicts to declare.
Acknowledgements
The authors kindly acknowledge the financial support of the Interreg-funded project EnhanceMicroAlgae (EAPA_338/2016). GMFT also gratefully recognises the National Mexican Council for Science and Technology (CONACyT, 249472).
References
- N. Scarlat, J. F. Dallemand, F. Monforti-Ferrario and V. Nita, Environ. Dev., 2015, 15, 3–34 CrossRef.
-
C. Lago, I. Herrera, N. Caldés and Y. Lechón, in The Role of Bioenergy in the Emerging Bioeconomy: Resources, Technologies, Sustainability and Policy, Elsevier, 2018, pp. 3–24 Search PubMed.
- T. Suganya, M. Varman, H. H. Masjuki and S. Renganathan, Renewable Sustainable Energy Rev., 2016, 55, 909–941 CrossRef CAS.
- Y. Su, K. Song, P. Zhang, Y. Su, J. Cheng and X. Chen, Renewable Sustainable Energy Rev., 2017, 74, 402–411 CrossRef.
- L. Barsanti and P. Gualtieri, Algal Res., 2018, 31, 107–115 CrossRef.
- M. A. Scaife, A. Merkx-Jacques, D. L. Woodhall and R. E. Armenta, Renewable Sustainable Energy Rev., 2015, 44, 620–642 CrossRef.
- M. J. Groom, E. M. Gray and P. A. Townsend, Conserv. Biol., 2008, 22, 602–609 CrossRef PubMed.
- L. A. Ribeiro and P. P. da Silva, Renewable Sustainable Energy Rev., 2013, 25, 89–96 CrossRef CAS.
- A. I. Barros, A. L. Gonçalves, M. Simões and J. C. M. Pires, Renewable Sustainable Energy Rev., 2015, 41, 1489–1500 CrossRef.
- B. Colling Klein, A. Bonomi and R. Maciel Filho, Renewable Sustainable Energy Rev., 2018, 82, 1376–1392 CrossRef.
- M. K. Enamala, S. Enamala, M. Chavali, J. Donepudi, R. Yadavalli, B. Kolapalli, T. V. Aradhyula, J. Velpuri and C. Kuppam, Renewable Sustainable Energy Rev., 2018, 94, 49–68 CrossRef CAS.
- K. Chojnacka and A. Noworyta, Enzyme Microb. Technol., 2004, 34, 461–465 CrossRef CAS.
- M. Bekirogullari, I. S. Fragkopoulos, J. K. Pittman and C. Theodoropoulos, Algal Res., 2017, 23, 78–87 CrossRef.
- G. M. Figueroa-Torres, J. K. Pittman and C. Theodoropoulos, Biotechnol. Biofuels, 2021, 14, 64 CrossRef CAS.
- A. Kiparissides, M. Koutinas, C. Kontoravdi, A. Mantalaris and E. N. Pistikopoulos, Automatica, 2011, 47, 1147–1155 CrossRef.
- C. Jeffryes, J. Rosenberger and G. L. Rorrer, Algal Res., 2013, 2, 16–27 CrossRef.
- F. J. Fields, J. T. Ostrand and S. P. Mayfield, Algal Res., 2018, 33, 109–117 CrossRef.
- T. Wang, X. Tian, T. Liu, Z. Wang, W. Guan, M. Guo and J. Chu, Process Biochem., 2017, 60, 74–83 CrossRef CAS.
- F. Ji, Y. Zhou, A. Pang, L. Ning, K. Rodgers, Y. Liu and R. Dong, Bioresour. Technol., 2015, 184, 116–122 CrossRef CAS PubMed.
- C. Yu, H.-P. Wang, T. Qiao, Y. Zhao and X. Yu, Bioresour. Technol., 2021, 340, 125648 CrossRef CAS PubMed.
- P. Rattanapoltee, P. Dujjanutat, P. Muanruksa and P. Kaewkannetra, Biochem. Eng. J., 2021, 175, 108128 CrossRef CAS.
- R. Jain, S. Mishra and K. Mohanty, J. Environ. Manage., 2022, 304, 114213 CrossRef CAS PubMed.
- A. Velayudhan, Curr. Opin. Chem. Eng., 2014, 6, 83–89 CrossRef.
- M. Bekirogullari, G. M. Figueroa-Torres, J. K. Pittman and C. Theodoropoulos, Biotechnol. Adv., 2020, 44, 107609 CrossRef CAS PubMed.
- M. Bekirogullari, J. K. Pittman and C. Theodoropoulos, Bioresour. Technol., 2018, 269, 417–425 CrossRef CAS PubMed.
-
E. H. Harris, D. B. Stern and G. Witman, The Chlamydomonas Sourcebook, Academic, 2008 Search PubMed.
- X. Wen, H. Tao, X. Peng, Z. Wang, Y. Ding, Y. Xu, L. Liang, K. Du, A. Zhang, C. Liu, Y. Geng and Y. Li, Biotechnol. Biofuels, 2019, 12, 27 CrossRef PubMed.
- X.-W. Zhang, F. Chen and M. R. Johns, Process Biochem., 1999, 35, 385–389 CrossRef CAS.
- J. Fan, C. Yan, C. Andre, J. Shanklin, J. Schwender and C. Xu, Plant Cell Physiol., 2012, 53, 1380–1390 CrossRef CAS PubMed.
- G. Markou, I. Angelidaki and D. Georgakakis, Appl. Microbiol. Biotechnol., 2012, 96, 631–645 CrossRef CAS PubMed.
- I. Brányiková, B. Maršálková, J. Doucha, T. Brányik, K. Bišová, V. Zachleder and M. Vítová, Biotechnol. Bioeng., 2010, 108, 766–776 CrossRef PubMed.
- B. J. Cade-Menun and A. Paytan, Mar. Chem., 2010, 121, 27–36 CrossRef CAS.
- G. Dragone, B. D. Fernandes, A. P. Abreu, A. a. Vicente and J. a. Teixeira, Appl. Energy, 2011, 88, 3331–3335 CrossRef CAS.
- T. Lacour, A. Sciandra, A. Talec, P. Mayzaud and O. Bernard, J. Phycol., 2012, 48, 647–656 CrossRef CAS PubMed.
-
S. G. Ball and P. Deschamps, The Chlamydomonas Sourcebook: Starch Metabolism, Elsevier, 2009 Search PubMed.
- K. A. Bogaert, E. Perez, J. Rumin, A. Giltay, M. Carone, N. Coosemans, M. Radoux, G. Eppe, R. D. Levine, F. Remacle and C. Remacle, Cells, 2019, 8, 1367 CrossRef CAS.
- X. Johnson and J. Alric, Eukaryotic Cell, 2013, 12, 776–793 CrossRef CAS PubMed.
- M. A. M. Mirzaie, M. Kalbasi, S. M. Mousavi and B. Ghobadian, Prep. Biochem. Biotechnol., 2016, 46, 150–156 CrossRef PubMed.
- M. Kamalanathan, P. Chaisutyakorn, R. Gleadow and J. Beardall, Phycologia, 2018, 57, 309–317 CrossRef CAS.
- N. G. Schoepp, R. L. Stewart, V. Sun, A. J. Quigley, D. Mendola, S. P. Mayfield and M. D. Burkart, Bioresour. Technol., 2014, 166, 273–281 CrossRef CAS PubMed.
- M. R. Droop, J. Mar. Biol. Assoc. U. K., 1968, 48, 689–733 CrossRef CAS.
- J. A. Bonachela, M. Raghib and S. A. Levin, Proc. Natl. Acad. Sci. U. S. A., 2011, 108, 20633–20638 CrossRef CAS.
- A. Grossman, Protist, 2000, 151, 201–224 CrossRef CAS PubMed.
- P. B. Heifetz, B. Förster, C. B. Osmond, L. J. Giles and J. E. Boynton, Plant Physiol., 2000, 122, 1439–1446 CrossRef CAS PubMed.
-
E. H. Harris, The Chlamydomonas Sourcebook: a Comprehensive Guide to Biology and Laboratory Use, Academic Press, 1989 Search PubMed.
- G. M. Figueroa-Torres, J. K. Pittman and C. Theodoropoulos, Bioresour. Technol., 2017, 241, 868–878 CrossRef CAS PubMed.
- A. K. Bajhaiya, A. P. Dean, L. A. H. Zeef, R. E. Webster and J. K. Pittman, Plant Physiol., 2016, 170, 1216–1234 CrossRef CAS PubMed.
- A. Vlysidis, M. Binns, C. Webb and C. Theodoropoulos, Biochem. Eng. J., 2011, 58–59, 1–11 CrossRef CAS.
- N. Eriksen, F. Riisgård, W. Gunther and J. Lønsmann Iversen, J. Appl. Phycol., 2007, 19, 161–174 CrossRef CAS PubMed.
Footnotes |
† Electronic supplementary information (ESI) available. See https://doi.org/10.1039/d2se00124a |
‡ Currently at Chemical and Process Engineering, University of Strathclyde, Scotland, UK |
|
This journal is © The Royal Society of Chemistry 2022 |