DOI:
10.1039/D2SD00038E
(Communication)
Sens. Diagn., 2022,
1, 465-468
Single-channel digital LAMP multiplexing using amplification curve analysis†
Received
6th March 2022
, Accepted 9th April 2022
First published on 11th April 2022
Abstract
Loop-mediated isothermal amplification assays are currently limited to one target per reaction in the absence of melting curve analysis, molecular probes or restriction enzyme digestion. Here, we demonstrate multiplexing of five targets in a single fluorescent channel using digital LAMP and the machine learning-based method amplification curve analysis, resulting in a classification accuracy of 91.33% on 54
186 positive amplification events.
Nucleic acid amplification tests for diagnosis and epidemiological surveillance of infectious disease are essential in the fight against outbreaks such as the ongoing COVID-19 pandemic. In addition to the gold standard polymerase chain reaction (PCR), loop-mediated isothermal amplification (LAMP) has become a popular alternative due to its high sensitivity, specificity, and rapidness. Although numerous LAMP assays have been developed in the last two decades, they have commonly been restricted to detect one target per reaction, limiting the throughput of technologies that rely on LAMP. Several methods have been employed to increase the number of targets in a single LAMP reaction, including: (i) fluorescence-based detection at different excitation wavelengths through the incorporation of a specific quencher–fluorophore pair per each target,1–7 (ii) DNA restriction enzyme digestion followed by gel electrophoresis,8,9 and (iii) melting curve analysis.10,11 However, probe-based approaches are still limited by the number of fluorescence channels present in the PCR platform and the increased cost of reagents, whereas post-PCR analysis requires more complex instrumentation, longer protocols and exposes the reaction to a greater risk of DNA contamination.12 In an effort to enhance real-time instruments capabilities for identification of multiple target using single channel detection, our team has demonstrated that kinetic information embedded in an amplification curve can be used to distinguish nucleic acid targets.13–17 This novel approach, named as data-driven multiplexing, utilise mathematical algorithms to extract target specific features from real-time amplification data which can be used as input to a classifier. In particular, this work explores the use of the amplification curve analysis (ACA) classifier, which consists of a supervised machine learning model (i.e., k-nearest neighbours) using the entire real-time curve from each amplification event. Leveraging on the advantages that digital amplification offers, such as absolute quantification, high resolution and particularly high-throughput,18 this manuscript demonstrates the first application of ACA in digital LAMP (dLAMP) for multiplexing five LAMP assays (5plex-LAMP) in a single reaction with a non-specific intercalating dye (EvaGreen), therefore using a single-fluorescent channel. As a case study, this work focuses on the detection of five respiratory pathogens which present similar flu-like symptoms:19–21 human influenza A virus (IAV), human influenza B virus (IBV), severe acute respiratory syndrome coronavirus 2 (SARS-CoV-2), human adenovirus (hAdV) and Klebsiella pneumoniae (KP). Publicly available assays were used to demonstrate the applicability of the ACA method for multiplexing in dLAMP without lengthy assay optimisation. All primer LAMP sequences used in this study are detailed in Table S1.†10,22–25 The LAMP assay for IAV (targeting M gene) was designed in-house. Performance of the 5plex-LAMP assay was evaluated with a fluorescence-based real-time instrument (LightCycler 96 system, Roche) using a 10-fold serial dilution of synthetic DNA, at concentrations ranging from 1.8 × 107 to 1.8 × 102 copies per reaction (Fig. S1A–E†). All assays amplified their specific target down to 180 copies per reaction. Melting curve analysis was used to confirm the target-specific amplification; obtained melting temperature peak values (Tm) for IAV, IBV, SARS-CoV-2, hAdV and KP were 88.5 °C, 83.5 °C, 86.5 °C, 89.5 °C and 88 °C, respectively (Fig. S1F†). Self-dimer or cross-primer formation was not observed in the non-template control (NTC) during the 35 cycle (1 min per cycle) run.
The 5plex-LAMP was then tested in a digital real-time instrument, dLAMP. In total, 110
880 amplification events were generated including 54
186 positive amplification reactions. Examples of dLAMP real-time amplification curves and time-to-positive distribution obtained with the 5plex-LAMP assay are provided in Fig. S2 and S3,† respectively. Between 6000 to 14
000 positive amplification events were obtained per target, and an adequate number of NTC reactions (N = 6930) were included to verify the absence of contamination, formation of any detectable secondary structure or primer dimerisation. The obtained data was first evaluated by unsupervised machine learning using the uniform manifold approximation and projection (UMAP) method to visualise how distinguishable the amplification curves were per target.26 Classification and clustering considered all available real-time data (in this case, 40 data-point per amplification reaction). After dimensionality reduction into a 3D space (Fig. 1A), it can be observed that amplification curves obtained per each target formed differentiable clusters. Subsequently, supervised machine learning was employed to classify the amplification curves demonstrating the capability of the ACA method for single-channel multiplexing in dLAMP. The selected classification algorithm was k-nearest neighbour (k-NN, with parameter k = 10).13,27 The overall classification accuracy of the ACA method was 91.33% ± 0.33% (mean ± std), represented by the confusion matrix shown in Fig. 1B. In addition, the accuracy, sensitivity, and specificity for the one-vs.-one classifiers is shown in Table 1, which demonstrates that the 5 targets can be distinguished with a classification accuracy ranging from 91.10% to 99.15%.
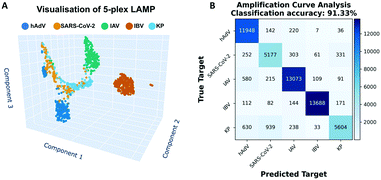 |
| Fig. 1 Performance of the multiplex LAMP assay using the ACA machine-learning based method in real-time digital LAMP. (A) Visualisation of the similarity of real-time LAMP amplification curves using the uniform manifold approximation and projection algorithm. (B) Confusion matrix showing prediction performance of ACA for each of the selected targets in the 5plex-LAMP: human influenza A virus (IAV), human influenza B virus (IBV), severe acute respiratory syndrome coronavirus 2 (SARS-CoV-2), human adenovirus (hAdV) and Klebsiella pneumoniae (KP). | |
Table 1 ACA classification performance by one-vs.-one classifiers
Targets |
Accuracy |
Sensitivity |
Specificity |
hAdV vs. SARS-CoV-2 |
97.40% |
98.74% |
94.69% |
hAdV vs. IAV |
97.22% |
98.32% |
96.25% |
hAdV vs. IBV |
99.15% |
99.88% |
98.51% |
hAdV vs. KP |
97.55% |
99.42% |
94.45% |
SARS-CoV-2 vs. IAV |
97.03% |
94.02% |
98.34% |
SARS-CoV-2 vs. IBV |
98.64% |
98.64% |
98.63% |
SARS-CoV-2 vs. KP |
91.10% |
93.08% |
89.48% |
IAV vs. IBV |
98.96% |
99.30% |
98.63% |
IAV vs. KP |
97.94% |
99.03% |
95.86% |
IBV vs. KP |
98.25% |
97.93% |
98.86% |
Furthermore, we compared these results with two alternative machine learning based methods commonly used for the identification of multiple targets in single-well PCR multiplex assays; final fluorescence intensity (FFI) and melting curve analysis (MCA).15 The obtained classification accuracy of the MCA method was 94.55% ± 0.33% (melting curves distribution and confusion matrix are shown in Fig. 2A and B), which represents a 3.41% ± 0.33% improvement compared to the ACA. Whereas the results obtained with the FFI method reported a classification accuracy of 48.32% ± 0.56% (Fig. 2C), showing a 43.01% ± 0.56% decreased classification accuracy compared to ACA. The FFI values were similar across different assays, and consequently the LAMP mechanism may difficult the classification based on this parameter (Fig. 2D). It is important to note that the 5plex-LAMP has not been optimised for any of the used methods, neither for ACA, MCA nor FFI analysis, therefore obtained results could have been improved. Furthermore, this is the first time FFI has been applied for target identification in LAMP. The combination of ACA and MCA methods, named amplification and melting curve analysis (AMCA) has been previously reported by Moniri et al.15 and Miglietta et al.14 as an approach that combines coefficients from both classifiers improving overall accuracy (as shown in Fig. S4†). As depicted in Fig. 3, all methods except FFI achieved a classification accuracy superior to 90% requiring 103 training data points. Although MCA and AMCA have shown superior performance compared to the ACA, the limitations that MCA impose in terms of accurate thermal control restrict its future use in combination with LAMP, particularly for point-of-care applications.
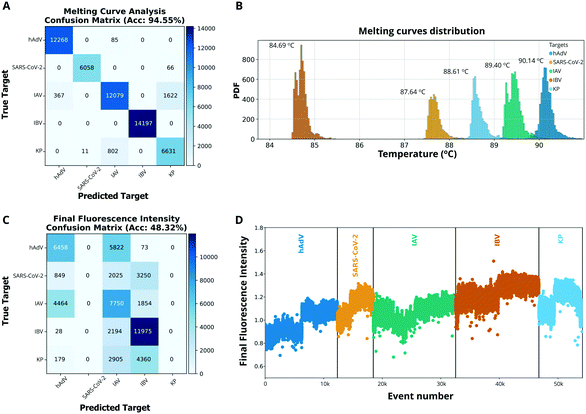 |
| Fig. 2 Performance of melting curve analysis (MCA) and final fluorescence intensity (FFI) machine-learning based methods in real-time digital LAMP. (A) Confusion matrix showing the prediction performance of MCA for each of the targets in the respiratory panel. (B) Melting curve distributions for each target showing the median temperature of the distribution. (C) Confusion matrix showing the prediction performance of FFI for each of the targets in the respiratory panel. (D) Distribution of FFI across the five targets. | |
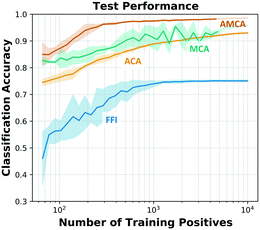 |
| Fig. 3 Effect of training data size on the classification accuracy using 5000 out-of-sample data points (10 iterations). | |
The achieved throughput and turnaround time (<35 min) in a single well reaction leverages target identification accuracy of several pathogens. This proof-of-concept study demonstrates that the ACA method can be used to multiplex LAMP assays using only the amplification curves. No further primer design optimisation, modifications in the reaction, incorporation of molecular probes or accurate thermal cycling are needed. Furthermore, we observed that 5plex LAMP assays did not generate non-specific products (e.g., primer dimerisation). Although there may be a limitation in the maximum number of assays that can be multiplexed in a single well, the 5plex-LAMP used here has proven to be equal or higher than the currently used methods for multiplexing in LAMP which rely on molecular probes,2 melting curve analysis10 or restriction enzyme digestion.9
Notwithstanding the achieved results, limitations to this study include the fact that real-time digital instruments are not commonly available, and therefore, the performance of the evaluated methods for target classification using data from a conventional real-time instrument should be further assessed. This will also require verifying if the trained data is transferable across instruments such that the proposed methodologies could be implemented in conventional real-time instruments, and ultimately in affordable devices for point-of-care diagnostics. Lastly, the conducted experiments for the demonstration of data-driven multiplexing with LAMP only considered the presence of synthetic pure DNA targets. Co-infections are likely to occur, especially in the field of infectious diseases where it is common to find patients presenting more than one disease. The use of dLAMP with single molecule resolution will increase the accuracy in determining the presence of co-infections. This could also be further investigated in future work, as well as the validation of the proposed method with clinical samples to determine its robustness and performance for multiplexing.
Conclusions
We have demonstrated that multiplexing five LAMP assays in a single well reaction using a single fluorescent channel can be achieved with the ACA method with high accuracy, limited assay design, and without the need of downstream experiments. We envision that the proposed method could be applicable for multiplexing any desired LAMP assay at standard laboratory settings enhancing the current testing capabilities, and at the point-of-care once integrated in portable devices that acquire real-time data.
Author contributions
Conceptualization – JRM, KMC and LM; investigation – KMC and LM. Data curation – LM, TP, AM and KMC. Formal analysis and software – LM and TP. Methodology – KMC and LM. Project administration – JRM and KMC. Funding acquisition – JRM, PG and AH. Writing original draft – KMC, LM and JRM. Writing review and editing – KMC, LM, TP, AM, JRM, PG and AH.
Conflicts of interest
There are no conflicts to declare.
Acknowledgements
This work was supported by the Imperial COVID-19 Research Fund (WDAI.G28059); the Department of Health and Social Care-funded Centre for Antimicrobial Optimisation (CAMO) at Imperial College London; the Imperial College Healthcare Trust NIHR Biomedical Research Centre (P80763); the EPSRC HiPEDS CDT (EP/L016796/1 to K. M. C.); and EPSRC Doctoral Prize Fellowship (WDAI_PA2018 to K. M.-C.). Authors AH, PG and JRM are affiliated with the NIHR Health Protection Research Unit (HPRU) in Healthcare Associated Infections and Antimicrobial Resistance at Imperial College London in partnership with the UK Health Security Agency (previously PHE) in collaboration with, Imperial Healthcare Partners, the University of Cambridge and the University of Warwick. The views expressed in this publication are those of the authors and not necessarily those of the NHS, the National Institute for Health and Care Research, the Department of Health and Social Care, or the UK Health Security Agency. AH is a National Institute for Health and Care Research (NIHR) Senior Investigator.
Notes and references
- J. H. Kim, M. Kang, E. Park, D. R. Chung, J. Kim and E. S. Hwang, BioChip J., 2019, 13, 341–351 CrossRef CAS PubMed.
- J. Kim, B. G. Park, D. H. Lim, W. S. Jang, J. Nam, D.-C. Mihn and C. S. Lim, PLoS One, 2021, 16, e0244753 CrossRef CAS PubMed.
- I. Takayama, M. Nakauchi, H. Takahashi, K. Oba, S. Semba, A. Kaida, H. Kubo, S. Saito, S. Nagata, T. Odagiri and T. Kageyama, J. Virol. Methods, 2019, 267, 53–58 CrossRef CAS PubMed.
- N. A. Tanner, Y. Zhang and T. C. Evans, BioTechniques, 2012, 53, 81–89 CrossRef CAS PubMed.
- C. S. Ball, Y. K. Light, C.-Y. Koh, S. S. Wheeler, L. L. Coffey and R. J. Meagher, Anal. Chem., 2016, 88, 3562–3568 CrossRef CAS PubMed.
- L. Becherer, M. Bakheit, S. Frischmann, S. Stinco, N. Borst, R. Zengerle and F. von Stetten, Anal. Chem., 2018, 90, 4741–4748 CrossRef CAS PubMed.
- L. Becherer, N. Borst, M. Bakheit, S. Frischmann, R. Zengerle and F. von Stetten, Anal. Methods, 2020, 12, 717–746 RSC.
- R. Yang, H. Zhang, X. Li, L. Ye, M. Gong, J. Yang, J. Yu and J. Bai, Biosci. Rep., 2018, 38, BSR20180425 CrossRef PubMed.
- L.-L. Zhong, Q. Zhou, C. Tan, A. P. Roberts, M. A. E.-G. El-Sayed Ahmed, G. Chen, M. Dai, F. Yang, Y. Xia, K. Liao, Y. Liang, Y. Yang, S. Feng, X. Zheng and G.-B. Tian, Infect. Drug Resist., 2019, 12, 1877–1887 CrossRef CAS PubMed.
- J. Mahony, S. Chong, D. Bulir, A. Ruyter, K. Mwawasi and D. Waltho, J. Clin. Virol., 2013, 58, 127–131 CrossRef CAS PubMed.
- N. Liu, D. Zou, D. Dong, Z. Yang, D. Ao, W. Liu and L. Huang, Sci. Rep., 2017, 7, 45601 CrossRef CAS PubMed.
- Z. K. Njiru, A. S. J. Mikosza, T. Armstrong, J. C. Enyaru, J. M. Ndung'u and A. R. C. Thompson, PLoS Neglected Trop. Dis., 2008, 10, 1371 Search PubMed.
- A. Moniri, L. Miglietta, K. Malpartida-Cardenas, I. Pennisi, M. Cacho-Soblechero, N. Moser, A. Holmes, P. Georgiou and J. Rodriguez-Manzano, Anal. Chem., 2020, 92, 13134–13143 CrossRef CAS PubMed.
- L. Miglietta, A. Moniri, I. Pennisi, K. Malpartida-Cardenas, H. Abbas, K. Hill-Cawthorne, F. Bolt, E. Jauneikaite, F. Davies, A. Holmes, P. Georgiou and J. Rodriguez-Manzano, Front. Mol. Biosci., 2021, 8, 1–11 Search PubMed.
- A. Moniri, L. Miglietta, A. Holmes, P. Georgiou and J. Rodriguez-Manzano, Anal. Chem., 2020, 92, 14181–14188 CrossRef CAS PubMed.
- A. Moniri, J. Rodriguez-Manzano, K. Malpartida-Cardenas, L. S. Yu, X. Didelot, A. Holmes and P. Georgiou, Anal. Chem., 2019, 91, 7426–7434 CrossRef CAS PubMed.
- J. Rodriguez-Manzano, A. Moniri, K. Malpartida-Cardenas, J. Dronavalli, F. Davies, A. Holmes and P. Georgiou, Anal. Chem., 2019, 91, 2013–2020 CrossRef CAS PubMed.
- Y. Yu, Z. Yu, X. Pan, L. Xu, R. Guo, X. Qian and F. Shen, Analyst, 2022, 147, 625–633 RSC.
- X. Zhu, Y. Ge, T. Wu, K. Zhao, Y. Chen, B. Wu, F. Zhu, B. Zhu and L. Cui, Virus Res., 2020, 285, 198005 CrossRef CAS PubMed.
- Y. Li, H. Wang, F. Wang, X. Lu, H. Du, J. Xu, F. Han, L. Zhang and M. Zhang, Medicine, 2021, 100, e24315 CrossRef CAS PubMed.
- N. Zhang, L. Wang, X. Deng, R. Liang, M. Su, C. He, L. Hu, Y. Su, J. Ren, F. Yu, L. Du and S. Jiang, J. Med. Virol., 2020, 92, 408–417 CrossRef CAS PubMed.
- L. L. M. Poon, C. S. W. Leung, K. H. Chan, J. H. C. Lee, K. Y. Yuen, Y. Guan and J. S. M. Peiris, J. Clin. Microbiol., 2005, 43, 427–430 CrossRef CAS PubMed.
- D. Dong, W. Liu, H. Li, Y. Wang, X. Li, D. Zou, Z. Yang, S. Huang, D. Zhou, L. Huang and J. Yuan, Front. Microbiol., 2015, 6, 1–6 Search PubMed.
- F. Li, L. Zhao, J. Deng, R. Zhu, Y. Sun, L. Liu, Y. Li and Y. Qian, Zhonghua Erke Zazhi, 2013, 51, 52–57 Search PubMed.
- J. Rodriguez-Manzano, K. Malpartida-Cardenas, N. Moser, I. Pennisi, M. Cavuto, L. Miglietta, A. Moniri, R. Penn, G. Satta, P. Randell, F. Davies, F. Bolt, W. Barclay, A. Holmes and P. Georgiou, ACS Cent. Sci., 2021, 7, 307–317 CrossRef CAS PubMed.
-
L. McInnes, J. Healy and J. Melville, ArXiv e-prints, last revised 18 Sep 2020 (this version, v3).
- Z. Zhang, Ann. Transl. Med., 2016, 4, 218 CrossRef PubMed.
|
This journal is © The Royal Society of Chemistry 2022 |