DOI:
10.1039/D2SD00022A
(Paper)
Sens. Diagn., 2022,
1, 810-820
Automated passive serial dilution microfluidic chip for calcium quantification based on the Arsenazo III method†
Received
1st February 2022
, Accepted 5th June 2022
First published on 13th June 2022
Abstract
In this study, a valveless, resistance-based method was used to design and develop a passive microfluidic chip to implement an automated calcium assay in urine samples using the Arsenazo III method with a 3D printed mold. The resistive design of the channels provides a precise mixing in the assay at a ratio of 250
:
25
:
2 for water, reagents, and samples, respectively, with an added advantage of reduced process cost due to the 3D printed mold. The reaction between Arsenazo III and calcium in the urine results in a purple-colored complex that is quantified using a visible light source spectrophotometer, a portable fiber-optic spectrophotometer with a UV (ultraviolet) source and a smartphone camera capable of capturing RGB values. A linear range from 1.62–9.59 mg dL−1 (limit of detection: 0.205 mg dL−1) for calcium concentration in urine was obtained using a visible light spectrophotometer with R2 = 0.99. Similarly, the linear range obtained for the portable spectrometer and smartphone camera was 1.62–6.50 mg dL−1 with R2 = 0.93 and R2 = 0.97, respectively. The chip fabrication method and assay were found to be highly reproducible (σ < 0.001). The approach becomes highly portable with the use of a smartphone camera for quantification. The proposed microfluidic system can successfully quantify calcium in a relevant range in urine samples and is sensitive enough for an automated urinalysis system inside a smart toilet. Additionally, it should also be applicable to other assays by changing channel resistance according to the desired dilution or mixing ratio.
1. Introduction
Urinalysis is the first step for diagnosis and detection of various kidney-related diseases, drug consumption, toxic substances or pharmaceutical components, and the possibility of exposure to such chemicals.1–4 The diagnosis involves checking the appearance, concentration, and content of urine samples by the process of sample collection, preparation, and quantification with necessary reagents.5 For example, the quantification process of calcium in a urine sample conventionally uses an Arsenazo III complex assay. In this assay a urine calibrant is diluted in DI water to create a range of samples with different calcium concentrations to form a calibration curve based on biochromatic detection.6 An unknown sample can then be processed identically and measured.
Several researchers have indicated an interest in developing a “smart toilet”, or a toilet that would automatically process urine samples in the toilet and report the health status of the user. A smart toilet would include point-of-care (POC) devices would enable rapid processing of biological samples with quick and precise diagnosis and detection of many diseases. One approach to POC systems is to incorporate microfluidic devices to handle fluid preparation, transfer and analysis in diagnostic platforms. The capacity for absolute control over geometry, high repeatability, fast reaction times, and reduced consumption of expensive reagents in microfluidic system can facilitate multiple tests on the same POC platform and render the system cost-effective, portable, and efficient.7–9 Various microfluidic chips have been presented in the literature for performing the sample preparation step in urinalysis10,11 for the detection and measurement of glucose,12 urinary proteins,13–15 hormones,16 biomarkers for cancers,17 leukocytes,18 nitrate, urinary ketones and electrolytes.19,20 The primary requirement for such a microfluidic system is maintaining precise mixing of calibrants in a specific ratio. These platforms utilize motive forces, including pneumatic,21 droplet,22 centrifugal,23 and digital microfluidics24 for performing functions like pumping, valving, and mixing. The network's complexity increases with the number of chemical reagents in the assay and mechanical components in the device. Such platforms could require multiple flow sources and become challenging to operate, repair and maintain. Another requirement of such urinalysis is precise mixing of reagents and several methods are presented in literature25–27 for a pressure-driven microfluidic system in terms of achieving that. In a traditional soft-lithography manufactured device, the microfluidic chip contains valves, channels, and mixing chambers where an external pump or pneumatics controls the pressure, valving, and flow rate.28,29 The on-chip valves operate based on the applied pneumatic force and provide advantages of precise fluid metering, minimal reagent usage, adaptability, and low operation time. However, integrating on-chip valves makes the design complex and usually requires multiple fabrication layers to operate. Increased complexity in design increases fabrication cost, and manufacturing scaleup becomes limited to flexible materials like PDMS. Another method is to control the flow by a combination of stepper motors and a chip with a syringe cartridge-like design. The cartridge meters and mixes the fluids in a chamber, which is then analyzed.30,31 While this method can provide exact metering in a valve-less system, the hardware's complexity and cost are higher at the expense of a simple chip design. Hence in this study, we demonstrate a passive resistive network microfluidic device with a simple design for calcium analysis in urine that would be easy to implement in a smart toilet using only the local pressure and water source and does not require any valves or metering for precise control over mixing ratios.
In the proposed device, a pressure-driven network design approach consisting of a resistive-valveless circuit system32,33 is used. It utilizes a resistive serial dilution network where each branch has a fixed hydraulic resistance equivalent to electrical resistance in an electrical network, which produces specific flow rate conditions in each inlet channel, assuming a uniform pressure input.34 The design of such systems enables analysis similar to that of an electrical circuit, leading to a microfluidic design without valves. This method provides straightforward hardware at the expense of a more calculation-heavy chip design and the need for more precise and consistent chip manufacturing. However, once the circuit calculations are made, chip operation and fabrication are straightforward. This solution mitigates the aforementioned limitations of other methods and produces the required dilution ratios by applying equal pressure to all inlet fluids. Mixing ratios can be obtained for a wide range of assays with different dilution ratios. Such networks are yet to be explored for urinalysis. If assays of this type are to be used in smart toilets, simple manufacturing and operation, reusability, and low reagent usage will be required to make them practical. These passive resistive designs may be the best solution.
In this paper, a calcium Arsenazo assay is demonstrated with a minimal footprint fluid handling system for urinalysis utilizing resistive flow circuits to precisely meter and mix a sample with buffer and reagents for subsequent analysis. The design includes three inlets and one outlet, applying a constant input pressure of 34.5 kPa (5 psi). The mixing ratio was targeted to achieve 25(reagent)
:
225(DI)
:
2(sample) volumes. This design's primary advantages include simple chip operation, passive hardware, a readily reusable and low-cost chip, easily adaptable design elements for incorporating other urinalysis assays, low reagent consumption, and well-established chip manufacturing methods. The chip was fabricated with a 3D printed mold35 which enables precise chip manufacturing capable of minimal reagent use. The performance of the system was quantified by measuring the absorbance of calcium Arsenazo III complex bichromatically at 650 nm using a visible light source spectrophotometer and a fiber optic-based portable spectrophotometer for a range of urine samples with different calcium concentrations. Moreover, using a smartphone camera as a quantification method was also evaluated for the urinalysis chip. As a result, we demonstrate a fully functional fluid handling chip capable of continuously processing a calcium Arsenazo assay on urine samples. The results show a highly linear and robust method for calcium analysis in urine samples. The linear range achieved is suitable for implementing the chip in an automated urinalysis system connected to a toilet bowl or a “smart toilet.” With a built-in optical data acquisition capability, continuous monitoring using this assay could readily be accomplished. The demonstrated chip could represent a class of urinalysis chips readily implementable into a “smart toilet.”
2. Materials and methods
2.1 Analytical design based on passive-resistive circuit method
The implementation of the required mixing ratio for the calcium Arsenazo assay without any valves or volume controls requires a careful flow resistance analysis to ensure the proper dilution and mixing ratios. A common approach is to use the analogous behavior of hydraulic and electric circuits based on the Hagen–Poiseuille equation and Ohm's law.32 In this analogy, the hydraulic network's pressure correlates with the electric circuit's voltage, volumetric flow rate correlates to current, and the hydraulic resistance to the electric resistance, assuming that the flow is laminar, viscous, and incompressible. Though such a method does not account for transient flow, this analogy is applicable for non-circular channels and can provide a good estimate when designing nearly one-dimensional channels.
Fig. 1a) shows a schematic of the hydraulic network designed for calcium quantification in urine and implements the resistive circuit method. The system has a total of three inlets to supply the calcium sample, the calcium Arsenazo reagent, and DI water for dilution. To determine the calcium concentration, a two-step mixing process is required in the network – first diluting the reagent with DI water and second, mixing the urine sample with the diluted reagent. The mixing ratio should be maintained as 225
:
25
:
2 (DI
:
reagent
:
sample). Fig. 1b) shows a proposed layout of the unit chip containing the network, and 1c) shows the analogous passive electric circuit diagram. In Fig. 1b) and c), the reagent, DI, and sample inlets are labeled as points A–C, respectively. The outlet is labeled as point F. The advantage of this resistive pressure balanced system is that only one pressure source (pneumatic pump/syringe pump/compressed air/household plumbing) will be needed at all three inlets. Any instabilities from the pressure source will be synchronized and will not impact the dilution ratio of the different mixing stages.
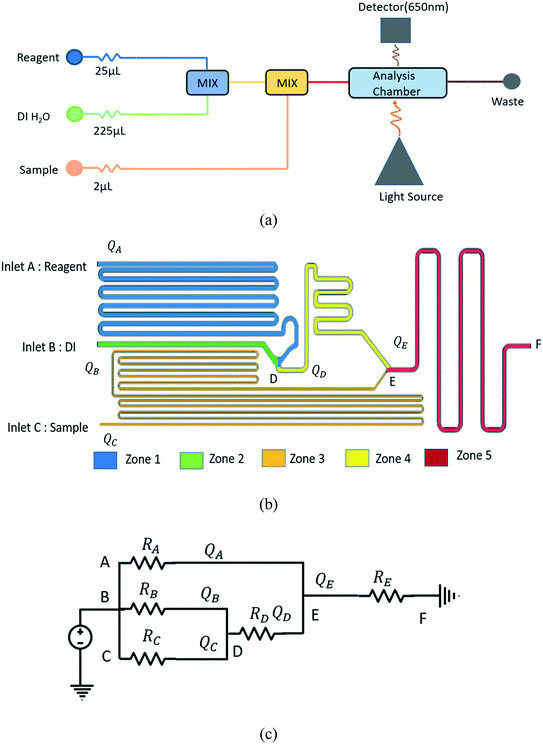 |
| Fig. 1 (a) A schematic of the resistive-circuit method with three inlets for DI water, reagent and sample. The mixed fluids are transferred to a detection chamber. (b) The proposed design of the chip that generates a dilution ratio of DI : reagent : sample = 225 : 25 : 2. The different flow areas are divided in to five different zones. (c) The microfluidic network hydraulic resistance analogous electrical circuit. | |
From Fig. 1b), it can be observed that the design is divided into five zones. Zone 1 is between inlet A and point D, zone 2 is between inlet B and point D, zone 3 is between inlet C and point E, zone 4 is between point D and point E, and zone 5 is between point E and point F. Each zone has its own hydraulic resistance and flow rate. Zone 4 starts after point D, where streams from zone 1 and 2 mix. The hydraulic resistance of this zone is represented as RD shown in Fig. 1c). Zone 5 starts when the fluid streams from zone 3 and zone 4 merges at point E. The variables representing volume flow rate and hydraulic resistances of each zone are presented in Fig. 1c). The three inlets have different fluids, but are all connected to the same pressure source. Even with the same pressure, the flow rates in each channel will be different depending on the channels' hydraulic resistance, and the resistances are designed to generate the desired fluid mixing ratios. The analogous electric circuit of the chip with the estimated hydraulic resistance can be seen in Fig. 1c). Here, the resistance ratios are to be maintained as RA
:
RB = 25
:
225 and
. Using these ratios, the flow rate at point D becomes QD = QA(1 + DR−1) and E QE = 126/125 QA ( 1+ DR−1). Here, DR is the dilution ratio at point D, and DR = 25
:
225.
Knowing the dilution ratios, channel dimensions can be generated that should provide the needed DR. The relationship between the pressure drop in a rectangular channel Δp and the volumetric flow rate Q for a pressure-driven, laminar flow of an incompressible and uniform viscous Newtonian fluid can be described by the Hagen Poiseuille's law.
RCh [Ps s
3 m
−1] is the hydraulic resistance and can be described by the following equation for non-circular channels.
|  | (2) |
Here,
L is the channel's length,
η is the dynamic viscosity of the fluid [s Pa
−1] and
rH is the hydraulic radius. For a rectangular channel, the hydraulic radius is

where
w and
h are the width and height of the microfluidic channel. This equation provides an approximate estimation with up to 20% error for a square cross-section.
32 The hydraulic resistance is constant for a homogenous viscous fluid and continuous channel cross-section. The equation implies that the hydraulic resistance is directly proportional to the channel length and inversely related to the hydraulic radius, suggesting that by changing the channel's geometry, the flow rate, and pressure drop through the channel can be controlled precisely.
The initial design of the microfluidic chip was developed using eqn (1) and (2) to constrain the channel geometries. Once a proposed design was created, the geometry of the model was implemented in SolidWorks, and 3D modeling, simulation, and optimization were performed using COMSOL Multiphysics to determine a final design that considered the 3D nature of the flow and compensated for any error caused by the hydrodynamic radius usage. The dilution ratio was then calculated using the obtained flow rate at the inlets and outlet. The geometry was adjusted until the desired dilution ratio was achieved, and a test chip was then fabricated.
2.2 Simulation model and optimization
The proposed chip was simulated using COMSOL Multiphysics for the simulation, a laminar, incompressible flow was assumed where the Reynolds number was well within the laminar range (Re ≪ 100). The fluid flow is described by the Navier–Stokes equation provided below: | ρu·∇u = −∇p + ∇·μ(∇u + ∇u)T) | (3) |
Here, ρ is the density (kg m−3), u the velocity (m s−1), μ denotes the viscosity (N s−1 m−2), and p presents the pressure (Pa). The model fluid is water with a viscosity of 1 × 10−3 N s−1 m−2 and a density of 1000 kg m−3. At the inlets, a parabolic velocity profile was assumed. The pressure at the outlet was zero, and the no-slip condition was applied.
The mass flux is given by diffusion and convection, and the mass balance equation is:
where
D is the diffusion coefficient (m
2 s
−1), and
c is the concentration (mol m
−3). Here a diffusion coefficient of 1 × 10
−11 m
2 s
−1 was used. The concentration of the fluid at the inlets are regarded as C
1 = 1.00 mol m
−3 and
C2 = 0 mol m
−3 for various conditions. As an example, for mixing at point D in
Fig. 1b), the concentration at inlet A and B was assigned
C1 = 1.00 mol m
−3 and
C2 = 0 mol m
−3 respectively. The mesh size was set to normal, with a maximum element size of 0.205 mm and a minimum element size of 0.0463 mm. The total mesh elements generated for the design was 292
![[thin space (1/6-em)]](https://www.rsc.org/images/entities/char_2009.gif)
954.
2.3 Device fabrication
The fabrication process of the device is shown in ESI† Fig. S1. The device was made using a 3D printed mold (Xometry, Bethesda, MD) and PDMS casting over the mold to create the microchannels. The material for the mold was Accura ABS Black (SL 7820) which cures PDMS without any reaction. The mold dimension was 79.00 mm × 53.97 mm × 4.00 mm. The cured PDMS layer thickness was 2 mm and achieved by placing 7.79 grams of PDMS in the mold (Slygard 184, PDMS: curing agent = 10
:
1). The cast was removed from the mold, and vias were created in the inlet and outlet locations using a 2 mm biopsy punch. The PDMS cast was then bonded to a glass slide (methanol washed glass, 50 mm × 75 mm × 1 mm) using standard air plasma activation.
2.4 Calcium assay
2.4.1 Calcium Aresenazo III quantification process.
The Arsenazo III complex assay and chemistry calibrant were used for calcium quantification in urine samples (Beckman Coulter DR0090). During the quantification process, calcium ions (Ca2+) react with Arsenazo III and form a complex. The absorption of the complex is determined at 650/700 nm wavelength. The measured absorption is directly proportional to the concentration in the sample. The urine calibrant was diluted in DI water to create a range of samples (1.69 mg dL−1 to 9.59 mg dL−1) with different calcium concentrations. The reaction is described by:
2.4.2 Quantification by spectrophotometer.
In absorption spectroscopy, light passes through a sample and is measured. The absorption coefficient is determined |  | (6) |
where, IT is the light intensity transmitted through the sample and I0 is the intensity transmitted through the blank.
The absorption A is related to the sample's concentration c and medium-specific molar absorptivity ε, and the path-length d by using Beer–Lambert's Law36
The relation between absorbance and concentration is not always linear because, with an increase in the concentration, the intra-molecular interaction affects the sample's absorptivity. Moreover, the refractive index of the sample may change with concentration.
2.4.3 Quantification by colorimeter.
Imaging devices like CCD and CMOS sensors detect light similarly to the way the human eye does. They register color as the intensity of three primary colors, red, green, and blue, where wavelengths are presented as RGB vectors. The spectral sensitivity of the devices to detect the RGB vector can vary depending on sensors and manufacturers. Considering the device's spectral sensitivity, the absorption can be calculated using Beer–Lamberts law.37 However, without specialized equipment, the sensitivity coefficient for any particular device is difficult to acquire, and an alternative approach for measuring absorbance has been suggested,38 where the relative absorbance is: | 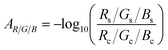 | (8) |
where s and c indicate the normalized values of the sample and blank, respectively.
2.5 Microfluidic device testing
The Arsenazo III assay was performed in the microfluidic chip. The constant low-resistance pressure set up for maintaining the pressure at 34.45 kPa (5 psi) is shown in Fig. 2. Due to the design of the chip, any microliter volume of the assay can be processed if given an appropriate amount of time. Once pressurized, the fluids flow into the chip and are continuously metered and mixed. The analysis can then occur on or off-chip, depending on the needs of the experiment. Once the reacted sample's desired volume is obtained, the chip is washed by flowing 1 mL of DI water through the channels. The desired volume for off-chip quantification is passed through a spectrophotometer to measure absorbance after collection. The observation window created in the design is for on-chip quantification using a portable spectrophotometer or smartphone camera. When the window is filled with a volume of mixed samples, a portable spectrometer and smartphone camera were used to quantify absorbance and RGB values, respectively.
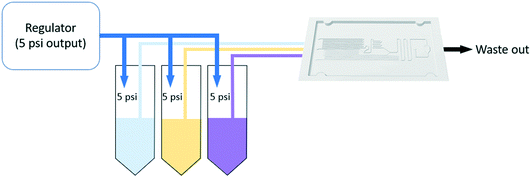 |
| Fig. 2 Experimental set up for constant pressure is shown here. | |
2.5.1 Issues with fabrication of the chip.
The 3D printing of the mold was an important contribution in terms of the fabrication of the chip. There were many issues faced during the printing process that needed to be addressed. The width of the sample channel was 200 μm and SLA printer from Xometry could provide the resolution for the sample channels. One of the main obstacles was the roughness of the mold surface. If the mold surface was not smooth enough, it caused issues with removal of the PDMS from the mold Moreover, the mold material needed to be compatible with the PDMS otherwise the layer does not cure and ruin the mold. After multiple trials, it was found that Accura Black (as produced by Xometry) could provide smooth, PDMS compatible molds that could be used repeatedly.
2.5.2 Off-chip quantification.
A spectrophotometer (WPA Biowave II Life Science Spectrophotometer) was used for sample analysis. For experimental simplicity, 100 μL samples were collected from the chip during operation and loaded into a custom flow cell having a path length of 1.2 mm. The flow cell was constructed from optically transparent polycarbonate, and the design is shown in Fig. 3. The figure represents the design, the cross-section of the flow cell, and the spectrophotometer used for analysis. A calibration curve was obtained using different samples of known calcium concentrations. Control samples were generated manually using standard pipetting techniques and are represented as manual data in the calibration curve. The reacted samples collected from the chip are described as ‘Chip’ in the calibration curve. Here, ‘Chip’ indicates that the reactions took place inside the chip but detected from outside of the chip and is categorized as off-chip quantification.
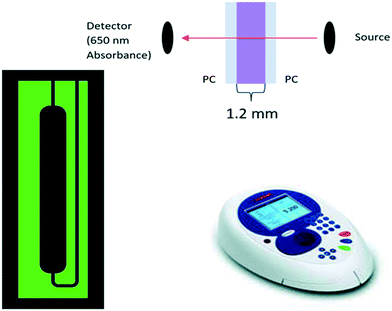 |
| Fig. 3 The flow cell design and cross-section as well as the spectrophotometer and analysis profile. | |
2.5.3 On-chip quantification.
The on-chip analysis of the urine concentration was performed by replacing the spectrophotometer with a portable spectrophotometer having a built in UV source (Ocean Optics USB 2000 +LS 450). The chip was integrated with a 3D printed manifold (Fig. 8b) acting as a gasket to avoid leaking in the PDMS chip. The manifold also incorporates the capability of adding optical fibers to the system for quantification which is suitable for on-chip quantification. An optical fiber connector was placed on both sides of the chip's observation window through the manifold to connect with the spectrophotometer and optical fiber. The quantification was performed using OceanView software which captured the absorbance of the sample at 650 nm. The experimental setup is shown in Fig. 4.
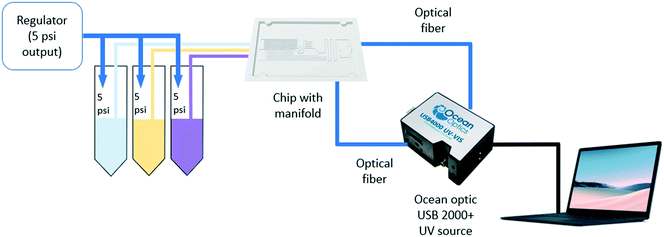 |
| Fig. 4 Experiment set up with Ocean Optics 2000 for on-chip quantification. | |
2.5.4 On-chip quantification with a smartphone camera.
A third on-chip quantification approach was demonstrated with a smartphone camera. The chip was placed inside a white box without the manifold for light reflection with a smartphone camera placed on top of it in a fixed position so that it focuses only on the observation window of the chip. A visible light source was used as an exciting source. The setup can be seen in Fig. 5. The Android application “Colorimeter” was used for capturing the image and generating the RGB value. The absorption coefficient was calculated considering the RGB (100%, 0%, 0%) channel from the obtained RGB values.
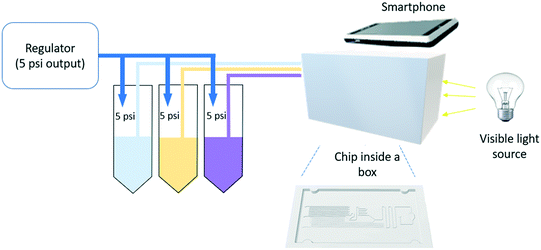 |
| Fig. 5 Experiment set up with smartphone for on chip quantification. | |
3. Results and discussion
3.1 Computation and simulation results
The designed chip's final layout with an observation window can be seen in Fig. 6. A square cross-section was used throughout the chip. The final channel sizes were determined as described in the methods and are presented in Table 1. While these sizes are used here, the chip design can be modified based on the assay and required volume of reagent or sample.
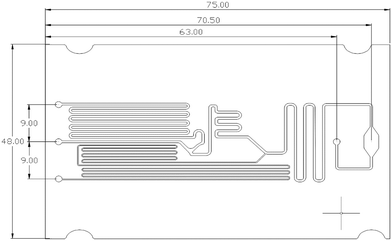 |
| Fig. 6 The final layout of the chip with an observation window. | |
Table 1 The final chip dimensions
Channel dimension |
Dimensions (W × H × L) |
Sample channel |
200 μm × 200 μm × 447.6 mm |
Reagent channel |
500 μm × 500 μm × 245 mm |
DI channel |
500 μm × 500 μm × 28.04 mm |
Mixing channel |
500 μm × 500 μm × 54.6 mm |
The microfluidic channel network was simulated using COMSOL Multiphysics. The results can be seen in Fig. 7. Fig. 7a) shows the concentration profile in mol m−3 for the final design. The concentration in the channel was set to 1 mol m−3 for the reagent, and 0 mol m−3 for DI water and sample. Concentration profiles showed the mixing between the DI water and reagent (point D in Fig. 1b) and can be seen in Fig. 7b. The mixing profile at the intersection (point E in Fig. 1b) was achieved and can be seen in Fig. 7c. Table 2 shows the simulation results, which indicate that for 34.45 kPa (5 psi) of pressure, the ratio of the channel array is close to the desired values (225 ± 0.007
:
25 ± 0.018
:
2) with adequate mixing.
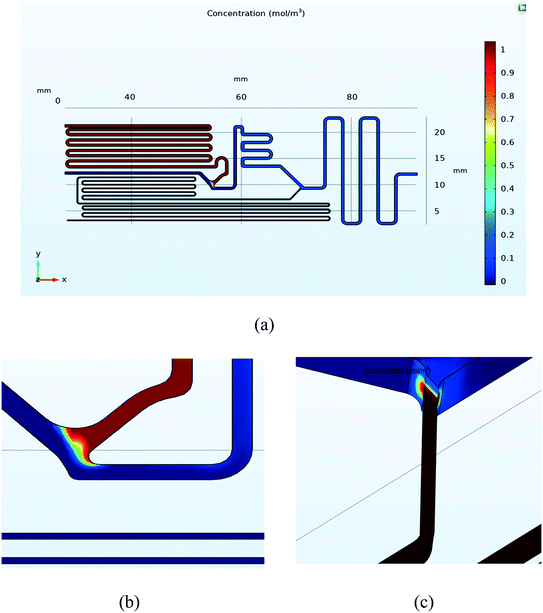 |
| Fig. 7 (a) Concentration profile of the final design considering reagent channel as 1 mol m−3 and other inlets as 0 mol m−3 (b) concentration profile at the intersection of the DI and reagent channels showing proper mixing (c) concentration profile at the intersection of the sample and the DI channels. The results indicate proper mixing at the intersection. | |
Table 2 Simulation results for the final design
Parameters |
Reagent |
Water |
Sample |
Inlet average u [m s−1] |
0.11 |
0.96 |
0.05 |
Inlet flow rate Q [μL s−1] |
26.26 |
239.12 |
2.14 |
Ratio |
24.54 |
223.47 |
2.00 |
Pressure [psi] |
5 |
5 |
5 |
Pressure [kPa] |
34.45 |
34.45 kPa |
34.45 kPa |
3.2 Experimental results
The fabricated microfluidic chip and the 3D printed manifold, shown in Fig. 8. The chip was tested for different calcium concentrations in urine samples (Liquichek-urine chemistry control levels 1 and 2).
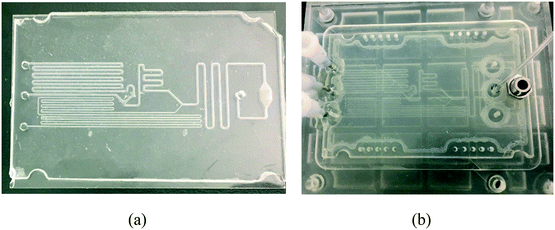 |
| Fig. 8 (a) Fabricated chip (b) the 3D printed manifold with the chip inside. | |
3.2.1 Off-chip quantification results.
The generated calibration curve for off chip quantification is presented in Fig. 9. Data indicate the metering and mixing of the assay by pipetting, and on-chip (using the flow chip) are highly similar. Experiments were replicated across multiple 3D-printed molds and castings to demonstrate fabrication repeatability. Fig. 9a) shows the calibration curve for a maximum calcium concentration of 9.59 mg dL−1 (level 2 of urine calibrant), and the associated data can be seen in Tables SI and SII.† The 3D printed manifold was not used for this experiment. The linear calibration equations for the manual and the on-chip assays in the relevant concentration range are y = 0.0019x + 0.0429 with R2 = 0.953 and y = 0.0023x + 0.048 with R2 = 0.985, respectively. The linear range of concentration detected for the equations ranges from 2.39–9.59 mg dL−1 (limit of detection: 0.932 mg dL−1) of calcium. The lower error bar indicates more repeatable results. Similarly, Fig. 9b) provides the results on a different day experiment with lower calcium concentration of urine sample (level 1) and the chip was inside the 3D printed manifold shown in Fig. 8b) while performing the experiment. The calibration curve is presented for a maximum concentration of 6.5 mg dL−1, and the data is provided in Table SIII and S4.† Both calibration curves follow a linear trend with R2 = 0.999 and R2 = 0.997 for on-chip and manual mixing, respectively. The linear equations for the mentioned conditions are y = 0.0028x + 0.0254 and y = 0.0022x + 0.0283 respectively, this indicates that the chip can be used for linear quantification of calcium in urine samples for the range 1.62–6.50 mg dL−1 (limit of detection: 0.205 mg dL−1). Here, the 3D printed manifold provided better connection of the tubes to the inlets and outlet of chip for which the linear calibration curve of the chip is comparable with the manual one.
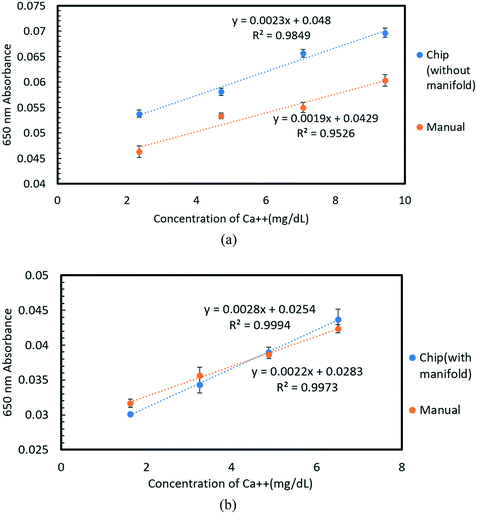 |
| Fig. 9 (a) Calibration curve of the Arsenazo assay for calcium concentrations of 2.39–9.59 mg dL−1. Manual and chip without manifold data show a linear trend. (b) Calibration curve of Arsenazo assay for calcium concentrations of 1.62–6.50 mg dL−1 with a linear trend for both manual and chip with manifold data. | |
3.2.2 On-chip quantification results.
Using the experimental set up presented in Fig. 4, the calibration curve for a urine sample was obtained for different concentrations and the results are presented in Fig. 10, and the maximum calcium concentration tested was 6.50 mg dL−1. The curve shows a linear trend, and the range of calcium concentration in urine samples with the setup is 1.62–6.50 mg dL−1 (limit of detection: 1.255 mg dL−1). The linear equation of the calibration curve is y = 0.0169x + 0.1129 with R2 = 0.93. This indicates that the calcium concentration in urine samples can be detected linearly with a portable spectrophotometer when the sample is in the chip's observation window.
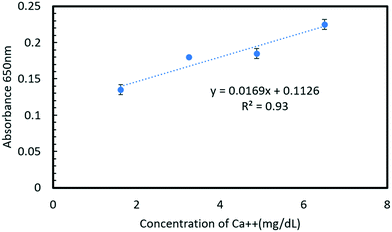 |
| Fig. 10 Calibration curve of Arsenazo assay with on-chip quantification using Ocean Optics 2000 which shows a linear trend. | |
3.2.3 On-chip quantification with a smartphone camera.
Using the set up presented in Fig. 5 for on-chip quantification with a smartphone camera, the calibration curve is presented in Fig. 11. The maximum concentration was 6.5 mg dL−1 and the curve was linear with a fit equation y = 0.0345x–0.0412 with R2 = 0.976, which indicates that data fits the linear model relatively well. Using this approach, we could determine the urine concentration within a range of 1.62–6.50 mg dL−1 (limit of detection: 0.522 mg dL−1) using a smartphone camera. Comparing the data using the smart phone camera with a portable spectrometer, we found that the R2 value is higher for the smartphone camera than the portable spectrophotometer, but with wider error bars, which can be resolved by an improved experimental set up. The smartphone camera data follows a linear trend and could potentially be a suitable alternative to a spectrophotometer.
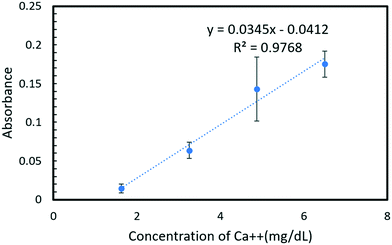 |
| Fig. 11 Calibration curve of Arsenazo assay for on-chip quantification using smartphone camera. | |
4. Conclusion
In this work, a fluid handling system containing a microfluidic chip was designed, built, and tested for precise replication of the standard calcium Arsenazo III urine assay. The system utilizes a simple resistive fluid network concept to precisely meter and mix components of the urine assay. The design favors simplicity in fabrication and operation by placing the metering and mixing requirements in precalculated resistive flow networks that only need one pressure source to run all of the channels, as might be found in a smart toilet results show that the system is more than sufficient to analyze calcium concentrations in a biologically relevant human urine sample range of 1.62–9.59 mg dL−1. Furthermore, the chip performance was demonstrated using three different measurement setups that represent 3 different use cases: a conventional spectrophotometer (for in a lab setting), a portable spectrophotometer (for a POC application or smart toilet), and a smartphone camera (for remote or at home assays). The smartphone camera and portable spectrophotometer show a comparable linear response to the laboratory-based system, suggesting the lab hardware can be easily replaced with a handheld smartphone camera. The proposed design and system are effective for use in a POC platform for calcium quantification in urinalysis. The comparison of different detection methods is provided in Table 3.
Table 3 Comparison of the detection methods
Detection methods |
Spectrophotometer |
Portable spectrophotometer |
Smartphone camera |
Type |
Off-chip- uses a flow chip for detection |
On-chip |
On-chip |
Calcium concentration range (mg dL−1) |
1.62–6.50 |
1.62–6.50 |
1.62–6.50 |
2.39–9.59 |
Slope |
0.002 |
0.02 |
0.03 |
Limit of detection (mg dL−1) |
0.21 |
1.25 |
0.52 |
Pros |
No separate software requirement |
Detection process is easier |
User friendly |
Reduced data variability |
Low data variability |
Does not require specialized equipment |
Detection process is easier |
Cons |
The detection process is laborious |
Requires specialized equipment and software |
Requires additional software |
Data variability depending on the set up |
In the future, because of its simplicity in design, the system is could readily be adapted to other urine assays like protein, albumin, creatinine, glucose, ions, and urea. Moreover, with a modular design, multiple assays could be implemented on one chip. For better on chip quantification with a smartphone camera, advanced software can be used for better quantification. The portable spectrophotometer uses a built-in UV source which can be replaced with a visible source for future experiments to compare its performance with the conventional system. This system is also well suited for scaleup manufacturing because the chip can be made using various low-cost techniques and materials such as injection molding. Overall, the proposed assay chip could enable rapid at home or POC urinalysis.
Author contributions
Conceptualization: Bruce K. Gale, Jeff Campbell, Christopher Jordon Lambert, Dhruv Patel, Mohammad-Hossein Mohammadi Shad, Nusrat Tazin. Methodology: Christopher Jordon Lambert, Dhruv Patel, Nusrat Tazin. Simulation: Nusrat Tazin, Mohammad-Hossein Mohammadi Shad. Resources: Bruce K. Gale, Jeff Campbell. Software: Bruce K. Gale. Supervision: Bruce K. Gale, Jeff Campbell. Validation: Nusrat Tazin, Christopher Jordon Lambert, Dhruv Patel. Investigation: Nusrat Tazin, Christopher Jordon Lambert, Dhruv Patel. Writing – original: Nusrat Tazin. Writing – review & editing: Nusrat Tazin, Christopher Jordon Lambert, Dhruv Patel, Bruce K. Gale.
Conflicts of interest
Jeff Campbell is an employee of Medic.Life.
Acknowledgements
The work was supported by Medic.Life.
References
- M. A. Perazella, The Urine Sediment as a Biomarker of Kidney Disease, Am. J. Kidney Dis., 2015, 66(5), 748–755, DOI:10.1053/j.ajkd.2015.02.342.
- K. E. Moeller, K. C. Lee and J. C. Kissack, Urine drug screening: Practical guide for clinicians, Mayo Clin. Proc., 2008, 83(1), 66–76, DOI:10.4065/83.1.66.
- W. Chen, C. Peng, Z. Jin, R. Qiao, W. Wang, S. Zhu, L. Wang, Q. Jin and C. Xu, Ultrasensitive immunoassay of 7-aminoclonazepam in human urine based on CdTe nanoparticle bioconjugations by fabricated microfluidic chip, Biosens. Bioelectron., 2009, 24(7), 2051–2056, DOI:10.1016/j.bios.2008.10.015.
- W. Su, X. Gao, L. Jiang and J. Qin, Microfluidic platform towards point-of-care diagnostics in infectious diseases, J. Chromatogr. A, 2015, 1377, 13–26, DOI:10.1016/j.chroma.2014.12.041.
- A. J. Callens and J. W. Bartges, Urinalysis, Vet. Clin. North Am. Small Anim. Pract., 2015, 45(4), 621–637, DOI:10.1016/j.cvsm.2015.02.001.
- K. F. Foley and L. Boccuzzi, Urine calcium: Laboratory measurement and clinical utility, Lab. Med., 2010, 41(11), 683–686, DOI:10.1309/LM9SO94ZNBHEDNTM.
- C. Y. Lee, C. L. Chang, Y. N. Wang and L. M. Fu, Microfluidic mixing: A review, Int. J. Mol. Sci., 2011, 12(5), 3263–3287, DOI:10.3390/ijms12053263.
- L. Gervais, N. De Rooij and E. Delamarche, Microfluidic chips for point-of-care immunodiagnostics, Adv. Mater., 2011, 23(24), H151–H176, DOI:10.1002/adma.201100464.
- L. Gervais, N. de Rooij and E. Delamarche, Microfluidic Diagnostic Devices: Microfluidic Chips for Point-of-Care Immunodiagnostics, Adv. Mater., 2011, 23(24), H208, DOI:10.1002/adma.201190098.
- P. Caglar, S. A. Tuncel, N. Malcik, J. P. Landers and J. P. Ferrance, A microchip sensor for calcium determination, Anal. Bioanal. Chem., 2006, 386, 1303–1312, DOI:10.1007/s00216-006-0776-8.
- Y. Boonyasit, T. Maturos, A. Sappat, A. Jomphoak, A. Tuantranont and W. Laiwattanapaisal, Passive micromixer integration with a microfluidic chip for calcium assay based on the arsenazo III method, BioChip J., 2011, 5, 1–7, DOI:10.1007/s13206-011-5101-8.
- S. A. Klasner, A. K. Price, K. W. Hoeman, R. S. Wilson, K. J. Bell and C. T. Culbertson, Paper-based microfluidic devices for analysis of clinically relevant analytes present in urine and saliva, Anal. Bioanal. Chem., 2010, 397, 1821–1829, DOI:10.1007/s00216-010-3718-4.
- K. M. Park, S. K. Lee, Y. S. Sohn and S. Y. Choi, BioFET sensor for detection of albumin in urine, Electron. Lett., 2008, 44(3), 185–186, DOI:10.1049/el:20083551.
- C. C. Lin, J. L. Hsu, C. C. Tseng and G. B. Lee, An integrated microfluidic system for the determination of microalbuminuria by measuring the albumin-to-creatinine ratio, Microfluid, Nanofluidics, 2011, 10, 1055–1067, DOI:10.1007/s10404-010-0734-9.
- T. Songjaroen, T. Maturos, A. Sappat, A. Tuantranont and W. Laiwattanapaisal, Portable microfluidic system for determination of urinary creatinine, Anal. Chim. Acta, 2009, 647(1), 78–83, DOI:10.1016/j.aca.2009.05.014.
- H. Wang, J. Li, X. Zhang, B. Hu, Y. Liu, L. Zhang, R. Cha, J. Sun and X. Jiang, A microfluidic indirect competitive immunoassay for multiple and sensitive detection of testosterone in serum and urine, Analyst, 2016, 141, 815–819, 10.1039/c5an01835h.
- L. G. Liang, M. Q. Kong, S. Zhou, Y. F. Sheng, P. Wang, T. Yu, F. Inci, W. P. Kuo, L. J. Li, U. Demirci and S. Q. Wang, An integrated double-filtration microfluidic device for isolation, enrichment and quantification of urinary extracellular vesicles for detection of bladder cancer, Sci. Rep., 2017, 7, 46224, DOI:10.1038/srep46224.
- D. S. Lee, B. G. Jeon, C. Ihm, J. K. Park and M. Y. Jung, A simple and smart telemedicine device for developing regions: A pocket-sized colorimetric reader, Lab Chip, 2011, 11, 120–126, 10.1039/c0lc00209g.
- N. Malcik, J. P. Ferrance, J. P. Landers and P. Caglar, The performance of a microchip-based fiber optic detection technique for the determination of Ca2+ ions in urine, Sens. Actuators, B, 2005, 107(1), 24–31, DOI:10.1016/j.snb.2004.09.049.
- P. Kubáň and P. C. Hauser, Evaluation of microchip capillary electrophoresis with external contactless conductivity detection for the determination of major inorganic ions and lithium in serum and urine samples, Lab Chip, 2008, 8, 1829–1836, 10.1039/b802973c.
- J. W. Hong, Y. Chen, W. F. Anderson and S. R. Quake, Molecular biology on a microfluidic chip, J. Phys.: Condens. Matter, 2006, 18, S691, DOI:10.1088/0953-8984/18/18/S14.
- K. Iwai, R. D. Sochol, L. P. Lee and L. Lin, Finger-powered bead-in-droplet microfluidic system for point-of-care diagnostics, Proc. - IEEE Annu. Int. Conf. Micro Electro Mech. Syst., 2012, 949–952, DOI:10.1109/MEMSYS.2012.6170343.
- M. J. Madou, L. J. Lee, S. Daunert, S. Lai and C. H. Shih, Design and fabrication of CD-like microfluidic platforms for diagnostic: Mircofluidic functions, Biomed. Microdevices, 2001, 3, 245–254, DOI:10.1023/A:1011419515576.
- V. Srinivasan, V. K. Pamula and R. B. Fair, An integrated digital microfluidic lab-on-a-chip for clinical diagnostics on human physiological fluids, Lab Chip, 2004, 4, 310–315, 10.1039/b403341h.
- D. Mark, S. Haeberle, G. Roth, F. Von Stetten and R. Zengerle, Microfluidic lab-on-a-chip platforms: Requirements, characteristics and applications, Chem. Soc. Rev., 2010, 39, 1153–1182, 10.1039/b820557b.
- S. Haeberle and R. Zengerle, Microfluidic platforms for lab-on-a-chip applications, Lab Chip, 2007, 7, 1094–1110, 10.1039/b706364b.
- G. Cai, L. Xue, H. Zhang and J. Lin, A review on micromixers, Micromachines, 2017, 8(9), 274, DOI:10.3390/mi8090274.
-
S. Haeberle, D. Mark, F. Von Stetten and R. Zengerle, Microfluidic platforms for lab-on-a-chip applications, in Microsystems and Nanotechnology, ed. Z. Zhou, Z. Wang and L. Lin, Springer, Berlin, 2012, pp. 853–895, DOI:10.1007/978-3-642-18293-8_22.
- C. Y. Lee, W. T. Wang, C. C. Liu and L. M. Fu, Passive mixers in microfluidic systems: A review, Chem. Eng. J., 2016, 288, 146–160, DOI:10.1016/j.cej.2015.10.122.
- O. Cakmak, E. Ermek, N. Kilinc, S. Bulut, I. Baris, I. H. Kavakli, G. G. Yaralioglu and H. Urey, A cartridge based sensor array platform for multiple coagulation measurements from plasma, Lab Chip, 2015, 15, 113–120, 10.1039/c4lc00809j.
- S. Smith, P. Madzivhandila, R. Sewart, U. Govender, H. Becker, P. Roux and K. Land, Microfluidic Cartridges for Automated, Point-of-Care Blood Cell Counting, SLAS Technol., 2017, 22(2), 176–185, DOI:10.1177/2211068216677820.
- K. W. Oh, K. Lee, B. Ahn and E. P. Furlani, Design of pressure-driven microfluidic networks using electric circuit analogy, Lab Chip, 2012, 12, 515–545, 10.1039/c2lc20799k.
- N. Vasilakis, K. I. Papadimitriou, H. Morgan and T. Prodromakis, Modular pressure and flow rate-balanced microfluidic serial dilution networks for miniaturised point-of-care diagnostic platforms, Sensors, 2019, 19(4), 911, DOI:10.3390/s19040911.
- C. Kim, K. Lee, J. H. Kim, K. S. Shin, K. J. Lee, T. S. Kim and J. Y. Kang, A serial dilution microfluidic device using a ladder network generating logarithmic or linear concentrations, Lab Chip, 2008, 8, 473–479, 10.1039/b714536e.
- M. de Almeida Monteiro Melo Ferraz, J. B. Nagashima, B. Venzac, S. Le Gac and N. Songsasen, 3D printed mold leachates in PDMS microfluidic devices, Sci. Rep., 2020, 10, 994, DOI:10.1038/s41598-020-57816-y.
- D. F. Swinehart, The Beer-Lambert law, J. Chem. Educ., 1962, 39(7), 333, DOI:10.1021/ed039p333.
- E. P. Moraes, M. R. Confessor and L. H. S. Gasparotto, Integrating Mobile Phones into Science Teaching To Help Students Develop a Procedure To Evaluate the Corrosion Rate of Iron in Simulated Seawater, J. Chem. Educ., 2015, 92(10), 1696–1699, DOI:10.1021/acs.jchemed.5b00274.
- T. S. Kuntzleman and E. C. Jacobson, Teaching Beer's Law and absorption spectrophotometry with a smart phone: A substantially simplified protocol, J. Chem. Educ., 2016, 93(7), 1249–1252, DOI:10.1021/acs.jchemed.5b00844.
|
This journal is © The Royal Society of Chemistry 2022 |