DOI:
10.1039/D1SD00046B
(Critical Review)
Sens. Diagn., 2022,
1, 673-685
Fast tracking of adulterants and bacterial contamination in food via Raman and infrared spectroscopies: paving the way for a healthy and safe world†
Received
8th November 2021
, Accepted 27th April 2022
First published on 4th May 2022
Abstract
Food adulteration adversely affects human health. Therefore, its fast and accurate detection is essentially required to alleviate health concerns. While there are several methods available for the detection of food adulterants, some of them are slow and require large amounts of samples for analysis. Optical spectroscopy methods, especially Raman and infrared (IR), are emerging techniques owing to their fast and accurate detection abilities as well as their ability to examine a very small amount of sample. This paper critically discusses the advancements in the detection of food adulterants using Raman and IR spectroscopies, with a major focus on identifying adulterants in milk, honey, olive oil, coconut water, clove essential oil, and spices (turmeric and saffron). We also discuss the role of Raman and IR spectroscopies in detecting bacterial contamination in food. The fundamentals and application of several Raman and IR-based techniques, namely, surface-enhanced Raman spectroscopy (SERS), Fourier transform (FT)-Raman, point-scan, portable Raman and IR, near and mid-IR, Fourier transform infrared (FTIR), and different associated types of chemometric methods are substantially discussed for the aforementioned applications. Finally, we also propose ways to further advance food security applications via these optical spectroscopy tools.
1. Introduction
Humans' highest hopes are to live a disease-free life and consume high-quality food, but in today's world, these are difficult. More than half of the groceries in the kitchen are adulterated.1 As per the 2018–2019 report released by the Food Safety and Standards Authority of India (FSSAI), 28% of the food samples tested were found to be contaminated or sub-standard in India.2 If humans continued to consume such adulterated food, their health would be severely affected. Adulteration of food is described as the intentional or unintentional substitution with inferior foreign particles in food or the extraction of a value-added food substitute from the main food component. Food adulteration involves injecting harmful chemicals into food, which lowers the quality of the food.3,4
Among the techniques available, optical spectroscopies are a powerful tool owing to their fast, easy, and accurate detection of food adulterants and bacterial contamination in food. Ultraviolet-visible (UV-vis) spectroscopy, fluorescence spectroscopy, IR spectroscopy, Raman spectroscopy, and nuclear magnetic resonance have shown huge potential in this regard. Table 1 shows a brief overview of Raman and IR techniques and their advantages. In this review, we will focus on Raman and IR spectroscopy for the detection of food adulterants and bacterial contamination in food. We will review the fundamentals of Raman and IR spectroscopies, critically review the progress of these techniques for the diagnosis of food adulterants and contaminants, and discuss future prospects.
Table 1 Raman and IR spectroscopies and their advantages
Techniques |
Advantages |
Disadvantages |
Raman spectroscopy |
|
|
1. Dispersive Raman spectroscopy |
Ideal for aqueous samples, samples measured at a higher temperature, etc.5 |
More time-consuming than FT-Raman |
2. FT-Raman spectroscopy |
Reduces fluorescence, simplifies FTIR spectrometer work, and has a high spectral resolution |
Black samples can strongly absorb, heat up, and produce intense background emissions |
3. SERS |
High sensitivity, resolution, and specificity |
A short-range chemical amplification mechanism results in signal suppression6 |
IR spectroscopy |
Fast, non-destructive, and reliable7 |
Some of the bonds, mainly hydrogen bonds such as –C–H, –S–H, –N–H, –O–H, are less intense or overlap |
2. Fundamentals of Raman and IR spectroscopy
Initially introduced in the early twentieth century, vibrational spectroscopy methods such as IR spectroscopy8 and Raman spectroscopy9 have quickly emerged as speedy and non-destructive tools in a variety of sectors. Raman spectroscopy is based on the inelastic scattering of photons by phonons. For molecular vibrations to be Raman active, they must cause a shift in polarizability, a parameter that describes how easily electrons respond to an electric field of light. When a laser beam falls on a sample, some of the photons are inelastically scattered. As a result, the molecule's vibrational energy is altered, and the dispersed radiation is transferred to a new wavelength. A Raman shift is defined as the difference in frequency between scattered and incident radiation. Scattered photons are transferred to longer wavelengths, resulting in a Stokes line in the Raman spectrum.9 Otherwise, the wavelengths are shifted to shorter wavelengths, which leads to an anti-Stokes line. Fig. 1 depicts a Raman scattering energy level diagram. Scattered light frequency shifts can be analyzed and interpreted as spectra. The spectra can serve as a fingerprint for a specific substance, making it easier to analyze this compound in a variety of samples and providing a foundation for structural and qualitative research.5 In recent years, Raman spectroscopy has gained interest in biological measurements because of its rapid and non-destructive analysis, and the requirement of a small amount of sample. Lately, Raman spectroscopy has been upgraded in terms of applications, for example, resonance Raman spectroscopy, surface-enhanced Raman spectroscopy (SERS), tip-enhanced Raman spectroscopy (TERS), etc.10 In SERS, the Raman scattering signals can be enhanced by several orders of magnitude compared to the common Raman scattering. SERS can be employed in food analysis for the detection of thiram, melamine, and ciprofloxacin.
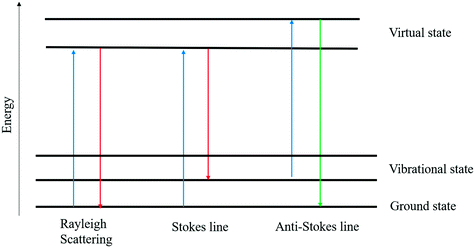 |
| Fig. 1 Light–matter interaction and associated elastic and inelastic scattering. | |
FTIR spectroscopy is a type of vibrational spectroscopy that uses electromagnetic radiation to interact with functional groups in analyzed samples, resulting in vibrational energy levels. FTIR spectroscopy has also emerged as a fast and non-destructive technique for authentication analysis, particularly when using attenuated total reflectance (ATR) and chemometric models. Based on FTIR spectral characteristics, this technique can qualitatively and quantitatively distinguish between authentic and adulterated foods. The IR radiation covers wavenumbers (ν) from 14
000–50 cm−1 with near IR (NIR) from 14
000 to 4000 cm−1, mid-IR (MIR) from 4000 to 400 cm−1, and far IR (FIR) from 400 to 50 cm−1. MIR is the most commonly used method for the detection of functional group authentication among these three spectral regimes.7 The combination of spectroscopy and chemometrics could provide a relatively inexpensive, fast, and non-destructive method for testing food microbiological safety. Fig. 2 shows the basic instrumentation for FT-IR spectroscopy.
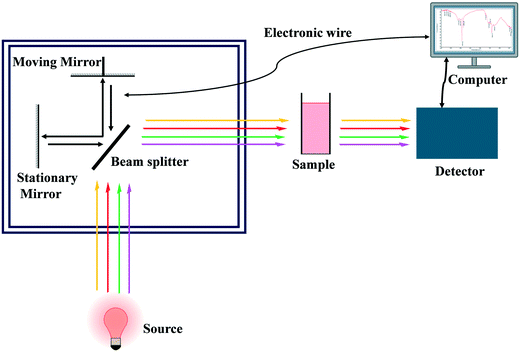 |
| Fig. 2 Schematic diagram of FT-IR spectroscopy. | |
3. SERS
SERS has emerged as a popular tool for analyzing food adulterants and biological samples.11 Jeanmaire and Van Duyne12 conducted pyridine spectroscopy analysis around four decades ago and discovered that using a SERS substrate significantly improved the Raman signals. Generally, analytical approaches that are used to deal with SERS either identify or quantify molecules or both.13 A SERS characteristic is a vibrational fingerprint of the species being studied that can be used to identify it directly.14 Its high sensitivity makes SERS capable of detecting analytes even when their concentration in solution is very low. Recent advances in nanotechnology have made it possible to design, fabricate, and synthesize nanostructures at a breakneck pace.15 In SERS research, the substrate reproducibility problem has also been attempted to be minimized.15,16 Researchers have presented several approaches for substrate preparation, and each of them has its pros and cons. Now, commercial SERS substrates are also available on the market.16 The functioning of SERS substrates and the purity of materials are ensured using Raman peaks.17 SERS is now employed in food analysis for the detection of thiram,18 melamine,19 and ciprofloxacin.20
4. Detection of food adulterants
Adulteration is a major problem worldwide; it is complex to analyze it because adulterated foods are similar to authentic materials.21 Traditional techniques for investigating food adulteration include gas chromatography-mass spectrometer (GS-MS), gas chromatography (GS), high-performance liquid chromatography (HPLC), capillary electrophoresis, ion chromatography, hydrophilic interaction liquid chromatography (HILIC), electrospray ionization mass spectroscopy (ESIMS), etc. These traditional methods require large amounts of solvents and reagents and sample pretreatment, and affect the sample in the process of detection. Additionally, many of these methods are complex, slow, labor-intensive, costly, and not conducive to large-scale applications. By contrast, Raman and IR spectroscopies have shown promise as simple, fast, and non-invasive tools for the identification of adulterants. In this section, we will comprehensively discuss the detection of adulterants in essential food products such as milk, honey, olive oil, coconut water, clove essential oil, and different spices using Raman and IR spectroscopic methods. We will also discuss the applications of Raman spectroscopy, IR spectroscopy, and surface plasmon resonance (SPR) techniques for the detection of bacterial contamination in food.
4.1 Probing the adulterants in milk
Milk is a commonly consumed food with significant nutritional (protein, fat, carbohydrate, vitamins, and minerals) and economic values. The reconstitution of milk is a process that partially or fully replaces raw milk with milk powder. Adulteration is possible in both raw milk and milk powder, requiring fast, easy, and automated methods for detecting the adulterants.22 The major milk adulterants include urea, melamine, boric acid, ammonia, formalin, hydrogen peroxide, benzoic acid, sucrose, dicyandiamide, and ammonium sulfate.23 Kuanglin et al. used a point-scan Raman spectroscopy device with a 16-bit charge-coupled device (CCD) camera to detect melamine in milk.24 The light source for the analysis of the milk sample was a laser (785 nm) with a maximum power output of 430 mW; Raman spectroscopy successfully identified the melamine in the milk sample.24 Zhang et al. reported the application of SERS to detect melamine in milk, whereby a silver-based SERS substrate caused several-fold enhancement of the Raman signals.25 The limit of detection of this method was 0.01 μg mg−1 for melamine samples. The results of this method are as accurate and reliable as those predicted by liquid chromatography/tandem mass spectrometry. Rajapandiyan et al. and Mecker et al. also reported the application of SERS for the detection of melamine in liquid milk and milk powder, whereby the signal was enhanced by employing silver nanoparticles (AgNPs).26,27 Hu et al. reported the detection of melamine in whole milk using a novel biosensor that combines molecularly imprinted polymers with SERS (MIPs–SERS).28 MIPs were found to be effective in separating melamine from whole milk. The signal was collected using a silver dendrite nanostructure that was SERS-active. The Raman signatures of whole milk samples with different melamine concentrations were separated by principal component analysis (PCA) and hierarchical cluster analysis (HCA). The intensity of the melamine peak (at 703 cm−1) was found to have a strong linear relationship with the concentration of melamine from 0.005 to 0.05 mmol L−1. The detection and quantification limits were 0.012 and 0.039 mmol L−1, respectively, demonstrating the biosensor's high sensitivity in detecting melamine in whole milk, as illustrated in Fig. 3.
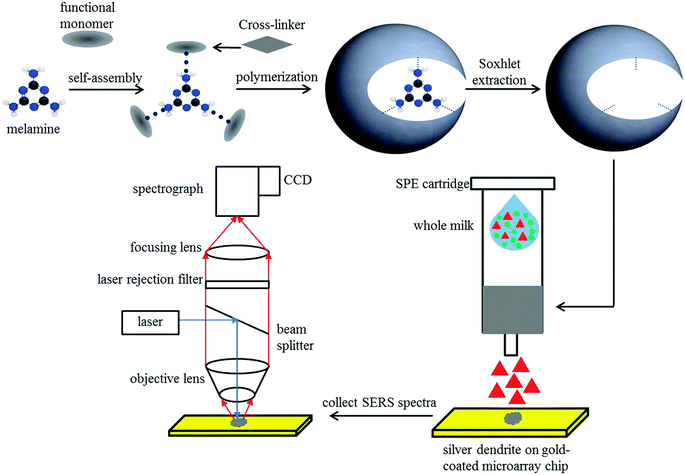 |
| Fig. 3 MIPs–SERS biosensor schematic diagram of melamine detection in whole milk. Reproduced with permission from ref. 28. | |
Almeida et al. employed a combination of Raman spectroscopy and chemometric modeling for evaluating the consistency of milk powder samples.29 Separation methods and other stages of sample preparation are not needed for this approach. As a result, the analysis can be completed easily and without producing waste. Du et al. reported the partial least-squares-discriminant analysis (PLS-DA) model that yielded adequate separation of spectral fingerprints, indicating that FTIR spectroscopy has great potential for controlling milk fraud.22 Jawaid et al. reported the use of the single bounce (SB)-ATR method of FTIR to detect and quantify melamine in both liquid and powdered milk.30 PLS models were developed for the correlation of melamine concentration with spectral results. Cassoli et al. collected samples of milk from 100 farms for the construction of calibration systems.31 Three separate adulterants that are widely used in raw milk adulteration were found in the samples: sodium citrate (SC), sodium bicarbonate (SB), and cheese whey (W), and three different concentrations of each adulterant were studied. The results show that the FTIR methodology can be used to determine the concentration of sodium citrate, sodium bicarbonate, and whey in raw milk. Nicolaou et al. demonstrated that in binary and tertiary milk mixtures, FTIR spectroscopy combined with chemometric models, such as linear, PLS, and nonlinear Kernel PLS, can provide accurate, simple, and rapid detection of adulteration of sheep, goat, and cow milk.32 For all milk species, the average errors were in the range of 5 to 8%. Due to the high speed of analysis (30 s per sample in batches of 96 or 384) and good accuracy, it was concluded that FTIR spectroscopy can substitute less effective and more time-consuming techniques for detecting milk adulterants in the food industry. Furthermore, He et al. used IR techniques in conjunction with a two-dimensional (2D) correlation study to review and analyze adulterants in milk.33 They first recorded the spectra of fresh raw milk and noted characteristic peaks. Subsequently, the adulterated milk samples were tested and their spectra were compared to those of fresh milk, as shown in Fig. 4. 2D correlation spectroscopy based on the intensity, position, and shape of the absorption peaks may be utilized to identify the adulterants in the milk sample.
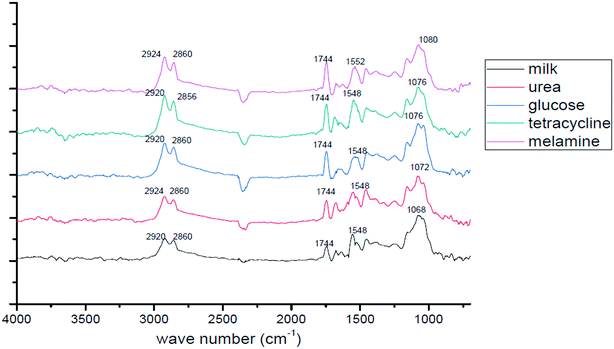 |
| Fig. 4 FTIR absorbance spectra of milk without and with adulterants. Some of the figures were reproduced with the permission from ref. 33. | |
With the help of the PLS algorithm, Raman spectroscopy was utilized for the quantitative identification of urea in milk powder.34 The findings reveal higher accuracy (greater than 90%) and the ability to detect urea levels below the suggested cut-off limit (70 mg dl−1). For the analysis of milk powder samples, Limm et al. used portable MIR spectroscopy as well as NIR spectroscopy combined with non-targeted chemometrics (SIMCA).35 Melamine, dicyandiamide, ammonium sulfate, and sucrose are all detectable in milk using portable Raman spectrometers.36 The capability of low-cost and compact Raman spectrometers for quick screening of sucrose and N-rich molecules provides an efficient way to monitor milk adulterants from farm to factory.37 The excessive use of tetracycline antibiotics in cattle causes contamination in milk; the high level of tetracycline in milk affects human health.37 With the help of the PCA method, Marques et al. used a paper-based SERS platform for the one-step detection of tetracycline.38 They used an aluminum layer deposited with silver nanoparticles as the substrate for the SERS platform. Fig. 5 displays the process for the detection of adulterants using Raman and FTIR spectroscopies.39Table 2 represents Raman and IR-based spectroscopies for the detection of adulterants in milk.
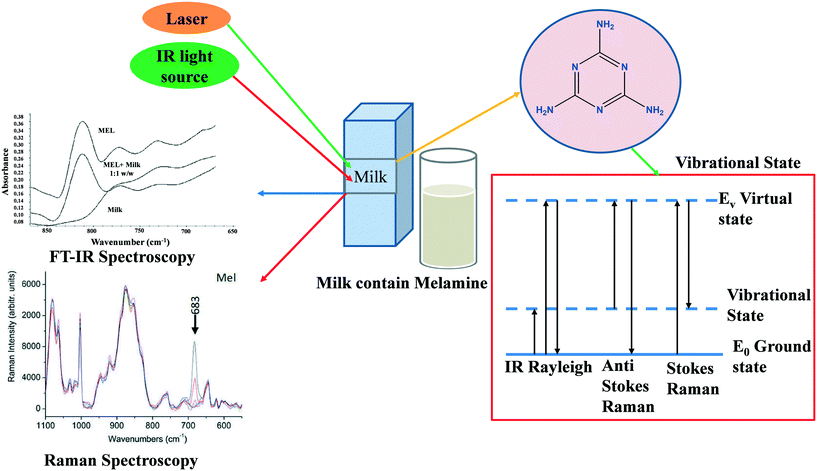 |
| Fig. 5 The schematic diagram for milk adulteration detection using Raman and FT-IR. Reproduced with permission from ref. 39. | |
Table 2 Raman and IR-based spectroscopies for the identification of milk adulterants
Adulterant |
Method for detection |
Chemometrics |
Accuracy/LOD |
Ref. |
Addition of sucrose |
Normal mid-infrared spectra |
For classification: PCA and SIMCA |
100% |
40
|
Quantification using polymerase chain reaction (PCR) and PLS |
Addition of water, starch, sodium citrate, formaldehyde, and sugar |
Mid-infrared-spectra in the range of 4000–600 cm−1 |
PLS-DA |
0.5–10% w/v |
41
|
Addition of melamine |
Normal NIR spectra |
One class partial least squares (OCPLS) |
89% |
42
|
Chloride, citrate, hydroxide, formaldehyde, hydrogen peroxide bicarbonate, carbonate, hypochlorite, starch, sucrose, and water |
MIR from 1000 to 4000 cm−1 |
PCA was used to visualize the sample distribution, and SIMCA was used to classify milk |
93.10 |
43
|
Tetracycline's residue (tetracycline, chlortetracycline, and oxytetracycline) |
MIR (FTIR) from 4000 to 550 cm−1 |
SIMCA for classification, PLS, and PCR for quantification of tetracycline residue |
99% |
44
|
Sodium hypochlorite |
FTIR spectra in the range of 4000–650 cm−1 |
SIMCA for classification |
56.7% |
43
|
Sweet whey |
Raman spectra from 800 to 1800 cm−1 |
ANN for quantification and PLS for corrected prediction |
— |
— |
4.2 Detection of adulterants in honey
Honey is a nutrient-dense food with many medicinal properties, including antibacterial, anti-inflammatory, antioxidant, and cell-growth properties;45,46 it is easy to contaminate it with other sugar syrups and hence the honey loses its purity. Honey has 80% carbohydrate content (glucose, fructose, and sucrose) along with vitamins, minerals, proteins, and amino acids.47 Because of its complexity, honey comes in a wide variety across the world.48 Raman spectroscopy identifies precise bands that characterize a molecular mixture, allowing for high-quality measurements. Özbalci et al. reported the application of Raman spectroscopy to assess the amount of glucose, fructose, sucrose, and maltose in aqueous solutions and commercial honey, and spectral data were analyzed using PCA, PLS, and artificial neural network (ANN) methods.49 These findings show that using Raman spectroscopy in conjunction with suitable multivariate techniques, it is possible to quantitatively evaluate the amounts of glucose, fructose, sucrose, and maltose in honey samples without the use of any treatment or chromatographic methods. Mignani et al. used Raman spectroscopy with an excitation wavelength of 1064 nm to analyze honey adulterants and nutraceutical indicators such as amino acids, proteins, enzymes, and other minerals.50 Here PLS regression was used for analyzing the concentration of the sugars and potassium from Raman spectral bands while PCA was used for data dimensionality reduction and explorative analysis. Oliveira et al. reported the use of FT-Raman spectroscopy to analyze the liquid and crystalline states of honey; they showed that crystallized samples have well-resolved distinctive spectral bands compared to liquid samples.51 Pierna et al. also employed FT-Raman spectroscopy, working with PCL-DA and support vector machines (SVM), for the detection of honey adulterants.52 Li et al. reported the application of Raman spectroscopy to detect the high fructose corn syrup (HFCS) and maltose syrup (MS) in honey; the results yielded a total accuracy of detection of about 84.4%.53 NIR spectroscopy was also used for qualitative and quantitative detection of honey adulterated with HFCS or MS.54 The main variables were chosen using competitive adaptive reweighted sampling (CARS). The adulterated honey samples were classified using PLS-linear discriminate analysis (LDA). The accuracy of the CARS–PLS–LDA models was 86.3% for honey versus contaminated honey with HFCS, and 96.1% for honey versus contaminated honey with MS. Oroian et al. employed Raman spectroscopy combined with PLS–LDA, PLS regression (PLSR), and PCR to detect honey adulterants.55 Guelpa et al. used NIR spectroscopy combined with PLS-DA and found 90% accuracy in the detection of honey adulterants.56 Subari et al. compared the LDA and PCA methods for identifying adulterants in honey and found that the LDA method performs better than the PCA method.57 Basar and Ozdemir investigated honey adulterants (corn syrup, beet sugar, and water) through ATR-FTIR employing genetic algorithm-based inverse least squares (GILS) and PLS analytical techniques and observed that GILS provides a better model compared with PLS.58 Magdas et al. also used Raman spectroscopy with SIMCA and machine learning (ML) approaches to determine the authenticity of honey.59Fig. 6 shows the procedure for the detection of adulterated honey through typical Raman and FTIR spectroscopy with various analysis approaches. Csilla et al. suggest a new method for the preparation of green samples for the detection of honey adulterants using Raman spectroscopy.60 It was proposed that honey dilution with distilled water during sample preparation helps to get reliable and reproducible spectra. Table 3 shows a summary of the results of detection of adulterants in honey using Raman and IR-based spectroscopies.
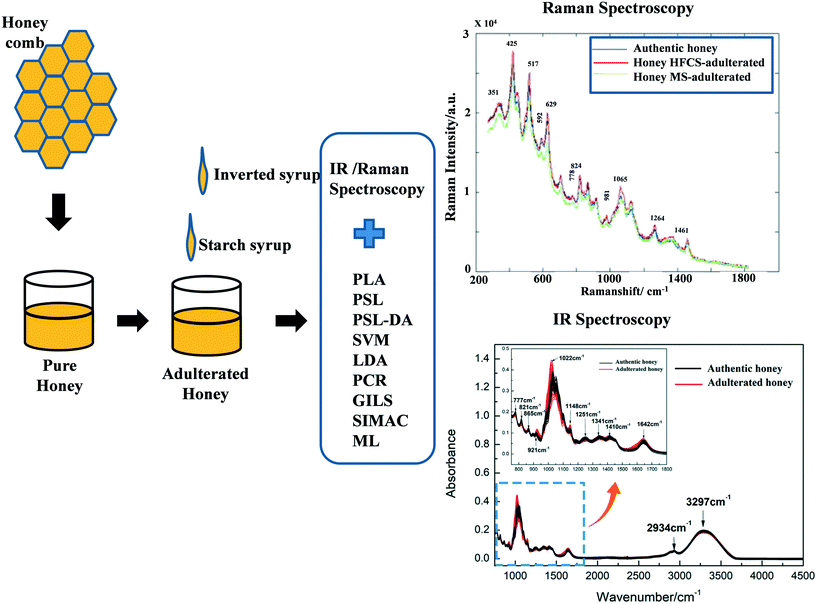 |
| Fig. 6 The schematic diagram shows the procedure for the detection of adulterated honey through typical Raman and FTIR spectroscopy with various analysis methods. Reproduced with permission from ref. 53 and 65. | |
Table 3 Raman and IR-based spectroscopies for honey adulteration detection
Adulterant |
Method for detection |
Chemometrics |
Accuracy/LOD |
Ref. |
Sugar syrup |
FT-MIR spectroscopy in the range of 4000–600 cm−1 |
PCA, HCA, and SVM |
100% |
61
|
HFCS |
Visible NIR spectroscopy |
PCA, HCA, and LDA |
100% |
62
|
Sweetener's materials |
NIR spectroscopy |
PCA, and least squares support vector machine (LS-SVM) |
95.1% |
63
|
Beet syrup, high-fructose corn syrup, partial invert cane syrup, dextrose syrup, and beet sucrose |
IR spectroscopy with MIR spectra from 800 to 4000 cm−1 |
SIMCA, and PLS |
96.2%, 97.5%, 95.8%, and 91.7% |
64
|
4.3 Detection of adulterants in olive oil
Olive oil is made from olives. Saturated fat makes up 14% of the oil, while polyunsaturated fats like omega-6 and omega-3 fatty acids make up 11%. Olive oil's most abundant fatty acid is oleic acid, a monounsaturated fat that accounts for 73% of the total oil content. Extra virgin olive oil (EVOO) is a nutrient-dense oil that is also high in antioxidants. However, a lot of adulteration agents are mixed with olive oil, so pure virgin olive oil is seldom available. An accurate and fast method for identifying the adulterants in olive oil is needed within an hour. Combining FT-Raman spectroscopy with multivariate statistical methods can be a rapid and accurate technique for the detection of adulterants in virgin olive oil. Olive oil is generally contaminated with soybean, corn oil, and olive residue (olive pomace). The PCR and stepwise linear discriminate analysis (SLDA) methods of FT-Raman can be used to distinguish between authentic and adulterated samples.66,67 Similarly, NIR with PCA has been used to accurately identify the adulterants in olive oil.68 Raman spectra of olive oil samples were analyzed on-site and in real-time with a portable Raman spectrometer.69 Authentic olive oil can be separated from olives having 5% or more of other edible oils, based on Raman bands. Also, some researchers have been able to rapidly screen and assess the quality of olive oil with the help of low-resolution portable Raman spectroscopy.70,71 NIR spectroscopy was used for the detection of adulterants in EVOO (adulterated with corn oil in an amount ranging from 2.7 to 25 w/w%).72 Many researchers have used FTIR, FT-Raman, and Raman spectroscopy and chemometric methods to analyze EVOO adulterated with various concentrations of soybean, peanut, olive pomace, and sunflower oil.73–78 These studies demonstrated the potential of optical spectroscopy in detecting olive oil adulterants. Waste cooking oil adulterant for olive oil was identified using Raman spectroscopy and associated chemometric methods such as interval partial least squares (IPLS) and synergy interval partial least squares (SIPLS).79 The root mean square errors of calibration and validation are found to be 0.0503 and 0.0485, respectively. This method is a fast and easy way to predict adulteration in olive oil. In another study, Joshi et al. also reported the detection of an olive oil mixture in argan oil by using portable Raman spectroscopy.80 The analysis of EVOO combined with grapeseed and canola oils at 0–100% was performed using a short distance standoff Raman detection instrument attached to a 785 nm laser source.81 Further, Raman spectroscopy with exponential equations has been used to predict the adulteration in olive oil.82 Exponential equations are used to measure the volume fraction (μ) of adulterants like rapeseed, corn, and sunflower oils in olive oil. Table 4 shows a summary of the results of detection of adulterants in olive oil using Raman and IR-based spectroscopies.
Table 4 Raman and IR-based spectroscopies for the detection of adulterants in olive oil
Adulterant |
Method for detection |
Chemometrics |
Accuracy/LOD |
Ref. |
2% (volume percentage) of three other edible oils, i.e., soybean oil, sunflower seed oil, and corn oil |
Raman spectroscopy |
External standard method (ESM) |
99% |
78
|
Soybean, corn, and raw olive residue (olive pomace) oils at 1, 5, and 10%, respectively |
FT-Raman spectroscopy |
PCR |
91.3% |
67
|
Peanut oil (PEO) |
FTIR with ATR sampling |
PCR, PLS-R, and LDA |
LDA provided 92.3% correct classification for the calibration set; the lowest detection limit for PEO in EVOO was about 0.5% V/V |
83
|
4.4 Detection of adulterants in coconut water and clove oil
Coconut water is a good source of many nutrients, like fiber, protein, magnesium, potassium, sodium, vitamins, and carbohydrate.84 It contains antioxidants that protect the cells from damage caused by free radicals. It also improves blood sugar control, increases insulin sensitivity, prevents kidney stones by reducing stone formation, and helps lower blood pressure. Coconut water can be adulterated with normal water, sugar, corn syrup, and other chemical components.85 The quantification of adulterants was performed using Raman spectroscopy (785 nm excitation source) with chemometric methods, and three types of adulterants were detected, namely, single sugar, mixed sugar, and HFCS.86 FTIR spectroscopy also has the potential to analyze sugars in fruit juices with the help of the PLSR approach.87 Clove essential oil is used for its antimicrobial activity, for preventing cavities, and for relieving oral pain, and it may also be used for its anticancer activity.88 Clove oil is also adulterated with foreign materials due to its high cost. Handheld Raman spectroscopy has been used to detect benzyl alcohol and vegetable oil adulteration in clove oil.89 The FTIR-ATR method was adopted for the detection of adulterants in clove oil.90
4.5 Detection of adulterants in spices
Turmeric is an indispensable ingredient in the Asian kitchen and is used not only as a flavoring agent and seasoning but also as a medicine for treating colds, coughs, ulcers, and various diseases and inflammations. It is also known for its antiviral, antibacterial, antifungal, anticancer, and antioxidant properties. This is mainly due to its high curcumin content. Turmeric is mostly contaminated with metanil yellow, starch, Sudan red, lead chromate, and white turmeric. The long-term use of azo dye (metanil yellow, Sudan red) and lead chromate contaminated turmeric can cause tumors, carcinoma, neurotoxicity, liver diseases, etc.91–98
FT-Raman and IR spectroscopies have proved to be potential tools for the detection of turmeric adulterants. Some of the vibrational spectra of IR spectroscopy are not present in Raman spectroscopy or vice versa, due to molecular symmetric and asymmetric properties. So, for a complete analysis, both IR and Raman spectra are recorded and analyzed. In the case of the white turmeric adulterant, the Raman spectra have no distinct spectral peaks thus the white and yellow turmeric adulteration mixture cannot be detected using the Raman spectroscopy method. However, IR spectroscopy shows a distinct spectral peak (1078 cm−1) in the white and yellow turmeric mixture sample. The positions of the Raman and IR spectral peaks are used to identify the chemical component, and the spectral peak intensity is used to estimate the concentration of the component. The majority of the spectral peaks of turmeric and adulterants overlapped, but some of them which did not overlap act as a fingerprint of adulterants. These fingerprint peaks are utilized to detect and analyze the additives in turmeric powder.91–98 Mostly, FT-Raman and FTIR show the 1406 cm−1 and 1140 cm−1 peaks, respectively, as non-overlapped spectral peaks in turmeric-metanil yellow adulterant samples, which is helpful to detect and analyze the adulterant components and their concentration levels. However, the FTIR fingerprint peak disappears at lower adulterant concentrations, due to the dominance of turmeric. FTIR and Raman show minimal detection levels of 5% and 2%, respectively, of the metanil adulteration.91,95 Erasmus et al. reported lead chromate adulterant detection through FT-Raman with the help of LDA and the PLSR algorithm. This method of detection achieved a 0.5% detection level of the lead chromate concentration level.93 Dhakal et al. proposed Sudan red as an adulterant in turmeric through FTIR with the help of PLSR, and a 748 cm−1 Sudan red fingerprint spectral peak was used to analyze the contamination.94 Recently, NIR and FT-NIR have received attention for the detection of mentanil yellow and adulterants in turmeric; they have achieved a 0.3% concentration detection level with the help of PCR and PLSR chemometric algoritms.97
Saffron (Crocus sativus L.), also known as red gold, is one of the most valuable and expensive spice crops in the world. The majority of consumers place a high value on saffron quality. As a result, assessing the purity and authenticity of a product as well as grading its quality is critical in the saffron trade. Saffron quality is determined by the amount of crocin, picrocrocin, and safranal, which are responsible for saffron's colour, flavor, and aroma.99 The higher the concentration of these compounds, the higher the purity of saffron. However, due to high market demand, saffron contamination with various food colorants (tartrazine, sunset yellow, azorubine, quinoline-yellow, allura red, and Sudan II) and other plant materials (safflower, marigold, and turmeric) is very common.100–105 Eman Shawky et al. employed NIR spectroscopy with the PLSR method to record three different fingerprint spectral regions, which were utilized to achieve the 1% detection limit of different plant adulterants in saffron Table 5.104
Table 5 Detection of adulterants in spices
Spices |
Spectroscopy and chemometrics method |
Adulterants |
Accuracy/LOD |
Ref. |
Turmeric powder |
FT-Raman |
Metanil yellow |
1% |
91, 95 |
FT-IR |
Metanil yellow |
5% |
91, 95 |
FT-Raman-PLSR |
Lead chromate |
0.5% |
93
|
FT-IR-PLSR |
Sudan red |
1% |
94
|
Handheld NIR |
Metanil yellow |
0.33% |
97
|
Handheld FT-IR |
Corn starch |
2% |
106
|
Metanil yellow |
2% |
Sudan dye IV |
2% |
Saffron |
Diffuse reflectance infrared Fourier transform spectroscopy (DRIFTS) with PLSR–DA |
Calendula |
1.9% |
105
|
Safflower |
2.1% |
Turmeric |
1,0% |
NIR with PLSR-VIP |
Plant materials |
1% |
104
|
4.6 Detection of bacterial contamination in food
Foodborne diseases cause significant morbidity and mortality worldwide and hence are a significant public health concern.4,107,108 The growth of microorganisms in food can change its appearance, flavour, odour, and other characteristics. Food quality is affected by the degradative processes caused by microorganisms, such as putrefaction, fermentation, and rancidity, as well as the substances they produce, such as pigments and slimes. Bacterial detection methods that have been used over the years are based on growing bacteria in culture media, isolating bacteria using selective media, and finally identifying bacteria based on morphological, physiological, and biochemical characteristics.109 These conventional methods are labor-intensive and time-consuming, but they produce reliable results. Preliminary identification can take anywhere from 48 to 72 hours, and confirmation of the pathogen species can take up to a week. Because products may degrade while testing results are available, faster tools are necessarily required.110,111 Numerous rapid methods for detecting foodborne bacteria have been developed including immunological, nucleic acid-based, and vibrational spectroscopy. IR and Raman spectroscopy can be used to determine the characteristics of whole organisms based on the vibrational energy of the bonds within functional groups. Increasingly popular in microbiology, vibration spectroscopy offers the advantages of high speed, low cost, and high throughput.112,113 This method can be used as a rapid screening tool for contaminated liquids because it does not require culturing bacteria or amplifying certain genes. A cost-effective, simple, and accurate approach to microbial identification is needed by the food sector to maintain safe products and diagnose contamination.114 SERS is one of the important Raman methods for the analysis of bacterial contamination in food. For SERS to function, no complicated sample pre-treatment process is needed, and no expensive solvent or column is necessary. In comparison with HPLC and GC, SERS is carried out in a matter of seconds. The SERS approach has a lot of potential for the detection and identification of chemical and microbiological contaminants in foods, as well as food quality assessments.115,116 SERS can also be used to detect food-borne microbes, like Salmonella, Staphylococcus aureus, and Escherichia coli, through Raman fingerprinting. The second derivative transformation of the spectra resulted in unique FT-NIR characteristics in the 7000–4000 cm−1 range, allowing PCA to reliably classify the data into discrete clusters.117 Nicolaou and Goodacre were able to use FT-IR ATR and high throughput (HT) techniques as well as multivariate statistical methods like principal components–discriminant function analysis (PC-DFE) and PLSR, to analyse milk samples and estimate microbial loads accurately and rapidly, in under 30 seconds, with minimal sample preparation.118 Assaf et al. experimented with various methods for identifying Salmonella spp. in rice, oats, wheat, maize, poultry, and pork using Raman spectroscopy and following ISO standards.119 Wang et al. used spectral characteristics of the SERS tag to detect S. aureus and S. enterica from spinach and peanut butter.120 Wu et al. employed silver nanorod arrays functionalized with vancomycin substrates to identify Salmonella spp., Escherichia coli, and Staphylococcus epidermidis in mung bean sprouts using Raman spectroscopy.121 The information acquired from the matrix, such as carbohydrates, proteins, and lipid, is highly linked to bacterial growth behavior, according to the SERS study, and chemical fluctuation during biofilm development can disclose whether Gram-positive or Gram-negative bacteria are present.122 Knowing their robustness, their distinctive ability to distinguish isolates based on changes in incubation time and medium, and their accuracy in identifying microbes down to the strain level, FTIR spectroscopy and matrix-assisted laser desorption/ionization time-of-flight (MALDI-TOF) mass spectrometry were examined for their utility and applicability in routine food-related microbiology diagnostics. Wenning et al. investigated the accuracy of detection of a large number of food-related bacteria from an in-house strain collection and different fresh isolates from regular food analysis.114 FTIR and MALDI-TOF produce accurate identification of bacterial strains. Yang and Irudayaraj investigated whole apple surfaces non-destructively using FT-Raman spectroscopy. The five separate strains of E. coli were distinguished with 100% accuracy. FT-Raman can be used for measuring food products directly from the sample or, by using the focused laser, for obtaining the spectra much quicker than FTIR.123 Klein et al. have constructed and demonstrated, with an accuracy of 96.6 percent, a model for discriminating the examined microorganisms at the genus, species, and strain levels.142 This was accomplished despite the addition of diverse stress conditions and at times after the bacteria had been incubated. Zajac et al. successfully showed that rotting chicken meat may be detected using FTIR and Raman spectroscopies.124 The Raman spectrum shows that the protein content (bands at 1655 and 1320 cm−1) decreases during spoiling within 10 days, while the amounts of amino acids (band at 1657 cm−1) increase.124 By using SERS microscopy and silver nanoparticles paired with PCA, L. monocytogenes and Salmonella spp. were found in smoked salmon and ham, Salmonella spp. was found in eggs, and Cronobacter spp. was found in powdered infant formula and mixed herbs.125 There are other optical techniques such as surface plasmon resonance (SPR) that have shown potential for the detection of adulterants and bacterial contamination in food (ref. 126–141), as discussed in detail in the ESI.†
5. Conclusion and future prospects
Raman and IR spectroscopies are simple and fast methods for the detection of food adulterants and bacteria. Different analysis methods have been adopted to discriminate between adulterants and bacteria in samples. While these techniques have shown huge potential for fast and accurate detection, they are not widely commercially deployed so far. One of the reasons for this could be the size of the optical apparatus. Nevertheless, in the recent past, handheld SERS-based Raman spectrophotometers have attracted huge attention, which provide fast and accurate results and avoid the size limitation concern.
While unexpected progress has been achieved over the years on SERS for analytical characterization studies including food analysis, many concerns have yet to be resolved for their widespread commercial application. As a technique, SERS is regarded as an irreproducible technique. It has been found that SERS substrates (metallic nanostructured surfaces supporting the effect) are responsible for the lack of reproducibility. Deviations in size, shape, and orientation of nanoparticles reduce the reproducible characteristics of the SERS substrate. Thus, considerable research has to be performed to develop high-quality SERS substrates with reproducible results. Furthermore, hand-held Raman spectrophotometers, as well as portable IR spectrophotometers, have huge potential and can bring lab-scale research to commercial scale products for the identification of adulterants. Next, the addition of big data analysis tools can help to further enhance the accuracy of these optical methods for the authentication of foods. Thus, the future of optical spectroscopy methods, especially Raman and IR, for biological and adulteration detection is bright, and many new analysis algorithms are expected to be discovered for further advancement in this field.
Author contributions
N. D. conceived the idea and planned the review manuscript. R. K. S., K. K., P. P., J. V., C. D., and N. D. wrote the initial draft of the manuscript. R. K. S. took the lead in writing the review. G. G., S. N. M., A. M., J. P. C., S. A. R. H., D. P. M., P. R. S., and A. K. S. critically reviewed the manuscript and gave their inputs to improve the manuscript draft. All the authors thoroughly read the final manuscript draft and gave permission for its submission.
Conflicts of interest
There are no conflicts to declare.
Acknowledgements
The authors Raj Kumar Sen and Jeet Vishwakarma are thankful to the Council of Scientific and Industrial Research (CSIR), India for the award of the Junior Research Fellowship. This research is partially supported by Science and Engineering Research Board (SERB), India through the project SRG/2020/001215.
References
-
C. Cavin, G. Cottenet, C. Fuerer, L. A. Tran and P. Zbinden, Food fraud vulnerabilities in the supply chain: an industry perspective, in Encyclopedia of food chemistry, ed. L. Melton, F. Shahidi and P. Varelis, Elsevier, 2019, vol. 1, pp. 670–678 Search PubMed.
-
FSSAI, Food Safety and Standards Authority of India (FSSAI) annual report 2018–2019, p. 131 Search PubMed.
- A. Marques, B. Veigas, A. Araújo, B. Pagará, P. V. Baptista, H. Águas, R. Martins and E. Fortunato, Sci. Rep., 2019, 9, 17922 CrossRef PubMed.
- S. Balbinot, A. Mohan Srivastav, J. Vidic, I. Abdulhalim and M. Manzano, Plasmonic biosensors for food control, Trends Food Sci. Technol., 2021, 111, 128–140 CrossRef CAS.
- D. Yang and Y. Ying, Appl. Spectrosc. Rev., 2011, 46, 539–560 CrossRef.
- J. Langer, D. J. de Aberasturi, J. Aizpurua, R. A. Alvarez-Puebla, B. Auguié, J. J. Baumberg, G. C. Bazan, S. E. J. Bell, A. Boisen, A. G. Brolo, J. Choo, D. Cialla-May, V. Deckert, L. Fabris, K. Faulds, F. J. G. de Abajo, R. Goodacre, D. Graham, A. J. Haes, C. L. Haynes, C. Huck, T. Itoh, M. Käll, J. Kneipp, N. A. Kotov, H. Kuang, E. C. Le Ru, H. K. Lee, J.-F. Li, X. Y. Ling, S. A. Maier, T. Mayerhöfer, M. Moskovits, K. Murakoshi, J.-M. Nam, S. Nie, Y. Ozaki, I. Pastoriza-Santos, J. Perez-Juste, J. Popp, A. Pucci, S. Reich, B. Ren, G. C. Schatz, T. Shegai, S. Schlücker, L.-L. Tay, K. G. Thomas, Z.-Q. Tian, R. P. Van Duyne, T. Vo-Dinh, Y. Wang, K. A. Willets, C. Xu, H. Xu, Y. Xu, Y. S. Yamamoto, B. Zhao and L. M. Liz-Marzán, ACS Nano, 2020, 14, 28–117 CrossRef CAS PubMed.
- A. Rohman, M. A. I. B. Ghazali, A. Windarsih, Irnawati, S. Riyanto, F. M. Yusof and S. Mustafa, Molecules, 2020, 25, 5485 CrossRef PubMed.
- H. M. Randall, Science, 1927, 65, 167–173 CrossRef CAS PubMed.
- C. V. Raman and K. S. Krishnan, Nature, 1928, 121, 501–502 CrossRef CAS.
- R. R. Jones, D. C. Hooper, L. Zhang, D. Wolverson and V. K. Valev, Nanoscale Res. Lett., 2019, 14, 231 CrossRef PubMed.
- V. Shvalya, G. Filipič, J. Zavašnik, I. Abdulhalim and U. Cvelbar, Appl. Phys. Rev., 2020, 7, 031307 CAS.
- D. L. Jeanmaire and R. P. Van Duyne, J. Electroanal. Chem. Interfacial Electrochem., 1977, 84, 1–20 CrossRef CAS.
- K. Gracie, E. Correa, S. Mabbott, J. A. Dougan, D. Graham, R. Goodacre and K. Faulds, Chem. Sci., 2014, 5, 1030–1040 RSC.
- E. Le Ru and P. Etchegoin, Annu. Rev. Phys. Chem., 2012, 63, 65–87 CrossRef CAS PubMed.
- R. Shi, X. Liu and Y. Ying, J. Agric. Food Chem., 2017, 66, 6525–6543 CrossRef PubMed.
- M. Fan, G. F. S. Andrade and A. G. Brolo, Anal. Chim. Acta, 2011, 693, 7–25 CrossRef CAS PubMed.
- E. Massarini, P. Wästerby, L. Landström, C. Lejon, O. Beck and P. O. Andersson, Sens. Actuators, B, 2015, 207, 437–446 CrossRef CAS.
- P. Guo, D. Sikdar, X. Huang, K. J. Si, W. Xiong, S. Gong, L. W. Yap, M. Premaratne and W. Cheng, Nanoscale, 2015, 7, 2862–2868 RSC.
- T. Lang, S. Pang and L. He, Anal. Methods, 2015, 7, 6426–6431 RSC.
- Y. Xie, P. Li, J. Zhang, H. Wang, H. Qian and W. Yao, Spectrochim. Acta, Part A, 2013, 114, 80–84 CrossRef CAS PubMed.
-
D. P. Attrey, Food Safety in the 21st Century, 2017, pp. 129–143, DOI:10.1016/B978-0-12-801773-9.00010-8.
- L. Du, W. Lu, B. Gao, J. Wang and L. Yu, J. Food Qual., 2019, 2019, 5487890 CrossRef PubMed.
- T. Azad and S. Ahmed, Int. J. Food Contam., 2016, 3, 22 CrossRef.
- C. Kuanglin, D. Sagar, Q. Jianwei, K. Moon and B. Abigail, Proc. SPIE, 2016, 9864 Search PubMed.
- X.-F. Zhang, M.-Q. Zou, X.-H. Qi, F. Liu, X.-H. Zhu and B.-H. Zhao, J. Raman Spectrosc., 2010, 41, 1655–1660 CrossRef.
- P. Rajapandiyan, W.-L. Tang and J. Yang, Food Control, 2015, 56, 155–160 CrossRef CAS.
- L. C. Mecker, K. M. Tyner, J. F. Kauffman, S. Arzhantsev, D. J. Mans and C. M. Gryniewicz-Ruzicka, Anal. Chim. Acta, 2012, 733, 48–55 CrossRef CAS PubMed.
- Y. Hu, S. Feng, F. Gao, E. C. Y. Li-Chan, E. Grant and X. Lu, Food Chem., 2015, 176, 123–129 CrossRef CAS PubMed.
- M. R. de Almeida, K. de Sá Oliveira, R. Stephani and L. F. C. de Oliveira, Anal. Lett., 2012, 45, 2589–2602 CrossRef CAS.
- S. Jawaid, F. N. Talpur, S. T. H. Sherazi, S. M. Nizamani and A. A. Khaskheli, Food Chem., 2013, 141, 3066–3071 CrossRef CAS PubMed.
- L. Cassoli, B. Sartori and P. Machado, Rev. Bras. Zootec., 2011, 40, 2591–2596 CrossRef.
- N. Nicolaou, Y. Xu and R. Goodacre, J. Dairy Sci., 2010, 93, 5651–5660 CrossRef CAS PubMed.
- B. He, R. Liu, R. Yang and K. Xu, Proc. SPIE, 2010, 7572, 160–168 CrossRef.
- K. Khan, H. Krishna, S. Majumder and P. Gupta, Food Anal. Methods, 2015, 8, 93–102 CrossRef.
- W. Limm, S. R. Karunathilaka, B. Yakes and M. Mossoba, Int. Dairy J., 2018, 85, 177–183 CrossRef CAS.
- S. R. Karunathilaka, B. Yakes, K. He, J. Chung and M. Mossoba, Heliyon, 2018, 4, e00806 CrossRef PubMed.
- M. Nieuwoudt, S. Holroyd, C. McGoverin, M. Simpson and D. Williams, Appl. Spectrosc., 2016, 71, 308–312 CrossRef PubMed.
- A. Marques, B. Veigas, A. Araújo, B. Pagará, P. Baptista, H. Águas, R. Martins and E. Fortunato, Sci. Rep., 2019, 9, 17922 CrossRef PubMed.
- D. Ellis, V. Brewster, W. Dunn, J. Allwood, A. Golovanov and R. Goodacre, Chem. Soc. Rev., 2012, 41, 5706–5727 RSC.
- M. De Marchi, M. Penasa, A. Zidi and C. L. Manuelian, J. Dairy Sci., 2018, 101, 10589–10604 CrossRef CAS PubMed.
- B. G. Botelho, N. Reis, L. S. Oliveira and M. M. Sena, Food Chem., 2015, 181, 31–37 CrossRef CAS PubMed.
- H. Chen, C. Tan, Z. Lin and T. Wu, Spectrochim. Acta, Part A, 2017, 173, 832–836 CrossRef CAS PubMed.
- C. S. Gondim, R. G. Junqueira, S. V. C. Souza, I. Ruisánchez and M. P. Callao, Food Chem., 2017, 230, 68–75 CrossRef CAS PubMed.
- L. Casarrubias-Torres, O. Meza-Márquez, G. Osorio-Revilla and T. Gallardo-Velazquez, Acta Vet. Brno, 2018, 87, 181–188 CrossRef.
- H. Sipahi, G. Aydogan, S. Helvacıoglu, M. Hamitoğlu, E. Guzelmeric and A. Aydin, FABAD J. Pharm. Sci., 2017, 42, 7–13 Search PubMed.
- G. Beretta, P. Granata, M. Ferrero, M. Orioli and R. Facino, Anal. Chim. Acta, 2005, 533, 185–191 CrossRef CAS.
- M. Megherbi, B. Herbreteau, R. Faure and A. Salvador, J. Agric. Food Chem., 2009, 57, 2105–2111 CrossRef CAS PubMed.
- S. E. K. Wei, R. Wahab, S. S. Yaacob and S. Ghoshal, Food Res. Int., 2019, 80, 16–32 Search PubMed.
- B. Özbalci, İ. H. Boyaci, A. Topcu, C. Kadılar and U. Tamer, Food Chem., 2013, 136, 1444–1452 CrossRef PubMed.
- A. G. Mignani, L. Ciaccheri, A. Mencaglia, R. Di Sanzo, S. Carabetta and M. Russo, J. Lightwave Technol., 2016, 34, 1 Search PubMed.
- L. F. De Oliveira, R. Colombara and H. Edwards, Appl. Spectrosc., 2002, 56, 306–311 CrossRef CAS.
- J. F. Pierna, O. Abbas, P. Dardenne and V. Baeten, Biotechnol. Agron. Soc. Environ., 2011, 15, 75–84 CAS.
- S. Li, Y. Shan, X. Zhu, X. Zhang and G. Ling, J. Food Compos. Anal., 2012, 28, 69–74 CrossRef CAS.
- M. Ferreiro-González, E. Espada-Bellido, L. Guillén-Cueto, M. Palma, C. Barroso and G. Barbero, Talanta, 2018, 188, 288–292 CrossRef PubMed.
- M. Oroian, S. Ropciuc and S. Paduret, Food Anal. Methods, 2018, 11, 959–968 CrossRef.
- A. Guelpa, F. Marini, A. Du Plessis, R. Slabbert and M. Manley, Food Control, 2016, 73, 1388–1396 CrossRef.
- N. Subari, J. Mohamad-Saleh, A. Y. Md shakaff and A. Zakaria, Sensors, 2012, 12, 14022–14040 CrossRef CAS PubMed.
- B. Başar and D. Ozdemir, J. Sci. Food Agric., 2018, 98, 5616–5624 CrossRef PubMed.
- D. A. Magdas, F. Guyon, C. Berghian-Grosan and C. Muller Molnar, Food Control, 2021, 123, 107769 CrossRef CAS.
- M. M. Csilla, C. Grosan and A. Magdas, Talanta, 2019, 208, 120432 Search PubMed.
- O. Elhamdaoui, A. El Orche, A. Cheikh, B. Mojemmi, R. Nejjari and M. Bouatia, J. Anal. Methods Chem., 2020, 2020, 8816249 Search PubMed.
- M. Ferreiro-González, E. Espada-Bellido, L. Guillén-Cueto, M. Palma, C. G. Barroso and G. F. Barbero, Talanta, 2018, 188, 288–292 CrossRef PubMed.
- X. Zhu, S. Li, Y. Shan, Z. Zhang, Y. Li, D. Su and F. Liu, J. Food Eng., 2010, 101, 92–97 CrossRef CAS.
- J. Kelly, C. Petisco and G. Downey, J. Agric. Food Chem., 2006, 54, 6166–6171 CrossRef CAS PubMed.
- F. Huang, H. Song, L. Guo, P. Guang, X. Yang, L. Li, H. Zhao and M. Yang, Spectrochim. Acta, Part A, 2020, 235, 118297 CrossRef CAS PubMed.
- V. Baeten and M. Meurens, J. Agric. Food Chem., 1996, 44, 2225–2230 CrossRef CAS.
- V. Baeten, M. Meurens, M. T. Morales and R. Aparicio, J. Agric. Food Chem., 1996, 44, 2225–2230 CrossRef CAS.
- I. Wesley, F. Pacheco and A. McGill, J. Am. Oil Chem. Soc., 1996, 73, 515–518 CrossRef CAS.
- M.-Q. Zou, X.-F. Zhang, X.-H. Qi, H.-L. Ma, Y. Dong, C.-W. Liu, X. Guo and H. Wang, J. Agric. Food Chem., 2009, 57, 6001–6006 CrossRef CAS PubMed.
-
C. Salvan, M. Jiménez and C. Carretero, Application of Raman spectroscopy for fast screening and assessment of olive oil quality, 2017 Search PubMed.
- E. Guzmán, V. Baeten, J. Fernández Pierna and J. A. Garcia Mesa, Food Control, 2011, 22, 2036 CrossRef.
- N. Vanstone, A. Moore, P. Martos and S. Neethirajan, Food Qual. Saf., 2018, 2, 189–198 CrossRef CAS.
- T. de Oliveira Mendes, R. Rocha, B. Porto, M. Oliveira, V. Anjos and M. J. Bell, Food Anal. Methods, 2015, 8, 2339 CrossRef.
- G. Gurdeniz and B. Ozen, Food Chem., 2009, 116, 519–525 CrossRef CAS.
- M. Vasconcelos, L. Coelho and J. De Almeida, Cogent Food Agric., 2015, 1, 1–13 Search PubMed.
- H. Yang and J. Irudayaraj, J. Am. Oil Chem. Soc., 2001, 78, 889–895 CrossRef CAS.
- A. Tay, R. Singh, S. Krishnan and J. Gore, LWT--Food Sci. Technol., 2002, 35, 99–103 CrossRef CAS.
- X. F. Zhang, M.-Q. Zou, X. H. Qi, F. Liu, C. Zhang and F. Yin, J. Raman Spectrosc., 2011, 42, 1784–1788 CrossRef CAS.
- Y. Li, T. Fang, S. Zhu, F. Huang, Z. Chen and Y. Wang, Spectrochim. Acta, Part A, 2017, 189, 37–43 CrossRef PubMed.
- R. Joshi, B.-K. Cho, R. Joshi, S. Lohumi, M. A. Faqeerzada, H. Amanah, J. Lee, C. Mo and H. Lee, J. Agric. Sci., 2019, 46, 183–194 CrossRef CAS.
- C. Farley, A. Kassu, N. Bose, A. Jackson-Davis, J. Boateng, P. Ruffin and A. Sharma, Appl. Spectrosc., 2016, 71, 1340–1347 CrossRef PubMed.
- T. K. de Lima, M. Musso and D. B. Menezes, Food Chem., 2020, 333, 127454 CrossRef CAS PubMed.
- M. Vasconcelos, L. Coelho, A. Barros and J. M. M. M. de Almeida, Cogent Food Agric., 2015, 1, 1018695 CrossRef.
- T. Lima, M. Musso and D. B. Menezes, Food Chem., 2020, 333, 127454 CrossRef PubMed.
- A. Prades, M. Dornier, N. Diop and J.-P. Pain, Fruits, 2012, 67, 87–107 CrossRef CAS.
- P. Richardson, H. Muhamadali, D. Ellis and R. Goodacre, Food Chem., 2018, 272, 157–164 CrossRef PubMed.
- L. E. Rodriguez-Saona, F. S. Fry, M. A. McLaughlin and E. M. Calvey, Carbohydr. Res., 2001, 336, 63–74 CrossRef CAS PubMed.
- K. V. Peter, Handb. Herbs Spices, 2012, 1, 1–607 Search PubMed.
- P. Vargas Jentzsch, F. Gualpa, L. A. Ramos and V. Ciobotă, Flavour Fragrance J., 2018, 33, 184–190 CrossRef CAS.
- D. Wicochea, S. Peyron, P. Rigou and P. Chalier, PLoS One, 2018, 13, e0207401 CrossRef PubMed.
- D. Sagar, C. Kuanglin, Q. Jianwei, K. Moon, S. Walter and C. Dian, Foods, 2016, 5, 36 CrossRef PubMed.
- K. Chao, S. Dhakal, W. F. Schmidt, J. Qin, M. Kim, Y. Peng and Q. Huang, Food Chem., 2020, 320, 126567 CrossRef CAS PubMed.
- S. W. Erasmus, L. van Hasselt, L. M. Ebbinge and S. M. van Ruth, Food Control, 2021, 121, 107714 CrossRef CAS.
- S. Dhakal, W. F. Schmidt, M. Kim, X. Tang, Y. Peng and K. Chao, Foods, 2019, 8, 143 CrossRef CAS PubMed.
- S. Dhakal, Foods, 2016, 5, 36 CrossRef PubMed.
- S. Kar, B. Tudu, A. Bag and R. Bandyopadhyay, Food Anal. Methods, 2018, 11, 1291–1302 CrossRef.
- I. Rukundo, M.-G. Danao, C. Weller, R. Wehling and K. Eskridge, J. Near Infrared Spectrosc., 2020, 28, 096703351989888 CrossRef.
- S. Kar, B. Tudu, A. Jana and R. Bandyopadhyay, Food Addit. Contam., Part A, 2019, 36, 863–875 CrossRef CAS PubMed.
-
A. Koocheki and E. Milani, in Saffron, ed. A. Koocheki and M. Khajeh-Hosseini, Woodhead Publishing, 2020, pp. 321–334, DOI:10.1016/B978-0-12-818638-1.00020-4.
- S. Karimi, J. Feizy, F. Mehrjo and M. Farrokhnia, RSC Adv., 2016, 6, 23085–23093 RSC.
- E. Cusano, R. Consonni, E. Petrakis, K. Astraka, L. Cagliani and M. Polissiou, Phytochem. Anal., 2018, 29, 476–486 CrossRef CAS PubMed.
- F. Y. Lee, T. Htar and G. Akowuah, Int. J. Food Prop., 2014, 18, 1773–1783 CrossRef.
- S. Er, H. Ekşi, H. Yetim and I. Boyacı, Food Anal. Methods, 2017, 10, 1547–1555 CrossRef.
- E. Shawky, R. Abu El-Khair and D. Selim, LWT--Food Sci. Technol., 2020, 109032, DOI:10.1016/j.lwt.2020.109032.
- E. Petrakis and M. Polissiou, Talanta, 2016, 162, 558–566 CrossRef PubMed.
-
R. Ranjan, N. Kumar, A. Kiranmayee and P. Panchariya, Application of Handheld NIR Spectroscopy for Detection of Adulteration in Turmeric Powder, 2021 Search PubMed.
- O. L. Henao, T. F. Jones, D. J. Vugia and P. M. Griffin, Foodborne Diseases Active Surveillance Network, Emerging Infect. Dis., 2015, 21, 1529–1536 CrossRef CAS PubMed.
- R. V. Tauxe, Int. J. Food Microbiol., 2002, 78, 31–41 CrossRef CAS PubMed.
- Y.-D. Wang, X.-L. Li, Z.-X. Liu, X.-X. Zhang, J. Hu and J.-H. Lü, Nucl. Sci. Tech., 2017, 28, 49 CrossRef.
- J. W.-F. Law, N.-S. Ab Mutalib, K.-G. Chan and L.-H. Lee, Front. Microbiol., 2015, 5, 770 Search PubMed.
- C. Quintelas, E. C. Ferreira, J. A. Lopes and C. Sousa, Biotechnol. J., 2018, 13, 1700449 CrossRef PubMed.
- P. Lasch, M. Stämmler, M. Zhang, M. Baranska, A. Bosch and K. Majzner, Anal. Chem., 2018, 90, 8896–8904 CrossRef CAS PubMed.
- J. Lee, M. S. Ahn, Y. L. Lee, E. Y. Jie, S. G. Kim and S. W. Kim, J. Appl. Microbiol., 2019, 126, 864–871 CrossRef CAS PubMed.
- M. Wenning, F. Breitenwieser, R. Konrad, I. Huber, U. Busch and S. Scherer, J. Microbiol. Methods, 2014, 103, 44–52 CrossRef CAS PubMed.
- M. Moskovits, J. Raman Spectrosc., 2005, 36, 485–496 CrossRef CAS.
-
Z. Zhang, in Sensing Techniques for Food Safety and Quality Control, The Royal Society of Chemistry, 2017, pp. 1–16, 10.1039/9781788010528-00001.
- L. E. Rodriguez-Saona, F. M. Khambaty, F. S. Fry and E. M. Calvey, J. Agric. Food Chem., 2001, 49, 574–579 CrossRef CAS PubMed.
- N. Nicolaou and R. Goodacre, Analyst, 2008, 133, 1424–1431 RSC.
- A. Assaf, C. B. Y. Cordella and G. Thouand, Anal. Bioanal. Chem., 2014, 406, 4899–4910 CrossRef CAS PubMed.
- Y. Wang, S. Ravindranath and J. Irudayaraj, Anal. Bioanal. Chem., 2011, 399, 1271–1278 CrossRef CAS PubMed.
- X. Wu, C. Xu, R. A. Tripp, Y.-W. Huang and Y. Zhao, Analyst, 2013, 138, 3005–3012 RSC.
- Y. Chao and T. Zhang, Anal. Bioanal. Chem., 2012, 404, 1465–1475 CrossRef CAS PubMed.
- H. Yang and J. Irudayaraj, J. Mol. Struct., 2003, 646, 35 CrossRef CAS.
- A. Zając, L. Dymińska, J. Lorenc and J. Hanuza, Food Anal. Methods, 2017, 10, 640–648 CrossRef.
- E. Witkowska, D. Korsak, A. Kowalska, M. Księżopolska-Gocalska, J. Niedziółka-Jönsson, E. Roźniecka, W. Michałowicz, P. Albrycht, M. Podrażka, R. Hołyst, J. Waluk and A. Kamińska, Anal. Bioanal. Chem., 2017, 409, 1555–1567 CrossRef CAS PubMed.
- D. G. Drescher, N. A. Ramakrishnan and M. J. Drescher, Methods Mol. Biol., 2009, 493, 323–343 CrossRef CAS PubMed.
- H. H. Nguyen, J. Park, S. Kang and M. Kim, Sensors, 2015, 15, 10481–10510 CrossRef CAS PubMed.
- A. M. Shrivastav, U. Cvelbar and I. Abdulhalim, Commun. Biol., 2021, 4, 70 CrossRef CAS PubMed.
- Y. Tang, X. Zeng and J. Liang, J. Chem. Educ., 2010, 87, 742–746 CrossRef CAS PubMed.
- R. Bakhtiar, J. Chem. Educ., 2013, 90, 203–209 CrossRef CAS.
- K. R. Srivastava, S. Awasthi, P. Mishra and P. Srivastava, Waterborne Pathog., 2020, 237–277, DOI:10.1016/B978-0-12-818783-8.00013-X.
- Y. W. Fen and W. M. Mat Yunus, Sens. Rev., 2013, 33, 305–314 CrossRef.
- S. Shen, T. Liu and J. Guo, Appl. Opt., 1998, 37, 1747–1751 CrossRef CAS PubMed.
- C. S. Schneider, A. G. Bhargav, J. G. Perez, A. S. Wadajkar, J. A. Winkles, G. F. Woodworth and A. J. Kim, J. Controlled Release, 2015, 219, 331–344 CrossRef CAS PubMed.
- K. V. Gobi, H. Iwasaka and N. Miura, Biosens. Bioelectron., 2007, 22, 1382–1389 CrossRef CAS PubMed.
- R. J. Green, R. A. Frazier, K. M. Shakesheff, M. C. Davies, C. J. Roberts and S. J. Tendler, Biomaterials, 2000, 21, 1823–1835 CrossRef CAS PubMed.
- Y. W. Fen, W. M. Mat Yunus and Z. Talib, Optik, 2013, 124, 126–133 CrossRef CAS.
- N. Zainuddin, Y. W. Fen, A. Alwahib, M. Yaacob, N. Bidin and M. A. Mahdi, Optik, 2018, 168, 134–139 CrossRef CAS.
- Y. Lu, Y. Xia, M. Pan, X. Wang and S. Wang, J. Agric. Food Chem., 2014, 62, 12471–12476 CrossRef CAS PubMed.
- S. D. Mazumdar, M. Hartmann, P. Kämpfer and M. Keusgen, Biosens. Bioelectron., 2007, 22, 2040–2046 CrossRef CAS PubMed.
- V. Yadav, M. Yadav, P. Kumar and R. K. Verma, Food Chem., 2020, 332, 127346 CrossRef PubMed.
- D. Klein, R. Breuch, J. Reinmüller, C. Engelhard and P. Kaul, Foods, 2021, 10, 1850 CrossRef CAS PubMed.
|
This journal is © The Royal Society of Chemistry 2022 |
Click here to see how this site uses Cookies. View our privacy policy here.