DOI:
10.1039/D2RA05751D
(Paper)
RSC Adv., 2022,
12, 30181-30200
Gaussian field-based 3D-QSAR and molecular simulation studies to design potent pyrimidine–sulfonamide hybrids as selective BRAFV600E inhibitors†
Received
12th September 2022
, Accepted 14th October 2022
First published on 21st October 2022
Abstract
The “RAS-RAF-MEK-ERK” pathway is an important signaling pathway in melanoma. BRAFV600E (70–90%) is the most common mutation in this pathway. BRAF inhibitors have four types of conformers: type I (αC-IN/DFG-IN), type II (αC-IN/DFG-OUT), type I1/2 (αC-OUT/DFG-IN), and type I/II (αC-OUT/DFG-OUT). First- and second-generation BRAF inhibitors show resistance to BRAFV600E and are ineffective against malignancies induced by dimer BRAF mutants causing ‘paradoxical’ activation. In the present study, we performed molecular modeling of pyrimidine–sulfonamide hybrids inhibitors using 3D-QSAR, molecular docking, and molecular dynamics simulations. Previous reports reveal the importance of pyrimidine and sulfonamide moieties in the development of BRAFV600E inhibitors. Analysis of 3D-QSAR models provided novel pyrimidine sulfonamide hybrid BRAFV600E inhibitors. The designed compounds share similarities with several structural moieties present in first- and second-generation BRAF inhibitors. A total library of 88 designed compounds was generated and molecular docking studies were performed with them. Four molecules (T109, T183, T160, and T126) were identified as hits and selected for detailed studies. Molecular dynamics simulations were performed at 900 ns and binding was calculated. Based on molecular docking and simulation studies, it was found that the designed compounds have better interactions with the core active site [the nucleotide (ADP or ATP) binding site, DFG motif, and the phospho-acceptor site (activation segment) of BRAFV600E protein than previous inhibitors. Similar to the FDA-approved BRAFV600E inhibitors the developed compounds have [αC-OUT/DFG-IN] conformation. Compounds T126, T160 and T183 interacted with DIF (Leu505), making them potentially useful against BRAFV600E resistance and malignancies induced by dimer BRAF mutants. The synthesis and biological evaluation of the designed molecules is in progress, which may lead to some potent BRAFV600E selective inhibitors.
1. Introduction
Melanomas arise from uncontrolled proliferation of melanocytes, which are found throughout the body and determine the color of the skin, eye, inner ear, iris, rectum, leptomeninges, and basal layer of the hair bulb matrix.1,2 For all sight of skin cancer melanoma account for only 4% but have death rate higher than 50%.3 In 2020, 19
292
789 new cases were estimated worldwide for all cancers, including 324
635 (1.7%) melanoma cases. The total number of cancer deaths was 9
958
133, of which 57
043 (0.6%) were due to melanoma.4
The “RAS-RAF-MEK-ERK” pathway is the main component of a signal transduction pathway that regulates gene expression and supports mammalian cells' proliferation and survival.5 The RAS-RAF-MEK-ERK pathway is responsible for the activation of BRAF and regulates cell proliferation and differentiation.6 Activation of the small G protein Ras leads to activation of BRAF.7 BRAF activates the MAP kinase/ERK signaling pathway for normal cell control and proliferation.8 Normal and oncogenic BRAF signaling pathway is depicted in Fig. 1a.
 |
| Fig. 1 (a) Normal and oncogenic BRAF signaling pathway notes: normal activation of RAS-RAF pathway shows normal cell proliferation and survival. Oncogenic BRAF signaling causes an increase in cell proliferation and survival from benign nevus to metastatic melanoma (b) V600E mutation in BRAF protein structure (c) BRAF inhibitor binding mode with conformations (αC-helix and DFG motif). | |
BRAF is the most frequently mutated protein kinase in human melanomas.9 The prevalence of BRAF mutations in human malignancies varies, with melanoma having between 70–90% of all mutations,10,11 5–20% in colon cancer,12 30–50% in thyroid cancer, ∼10% in papillary thyroid cancer,13 5–20% in colorectal cancer, and 5–30% in ovarian cancer.14 Among BRAF gene mutations, the BRAFV600E gene is the most common mutation in melanoma, accounting for 70–90%.15,16 It occurs at position 600 with a single point mutation (Val600 → Glu), in which the hydrophobic valine is replaced by polar, hydrophilic glutamic acid.17 BRAFV600WT is the second most common mutation of BRAF in this BRAFV600K, (10–30%).18 The rarer codon mutations BRAFV600R,V600D (6–3%), BRAFV600E2,600MandV600G (<1%)19 occur in a small percentage of patients and are considered 8% to 10% of all BRAF mutations.20
1.1 BRAF kinase conformations
The BRAF kinase domain contains two lobes: small N and large C-terminal lobe, which are connected by a catalytic cleft or flexible hinge region. The catalytic site is located in the cleft between two lobes and contains the ATP-binding site. This region is divided into a front cleft (containing the ATP-binding site), a gate area, and a back cleft. The small N-terminal domain lobe has an αC-helix, predominantly antiparallel β-sheet anchors, a glycine-rich ATP-phosphate-binding loop (P-loop), and ATP orients. The large C-terminal lobe consists of α-helices.
At the interface of the N-and C-terminal lobes, the nucleotide (ADP or ATP) binding site, the magnesium binding site (DFG [Asp594–Phe595–Gly596] motif), and the phospho-acceptor site [activation segment (AS)] are located near the DFG motif, which are three major active sites.8,21
The ATP pocket consists of the following main regions. Adenine region (1) is similar to the hinge region. Sugar region (2) is where ATP ribose is located, while other three regions include hydrophobic (3), type-I (4) and type-II (5) regions. The solvent-accessible region is also part of the ATP region, but generally, ATP is not located in this region.22,23 The structural domain of the BRAF between the N-terminal and C-terminal lobes contains three conserved regions (CR): CR1, CR2, and CR3.24 In humans, the BRAF protein kinase contains 766 amino acids, with key amino acid residues listed in Table 1.25,26 BRAF protein structure and different binding conformations (αC-helix and DFG) motif with inhibitors are depicted in Fig. 1b and c.
Table 1 Important amino acid sequence in BRAF protein
BRAF protein region |
Amino acid sequence |
Different binding pocket between N- and C-lobe |
Amino acid sequence |
Raf selective pocket contain (DIF and DFG motif). |
CR1 |
150–290 |
RBD |
155–227 |
Glycine-rich ATP-phosphate-binding loop (P-loop) and ribose pocket |
463–471 |
CRD |
234–280 |
CR2 |
360–375 |
αC-Helix |
492–504 |
CR3 (protein kinase domain) |
457–717 |
RAF selective pocketa |
DIF |
504–511 |
DFG motif |
594–596 |
Gatekeeper residue |
T529 |
Hinge region (adenine region) |
530–535 |
Catalytic loop |
574–581 |
Activation segment |
599–623 |
1.2 Different binding modes of BRAF protein with inhibitors
The DFG motif and the αC-helix represent an important structural element of kinases. In physiological kinase activation, the DFG motif plays a fundamental role as it shifts from the DFG-OUT (inactive or closed state occupying part of the ATP-binding site) to the DFG-IN (active or open state), where it moves towards the base of the αC-helix, which occupies part of the allosteric binding site and influences the catalytic process.
When the DFG motif is in the IN position, the Asp594 residue faces the active site of the kinase. In this condition, the glycine-rich loop and the activation segment of the BRAF kinase are separated from one another, the hydrophobic connection between these two areas is disrupted. As a result, the catalytic cleft accessible to ATP. In this conformation, the inhibitors form interactions with the hinge region (ATP-binding site) by forming ∼1–3 hydrogen bonds and hydrophobic interaction around the adenine regions of the ATP-binding site of the protein. The inhibitors also interact with other different regions of ATP, including hydrophobic regions adjacent to the adenine region, the ribose region, and solvent accessible region.
When the DFG motif is in the OUT position the aspartate residue (Asp594) displaced from the active site, therefore hydrophobic connection between the glycine rich loop and activation section of the BRAF kinase domain, which is brought near to one another. This contact renders ATP unavailable to the catalytic cleft of BRAF. DFG-OUT conformation, Asp594 residue supports allosteric movement extending outward from the ATP-binding site, and inhibitors bind to allosteric site that is close to the ATP binding pocket. The αC-helix near the ATP site is called the αC-IN position, the αC-helix away from the ATP site is called the αC-OUT position.27–33 Based on αC-helix and DFG motif, four structural types of inhibitors are currently being developed. (1) Type I, (αC-IN/DFG-IN). (2) Type II, (αC-IN/DFG-OUT). (3) Type I1/2, (αC-OUT/DFG-IN). (4) Type I/II, (αC-OUT/DFG-OUT).34–37
1.3 Pyrimidine–sulfonamide hybrids BRAF inhibitors
Dabrafenib mesylate type I1/2, (αC-OUT/DFG-IN) inhibitor is an FDA-approved pyrimidine-thiazole based diphenyl sulfonamide derivative for the treatment of metastatic melanoma in patients with BRAFV600E mutation.38,39 Dabrafenib was first developed by GlaxoSmithKline (GSK) under the name GSK2118436. It is an ATP-competitive inhibitor of BRAF kinases. Dabrafenib inhibits BRAFV600E most potently but is less effective against BRAFV600WT.40,41 In 2011, vemurafenib, a pyrolo-pyrimidine based diphenyl sulfonamide derivative42 of type I1/2, (αC-OUT/DFG-IN)43 received FDA approval for first oral BRAFV600E inhibitor.44,45 Encorafenib (LGX-818), a pyrazolo-pyrimidine based phenyl sulfonamide derivative,39 was approved by the FDA in June 2018 for the treatment of BRAFV600E/K mutated cancer. Patients with metastatic melanoma can take a combination of encorafenib and binimetinib (inhibitors of BRAF and MEK).46,47 The FDA-approved first- and second-generation adenosine triphosphate (ATP) competitive BRAF inhibitors are shown resistance against monomeric BRAFV600 species mutations and also ineffective against malignancies driven by dimeric BRAF mutants causing ‘paradoxical’ activation.48 Preclinical studies of BRAF inhibitors in melanoma shown that the development of resistance is more complex compared to single mutations.49 BRAF inhibitor resistance depends on epigenetic and transcriptomic mechanisms of MAPK/ERK or PI3K/Akt activation of receptor tyrosine kinases.50
In 2019 Abdel-Maksoud et al. synthesized BRAF inhibitors possessing 5-(pyrimidin-4-yl)imidazo[2,1-b]thiazole sulfonamide containing scaffold,51 and in 2021, Ali et al. synthesized novel (imidazole-5-yl)pyrimidine–sulfonamide hybrids derivatives.52 In 2020 Ammar et al. synthesized imidazothiazole moiety containing pyrimidine–sulfonamide derivatives. The synthesized compounds showed good BRAFV600E kinase inhibition values in in vitro experiments.53 Abdel-Maksoud MS, et al. synthesized a new series of 4-(1H-benzo[d]imidazole-1-yl)pyrimidin-2-amine linked sulfonamide derivatives in 2021. The IC50 of the synthesized compounds were evaluated against BRAFV600E, wild-type BRAF, and CRAF and potential inhibitory activity was found.54 In 2012, Li et al. synthesized a new series of ATP-competitive BRAF inhibitors based on aryl/heteroaryl and o-substitutions on the pyrazolo[1,5-a] pyrimidine scaffold sulfonamide derivative with highly cellular potency and BRAF selectivity.55 To date, the FDA has approved four drugs for the treatment of melanoma, three of which contain a sulfonamide and pyrimidine moiety respectively (Fig. 2). BRAF inhibitors having pyrimidine and sulfonamide moiety (1–51) are also depicted in Fig. 2.
 |
| Fig. 2 Pyrimidine and sulfonamide moiety containing FDA approved and potent BRAFV600E inhibitors. | |
2. Computational approach for designing of new pyrimidine–sulfonamide hybrid containing compounds as potent BRAFV600E inhibitors
Literature reports indicate that pyrimidine and sulfonamide moieties play a very important role in BRAF mutant inhibitor development. The aim of this work is to investigate the structural requirements of pyrimidine–sulfonamide hybrid derivatives as inhibitors of BRAFV600E mutant. Typically, 3D-QSAR investigations are employed for statistical analysis of SAR for a group of compounds with similar structures and to look into the characteristics of an interaction between a ligand and its target protein.56
Molecular docking is used to understanding the structural relationship between a ligand and its target and predicting the best binding conformation.57 The flexibility, changes in the structural conformation of the target binding site, and stability of a ligand–receptor complex suggested by molecular docking was studied in details by the use of molecular dynamics (MD) simulations.58 As a result, it is thought that combining 3D-QSAR, molecular docking, and MD simulations provides an efficient way to examine how the ligand and receptor attach to one another. By utilizing 3D-QSAR, molecular docking, and MD simulations in the current study, we have successfully proven molecular modelling of inhibitors of pyrimidine–sulfonamide hybrids. To the best of our knowledge, no 3D-QSAR studies have been reported for these types of compounds.59
In this study, we built more selective 3D-QSAR models based on Gaussian techniques and SAR of these was summarized. The results of the 3D-QSAR investigation were validated using molecular docking, and the interactions between proteins and ligands were examined. We applied MD simulations to understand the flexibility, structural conformational changes and to estimate the free energy of binding of mutant BRAF kinases with the designed compounds.
2.1 Materials and methods
2.1.1 Gaussian based 3D QSAR.
2.1.1.1 Dataset collection. Structurally different pyrimidine–sulfonamide hybrid derivatives (1–51) were selected from the literature, exhibiting a broad spectrum of BRAFV600E inhibitory activities (IC50 1.20–1910 nM).51–54 The 2D structures of the pyrimidine–sulfonamide hybrid derivatives (1–51) were prepared using the Chem 2D program. The geometry of all these molecules was converted to a 3D structure using Schrodinger's Maestro 12.9. The following options were chosen: (i) the force field used was OPLS 2005, (ii) one low energy ring conformation per ligand was generated, (iii) all possible ionization states at biological pH (iv) depending on the number of chiral centers, probable tautomers and stereospecificity were considered when processing the 3D structure of the molecules with the LigPrep module.59,60
2.1.1.2 Training and test set selection. The IC50 values of the data set were changed to negative logarithmic forms [pIC50 = −log10(IC50)] to linearize the data. pIC50 values between 5.719 and 8.921 were used in this study as dependent variable for the development of 3D-QSAR models. The dataset was divided into training and test (validation) set (70
:
30) (Table 2) using random selection tool present in 3D-Gaussian based QSAR of Maestro 12.9.59,60
Table 2 Experimental and predicted BRAFV600E inhibitory IC50 values of pyrimidine–sulfonamide hybrid derivatives used in current study
S. No. |
R1 |
X |
n |
R2 |
IC50 (nM) |
pIC50 |
Predicted pIC50 values (Gaussian) |
Ref. |
1 |
 |
F |
1 |
OH |
5.620 |
8.250 |
7.802 |
52 |
2 |
 |
F |
1 |
OH |
26.300 |
7.580 |
7.901 |
52 |
3 |
 |
F |
1 |
OH |
30.300 |
7.519 |
7.748 |
52 |
4 |
 |
F |
1 |
OH |
8.680 |
8.061 |
7.858 |
52 |
5 |
 |
F |
1 |
OH |
2.490 |
8.604 |
8.436 |
52 |
6 |
 |
Cl |
1 |
OH |
4.250 |
8.372 |
7.824 |
52 |
7 |
 |
Cl |
1 |
OH |
21.800 |
7.662 |
7.923 |
52 |
8 |
 |
Cl |
1 |
OH |
14.800 |
7.830 |
7.770 |
52 |
9 |
 |
Cl |
1 |
OH |
7.810 |
8.107 |
8.464 |
52 |
10 |
 |
— |
2 |
F |
22.300 |
7.652 |
7.440 |
51 |
11 |
 |
— |
3 |
F |
89.000 |
7.051 |
7.376 |
51 |
12 |
 |
— |
3 |
F |
26.000 |
7.585 |
7.575 |
51 |
13 |
 |
— |
3 |
F |
25.100 |
7.600 |
7.773 |
51 |
14 |
 |
— |
3 |
F |
16.200 |
7.790 |
7.778 |
51 |
15 |
 |
— |
3 |
F |
9.300 |
8.032 |
7.778 |
51 |
16 |
 |
— |
2 |
 |
28.300 |
7.543 |
7.615 |
53 |
17 |
 |
— |
2 |
 |
59.100 |
7.228 |
7.403 |
53 |
18 |
 |
— |
2 |
 |
141.000 |
6.851 |
7.323 |
53 |
19 |
 |
— |
2 |
 |
23.400 |
7.631 |
7.375 |
53 |
20 |
 |
— |
2 |
 |
16.700 |
7.777 |
7.611 |
53 |
21 |
 |
— |
2 |
 |
73.700 |
7.133 |
7.598 |
53 |
22 |
 |
— |
2 |
 |
12.700 |
7.896 |
8.112 |
53 |
23 |
 |
— |
2 |
 |
152.000 |
6.818 |
6.651 |
53 |
24 |
 |
— |
3 |
 |
13.500 |
7.870 |
8.067 |
53 |
25 |
 |
— |
3 |
 |
11.400 |
7.943 |
7.721 |
53 |
26 |
 |
— |
3 |
 |
20.400 |
7.690 |
8.326 |
53 |
27 |
 |
— |
3 |
 |
4.310 |
8.366 |
8.332 |
53 |
28 |
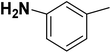 |
— |
3 |
 |
6.210 |
8.207 |
8.286 |
53 |
29 |
 |
— |
3 |
 |
24.500 |
7.611 |
7.758 |
53 |
30 |
 |
— |
3 |
 |
1.200 |
8.921 |
8.331 |
53 |
31 |
 |
— |
3 |
 |
4.310 |
8.366 |
8.332 |
53 |
32 |
 |
— |
3 |
 |
6.210 |
8.207 |
8.286 |
53 |
33 |
 |
— |
3 |
 |
25.100 |
7.600 |
7.507 |
53 |
34 |
 |
— |
3 |
 |
9.300 |
8.032 |
7.741 |
53 |
35 |
 |
— |
3 |
 |
131.0 |
6.883 |
7.454 |
53 |
36 |
 |
— |
3 |
 |
20.0 |
7.699 |
7.453 |
53 |
37 |
 |
— |
3 |
 |
35.0 |
7.456 |
7.407 |
53 |
38 |
 |
— |
2 |
— |
1910.0 |
5.719 |
5.987 |
54 |
39 |
 |
— |
2 |
— |
740.0 |
6.131 |
6.055 |
54 |
40 |
 |
— |
2 |
— |
820.0 |
6.086 |
6.066 |
54 |
41 |
 |
— |
2 |
— |
910.0 |
6.041 |
6.066 |
54 |
42 |
 |
— |
2 |
— |
620.0 |
6.208 |
6.183 |
54 |
43 |
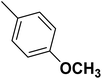 |
— |
2 |
— |
980.0 |
6.009 |
6.043 |
54 |
44 |
 |
— |
2 |
— |
790.0 |
6.102 |
5.993 |
54 |
45 |
 |
— |
3 |
— |
1120.0 |
5.951 |
6.045 |
54 |
46 |
 |
— |
3 |
— |
530.0 |
6.276 |
6.062 |
54 |
47 |
 |
— |
3 |
— |
790.0 |
6.102 |
6.056 |
54 |
48 |
 |
— |
3 |
— |
810.0 |
6.092 |
6.043 |
54 |
49 |
 |
— |
3 |
— |
490.0 |
6.310 |
6.259 |
54 |
50 |
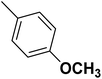 |
— |
3 |
— |
820.0 |
6.086 |
6.185 |
54 |
51 |
 |
— |
3 |
— |
1280.0 |
5.893 |
6.409 |
54 |
2.1.1.3 Alignment procedure. Correct alignment of optimized conformers from selected dataset in a fixed lattice is the most crucial need for successful generation of models. It depends on the correct relative placement of the molecules from the dataset. In this study, the molecules were aligned using the structure alignment tools (flexible shape-based alignment, common scaffold alignment, maximum common substructure, smarts) of Maestro 12.9 tools.60,61
2.1.1.4 Generation of 3D QSAR model. Models were generated using a grid spacing of 0.1 Å and extending the grid by 3.0 Å beyond the limits of the training set using Maestro 12.9. The force field values were set as ignore force field within 2.0 Å. The truncated steric and electrostatic force field was preset to 30.0 kcal mol−1. Variables were removed with a standard deviation (SD) <0.01 and the number of ligands to exclude from the cross-validation was set as 1. Five features including steric, electrostatic, H-bond acceptor, H-bond donor, and hydrophobic field was considered for model buildup.60,62
2.1.1.5 Partial least square (PLS) analysis. The partial least squares (PLS) were used to establish the 3D-QSAR model. In this various statistical parameters such as standard deviation (SD), non-cross-validated correlation coefficient (r2), cross-correlation validation, (rCV2), r2 scramble, Fischer's test (F-test), variance ratio (p value), root mean squared error (RMSE), Pearson-r values, leave-one-out (LOO) cross-validated correlation coefficient (q2) and Pearson-r were considered for validation of the developed models. The quality of the developed models was examined by SD of regression < SD of actual activities (1), 1 > r2 > 0.5, rCV2 > 0.6, rscramble2 < 0.6, Fischer's test (F-test) value (as large as possible), p value (as less as possible), q2 > 0.5, Pearson-r values, and RMSE close to 1. To evaluate the predictive ability of the QSAR models developed, pIC50 values as dependent variables and Gaussian intensities as descriptors (independent variables) were used.60,63
2.1.2 Molecular docking. The 3D structure of human BRAF kinase (hBRk) with V600E mutation was taken from the research collaboratory for structural bioinformatics (RCSB) protein database,64 PDB ID 4XV2.65 Dabrafenib (N-{3-[5-(2-aminopyrimidin-4-yl)-2-tert-butyl-1,3-thiazol-4-yl]-2-fluorophenyl}-2,6-difluorobenzenesulfonamide) is bound in the catalytic pocket. Only the a chain of the kinase was retained, and dabrafenib and water molecules were removed. The hBRk was prepared for molecular docking with Chimera 1.14.66,67 All nonpolar hydrogens were merged, gasteiger charges were added to each atom, atom types were determined, and the final structure of the prepared receptor was saved in pdbqt file format. The center of the grid box was set at the position of the F39 atom of dabrafenib, with cartesian coordinates −0.4, −4.9, −21.6. The size of the box was 20 × 20 × 20 Å3 and both exhaustiveness and the number of modes were set to 100. The 3D structure of the ligands was determined by converting SMILES to 3D geometry and then optimizing the geometry in RDKit.68 The Auto Dock Tools 4 Python script prepare_ligand4.py69 was used to prepare the ligands. A threshold of 4 kcal mol−1 relative to the highest score was introduced to save docked conformations. All results were visually inspected, and the conformation with the lowest binding energy was retained. AutoDock Vina69 was used for molecular docking simulations. Dabrafenib was docked to the hBRk to validate our docking procedure.
2.1.3 Molecular dynamics simulations. The initial structures of the ligand–receptor complexes for the MD simulation were obtained by molecular docking experiment. The ligands were parametrized in the AMBER 20 Antechamber module70 using the GAFF force field.71 For the kinase, AMBER ff19SB72 force field was employed. The PDB2PQR web-server73 was accessed to determine the protonation state of the side chains of the residues at physiological pH. The ligand–receptor complex was impregnated with pre-equilibrated TIP3P water molecules in a truncated octahedral periodic box. The minimum distance between the edges of the water box and the nearest atom of the complex was set at 12 Å. The system was neutralized by six Cl− anions, followed by the addition of Na+ and Cl− ions according to the recommendations of Machado and Pantano74 to achieve a neutral environment with a salt concentration of 0.15 M.The minimization–heating–equilibration–production protocol was used. First, the system was subjected to geometry optimization with periodic boundary conditions in all directions. In 10
000 optimization cycles (4000 steepest descent + 6000 conjugate gradient), both the protein and the ligand were constrained with harmonic potential k = 10.0 mol−1 Å−2. The system was heated stepwise from 0 K to 310 K in 500 ps without any constraints. After an equilibration period of 500 ps, a productive unconstrained molecular dynamics (MD) simulation of 1 μs duration was started, except for the drug dabrafenib, for which the total simulation time was 300 ns. A time step of 2 fs at constant pressure (1 atm) and temperature (310 K) was used. A langevine thermostat with a collision frequency of 1 ps−1 was used for temperature control. In all simulation protocols, hydrogen atoms were constrained by the SHAKE algorithm.75 The cut-off distance for non-bonded interaction was 11 Å, while for long-range electrostatic interactions, the particle mesh Ewald method76 was used. Periodic boundary conditions were employed in all directions. MD simulations were performed using the molecular dynamics package Amber.77 MD simulations were performed on the Isabella cluster of the University Computing Center, University of Zagreb, Croatia.
2.1.3.1 Binding free energy calculation. The free energies of binding (ΔGbind) between a series of potential drugs (ligands) and the hBRk were calculated according to well-established molecular mechanics/generalized Born surface area (MM/GBSA) protocol.78 The formula |
ΔGbind = ΔH − TΔS ≈ ΔEMM + ΔGsol − TΔS
| (1) |
|
ΔEMM = ΔEinternal + ΔEelectrostatic + ΔEvdW
| (2) |
within single-trajectory approach is implemented in the MMPSBA.py script of the AmberTools package. The sum of the bond, angle and dihedral energy (ΔEinternal), electrostatic (ΔEelectrostatic) and van der Waals (ΔEvdW) energies is ΔEMM, the change in MM energy contribution in the gas phase. ΔGsol is a change in solvation free energy, with a polar component (ΔGGB, electrostatic solvation energy) and a non-polar, non-electrostatic solvation contribution (ΔGSA). TΔS is the conformational entropy at binding. The production phase trajectory was divided into 20 segments of 50 ns length. Except for the drug dabrafenib (DAB), where the 300 ns trajectory was divided into 6 segments of 50 ns length. From each segment, 100 geometries were sampled at regular time steps and ΔGbind was calculated. The final ΔGbind was expressed as the mean ± standard deviation for all 20 segments. The calculated MM/GBSA free energies of binding were further decomposed into the specific contributions for each residue. In this way, the contributions of each amino acid side chain to ΔGbind were determined and the nature of the energy change in terms of interaction and solvation energies, or entropic contributions were identified.79 Since we are comparing the energies of the same receptor and of ligands with approximately the same size and number of rotatable bonds, entropic contributions (–TΔS) were neglected.
2.1.3.2 Cluster analysis. The structures of each complex were clustered based on the RMSD of the Cα atoms of each residue using the k-means algorithm. The maximum number of iterations was set to 1000, with randomized initial set of points and sieving set was equal to 10. The frames closest to the centroids of the clusters were identified and considered as representative structures of the conformations. The CPPTRAJ module80 was used to perform the cluster analysis.
3. Results and discussion
3.1 Gaussian-based QSAR model generation
Model was generated on structurally aligned pyrimidine–sulfonamide hybrid derivatives (1–51) with BRAFV600E inhibitory activity. There was a moderate disparity between the actual and predicted values of the training and test molecules, which is known as residual activity, and it was within the Gaussian models' allowed ranges (<1), this indicates strong linear correlation.
The developed QSAR models were evaluated using the following parameters: squared correlation coefficient (r2), cross-validated correlation coefficient (q2 training), r2 scramble, stability, Fisher's test (the variance ratio indicating the statistical significance of the model), p-value (magnitude of the variance ratio, where small p-values usually indicate a higher degree of significance), SD (SD of the regression), RMSE test (root-mean-square error of the test set), q2 test (value for the predicted activities of the test set) and Pearson-r (correlation coefficient between observed and predicted activities for the test set).81
3.1.1 Gaussian-based 3D-QSAR model. Model was generated using PLS with five factors, including steric, electrostatic, hydrophobic, HBD, and HBA fields of the training set.59 The rCV2 value of 0.7873 was derived from the cross-validation leave one out (LOO) method, while non-cross-validation analysis yielded an r2 value of 0.9603. SD of the regression is 0.1855, Fischer's ratio value is 140.5, and stability of the model is 0.87. The statistical summary of the model is listed in Table 3.
Table 3 Statical results obtained using Gaussian-based 3D-QSAR models
Statistical parameters of generated 3D QSAR model |
PLS data |
Gaussian |
Acceptable value |
SD |
0.1855 |
SD of regression < SD of actual activities (1) |
r2 |
0.9603 |
1 > r2 > 0.5 |
rCV2 |
0.7873 |
>0.6 |
r2 scramble |
0.6879 |
<0.6 |
Stability |
0.87 |
>0.6 or = 1 |
F |
140.5 |
Larger values of F |
p |
2.10 × 10−19 |
Smaller values of p, greater degree of confidence |
RMSE |
0.53 |
Close to 1 |
q2 |
0.6384 |
>0.5, and (R2 − Q2) 0.3 |
Pearson-r |
0.806 |
Close to 1, lesser scattered predictive activities in the plot of observed vs. predicted activities |
Field contribution (%) |
Gaussian steric |
0.416 |
Gaussian electrostatic |
0.114 |
Gaussian hydrophobic |
0.189 |
Gaussian H-bond acceptor |
0.118 |
Gaussian H-bond donor |
0.184 |
The results for this model showed that the steric and electrostatic contributions were, respectively, 0.416 and 0.114. This implies that for protein–ligand interactions, the steric field is more significant than the electrostatic groups. Steric and electrostatic field contributions are given in (Table 3 and Fig. 3). The pIC50 values were predicted using 15 test set inhibitors for model validation. The predictive q2 for these models was 0.6384, which shows that they have a respectable level of predictive power. The contributions of the hydrophobic, HBD, and HBA fields were 0.169, 0.184, and 0.118, respectively (Table 3). The field contributions of the steric and hydrophobic intensities were more than those of the electrostatic, HBA, and HBD fields, indicating that the importance of the steric and hydrophobic fields for protein–ligand interactions is greater.
 |
| Fig. 3 Gaussian based 3D-QSAR model. (a) Potent BRAFV600E inhibitor [IC50 = 1.200 nM; (S. No. 30)] (b) steric contour map (c) electrostatic contour map (d) hydrophobic contour map (e) H-bond acceptor contour map (f) H-bond donor contour map. Relation between experimental and predicted BRAFV600E inhibitory activity values of (g) all set (pIC50) (h) test set (pIC50) (i) training set (pIC50). | |
3.1.2 Different Gaussian contour maps. The contours of each of the five PLS components of the training set are described and shown in Fig. 3. In the Gaussian steric contour map (Fig. 3b), the phenyl ring (green contours) and the imidazo[2,1-b] thiazole moiety (red contours) are shown as favourable and unfavorable sites for steric interactions respectively.The imidazo[2,1-b] thiazole moiety (green contours) with electropositive groups and the phenyl ring (red contours) with electronegative groups are active in the Gaussian electrostatic contour map (Fig. 3c). The green contour maps over the phenyl ring (Fig. 3d) of the Gaussian hydrophobic contour map show that they are hydrophobic, whereas the red contour maps over the imidazo[2,1-b] thiazole moiety show that they are not hydrophobic and may reduce BRAFV600E activity. The hydrogen bond acceptor (HBA) groups in the phenyl ring (red contour) are unfavorable, whereas the HBA groups in the sulfonamide moiety (green contour) are favorable for activity, in the Gaussian HBA contour map (Fig. 3e). The green contour with the phenyl ring has a favorable hydrogen bond donor (HBD) group in the Gaussian HBD contour map (Fig. 3f), and substituting HBD groups at this position may boost BRAFV600E activity.
3.1.3 Validation of Gaussian-based 3D-QSAR model. Predicted versus experimental activity of the training and test sets was plotted to demonstrate the model's validity see Fig. 3g–i. Comparison of the experimentally observed and predicted pIC50 values of pyrimidine–sulfonamide hybrid inhibitors, shows that the Gaussian model performs well in predicting the activities for both training and test molecules. After analyzing the statistical parameters, we came to the conclusion that the models had good predictability and offered precise information about the chemical properties of the ligands, which would contribute to antagonistic activity against BRAFV600E.Fig. 4 shows the structural requirements of the designed pyrimidine–sulfonamide hybrid derivatives based on 3D-QSAR. In designed compound Fig. 4, the alkyl chain of potent compound Fig. 3a is replaced by phenyl ring due to its higher hydrophobicity and imidazothiazole moiety was eliminated. Structure activity relationship (SAR) of designed compound on the basis of different parameters like hydrogen bond acceptor (HBA), (hydrogen bond donor) HBD, electrostatic hydrophobic and steric requirements at specific position are also depicted in Fig. 4.
 |
| Fig. 4 Structural requirements of pyrimidine–sulfonamide hybrid derivatives for BRAFV600E activity and selectivity based on the analysis of Gaussian based contour maps. | |
3.2 Rationality of designed compound compared with USFDA approved BRAF inhibitors
Based on a Gaussian-based 3D-QSAR model, the developed pyrimidine–sulfonamide hybrid derivative's structural moieties were assessed with the FDA-approved BRAF inhibitors: first-generation – sorafenib and second generation – dabrafenib, vemurafenib, and encorafenib for rationality.
Pyrimidine and sulfonamide (SO2NH2) moieties were selected as a core moiety for development of new BRAFV600E inhibitors. The pyrimidine moiety is similar to the most potent drugs on the market, dabrafenib and encorafenib. The sulfonamide moiety is similar to all 2nd generation drugs. The N-phenyl ring moiety has similarity with sorafenib (1st generation), dabrafenib, vemurafenib, and encorafenib (2nd generation). The rational of the designed compound is depicted in Fig. S1.†
3.2.1 Different derivatives of designed pyrimidine–sulfonamide hybrid moieties. Importance of different function groups, such as OH (HBA/HBD), NO2, Cl, Br, F, and I (electron-withdrawing), OCH3, OH (electron-donating group), different heterocyclic moieties, such as quinoline, pyrrole, thiophene, and naphthol (steric requirements), were chosen based on the SAR of the designed compound (Fig. 4). On the basis of above findings, a library of 88 newly designed compounds was created and is shown in Fig. 5 and Table S1.†
 |
| Fig. 5 Structure of new designed pyrimidine–sulfonamide hybrid compounds. | |
3.3 Molecular docking and molecular dynamics simulations
Being aware of the potential problems with molecular docking experiments,82 we docked 92 compounds (88 designed compounds and 4 FDA approved BRAF inhibitor drugs) to the kinase receptor. The docking procedure was validated by redocking dabrafenib to the hBRk binding site. The root mean squared deviation (RMSD) between the experimental and docked structure is small (0.47 Å). Additionally, the rotation of the dabrafenib's methyl group contributes the most to the overall RMSD, so we have demonstrated the correctness of our approach. The superimposed experimental and docked dabrafenib structures in the enzyme pocket are displayed in Fig. S2.† Among all docked compounds, dabrafenib performed best with a docking score of −12.7 kcal mol−1. Four molecules, T109, T183, T160 and T126 had scores below −12 kcal mol−1, so they were selected for detailed studies. Binding poses of T109 (red), T126 (orange), T160 (sea green), T183 (sky blue), and the reference drug dabrafenib (magenta) as determined by molecular docking to the BRAF kinase protein pocket are depicted in Fig. S3.† It is interesting that none of the docked hit molecules nor dabrafenib (docked or experimentally determined structure) are in contact with Glu600. Fig. S4† shows docked T160 (sea green) and dabrafenib (magenta) to BRAF. CA atom of Glu600 (black) is more than 14 Å apart from the nearest non-hydrogen atom of T160/dabrafenib ligands. All docking scores are collected in Table S2.†
After visual inspection of the best docked poses of the top 4 compounds forming our group of hit molecules, they served as initial geometries for MD simulations. Before conclusions were drawn, the conformational dynamics and stability of each complex were examined using a 1 μs long molecular dynamics simulation. The RMSD and radius of gyration (RoG) of the backbone αC atoms of the receptor relative to the initial structures were calculated and the stability of the complexes was monitored. Flexibility of the residues' side chain was evaluated using RMSF. The compounds were ranked based on their ability to bind to hBRk, and their interaction pattern was investigated.
3.3.1 Molecular dynamics simulation study of compound T 160. In the first 900 ns of the simulation, the RMSD fluctuated around a mean value of 2.44 Å. Then an increase in RMSD was observed, and the mean value for the last 100 ns was 3.05 Å. These changes prompted us to perform a cluster analysis to identify relevant changes in the complex structure. We chose to use the k-means algorithm, where k ranges from 2 to 5. The results were analyzed using the Davies–Bouldin index (DBI), the pseudo-F statistic (pSF), and the ratio between the sum of squares of the regression and the sum of squares of error (SSR/SST) (Table S3†). The analysis confirmed the presence of three relevant conformations with selected parameters collected in Table S4.† For each conformation, a representative structure was identified as a frame closest to the cluster centroid. The distribution of the conformations of the T160:BRAF kinase (T160:hBRk) complex along the trajectory and their RMSD relative to the initial frame are shown in Fig. 6. Along with the increase in the mean RMSD values after the 900 ns mark, a slight decrease in the radius of gyration (RoG) from 19.24 Å to 19.15 Å was observed.
 |
| Fig. 6 Molecular dynamics trajectory analysis for T160:hBRk complex. RMSD (left) and RoG (right). | |
In all three conformations, the secondary structure was generally well preserved. However, there were some minor changes in the secondary structure that could affect the geometry of the catalytic pocket Fig. 7. The first region includes residues that are in direct contact with the ligand – Arg462, Ile463, Gly464, and Ser465, while these residues do not have a defined secondary structure in the dominant conformation A, they form a β-sheet in conformations B and C. The second region with considerable changes is the region between Ile592 and Phe 635, which is almost completely without defined secondary structure. In the crystal structure (Fig. S5†)83 and in conformation A, only Pro622 to Ile625 from this region form an H4 α-helix. In conformation B, the H4 helix is absent but two new helices are formed (Glu611-Leu613 and Ile617-Trp619). The H4 helix and the Ile617–Trp619 helix are present in the C conformation.
 |
| Fig. 7 Structural differences between conformations A (blue), B (red) and C (green). Superposition of three structures of the T160:hBRk complex representing three conformations. | |
The flexibility of the residues of the hBRk protein in the complex was examined by calculating root mean squared fluctuations (RMSF) for each residue (Fig. 8). High RMSF values indicated greater fluctuations and flexibility. The averaged RMSF value was 1.55 Å. Almost all of the first 50 residues were having higher than average RMSF values, indicating high flexibility of the N-terminus of the kinase. The highest RMSF value was having Met627, a residue whose side chain pointed toward the solvent, far from the binding site of the ligand. The previously mentioned unstructured region was having two fragments with RMSF values above 3 Å. These two fragments, Ser607–Ser614 and Ile625–Asn631, were not in direct contact with the ligand. It is important to note that the catalytically relevant residues Asp594, Phe595 and Gly596 have RMSF values below 1 Å.
 |
| Fig. 8 Root mean square fluctuations per residue of the T160:hBRk complex. | |
Hydrogen bond analysis revealed that on average 2.11 hydrogen bonds were established between T160 and the kinase. This is on average only 0.02 less than in the complex with T183 and the hBRk. The predominant hydrogen bond formed in almost 75% of the simulation time was between the hydroxyl group of Thr529 (hydrogen acceptor) and the N4 atom of T160 with an average bond length of 1.89 ± 0.08 Å. Changes in the 3D structure of the protein were reflected in the hydrogen bonding patterns in different conformations. For example, in A, T160 formed four hydrogen bonds with three residues (two with Ser465 and one with Thr529 and Asp594). In addition, 15 residues had at least one heavy atom in a 4.0 Å zone away from the non-hydrogen atoms of T160. This illustrated the large number of stabilizing van der Waals interactions. In conformation B, only two hydrogen bonds were formed (with Lys483 and Thr529). Finally, conformation C exhibited three hydrogen bonds. The detailed interactions are shown in Fig. 9.
 |
| Fig. 9 Hydrogen bonds patterns in three T160:hBRk conformations. | |
Although the MD simulation for DAB was 300 ns long, some conclusions can be drawn. The flexibility of residues changes slightly when T160 is replaced in the catalytic pocket by dabrafenib, the drug approved for the treatment of melanoma with V600E or V600K mutation (Fig. S6†), with a slight increase in the flexibility of residues Ala544–Lys548 and a decrease in the flexibility of Ile625–Asn631. In addition, dabrafenib has on average one more hydrogen bond than T160, being predominantly (90% of the simulation time) bound to the Gly593 oxygen atom with an average hydrogen bond length of 1.84 Å, which is shorter than the hydrogen bond between Thr529 and T160. Numerous van der Waals interactions together with a higher number of hydrogen bonds could be responsible for the higher binding affinity to hBRk than T160.
3.3.2 Calculation of the free energy of binding. The binding affinity of the hit molecules and the reference drug dabrafenib was estimated using the MM/GBSA approach, in which the free energies of solvation were determined by solving the generalized Born equation. For the complex T160:hBRk, the free energy of binding without entropy contribution was −59.1 ± 2.6 kcal mol−1. T160 had the highest binding potential to hBRk of all the compounds studied. The MM/GBSA binding free energy decomposition was used to identify key residues with dominant contribution to protein–ligand binding. In the study of the SARS-CoV-2 virus, its main protease84 and the NS3 protease of Kyasanur forest disease virus,85 a threshold of −1.5 kcal mol−1 was set for the free energy of binding of a single residue to classify it as a residue with dominant contribution, and the same criteria was applied in the present study. Table 4 lists the residues with dominant contributions for T109, T126, T160, and T183 and dabrafenib. Fig. 10 shows the main interactions based on the decomposition of the binding free energy. In addition to the polar and uncharged Thr529 and the positively charged Lys483, four hydrophobic residues (Val471, Leu505, Leu514, Trp531, and Phe583) contributed significantly to the binding free energy of T160. This finding confirmed the results of previous analyzes on the importance of van der Waals interactions.
Table 4 Contributions of the most important amino acid residues for the binding of T109, T126, T160, T183 and dabrafenib to hBRk
T109 |
T126 |
T160 |
T183 |
Dabrafenib (DAB) |
Residue |
ΔGbind |
Residue |
ΔGbind |
Residue |
ΔGbind |
Residue |
ΔGbind |
Residue |
ΔGbind |
Lys483 |
−6.86 |
Lys483 |
−2.68 |
Thr529 |
−3.63 |
Lys483 |
−3.09 |
Gly593 |
−3.45 |
Asp594 |
−3.93 |
Phe583 |
−2.27 |
Lys483 |
−2.74 |
Asp594 |
−2.38 |
Trp531 |
−2.63 |
Ile527 |
−2.21 |
Leu514 |
−2.19 |
Leu514 |
−2.43 |
Val471 |
−2.08 |
Phe583 |
−2.36 |
Val471 |
−2.07 |
Val471 |
−2.16 |
Val471 |
−2.05 |
Gly593 |
−2.07 |
Thr529 |
−2.23 |
Phe583 |
−1.77 |
Thr529 |
−1.80 |
Leu505 |
−1.85 |
Ile463 |
−2.02 |
Leu514 |
−1.93 |
Leu514 |
−1.51 |
Ile463 |
−1.73 |
Phe583 |
−1.77 |
Leu514 |
−1.97 |
Val471 |
−1.89 |
|
|
Leu505 |
−1.58 |
Trp531 |
−1.62 |
Ile527 |
−1.92 |
Ile527 |
−1.81 |
|
|
|
|
|
|
Phe583 |
−1.91 |
Cys532 |
−1.70 |
|
|
|
|
|
|
Leu505 |
−1.56 |
|
|
 |
| Fig. 10 Insight into the catalytic site neighborhood of T160 in hBRk, with residues with the most favorable single residue binding free energy. | |
As expected, the Gly593 residue, which forms the hydrogen bond, has the largest contribution to the binding of dabrafenib (DAB). Other stabilizing interactions are established with the electron-rich residues Phe583 and Trp531, the hydrophobic Val471, Leu514, and Ile527, and the polar Thr529 and Cys532. The free energy of binding for the DAB:hBRk complex was estimated to be −61.7 ± 1.0 kcal mol−1, slightly better than for T160:hBRk.
The results obtained by the analysis of Gaussian-based contour maps suggest that the introduction of the HBA group at the phenyl moiety should increase the activity of the pyrimidine–sulfonamide hybrid derivatives. Comparing compounds T160 and T126, which differ only in the presence of a nitro group at the para position of the phenyl moiety in T160, one can observe a drastic change in the binding free energy for these two compounds. While the binding free energy for T160 is −59.1 ± 2.6 kcal mol−1, it is only −39.7 ± 2.2 kcal mol−1 for T126 (vide infra). The MD simulations revealed that the nitro group sits in a hydrophobic pocket and is surrounded by hydrogen atoms. To gain a deeper insight into the nature of why some structural modifications predicted by Gaussian-based contour maps contribute to higher selectivity, additional MD simulations are needed, including non-substituted pyrimidine–sulfonamide scaffolds, but this is beyond the scope of the present manuscript.
3.3.3 Compound T109, T126 and T183 molecular dynamics simulations study. The same protocol used for the analysis of the T160:hBRk complex was applied to the analysis of the T109:hBRk, T126:hBRk, and T183:hBRk complexes. The stability of the complexes was confirmed by tracking RMSD and RoG along the trajectory (Fig. S7†).All complexes remained stable but had higher averaged RMSD values compared to the T160:hBRk complex. The RoG values were very similar and range from 18.93 Å to 19.29 Å. The existence of multiple conformations was confirmed by cluster analysis. The cluster analysis data are summarized in Tables S5–S10.† Three complexes shared the presence of an initial short-lived conformation that converts to another conformation within 100 ns. The relative ratios between the populations of conformations A and B are approximately 0.6 to 0.3.
The primary geometric difference between the conformations of T109:hBRk and T126:hBRk, just as with T160:hBRk, was in unstructured fragments. However, in T183:hBRk, conformations A and B showed differences in secondary structure motifs compared to the representative structure of the initial conformation C (Fig. 11). For example, a shift of the H6 α-helix axis was observed. Slightly less pronounced was the shift of the H1 α-helix axis, but it played a larger role in the geometry of the binding site. While the C-terminus of the H1 α-helix had van der Waals contacts with the catalytic Phe595 residue, the N-terminus is tilted toward the unstructured loop region, reducing the volume of the cleft.
 |
| Fig. 11 Superposition of three structures of the T183:hBRk complex representing three conformations with circled regions of major structural rearrangements. Conformations A (blue), B (red) and C (green). | |
hBRk residues had higher average RMSF values, 1.81 Å and 1.80 Å, when T183 and T126 were bound in the active pocket, respectively, compared with T109 (1.55 Å). The RMSF diagram (Fig. S8†) shows similar flexibility patterns. Again, the N-terminus and unstructured regions exhibited above average flexibility, while the conserved triad, which was important for catalysis, exhibited low flexibility. T126 had the lowest average number of hydrogen bonds (0.95) between ligand and the receptor. The hydrogen bond connecting the hydroxyl oxygen of Thr529 and the NH group of T126 was present only during 23% of the simulation time, with an average length of 1.91 Å. For comparison, the analogous bond in T160:hBRk was present for about 75% of the simulation time. In T183:hBRk, Gly593 acted as a hydrogen bond acceptor, and the 1.83 ± 0.10 Å long bond was present during 82% of the trajectory. In addition to hydrogen bonding, van der Waals interactions also contributed to free energy of binding. While the free energy of binding of T109 and T183 was almost within 1 kcal mol−1 (−55.5 ± 3.1 kcal mol−1 and −56.6 ± 3.0 kcal mol−1, respectively), the value for T126 is only 39.7 ± 2.2 kcal mol−1. T109, T160, and T183 can serve as excellent starting points for introducing chemical modifications to optimize their potential to inhibit BRAF kinase.
4. Binding study of BRAF inhibitors and designed compounds with DFG motif and αC-helix of BRAFV600E protein and their conformation
BRAF inhibitors are having 4 different types binding conformations with DFG motif and αC-helix.86 Employing this notion, by using molecular docking and simulation studies, we succeeded in locating our designed molecule's binding conformation with the DFG motif and αC-helix (Fig. 12).
 |
| Fig. 12 FDA inhibitors and designed compound interaction with different region of BRAFV600E and their binding conformation on the basis of DFG motif and αC-helix. | |
The Asp594 residue of the DFG motif was facing the active site of the kinase in the designed compounds (T109, T126, T160 and T183). Compounds T109, T126, T160 and T183 have interactions with the hinge region (ATP-binding site) by forming ∼1–3 hydrogen bonds and hydrophobic interaction around the adenine regions of the ATP binding site of the protein. The designed compounds also has interactions with other various regions of ATP, including hydrophobic regions adjacent to the adenine region, the ribose region, and the solvent assessable region (DFG-IN). The αC-helix away from the ATP site is referred to as the αC-OUT position. Thus, designed compounds interacted with the [αC-OUT/DFG-IN] confirmation, just like dabrafenib, encorafenib and vemurafenib.23,87 Additionally, compounds T126, T160, and T183 interact with the Leu505 amino acid sequence at the dimerization interface (DIF). BRAF dimerization contributes to the pathogenic function of disease-associated mutant Raf proteins and exhibits activity similar to the constitutively active BRAFV600E mutant once this activated state has been attained. It has also been found to alter therapeutic responses and disease progression in patients treated with BRAF inhibitors.88 Oncogenic BRAF dimers shown resistance against BRAF inhibitors and causes paradoxical activation.89
5. Conclusion
The pyrimidine–sulfonamide hybrid derivatives were designed based on the different structural moieties of the first and second-generation BRAF inhibitors. 3D-QSAR and molecular docking studies were performed for the designed compounds to determine their binding affinity. Molecular dynamics simulations were performed to understand the flexibility, structural conformation changes and interaction pattern of the mutant BRAF kinase with hit compounds. Analysis of the Gaussian-based 3D-QSAR models showed that the contribution of steric and hydrophobic fields was higher than the other field intensities. These models provided considerable insight into the key properties of the inhibitors, their structural features, and their inhibitory potential. The developed compounds showed good interactions with the core active site [the nucleotide (ADP or ATP) binding site, DFG motif, the phospho-acceptor site (activation segment), adjacent to the DFG motif and the αC-helix] of the BRAFV600E protein. Similar to the FDA-approved BRAFV600E inhibitors the developed compounds have [αC-OUT/DFG-IN] conformation and compound T126, T160, and T183 interacted with dimerization interface and may effective against malignancies driven by dimer interface. The synthesis of these designed molecules is in progress. The synthesized molecules will be tested further to support the results of in silico studies.
Author contributions
Conceptualization: Pradeep Kumar; computational methodology and data interpretation: Ankit Kumar Singh, Jurica Novak, Adarsh Kumar, Vikas Pathania, Suresh Thareja, Prateek Pathak, Maria Grishina and Mariusz Jaremko; data curation and figure drawing: Harshwardhan Singh, Jagat Pal Yadav, and Jurica Novak. The interpretation of the results, writing, and reviewing of the manuscript: Ankit Kumar Singh, Prateek Pathak, Hemraj Nandanwar, Jurica Novak, Maria Grishina, Amita Verma, Abdul-Hamid Emwas, Habibullah Khalilullah and Pradeep Kumar.
Abbreviations
ADP | Adenosine diphosphate |
ATP | Adenosine triphosphate |
CR | Conserved regions |
CRD | Cysteine-rich domain |
DAB | Dabrafenib |
DIF | Dimerization interface |
FDA | Food and drug administration |
3D-QSAR | Three-dimensional quantitative structure–activity relationship |
hBRk | Human BRAF kinase |
LOO | Leave one out |
MAPK | Mitogen-activated protein kinase |
MD | Molecular dynamics |
MM/GBSA | Molecular mechanics/generalized Born surface area |
PLS | Partial least square |
RMSD | Root mean squire deviation |
RMSE | Root mean squire error |
RMSF | Root mean squared fluctuations |
RoG | Radius of gyration |
Conflicts of interest
The authors declare no competing interest.
Acknowledgements
The APC was funded by King Abdullah University of Science and Technology (KAUST), Thuwal, Jeddah, Saudi Arabia. Authors are thankful to Central University of Punjab, Bathinda, DST-FIST, India; Isabella cluster of the University Computing Center, University of Zagreb, Croatia; South Ural State University, Chelyabinsk, Russia; for providing the necessary facilities to execute this research. Dr Prateek Pathak and Dr Maria Grishina also acknowledged that their part of the work was supported by Ministry of Science and Higher Education of Russia (Grant FENU-2020-0019).
References
- H. M. Gloster Jr and K. Neal, J. Am. Acad. Dermatol., 2006, 55, 741–760 CrossRef.
- J. Y. Lin and D. E. Fisher, Nature, 2007, 445, 843–850 CrossRef CAS PubMed.
- A. Sample and Y. Y. He, Photodermatol., Photoimmunol. Photomed., 2018, 34, 13–24 CrossRef CAS.
- H. Sung, J. Ferlay, R. L. Siegel, M. Laversanne, I. Soerjomataram, A. Jemal and F. Bray, Ca-Cancer J. Clin., 2021, 71, 209–249 CrossRef.
- J. A. McCubrey, L. S. Steelman, W. H. Chappell, S. L. Abrams, E. W. Wong, F. Chang, B. Lehmann, D. M. Terrian, M. Milella and A. Tafuri, Biochim. Biophys. Acta, Mol. Cell Res., 2007, 1773, 1263–1284 CrossRef CAS.
- C. M. Beneker, M. Rovoli, G. Kontopidis, M. Röring, S. Galda, S. Braun, T. Brummer and C. McInnes, J. Med. Chem., 2019, 62, 3886–3897 CrossRef CAS PubMed.
- X. R. Han, L. Chen, Y. Wei, W. Yu, Y. Chen, C. Zhang, B. Jiao, T. Shi, L. Sun and C. Zhang, J. Med. Chem., 2020, 63, 4069–4080 CrossRef CAS.
- H. B. El-Nassan, Eur. J. Med. Chem., 2014, 72, 170–205 CrossRef CAS.
- G. Bollag, P. Hirth, J. Tsai, J. Zhang, P. N. Ibrahim, H. Cho, W. Spevak, C. Zhang, Y. Zhang and G. Habets, Nature, 2010, 467, 596–599 CrossRef CAS PubMed.
- A. Kiełbik, P. Wawryka, A. Chwiłkowska, J. Saczko and J. Kulbacka, Med. Res. J., 2019, 4, 184–188 CrossRef.
- B. Domingues, J. M. Lopes, P. Soares and H. Pópulo, ImmunoTargets Ther., 2018, 7, 35 CrossRef CAS.
- G. Saldanha, L. Potter, P. DaForno and J. H. Pringle, Clin. Cancer Res., 2006, 12, 4499–4505 CrossRef CAS PubMed.
- J. Zhang, B. Lu, D. Liu, R. Shen, Y. Yan, L. Yang, M. Zhang, L. Zhang, G. Cao and H. Cao, Cancer Biol. Ther., 2016, 17, 199–207 CrossRef CAS PubMed.
- P. N. Ibrahim, J. Zhang, C. Zhang and G. Bollag, in Ann. Rep. Med. Chem., Elsevier, 2013, vol. 48, pp. 435–449 Search PubMed.
- M. W. Rowbottom, R. Faraoni, Q. Chao, B. T. Campbell, A. G. Lai, E. Setti, M. Ezawa, K. G. Sprankle, S. Abraham and L. Tran, J. Med. Chem., 2012, 55, 1082–1105 CrossRef CAS PubMed.
- A. B. Umar, A. Uzairu, G. A. Shallangwa and S. Uba, Future J. Pharm. Sci., 2020, 6, 1–10 CrossRef.
- N. Dhomen and R. Marais, Hematol. Oncol. Clin., 2009, 23, 529–545 CrossRef PubMed.
- E. Dratkiewicz, A. Simiczyjew, K. Pietraszek-Gremplewicz, J. Mazurkiewicz and D. Nowak, Int. J. Mol. Sci., 2019, 21, 113 CrossRef PubMed.
- M. Ottaviano, E. F. Giunta, M. Tortora, M. Curvietto, L. Attademo, D. Bosso, C. Cardalesi, M. Rosanova, P. De Placido and E. Pietroluongo, Int. J. Mol. Sci., 2021, 22, 3474 CrossRef CAS.
- P. A. Ascierto, J. M. Kirkwood, J.-J. Grob, E. Simeone, A. M. Grimaldi, M. Maio, G. Palmieri, A. Testori, F. M. Marincola and N. Mozzillo, J. Transl. Med., 2012, 10, 1–9 CrossRef.
- O. P. Van Linden, A. J. Kooistra, R. Leurs, I. J. De Esch and C. De Graaf, J. Med. Chem., 2014, 57, 249–277 CrossRef CAS PubMed.
- A. Vulpetti and R. Bosotti, Il Farmaco, 2004, 59, 759–765 CrossRef CAS PubMed.
- A. K. Singh, A. Kumar, S. Thareja and P. Kumar, Anti-Cancer Agents Med. Chem., 2022 DOI:10.2174/1871520622666220624164152.
- T. Niihori, Y. Aoki, Y. Narumi, G. Neri, H. Cavé, A. Verloes, N. Okamoto, R. Hennekam, G. Gillessen-Kaesbach and D. Wieczorek, Nat. Genet., 2006, 38, 294–296 CrossRef CAS PubMed.
- H. C. Tang and Y. C. Chen, Int. J. Nanomed., 2015, 10, 3131 CrossRef CAS.
- M. Dankner, A. A. Rose, S. Rajkumar, P. M. Siegel and I. R. Watson, Oncogene, 2018, 37, 3183–3199 CrossRef CAS PubMed.
- B. Agianian and E. Gavathiotis, J. Med. Chem., 2018, 61, 5775–5793 CrossRef CAS PubMed.
- M. A. Rahman, A. Salajegheh, R. A. Smith and A. Y. Lam, Crit. Rev. Oncol. Hematol., 2014, 90, 220–232 CrossRef CAS PubMed.
- A. Zambon, I. Niculescu-Duvaz, D. Niculescu-Duvaz, R. Marais and C. J. Springer, Bioorg. Med. Chem. Lett., 2012, 22, 789–792 CrossRef CAS PubMed.
- H. F. Li, Y. Chen, S. S. Rao, X. M. Chen, H. C. Liu, J. H. Qin, W. F. Tang, X. Zhou and T. Lu, Curr. Med. Chem., 2010, 17, 1618–1634 CrossRef CAS PubMed.
- J. H. Pan, H. Zhou, S. B. Zhu, J. l. Huang, X. X. Zhao, H. Ding and Y. l. Pan, Cancer Manage. Res., 2018, 10, 2289 CrossRef CAS PubMed.
- P. Wu, T. E. Nielsen and M. H. Clausen, Trends Pharmacol. Sci., 2015, 36, 422–439 CrossRef CAS PubMed.
- M. Angiolini, Struct. Biol. Drug Discovery, 2020, 363–393 CAS.
- Z. Zhao, H. Wu, L. Wang, Y. Liu, S. Knapp, Q. Liu and N. S. Gray, ACS Chem. Biol., 2014, 9, 1230–1241 CrossRef CAS PubMed.
- S. Whittaker, R. Kirk, R. Hayward, A. Zambon, A. Viros, N. Cantarino, A. Affolter, A. Nourry, D. Niculescu-Duvaz and C. Springer, Sci. Transl. Med., 2010, 2, 35ra41 Search PubMed.
- I. C. Waizenegger, A. Baum, S. Steurer, H. Stadtmüller, G. Bader, O. Schaaf, P. Garin-Chesa, A. Schlattl, N. Schweifer and C. Haslinger, Mol. Cancer Ther., 2016, 15, 354–365 CrossRef CAS PubMed.
- G. Verkhivker, Mol. BioSyst., 2016, 12, 3146–3165 RSC.
- M. Imran, S. M. B. Asdaq, S. A. Khan, D. Unnikrishnan Meenakshi, A. S. Alamri, W. F. Alsanie, M. Alhomrani, Y. Mohzari, A. Alrashed and M. AlMotairi, Pharmaceuticals, 2021, 14, 710 CrossRef CAS PubMed.
- L. E. Campos, F. Garibotto, E. Angelina, J. Kos, T. Gonec, P. Marvanova, M. Vettorazzi, M. Oravec, I. Jendrzejewska and J. Jampilek, Bioorg. Chem., 2020, 103, 104145 CrossRef CAS.
- A. D. Ballantyne and K. P. Garnock-Jones, Drugs, 2013, 73, 1367–1376 CrossRef CAS PubMed.
- G. T. Gibney and J. S. Zager, Expert Opin. Drug Metab. Toxicol., 2013, 9, 893–899 CrossRef CAS PubMed.
- R. H. Smith, Z. M. Khan, P. M.-U. Ung, A. P. Scopton, L. Silber, S. M. Mack, A. M. Real, A. Schlessinger and A. C. Dar, Biochemistry, 2021, 60, 289–302 CrossRef CAS PubMed.
- J. Kim, B. Choi, D. Im, H. Jung, H. Moon, W. Aman and J.-M. Hah, J. Enzyme Inhib. Med. Chem., 2019, 34, 1314–1320 CrossRef CAS PubMed.
- S. Jang and M. Atkins, Clin. Pharmacol. Ther., 2014, 95, 24–31 CrossRef CAS PubMed.
- G. Kim, A. E. McKee, Y.-M. Ning, M. Hazarika, M. Theoret, J. R. Johnson, Q. C. Xu, S. Tang, R. Sridhara and X. Jiang, Clin. Cancer Res., 2014, 20, 4994–5000 CrossRef CAS PubMed.
- V. Asati, S. K Bharti and D. K Mahapatra, Anti-Cancer Agents Med. Chem., 2016, 16, 1558–1575 CrossRef CAS PubMed.
- A. A. Rose, Drugs Today, 2019, 55, 247–264 CrossRef CAS PubMed.
- J. V. Cohen and R. J. Sullivan, Clin. Cancer Res., 2019, 25, 5735–5742 CrossRef CAS PubMed.
- S. A. Luebker and S. A. Koepsell, Front. Oncol., 2019, 9, 268 CrossRef PubMed.
- I. Proietti, N. Skroza, N. Bernardini, E. Tolino, V. Balduzzi, A. Marchesiello, S. Michelini, S. Volpe, A. Mambrin and G. Mangino, Cancers, 2020, 12, 2801 CrossRef CAS PubMed.
- M. S. Abdel-Maksoud, U. M. Ammar and C.-H. Oh, Bioorg. Med. Chem., 2019, 27, 2041–2051 CrossRef CAS PubMed.
- E. M. Ali, R. F. A. El-Telbany, M. S. Abdel-Maksoud, U. M. Ammar, K. I. Mersal, S.-O. Zaraei, M. I. El-Gamal, S.-I. Choi, K.-T. Lee and H. K. Kim, Eur. J. Med. Chem., 2021, 215, 113277 CrossRef CAS PubMed.
- U. M. Ammar, M. S. Abdel-Maksoud, K. I. Mersal, E. M. Ali, K. H. Yoo, H. S. Choi, J. K. Lee, S. Y. Cha and C.-H. Oh, Bioorg. Med. Chem. Lett., 2020, 30, 127478 CrossRef CAS PubMed.
- M. S. Abdel-Maksoud, A. A. Mohamed, R. M. Hassan, M. A. Abdelgawad, G. Chilingaryan, S. Selim, M. S. Abdel-Bakky and M. M. Al-Sanea, Int. J. Mol. Sci., 2021, 22, 10491 CrossRef CAS PubMed.
- L. Ren, E. R. Laird, A. J. Buckmelter, V. Dinkel, S. L. Gloor, J. Grina, B. Newhouse, K. Rasor, G. Hastings and S. N. Gradl, Bioorg. Med. Chem. Lett., 2012, 22, 1165–1168 CrossRef CAS PubMed.
- H. Yuan, H. Liu, W. Tai, F. Wang, Y. Zhang, S. Yao, T. Ran, S. Lu, Z. Ke and X. Xiong, SAR QSAR Environ. Res., 2013, 24, 795–817 CrossRef CAS PubMed.
- L. Alonso-Cotchico, J. Rodríguez-Guerra, A. Lledos and J. D. Marechal, Acc. Chem. Res., 2020, 53, 896–905 CrossRef CAS PubMed.
- L. G. Ferreira, R. N. Dos Santos, G. Oliva and A. D. Andricopulo, Molecules, 2015, 20, 13384–13421 CrossRef CAS PubMed.
- S. Kalva, D. Vinod and L. M. Saleena, Med. Chem. Res., 2013, 22, 5303–5313 CrossRef CAS.
- Y. Singh, K. S. Sanjay, P. Kumar, S. Singh and S. Thareja, J. Biomol. Struct. Dyn., 2022, 1–18 CAS.
- K. Yao, P. Liu, H. Liu, Q. Wei, J. Yang, P. Cao and Y. Lai, J. Mol. Struct., 2019, 1189, 187–202 CrossRef CAS.
- J. Caballero, J. Mol. Graphics Modell., 2010, 29, 363–371 CrossRef CAS PubMed.
- M. K. Teli, Org. Med. Chem. Lett., 2012, 2, 1–10 CrossRef PubMed.
- R. Roskoski Jr, Pharmacol. Res., 2018, 135, 239–258 CrossRef PubMed.
- C. Zhang, W. Spevak, Y. Zhang, E. A. Burton, Y. Ma, G. Habets, J. Zhang, J. Lin, T. Ewing and B. Matusow, Nature, 2015, 526, 583–586 CrossRef CAS PubMed.
- E. F. Pettersen, T. D. Goddard, C. C. Huang, G. S. Couch, D. M. Greenblatt, E. C. Meng and T. E. Ferrin, J. Comput. Chem., 2004, 25, 1605–1612 CrossRef CAS PubMed.
- E. C. Meng, E. F. Pettersen, G. S. Couch, C. C. Huang and T. E. Ferrin, BMC Bioinf., 2006, 7, 1–10 CrossRef PubMed.
- M. Lovrić, J. M. Molero and R. Kern, Mol. Inf., 2019, 38, 1800082 CrossRef PubMed.
- G. M. Morris, R. Huey, W. Lindstrom, M. F. Sanner, R. K. Belew, D. S. Goodsell and A. J. Olson, J. Comput. Chem., 2009, 30, 2785–2791 CrossRef CAS PubMed.
- J. Wang, W. Wang, P. A. Kollman and D. A. Case, J. Mol. Graphics Modell., 2006, 25, 247–260 CrossRef CAS PubMed.
- W. R. Wang, R. J. Wolf, J. W. Caldwell and P. A. Kollman, J. Comput. Chem., 2004, 25, 92 Search PubMed.
- C. Tian, K. Kasavajhala, K. A. Belfon, L. Raguette, H. Huang, A. N. Migues, J. Bickel, Y. Wang, J. Pincay and Q. Wu, J. Chem. Theory Comput., 2019, 16, 528–552 CrossRef PubMed.
- T. J. Dolinsky, P. Czodrowski, H. Li, J. E. Nielsen, J. H. Jensen, G. Klebe and N. A. Baker, Nucleic Acids Res., 2007, 35, W522–W525 CrossRef PubMed.
- X. Duan, F. Liu, H. Kwon, Y. Byun, I. Minn, X. Cai, J. Zhang, M. G. Pomper, Z. Yang and Z. Xi, J. Med. Chem., 2020, 63, 3563–3576 CrossRef CAS PubMed.
- H. C. Andersen, J. Comput. Phys., 1983, 52, 24–34 CrossRef CAS.
- T. Darden, D. York and L. Pedersen, J. Chem. Phys., 1993, 10089 CrossRef CAS.
- D. Case, J. Berryman, R. Betz, D. Cerutti, T. Cheatham III, T. Darden, R. Duke, T. Giese, H. Gohlke and A. Goetz, AMBER 2016, University of California, San Francisco, 2016 Search PubMed.
- S. Genheden and U. Ryde, Expert Opin. Drug Discovery, 2015, 10, 449–461 CrossRef CAS PubMed.
- G. Rastelli, A. D. Rio, G. Degliesposti and M. Sgobba, J. Comput. Chem., 2010, 31, 797–810 CAS.
- D. R. Roe and T. E. Cheatham III, J. Chem. Theory Comput., 2013, 9, 3084–3095 CrossRef CAS PubMed.
- S. K. Verma and S. Thareja, PLoS One, 2017, 12, e0175318 CrossRef PubMed.
- Y. C. Chen, Trends Pharmacol. Sci., 2015, 36, 78–95 CrossRef PubMed.
- R. A. Laskowski, Nucleic Acids Res., 2001, 29, 221–222 CrossRef CAS PubMed.
- J. Novak, H. Rimac, S. Kandagalla, M. A. Grishina and V. A. Potemkin, Future Med. Chem., 2021, 13, 363–378 CrossRef CAS PubMed.
- S. Kandagalla, J. Novak, S. B. Shekarappa, M. A. Grishina, V. A. Potemkin and B. Kumbar, J. Biomol. Struct. Dyn., 2021, 1–17 CrossRef PubMed.
- X. Wang and J. Kim, J. Med. Chem., 2012, 55, 7332–7341 CrossRef CAS PubMed.
- S. B. Peng, J. R. Henry, M. D. Kaufman, W. P. Lu, B. D. Smith, S. Vogeti, T. J. Rutkoski, S. Wise, L. Chun and Y. Zhang, Cancer Cells, 2015, 28, 384–398 CrossRef CAS PubMed.
- N. P. Liau, A. Venkatanarayan, J. G. Quinn, W. Phung, S. Malek, S. G. Hymowitz and J. Sudhamsu, Biochem, 2020, 59, 3982–3992 CrossRef CAS PubMed.
- A. Tse and G. M. Verkhivker, PLoS One, 2016, 11, e0166583 CrossRef PubMed.
|
This journal is © The Royal Society of Chemistry 2022 |
Click here to see how this site uses Cookies. View our privacy policy here.