DOI:
10.1039/D2RA05176A
(Paper)
RSC Adv., 2022,
12, 32912-32922
Isolation of oligostilbenes from Iris lactea Pall. var. chinensis (Fisch.) Koidz and their anti-inflammatory activities†
Received
18th August 2022
, Accepted 10th November 2022
First published on 16th November 2022
Abstract
Iris lactea Pall. var. chinensis (Fisch.) Koidz (Iris lactea) is an herbaceous perennial widely distributed in China, India, and South Korea. Iris lactea has been extensively used in traditional Chinese medicine. The present study isolated a new oligostilbene (compound 1), together with three known oligostilbenes (compounds 2, 3 and 4) from the seeds of Iris lactea. The structures of these compounds were elucidated by HRESIMS, NMR, and chemical analyses. The network-based pharmacologic analysis platform was used to predict the target proteins related to inflammation of isolated compounds. Furthermore, the isolated compounds were tested for their anti-inflammatory effects in LPS-stimulated RAW 264.7 cells. In this network, 138 candidate targets of compounds related to its therapeutic effect on inflammation were identified. In addition, compounds 1, 2, 3 and 4 significantly decreased NO content and the IL-6 levels as well as the expression of COX-2 in LPS-stimulated RAW 264.7 cells.
Introduction
Iris lactea Pall. var. chinensis (Fisch.) Koidz (Iris lactea) is an herbaceous perennial that grows primarily in China, South Korea, Russia, and India. And Iris lactea is also widely distributed in Northeast China, North China, and Northwest China.1 Iris lactea is one of the most valuable iris plants. Because the flowers exhibit good appearance and gorgeous colors, it has high ornamental value and can be planted on the edges of garden roads, flower beds and flower borders, embellished in lawns, or directly used as ground cover plants.2 Iris lactea has a well-developed root system and strong drought resistance; therefore, it is also a useful sand-fixation and greening plant. In addition, as a halophyte, Iris lactea has strong salt resistance and can be used in the improvement of saline land.3 Iris lactea also showed strong Cd tolerance and accumulation ability, indicating significant potential for application in the phytoremediation of Cd-contaminated soil.4–6
In traditional Chinese medicine, Iris lactea is used to treat jaundice, pharyngitis, hemorrhoids, ulcer, blood vomiting and joint pain.7–9 Phytochemical studies identified flavones, isoflavones, glycosylflavones, xanthones, triterpenes, quinones, and stilbenes from Iris lactea.10–12 Remarkably, an increasing number of studies have been devoted to oligostilbenes, a group of natural products of stilbenes polymers.13,14 Oligostilbenes exhibit various pharmacological activities, including anti-fungal, anti-inflammation, anti-oxidation, anti-tumor, anti-HIV, anti-Alzheimer's disease and anti-diabetes.15–24 Our early study isolated three oligostilbenes from Iris lactea,25 to gain a more comprehensive understanding of the bioactive constituents of Iris lactea, we performed analyses of high-performance chromatography coupled to mass spectrometry and nuclear magnetic resonance. Four oligostilbenes, Vitisin A-13-O-β-D-glucoside (1), Vitisin D (2), hopeaphenol (3) and Vitisin A (4) were isolated. Among them, compound 1 is a new and to be identified in Iris lactea, whereas compound 3 is isolated from Iris lactea for the first time.
Inflammation is a protective response to harmful stimuli, including pathogen invasion. It is a critical procedure that the body can utilize to signal the immune system to maintain tissue homeostasis.26 However, failure to control inflammation can cause pathological changes that contribute to acute or chronic diseases, thereby resulting in a range of diseases including Alzheimer's disease, type 2 diabetes mellitus, and atherosclerosis.27 Therefore, it seems that anti-inflammation could be a crucial point of prevention of disorders. And natural compounds characterized as anti-inflammatory or immunomodulatory dietary supplements are hoped to be developed into health care product without side effects.28 There are accumulating studies have found that oligostilbenes exhibit strong anti-inflammatory. Ampelopsin C, Ampelopsin A and Vitisin B from Vitis thunbergii, and displayed strong PGE2 inhibition activities. Among, Ampelopsin C showed better PGE2 inhibitory effects than the other compounds surveyed, with an IC50 value of 15.52 μM.29 α-Viniferin was first isolated as an anti-inflammatory constituent of Caragana chamlagu, a Korean herbal medicine for arthritis. α-Viniferin at doses of 3–10 μM inhibited the synthesis of cyclooxygenase-2 (COX-2) transcription lipopolysaccharide (LPS)-stimulated murine RAW 264.7 macrophages.30
Network pharmacology is a platform for drug target analysis and molecular mechanism prediction, mainly constructs bio-information networks through methods such as literature mining, and analyzes network topology and selects specific signal nodes for multi-target prediction of drug action.31 Network pharmacology could be used to solve the problems caused by multi-component, multi-target and synergistic features in compound research,32 providing new approaches and strategies for new drug development.33
In this study, isolation and identification of four oligostilbenes from Iris lactea were studied. The anti-inflammation targets of all isolated compounds were predicted by network pharmacology. In addition, the effects of compounds 1, 2, 3 and 4 on anti-inflammation in LPS-stimulated RAW 264.7 cells were studied. These results suggest compounds 1, 2, 3 and 4 showed potent anti-inflammation activities.
Experimental
Collection of Iris lactea seeds and extraction of oligostilbenes
Seeds of Iris lactea were collected at Malian Lake of Inner Mongolia, China, in August 2018. The plant was verified by Dr Qingbo Gao (Northwest Institute of Plateau Biology, Chinese Academy of Sciences, Xining, China) after comparing with the voucher specimen in the Qinghai-Tibetan Plateau Museum of Biology (Xining, China) (reference no. 158719).
As previously described, oligostilbenes in Iris lactea were extracted under alkaline conditions followed by precipitation with acid.25 First, the seeds of Iris lactea were cleaned with water and dried in the shade. After de-coating, seed kernels (22.0 kg) were macerated with 5.0% NaOH aqueous solution (12 h, room temperature). The solution was filtrated and combined as the alkali extraction solution. The extraction solution was neutralized by H2SO4 which was added in a drop-wise manner under vigorous stirring. After realizing, a pH of 3.0, precipitation was initiated, which was allowed to finish automatically at room temperature. The precipitates were collected by centrifugation (4500×g, 10 min). Impurities were removed by re-dissolving the precipitates in 95% ethanol and drying under vacuum (60 °C). The resultant extracts (328.12 g) were stored at −20 °C.
Isolation of oligostilbenes
The oligostilbenes extracts (20.00 g) was dissolved in 50 mL of methanol and filtration (0.22 μm) to obtain a sample solution with the concentration of approximately 400 mg mL−1. The oligostilbenes extracts solution was further processed with preparative HPLC using a DAC50 column with the filler of Hedera C18 (particle size 10 μm). The mobile phase A was acetonitrile and the mobile B was water. The gradient elution step was 0–60 min, 20–30% A. The inject volume was 1 mL. The flow rate was 60 mL min−1. Four fractions (S1, S2, S3 and S4) were obtained through the above separation stage. Fraction S4 were determined to be monomeric compound after HPLC analysis and compound 4 was obtained without further separation or purification process. Then fraction S1, S2, and S3 were individually purified by a semi-preparative HPLC equipped with a Kromasil C18 (250 × 21.2 mm, 5 μm) and eluted with acetonitrile–water (30
:
70, v/v) to achieve compounds 1, 2, and 3, respectively.
The isolated compounds were subjected to HPLC analysis with a Kromasil C18 column (250 × 4.6 mm, 5 μm). Components were eluted with a 0–40 min gradient of 10–50% acetonitrile in water with a flow rate of 1 mL min−1. The detection wavelength was 210 nm. And the retention time was 25.5, 27.5, 28.0, and 32.5 min for compounds 1, 2, 3 and 4. Finally, four compounds with the amount of 137.0, 21.0, 18.0, and 1348.0 mg were obtained, respectively. The purity of each compound was 90.1%, 95.5%, 96.3% and 98.1%, as shown in Fig. S2.† And the yield of compounds 1, 2, 3 and 4 was 0.685%, 0.105%, 0.09% and 6.74%, respectively.
Compound 1. Vitisin A-13-O-β-D-glucoside. White amorphous powder, [[α]D +181° (c 0.1, MeOH)], possessed a molecular formula of C62H52O17 by the positive HRESI-MS (m/z 1091.3099 [M + Na]+, calcd 1091.3102), the 1H and 13C NMR data see Table 1.
Table 1 1H and 13C NMR data for compound 1 (CD3OD, δH: 3.30 ppm, δC: 49.0 ppm)
No. |
1H NMR |
13C NMR |
No. |
1H NMR |
13C NMR |
1a |
— |
133.8 (s) |
4c |
— |
158.8 (s) |
2a, 6a |
7.12 (2H, d, 8.6) |
128.2 (d) |
7c |
5.82 (1H, d, 11.4) |
89.1 (d) |
3a, 5a |
6.76 (2H, d, 8.6) |
116.3 (d) |
8c |
4.12 (1H, d, 11.4) |
49.7 (d) |
4a |
— |
158.5 (s) |
9c |
— |
142.5 (s) |
7a |
5.34 (1H, d, 6.5) |
94.8 (d) |
10c |
— |
121.1 (s) |
8a |
4.28 (1H, d, 6.5) |
58.1 (d) |
11c |
— |
158.5 (s) |
9a |
— |
146.8 (s) |
12c |
5.94 (1H, d, 2.3) |
101.0 (d) |
10a, 14a |
6.09 (2H, d, 2.2) |
107.4 (d) |
13c |
— |
156.8 (s) |
11a, 13a |
— |
160.0 (s) |
14c |
6.12 (1H, d, 2.3) |
104.8 (d) |
12a |
6.18 (1H, t, 2.2) |
102.3 (d) |
1d |
— |
136.0 (s) |
1b |
— |
129.1 (s) |
2d, 6d |
6.99 (2H, d, 8.7) |
129.2 (d) |
2b |
5.93 (1H, d, 2.0) |
133.0 (d) |
3d, 5d |
6.61 (2H, d, 8.7) |
115.5 (d) |
3b |
— |
132.9 (s) |
4d |
— |
155.9 (s) |
4b |
— |
155.7 (s) |
7d |
5.25 (1H, d, 3.8) |
41.1 (d) |
5b |
6.60 (1H, d, 8.3) |
115.5 (d) |
8d |
5.40 (1H, d, 3.8) |
41.8 (d) |
6b |
6.74 (1H, dd, 8.3, 2.0) |
123.6 (d) |
9d |
— |
141.6 (s) |
7b |
6.37 (1H, d, 16.2) |
132.1 (d) |
10d |
— |
121.0 (s) |
8b |
6.29 (1H, d, 16.2) |
122.0 (d) |
11d |
— |
160.4 (s) |
9b |
— |
137.3 (s) |
12d |
6.06 (1H, d, 2.1) |
96.1 (d) |
10b |
— |
122.2 (s) |
13d |
— |
158.8 (s) |
11b |
— |
162.4 (s) |
14d |
6.03 (1H, d, 2.1) |
110.4 (d) |
12b |
6.51 (1H, d, 2.0) |
98.3 (d) |
1′ |
4.89 (1H, d, 7.5) |
102.8 (d) |
13b |
— |
160.4 (s) |
2′ |
3.40–3.51 (m) |
75.0 (d) |
14b |
6.78 (1H, d, 2.0) |
106.4 (d) |
3′ |
3.40–3.51 (m) |
77.9 (d) |
1c |
— |
131.1 (s) |
4′ |
3.40–3.51 (m) |
71.2 (d) |
2c, 6c |
7.08 (2H, d, 8.6) |
130.3 (d) |
5′ |
3.40–3.51 (m) |
77.9 (d) |
3c, 5c |
6.70 (2H, d, 8.6) |
116.1 (d) |
6′ |
3.78 (1H, dd, 12.0, 4.1); 3.89 (1H, br d, 12.0) |
62.4 (t) |
Bioinformatic analysis
The database of DrugBank (https://www.drugbank.ca/), GeneCards (https://www.genecards.org/) and TTD (http://db.idrblab.net/ttd/) were used to construct inflammation-related target libraries by performing automated searches using keywords including “inflammation” and “inflammatory”. The molecular targets of all isolated compounds were predicted through PharmMapper (http://lilab.ecust.cn/pharmmapper/), which is a freely available web tool for identifying potential targets using a pharmacophore mapping method. The compound targets and inflammation-related targets were set as the background lists to get the overlapping targets using Venn diagrams (http://bioinformatics.psb.ugent.be/webtools/Venn/) web tool. Cytoscape 3.6.0 software was used to import the data to construct and visualize the compound-targets network.
Gene Ontology (GO) and Kyoto Encyclopedia of Genes and Genomes (KEGG) pathway enrichment analysis was performed based on the Metascape34 (https://metascape.org/gp/index.html#/main/step1). P < 0.05 was accepted to obtain main GO and KEGG pathways, and the first 20 pathways were screened out. Afterwards, the visualized diagrams of GO functional enrichment and KEGG pathway analysis were carried out through bioinformatics (http://www.bioinformatics.com.cn/login/) web tool.
Molecular docking
The molecular docking was used to analyze the interaction between all isolated compounds and COX-2. The analysis was conducted in Yinfo Cloud Platform (https://cloud.yinfotek.com/) and with the Autodock Vina program (version 1.1.2). The 3D structure of compounds 1, 2, 3 and 4 were constructed with energy minimization in MMFF94 force field. The crystal structures of COX-2 (PDB ID: 4M11)35 was obtained from RCSB Protein Data Bank (https://www.rcsb.org/). The crystal ligand was separated and used to define the binding pocket. AutoDock Vina program was utilized to perform semi-flexible docking with maximum 9 poses output after internal clustering.36
Anti-inflammatory assays
The RAW 264.7 murine macrophage cell line (TCM13, National Collection of Authenticated Cell Cultures, Shanghai, China) was cultured in DMEM (Dulbecco's modified Eagle medium) supplemented with 10% fetal bovine serum, antibiotics (100 U mL−1 penicillin and 100 U mL−1 streptomycin), and the cells were maintained at 37 °C in a humidified incubator containing 5% CO2.
The RAW 264.7 cells were seeded in 96-well plates (1 × 105/mL) for 24 h. The effects of different concentrations of compounds (0, 1, 5, 10, 20, 50 and 100 μM) on cell viability were measured by the MTT assay.37 Then, the RAW 264.7 cells were divided into three groups containing the control group, the model group (5 μg mL−1 LPS),38 and the sample group (5 μg mL−1 LPS and the compounds at concentrations of 10 μM) for 24 h. The NO concentration in the culture medium was measured by the Griess reagent.39 The IL-6 level in the culture medium was measured by an ELISA kit.40 The expression level of COX-2 was measured by western blot. Antibodies against COX-2 (#12282) and β-actin (#4970) were obtained from Cell Signaling Technology (Danvers, MA, USA) and the assays were conducted as previously reported.41
Statistical analysis
Data analyses were performed with GraphPad Prism 7.0 (GraphPad Software, San Diego, CA). Three or more replicates were performed for each experiment. One-way ANOVA was used for comparisons between three or more groups, whereas Student's t-test was used for comparisons between two groups. Results were presented as mean ± SD. P value less than 0.05 was considered significant.
Results and discussion
Preparation of oligostilbene extracts
Oligostilbenes in Iris lactea were extracted under alkaline conditions followed by precipitation with acid. Selection of the extraction method was based on the trade-off between cost and efficiency. In the published studies, extraction with organic solvents was preferred. However, utilization of multiple organic solvents (such as ethanol, methanol or acetone) and successive partitioning increases the costs. On the other hand, alkaline-based extraction followed by acid-induced precipitation has been widely used in extraction of natural products such as lignins, hemicelluloses and phenolic compounds.42–45 Lv et al. isolated Vitisin D, Ampelopsin B and cis-Vitisin A from Iris lactea by adopting the alkaline-acid method and verified the extracts with high-speed counter current chromatography method.25 The alkaline-acid method is of many advantages: environmental protection, easy operation, cost saving and low solvent contamination. Oligostilbenes contain hydroxyl groups and exhibit weak acidity. After interaction with the alkali, the water solubility of the oligostilbenes increase. After the addition of acid, the dissolved substances in the alkali solution could be precipitated. It should be noted that determination of the specific pH value for the precipitation of oligostilbenes is of special importance. After series of pilot tests, we found pH 3.0 could induce the proximal precipitation.
In general, extraction method of plant material applies the organic solvent rather than use the alkaline-acid method, which might have destroyed some of the key compounds of plant material. However, according to the above comparison combine with structural characteristics of oligostilbenes. We found that the alkaline-acid method is more advantageous. So, we applied the alkaline-acid method to obtain oligostilbenes extracts.
Identification of new compounds
Compound 1, obtained as white amorphous powder [[α]D +181° (c 0.1, MeOH)], with a molecular formula of C62H52O17 by the positive HRESI-MS (m/z 1091.3099 [M + Na]+, calcd 1091.3102), requiring 37 indices of hydrogen deficiency. The 1H NMR spectrum (Table 1) of methanol-d4 of compound 1 showed signals for three pairs of p-substituted benzene ring proton, three pairs of 1,3,4,5-tetrasubstituted benzene ring signals, a set of 1,2,4-trisubstituted benzene ring, a set of symmetrical 1,3,5-trisubstituted benzene, and a pair of trans-form olefinic protons at δH 6.29, 6.37 (each 1H, d, J = 16.2 Hz). In addition, three pairs of coupling aliphatic doublets were detected at δH 4.28, 5.34 (each 1H, d, J = 6.5 Hz), 4.12, 5.82 (each 1H, d, J = 11.4 Hz), and 5.25, 5.40 (each 1H, d, J = 3.8 Hz), as well as characteristic signals for β-glucopyranosyl at δH 4.89 (1H, d, J = 7.5 Hz, H-1′), 3.78 (1H, dd, J = 12.0, 4.1 Hz, H-6′a), 3.89 (1H, br d, J = 12.0 Hz, H-6′b), and 3.40–3.51 (4H, m, H-2′, H-3′, H-4′ and H-5′). The 13C NMR spectrum (Table 1) showed a total of 62 carbon resonances, including 50 downfield carbon signals (partially overlapping) assignable to eight benzene rings and a double-bond group, six aliphatic methine carbons (two oxygenated), as well as characteristic signals for β-glucopyranosyl at δC 102.8 (d, C-1′), 77.9 (d, C-3′ and C-5′), 75.0 (d, C-2′), 71.2 (d, C-4′) and 62.4 (t, C-6′). The above NMR features were very similar to those of Vitisin A,46 a stilbene tetramer isolated from the genus Vitis. The difference between the two was that the signals of β-glucopyranosyl moiety were only present in compound 1, suggesting it a glucoside derivative of Vitisin A. The obvious downfield shifts (Δ ≈ +0.35 ppm) of H-12b and H-14b in compound 1, compared with those of Vitisin A, indicates the localization of the sugar fragment at C-13b. Moreover, the HMBC correlations (Fig. 2a) between the H-1′ [δH 4.89 (1H, d, J = 7.5 Hz, H-1′)], H-12b [δH 6.51 (1H, d, J = 2.0 Hz)] and H-14b [δH 6.78 (1H, d, J = 2.0 Hz)] to C-13b [δC 160.4 (s)], further confirmed the glycosylation at C-13b. The sugar was identified as D-glucose by acid hydrolysis. Moreover, based on the coupling constant (7.5 Hz) of the anomeric proton, the sugar moiety was identified as β-configuration.47,48 In view of the roughly consistent NMR data (especially the coupling constants) and the NOESY correlations (Fig. 2b), the aglycone part of compound 1 was deduced to have the same stereochemistry as that of Vitisin A. Therefore, the structure of compound 1 was established as Vitisin A-13-O-β-D-glucoside (Fig. 1). The complete assignments of the 1H and 13C NMR spectroscopic data were summarized in Table 1 based on the extensive 2D NMR experiments. By comparing their NMR data with those reported in literature, structures of the other three compounds in the extracts were identified as Vitisin D (compound 2),49 hopeaphenol (compound 3)50,51 and Vitisin A (compound 4),46 respectively (Fig. 1).
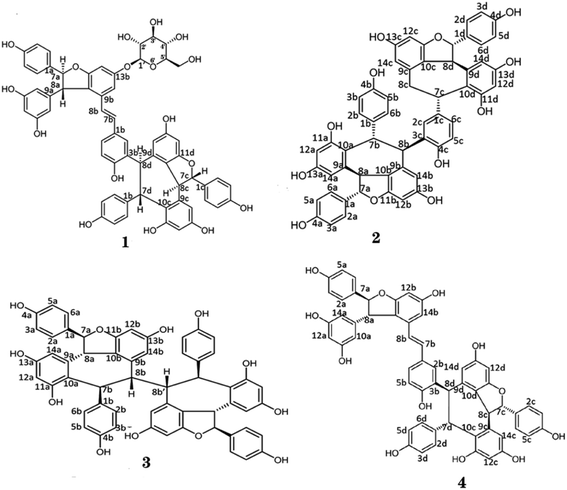 |
| Fig. 1 Chemical structures of isolated oligostilbenes from seeds of Iris lactea. | |
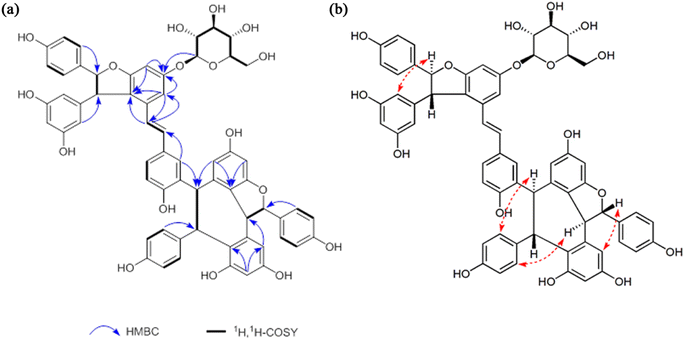 |
| Fig. 2 The correlations of compound 1. (a) The HMBC and 1H,1H-COSY correlations. (b) The NOESY correlations. | |
Naturally occurring oligostilbenes are hydroxylated stilbenes with complex structures and various biological activities. Oligostilbenes are mainly found in Vitaceae, Gnetaceae, Dipterocarpaceae, Leguminosae, Cyperaceae, Paeoniaceae, and Iridaceae.52–58 Hou et al., isolated trans-ε-viniferin and 2-r-viniferin from the seeds of Iris lactea.59 Lv et al., separated multiple oligostilbenes, including Vitisin B and Vitisin C, from the seed kernel of Iris lactea.57 In this study, we modified the alkaline-acid extraction method and isolated four oligostilbenes from the seeds of Iris lactea, including Vitisin A-13-O-β-D-glucoside, Vitisin D, hopeaphenol and Vitisin A.
Based on the above results, we found that the purity of compound 1 was 90.1%. To determine the impurity of compound 1, we analyzed the compound 1 with UPLC-TOF-MS. According to the retention time in UPLC-UV chromatogram and the masses of parent ions [M − H]+ and their fragment ions, we concluded the impurity is vaticaside B (Fig. S3†). The vaticaside B is glucosides of resveratrol oligomers. Compound 1 and compound 4 as resveratrol tetramer, a class of stilbenoids, are commonly biosynthesized by regioselective oxidative coupling of four units of resveratrol monomer.60 It has been reported that compound 4 more stable against degradation than native resveratrol dimer or monomer.61 In addition, there was use a rapid liquid chromatography-ultraviolet detection method for the assessment of stability of resveratrol and resveratrol tetramer hopeaphenol. The results indicated that the half-life of resveratrol is 1 h. However, the tetramer hopeaphenol was stable over the maximum incubation time of 72 h.62 Thus, we think resveratrol tetramer compound 1 is also stable. So, this impurity may be introduced due to unclean of separation process.
Bioinformatic analysis
After removing the repeated targets, a total of 1365 inflammation-related targets were integrated and identified through the DrugBank, GeneCards and TTD databases. 436 possible targets of 4 compounds were attained by using PharmMapper to screen and remove repetition. The Fig. 3a shows the Venn diagram of the compound and inflammation targets, such that the common part of the two was regarded as to include potential anti-inflammatory targets. There were 138 overlapping targets between the compound targets and inflammation-related targets. The correlations between the compound targets and overlapping targets were constructed and visualized by Cytoscape 3.6.0 software (Fig. 3b and Table S1†). The results show that the 10 targets with a higher degree value among the anti-inflammatory targets are BMP2 (P12643, degree = 4), CA2 (P00918, degree = 4), TTR (P02766, degree = 4), ALB (P02768, degree = 4), CASP7 (P55210, degree = 4), BCHE (P06276, degree = 4), MAPK1 (P28482, degree = 4), PIK3CG (P48736, degree = 4), GC (P02774, degree = 4) and MMP13 (P45452, degree = 4), which play a major role in the network.
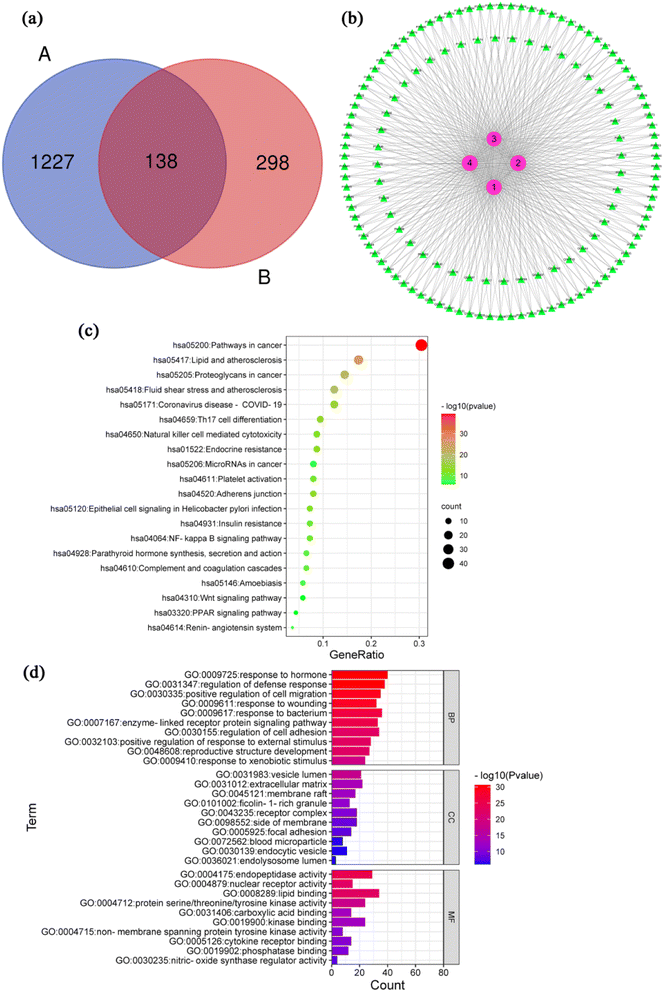 |
| Fig. 3 Bioinformatic analysis and molecular docking. (a) Venn diagram containing the inflammation-related targets (A) and compounds-related tergets (B). (b) Compound-target network diagram. (c) Top 20 components of the KEGG pathway analysis. (d) Top 10 components of the GO enrichment analysis. | |
The inflammatory process can trigger different diseases depending on the specific inflamed tissue or organ involved. However, all disorders have common features or a conjoint cellular process, such as the activation of a stress signaling pathway and the concomitant production of inflammatory cytokines.63 A total of 138 inflammation-related targets were input to the Metascape database, and 169 pathways that had statistical significance were input for pathway enrichment analysis. According to the results with significance (P < 0.05), enrichment analysis yielded 169 pathways and visualization analysis of the top 20 signaling pathways (Fig. 3c), including pathways in cancer, lipid and atherosclerosis, Th17 cell differentiation, NF-κB signaling pathway and coronavirus disease-COVID 19. Detailed information of KEGG pathway enrichment analysis was provided in ESI Table S2.† GO function of 138 action targets (P < 0.05) was enriched with Metascape (Table S3†). Top 10 terms are shown in Fig. 3d. The results showed that the main biological process were involved in response to hormone, regulation of defense response, positive regulation of cell migration, response to wounding and response to bacterium. The main cellular component was involved in vesicle lumen, extracellular matrix, membrane raft, receptor complex and side of membrane. The main molecular function were involved in endopeptidase activity, nuclear receptor activity, lipid binging, protein serine/threonine/tyrosine kinase activity and nitric-oxide synthase regulator activity.
NF-κB is the most ubiquitous transcription factor, and NF-κB signaling plays an important role in the expression of genes related to inflammatory response, including those encoding iNOS, COX-2 and pro-inflammatory cytokines.64 We use KEGG and GO pathway enrichment analyses and existing literature reports, the NF-κB-dependent signaling pathway was tentatively identified as the mechanism by which compounds 1, 2, 3 and 4 exerts its anti-inflammatory effects.
Molecular docking analysis
To investigate the possible mechanism anti-inflammatory activities, molecular docking studies (Autodock Vina program (version 1.1.2)) were conducted to investigate the interactions between the compounds and COX-2 protein. As far, the latest version of Autodock Vina program is v. 1.2.0. However, the Autodock Vina program (version 1.1.2) was used for the molecular docking studies. Compared to Autodock Vina program (version 1.1.2), Autodock Vina program (version 1.2.0) support for modeling specific features such as macrocycles or explicit water molecules. Additionally, Autodock Vina program (version 1.2.0) also support the AutoDock4.2 scoring function, simultaneous docking of multiple ligands, and a batch mode for docking a large number of ligands. Furthermore, it implemented Python bindings to facilitate scripting and the development of docking workflows.65 In general, the version updates have no effect on results of molecular docking. So, we think the Autodock Vina program (version 1.1.2) is still applicable to analyze molecular docking. The results revealed that compounds 1, 2, 3 and 4 showed strong affinities with COX-2 protein (Fig. 4). And the estimated binding free energy of compounds 1, 2, 3 and 4 for its binding with COX-2 was −10.9, −11.3, −8.2 and −9.4 kcal mol−1, respectively. For the interaction between compound 1 and COX-2, hydrogen bonds form at residues Gly135, Gln327, Lys317 and Asn34, and hydrophobic interaction occurs at residues His133, Tyr134, Glu326 and Tyr136. For the interaction between compound 2 and COX-2, hydrogen bonds form at residues Asp239 and Arg311, and hydrophobic interaction occurs at residues Lys243, Lys557, Val554, Phe247, Thr561 and His242, and π–π stacking at residues Phe247. For the interaction between compound 3 and COX-2, hydrogen bonds form at residues Asp158 and Tyr136, and hydrophobic interaction occurs at residues Pro154, Ala156, Asp157, Asp325 and Glu326. For the interaction between compound 4 and COX-2, hydrogen bonds form at residues Ser110, Arg109, Asp362 and Ser563, and hydrophobic interaction occurs at residues Pro106, Asp362, Asn560, Thr561, Lys243 and Gln270.
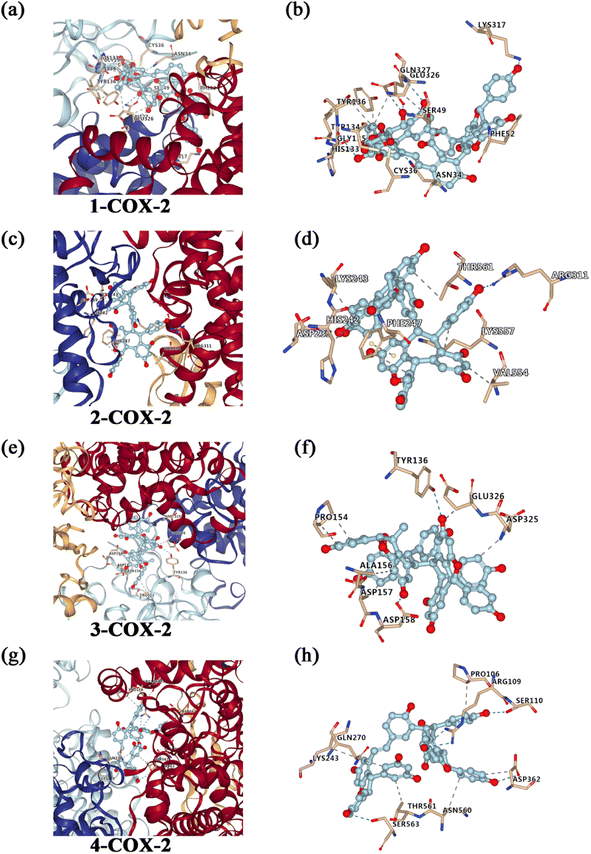 |
| Fig. 4 Molecular docking to predict the binding of compounds 1, 2, 3 and 4 to COX-2. (a) General overview of compound 1 docking into COX-2. (b) Demonstration of the predicted binding conformation and corresponding interaction amino acid residues in (a). (c) General overview of compound 2 docking into COX-2. (d) Demonstration of the predicted binding conformation and corresponding interaction amino acid residues in (c). (e) General overview of compound 3 docking into COX-2. (f) Demonstration of the predicted binding conformation and corresponding interaction amino acid residues in (e). (g) General overview of compound 4 docking into COX-2. (h) Demonstration of the predicted binding conformation and corresponding interaction amino acid residues in (g). | |
Anti-inflammatory effects
The MTT assay was preliminarily used to determine the cytotoxicity of isolated compounds in RAW 264.7 cells. As shown in Fig. 5a–d, compounds 1, 2, 3 and 4 (1–100 μM) had no cytotoxic effect on RAW 264.7 cell viability, compared to the control group. So, compounds 1, 2, 3 and 4 of 10 μM were used to investigate the potential anti-inflammatory activities of these compounds in LPS-stimulated RAW 264.7 cells. Sung et al. found that compound 4 at concentration of 1–10 μM had no cytotoxic effect on RAW 264.7 cells.66 Xu et al. suggested that compound 3 at concentration of 10 μM didn't exhibited cytotoxic effect on hCMEC/D3 cells.67 In addition, the effects of compounds 1, 2, 3 and 4 at concentration of 1–100 μM on cell toxicity were tested in PC12, SH-SY5Y, and HepG2 cell lines with MTT assay (Fig. S4†). The cell viability was increased after incubation with compounds 1 and 2 individually for 24 h at final concentrations of 0–50 μM. However, it should also be noted that compound 1 and compound 2 at 100 μM reduced the viability of HepG2 and PC12 cells, respectively. We found compound 3 at 1–100 μM increased the viability of the three cell lines after a 24 h treatment. In contrast, our results showed 24 h incubation with compound 4 at 0–20 μM increased the viability of PC12 and SH-SY5Y cells although a reduction of viability were observed when the dosage increased to 50 and 100 μM. In a word, compounds 1, 2, 3 and 4 at concentration of 1–10 μM had no caused the reduction of cell viability.
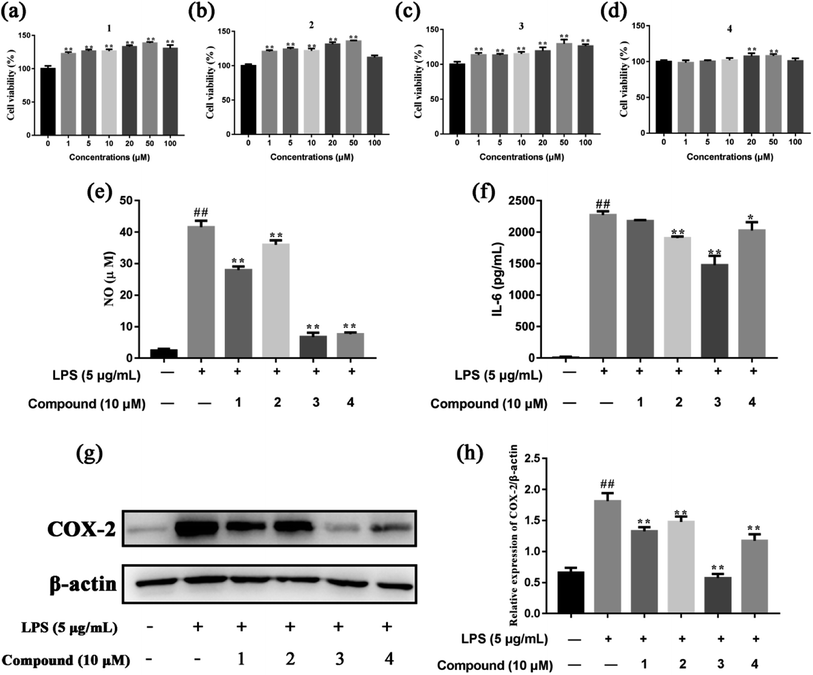 |
| Fig. 5 The anti-inflammatory effects of compounds 1, 2, 3 and 4 in LPS-stimulated RAW 264.7 cells. (a)–(d) The cell viability of compounds 1, 2, 3 and 4, respectively. (e) The NO production. (f) The level of IL-6. (g) and (h) The protein expression of COX-2. Data were expressed as mean ± SD of three independent experiments. #P < 0.05 and ##P < 0.01 compared to the control group. *P < 0.05 and **P < 0.01 compared to the LPS-stimulated group. | |
NO is known to be a potent pro-inflammatory mediator and possesses a dual regulatory function in the inflammatory reaction.68 NO plays anti-inflammatory roles under normal physiological conditions, however, excessive production of NO has been found associated with various inflammatory disorders.69 Therefore, we were investigated whether the four compounds affected NO production in LPS-stimulated RAW 264.7 cells. As shown in Fig. 5e, compounds 1, 2, 3 and 4 significant suppressed NO production in LPS-stimulated RAW 264.7 cells, compared to the model group. In addition, the effect of compounds 1, 2, 3 and 4 on the levels of pro-inflammatory cytokine IL-6 was evaluated. The results showed that compounds 2, 3 and 4 inhibited the release of IL-6 in the cell supernatant, compared to the model group (Fig. 5f). COX-2 is expressed upon induction by various factors, such as IL-1β, IL-6, TNFα and growth factors, in inflammation, pain response, or tissue damage.70 Compared to the model group, compounds 1, 2, 3 and 4 had the strongest inhibitory effect on the expression of COX-2 in LPS-stimulated RAW 264.7 cells (Fig. 5g and h). Compound 4 suppresses LPS-induced NO production in LPS-stimulated RAW 264.7 cells. The results are in agreement with other related researches.66
In this study, the main targets of the anti-inflammatory effects of compounds 1, 2, 3 and 4 and the related signaling pathways were initially predicted by network pharmacology. However, network pharmacology research is based on network modeling, database resource development and software application.71 Thus, we further investigated the anti-inflammatory effects of compounds 1, 2, 3 and 4 in LPS-stimulated RAW 264.7 cell. The results show compounds 1, 2, 3 and 4 inhibited the production of NO and IL-6, and the expression of COX-2. Taken together, our results exhibit compounds 1, 2, 3 and 4 have potential anti-inflammatory activity. However, further investigation is still needed as there are some limitations in our study. The main limitation of our study is that we didn't examine the anti-inflammatory role of compounds 1, 2, 3 and 4 in vivo. Therefore, further study should be performed to verify the role of compounds 1, 2, 3 and 4 in inflammatory animal models.
There were reported that stilbene extract with 99% purity (ST-99 extract) didn't exhibit genotoxic potential by Ames test or comet assay.72 Pharmacological activities of trans-resveratrol are well known through its antioxidant, antimutagenic,73 and anticarcinogenic.74 And resveratrol exhibits various biological effects such as antimutagenic and anticarcinogenic properties.75 However, compounds 1, 2, 3 and 4 as resveratrol tetramer, these compounds may have antimutagenic and anticarcinogenic activities like resveratrol or trans-resveratrol.
3′-Hydroxypterostilbene (3′-HPT) is a naturally occurring stilbenoid molecule present in the plants Sphaerophysa salsula. 90 day repeated oral dose and reproductive (developmental) toxicity of 3′-HPT as a test substance in rats were performed. Results showed no significant differences in haematology, reproductive and developmental parameters in the control/treatment groups. The findings suggested a no observed adverse effect level of 3′-HPT in rats after oral administration.76 Resveratrol protects against the cardiac developmental toxicity of trichloroethylene by inhibiting oxidative stress and cell proliferation.77 However, 100 and 200 μM resveratrol treatments resulted in a significant decrease in the number of offspring of Drosophila melanogaster. The results reveal that resveratrol may have negative effects on the development and reproduction of invertebrates.78 Based on the related studies, it is found that resveratrol on the development and reproduction has positive and negative effects. Compounds 1, 2, 3 and 4 as resveratrol tetramer, these compounds may have different effects on development and reproduction. However, the specific mechanism of action needs further explore.
Resveratrol attenuates methylmercury-induced neurotoxicity by modulating synaptic homeostasis.79 And resveratrol suppresses rotenone-induced neurotoxicity through activation of SIRT1/Akt1 signaling pathway.80 Compound 4 from Vitis amurensis against sodium nitroprusside-induced neurotoxicity in human neuroblastoma SH-SY5Y cells.81 However, compounds 2 and 4 as isomer, we predict that the compound 2 also have neuroprotective effects.
Oligostilbenes are resveratrol derivatives, one of the stilbenoids produced by plants as a defense mechanism in response to microbial attack, poisons, diseases, or UV-radiation. Overall, oligostilbenes are useful in medical technology and in the pharmaceutical, agricultural, nutraceutical and cosmeceutical sectors.
Conclusions
In summary, phytochemical investigation of Iris lactea resulted in the isolation of four oligostilbenes. Among them, compound 1 was a new oligostilbene from Iris lactea and compound 3 was isolated from Iris lactea for the first time. Network pharmacology and anti-inflammatory assays showed that compounds 1, 2, 3 and 4 possessed inhibitory effect on associated inflammatory factors. Therefore, these oligostilbenes may be a candidate for the prevention and treatment of inflammatory disease. In addition, based on its advantage of abundant resources, good biological activity and environmental friendliness, Iris lactea will become a promising plant resource.
Author contributions
Fang-Fang Tie performed the experiments and wrote the preliminary manuscript. Yang-Yang Fu and Na Hu provide significant guidance in the biological experimental procedure. Zhi Chen modified the syntax. Hong-Lun Wang performed conceptualization and reviewed the final manuscript.
Conflicts of interest
The authors declare no conflict of interest.
Acknowledgements
This study was supported by the Qinghai Province Applied Basic Research Project (2020-ZJ-905).
References
- C. S. Gu, L. Q. Liu, Y. M. Deng, X. D. Zhu, S. Z. Huang and X. Q. Lu, Bull. Environ. Contam. Toxicol., 2015, 94, 247–253 CrossRef CAS PubMed.
- J. Tang, Q. Liu, H. Yuan, Y. Zhang, W. Wang and S. Huang, Genes Genomics, 2018, 40, 893–903 CrossRef CAS PubMed.
- C. S. Gu, S. Xu, Z. Q. Wang, L. Q. Liu, Y. X. Zhang, Y. M. Deng and S. Z. Huang, Plant Physiol. Biochem., 2018, 125, 1–12 CrossRef CAS PubMed.
- C. S. Gu, L. Q. Liu, Y. M. Deng, Y. X. Zhang, Z. Q. Wang, H. Y. Yuan and S. Z. Huang, Ecotoxicol. Environ. Saf., 2017, 144, 507–513 CrossRef CAS PubMed.
- X. X. Tian, P. C. Mao, Q. Guo and L. Meng, Southwest China Journal of Agricultural Sciences, 2019, 32, 2090–2096 Search PubMed.
- Q. Q. Liu, Y. X. Zhang, Y. J. Wang, W. L. Wang, C. S. Gu, S. Z. Huang and H. Yuan, J. Hazard. Mater., 2020, 400, 123165 CrossRef CAS PubMed.
- H. W. Kim, S. S. Kim, K. B. Kang, B. Ryu, E. Park, J. Huh, W. K. Jeon, H. S. Chae, W. K. Oh, J. Kim, S. H. Sung and Y. W. Chin, Molecules, 2020, 25, 1–12 Search PubMed.
- Y. Meng, G. Y. Xie, L. Shi and M. J. Qin, Chinese Wild Plant Resources, 2017, 36, 42–49 Search PubMed.
- Y. Wang, L. Wang, M. Yao, B. Zhao, Y. Xiong, J. J. Dou and S. F. Ni, Ningxia Journal of Agriculture and Forestry Science and Technology, 2012, 53, 69–70 Search PubMed.
- B. Lin, G. Wang, Q. Wang, C. Ge and M. Qin, Fitoterapia, 2011, 82, 1137–1139 CrossRef CAS PubMed.
- A. U. Rahman, M. I. Choudhary, M. N. Alam, P. O. Ndognii, T. Badarchiin and G. Purevsuren, Chem. Pharm. Bull., 2000, 48, 738–739 CrossRef CAS PubMed.
- K. Seki, K. Haga and R. Kaneko, Phenols and a dioxotetrahydrodibenzofuran from seeds of Iris pallasii, Phytochemistry, 1995, 38, 965–973 CrossRef CAS.
- Y. Gao, C. He, R. Ran, D. Zhang, D. Li, P. G. Xiao and E. Altman, J. Ethnopharmacol., 2015, 169, 24–33 CrossRef CAS PubMed.
- C. S. Yao and M. Lin, Nat. Prod. Res., 2005, 19, 443–448 CrossRef CAS PubMed.
- H. M. Ge, B. Huang, S. H. Tan, D. H. Shi, Y. C. Song and R. X. Tan, J. Nat. Prod., 2006, 69, 1800–1802 CrossRef CAS PubMed.
- D. T. Ha, H. Kim, P. T. Thuong, T. M. Ngoc, I. Lee, N. D. Hung and K. Bae, J. Ethnopharmacol., 2009, 125, 304–309 CrossRef CAS PubMed.
- H. Matsuda, Y. Asao, S. Nakamura, M. Hamao, S. Sugimoto, M. Hongo, Y. Pongpiriyadacha and M. Yoshikawa, Chem. Pharm. Bull., 2009, 57, 487–494 CrossRef CAS PubMed.
- K. T. Wang, L. G. Chen, S. H. Tseng, J. S. Huang, M. S. Hsieh and C. C. Wang, J. Agric. Food Chem., 2011, 59, 3649–3656 CrossRef CAS PubMed.
- A. Wibowo, N. Ahmat, A. S. Hamzah, A. S. Sufian, N. H. Ismail, R. Ahmad, F. M. Jaafar and H. Takayama, Fitoterapia, 2011, 82, 676–681 CrossRef CAS PubMed.
- G. X. Yang, J. T. Zhou, Y. Z. Li and C. Q. Hu, Planta Med., 2005, 71, 569–571 CrossRef CAS PubMed.
- N. K. Zawawi, N. Ahmat, M. Z. Mazatulikhma, R. M. Shafiq, N. H. Wahid and A. S. Sufian, Nat. Prod. Res., 2013, 27, 1589–1593 CrossRef CAS PubMed.
- N. Zga, Y. Papastamoulis, A. Toribio, T. Richard, J. C. Delaunay, P. Jeandet, J. H. Renault, J. P. Monti, J. M. Mérillon and P. Waffo-Téguo, J. Chromatogr. B: Anal. Technol. Biomed. Life Sci., 2009, 877, 1000–1004 CrossRef CAS PubMed.
- F. F. Tie, H. L. Wang, N. Hu and Q. Dong, CN Pat., 2020, 202210656255.8 Search PubMed.
- H. L. Wang, F. F. Tie and G. X. Luan, CN Pat., 109662962B, 2019 Search PubMed.
- H. Lv, W. Zhou, X. Wang, Z. Wang, Y. Suo and H. Wang, J. Chromatogr. Sci., 2016, 54, 744–751 CAS.
- N. Ren, E. Kim, B. Li, H. Pan, T. Tong, C. S. Yang and Y. Tu, J. Agric. Food Chem., 2019, 67, 5361–5373 CrossRef CAS PubMed.
- P. Libby, Nutr. Rev., 2007, 65, 140–146 CrossRef.
- R. Gautam and S. M. Jachak, Med. Res. Rev., 2009, 29, 767–820 CrossRef CAS PubMed.
- K. T. Wang, L. G. Chen, S. H. Tseng, J. S. Huang, M. S. Hsieh and C. C. Wang, J. Agric. Food Chem., 2011, 59, 3649–3656 CrossRef CAS PubMed.
- E. Y. Chung, B. H. Kim, M. K. Lee, Y. P. Yun, S. H. Lee, K. R. Min and Y. Kim, Planta Med., 2003, 69, 710–714 CrossRef CAS PubMed.
- J. Xue, Y. Shi, C. Li and H. Song, J. Cell. Biochem., 2019, 120, 6431–6440 CrossRef CAS PubMed.
- Q. Ge, L. Chen, M. Tang, S. Zhang, L. Liu, L. Gao, S. Ma, M. Kong, Q. Yao, F. Feng and K. Chen, Eur. J. Pharmacol., 2018, 833, 50–62 CrossRef CAS PubMed.
- A. S. Pereira, M. J. Bester and Z. Apostolides, Mol. Diversity, 2017, 21, 809–820 CrossRef CAS PubMed.
- Y. Zhou, B. Zhou, L. Pache, M. Chang, A. H. Khodabakhshi, O. Tanaseichuk, C. Benner and S. K. Chanda, Nat. Commun., 2019, 10, 1523 CrossRef PubMed.
- J. Maniewska, B. Wiatrak, Ż. Czyżnikowska and B. M. Szczęśniak-Sięga, Int. J. Mol. Sci., 2021, 22, 7818 CrossRef CAS PubMed.
- O. Trott and A. J. Olson, J. Comput. Chem., 2010, 31, 455–461 CAS.
- M. Zhang, H. Ren, K. Li, S. Xie, R. Zhang, L. Zhang, J. Xia, X. Chen, X. Li and J. Wang, BMC Complementary Med. Ther., 2021, 21, 149 CrossRef CAS PubMed.
- X. Liu, S. Quan, Q. Han, J. Li, X. Gao, J. Zhang and D. Liu, J. Ethnopharmacol., 2022, 282, 114626 CrossRef CAS PubMed.
- Y. Han, X. Li, X. Zhang, Y. Gao, R. Qi, R. Cai and Y. Qi, Int. Immunopharmacol., 2020, 84, 106528 CrossRef CAS PubMed.
- L. Chen and Z. Liu, Mol. Med. Rep., 2019, 20, 5345–5352 CAS.
- X. N. Li, J. L. Huang, X. D. Jiang, W. C. Yu, Y. M. Zheng, Q. B. Li and N. He, Sep. Purif. Technol., 2014, 124, 201–206 CrossRef CAS.
- S. L. Sun, J. L. Wen, M. G. Ma and R. C. Sun, Carbohydr. Polym., 2013, 92, 2224–2231 CrossRef CAS PubMed.
- G. H. Wang and H. Z. Chen, Sep. Purif. Technol., 2013, 105, 98–105 CrossRef CAS.
- X. H. Xiong, L. P. Zhao, Y. M. Chen, Q. J. Ruan, C. M. Zhang and Y. F. Hua, Food Bioprod. Process., 2015, 94, 239–247 CrossRef CAS.
- Y. Fang, L. Yang and J. He, Biomed. Pharmacother., 2021, 143, 112104 CrossRef CAS PubMed.
- J. Ito, K. Gobaru, T. Shimamura and M. Niwa, Absolute configurations of some oligostilbenes from Vitis coignetiae and Vitis vinifera ‘Kyohou’, Tetrahedron, 1998, 34, 1–13 Search PubMed.
- W. W. Zhao, W. W. Guo, J. F. Guo, X. Wang, X. Q. Chen and X. Wu, Nat. Prod. Res., 2021, 35, 49–56 CrossRef CAS PubMed.
- H. Wen, Y. Zang, Q. Zhu, S. Ouyang, J. Luo, N. Luo, H. Zhu and Y. Zhang, Nat. Prod. Res., 2022, 36, 3255–3261 CrossRef CAS PubMed.
- Y. Ohshima, K. Shinoda, Y. Takaya, T. Ohta, M. Niwa, K. Hisamichi and M. Takeshita, Heterocycles, 1997, 46, 169 CrossRef.
- H. A. Guebailia, K. Chira, T. Richard, T. Mabrouk, A. Furiga, X. Vitrac, J. P. Monti, J. C. Delaunay and J. M. Mérillon, J. Agric. Food Chem., 2006, 54, 9559–9564 CrossRef CAS PubMed.
- J. Kawabata, E. Fukushi, M. Hara and J. Mizutani, Magn. Reson. Chem., 1992, 30, 6–10 CrossRef CAS.
- I. Bayach, N. Manshoor, J. C. Sancho-García, M. I. Choudhary and J. F. F. Weber, Chem.–Asian J., 2015, 10, 198–211 CrossRef CAS PubMed.
- W. F. Chiou, C. C. Shen, C. C. Chen, C. H. Lin and Y. L. Huang, Planta Med., 2009, 75, 856–859 CrossRef CAS PubMed.
- C. N. He, Y. Peng, L. J. Xu, Z. A. Liu, J. Gu, A. G. Zhong and P. G. Xiao, Chem. Pharm. Bull., 2010, 58, 843–847 CrossRef CAS PubMed.
- T. Ito, H. Endo, H. Shinohara, M. Oyama, Y. Akao and M. Iinuma, Fitoterapia, 2012, 83, 1420–1429 CrossRef CAS PubMed.
- W. Jeong, E. K. Ahn, J. S. Oh and S. S. Hong, J. Asian Nat. Prod. Res., 2017, 19, 1143–1147 CrossRef CAS PubMed.
- H. Lv, H. Wang, Y. He, C. Ding, X. Wang and Y. Suo, J. Chromatogr. B: Anal. Technol. Biomed. Life Sci., 2015, 988, 127–134 CrossRef CAS PubMed.
- Y. Shimokawa, Y. Hirasawa, T. Kaneda, A. H. A. Hadi and H. Morita, Chem. Pharm. Bull., 2012, 60, 790–792 CrossRef CAS PubMed.
- W. Hou, Identification and study on the activity of natural compounds from the seeds of Iris lactea Pall. Var. chinensis (Fisch.) Koidz, Master's thesis, Liaoning Normal University, 2012.
- M. Lin and C. S. Yao, Stud. Nat. Prod. Chem., 2006, 33, 601–644 CAS.
- J. Shen, Q. Zhou, P. Li, Z. Q. Wang, S. S. Liu, C. N. He, C. H. Zhang and P. G. Xiao, Molecules, 2017, 24, 2050 CrossRef PubMed.
- I. Willenberg, W. Brauer, M. T. Empl and N. H. Schebb, J. Agric. Food Chem., 2012, 15, 7844–7850 CrossRef PubMed.
- G. Haegeman, Eur. Respir. J., 2003, 44, 16–19 CrossRef PubMed.
- D. Capece, D. Verzella, I. Flati, P. Arboretto, J. Cornice and G. Franzoso, Trends Immunol., 2022, 43, 757–775 CrossRef CAS PubMed.
- J. Eberhardt, D. Santos-Martins, A. F. Tillack and S. Forli, J. Chem. Inf. Model., 2021, 61, 3891–3898 CrossRef CAS PubMed.
- M. J. Sung, M. Davaatseren, W. Kim, S. K. Park, S. H. Kim, H. J. Hur, M. S. Kim, Y. S. Kim and D. Y. Kwon, Int. Immunopharmacol., 2009, 9, 319–323 CrossRef PubMed.
- L. Xu, Z. Q. Yu, Y. Uekusa, S. Kawaguchi, H. Kikuchi, K. Daitoku, M. Minakawa, S. Motomura, K. I. Furukawa, Y. Oshima, K. Seya and T. Imaizumi, J. Pharmacol. Sci., 2022, 149, 147–157 CrossRef CAS PubMed.
- S. R. Lee, S. Lee, E. Moon, H. J. Park, H. B. Park and K. H. Kim, Bioorg. Chem., 2017, 70, 94–99 CrossRef CAS PubMed.
- Y. L. Tang, X. Zheng, Y. Qi, X. J. Pu, B. Liu, X. Zhang, X. S. Li, W. L. Xiao, C. P. Wan and Z. W. Mao, Bioorg. Chem., 2020, 98, 103748 CrossRef CAS PubMed.
- V. B. Grace, D. D. Wilson, R. Anushya and S. Siddikuzzaman, Life Sci., 2021, 285, 119967 CrossRef CAS PubMed.
- Z. Z. Yuan, Y. Y. Pan, T. Leng, Y. Chu, H. J. Zhang, J. R. Ma and X. J. Ma, J. Pharm. Pharm. Sci., 2022, 25, 218–226 Search PubMed.
- C. Medrano-Padial, A. I. Prieto, M. Puerto and S. Pichardo, Food Chem. Toxicol., 2021, 150, 112065 CrossRef CAS PubMed.
- U. Subbiah and M. Raghunathan, J. Biomol. Struct. Dyn., 2008, 25, 425–434 CrossRef CAS PubMed.
- M. Athar, J. H. Back, L. Kopelovich, D. R. Bickers and A. L. Kim, Arch. Biochem. Biophys., 2009, 486, 95–102 CrossRef CAS PubMed.
- S. Pervaiz and A. L. Holme, Antioxid. Redox Signaling, 2009, 11, 2851–2897 CrossRef CAS PubMed.
- M. Muhammed, B. Sarang, N. Sankaran, P. Anjali and S. Naveed, PLoS One, 2017, 3, e0172770 Search PubMed.
- Y. J. Huang, Y. Xia, Y. Z. Tao, H. M. Jin, C. Ji, S. Aniagu, T. Chen and Y. Jiang, Toxicology, 2021, 30, 452 Search PubMed.
- E. Atli and E. Tamtürk, Toxicol. Res., 2021, 27, 101–107 Search PubMed.
- W. J. Wang, C. Y. Deng, F. Chen, L. Zhang, Y. Hu, Q. Lu and A. Zhang, Toxicol. Appl. Pharmacol., 2022, 440, 115952 CrossRef CAS PubMed.
- H. Wang, X. G. Dong, Z. X. Liu, S. W. Zhu, H. L. Liu, W. C. Fan, Y. L. Hu and J. H. Sun, Anat. Rec., 2018, 301, 1115–1125 CrossRef CAS PubMed.
- M. Jang, E. J. Cho and X. L. Piao, Arch. Pharmacal Res., 2015, 38, 1263–1269 CrossRef CAS PubMed.
|
This journal is © The Royal Society of Chemistry 2022 |