DOI:
10.1039/D2RA05143E
(Paper)
RSC Adv., 2022,
12, 31124-31141
Integrating network pharmacology and an experimental validation strategy elucidates the protective effect and mechanism of callicarpa nudiflora against neuroinflammation†
Received
17th August 2022
, Accepted 20th October 2022
First published on 31st October 2022
Abstract
Abnormal activation of microglia promotes neuroinflammation (NI) in Alzheimer's disease (AD). Callicarpa nudiflora Hook et Arn. (CN) is a traditional Chinese herb with a wide range of clinical applications and definite anti-inflammatory effects. However, the anti-inflammatory action and mechanism of NI are not known. The purpose of this research was to survey whether CN could inhibit lipopolysaccharide (LPS)-induced inflammatory activation in BV-2 microglia. This study used a network pharmacology and pharmacophore model-based approach to explore the molecular mechanism of CN anti-NI by combining molecular docking and experimental validation. First, we screened the key active components and targets of CN anti-NI by network pharmacology. Then, the common structural features of these functional molecules in the treatment of neuroinflammation were predicted by 3D-QSAR pharmacodynamic modeling. Finally, the molecular mechanism of the active ingredient 5-hydroxy-3,7,4′-trimethoxyflavone (THF) against neuroinflammation was validated by molecular docking and in vitro experiments. In conclusion, this study established the structure–activity relationships of the active components of CN anti-NI and provided new insights into the pharmacological mechanisms of CN anti-NI at an integrative level.
1. Introduction
Alzheimer's disease (AD) is a progressive neurodegenerative disease that is the main cause of the progression of dementia.1 As the disease progresses, AD patients experience a variety of symptoms, beginning with impaired speech and memory, leading to a vegetative state and eventual death.2 Currently, about 50 million people worldwide suffer from dementia,3 most of whom are affected by AD.4 And the number of people with dementia in various stages is still increasing rapidly, making it a major public health problem around the world and a huge social and economic burden for patients and their families.5 AD is mainly characterized by senile macules or Aβ spots resulting from abnormal changes in amyloid precursor protein (APP) and neurofibrillary tangles (NFTs) with hyperphosphorylated tau (P-tau).6,7 Now, these two hypotheses have been in-depth studies and are thought to be important targets for the treatment of AD. Ever, up until now, clinical trials modulating both pathological proteins by direct amyloid or tau immunotherapy have shown disappointing results. Abundant nitric oxide in the brain triggers oxidative damage to neurons and contributes to the activation of apoptosis.8 Hence, effective inhibition of neuroinflammation represents a critical strategy for the treatment of AD. Neuroinflammation is an immune response activated by microglia and astrocytes in the CNS, which leads to the emission of pro-inflammatory cytokines and chemokines.9 Microglia have a role in the CNS response with neuromodulatory, neurotrophic and neuroimmune roles. When the CNS is injured, microglia are activated and translocate to the site of injury, unleashing a host of pro-inflammatory, anti-inflammatory, and neurotoxic responses.10–12 Thus, suppressing microglia activation may be an effective strategy to combat neuroinflammation. Lipopolysaccharide (LPS), an inflammation-inducing endotoxin, is frequently utilized to boost microglia and build a useful in vitro model to research the mechanism of neuronal injury.13 Over recent years, several reports on LPS-activated microglia modeling neuroinflammation have been published.14,15 Nevertheless, the in vivo effectiveness of anti-neuroinflammatory and micro-glia-targeting drugs remains undefined.16 It is therefore imperative to search for a new drug with few or no side affects.17
Traditional Chinese medicine (TCM) has a multi-target and multi-component history of preventative and curative treatment of different disorders for thousands of years18 and performed an outstanding effect. TCM is often considered to be an easily accessible, inexpensive, and less harmful manner of therapeutics. Traditional Chinese herbal medicine can be an effective alternative treatment tactic for a wide range of multifactorial and complex chronic diseases, of which AD is one.19 For this reason, the search for herbal remedies for NI has become a hot spot in modern pharmacological research. CN is a member of the Verbenaceae family, widely scattered in South China.20 CN has tremendous anti-inflammatory properties. Crude extracts and extracted fractions of CN have been reported to be found to have good anti-inflammatory activity.20,21 In addition, Sun et al. found that nine previously undescribed Seco-labdane diterpenoids isolated from the leaves of CN exhibited significant inhibition of nitric oxide (NO) production in LPS-induced mouse microglial BV2 cells.22 Although studies have documented the biological activity of CN as an anti-inflammatory agent, reports on the molecular mechanisms of the anti-inflammatory effects of CN remain scarce due to the complexity of its chemical composition. However, efforts are necessary to elucidate the molecular mechanisms by which CN regulates microglia activation in the context of AD.
Network pharmacology is a research approach developed based on systems biology and multifaceted pharmacology.23 It shows a high degree of congruence with the holistic dynamic approach and multi-component, multi-target, and multi-pathway interactions of TCM in the treatment of disorders.24 The network pharmacology approach can be an effective way to identify the critical role of herbal medicine in the treatment of diseases.25 This approach reflects the complex and diverse relationships among drugs, targets, diseases, and signaling pathways in the form of a network, thereby demonstrating the workings of various active ingredients, helping us to better understand the effects of drugs and facilitating the development of new drugs. Pharmacophore modeling is a widely applicable and effective method for the discovery of new active compounds. It is based on the common characteristics of molecules and is studied by using mathematical and statistical methods and reveals a series of ligand molecules that are shared by chemical characteristics, and based on the structure of these standard features than composite pharmacophore model of automatic generation, thus further compounds to search the database to find possible precursor molecules and explore the structure–activity relationships of a series of molecules with similar activity but different structures.26 In a nutshell, molecular docking can predict the affinity of the ligand by calculating the interaction between the ligand and the protein, illustrating the mechanism of interaction between the two at the molecular level.27
The purpose of this effort was to identify the molecular mechanism of action of the anti-neuritis activity of CN. We used network pharmacology to analyze the compound–target interactions and relevant signaling pathways of CN. And then, a 3D-QSAR pharmacophore model based on molecular co-features was built using the HipHop method to anticipate the common structural characterization of the active components in CN against NI. Finally, molecular docking showed that these compounds docked tightly with the predicted target proteins. We further established a NI model of LPS-induced BV2 microglia to evaluate the anti-NI effect of CN, which provides a basis for the rational use and in-depth development of new drugs. This workflow is shown in Fig. 1.
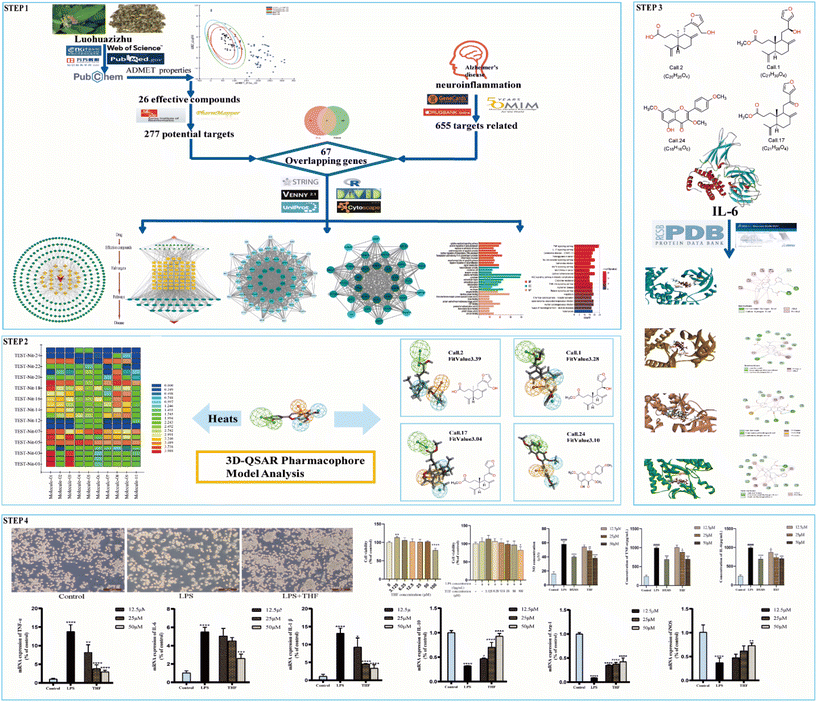 |
| Fig. 1 Schematic diagram of the anti-NI mechanism of CN. Network pharmacology analyzed the active components and core targets of CN for NI treatment; the common structural features of four anti-NI active compounds in CN were identified by 3D-QSAR pharmacological modeling; molecular docking showed that all four drug candidates could bind well to interleukin-6 targets. Cellular experiments confirmed that the candidate compound 5-hydroxy-3,7,4′-trimethoxyflavone can polarize microglia from pro-inflammatory to anti-inflammatory phenotypes by modulating the expression of inflammatory factors and promoting the anti-inflammatory phenotype. | |
2. Materials and methods
2.1. Network pharmacology
2.1.1. Screening active compounds and prediction of ADMET. By analyzing the physicochemical and structural characteristics of drug candidates, researchers have summarized the concept of drug-like properties, which has been widely used in the screening of lead compounds. Thus, as an established concept for drug design, ADMET can estimate which compounds have “drug-like” characteristics. We systematically and comprehensively investigated the chemical composition of CN publicly reported in CNKI, Wanfang, Web of Science database, and PubMed database,28 and ChemDraw29 is used to draw molecular formulas of compounds, entering the canonical (SMILES) information. Import it into the Discovery Studio software (Discovery Studio 2019; BIOVIA; San Diego, USA) and select “calculate molecular properties” in the “small molecules” module. In the “calculate molecular properties” module, click on “ADMET descriptors” to set the parameters, and the levels can be classified as 0, 1, 2, and 3, representing very good, good, poor, and very poor intestinal absorptivity and blood–brain barrier penetration, respectively, according to the human intestinal absorptivity model blood–brain barrier penetration model, relatively poor and very poor. The greater the Mahalanobis distance (MD) and the smaller the p-value of MD (MD p-value) in the hepatotoxicity and plasma protein binding rate of the drug, the less reliable the predicted results. According to the hepatotoxicity measure, 0 and 1 levels represent no hepatotoxicity and hepatotoxicity, respectively. Compounds with no hepatotoxicity and small MD values and large MD p-values are considered ideal.
2.1.2. Collection and filter of active compounds and NI-related targets. The targets relevant to each candidate compound were looked for from the PharmMapper database (https://www.lilab-ecust.cn/pharmmapper)30 and then converted to the corresponding gene names from the Uniprot database (https://www.uniprot.org/).31 Furthermore, the SMILES formula of the candidate components was used to seek potential targets on the SwissTargetPredictioninterface (https://www.swisstargetprediction.ch/).32 Finally, the constituent targets derived from the two databases were then combined to eliminate duplicate terms.Known disease targets were located and filtered by the keywords “neuroinflammation” by using GeneCardsdatabase (https://www.genecards.org/),33 OMIM database (https://omim.org/),34 and DrugBank database (https://www.drugbank.ca/).35 Afterward, all results were then consolidated and intersecting targets were excluded.
2.1.3. Obtain intersection target genes and draw a Venn-diagram. To obtain potential targets for CN treatment of NI, the targets of CN components collected in the previous study were incorporated with the NI-related targets to obtain the co-targets of both. The co-targets of both were then input to Draw Venn Diagram (https://bioinformatics.psb.ugent.be/webtools/Venn/) Center for analysis,36 and subsequently, their co-targets were used as the core targets for follow-up analysis.
2.1.4. Protein-protein-interaction (PPI) and hub target analysis. To recognize the core modulation targets, we input the common targets of CN anti-NI obtained earlier into the STRING database (https://string-db.org/)37 for PPI analysis. The condition was selected as “Homo sapiens”, and the default confidence level was greater than or equal to 0.4; other parameters remained unchanged. Afterward, Cytoscape 3.7.2 was used to visualize the interaction information in the network. The CytoNCA plugin of Cytoscape38,39 were selected for analyzing the topological properties of the data. Among these, betweenness centrality (BC), closeness centrality (CC), and degree centrality (DC) were selected to assess the significance of nodes in the network. The greater the value of these three, the more critical the target pointed is in the network. In the PPI network, BC, CC, and DC are selected as variables for the core targets, and the core target network correlation diagram is constructed on the result of the selection.
2.1.5. GO and KEGG pathway enrichment analysis. To further analyze the specific mechanism of action of CN in anti-NI, we entered the common targets of active ingredients and diseases into the DAVID database (https://david.ncifcrf.gov/summary.jsp) for biological procedure enrichment analysis and pathway enrichment analysis, selecting “Homo sapiens” and the significance condition P < 0.01. The results of the enrichment analysis were displayed visually based on R 4.0.2 and related R packages (cluster profile, ggplot2, enrichplot, preview, and BiocManager).
2.1.6. Network construction. Network building was done using the network visualization software Cytoscape 3.7.2, which can intuitively show the compound–target–pathway association between CN and NI, including 2 networks, respectively: (1) compound-predicted target for CN network; (2) effective compound–hub target–pathway network for CN anti-NI.
2.2. Construction of the 3D-QSAR pharmacophore model
2.2.1. Construction of pharmacophore model based on common molecular feature. Twenty-six IL-6 inhibitors that passed the laboratory analysis were selected from the published papers as the training set.40. (ESI Fig. 1†). The structures of these compounds were drawn using ChemDraw, and the energies of the molecules were energy minimized by the Minimization module in Discovery Studio software (Discovery Studio 2019; BIOVIA; San, USA). Based on the common structural characteristics of the 26 compounds in the training set, the ultimate pharmacophore model has been created utilizing the HipHop approach of the Common Feature Pharmacophore generation module under the Pharmacophore item in the Discovery Studio software.41 Briefly, the principal value of the active compound was set to 2, and the MaxOmitFeat value was set to 1, indicating the conformational space of the established pharmacophore reference ligand. The hydrogen bond acceptor, hydrogen bond donor, hydrophobic center, positively charged ion center, and aromatic ring center were selected as the characteristic elements of pharmacophore in the HipHop module, and the range of each pharmacophore was set as 0–5. The best mode was adopted for superposition, the upper limit of conformation generated by each compound was set at 255, and only the model whose energy difference with the lowest conformation was less than 20 kcal mol−1 was saved. After the analysis, only the 10 highest-rated pharmacophore models were maintained.
2.2.2. 3D-QSAR pharmacophore verification. The top 10 generated pharmacophores were evaluated using the Ligand Profiler module in Discovery Studio. 21 active compounds with IL-6 inhibition published in the literature and 5 with no reported activity were used as the test set for the generated pharmacophores (ESI Fig. 2†). The final selected pharmacophore was required to meet the characteristics of having a high match to the active compound and a low match to the inactive compound with the highest possible scoring value. After obtaining the preferred pharmacophore, the 26 active ingredients predicted by network pharmacology were matched to the pharmacophore and the results were analyzed.
2.3. Molecular docking
2.3.1. Reliability verification of molecular docking process. In the current study, we selected four active components estimated by the pharmacological model as ligand compounds, and selected IL-6, which had the highest score in the network pharmacological search filter, as the receptor protein, and downloaded the crystal structure of the protein. Discovery Studio was used for molecular docking, and the ligand expansion method was used to determine the active site. This means that the ligand is centered on the location of the ligand and then expands outward to a certain extent, generally with a radius of 9 Å. The receptor residues within this range constitute the relevant active site. Before the molecular docking of the four active ingredients, the accuracy and reliability of the docking process should be evaluated. The target protein complex containing the original ligand was first obtained from the RCSB PDB database (https://www.rcsb.org/), after which the original ligand was abstracted and then docked to the binding pocket of the complex, and the root-mean-square deviation (RMSD) of the conformation of the docked ligand from the conformation of the ligand in the original crystal structure was calculated. It is generally believed that the method is reliable when RMSD is less than or equal to 2.0.
2.3.2. Molecular docking of four active ingredients. The prepared target proteins and four active ingredients are imported into Discovery Studio and docked using the LibDock module. This method is fast and accurate and is suitable for fast and accurate virtual screening of large databases. The parameters' active site radius is set to 9, the number of Hotspots is set to 100, and the rest of the parameters are Default.
2.4. Experimental verification in vitro
2.4.1. Microglial BV2 cell culture. The BV2 microglial cell line was purchased from the China Typical Culture Collection of Wuhan University. Cells were cultured in Dulbecco's Modified Eagle Medium (DMEM) (Solarbio, China) containing 10% fetal bovine serum (FBS, Gibico, Mexico origin). Cells were incubated at 37 °C and 5% CO2.
2.4.2. Cell viability assay. The cell viability is evaluated via the Cell Counting Kit-8 (CCK-8) assay (Beyotime, China). BV2 cells at the logarithmic growth stage were inoculated in 96-well plates at a density of 9 × 103 per well, 100 μL per well, and after the cells were plastered, the experiment was divided into the blank group; the LPS group (1 μg mL−1); THF in each dose group (0, 3.125, 6.25, 12.5, 25, 50 and 100 μM), Each group was repeated 5 times, the blank group with fresh culture medium, dosing group pretreated for 3.5 h before adding LPS stimulation or THF alone. After incubation for 24 h, 10% CCK-8 medium was added and incubated for 2 h at 37 °C. After that, the absorbance was tested at 450 nm.
2.4.3. Nitric oxide (NO) assay. The concentration of NO in the cell culture supernatant was determined by the Griess method. Cells were inoculated in 96-well plates at a density of 9 × 103 per well. After the cells were plastered, the experiment was divided into the blank group; LPS group (1 μg mL−1); THF each dose group (12.5 μM, 25 μM and 50 μM), with 5 parallel sub-wells in each group, and the blank group was added with fresh culture medium, and the drug administration group was pretreated for 3.5 h before adding LPS stimulation. After 24 h of incubation, supernatants were collected and assayed without following the manufacturer's protocol (Beyotime, China).
2.4.4. Enzyme-linked immunosorbent assay. Cellular inflammatory factors tumor necrosis factor TNF-α and interleukin-6 were evaluated using an enzyme-linked immunosorbent assay (ELISA) kit (Beyotime, China). Cells were cultured in 24-well plates at a density of 1 × 105 per well. After allowing the cells to adhere to the walls, they were processed as described in Section 2.4.3. After 24 h of enculturation, the cell culture supernatant was obtained and the supernatant was collected through centrifugation (4 °C, 1000 × g, 10 min) and then added to a 96-well plate in the ELISA kit at 100 μL per well. IL-6 and TNF-α concentrations were measured with the ELISA manufacturer's instructions.
2.4.5. RNA isolation and quantitative real-time polymerase chain reaction. Total sample RNA was extracted using Trizolszol up (TransGen Biotech, China) and reverse transcribed into cDNA using the TransScript One-Step gDNA Removal and cDNA synthesis SuperMix (TransGen Biotech., China) according to the manufacturer's guidelines, qPCR was performed on an ABI Step One system (Thermo, USA) using the TransStrart Green QpcrSuperMix kit (TransGen Biotech, China). The primer sequences used in this study are listed in Table 1 β-actin was used as a normalization control. The relative RNA expression of each gene was analyzed by the 2−ΔΔCT method.
Table 1 67 Potential targets of CN anti-NI
No. |
Gene |
No. |
Gene |
No. |
Gene |
No. |
Gene |
No. |
Gene |
1 |
UGT2B7 |
15 |
ACE |
29 |
IL6 |
43 |
EGFR |
57 |
MAP2K1 |
2 |
AR |
16 |
F2 |
30 |
PTGS2 |
44 |
TRPV1 |
58 |
SNCA |
3 |
NR1H3 |
17 |
NOS3 |
31 |
STAT3 |
45 |
ACHE |
59 |
CASP8 |
4 |
MME |
18 |
NOS2 |
32 |
CYP1B1 |
46 |
BCL2 |
60 |
FOS |
5 |
ESR1 |
19 |
KIT |
33 |
PLG |
47 |
RET |
61 |
MET |
6 |
NR3C1 |
20 |
CA2 |
34 |
ABCC1 |
48 |
SKY |
62 |
TLR2 |
7 |
TP53 |
21 |
NOS1 |
35 |
AKT1 |
49 |
MAPT |
63 |
NLRP3 |
8 |
IL1B |
22 |
IL6ST |
36 |
VEGFA |
50 |
SRC |
64 |
MAPK1 |
9 |
MIF |
23 |
PPARG |
37 |
CASP3 |
51 |
CYP2C19 |
65 |
MAPK14 |
10 |
MMP3 |
24 |
JAK1 |
38 |
TTR |
52 |
CYP3A4 |
66 |
MAPK3 |
11 |
MMP13 |
25 |
TLR1 |
39 |
ABCB1 |
53 |
CYP2C9 |
67 |
NTRK1 |
12 |
MMP9 |
26 |
COMT |
40 |
PIK3CA |
54 |
CYP1A2 |
|
|
13 |
MMP2 |
27 |
APP |
41 |
RELA |
55 |
BCHE |
|
|
14 |
MAPK8 |
28 |
TNF |
42 |
TERT |
56 |
PDGFRA |
|
|
2.4.6. Statistical analysis. GraphPad Prism 8.0 software (La Jolla, CA, USA) was utilized. Statistical analysis of the data was performed. The measured data were represented as mean and standard deviation (SD). Differences between the two sample means were statistically analyzed by t-test, and P-values < 0.05 were deemed statistically significant. The model and control groups were considered statistically significant at #p < 0.05, ##p < 0.01, ##p < 0.001, ####p < 0.0001. Values of *p < 0.05, **p < 0.01 and ***p < 0.001 indicate statistical significance between the treatment and model groups.
3. Results
3.1. Network pharmacology analysis
3.1.1. The acquisition of active compounds using ADMET screening. In total, we identified 137 drug-related components from the published literature. In this study, the solubility, human intestinal absorption, blood–brain barrier penetration, hepatotoxicity, and plasma protein binding of these compounds were predicted using ADMET, resulting in the screening of a total of 26 CN anti-NI active compounds. Among them, Fig. 2 shows the distribution of only 26 active compounds in the 95% and 99% confidence intervals of the blood–brain barrier permeability and intestinal absorption models. Specific information on the other models is shown in ESI Table 1,† and information on the 26 active compounds is shown in ESI Table 2.†
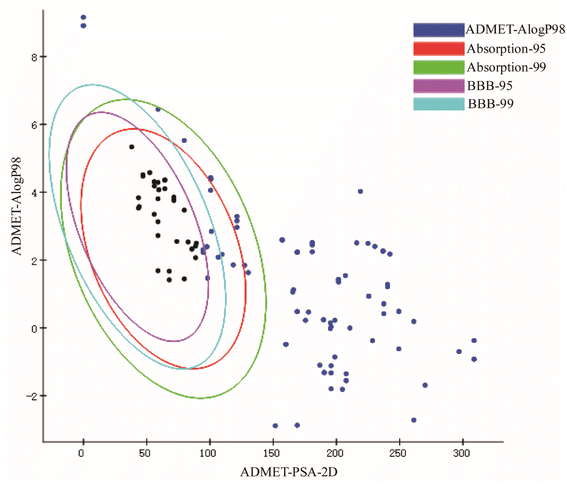 |
| Fig. 2 Distribution of intestinal absorption and blood–brain barrier penetration of 137 CN compounds predicted by ADMET. | |
3.1.2. Targets of bioactive compounds in CN and NI-related targets. We identified the SMILES formula of these compounds by searching in PubChem. 227 potential targets corresponding to the above compounds were retrieved according to the structural similarity of the compounds from SwissTar-get prediction and PharmMapper databases. In addition, 602 NI-related gene targets were gained from the GeneCards database, 40 gene targets from the OMIM database, and 31 gene targets from the DrugBank database. After duplication removal, a total of 655 NI-associated gene targets were acquired. Next, the Venn diagram showed that there were 67 intersections of disease-related gene targets and drug-related gene targets (Fig. 3A), and the specific target information is shown in Table 1, and these targets were determined as potential targets for CN anti-NI.
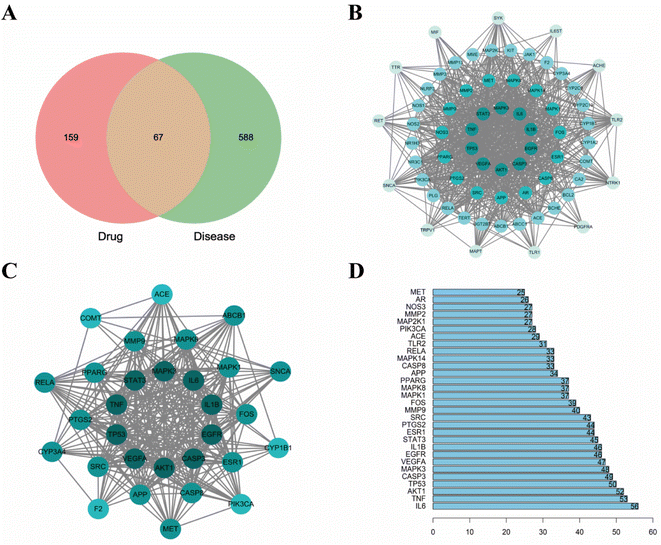 |
| Fig. 3 Drug-disease target intersecting Venn diagrams with protein–protein interaction networks. (A) Venn diagram of 227 targets of CN intersected with 655 targets of NI, (B) PPI network of shared targets correlated with CN and NI. Darker colors indicate larger degree values and greater importance in this network, (C) a protein–protein cluster containing 30 nodes and 316 edges, (D) top 30 core targets of the PPI network (Y-axis shows the top 30 important targets, X-axis shows the number of interconnected targets). | |
3.1.3. Protein-protein-interaction (PPI) network construction. The 67 shared targets were entered into the STRING database, and then the data acquired from this center were entered into Cytoscape 3.7.2 for network mapping and topology analysis (Fig. 3B). The PPI network results showed that it was composed of 67 nodes and 861 edges. The topological properties of the shared targets were analyzed through the CytoNCA plugin with median BC, CC, and DC values of 15.80787644, 0.6, and 24, respectively. 30 genes were finally found to have target values above the median, and these genes were deemed to be the core targets for CN treatment of NI (Fig. 3C). The number of those nodes' edges was 56 in IL-6, 53 in TNF, 52 in AKT1, 50 in TP53, 49 in CASP3, 48 in MAPK3, 47 in VEGFA, 46 in EGFR, 46 in IL1B, 45 in STAT3, 44 in ESR1, and 44 in PTGS2, respectively. It is suggested that these genes may be the core genes for the CN treatment of NI. The degree values of all protein nodes correlated with the targets are shown in the bar graph (Fig. 3D).
3.1.4. GO and KEGG pathway enrichment analyses. To explain the therapeutic mechanism of CN anti-NI more clearly, we analyzed 30 shared targets with GO annotation and KEGG pathway analysis utilizing the DAVID database. After screening with p-values ≤ 0.01, 239 GO terms were derived, including 172 for biological processes (BP), 50 for molecular functions (MF), and 17 for cellular constituents (CC). The enrichment results were sorted depending on the p-value of each biological process and pathway. This is illustrated in Fig. 4. Among the 172 BP GO terms, the most enriched BP terms were the transcriptional promotion of RNA polymerase II promoter (GO:0045944), and cytokine-mediated signaling pathway (GO:0019221). And among the 50 MF GO terms, the most significant terms relevant to NI treatment are identical protein binding (GO:0042802), and ATP binding (GO:0005524). Among the 17 CC GO terms correlated with NI treatment, plasma membrane (GO:0005886), and cytoplasm (GO:0005737) were listed in the top 10 entries.
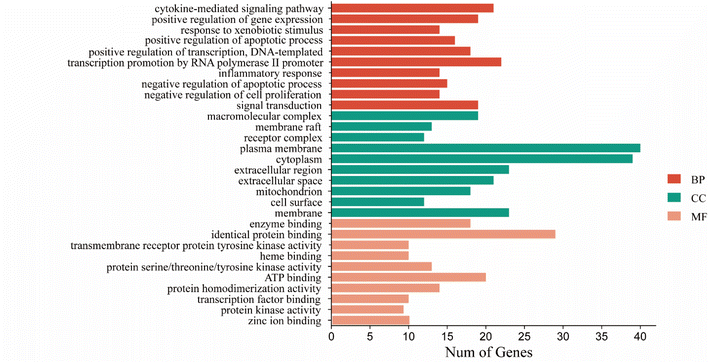 |
| Fig. 4 CN annotation of GO functions for NI. The bar chart shows the top 10 GO enrichment items for BP, CC, and MF. | |
The KEGG pathway enrichment analysis yielded as many as 161 pathways, and the top 20 pathways were displayed for graphical visualization. The data were ranked per their p-values and then converted into histograms and bubble plots for presentation, respectively (Fig. 5A and B). The results showed that the common signaling pathways were mainly focused on the MAPK signaling pathway (hsa04010), IL-17 signaling pathway (hsa04657), lipid and atherosclerotic signaling pathway (hsa05417), Toll-like receptor signaling pathway (hsa04620), and TNF signaling pathway (hsa04668). This suggests that the active components of CN may exert anti-NI effects by participating in various bioregulatory processes.
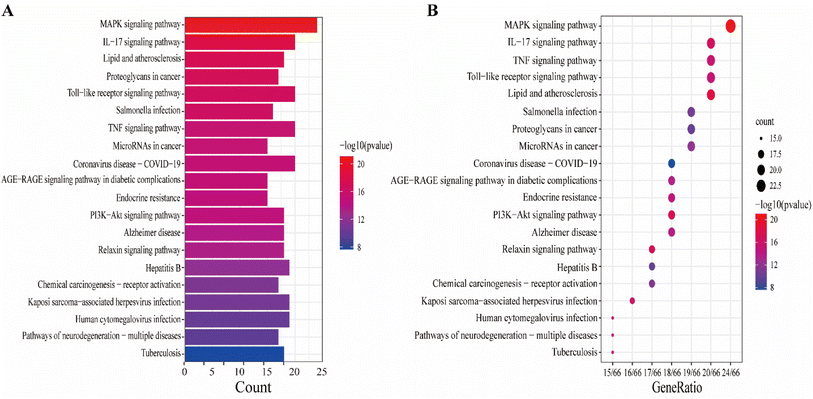 |
| Fig. 5 Kyoto Encyclopedia of Genes and Genomes (KEGG) enrichment analysis of 67 common targets of CN anti-NI, (A) histogram of the top 20 KEGG pathways, (B) bubble plot of the top 20 KEGG pathways. | |
3.1.5. Network construction of compound-predicted target of CN. As shown in Fig. 6, a total of 26 effective components and 277 predicted targets in CN were derived. The Herbs–compound–predicted target network (H–C–P) includes 253 nodes (16 compounds and 285 targets) and 407 edges. In this network, 5-hydroxy-3,7,4′-trimethoxyflavone (degree = 28), callicarpaolide (degree = 25), 7α-hydroxy sandaracopimaric acid (degree = 24), and nudiflflopenes I (degree = 22) were the main active ingredients of CN for the treatment of various diseases. It is furthermore that multiple targets are regulated by multiple chemical components at the same time. These results demonstrate that CN-active compounds have an important role in disease treatment through multi-target modulation.
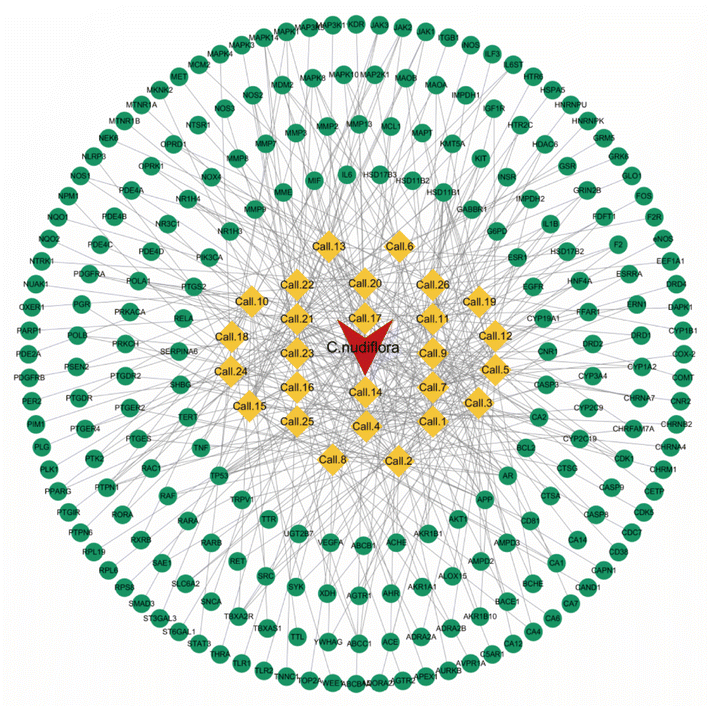 |
| Fig. 6 The herb-compound-prediction target network (H–C–P) is composed of 253 nodes (16 ingredients and 285 targets) and 407 edges. | |
3.1.6. Construction of effective compounds-hub targets-pathway network of CN for anti-NI. To further reveal the mechanism of CN action on NI, this section constructs a systematic and mature herbal-active-component–center–target–pathway–disease network (C–E–H–P–D) through 26 active ingredients, 67 shared targets, and 30 KEGG signaling pathways (Fig. 7). The network consists of 125 nodes and 662 edges, and a single compound in the CN can interact with multiple targets, and a single target can be regulated by multiple components. For example, 7α-hydroxy sandaracopimaric acid targets 14 central targets, including MIF, TNF, IL6, IL1A, PIK3CA, RELA, JAK1, BCL2, NOS1, TERT, TRPV1, MME, ESR1, and CA2. 5-Hydroxy-3,7,4′-trimethoxyflavone also modulates 9 central targets such as MAPK8, TNF, TP53, CASP8, NOS1, IL1B, ABCC1, MAPT, and ACHE. There are other compounds and related targets, reflecting the positive multi-component and multi-target intervention of CN in the treatment of NI. In the bioinformatics network, purple represents herb; green indicates bioactive compounds from CN; yellow stands for the target genes; blue represents signal pathway; red is the disease. One target simultaneously corresponds to multiple components, and besides a potential active ingredient also responds to multiple targets. It can be seen that the neuroprotective mechanism of Chinese medicine is featured multiple ingredients, multiple targets, and multiple mechanisms. The details of the effective compound–hub target–pathway–disease network of Chinese medicine are illustrated in Fig. 5 and 7. The C–E–H–P–D system network indicates that many target genes of CN are closely related to inflammation-related signaling pathways, including the IL-17 signaling pathway (hsa04657), TNF signaling pathway (hsa04668), Toll-like receptor signaling pathway (hsa04620) and MAPK signaling pathway (hsa04010), and the schematic diagram of inflammation-related signaling pathways is presented in Fig. 8.
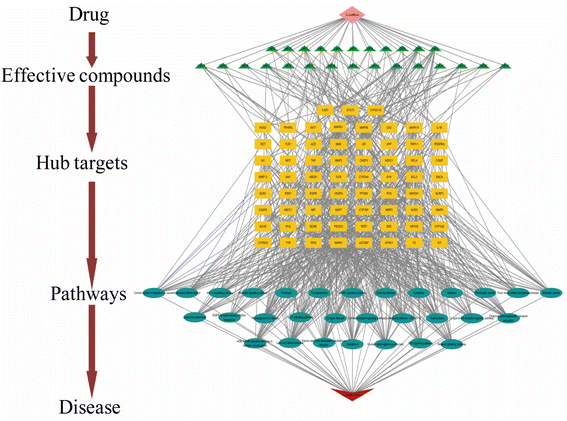 |
| Fig. 7 Chinese medicines-effective compounds–hub targets–pathways–disease (C–E–H–P–D) network of Callicarpa nudiflora Hook et Arn. (CN) an-ti-neuroinflammation (NI). The C–E–H–P–D network of CN anti-NI was composed of 26 ingredients, 67 common targets, and 30 pathways, covering 125 nodes and 662 edges. The purple color represents herbs; green indicates bioactive compounds from CN; yellow stand for the target genes; blue represents signal pathway; red is the disease. | |
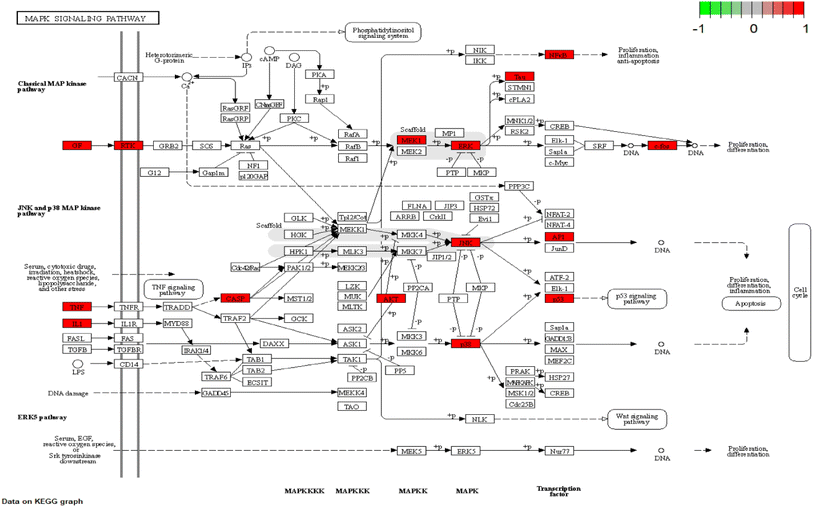 |
| Fig. 8 Schematic diagram of MAPK signaling pathway. The red nodes represent CN–NI-related genes. | |
3.2. 3D-QSAR pharmacophore model analysis
Although the network pharmacology screening successfully anticipated important active components of CN for the treatment of NI and the core anti-NI target of CN, interleukin-6. However, to further elucidate the common structural features of the key active ingredients predicted by network pharmacology in the treatment of neuroinflammatory disorders. In this section, a pharmacophore model based on common molecular features was constructed to further explore the structural commonality of active compounds in CN anti-NI, using the key target interleukin-6 as the active study target. It provides a basis for elucidating the material basis of CN anti-NI, expanding the scope of Chinese medicine research, and enriching the theory of Chinese medicine.
3.2.1. Construction and evaluation of 3D-QSAR pharmacophore model. To elucidate the common structural features among the CN anti-NI active components and to find the best pharmacophore model for CN anti-NI, ten 3D-QSAR pharmacophore models were developed in this section using the HipHop method. Table 2 shows the resulting parameters of the ten pharmacophore models. Each row in the table represents one pharmacophore. As shown in the table, the first pharmacophore has a higher score. Among them, pharmacophore 01 has the feature RHHA, which indicates that the pharmacophore contains one aromatic ring center, two hydrophobic features, and one hydrogen bond acceptor feature. The ranking shows that this pharmacophore has a score of 109.898; a Direct Hit indicates that the pharmacophore features match 4 small molecules. Partial Hit indicates that the number of partially matched pharmacophores containing 4 small molecules is 0. Max Fit indicates that all 4 pharmacophore features can be matched (Table 3).
Table 2 Parameters of 10 common features of the pharmacophore
Pharmacophore |
Feature |
Rank |
Direct Hit |
Partial Hit |
Max Hit |
01 |
HHHA |
109.898 |
11111111111110 |
00000000000001 |
4 |
02 |
HHHA |
109.617 |
11111111111110 |
00000000000001 |
4 |
03 |
HHHA |
107.512 |
11111111111110 |
00000000000001 |
4 |
04 |
RHHA |
107.175 |
11111111111110 |
00000000000001 |
4 |
05 |
RHHA |
104.751 |
11111111111110 |
00000000000001 |
4 |
06 |
RHHA |
104.386 |
11111111111110 |
00000000000001 |
4 |
07 |
HHHA |
103.734 |
11111111111110 |
00000000000001 |
4 |
08 |
HHHA |
103.709 |
11111111111110 |
00000000000001 |
4 |
09 |
HHHA |
103.257 |
11111111111110 |
00000000000001 |
4 |
10 |
RHHA |
102.531 |
11111111111110 |
00000000000001 |
4 |
Table 3 qPCR primer sequence
Gene |
|
5′ to 3′ |
TNF-a |
Forward |
ATGGCCTCCCTCTCATCAGT |
Reverse |
TTTGCTACGACGTGGGCTAC |
IL-6 |
Forward |
CTCCCAACAGACCTGTCTATAC |
Reverse |
CCATTGCACAACTCTTTTCTCA |
IL-10 |
Forward |
ACATACTGCTAACCGATCCTT |
Reverse |
GCTCCACTGCCTTGCTCTT |
Arg-1 |
Forward |
ATGCTCACACTGACATCAACAC |
Reverse |
CTTCCATCACCTTGCCAATCC |
IL-4 |
Forward |
GCTAGTTGTCATCCTGCTCTTC |
Reverse |
GGTGTTCTTCGTTGCTGTGA |
iNOS |
Forward |
ACTACTGCTGGTGGTGACAA |
Reverse |
CCTGAAGGTGTGGTTGAGTTC |
β-actin |
Forward |
AAGTGTGACGTTGACATCCG |
Reverse |
TCTGCATCCTGTCAGCAATG |
To validate the validity of the pharmacophore model constructed by HipHop, we need to verify whether the pharmacophore has a good ability to distinguish between active and inactive molecules by using the training set of known compounds with activity. Fig. 9 shows the heat map of the ten 3D-QSAR pharmacophore models used to predict the training set compounds. ESI Table 3† shows the corresponding fits. The closer the fit value is to 4, the better the compound fits the model; the closer the fit value is to 0, the lower the compound fits the model. As shown in Fig. 9, the FitValue values of the compounds with higher activity in “Pharmacophore 01” were higher than those with lower activity in “Pharmacophore 01”. This result indicates that “Pharmacophore 01” is the best 3D-QSAR pharmacophore model to predict the activity of CN compounds. The pharmacophore model may be the decisive therapeutic pharmacophore for CN anti-NI.
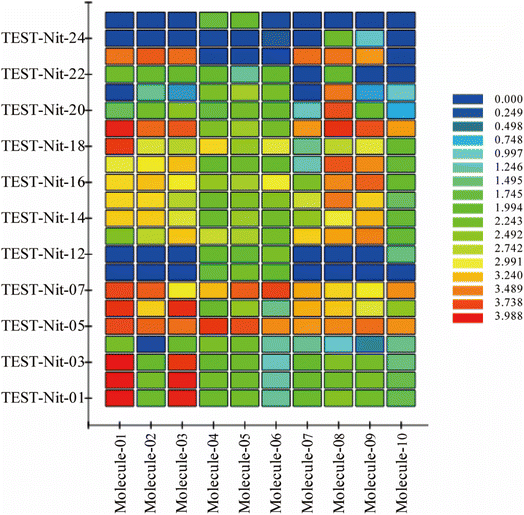 |
| Fig. 9 Heat map of the 10 pharmacological models predicted for the test group of ingredients. | |
In summary, network pharmacology was used to successfully screen for highly active compounds for CN anti-NI. Then, a HipHop approach was used to construct a pharmacophore model predicting the common molecular structure-based features possessed by CN active molecules in the treatment of neuroinflammation. Finally, the validity of the 3D-QSAR pharmacophore was verified by a training set of compounds with known activity, and the possible decisive therapeutic effect of “pharmacophore 01” was postulated. Therefore, we will validate it by molecular docking and in vitro experiments to further explore the molecular mechanism of CN anti-NI.
3.2.2. Prediction of CN activity based on the 3D-QSAR pharmacophore model. For the exploration of the structural characteristics of CN anti-NI, this part used the HipHop method to forecast the anti-NI activity of CN compounds by building 3D-QSAR pharmacophore models which are based on molecular common features. As shown in Fig. 10, 5-hydroxy-3,7,4′-trimethoxyflavone, ent 3,4-seco-16-hydroxy-12,15-epoxy-4(18),8(17),12,14-labdatetraen-3-oic acid, nudiflflopenes C, nudiflflopenes M displayed higher activity in the pharmacophore model with FitValues of 3.39, 3.28, 3.10 and 3.04, respectively. Furthermore, in the H–C–P network, 5-hydroxy-3,7,4′-trimethoxyflavone, Callicarpaolide, 7α-hydroxy sandaracopimaric acid, and nudiflflopenes I was suggested to be essential active ingredients of CN for the treatment of various diseases. Both models showed good results for the prediction of 5-hydroxy-3,7,4′-trimethoxyflavone. Furthermore, we choose the predicted values of the 3D-QSAR pharmacophore model established by this method as a follow-up study because of the better accuracy of the pharmacophore model built by 3D-QSAR. In parallel, we selected 5-hydroxy-3,7,4′-trimethoxyflavone (THF), a common ingredient predicted by the network pharmacology and 3D-QSAR pharmacophore model, as the active ingredient for in vitro experimental validation.
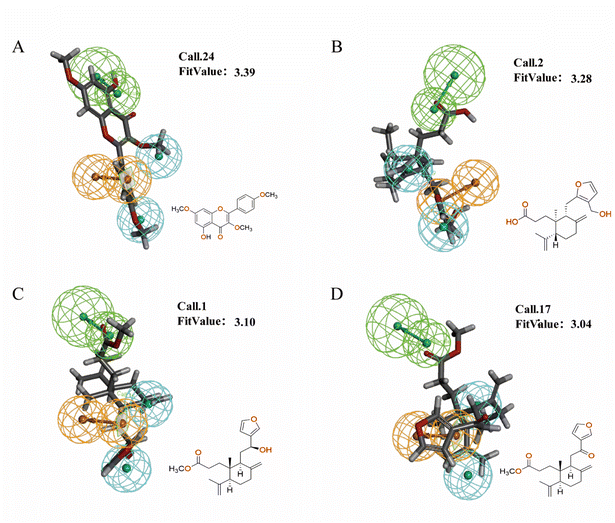 |
| Fig. 10 Pharmacophore matching of 4 potential IL-6 inhibitory active components. (A) Pharmacophore 10 with ent 3,4-eco-16-hydroxy-12,15-epoxy-4(18), 8(17),12,14-labdatetraen-3-oic acid (Call.2), (B) pharmacophore 10 with nudiflflopenes C (Call.1), (C) pharmacophore 10 with 5-hydroxy-3,7,4′-trimethoxyflavone (Call.24), (D) pharmacophore 10 with nudiflflopenes M (Call.17). | |
3.3. Molecular docking results
In molecular docking, the ligand is bound to one or more amino acid residues through hydrogen bonds in the active pocket, participating in the process of conformational change and energy complementation.42 The binding site, binding fraction, and RMSD value can visualize the interaction and stability of the docking model. Here, we constructed protein receptors based on IL-6, the core target of PPI. Based on the 3D-QSAR pharmacophore model, four highly active compounds were screened from CN for molecular docking. Before performing molecular docking of CN components, we first verified the reliability of the molecular docking process. Since the crystal structure of the IL-6 (6CQ5) target protein contains the original ligand of F8S. We extracted the ligand molecules from the complex and then docked them into the binding pocket of the complex using LibDock. The root-mean-square deviation (RMSD) of the docked ligand from the ligand conformation in the original crystal structure was calculated to be 1.41428, which is less than 2. 0, indicating that this docking process has good accuracy for this target. Subsequently, we used ent 3,4-seco-16-hydroxy-12,15-epoxy-4(18),8(17),12,14-labdatetraen-3-oic acid, nudiflflopenes C, 5-hydroxy-3,7,4′-trimethoxyflavone, nudiflflopenes M four active ingredients as ligands and key target proteins as receptors for molecular docking. The docking results are shown in ESI Table 4.† The docking results showed that the docking scores of the four active compounds to the core target IL-6 were greater than 90, indicating relatively stable binding between the ligand and the receptor (Fig. 11).
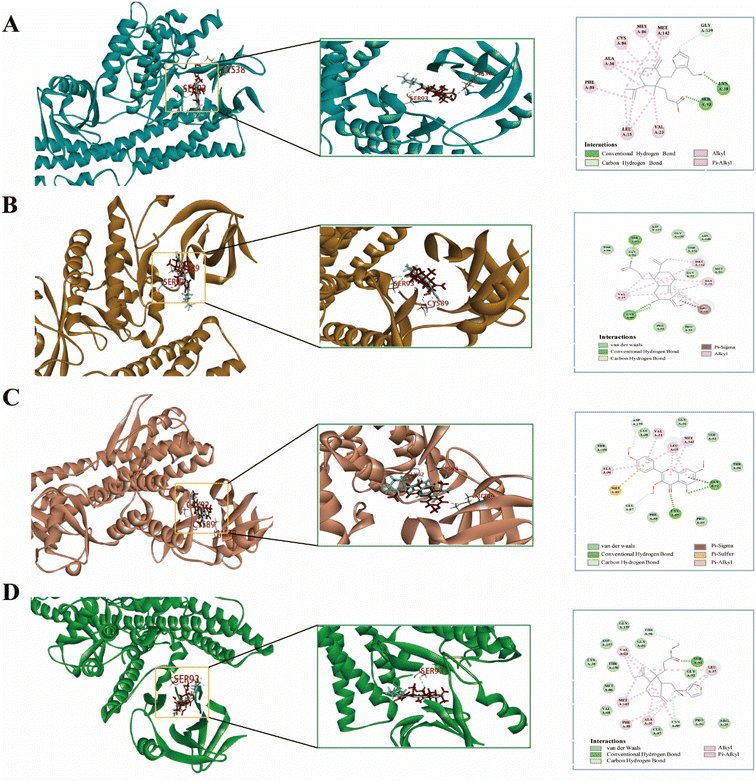 |
| Fig. 11 Molecular docking of the CN–NI active compound to the receptor protein. Gray represents the original ligand of the crystal structure. Red represents CN–NI compounds. (A) Ent 3,4-seco-16-hydroxy-12,15-epoxy-4(18),8(17),12,14-labdatetraen-3-oic acid with IL-6, (B) nudiflflopenes C with IL-6, (C) 5-hydroxy-3,7,4′-trimethoxyflavone with IL-6, (D) nudiflflopenes M with IL-6. | |
Fig. 11 shows the binding pattern of the IL-6 target protein to its corresponding original ligand and the four CN active compounds mentioned above. A high similarity was observed between the active compounds and the original ligands of the proteins, which occupy almost the same active site. The binding pattern of ent 3,4-seco-16-hydroxy-12,15-epoxy-4(18),8(17),12,14-labdatetraen-3-oic acid at the IL-6 active site is shown in Fig. 11A in three- and two-dimensional modes, respectively. Two amino acid residues formed hydrogen bond interactions with ent3,4-seco-16-hydroxy-12,15-epoxy-4(18),8(17),12,14-labdatetraen-3-oic acid, and the phenolic hydroxyl group on this compound was attached to Ser93 and Lys38 residues via two hydrogen bonds, respectively. Fig. 11B shows the mode of binding of nudiflflopenes C to the IL-6 active site. The molecule is located in the binding capsule surrounded by residues Gys89 and Ser93. The binding pattern between 5-hydroxy-3,7,4′-trimethoxyflavone and IL-6 is shown in Fig. 8C. The residues of Cys89 and Gly92 form stable hydrogen bond interactions with the carbonyl group in 5-hydroxy-3,7,4′-trimethoxyflavone and the hydroxyl group on the benzene ring, respectively. Fig. 11D shows the binding pattern between nudiflflopenes M and IL-6, forming a hydrogen bond to the carbonyl group with the Ser93 residue in IL-6.
Taken together, these docking results provide evidence that the active compound of CN can bind stably to the active pocket of the receptor protein, forming a stable binding conformation.
3.4. Experimental verification in vitro
3.4.1. THF inhibits the production of pro-inflammatory cytokines. CCK-8 assay was performed after treatment with different concentrations of THF to determine the effect on the viability of BV2 microglia. Fig. 12B shows that none of the THF concentrations in the range of 12.5–50 μM affected the viability of BV2 microglia (P > 0.05). Therefore, the concentration range of 12.5–50 μM was used in subsequent experiments. In addition, to determine the combined toxicity of LPS and THF on BV2 microglia, we added LPS (1 μg mL−1) on top of the previous one to determine cell viability (Fig. 12C). There was no significant effect on cell viability (P > 0.05). To determine the effect of THF on LPS-induced activation of BV2 cells, the morphological changes of BV2 cells were observed in a bright field, and it was found that the cells in the normal group growing well and BV2 cells were in a quiescent state, with semi-adherent round or spindle-shaped cells and smaller cytosol. After LPS intervention for modeling, most BV2 cells tended to a pro-inflammatory amoeboid morphology; in contrast, some cells reverted to a round or spindle shape after pretreatment with THF (50 μM) (Fig. 12A). To investigate the potential modulatory effect of THF on pro-inflammatory responses, BV2 microglia were treated as described above. After LPS treatment, NO levels were significantly increased, whereas pretreatment with THF (50 μM) significantly reduced LPS-induced production of these pro-inflammatory cytokines in the extracellular medium (Fig. 12D).
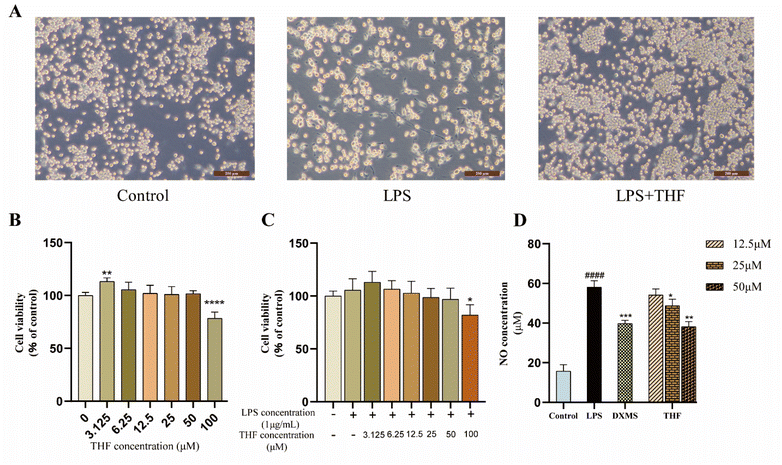 |
| Fig. 12 Effect of THF on the viability and activation state of BV2 microglia and the inhibitory effect of NO. (A) Changes in cell morphology under different treatments. (B) Effect of different concentrations of THF on cell viability. (C) Effect of different concentrations of THF and LPS (1 μg mL−1) on cell viability. (D) THF inhibits NO production in LPS-stimulated BV2 microglia. | |
3.4.2. THF up-regulates expression of M2 stage-related genes. We hypothesized that THF may exert its anti-inflammatory effects by promoting the M2 polarization state as well as by altering the M1 polarization of BV2 microglia. First, we examined the inhibition of inflammatory factors IL-6 and TNF-α by THF in LPS-induced BV2 cells by ELISA kits (Fig. 13A and B). After that, we investigated the changes in the expression levels of M1 and M2 status marker genes in LPS-induced BV2 cells after THF pretreatment. In LPS-stimulated BV2 cells, THF pretreatment significantly suppressed the mRNA expression levels of M1 status-related genes (Fig. 13C–E). Meanwhile, THF pretreatment increased the mRNA expression levels of M2-related genes (Fig. 13F–H). These results suggest that THF may be able to shift the phenotype of LPS-stimulated microglia from M1 polarization to M2 polarization.
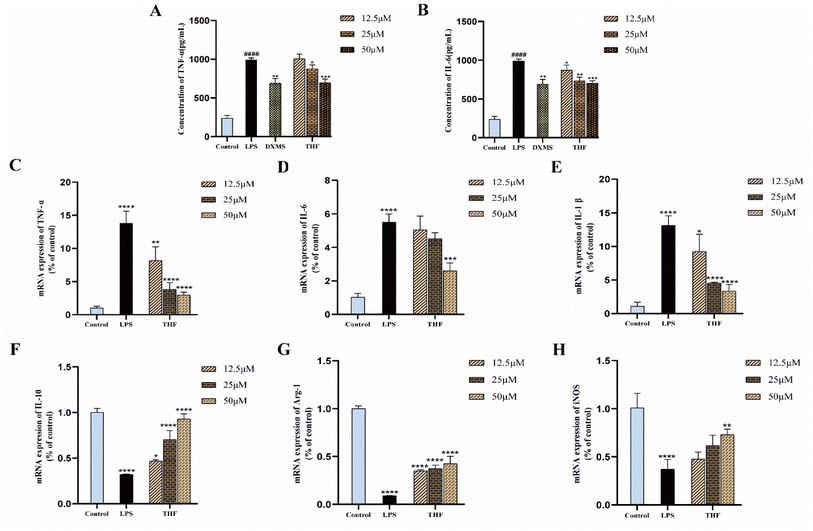 |
| Fig. 13 Impact of THF on LPS-induced inflammatory cytokines in BV2 microglia. (A) TNF-αlevels in BV2 microglia. (B) IL-6 levels in BV2 microglia. (C–E) THF attenuates the expression of M1 status-related genes in BV2 microglia. (F–H) THF upregulates the expression of M2 status-related genes in BV2 microglia. | |
4. Discussion
Neuroinflammation is a reaction involving all cells in the Central Nervous System (CNS), contains neurons, glial cells, etc., and specifically refers to inflammation occurring in brain tissue. When pathogens invade brain tissue, they activate various genes and proteins to produce proinflammatory factors and cytotoxic factors, for example, inducible Nitric Oxide Synthase (iNOS), interleukin-1β (IL-1β), interleukin-6 (IL-6), tumor necrosis factor-α (TNF-α), cyclooxygenase-1 (Cox-1) and cyclooxygenase-2 (COX-2).43–46 When these cytokines are overproduced, the toxicity expands, causing nerve cell damage and death, leading to long-term neurodegeneration. In recent decades, there have been many studies on neuroinflammation and some results have been achieved. But no better drugs or measures have been developed. In recent years, the potential neuroprotective effects of the main active components of herbal extracts in various neurological disorders have been extensively investigated.47,48 Numerous studies have shown that CN is one of the herbal medicines with anti-inflammatory, neuroprotective, antithrombotic, analgesic, and antioxidant activities.49,50 In this study, we screened the key active ingredients and targets of CN anti-NI by network pharmacology. Through literature search and ADMET screening, 26 compounds were confirmed to have good blood–brain barrier permeability and intestinal absorption, which further provided a basis for the theoretical drug-like ability of the drugs. Then, the optimal 3D-QSAR pharmacophore model for predicting key active ingredients was developed, four highly active compounds were successfully screened, and the standard structural features shared by the active molecules in the treatment of neuroinflammation were further analyzed. Finally, we established a model of LPS-induced neuroinflammation in BV2 microglia to further explore the potential mechanism of action of CN anti-NI.
Combined with network pharmacology analysis, we revealed that CN acts on multiple targets through multiple signaling pathways, mainly IL-6, TNF, AKT1, and TP53, which may play a key role in the occurrence and development of neuroinflammation. Promoting inflammatory cytokines, the likes of IL-1β, TNF-α, and IL-6, have multiple functions in neurodegeneration and protection. It has been previously demonstrated that IL-6 and TNF-α are overexpressed in activated microglia and lead to neurodegeneration.51 Each of these acute phase response proteins is contributing to the progression as well as to the resolution of both acute and chronic inflammation.52 IL-6 is a polymorphic cytokine produced mainly by microglia and astrocytes in various brain regions. It protects the function of the central nervous system by promoting the survival and regeneration of neurons. Besides IL-6 secretion, astrocytes positively regulate microglia at multiple levels during inflammatory damage and recovery.53 AKT, also known as PKB (protein kinase B), is a serine/threonine kinase that comes in three subtypes. Different AKT subtypes have different effects on nerve cell inflammation, and AKT1 is the most widely expressed subtype in the brain.54 Studies have shown that Akt1 deficiency promotes the classical activation of macrophages and enhances phagocytosis.55 P53 has long been considered a key transcription factor.56 TP53 induced glycolysis and apoptosis regulator (TIGAR) is an important p53 target, it has a critical role in the balancing between glycolysis and redox.57 TIGAR is broadly located in neurons and has a critical role in the CNS. These discoveries are consistent with our results that IL-6, TNF, AKT1, and TPT3 are extremely vital and may be engaged in the CN anti-NI process.
To explain the functions and related pathways of 277 protein targets, further, GO enrichment analysis and KEGG pathway enrichment analysis were performed. The GO results showed that the target genes were mainly concentrated in membrane microregions and biological functions such as protein tyrosine kinase activity and monooxygenase activity. KEGG data showed that the MAPK signaling pathway is the most abundant number of genes among NI-related signaling pathways and is the most critical signaling pathway in NI treatment. IL-6, as a key node in the MAPK signaling pathway, is involved in the process of NI occurrence and development. MAPK signaling pathway is an important cell signaling pathway that plays an important role in mediating various processes such as cell proliferation, apoptosis, necrosis, and inflammation. MAPK signaling pathway consists of extracellular signal-regulated protein kinase (ERK), Jun-N-terminal Kinase (JNK), and P38. MAPK is considered to be an important signaling pathway in the inflammatory process mimicked by LPS. It has been shown that the p38MAPK protein is a key target of this signaling pathway.58 It has been shown that the p38MAPK receptor initiates the release of inflammatory cytokines (IL-6, TNF-α and NO), so inhibition of P38MAPK phosphorylation inhibits the release of inflammatory cytokines and other cytotoxic factors from microglia and reduces neuroinflammation.59 These findings are consistent with our view that the MAPK signaling pathway is the most important CN–NI signaling pathway and may be involved in the anti-NI mechanism of CN.
In addition, the best pharmacophore model for CN anti-NI could be found from the 3D-QSAR pharmacophore model results, which consisted of an aromatic ring group, two hydrophobic groups, and a hydrogen bond acceptor group. Meanwhile, the flavonoid component 5-hydroxy-3,7,4′-trimethoxyflavone (THF) was screened for its good anti-inflammatory activity. Therefore, we further analyzed the relationship between the two from the perspective of the structure–activity relationship. Studies have shown that the hydrophobic groups of flavonoids play an important role in the anti-inflammatory properties. In these compounds, hydrophobic substituents, such as amyl, alkyl chains, and oxygen-containing heterocyclic parts, usually enhance the anti-inflammatory activity of flavonoids.60 O-methylation is commonly used in the synthesis of plant and microbial secondary metabolites, by which the methyl group replaces the hydroxyl group of the receptor compound to increase the hydrophobicity of the latter molecule thereby enhancing the activity.61,62 Tewtrakul et al. investigated the inhibitory activity of compounds isolated from the rhizomes of Kaempferia parviflora Wall on nitric oxide (NO) production. The results showed that 5-hydroxy-3,7,3′,4′-tetramethoxy flavonoid expressed the highest NO inhibitory activity with an IC50 of 16.1 mM, followed by 5-hydroxy-7,4′-dimethoxyflavin (IC50 = 24.5 mM) and 5-hydroxy-3,1,7′-trimethoxy flavonoids (IC50 = 30.6 mM), while the other compounds exhibited moderate to weak inhibitory activity. In addition, the anti-inflammatory activity exhibited by flavonoids is partly related to their inherent antioxidant capacity.63 The scavenging ability of flavonoids is due to the presence of a double bond between carbon 2 and carbon 3 in the C ring of the flavonoid backbone. The C-ring 2 and 3 double bonds in the parent nucleus structure of natural flavonoids may be important in inhibiting microglia activation, and the strength of their inhibitory effect depends on the type of substitution of the molecule. Methylation of 3-hydroxyl groups also exhibits a higher inhibitory effect on NO production, e.g., rhamnolins < lzalpinin, ombuine < ayanin.64 These studies further support our prediction by the 3D-QSAR pharmacophore model.
Based on the 3D-QSAR results, we performed molecular docking to further validate the predicted results. The docking results showed that ent 3,4-seco-16-hydroxy-12,15-epoxy-4(18),8(17),12,14-labdatetraen-3-oic acid, nudiflflopenes C, 5-hydroxy-3,7,4′-trimethoxyflavone and nudiflflopenes M bound well to IL-6, respectively, with 5-hydroxy-3,7,4′-trimethoxyflavone having the highest docking score with IL-6, indicating the most stable binding. Based on the results of the above analysis, we constructed a model of LPS-induced neuroinflammation in BV2 microglia for in vitro experimental evaluation. Experimental validation showed that THF, an important active component of CN, effectively inhibited the inflammatory response of BV2 microglia, suppressed the expression of TNF-α and IL-6 mRNA, and shifted the phenotype of LPS-stimulated BV2 cells from M1 to M2 polarization, thereby treating neuroinflammation. However, the present study has several limitations according to the corresponding guidelines.65 First, we constructed pharmacological models based on common characteristics of the molecules to find possible lead molecules. However, screening for compounds with clear activity values remains our endeavor. Therefore, the study about searching more pharmacophore features by 26 active ligand small molecules is still imperfect and needs to be further developed. However, in future studies, we will construct a receptor structure-based pharmacological model (SBP) to maximize the use of known receptor structures and drug receptor information to effectively overcome the de-criticalization of existing drug design tools and accelerate the prediction and development of new drugs faster.
5. Conclusions
In conclusion, this study provides a systematic and intuitive overview of the possible molecular mechanisms and signaling pathways of how CN exerts its anti-NI effects. Meanwhile, a 3D-QSAR pharmacophore model based on molecular common features was constructed to predict 5-hydroxy-3,7,4′-trimethoxyflavone (THF) as a good candidate for the treatment of NI. Consistent with the prediction results, the experimental results also demonstrated that the prediction based on the network pharmacology approach and pharmacophore model was accurate and credible, and the relationship between them was further analyzed from the perspective of the structure–activity relationship.
Author contributions
Conceptualization and methodology, G. Y. and Y. L.; software and visualization, J. C. and Y. L.; validation, data curation, and formal analysis, G. Y. and Y. M.; investigation, Y. L., and Y. M.; writing—original draft preparation, G. Y. and Y. L.; supervision, project administration, and writing—review and editing, J. C. All authors have read and agreed to the published version of the manuscript.
Conflicts of interest
The authors declare no conflict of interest.
Acknowledgements
This study was conducted with the support of a research grant from Jiangzhong Pharmaceutical Co. Ltd.
References
- P. Liu, Y.-Y. Wang, Y. Sun and G.-P. Peng, Clin. Interventions Aging, 2022, 17, 665–674 CrossRef.
- Alzheimer's Association, Alzheimer's Dement., 2019, 15, 321–387 CrossRef.
- G. Pottiez, L. Yang, T. Stewart, N. Song, P. Aro, D. R. Galasko, J. F. Quinn, E. R. Peskind, M. Shi and J. Zhang, J. Proteome Res., 2017, 16, 1228–1238 CrossRef CAS.
- B. R. Wang, Z. Ou, X. H. Gu, C. S. Wei, J. Xu and J. Q. Shi, Int. J. Geriatr. Psychiatr., 2017, 32, e173–e179 CrossRef PubMed.
- L. Rizzi, I. Rossetand and M. Roriz-Cruz, Biomed Res. Int., 2014, 2014, 908915 Search PubMed.
- A. Videnovic, A. S. Lazar, R. A. Barker and S. Overeem, Nat. Rev. Neurol., 2014, 10, 683–693 CrossRef.
- L. M. Ittner and J. Götz, Nat. Rev. Neurosci., 2011, 12, 67–72 CrossRef PubMed.
- T. Wei, C. Chen, J. Hou, W. Xin and A. Mori, Biochim. Biophys. Acta, Mol. Cell Res., 2000, 1498, 72–79 CrossRef CAS.
- R.-R. Ji, A. Nackley, Y. Huh, N. Terrando and W. Maixner, Anesthesiology, 2018, 129, 343–366 CrossRef PubMed.
- J. K. Olson and S. D. Miller, J. Immunol., 2004, 173, 3916–3924 CrossRef CAS.
- M. L. Block and J.-S. Hong, Prog. Neurobiol., 2005, 76, 77–98 CrossRef CAS.
- R. J. Horvath, N. Nutile-McMenemy, M. S. Alkaitis and J. A. Deleo, J. Neurochem., 2008, 107, 557–569 CrossRef CAS PubMed.
- H. Y. Park, G.-Y. Kim and Y. H. Choi, Int. J. Mol. Med., 2012, 30, 204–210 CAS.
- K. W. Lee, S. Y. Jung, S. M. Choi and E. J. Yang, BMC Complementary Altern. Med., 2012, 12, 196 CrossRef CAS.
- J. Xu, C. Yuan, G. Wang, J. Luo, H. Ma, L. Xu, Y. Mu, Y. Li, N. P. Seeram, X. Huang and L. Li, J. Agric. Food Chem., 2018, 66, 3571–3580 Search PubMed.
- W. Y. Fu, X. Wang and N. Y. Ip, ACS Chem. Neurosci., 2019, 10, 872–879 CrossRef CAS PubMed.
- X. Jin, M. Liu, D. Zhang, X. Zhong, K. Du, P. Qian, W. Yao, H. Gao and M. Wei, CNS Neurosci. Ther., 2019, 25, 575–590 CrossRef CAS.
- S. Tabassum, H. R. Khalid, W. U. Haq, S. Aslam, A. Alharbi, M. Alshammari, M. S. Riaz Rajoka, M. Khurshid and U. A. Ashfaq, Processes, 2022, 10, 298 CrossRef CAS.
- L. Li, L. Zhang and C. Yang, Curr. Top. Med. Chem., 2016, 16, 537–548 CrossRef CAS PubMed.
- L. Dong, X. P. Zhang, M. S. Liu, Y. M. Li, J. H. Wang and Y. Wang, J. Asian Nat. Prod. Res., 2013, 15, 30–34 CrossRef CAS PubMed.
- Y. M. Li, Y. F. Yang, X. D. Kang, X. F. Li, Y. Z. Wu, J. P. Xiao, Y. Ye, J. Q. Yang, Y. Yang and H. Liu, Front. Pharmacol., 2022, 12, 806808 CrossRef PubMed.
- X. Sun, F. Liu, X. Yang, J. Wang, B. Dong, C. Xie, D.-Q. Jin, J. Zhang, D. Lee, Y. Ohizumi, J. Xu and Y. Guo, Phytochemistry, 2018, 149, 31–41 CrossRef CAS PubMed.
- Z. Zhai, X. Tao, M. M. Alami, S. Shu and X. Wang, Curr. Issues Mol. Biol., 2021, 43, 65–78 CrossRef PubMed.
- Z. Ding, F. Xu, Q. Sun, B. Li, N. Liang, J. Chen and S. Yu, J. Evidence-Based Complementary Altern. Med., 2021, 2126967 Search PubMed.
- Y. Qian, X. Sun, X. Wang, X. Yang, M. Fan, J. Zhong, Z. Pei and J. Guo, J. Diabetes Res., 2021, 5477941 CAS.
- S. Y. Yang, Drug Discovery Today, 2010, 15, 444–450 CrossRef CAS PubMed.
- L. Pinzi and G. Rastelli, Int. J. Mol. Sci., 2019, 20, 4331 CrossRef CAS PubMed.
- S. Kim, P. A. Thiessen, E. E. Bolton, J. Chen, G. Fu, A. Gindulyte, L. Han, J. He, S. He, B. A. Shoemaker, J. Wang, B. Yu, J. Zhang and S. H. Bryant, Nucleic Acids Res., 2016, 44, D1202–D1213 CrossRef CAS PubMed.
- D. A. Evans, Angew. Chem., Int. Ed. Engl., 2014, 53, 11140–11145 CrossRef CAS PubMed.
- X. Wang, Y. Shen, S. Wang, S. Li, W. Zhang, X. Liu, L. Lai, J. Pei and H. Li, Nucleic Acids Res., 2017, 45, W356–W360 CrossRef CAS PubMed.
- C. The UniProt, Nucleic Acids Res., 2021, 49, D480–D489 CrossRef PubMed.
- D. Gfeller, A. Grosdidier, M. Wirth, A. Daina, O. Michielin and V. Zoete, Nucleic Acids Res., 2014, 42, W32–W38 CrossRef CAS.
- M. Rebhan, V. Chalifa-Caspi, J. Prilusky and D. Lancet, Trends Genet., 1997, 13, 163 CrossRef CAS.
- J. S. Amberger, C. A. Bocchini, F. Schiettecatte, A. F. Scott and A. Hamosh, Nucleic Acids Res., 2015, 43, D789–D798 CrossRef.
- D. S. Wishart, Y. D. Feunang, A. C. Guo, E. J. Lo, A. Marcu, J. R. Grant, T. Sajed, D. Johnson, C. Li, Z. Sayeeda, N. Assempour, I. Iynkkaran, Y. Liu, A. Maciejewski, N. Gale, A. Wilson, L. Chin, R. Cummings, D. Le, A. Pon, C. Knox and M. Wilson, Nucleic Acids Res., 2018, 46, D1074–d1082 CrossRef CAS PubMed.
- L. Sun, S. Dong, Y. Ge, J. P. Fonseca, Z. T. Robinson, K. S. Mysore and P. Mehta, Front. Genet., 2019, 10, 421 CrossRef CAS PubMed.
- D. Szklarczyk, A. L. Gable, D. Lyon, A. Junge, S. Wyder, J. Huerta-Cepas, M. Simonovic, N. T. Doncheva, J. H. Morris, P. Bork, L. J. Jensen and C. V. Mering, Nucleic Acids Res., 2019, 47, D607–d613 CrossRef CAS.
- Y. Tang, M. Li, J. Wang, Y. Pan and F.-X. Wu, Biosystems, 2015, 127, 67–72 CrossRef CAS PubMed.
- M. Kohl, S. Wiese and B. Warscheid, Methods Mol. Biol., 2011, 696, 291–303 CrossRef CAS.
- S. Kaur, Y. Bansal, R. Kumar and G. Bansal, Bioorg. Med. Chem., 2020, 28, 115327 CrossRef CAS.
- M. Kim, G. Kim, M. Kang, D. Ko, Y. Nam, C. S. Moon, H. M. Kang, J. S. Shin, O. Werz, K. T. Lee and J. Y. Lee, Bioorg. Med. Chem. Lett., 2021, 41, 127992 CrossRef CAS.
- S. Feng, T. Wang, L.-m. Fan, X.-x. An, X.-n. Ding, M.-j. Wang, X.-f. Zhai, Y.-j. Cao, J. He and Y. Li, RSC Adv., 2022, 12, 2181–2195 RSC.
- H. S. Kwon and H. Koh, Transl. Neurodegener., 2020, 9, 42 CrossRef.
- S. E. Park, K. Sapkota, S. Kim, H. Kim and S. J. Kim, Br. J. Pharmacol., 2011, 164, 1008–1025 CrossRef CAS PubMed.
- C. S. Subhramanyam, C. Wang, Q. Hu and S. T. Dheen, Semin. Cell Dev. Biol., 2019, 94, 112–120 CrossRef CAS PubMed.
- X. Wei, K. S. Cho, E. F. Thee, M. J. Jager and D. F. Chen, J. Neurosci. Res., 2019, 97, 70–76 CrossRef CAS.
- O. A. Olajide and S. D. Sarker, Inflammopharmacology, 2020, 28, 1439–1455 CrossRef.
- J. Zhang, Y. Zheng, Y. Luo, Y. Du, X. Zhang and J. Fu, Mol. Immunol., 2019, 116, 29–37 CrossRef CAS PubMed.
- K. A. Koo, S. H. Kim, T. H. Oh and Y. C. Kim, Life Sci., 2006, 79, 709–716 CrossRef CAS.
- Y. Tu, L. Sun, M. Guo and W. Chen, J. Ethnopharmacol., 2013, 146, 465–481 CrossRef CAS PubMed.
- M. Guo, J. Jin, D. Zhao, Z. Rong, L.-Q. Cao, A.-H. Li, X.-Y. Sun, L.-Y. Jia, Y.-D. Wang, L. Huang, Y.-H. Li, Z.-J. He, L. Li, R.-K. Ma, Y.-F. Lv, K.-K. Shao and H.-L. Cao, Front. Oncol., 2022, 12, 866154 CrossRef PubMed.
- M. N. Catorce and G. Gevorkian, Curr. Neuropharmacol., 2016, 14, 155–164 CrossRef CAS.
- L. Sun, Y. Li, X. Jia, Q. Wang, Y. Li, M. Hu, L. Tian, J. Yang, W. Xing, W. Zhang, J. Wang, H. Xu, L. Wang, D. Zhang and H. Ren, Oncotarget, 2017, 8, 40065–40078 CrossRef.
- H.-X. Xiao, B. Song, Q. Li, Y.-M. Shao, Y.-B. Zhang, X.-L. Chang and Z.-J. Zhou, Toxicol. Lett., 2022, 355, 116–126 CrossRef CAS PubMed.
- A. Arranz, C. Doxaki, E. Vergadi, Y. Martinez de la Torre, K. Vaporidi, E. D. Lagoudaki, E. Ieronymaki, A. Androulidaki, M. Venihaki, A. N. Margioris, E. N. Stathopoulos, P. N. Tsichlis and C. Tsatsanis, Proc. Natl. Acad. Sci. U. S. A., 2012, 109, 9517–9522 CrossRef CAS PubMed.
- W. Hu, S. Chen, R. F. Thorne and M. Wu, Adv. Exp. Med. Biol., 2019, 1206, 127–149 CrossRef CAS.
- S.-s. Huang, Y.-c. Sheng, Y.-y. Jiang, N. Liu, M.-m. Lin, J.-c. Wu, Z.-q. Liang, Z.-h. Qin and Y. Wang, Neurochem. Int., 2022, 152, 105244 CrossRef CAS.
- H.-J. Shim, S.-w. Park, J.-W. Lee, H.-J. Park, S.-H. Baek, E.-K. Kim and S.-W. Yu, Am. J. Chin. Med., 2016, 44, 119–132 CrossRef PubMed.
- C.-S. Yoon, D.-C. Kim, D.-S. Lee, K.-S. Kim, W.-m. Ko, J.-H. Sohn, J.-H. Yim, Y.-C. Kim and H.-c. Oh, Int. Immunopharmacol., 2014, 23, 568–574 CrossRef CAS.
- L. Chen, H. Teng, Z. Xie, H. Cao, W. S. Cheang, K. Skalicka-Woniak, M. I. Georgiev and J. Xiao, Crit. Rev. Food Sci. Nutr., 2018, 58, 513–527 CrossRef CAS.
- B. G. Kim, B. R. Jung, Y. Lee, H. G. Hur, Y. Lim and J. H. Ahn, J. Agric. Food Chem., 2006, 54, 823–828 CrossRef CAS PubMed.
- S. H. Kim, C. D. Jun, K. Suk, B. J. Choi, H. Lim, S. Park, S. H. Lee, H. Y. Shin, D. K. Kim and T. Y. Shin, Toxicol. Sci., 2006, 91, 123–131 CrossRef CAS.
- S. Tewtrakul, S. Subhadhirasakul, C. Karalai, C. Ponglimanont and S. Cheenpracha, Food Chem., 2009, 115, 534–538 CrossRef CAS.
- M. A. Soobrattee, V. S. Neergheen, A. Luximon-Ramma, O. I. Aruoma and T. Bahorun, Mutat. Res., 2005, 579, 200–213 CrossRef CAS PubMed.
- S. Li, World J. Tradit. Chin. Med., 2021, 7, 165–166 Search PubMed.
|
This journal is © The Royal Society of Chemistry 2022 |