DOI:
10.1039/D2RA04794B
(Paper)
RSC Adv., 2022,
12, 27421-27430
Developing a DNA logic gate nanosensing platform for the detection of acetamiprid
Received
1st August 2022
, Accepted 13th September 2022
First published on 27th September 2022
Abstract
This paper reports a novel fluorescence and colorimetric dual-signal-output DNA aptamer based sensor for the detection of acetamiprid residue. Acetamiprid is a new systemic broad-spectrum insecticide with high insecticidal efficiency that is widely used worldwide, but there is a risk of adverse neurological reactions in humans and animals. The dual-mode output principle designed in this paper, consisting of a fluorescence signal and colorimetric signal, is based on the relevant reaction of the special domain of a G-quadruplex, bidding farewell to a classical single-signal output, with a target-recognition cycle used to complete signal amplification through a hybridization chain reaction. Upgraded detection sensitivity and the qualitative and semi-quantitative detection of acetamiprid are achieved based on the fluorescence signal output and visual discrimination observations during colorimetric experiments. This model was applied to the determination of acetamiprid residue in fruits and vegetables. The dual-detection platform further reduced systematic error, with a detection limit of 27.7 pM. When applied in a comparative detection study using three different pesticides, the system shows excellent discrimination specificity and it performs well in actual sample detection and has a fast response time. Designing DNA logic gates that operate in the presence of targets and molecular-switch-based detection platforms also involves the intersection of biology and computational modeling, providing new ideas for biological platforms.
Introduction
Acetamiprid (Ace) is a representative new nicotine insecticide. It is often used to control herbivorous pests affecting fruits and vegetables, such as garlic, celery, tea, and strawberries. The control effect is more than 90%, it is compatible with the environment, and the shelf life is about 20 days.1 Acetamiprid has biologically toxic effects on the human body;2–4 it can enter food and human tissue during daily routines, stimulate neurotoxicity caused by acetylcholine receptors, penetrate human peripheral blood lymphocytes, and cause DNA damage.5,6 Acetamiprid acts as an agonist of nicotinic acetylcholine receptors on neuronal postsynaptic membranes and it inhibits normal conduction in the central nervous system.7,8 In addition to acute toxicity, acetamiprid has potential long-term and chronic toxic effects, including developmental neurotoxicity, genotoxicity, and other toxicities.9 Therefore, there is an urgent need to design an efficient, fast, and inexpensive detection method. For acetamiprid, common official methods for its determination are high-performance liquid chromatography (HPLC),10 liquid chromatography-mass spectrometry (LC-MS),11,12 high-performance liquid chromatography (HPLC)-UV,13 and gas chromatography-mass spectrometry (GC-MS).14,15 It is well known that although these methods can achieve high sensitivities and low detection limits, they all have limitations, such as cumbersome pretreatment methods, long detection times, and the need for expensive instruments, time-consuming sample preparation methods, specialized equipment, and experienced laboratory personnel. In addition, electrochemical immunoassay and enzyme-linked immunosorbent assay (ELISA)16 antibodies have short shelf lives, are susceptible to interference, and are very expensive and time-consuming to produce, severely limiting their daily application.17,18 Therefore, there is an urgent need to develop a reliable, effective, and rapid method for the detection of aminopyralid in the environment and agricultural products19 to ensure food safety for human health. To improve the above-mentioned conventional assays, we have constructed a biosensor to achieve assay optimization, substantially reduce assay costs, and improve the ease of operation. The biosensor utilizes the high affinity of the target and aptamer using the systematic evolution of ligands by exponential enrichment (SELEX) method20 to screen ligands with high affinity and specificity from a random oligonucleotide library synthesized in vitro. The aptamer for the target pesticide acetamiprid in this study was selected using the SELEX method.21,22 Via a hybridization chain reaction (HCR),23 signal amplification of the biosensor is achieved; this is a process that does not require the involvement of proteases or DNA enzymes, and only a strand-hybridization process occurs. The reaction cannot occur at room temperature due to short-loop interference, and it requires primers to activate a stable strand for hybridization. In a HCR, once the target DNA is inserted, two synthetic DNA hairpins coexist in solution and hybridize into continuous linear DNA. HCR has distinct advantages, such as high amplification rates,24 controlled kinetics, and non-enzymatic properties.25 An enzyme-free labeling technique is preferred in this sensor because it avoids the expensive and complex process of fluorescent labeling and the side-reactions generated during labeling. We used an enzyme-free labeling technique in our study, and special DNA structures (G-quadruplexes) were introduced using the HCR principle.26,27
A G-quadruplex is a specialized DNA structure consisting of a sequence of guanine-rich nucleic acids that has received significant attention due to growing evidence for its role in important biological processes and as a therapeutic target.28–31 A G-quadruplex is formed via the repetitive folding of a single polynucleotide molecule or of two or four molecules. The structure consists of stacked G-quadruplexes, which are rectangular coplanar arrays with four guanine bases each.27 Metalloporphyrins are widely found in nature, and they are used by organisms as cofactors for various enzymes and other specific proteins. Metalloporphyrins are versatile and they can be involved in oxygen transfer, electron transfer, and various redox chemical reactions, such as those related to catalase, peroxidase, and monooxygenase. N-Methylmethylporphyrin IX (NMM) is a water-soluble asymmetric porphyrin with excellent optical properties and it is sensitive to G-quadruplexes; it has been studied in relation to structural interactions,32–34 and NMM is particularly selective for G-quadruplexes compared to double-stranded DNA, and it can be used for fluorescence signal output in the presence of ions, with exponentially increased output signal results35,36. It has a wide range of applications in biology and chemistry and, therefore, NMM is known as a ligand for G-quadruplexes. The detection of the fluorescence signal in this study was done via exciting the sensor system in the presence of the target to produce G-quadruplexes, which led to a fluorescence signal change upon interaction with NMM.
Colorimetric aptamer sensors are favored by researchers due to the remarkable advantages of easy operation, low cost, and easy observation with the naked eye.37 Aptamers can modulate the catalytic performance of DNase, thereby allowing the visual detection of pesticide residues.38 3,3′,5,5′-Tetramethylbenzidine (TMB) is a substrate of a DNA enzyme catalytic system consisting of a guanine tetrameric DNA molecule and hemin,39–41 involving a class of catalytically active nucleic acid, a commonly studied deoxyribonuclease. The deoxyribonuclease consists of hemin and a guanine (G) tetraspanin DNA sequence bound to hemin. Hemin itself is a potent cofactor, and G-quadruplexes with low peroxidase-like activity can bind to hemin and greatly enhance the hemin activity42. During the oxidation of hydrogen peroxide by the G-quadruplex–hemin complex, TMB can act as a hydrogen donor along with a peroxidase, such as horseradish peroxidase, to reduce it to water. The oxidation of TMB was shown to give a blue color to the solution due to the oxidation of TMB to 3,3′,5,5′-tetramethylbenzidine diimine.43
The design of DNA logic gates has allowed the outstanding use of DNA Boolean logic gates in the field of biosensors.44 This has shown great potential in the area of biosensing because logic analysis can be performed on multiple targets at the same time. The pattern change of a sensor biosignal is very similar to the input and output of a logic-based system.45 In this paper, we design “AND” gates and “OR” gates based on the input and output of different detection signals when the target is input into the logic system. DNA logic gates are promising multi-input analysis tools, laying a foundation for intelligent diagnosis, and new ideas will be provided for the development of traditional logic gates.
An enzyme-free label-free biosensor was designed to achieve the detection of acetamiprid residue, and we used the fluorescent signal of G-quadruplexes/NMM, which was further amplified using a HCR. More importantly, we performed a series of computer simulations prior to the bioassay to simplify the subsequent experimental steps and eliminate some negative effects. In the presence of aminopyralid, two designed DNA hairpins are triggered by the reaction of the trigger strand S, which sequentially opens H1 and H2 and self-assembles into an embedded G-quadruplex, thus significantly enhancing the fluorescence signal in the presence of NMM; also, color analysis can be accomplished based on the reaction of the G-quadruplex with TMB, leading to color development, and both approaches lead to a signal output after the experiments are completed. Our method is sensitive, rapid, and selective, and it has practical application in detection processes, where it may open new avenues for the application of biosensors in food control and quality testing. Via analyzing the signals of biological gates, basic logical “OR” and “AND” gates are constructed to convert the input and output into logical signals, and the conversion of biological signals to digital information is realized.
Materials and methods
Reagents
Acetamiprid (Ace), chlorpyrifos (Chl), and imidacloprid (Imi) used in these experiments were purchased from Shanghai Yuanye Biological Co., Ltd, and multiple oligonucleotide sequences (aptamer, S, H1–H2) were purified via HPLC by Shanghai Sangon Bioengineering Co., Ltd and purchased. N-Methyl mesoporphyrin IX, NMM, was purchased from Beijing Bailingwei Technology Co., Ltd. Tris–HCl buffer (NaCl, MgCl), centrifuge tubes, consumables, and potassium chloride (KCl) were purchased from Xi'an Jingbo Biotechnology Co., Ltd. TMB chromogenic solution (3,3′,5,5′-tetramethylbenzidine) and hemin were purchased from Beijing Soleibao Technology Co., Ltd.
Instrumentation
The fluorescence spectra of NMM were measured using a fluorescence scanning spectrometer with an excitation wavelength of 399 nm and an emission wavelength of 610 nm using a BioTek H1 multifunctional microplate reader from Berton Instruments, Inc., USA. TMB absorbance was measured with an OD scanning spectrometer emitting at 650 nm using a BioTek FX multi-function microwave detector from BioTek FX Multi-function Microplate Detector, USA.
Experimental operation detection
Before experiments, a diluted solution containing H1 (1 μM) and H2 (1 μM) was heated at 95 °C for 10 min for a PCR reaction, and then slowly cooled to room temperature to form a hairpin-like structure. Also, Apt (1 μM) and S (1 μM) were mixed to form a double-stranded structure. Then, different concentrations of acetamiprid and Apt-S (100 nM) buffer were mixed and incubated at 37 °C for 30 min, and then H1 (350 nM) and H2 (350 nM) were added to the above reaction solution for self-assembly, with incubation at 37 °C for 90 min. After the post-addition process was completed, one half of the solution was added to NMM (1.5 μM) and incubated at 25 °C for a further 20 min, and the other half was added to hemin (10 μL) and further incubated at 25 °C for 30 min. Finally, 50 μL of the product was added to 450 μL of TMB/H2O2 and mixed for 15 min. The two different solutions were transferred to two 96-well plates, and the fluorescence intensities were detected and recorded using the above-mentioned instrument.
Result and discussion
Principle of the detection mechanism
The biosensing detection response is based on signal recognition in the system first. When the target acetamiprid does not appear in the system, the single strand of the aptamer will be paired with the complementary base of the trigger strand, and the trigger strand cannot be released into the reaction system (Fig. 1). There will be no follow-up effects. However, the appearance of the target molecule acetamiprid will lead to spatial coupling with the special structure of the aptamer, a single chain of the aptamer will be pulled out from the double-stranded structure in the form of the trigger chain S (green), and the trigger chain will be released into the reaction system. When the trigger strand S is added to the H1/H2 cycle, it first searches for a foothold (green segment) for complementary base pairing at the 5′ end of the hairpin H1, and it gradually displaces the “stem” of the initial H1 hairpin during the pairing process. Complementarily, in this process, the original 3′ end foothold of the H1 hairpin is opened to free exposure (orange fragment), which will remove the original H1 “signal”, and the special design of the end segment sequence will be the 5′ end of the H2 chain. The segment sequence is opened to complete the dynamic opening of the two hairpins. When the H1–H2 double chain is formed, the original partially paired trigger chain S is replaced, so that the S chain returns to the reaction system to stimulate a new round of hairpin formation. Clip opening and closing was based on this. Since guanine-rich bases were present by design in the “stem” of the hairpin at the beginning of the process, H1 was not activated alone in the reaction system, and G-quadruplexes could not be formed when H2 was turned on, unless both hairpins were turned on. At the same time, the base structures at the ends of the two strands can form a double-stranded structure with G-quadruplexes (red) at both ends. Two types of experimental data, fluorometric and absorbance, were measured using a multi-function microwave detector. The fluorescence data from the G-quadruplexes in the experimental reactions were measured at excitation and emission wavelengths of 399 and 610 nm and used for analysis; the absorbance of TMB/H2O2 oxidised with haemoglobin was measured with an OD scanning spectrometer at 650 nm via absorbance experiments, and a visual qualitative judgement can be made based on a colour reaction and the absorbance data (Table 1).
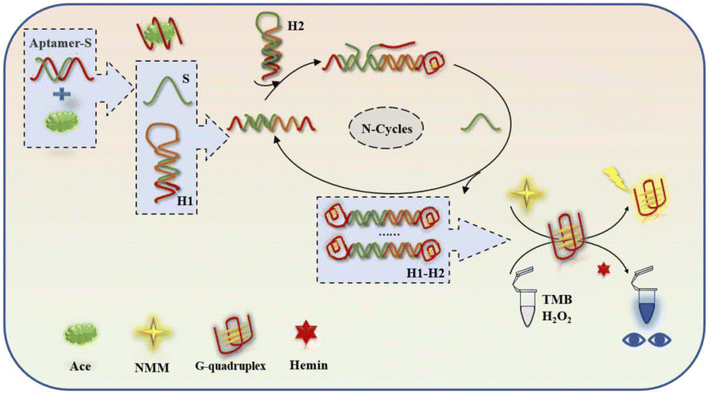 |
| Fig. 1 A cyclic dual-signal biosensing device based on G-quadruplexes for the detection of acetamiprid. | |
Table 1 Nucleic acid sequences required for sensors
Name |
Sequence (5′-3′) |
Apt |
CTG ACA CCA TAT TAT GAA GA |
S |
AGTCACACGTCTTGATAATAT G |
H1 |
GGGCCATCAAGACGTGTGACTCGGTAGTGACACCTCTTGACGGGC GGGTAGGG |
H2 |
GGGATCAAGACGTGTCACTACCGAGTGACACGTCTTGATGGGGGCGGGTAGGG |
Sequence design and analysis
All sequences used in the experiments are designed, and the designed hairpin DNA is preliminarily predicted via NUPACK software thermodynamic simulations based on thermodynamic and concentration simulations. As can be seen from the data in Fig. 2 and 3, we used hairpin structures and reaction conditions that may exist in a solution system to simulate concentrations and thermodynamics for preliminary analysis regarding viability. Both are simulated in an environment at 37 °C. The free energy of the secondary structure of the hairpin H1 is −10.58 kcal mol−1 and the free energy of the secondary structure of the hairpin H2 is −18.69 kcal mol−1. The hairpin DNA structures are relatively stable at 37 °C, but as the design relies on a difference for the reaction sequence, we set the stability of H2 to be greater than that of H1, because H1 as an intermediate product needs to be combined with the shorter trigger chain S and open, while H2 needs to be activated only after H1 is fully opened. If it is not stable, the hairpin may open out of sequence, leading to the possibility of increased background fluorescence.
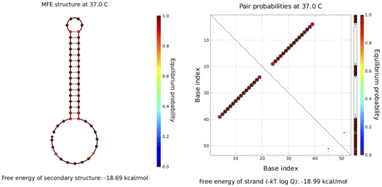 |
| Fig. 2 The simulated structure diagram (left) and secondary structure thermodynamic free energy plot (right) of the hairpin probe H1. | |
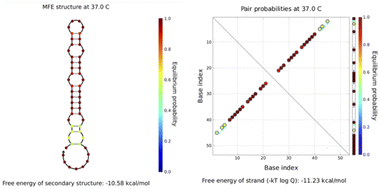 |
| Fig. 3 The simulated structure diagram (left) and secondary structure thermodynamic free energy plot (right) of hairpin probe H2. | |
Putting H1–H2 (Fig. 4) into the simulated environment at the same time, the secondary structure has a free energy of −43.58 kcal mol−1 and very stable hairpin binding; this assists in triggering the elimination of the chain S by the hairpin after it triggers the reaction, and S reenters the cycle to continue the cycle. These computer simulation results provide data to support the empirical feasibility of this method.
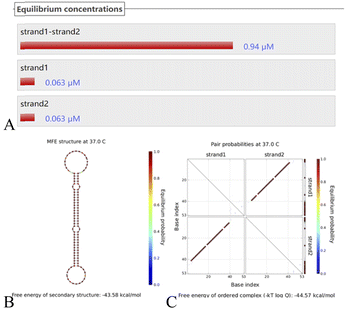 |
| Fig. 4 (A) The H1–H2 equilibrium concentration. (B) The structure diagram of the H1–H2 hairpin probe and (C) the secondary structure thermodynamic free energy plot. | |
DNA logic gate construction
A biologic gate is an intelligent probe capable of responding to biological conditions with behavior similar to that of a computer logic gate, where the input information can be relevant information relating to physical or biological characteristics, such as the response to laser or ultrasonic illumination or structural changes caused by changes in biological properties. The logic gate output can be classified as the release of cargo, for example, the release of a secured or inactive drug, a measurable change in state, a fluorescent signal, or nanoparticle aggregation. Because biological conditions involve many parameters, leading to many possible inputs and desired outputs, any logic gate development needs to first identify the target conditions or target information to be evaluated based on logic manipulation. Therefore, although biologic gates use logic operations similar to those performed at the molecular level in terms of computer behavior, the input and output signals cannot be simply defined as conversions of electrical signals, as in computer logic, and the designer needs to indicate in advance the type of input and the ascribed Boolean value of the output signal. Fluorescence or absorbance signals are used in this paper as outputs to construct biologic gates. In logical binary operations, 1 and 0 are the optimal values to enable logic gates to adapt themselves to different application requirements. In logical binary operations, the input is encoded using 1 and 0, and 1 and 0 are assigned to the presence and absence of the target, respectively. On the output side, 1 is a high signal and 0 is a low signal.
In the design in this paper, the universal platform is based dually on the fluorescent-based binding of G-quadruplexes and NMM and a colorimetric interaction involving G-quadruplexes and TMB/H2O2. To achieve the objective of detecting aminopyralid via a dual “AND” and “OR” fluorescence/colorimetric detection logic platform, the main design used the presence of the specific target, DNA probe, and NMM/TMB as input signals to the logic gates and the detection results as output signals. The principle of the constructed dual “AND” biologic gate is shown in Fig. 5. The input signal (input) of the logic platform is divided into three components: acetamiprid, Apt-S, H1/H2, and NMM/hemin; the output signal (output) is based on the fluorescence intensity or TMB colorimetric output, with “0”/“1” as the signal result. The specifically designed aptamer is the basic building element of the logic gate platform, and the logic structure output is based on the presence of the specifically designed aptamer. When the signal from acetamiprid is missing in the system (input 1 is “0”), there is no obvious fluorescence signal. When Apt-S, H1, or H2 is missing (input 2 is “0”) or when NMM/hemin is missing (input 3 is “0”), then the signal output is “0”. When acetamiprid, Apt-S, H1, H2, and NMM/hemin enter the logical platform at the same time (input 1, input 2, and input 3 are “1”), a significant fluorescence/colorimetric signal is expressed, and the output “1”.
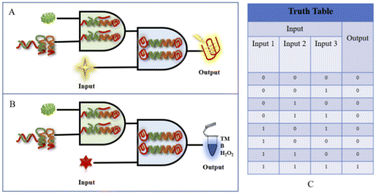 |
| Fig. 5 (A) A schematic diagram of the fluorescence-based “AND” logic gate. (B) A schematic diagram of the absorbance-based colorimetric “AND” logic gate. (C) The corresponding truth table. | |
The goal of the fluorescence/colorimetric detection of acetamiprid is achieved through the “OR” logic platform, which is mainly designed for a specific signal molecule, and the “OR” gate principle is shown in Fig. 6. The input signal (input) of the logic platform is divided into two components: NMM and hemin; the output is based on the fluorescence intensity/TMB colorimetric response, with “0” and “1” as possible signal results. The specifically designed aptamer is the basic building block of the logic gate platform, and the output of the logic structure is based on the presence of the specifically designed aptamer. When hemin is missing in the system but NMM is present (input 1 is “1” and input 2 is “0”), then a distinct fluorescence signal appears in the system, and the output fluorescence signal is “1”. When NMM is missing but hemin is present (input 1 is “0”, input 2 is “1”), the output colorimetric signal is “1”. When NMM and hemin are both missing (input 1 and input 2 are both “0”), the output of the dual-signal platform is “0”. When both NMM and hemin enter the logical platform (input 1 and input 2 are both “1”), significant fluorescence and colorimetric signals are expressed, and the output is “1”.
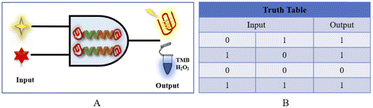 |
| Fig. 6 (A) A schematic diagram of the “OR” logic gate sensor. (B) The corresponding truth table for the logic gate. | |
Biological experiments to verify its feasibility
Based on fluorescence intensity scans at 610 nm, it is not difficult to see the differentiating characteristics of the five obtained sets of data (Fig. 7). When only the hairpin structure H1 or H2 appears in the reaction system (Fig. 7, purple and green curves, respectively), it is not difficult to see that the fluorescence output is not high, indicating that the hairpin structure in the system is stable and there is no obvious self-formation of G-quadruplexes. When the two kinds of hairpin, H1 and H2, and the Apt-S double-stranded structure are present in the reaction system, the fluorescence signal intensity shows a small increase; this is because when H1 and H2 coexist, this will inevitably occur due to weak interactions resulting in a small amount of H1/H2 double-stranded structure containing G-quadruplexes at both ends due to hybridization, possibly because of the stability of the hairpin structure in the early stage and the reaction conditions. In simulations, there are few occurrences of this, and it will not affect the feasibility of the experiment too much. The principle of using Apt-S, designed in this experiment, is that in the presence of the target acetamiprid, through spatial coupling between the target and the special structure of the aptamer, the trigger chain S is released, and a subsequent cycle involving H1 and H2 can be completed. In the experiment, the target trigger chain S is directly introduced into the reaction system, which can directly let the trigger chain S complete the above experimental process, without the need for the spatial coupling reaction between the target and the aptamer trigger chain Apt-S, and proceed with the sequence. The fluorescence intensity signal obtained is shown in the pink curve in Fig. 7, and the strong fluorescence intensity clearly indicates that the trigger chain S is capable of completing the experimental path and accurately completing the two-step process of opening the hairpin to form a G-quadruplex structure; this allows the catalytic hairpin self-assembly process to be achieved. When the target acetamiprid enters the detection system, the trigger chain S should be released through spatial coupling between the target and the aptamer. The experiments show that the trigger chain S can indeed be released through target recognition, and the fluorescence intensity data indicates the feasibility of the reaction to detect the target acetamiprid.
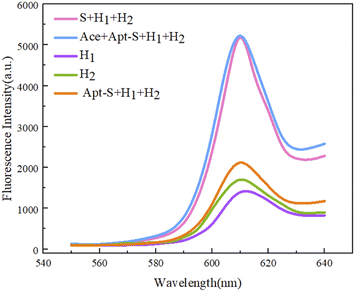 |
| Fig. 7 Assessing the feasibility of a sensor based on dual hairpin cyclic amplification via visualizing the fluorescence output. | |
In addition to the fluorescence signal intensity output, we also used the chromogenic reaction due to the binding of the G-quadruplex and hemin as a qualitative detection method for acetamiprid visualization with the naked eye (Fig. 8). Depending on differences in the DNase activities at different concentrations in the designed experimental test tubes, the colors of samples are different, and the presence of the target acetamiprid can be visualized (blue color). We designed 7 sets of experimental test samples, labeled 1–7. No. 1 was an experimental control sample; No. 2 was the Apt-S double-stranded structure; No. 3 contained Apt-S and the target acetamiprid; No. 4 contained hairpins H1 and H2; No. 5 contained the Apt-S double-stranded structure and hairpins H1 and H2; No. 6 contained the Apt-S double-stranded structure, hairpins H1 and H2, and acetamiprid (50 nM); and No. 7 contained the Apt-S double-stranded structure, hairpins H1 and H2, and acetamiprid (100 nM). The absorbance intensity data indicated the feasibility of this method for the detection of acetamiprid.
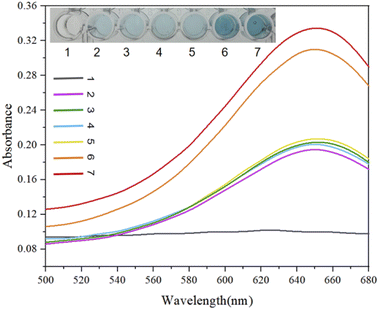 |
| Fig. 8 Feasibility analysis and colorimetric visualization for light-absorption-based detection. No. 1: blank; No. 2: Apt-S; No. 3: Apt-S and acetamiprid; No. 4: H1 and H2; No. 5: Apt-S and hairpins H1 and H2; No. 6: Apt-S, H1 and H2, and acetamiprid (50 nM); and No. 7: Apt-S, H1 and H2, and acetamiprid (100 nM). | |
Condition optimization
The reaction was first optimized for the K+ concentration, based on the use of ions to allow the embedded space of the G-quadruplex to provide an ionic basis for experimental NMM staining; as shown in Fig. 9(A), with an increase in the ion amount, there is a general trend for the fluorescence output to be enhanced. This is based on the F–F0 fluorescence signal output response data, where the SD is obtained based on three repeated experiments. Finally, an ion concentration of 25 mM was used for experimental detection.
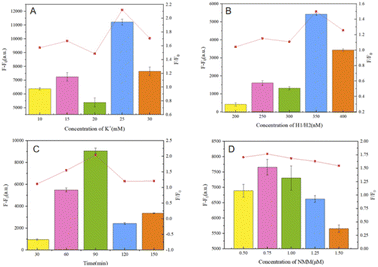 |
| Fig. 9 (A) The effects of the K+ concentration on the fluorescence response of the system. (B) The effects of the H1 and H2 hairpin concentrations on the fluorescence response of the system. (C) The effects of the hairpin reaction binding time on the fluorescence response of the system. (D) The effects of the NMM concentration on the fluorescence response of the system. Error bars: SD; n = 3. | |
Subsequently, condition optimization was carried out relating to the concentrations of the hairpins (H1, H2), as shown in Fig. 9(B). The hairpins are the core of the cyclic amplification model for biosensing. In the cascade reaction, a concentration that is too low will affect the opening and assembly of the double hairpin structure, but a too-high concentration will produce a small probability of the weak opening of the double hairpin, which will enhance the background noise. Therefore, 350 nM is ultimately selected as the optimum concentration through experimental analysis.
In addition, the hairpin reaction time is also a parameter related to cyclic amplification that can affect the self-assembly efficiency, as shown in Fig. 9(C). When the time is too short, the trigger chain S cannot be fully released due to the need for the target and inadequate double chain reactions, resulting in an inability to ensure that hairpins H1 and H2 are triggered by the trigger chain S; when the time is too long, as shown by the ratio F/F0, the trigger efficiency decreases and the time cost increases, which is not conducive to comprehensive sensor performance. Therefore, a hairpin reaction time of 90 min is considered the optimal hairpin self-assembly time to complete biosensor detection experiments.
The concentration of NMM and the reaction time of NMM with the G-quadruplex structures have great influence on the fluorescence intensity. In these experiments, as shown in Fig. 9(D) and based on the results of F–F0 data analysis, an NMM concentration of 0.75 μM (1.5 μL) resulted in the highest fluorescence intensity value. A too-low concentration of NMM cannot provide sufficient fluorescence intensity from the G-quadruplexes generated during the reaction, and the higher the concentration of NMM, the higher the background fluorescence signal. Also, based on time-gradient detection, the reaction time of NMM is very important in the test itself. The effect is slight, so 15 min was ultimately adopted as the reaction time for NMM.
Sensitivity and specificity
Based on the optimal experimental conditions obtained from the above single-factor experiments, we evaluated the sensitivity of the sensor via changing the concentration of acetamiprid and further analyzed the detection performance. As shown in Fig. 10(A), from the purple curve to the red curve, the concentrations of acetamiprid samples to be tested were set to 0 nM, 5 nM, 10 nM, 15 nM, 20 nM, 25 nM, 30 nM, 50 nM, and 100 nM; increasing the concentration of acetamiprid resulted in a gradual increase in the fluorescence signal. Through further observation, it was found that the fluorescence response of NMM at 610 nm was linearly related to the logarithm of the acetamiprid concentration between 0 nM and 30 nM (Fig. 10(B)). The linear regression equation is expressed as y = 331.31x + 3543.1 (x and y are the acetamiprid concentration and fluorescence intensity, respectively), and the correlation coefficient R2 is 0.9858. In addition, the acetamiprid limit of detection (LOD) calculated according to the formula 3σ/S (σ is the standard deviation of the control solution and S is the slope of the linear regression equation) was 27.7 pM. When the acetamiprid concentration of the solution being tested is outside the linear range (i.e., 27.7 pM to 30 nM), it is sufficient to dilute the actual sample with buffer to a concentration range that satisfies the linearity conditions, and the target concentration of acetamiprid can be estimated quantitatively based on the dilution factor. SD was calculated based on three replicate experiments.
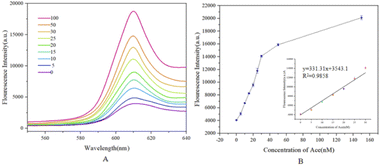 |
| Fig. 10 (A) Fluorescence spectra obtained upon detecting different concentrations of acetamiprid. The concentrations are 0, 5, 10, 15, 20, 25, 30, 50, and 100 nM. (B) A linear plot of acetamiprid concentration (from 0 to 30 nM) versus fluorescence intensity at 610 nm, with a detection limit of 27.7 pM. | |
The visual colorimetric method was then used to determine the sensitivity of the colorimetric sensor; different concentrations of acetamiprid were input, and the color change and biosensor detection performance were further analyzed. As shown in Fig. 11, from the purple curve to the red curve, the acetamiprid concentrations of the samples to be tested were 0 nM, 0.1 nM, 0.5 nM, 5 nM, 25 nM, 50 nM, 250 nM, and 500 nM. An increase in the acetamiprid concentration led to the enhancement of the absorbance signal. Color gradient analysis revealed that the color contrast of the biosensor was significant as the concentration of acetamiprid increased, indicating that colorimetric visualization detection could be achieved with the biosensor.
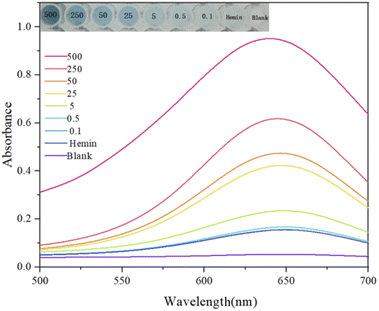 |
| Fig. 11 Absorbance spectra obtained via detecting different concentrations of acetamiprid from 0.1 to 500 nM. | |
The key to visualized absorbance experimental detection lies in naked eye identifiability, so the concentration range and gradient were chosen to be larger when selecting the test sample concentration. This is to facilitate the qualitative and semi-quantitative rapid detection of acetamiprid, which can be used as an auxiliary means in addition to accurate fluorescence detection. Fig. 12 shows the color changes for the chromogenic TMB reaction in response to different selected concentrations, and the target concentration can be roughly distinguished in this range based on the color change.
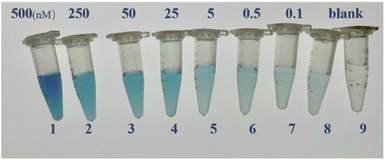 |
| Fig. 12 The colorimetric reaction of TMB for the detection of acetamiprid at different concentrations. (1) 500 nM; (2) 250 nM; (3) 50 nM; (4) 25 nM; (5) 5 nM; (6) 0.5 nM; (7) 0.1 nM; (8) hemin; and (9) blank. | |
To examine the effects of color vision bias of the naked human eye with respect to the TMB colorimetric response at different concentrations, as shown in Fig. 13, we selected five standard concentrations of acetamiprid samples for naked eye recognition by members of the research group who did not know the concentrations beforehand, and the possible color-based responses provided were: (A) 500 nM; (B) 250 nM; (C) 25 nM; (D) 0.5 nM; and (E) 0 nM. These researchers could divide the acetamiprid samples into five color gradients, and the experimenter could judge which concentration a sample solution is closest to using the naked eye and without using absorbance testing based on an enzyme standard; this can allow the semi-quantitative detection of acetamiprid through visualization. This method does not need to use an instrument to distinguish the different concentration gradients, it is easy and fast to carry out, and it can be used as an auxiliary detection method.
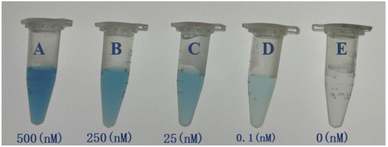 |
| Fig. 13 A visual comparison chart based on detection solutions with five standard concentrations. (A) 500 nM; (B) 250 nM; (C) 25 nM; (D) 0.1 nM; (E) 0 nM. | |
To verify the specificity of the established sensor for acetamiprid (Ace), the sensor was also tested in the presence of other comparable novel pesticides, chlorpyrifos (Cla) and imidacloprid (Imi), under the same conditions. Imidacloprid and chlorpyrifos, which are structurally comparable to acetamiprid (Fig. 14), may cause severe interference.46
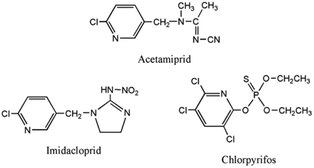 |
| Fig. 14 The structures of the pesticides used for specificity analysis. | |
We experimentally developed five sets of samples for assays. As shown in Fig. 15, the concentrations of Cla (2) and Imi (3) in experiments were 100 nM and the concentration of acetamiprid (1) was 10 nM. It is evident from the fluorescence measurements that even if other insecticides were present at concentrations 10 times higher than that of acetamiprid, only small changes in the fluorescence intensity were observed, and the intensities were not substantially different from the blank control (5) group. The presence of acetamiprid induced a significant increase in fluorescence intensity. In addition, the fluorescence signal from a mixture of acetamiprid and the other insecticides (4) was comparable to that of the acetamiprid group. The results of all the above experiments implied that the specificity of the sensing method for acetamiprid detection was substantial.
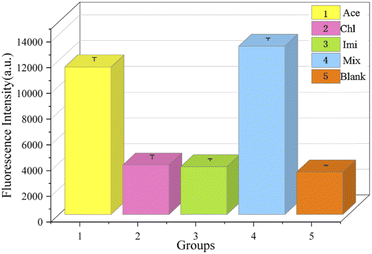 |
| Fig. 15 A comparison of the fluorescence responses between control samples and experimental targets to analyse specificity. (1) Acetamiprid; (2) chlorpyrifos; (3) imidacloprid; (4) acetamiprid, chlorpyrifos, and imidacloprid; (5) blank. | |
Application in practical samples
To ensure the application of the sensor in actual samples, we selected crops commonly exposed to acetamiprid, and cucumber and cabbage were used for testing the recovery rate of the biosensor platform, as shown in Table 2. The recovery is the ratio of the result obtained upon adding a quantitative amount of standard to a sample matrix and analyzing it according to the sample processing steps to the amount of standard added; recovery is an indicator of the stability of a sensor. In this paper, we subjected samples of known concentrations of acetamiprid to recovery testing in our experiments, and in order to ensure experimental stability, we took an average of three measurements to calculate the final recovery rate.
Table 2 Real-world sample detection
Sample |
Added (nM) |
Found (nM) |
Recovery (%) |
RSD (%) |
Cucumber |
1 |
10 |
10.36 |
103.6 |
3.2 |
2 |
50 |
50.3 |
100.6 |
1.9 |
Cabbage |
1 |
10 |
9.87 |
98.7 |
0.89 |
2 |
50 |
50.12 |
100.24 |
2.72 |
Both of the above vegetables were purchased from local farmers' markets and were not otherwise processed. 1 g of cucumber or cabbage was placed in an ultrasonic crusher for 5 min in 10 mL of buffer, and the supernatant was extracted after centrifugation for 10 min. During the detection process, 10 nM or 50 nM of acetamiprid was added to the cucumber and cabbage samples, and the experimental results were measured. The recovery rates were between 98.7% and 103.6%, and the RSD (relative standard deviation) values were between 0.89% and 3.2%. The results show that the biosensor has good accuracy during sample detection.
This method has been compared with common methods used to detect acetamiprid published in the literature, as shown in Table 3. For comparison with the biosensor detection platform designed in this paper, the differences between detection limits were analyzed and detection sample types were compared. In this study, due to the design of the strand displacement cyclic amplification method via a double-hairpin self-assembly model, the target detection limit can be reduced about 6-fold and the detection range is wider than those for detection methods without cyclic amplification technology previously studied by researchers. This method has a higher degree of recognition for trace levels of acetamiprid and, compared with other traditional detection methods, this detection method has certain advantages. The test samples herein are most common fruits and vegetables, which are also the most likely sources of acetamiprid residue absorbed by the human body.
Table 3 A comparison with other detection methods
Detection method |
Sample |
Detection limit |
Reference |
Colorimetric sensing |
Soil |
0.5 nM |
47 |
LC-MS/MS |
Bovine liver |
0.2 mg kg−1 |
48 |
MIP-MSPD-LC-MS/MS |
Rice |
2.4 ng g−1 |
49 |
Based on apt-CQDs |
Serum and vegetables |
0.04 ng L−1 |
50 |
Aptamer-based fluorometric method |
Serum and water |
172 pM |
51 |
Electrochemical aptamer-based assay |
Water and serum |
153 pM |
52 |
FRET-based chemosensor |
Water samples |
0.02 μM |
53 |
Fluorescence |
Vegetables |
1.08 μg L−1 |
54 |
Fluorescence |
Vegetables |
27.7 pM |
Present work |
Conclusions
In this paper, we designed a novel sensor for the nicotine insecticide acetamiprid, which features dual fluorescence and colorimetric detection signal outputs and is based on a dual hairpin cascade amplification reaction. This detection method is different from single-signal detection, as naked-eye and instrument-based detection complement each other. The sensitivity of the aptamer biosensor, designed based on G-quadruplexes and a hybrid chain reaction, was studied under the optimal test conditions, and the linear regression equation can be expressed as y = 331.31x + 3543.1 (x and y are the Ace concentration and fluorescence intensity, respectively) with a correlation coefficient R2 of 0.9858. In addition, according to the formula 3σ/S (where σ is the standard deviation of the control solution and S is the slope of the linear regression equation), the limit of detection (LOD) toward acetamiprid was calculated to be 27.7 pM. Furthermore, the colorimetric absorbance output enables qualitative target determination without depending on instruments. This sensing platform is a low-cost flexible platform that can satisfy the demands of future practical applications. Genomics technology could be utilized to amplify and increase the detection signal. In addition, the platform could be used to detect other targets via modifying the aptamer and target pair. The sophisticated design of this biosensor means it will have potential uses in chemistry, biomedicine, and environmental monitoring.
Conflicts of interest
There are no conflicts to declare.
Acknowledgements
This work is supported by the National Natural Science Foundation of China (No. 62073207) and the Basic Natural Science Research Program of Shaanxi Province (No. 2020JM-298).
Notes and references
- P. Jeschke and R. Nauen, Pest Manag. Sci., 2008, 64, 1084–1098 CrossRef CAS.
- J. Liu, F. Zha, Y. Xu, J. Qiu and Y. Qian, Foods, 2021, 10, 4–835 Search PubMed.
- A. Y. Kocaman and M. Topaktas, Environ. Toxicol., 2010, 25, 157–168 CAS.
- E. S. Istifli, M. Buyukleyla, E. Rencuzogullari and M. Topaktas, Toxicol. Ind. Health, 2013, 29, 23–37 CrossRef.
- J. J. Xiong, H. Z. Li, X. Ma and J. You, J. Separ. Sci., 2018, 41, 525–533 CrossRef CAS.
- L. Madianos, G. Tsekenis, E. Skotadis, L. Patsiouras and D. Tsoukalas, Biosens. Bioelectron., 2018, 101, 268–274 CrossRef CAS PubMed.
- Y. M. Kim, C. Liu and W. H. Tan, Biomarkers Med., 2009, 3, 193–202 CrossRef CAS PubMed.
- A. Marin, J. Vidal, F. Gonzalez, A. G. Frenich, C. R. Glass and M. Sykes, J. Chromatogr., B: Anal. Technol. Biomed. Life Sci., 2004, 804, 269–275 CrossRef CAS PubMed.
- H. Wenchao, T. Ying and S. Xiaoming, Chemosphere, 2018, 192, 59–65 CrossRef PubMed.
- J. Vichapong, R. Burakham and S. Srijaranai, Chromatographia, 2016, 79, 285–291 CrossRef CAS.
- O. Lopez-Fernandez, R. Rial-Otero and J. Simal-Gandara, Anal. Bioanal. Chem., 2015, 407, 7101–7110 CrossRef CAS.
- H. Obana, M. Okihashi, K. Akutsu, Y. Kitagawa and S. Hori, J. Agric. Food Chem., 2003, 51, 2501–2505 CrossRef CAS PubMed.
- T. Yukari, N. Yumiko, T. Yasuhide, K. Yoshihisa, T. Yuzo and S. Tadashi, Food Hyg. Saf. Sci., 1998, 39, 2 Search PubMed.
- X. A. Zhang, N. Mobley, J. G. Zang, X. M. Zheng, L. Lu, O. Ragin and C. J. Smith, Agric. Food Chem., 2010, 58, 1553–11560 Search PubMed.
- R. Marina, G. Svetlana, V. Tatjana and L. Mila, Food Chem., 2009, 112, 712–719 Search PubMed.
- W. Shigeyuki, I. Shigekazu, K. Yoshio, O. Naiki, Y. Tetsuo, M. Hiroshi, K. Takashi and Y. Yojiro, Anal. Chim. Acta, 2001, 427 Search PubMed.
- K. M. Fan, W. K. Kang, S. F. Qu, L. Li, B. H. Qu and L. H. Lu, Talanta, 2019, 197, 645–652 CrossRef CAS PubMed.
- A. Y. Kocaman and M. Topaktas, Environ. Toxicol., 2010, 25, 157–168 CAS.
- A. Bahreyni, R. Yazdian-Robati, M. Ramezani, K. Abnous and S. M. Taghdisi, Mikrochim. Acta, 2018, 185, 272 CrossRef PubMed.
- R. Stoltenburg, C. Reinemann and B. Strehlitz, Biomol. Eng., 2007, 24, 381–403 CrossRef CAS PubMed.
- J. A. He, Y. A. Liu, M. T. Fan and X. J. Liu, J. Agric. Food Chem., 2011, 59, 1582–1586 CrossRef CAS PubMed.
- D. Mariia, R. Silvie, G. Helena and R. Tomas, Biotechnol. Adv., 2015, 33, 1141–1161 CrossRef.
- W. T. Yang, X. X. Zhou, J. M. Zhao and W. J. Xu, Mikrochim. Acta, 2018, 185, 100 CrossRef PubMed.
- C. Ma, H. Y. Liu, T. Tian, X. R. Song, J. H. Yu and M. Yan, Biosens. Bioelectron., 2016, 83, 15–18 CrossRef CAS PubMed.
- R. Dongxia, S. Chengjun, H. Zhijun, L. Zewei, Z. Chen and L. Yongxin, Sensor. Actuator. B Chem., 2019, 296 Search PubMed.
- R. V. Ravindranathan and H. T. Miles, Biochim. Biophys. Acta, 1965, 94, 603–606 CrossRef CAS.
- C. Schaffitzel, I. Berger, J. Postberg, J. Hanes, H. J. Lipps and A. Pluckthun, Proc. Natl. Acad. Sci. U. S. A., 2001, 98, 8572–8577 CrossRef CAS PubMed.
- T. Simonsson, Biol. Chem., 2001, 382, 621–628 CAS.
- T. Simonsson, P. Pecinka and M. Kubista, Nucleic Acids Res., 1998, 26, 1167–1172 CrossRef CAS.
- J. T. Davis, Angew. Chem., Int. Ed., 2004, 43, 668–698 CrossRef CAS.
- W. Guliang and M. V. Karen, DNA Repair, 2014, 19, 143–151 CrossRef PubMed.
- J. M. Nicoludis, S. T. Miller, P. D. Jeffrey, S. P. Barrett, P. R. Rablen, T. J. Lawton and L. A. Yatsunyk, J. Am. Chem. Soc., 2012, 134, 20446–20456 CrossRef CAS PubMed.
- J. M. Nicoludis, S. P. Barrett, J. Mergny and L. A. Yatsunyk, Nucleic Acids Res., 2012, 40 Search PubMed.
- A. Ambrus, D. Chen, J. Dai, T. Bialis, R. A. Jones and D. Yang, Nucleic Acids Res., 2007, 34, 2440–2450 Search PubMed.
- W. XiaoFeng, W. YongSheng, X. XiLin, L. Wen-Bo, Z. Bin, C. SiHan and X. JinHua, Anal. Methods, 2018, 10, 1039 Search PubMed.
- H. Xi, M. Juhas and Y. Zhang, Biosens. Bioelectron., 2020, 167, 112–122 CrossRef.
- L. Mei, K. Arshad, W. Zhifei, L. Yuan, Y. Gaojian, D. Yan and H. Nongyue, Biosens. Bioelectron., 2019, 130, 174–184 CrossRef PubMed.
- P. Weerathunge, R. Ramanathan, R. Shukla, T. K. Sharma and V. Bansal, Anal. Chem., 2014, 86, 11937–11941 CrossRef CAS PubMed.
- B. L. Li, Y. Du, T. Li and S. J. Dong, Anal. Chim. Acta, 2009, 651, 234–240 CrossRef CAS PubMed.
- P. Travascio, P. K. Witting, A. G. Mauk and D. Sen, J. Am. Chem. Soc., 2001, 123, 1337–1348 CrossRef CAS PubMed.
- J. Chen, J. Pan and S. Chen, Chem. Sci., 2018, 9, 300 RSC.
- Y. Song, K. Qu, C. Zhao, J. Ren and X. Qu, Adv. Mater., 2010, 22, 2206–2210 CrossRef CAS.
- T. Paola, L. Yingfu and S. Dipankar, Chem. Biol., 1998, 5, 505–517 CrossRef.
- S. Zhao, L. Yu, S. Yang, X. Tang, K. Chang and M. Chen, Nanoscale Horiz., 2021, 6, 298–310 RSC.
- X. Liu, F. Meng and R. Sun, et al., Chem. Commun., 2021, 57, 2629–2632 RSC.
- P. D. Josephy, T. Eling and R. P. Mason, J. Biol. Chem., 1982, 257, 3669–3675 CrossRef CAS.
- H. J. Shi, G. H. Zhao, M. C. Liu, L. F. An and T. C. Cao, J. Hazard. Mater., 2013, 260, 754–761 CrossRef CAS PubMed.
- Z. M. Xiao, X. W. Li, X. L. Wang, J. Z. Shen and S. Y. Ding, J. Chromatogr., B: Anal. Technol. Biomed. Life Sci., 2011, 879, 117–122 CrossRef CAS.
- L. G. Chen and B. Li, J. Chromatogr., B: Anal. Technol. Biomed. Life Sci., 2012, 897, 32–36 CrossRef CAS PubMed.
- Z. Jiao, H. F. Zhang, S. H. Jiao, Z. N. Guo, D. Zhu and X. F. Zhao, Food Anal. Methods, 2019, 12, 668–676 CrossRef.
- K. Abnous, N. Danesh, M. Ramezani, M. Alibolandi, P. Lavaee and S. Taghdisi, Microchim. Acta, 2017, 184, 81–90 CrossRef CAS.
- B. Wang, Y. F. Chen, Y. Y. Wu, B. Weng, Y. S. Liu, Z. S. Lu, C. M. Li and C. Yu, Biosens. Bioelectron., 2016, 78, 23–30 CrossRef CAS PubMed.
- L. Xiang and J. S. Tang, RSC Adv., 2017, 7, 8332–8337 RSC.
- J. Wang, Y. Wu, P. Zhou, W. Yang, H. Tao, S. Qiu and C. Feng, Analyst, 2018, 143, 5151–5160 RSC.
|
This journal is © The Royal Society of Chemistry 2022 |