DOI:
10.1039/D2RA03379H
(Paper)
RSC Adv., 2022,
12, 18884-18888
The discovery of penta-peptides inhibiting the activity of the formylglycine-generating enzyme and their potential antibacterial effects against Mycobacterium tuberculosis†
Received
31st May 2022
, Accepted 16th June 2022
First published on 29th June 2022
Abstract
The formylglycine-generating enzyme is a key regulator that converts sulfatase into an active form. Despite its key role in many diseases, enzyme activity inhibitors have not yet been reported. In this study, we investigated penta-peptide ligands for FGE activity inhibition and discovered two hit peptides. In addition, the lead peptides also showed potential antibacterial effects in a Mycobacterium tuberculosis model.
Introduction
The formylglycine-generating enzyme (FGE) is a metalloenzyme that can recognize substrates that have conserved CxPxR-sequence pentapeptides and can then convert cysteine into formylglycine (fGly) through post-translational modification via oxygen-dependent catalysis.1 In many organisms, the FGE is an essential enzyme for activating sulfatases, where the active form contains fGly at the enzyme active site for hydrolysis reactions with the sulfate ester groups of substrates. Since sulfatases have a vast array of both normal biological and pathophysiological roles in host systems, they are highly associated with many diseases, including multiple sulfatase deficiency (MSD), metachromatic leukodystrophy, and infections.2 Among these, MSD is a fatal inherited metabolic disorder in humans that is induced by a lack of FGE activity.3 40 different genetic mutations of the FGE have been reported to cause MSD, resulting in short life expectancy.3b Due to its high lethality, it is difficult to study the mechanism of the progression of MSD using genetic alteration models, and there is a high demand for a suitable biological model for studying the biomolecular changes that occur upon the inhibition of FGE activity. However, mechanism of action studies involving the early stages of MSD and related perturbed pathway studies face a hurdle due to the lack of ligands that can inhibit FGE activity. In addition, previous studies have also shown that both enteric commensal and pathogenic bacterial species employ sulfatases to metabolize host metabolites, including sulfated glycosaminoglycan (GAG),4 and genetic mutations relating to sulfatase can affect their survival. In particular, it has been suggested that mycobacterial sulfatases act on extracellular sulphated GAG, thereby facilitating the adhesion of Mycobacterium tuberculosis to epithelial cells,5 eventually changing the gut microbiome composition and potentially affecting the nutrition, physiology, and immune function of the host. Despite these biological impacts, FGE activity modulators and inhibitors have not yet been developed. In this study, we report the first discovery of FGE activity inhibitors using canonical peptides.
Results and discussion
In vitro FGE activity assay setup
To screen activity inhibitors, we initially set up an in vitro enzyme activity assay based on the LCMS profile of a natural substrate peptide (15-mer: ALCTPSRGSLFTGRY). FGEs from M. tuberculosis were cloned into a pET15b plasmid with an additional purification tag at the N-terminal (detailed information is given in the ESI†), and we optimized the assay conditions for enzyme activity measurements. As previously reported, the activity of FGE was sensitive to the amount of copper ions and the reducing agent DTT.6 We also confirmed the enzyme kinetics using the natural substrate and found that the enzyme exhibited moderate affinity for the substrate (Km = 40 μM) and a fast product turnover (kcat = 5.6 min−1; kcat/Km = 1.4 × 106 M−1 s−1) (Fig. 1). Using the optimized assay conditions, we first examined a simple peptide that contains serine (LS*TPSR) instead of cysteine, based on the natural substrate, but this did not inhibit the FGE activity up to 250 μM (Fig. S1†). This result showed that subtle changes to the natural substrate can significantly alter the activity modulation effects, and we required large-size enzyme–ligand binding evaluation data to find activity inhibitors.
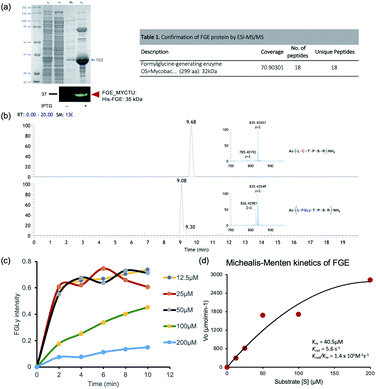 |
| Fig. 1 FGE activity assays. (a) The affinity enrichment of overexpressed FGE protein resolved on 10% SDS-PAGE, with the identity of the purified protein confirmed via antibody and nano-LCMS/MS methods. (b) Mass chromatograms and corresponding MS spectra of the substrate (Ac-ALCTPSRGSLFTGRY-NH2; 835.42657 (z = 2)) and product (Ac-AL[FGLy]TPSRGSLFTGRY-NH2; hydrated formylglycine form: 835.43579 (z = 2)). (c) FGE conversion efficiency as a function of time at different substrate concentrations. (d) Kinetics data fitted to the Michaelis–Menten equation via nonlinear regression. | |
Primary screening: in silico docking simulations
For primary screening, we conducted a brute-force search campaign for FGE active-site binders based on pentamer peptides using docking simulations. We used the human FGE crystal structure (PDB: 1Y1E) because there is no crystal structure available for the FGE from M. tuberculosis. However, it is known that active sites are highly conserved between species, and the overall sequence homology between human and mycobacteria examples is 43%, which is also high (Fig. S2†). Additionally, our human FGE crystal structure and an AlphaFold2 model structure predicted based on FGE sequence information from M. tuberculosis were significantly similar (Fig. 2a). Since the current performance of docking simulations can only provide correlation coefficients of 0.4–0.5 between the docking score and experimental binding data,7 we decided to choose several peptides depending on docking score ranges, instead of simply focusing on top-tier candidates. For this purpose, we did not have to cover the total search space (205 = 3
200
000). Instead, we examined almost half of the options, consisting of peptides with one of 11 randomly selected amino acids as the first residue (xXXXX: x is C, D, E, H, K, L, M, P, Q, R, or Y; and X is any amino acid).
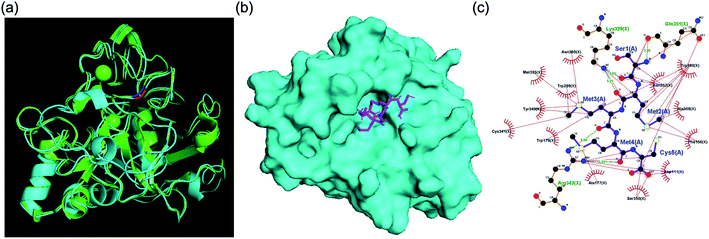 |
| Fig. 2 (a) Structural similarity of FGEs from humans (PDB: 1Y1E) and M. tuberculosis (AlphaFold2 model). (b) The binding mode of SMMMC and the human FGE. (c) Interaction mapping between SMMMC and 1Y1E. Residues that play a role in hydrophobic interactions are colored red and hydrogen bonds are marked with green dashed lines. | |
In this primary screening, the majority of penta-peptides showed binding affinity near the median (−8.26 kcal mol−1) with relatively narrow standard deviation (0.727 kcal mol−1). Based on a boxplot of the docking simulation results, we re-sampled a total of 20 penta-peptides, consisting of 2 to 6 peptides from 6 statistical regions (details in Fig. S3†), and conducted in vitro FGE activity inhibition assays to discover lead candidates.
FGE activity perturbation abilities of the candidate penta-peptides in vitro
For secondary in vitro assays, we measured the activity inhibition levels at 5 different penta-peptide concentrations (50, 100, 150, 200, and 250 μM) in the presence of the natural substrate peptide at 100 μM. A control sample without an additional penta-peptide showed clear consumption of the substrate after 10 min; thus, we measured activity inhibition based on the conversion level after 10 min of enzyme reaction. Surprisingly, only two candidate penta-peptides (SCGMM and SMMMC) exhibited more than 50% activity inhibition at a concentration equal to one equivalent of the natural substrate, while the rest showed subtle activity perturbation (Fig. 3 and S4–S23†). Based on the docking simulations, SMMMC exhibited reasonable binding at the active site (Fig. 2b). SMMMC undergoes hydrogen bonding with Lys329, Gln351, and Arg343, and hydrophobic interactions with Asp111, Phe156, Ala177, Trp179, Trp180, Trp299, Met335, Tyr340, Cys341, Ser350, Asp352, Ala358, and Asn360, as shown in Fig. 2c.
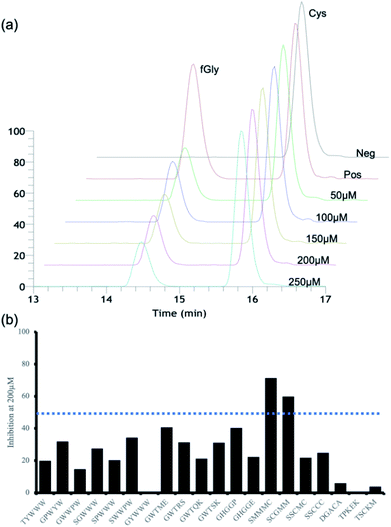 |
| Fig. 3 FGE activity inhibition profiles. (a) An illustration of the LCMS quantitation profiles of the 15-mer natural substrate (containing Cys) and its product (containing fGly). (b) In vitro enzyme activity inhibition profiles of 20 candidate peptides at 200 μM. | |
It is also noteworthy that the two hits had the lowest ranking in terms of the primary screening results. Many top-ranked candidates contain tryptophan residues (regions 5 and 6 in Fig. S3†), but none of those showed any marked activity inhibition effects, possibly highlighting the current prediction limits in relation to aromatic interactions during docking simulations.8 Although there seems to be more room for improving the inhibitory effects, these two penta-peptides are the first known FGE activity inhibitors, to the best of our knowledge.
M. tuberculosis infection model study
Encouraged by the FGE inhibitory effects of the two peptides, we further investigated the FGE inhibitory effects against M. tuberculosis infection. During infection, M. tuberculosis employs a slew of evasive strategies, including arresting its own biosynthetic machinery and utilizing alternative inorganic energy sources through sulfur metabolism,9 in which sulfatases play a crucial role. Although M. tuberculosis has many sulfatase genes, only one FGE gene has been identified.10 Thus, we envisioned that the inhibition of FGE activity may be an effective strategy to perturb all sulfatase classes and obtain antibacterial effects against tuberculosis. To prove our hypothesis, we chose Mycolicibacterium smegmatis as a tuberculosis model.11 Different concentrations of the hit peptides were incubated with the culture media, from 12.5 μM to 100 μM, for 3 days, and growth inhibition was measured via bacterial counting. Interestingly, both SMMMC and SCGMM exhibited a dose-dependent growth inhibition trend, and SMMMC and SCGMM inhibited the growth of M. smegmatis by up to 40% and 25%, respectively, at 100 μM (Fig. 4). Since the enzyme activity inhibition potency of SMMMC was superior to SCGMM, the bacterial growth inhibition effects of SMMMC were also higher than those of SCGMM. Compared to the other antimicrobial peptides (AMPs),12 our hit penta-peptides represent a new class of AMP with a unique target enzyme. Although the hits are relatively less potent from a growth inhibition point of view, there is much room to improve the potency and selectivity, considering the first-generation state of the lead compounds.
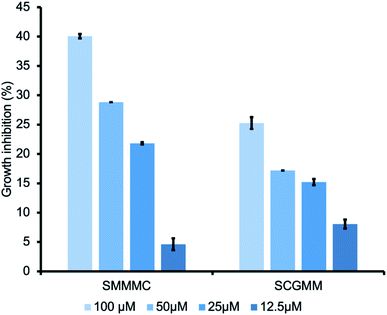 |
| Fig. 4 Growth inhibition of M. smegmatis upon treatment with the hit penta-peptides. A series of peptide doses was administrated in the growth medium, and the inhibition percentages were obtained after 3 days of culturing. Growth inhibition values were obtained from duplicate experiments. | |
Experimental
Preparation of protein expression vectors
The gene encoding M. tuberculosis FGE (Rv0712, residues 2–299) was amplified from the plasmid pBAD/myc-his A Rv0712 (FGE), a gift from Carolyn Bertozzi (Addgene plasmid #16132; https://n2t.net/addgene:16132; RRID: Addgene_16132), and it was subcloned into pET15b (Novagen) using NdeI and BamHI restriction sites. The resulting construct was transformed into DH5-α cells. Extracted plasmids were sequenced to verify the insertion points. Protein-encoding plasmids were transformed into BL21(DE3) cells (Enzynomics).
Protein expression and purification
FGE protein was expressed and purified as previously described, with a few exceptions.13 FGE-containing clones were incubated in Luria Bertani medium supplemented with ampicillin under shaking at 37 °C until A600 = 1.5–2. The cultures were diluted to 1
:
100 and incubated until OD600 reached 0.7–0.9. The culture flasks were placed in ice-water to lower the temperature to approximately 18–20 °C and then 250 μM isopropyl-1-thio-β-D-galactopyranoside was added. Cultures were incubated at 20 °C for 14–16 h. Cells were harvested, frozen for a minimum of 1 h, and resuspended (10 mg ml−1) in 30 ml of lysis buffer (50 mM triethanolamine, 250 mM NaCl, 10% glycerol, and 5 mM calcium acetate; pH 7.4) supplemented with 10 mM imidazole, DNase I (10 μg ml−1), lysozyme (1 mg ml−1), 2 mM dithiothreitol, 1 mM tris(2-carboxyethyl), and phosphine, at pH 7.4 and with 3× protease inhibitors. The suspension was incubated at room temperature under gentle shaking at room temperature for 30 min, followed by sonication. To avoid the precipitation of enzymes during cell lysis, we recommend that lysis should be carried out in the presence of millimolar concentrations of both DTT and TCEP and on ice, especially if a high protein yield is expected. The cell lysate was clarified via centrifugation, filtered through a 0.45 μm filter (Corning, Corning Incorporated, Germany), and incubated with lysis-buffer-equilibrated Ni-NTA agarose (Qiagen). The agarose was washed with 20 column volumes of lysis buffer containing 40 mM imidazole and 2 mM DTT, and the protein was eluted using lysis buffer containing 300 mM imidazole and 3 mM DTT. The eluted protein was concentrated to 0.5 ml. The protein was dialysed into dialysis buffer (25 mM triethanolamine, 150 mM NaCl, and 10% glycerol; pH 7.6) using SnakeSkin dialysis tubing (10 K MWCO, 22 mm; Thermo Fisher Scientific) under stirring for 36 h at 4 °C. The identity and purity of the product were assessed via MS/MS mass spectrometry (LC-Orbitrap Velos, Thermo Fisher Scientific).
FGE activity assay
The enzyme assay was carried out as described previously.13b Briefly, approximately 20 μM purified enzyme was added to room-temperature-equilibrated reaction buffer containing 25 mM triethanolamine at pH 7.4, 50 mM NaCl, 20 μM CuSO4, 2 mM DTT, 2× protease inhibitors, and 100 μM FGE substrate Ac-ALCTPSTGSLFTGRY-NH2. The reaction was incubated under gentle vortexing at RT for 1 h, followed by the addition of 100 mM HCl to terminate the reaction.
Kinetics assay
The enzyme was added to a prebuffered reaction mixture equilibrated at RT for 5 min containing the substrate peptide (at a concentration of 0–200 μM). The reaction was allowed to proceed at room temperature under gentle vortexing for 10 min. The reaction was stopped with 100 mM HCl, ziptip-desalted, and analysed via LC-ESI-MS. The peak area for the product was obtained and integrated to plot the Michaelis–Menten curve and calculate the Vmax, Km, and specific constant (kcat/Km) values for the FGE. The experiments were conducted in triplicate.
Enzyme inhibitor assay
All peptides used for this study were obtained from Peptron Inc. (Daejeon, South Korea) with 99% purity (based on 220 nm LC chromatograms). Native substrate (100 μM) and inhibitor (at concentrations ranging from 50–250 μM) were added to room-temperature-equilibrated reaction buffer containing 20 μM formylglycine-generating enzyme (FGE), equimolar CuSO4, 2 mM dithreitol (DTT), 50 mM sodium chloride, and 2× protease inhibitors. The reaction was conducted at RT for 1 h, followed by the addition of 100 mM HCl to terminate the reaction. The reaction products were desalted and then analysed using a binary-pump Thermoscientific Ultimate 3000 HPLC system (Thermo Scientific, Waltham, Massachusetts, United States) fitted with a reverse phase SCINChrom C18G 3 μm column (150 × 4.6 mm; 3.6 μm particle size) (Seoul, USA) and a dual wavelength absorbance detector (220 nm and 280 nm).
Assay conditions for LCMS. The desalted reaction products of FGE enzyme catalytic and inhibitory assays were analyzed using a binary-pump LC (Ultimate 3000 HPLC system; Thermo Scientific, Waltham, Massachusetts, United States) fitted with either a reverse-phase Luna Phenomenex C18 3 (50 × 2.0 mm, 3 μm particle size; Phenomenex, CA, USA) (Fig. S4–S8†) or reverse-phase SCINChrom C18G 3 μm (150 × 4.6 mm, 3.6 μm particle size; Scinco, Korea) (Fig. S9–S23†) column and a dual wavelength absorbance detector (220 nm and 280 nm). The LC elution conditions were identical for both columns and they were as follows: flow rate, 0.3 ml min−1; column temperature: 28 °C; 3% 0.1% formic acid in acetonitrile (solvent B) for 2 min; followed by elution with linearly graded solvent B with a gradient of up to 20% solvent B for 8 min, then up to 45% for 2 minutes, and then topping off with a final elution gradient of 70% solvent B for 3 min. The HPLC-resolved peptide substrate and enzymatic reaction products were simultaneously analysed via electrospray ionization – mass spectrometry (ESI-Velos). The MS conditions were as follows: MS was set to positive ESI mode with a scan range from 50–2000; the capillary voltage was 3.44 kV; the sampling cone was set to 30 and the extraction cone to 3.44; the source temperature was set to 301.32 °C and the desolvation temperature to 312 °C; the flow of the cone gas was 50 l h−1 and that of the desolvation gas was 800 l h−1. Mass spectrometry data were collected using Orbitrap apparatus (Thermo Fisher Scientific).
Docking simulations
The binding modes between the penta-peptide and FGE structures were evaluated based on AutoDock Vina docking simulations (version 1.1.2).14 The protein crystal structure was obtained from the PDB database (PDB code: 1Y1E) consisting of the human FGE protein at a resolution of 1.73 Å. The initial structures of the ligands for docking simulations were prepared using PyRosetta, and energy was briefly minimized to obtain optimum geometry.15 The generated peptide coordinates were stored in PDB and converted to PDBQT using the MGLTools prepare_ligand4.py routine. After running the docking simulation, each ligand docking result was examined, and the top binding energies were examined and visualized using a boxplot, as shown in Fig. S3.† The docking result conformations of ligands were visualized for interaction mapping using LigPlot+ (v2.2, EMBL-EBI).16
Conclusions
In short, we explored penta-peptide ligands for FGE activity inhibition both in silico and in vitro. From our two-step screening approach, we discovered FGE activity inhibitors, and the two hit penta-peptides showed dose-dependent growth inhibition in a Mycobacterium tuberculosis model. This is the first report of FGE inhibitors and their antibacterial effects against M. tuberculosis. It is also worth mentioning that our lead peptides have great potential to act as tools for investigating early endogenous changes in MSD patients, as a genetic knock-out model cannot be established due to the lethal effects. More related studies, including further optimization to obtain higher potency and mechanistic studies relating to MSD in a mammalian model, are underway.
Conflicts of interest
There are no conflicts to declare.
Acknowledgements
This work is supported by a Korea University Guro Hospital (KOREA RESEARCH-DRIVEN HOSPITAL) grant funded by Korea University Medicine (K2210341), Korea University Grant (K2110571), and the National Research Foundation (NRF-2018M3A9H4079286 and NRF-2020R1A2C2004422).
Notes and references
-
(a) M. J. Appel and C. R. Bertozzi, ACS Chem. Biol., 2015, 10, 72–84 CrossRef CAS PubMed;
(b) E. C. Ennemann, K. Radhakrishnan, M. Mariappan, M. Wachs, T. H. Pringle, B. Schmidt and T. Dierks, J. Biol. Chem., 2013, 288, 5828–5839 CrossRef CAS PubMed;
(c) A. Preusser-Kunze, M. Mariappan, B. Schmidt, S. L. Gande, K. Mutenda, D. Wenzel, K. von Figura and T. Dierks, J. Biol. Chem., 2005, 280, 14900–14910 CrossRef CAS PubMed.
-
(a) C. Settembre, I. Annunziata, C. Spampanato, D. Zarcone, G. Cobellis, E. Nusco, E. Zito, C. Tacchetti, M. P. Cosma and A. Ballabio, Proc. Natl. Acad. Sci. U. S. A., 2007, 104, 4506–4511 CrossRef CAS PubMed;
(b) M. P. Cosma, S. Pepe, I. Annunziata, R. F. Newbold, M. Grompe, G. Parenti and A. Ballabio, Cell, 2003, 113, 445–456 CrossRef CAS;
(c) Y. Takakusaki, S. Hisayasu, Y. Hirai and T. Shimada, Hum. Gene Ther., 2005, 16, 929–936 CrossRef CAS PubMed.
-
(a) S. Beck-Wodl, C. Kehrer, K. Harzer, T. B. Haack, F. Burger, D. Haas, A. Riess, S. Groeschel, I. Krageloh-Mann and J. Bohringer, JIMD Reports, 2021, 58, 80–88 CrossRef PubMed;
(b) N. Mohammadian Khonsari, B. Hakak-Zargar, T. Voth and S. Noorian, Endocrinol., Diabetes Metab. Case Rep., 2020, 2020, 20–0128 Search PubMed.
-
(a) J. E. Ulmer, E. M. Vilen, R. B. Namburi, A. Benjdia, J. Beneteau, A. Malleron, D. Bonnaffe, P. A. Driguez, K. Descroix, G. Lassalle, C. Le Narvor, C. Sandstrom, D. Spillmann and O. Berteau, J. Biol. Chem., 2014, 289, 24289–24303 CrossRef CAS PubMed;
(b) E. C. Martens, H. C. Chiang and J. I. Gordon, Cell Host Microbe, 2008, 4, 447–457 CrossRef CAS PubMed.
- J. D. Mougous, R. E. Green, S. J. Williams, S. E. Brenner and C. R. Bertozzi, Chem. Biol., 2002, 9, 767–776 CrossRef CAS PubMed.
-
(a) M. J. Appel, K. K. Meier, J. Lafrance-Vanasse, H. Lim, C. L. Tsai, B. Hedman, K. O. Hodgson, J. A. Tainer, E. I. Solomon and C. R. Bertozzi, Proc. Natl. Acad. Sci. U. S. A., 2019, 116, 5370–5375 CrossRef CAS PubMed;
(b) P. G. Holder, L. C. Jones, P. M. Drake, R. M. Barfield, S. Banas, G. W. de Hart, J. Baker and D. Rabuka, J. Biol. Chem., 2015, 290, 15730–15745 CrossRef CAS PubMed.
-
(a) N. T. Nguyen, T. H. Nguyen, T. N. H. Pham, N. T. Huy, M. V. Bay, M. Q. Pham, P. C. Nam, V. V. Vu and S. T. Ngo, J. Chem. Inf. Model., 2020, 60, 204–211 CrossRef CAS PubMed;
(b) Z. Wang, H. Y. Sun, X. J. Yao, D. Li, L. Xu, Y. Y. Li, S. Tian and T. J. Hou, Phys. Chem. Chem. Phys., 2016, 18, 12964–12975 RSC.
- M. Brylinski, Chem. Biol. Drug Des., 2018, 91, 380–390 CrossRef CAS PubMed.
- L. G. Wayne and C. D. Sohaskey, Annu. Rev. Microbiol., 2001, 55, 139–163 CrossRef CAS PubMed.
- K. E. Beatty, M. Williams, B. L. Carlson, B. M. Swarts, R. M. Warren, P. D. v. Helden and C. R. Bertozzi, Proc. Natl. Acad. Sci. U. S. A., 2013, 110, 12911–12916 CrossRef CAS PubMed.
-
(a) J. M. Reyrat and D. Kahn, Trends Microbiol., 2001, 9, 472–474 CrossRef CAS PubMed;
(b) V. Humnabadkar, P. Madhavapeddi, H. Basavarajappa, M. G. Sheikh, R. Rane, R. Basu, P. Verma, A. Sundaram, K. Mukherjee and S. M. de Sousa, J. Biomol. Screening, 2015, 20, 265–274 CrossRef PubMed.
- M. AlMatar, E. A. Makky, G. Yakici, I. Var, B. Kayar and F. Koksal, Pharmacol. Res., 2018, 128, 288–305 CrossRef PubMed.
-
(a) B. L. Carlson, E. R. Ballister, E. Skordalakes, D. S. King, M. A. Breidenbach, S. A. Gilmore, J. M. Berger and C. R. Bertozzi, J. Biol. Chem., 2008, 283, 20117–20125 CrossRef CAS PubMed;
(b) P. G. Holder, L. C. Jones, P. M. Drake, R. M. Barfield, S. Banas, G. W. de Hart, J. Baker and D. Rabuka, J. Biol. Chem., 2015, 290, 15730–15745 CrossRef CAS PubMed.
- J. Eberhardt, D. Santos-Martins, A. F. Tillack and S. Forli, J. Chem. Inf. Model., 2021, 61, 3891–3898 CrossRef CAS PubMed.
- S. Chaudhury, S. Lyskov and J. J. Gray, Bioinformatics, 2010, 26, 689–691 CrossRef CAS PubMed.
- R. A. Laskowski and M. B. Swindells, J. Chem. Inf. Model., 2011, 51, 2778–2786 CrossRef CAS PubMed.
|
This journal is © The Royal Society of Chemistry 2022 |
Click here to see how this site uses Cookies. View our privacy policy here.