DOI:
10.1039/D2RA02939A
(Paper)
RSC Adv., 2022,
12, 24319-24338
Oxa-376 and Oxa-530 variants of β-lactamase: computational study uncovers potential therapeutic targets of Acinetobacter baumannii†
Received
11th May 2022
, Accepted 17th August 2022
First published on 26th August 2022
Abstract
Antimicrobial resistance is a major global health crisis, resulting in thousands of deaths each year. Antibiotics' effectiveness against microorganisms deteriorates over time as multidrug resistance (MDR) develops, which is exacerbated by irregular antibiotic use, poor disease management, and the evasive nature of bacteria. The World Health Organization has recognized multidrug resistance as a critical public health concern, and Acinetobacter baumannii has been at the center of attention due to its ability to develop multidrug resistance (MDR). It generally produces carbapenem-hydrolyzing oxacillinase, which has been identified as the primary source of beta-lactam resistance in MDR bacteria. Recently, point mutations in A. baumannii have been identified as a key factor of multidrug resistance, making them a prime concern for researchers. The goal of the current work was to establish a unique way of finding multidrug-resistant variants and identify the most damaging mutations in the existing databases. We characterized the deleterious variants of oxacillinases using several computational tools. Following a thorough analysis, Oxa-376 and Oxa-530 were found to be more damaging when compared with the wild-type Oxa-51. The mutants' 3D structures were then prepared and refined with RaptorX, GalaxyRefine, and SAVES servers. Our research incorporates seven antimicrobial agents to illustrate the resistance capability of the variants of oxacillinase by evaluating binding affinity in Autodock-vina and Schrodinger software. RMSD, RMSF, Radius of gyration analysis, the solvent-accessible surface area (SASA), hydrogen bonding analysis and MM-GBSA from Molecular Dynamics Simulation revealed the dynamic nature and stability of wild-type and Oxa-376 and Oxa-530 variants. Our findings will benefit researchers looking for the deleterious mutations of Acinetobacter baumannii and new therapeutics to combat those variants. However, further studies are necessary to evaluate the mechanism of hydrolyzing activity and antibiotic resistance of these variants.
1 Introduction
Antibiotic resistance is a threat to human health responsible for millions of deaths, and this situation is worsening day by day. Recently, they have been rapidly losing their efficacy. The emergence of multi-drug resistance (MDR) reduces the potency of antibiotics against bacteria over time. Acinetobacter baumannii is one of the most dangerous bacteria, known to cause a wide variety of antibiotic resistances, making it nearly incurable.1 Researchers have brought Acinetobacter to the forefront of study due to the potential for acquiring drug-resistant genes. A. baumannii is an oxygen-dependent, catalase-positive, Gram-negative, bacterium that belongs to the coccobacillus class of bacteria.2 It is an opportunistic bacterium capable of causing a variety of nosocomial diseases like meningitis, urinary tract infection, pneumonia, cardiomyopathy, wound infections.3 Beijerinck, a Dutch microbiologist, first isolated this organism from the soil in 1911 using calcium acetate media.4 It has a genomic part of 86-kb with a distinctive structure comprising about 45 resistant genes.5 Recently, A. baumannii has been recognized as a “red alert” human microorganism due to its aggressive characteristics and a broad range of antibiotic resistance.6 A. baumannii is capable of mutating, receiving, and exchanging resistance genes associated with insertion sequences, chromosomal resistance, and plasmids all of which show varying degrees of diversity.7 Accumulation of multiple antibiotic-resistant genes in A. baumannii can result in a multiple drug-resistant organism.8 A. baumannii produces carbapenem-hydrolyzing oxacillinases against beta-lactam antibiotics after acquiring the resistance mechanism. Beta-lactamases may be divided into four categories based on the nature of the active zone, utilizing the Ambler categorization approach: class A, B, C, and D. Beta-lactamases (oxacillinase) are members of class D, which is substantially more complex than classes A, B, and C.9 Beta-lactamases can further be categorized into four subtypes. Subtypes 1 and 4 include plasmid-encoded OXA-23, OXA-58,10 and subtypes 2 and 3 include chromosomally encoded OXA-24, OXA-51, and their variants.11 Among all these variants, Oxa-51 is a powerful variant that develops widespread resistance and has caught the attention of scientists.
Beta-lactam antibiotics are widely used against A. baumannii for their reasonable cost, effectiveness, and fewer side effects.12 Penicillin (Amoxicillin), Cephalosporin (Ceftriaxone, Cefuroxime), Carbapenem (Meropenem), and Monobactam (Aztreonam) are beta-lactam antibiotics with four-membered lactam rings containing nitrogen attached to beta-carbon.13,14 These antibiotics are called beta-lactams and they destroy bacteria by interfering with cell wall formation. However, due to its evasive nature, A. baumannii develops a unique mechanism of escaping antibiotic action by producing the enzyme oxacillinase. Beta-lactamases, one of the primary components secreted by A. baumannii, breaks the lactam ring, which is required for beta-lactam activity. Therefore, impaired beta-lactams cannot prevent cross-linking of the NAG–NAM chain to create peptidoglycan by transpeptidase enzyme (penicillin-binding protein). As a result, bacterial cell wall formation continues resulting in resistance to the antibiotics.15
Antibiotic resistance has risen dramatically because of antibiotic abuse or overuse owing to a lack of expert advice, placing individuals at a risk of getting antibiotic-resistant infections while receiving little medical benefit. The development of β-lactamase enzymes in microbes that bind and prevent β-lactams is the most crucial reason behind MDR. Another reason is the efflux pump that drags the antimicrobials outside the microbe and prevents antibiotics' dispersion into the cytoplasm.16 In addition, (a) changes in the pattern of protein related to plasma membrane, (b) ribosomal methylation thwarting the activity of antimicrobials, (c) the presence of stimuli for debasing medicines, (d) formation of biofilm, (e) protection to the genetic material of non-pathogenic species and so on are also contributing. In these ways, the pathogen becomes more damaging and challenging to eliminate.17 Lately, point mutation within A. baumannii has been cited as an important reason behind various degrees of resistance.18 It is a characteristic that helps the infection in evading antibiotic treatments, which leads to resistance, although it is still poorly understood. Consequently, it makes β-lactams, erythromycin, fluoroquinolones, tetracyclines, and other antibiotics ineffective.19 Because of point mutations, new variants with higher antibiotic sensitivity are developing from wild types. As oxacillinases appear to be abundant in A. baumannii, single point mutations in these enzymes impair extended-spectrum antibiotic's antibacterial activity that has been selected by the low antibiotic concentrations obtained through research.20
Along with all other factors, now multi-drug resistance has become a burning issue causing millions of deaths. A study reported that antibiotic resistance is responsible for around 7 lakhs of deaths annually in the world, which is assumed to rise to 10 million by 30 years if no action is taken.21 Despite being a substantial threat, pharmaceutical companies are still incapable of developing more potent and effective antibiotics. Economic problems, costly hygiene maintenance, and a few other causes have been attributed to this.22 Therefore, we design this study to determine the point mutations of oxacillinase producing multidrug-resistant A. baumannii at a molecular level.
According to a prior study, Oxa-23 develops antibiotic resistance by hydrolyzing Meropenem.23 In this study, we have collected oxacillinase variants of A. baumannii using Protein BLAST. The focus of this work is to uncover point mutations in the NCBI (National Center for Biotechnology Information) database that have been submitted from all around the world. From the dataset, 10 point mutations have been identified as Oxa-132, Oxa-219, Oxa-376, Oxa-377, Oxa-411, Oxa-530, Oxa-688, Oxa-693, Oxa-702, Oxa-880. Among the available antibiotics in the market, we have picked 7 antibiotics namely ampicillin, Cefaclor, Imipenem, Aztreonam, DB04293, Cefotaxime, and Doripenem for the study to test their affinity toward oxacillinases enzyme. RaptorX software was used to predict 3d structures of protein, which were then refined and validated in GalaxyRefine and the SAVES server. The binding affinity of these reference drugs was evaluated to assess the hydrolytic activity of antibiotics on these variants. Subsequently, Molecular Dynamics Simulation was done to demonstrate the stability and dynamic nature of the variants. Following a thorough analysis, we found the most damaging point mutations that might transform the native oxacillinase enzyme into a multidrug resistant variant. Nevertheless, in vitro and in vivo analysis is required to validate our hypothesis. Our findings could contribute to discovering novel multidrug resistance variants and therapeutic options for the multidrug-resistant Acinetobacter infections.
2 Methods and materials
2.1 Sequence retrieval utilizing BLAST (Basic Local Alignment Search Tool)
Depending on the similarity between amino acid sequences, all the variants of OXA-51 in A. baumannii were retrieved using pBLAST (https://blast.ncbi.nlm.nih.gov/Blast.cgi). pBLAST is a Basic Local Alignment Search Tool available at the National Center for Biotechnology (NCBI) homepage that is used for retrieving sequences. The program scans a protein sequence database as per “query” sequence, with subsequent comparison of nucleotide or protein sequences to the query sequence and calculating the statistical significance of the matches.24 In this pBLAST tool, the query sequence is usually an accession number (Q5QT35), gi(s), or FASTA sequence, and the search for variants was done using several parameters such as database type, organism genus, and species with specific taxid, algorithm, and a few other pertinent options.
2.2 Identification of point mutation
Antibiotic resistance could arise from genetic mutations of OXA-51. From the retrieved sequences of OXA-51, variants with point mutation were sorted out which is the focus of this study.
2.3 Identification of the effects of point mutation
We utilized five different online tools with distinct algorithm to determine the functional impact of point mutation. Due to point mutation, the function and structure of the protein might change. Three of them SIFT, PROVEAN and PolyPhen-2 servers were used to compute the functional impact and two of them, I-Mutant3.0 and PredyFlexy, servers were used to predict the structural impact on the variants.
2.3.1 Determination of the functional impacts of point mutation.
2.3.1.1 SIFT. The SIFT server (https://sift.bii.a-star.edu.sg/) uses evolutionary and sequence homology information to compare the deleterious and benign amino acid mutations.25 It assigns the mutations based on a cutoff number of 0.05. The result above this threshold is regarded as neutral, while a result under this threshold is categorized as detrimental.26
2.3.1.2 PolyPhen-2. PolyPhen-2 (http://genetics.bwh.harvard.edu/pph2/) categorizes the amino acid substitutions based on phylogenetic, sequence-based, and structural characteristics.27 A threshold score closer to 0.0 is considered benign and closer to 1.0 is regarded harmful. It classifies the data as benign, probably damaging or possibly damaging.28
2.3.1.3 PROVEAN. The PROVEAN online application (http://provean.jcvi.org/index.php) which classifies the deleterious and benign mutation considering a cutoff PROVEAN score.29 The threshold score of 2.5 is used to determine which mutations have detrimental impacts on biological functions. Mutations with a value less than 2.5 are predicted to have a negative impact on biological functions.30
2.3.2 Determination of the structural impacts of point mutation.
2.3.2.1 I-Mutant3.0. The I-Mutant3.0 software (http://gpcr2.biocomp.unibo.it/cgi/predictors/I-Mutant3.0/I-Mutant3.0.cgi) uses an SVM prediction model to estimate the strength and stability of a protein after a point mutation is expressed. By calculating the difference in Gibbs free energy (ΔΔG), it illustrates the variable durability among the protein variants. Gibbs free energy less than −1.0 kcal mol−1, shows destabilizing mutations, Gibbs free energy greater than 1.0 kcal mol−1, represents the stabilizing mutations and Gibbs free energy between −1 to 1 kcal mol−1, depicts the neutral mutations.31
2.3.2.2 PredyFlexy. Predyflexy (https://www.dsimb.inserm.fr/dsimb_tools/predyflexy/index.html) analyzes flexibility using two separate parameters, root mean square fluctuations, obtained from molecular dynamics simulation and the B-factor, retrieved from X-ray analysis.32 It identifies three types of characteristics: flexible, intermediate and rigid, based on the LSP (Line Spectrum Pair) model. Prediction of flexible, intermediate and rigid nature of protein is denoted by 2, 1, and 0 respectively.33
2.4 Prediction of protein structures (wild and mutant type) by structure prediction tool (RaptorX)
After screening out variants with a point mutation, RaptorX has been used for modeling protein structures. It is an online-based tool that predicts the structural conformation of a protein (http://raptorx.uchicago.edu/). RaptorX is a computational homology modeling tool that employs protein template information to improve alignment accuracy.34 It can predict 3D structures for protein sequences outside the Protein Data Bank (PDB) database. After the submission of the FASTA sequence as an input, RaptorX sets confidence scores to indicate the quality of each predicted 3D model and predicts its secondary and tertiary structures, contacts, solvent accessibility, disordered regions, and binding sites.
2.5 Refining and validating protein structures (GalaxyRefine and SAVES server)
Computer-based validation tool GalaxyRefine was used to check the accuracy of protein models generated by online structure prediction models. GalaxyRefine (http://galaxy.seoklab.org/refine) is such a template-based homology model quality checker that has passed the CASP10 assessment. The program facilitates the improvement of the protein models with more accurate local and side-chain conformation and also helps in lowering the free energy with short molecular dynamics simulation.35 For verifying the accuracy of protein models, SAVES server (https://saves.mbi.ucla.edu/) was utilized. It has a set of six tools that gives output like RAMACHANDRAN plot that provides relevant information and percentages about the conformation of residues in favored, allowed, and disallowed regions and is used for structural validation of protein. The Ramachandran plot has proven to be an efficient validation test for protein structure models regardless of the experimental or computational model.36 The percentage over 90% for favored regions is considered a good model. The VERIFY3D and ERRAT services were also used to evaluate the reliability of protein models.
2.6 Molecular docking by Autodock-vina
The process of estimating the binding affinity by molecular docking involves binding of a ligand with receptor binding sites. Autodock-vina (http://vina.scripps.edu) was utilized to predict the binding affinity and bound conformation.37 In this program, a scoring function is thought to mimic the system's customary chemical potentials.38 To assess the binding energy of ligands with receptor proteins, this tool uses the Lamarckian Genetic Algorithm and accepts the PDBQT molecular structure file format for both input and output. The term “exhaustiveness” refers to the repetitions of quantifying a protein's binding site which was set to 10 in this study. Fpocket service of Galaxy Europe server (https://usegalaxy.eu/) was used to determine ligand binding sites which help to generate a grid box.39 The very same application was used to create grid units of the desired size. The center of the grid box for Oxa-51 was fixed where X = 29.823, Y = 25.74, Z = −45.458 with a dimension of 50 × 62 × 54 Å and for Oxa-376 the coordinate was fixed X = 18.484, Y = 28.729, Z = 24.946, with a fixed dimension of 52 × 46 × 48 Å, and another variant Oxa-530 was fixed at X = 41.009, Y = −3.532, Z = 7.795, with a fixed dimension of 42 × 46 × 62 Å in the grid box.
2.7 Glide docking and MM-GBSA analysis
The Oxa-51 has no crystallographic structure to validate autodock-vina result. Therefore, we employed Schrodinger software to validate the docking score and interaction with different algorithm. Ampicillin, Cefaclor, Imipenem, Aztreonam, DB04293, Cefotaxime, and Doripenem antibiotics were processed in LigPrep application by including hydrogens, eliminating salts and incorporate ions at pH (7 ± 2.0).40 The 3d protein structures of native and mutants were prepared in a protein preparation module by filling missing side chains, incorporating cap termini, generating disulfide bonds, introducing hydrogen atoms and defining the bond orders.40 Energy minimization was led by employing the OPLS3e force field.41 Previously identified binding regions of the proteins were used to prepare the Grid box. The grid size was fixed utilizing the Receptor Grid Generation module with standard parameters of a van der Waals radius scaling measure of 1.0 and a charge cutoff of 0.25. The Glide docking of the Oxa-51 variants were performed in the Ligand docking module using GLIDE XP settings. A standard scoring value of 0.15 and van der Waals radius scaling value of 0.80 were preset to the drugs.
Docking score = a × vdW + b × Coul + Hbond + Metal + Lipo + BuryP + RotB + Site |
here, a and b are co-efficient standards for vdW and Coul, respectively, vdW = van der Waals energy, Coul = Coulomb energy, Hbond = hydrogen bonding binding to the protein, Metal = association with metal, Lipo = standard form for lipophilic, BuryP = buried polar group penalty, RotB = rotatable bond penalty and Site = active site polar interaction.42
To further elucidate the binding interaction of the protein-ligand complexes, we utilized the pose view structure to determine the binding free energy in Prime MM-GBSA utility. Energy minimization of the docking structures were completed in native optimization tool of the Prime utilizing OPLS3e force field.
ΔGbind (binding free energy) = ΔEMM + ΔGsolv + ΔGSA |
here, ΔEMM represents the reduced energy variations among the total of the energies of the protein and ligand and the complex structure of protein–ligand. Δ
Gsolv demonstrate the variation in the GBSA solvation energy of the complex structure of ligand–protein, and the sum of the salvation energies for the ligand and protein. ΔGSA highlights the variation in the energies for the surface area of protein–ligand and the sum of the surface area of the ligand and protein.
43
2.8 Molecular dynamics simulation of protein
Molecular dynamics simulation (MDS) is a powerful way of investigating the stability, structural change of protein. It is a useful method for establishing the physical basis of a structure by examining thermal averages of molecular characteristics and determining thermally accessible conformations.44 GROMACS (Groningen Machine for Chemical Simulation) (https://simlab.uams.edu/) was used to perform MDS on structures that used topologies, such as the GROMOS96 43a1 force field. This program allows for the solvation of a three-dimensional protein structure, followed by neutralization with NaCl ion (0.15 M).45 For energy minimization, GROMACS contains a neutralized triclinic box set system and 5000 steps of steepest descent algorithms. The equilibration stage was performed at 310 K and 1.0 bar with NVT (constant volume) and NPT (constant pressure) experimental settings, integrating an equilibration time-frame of 5000. It provides outputs as the root mean square deviation (RMSD), the root mean square fluctuation (RMSF), the radius of gyration (Rg), the solvent-accessible surface area (SASA), and hydrogen bonds (HBs).
2.9 Molecular dynamic simulation and post MM-GBSA analysis of protein–ligand complexes
Molecular dynamic simulation contributes to the understanding of the flexibility and stability of the protein–ligand docked structural complex.46 The MD simulation of Oxa-51_Doripenem, Oxa-376_Doripenem and Oxa-530_Doripenem complexes was conducted on Desmond application of Schrodinger.47 Utilizing the system builder module, these complexes were solvated on orthorhombic single point charge (SPC) water model. The protein and solvated area were separated by a minimum buffer distance of 10 Å. Then, the derived system was neutralized by introducing Na+ ions until it attained the human body physiological salt concentration of 0.15 M. The energy of final system was minimized utilizing the built-in OPLS3e force field. The MDS was performed incorporating the isothermal isobaric ensemble (NPT) at 310° K and 1.013 bar pressure. The simulation run of 100 nanoseconds (ns) was accompanied by a recording period of 100 picoseconds (ps), in which 1000 frames were written to the trajectory. Finally, we examined the trajectory utilizing Simulation Interaction Diagram (SID) tool, and the exported results included protein contact mapping, root mean square deviation (RMSD), and root mean square fluctuation (RMSF), and ligand properties. The thermal MM-GBSA. py script was used to carry out the post-simulation MM-GBSA evaluation of the Oxa-51 Doripenem, Oxa-376 Doripenem, and Oxa-530 Doripenem complexes (provided in the ESI†). The post-MM-GBSA evaluation of binding free energy evaluation was performed creating 0–1000 frame.48
2.10 Molecular dynamics simulation of Apo proteins by iMODS server
The iMODS facility (https://imods.iqfr.csic.es/) was used to investigate the combined movements of native and mutant protein structures in greater detail.49 This server incorporates improved combined phase presentation, a novel NMA (Normal Mode Analysis) solution in dihedral geometry, and multi-scale structure-building to broaden its validity.50 The iMODS service calculates the interior coordinates of native and mutant structures employing the NMA method to assess the stability. The protein's stability is indicated by the main-chain elastic network model, covariance matrix, variance, eigenvalue, B-factor, and deformability.
3. Result
3.1 Sequence retrieval using BLAST
In the pBLAST program, the FASTA format was used as a query sequence in the BLAST submission page and a search was made to obtain OXA-51 variants. Several criteria were used to obtain results for our investigation, such as specifying Acinetobacter baumannii limited by taxid: 470, selecting Whole-genome shotgun contigs (wgs) in the Database option along with a few other relevant choices. After submitting the protein's FASTA sequence to the Blast of NCBI site, mutants were retrieved, which were further analyzed in the progression of this investigation. A total of 348 named and unnamed variants were downloaded from NCBI as of 2020 (ESI†). Complete methodology of this study was provided in the Fig. 1.
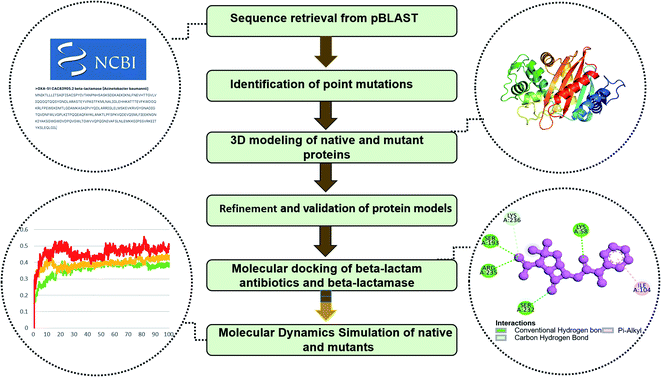 |
| Fig. 1 Complete workflow of the study. | |
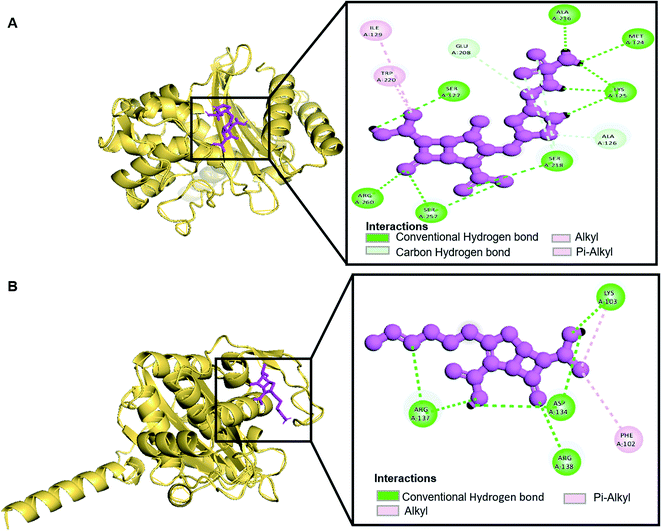 |
| Fig. 2 Schematic presentation of Oxa-51_Doripenem complex (A) and Oxa-51_Imipenem complex (B). | |
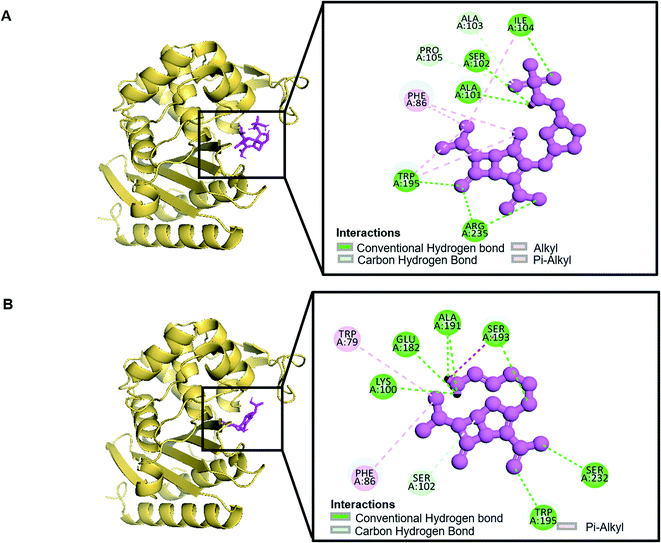 |
| Fig. 3 Schematic presentation of Oxa-376_Doripenem complex (A) and Oxa-376_Imipenem complex (B). | |
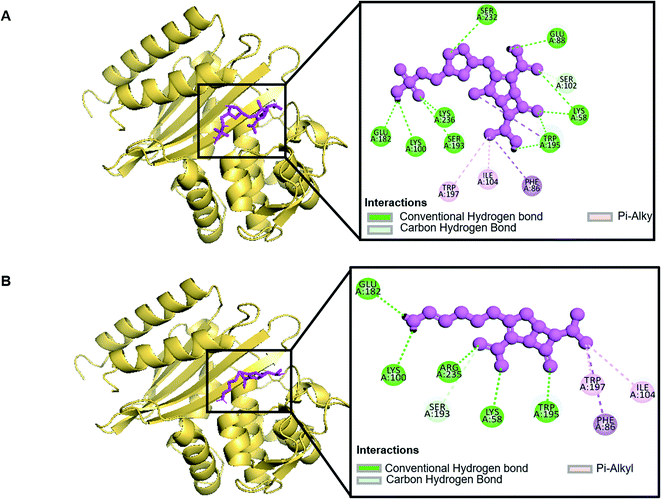 |
| Fig. 4 Schematic presentation of Oxa-530_Doripenem complex (A) and Oxa-530_Imipenem complex (B). | |
3.2 Identification of point mutations
After retrieving protein sequences, point mutations were identified for our study purpose. From 348 variants, 10 point mutations had been screened (Table 1). These mutations (Oxa-132, Oxa-219, Oxa-376, Oxa-377, Oxa-411, Oxa-530, Oxa-688, Oxa-693, Oxa-702, Oxa-880) in a single amino acid sequence may be damaging to the oxacillinase protein structures. Then, docking was carried out on these identified point mutations to reveal the fluctuation of binding affinity toward antibiotics.
Table 1 Details of selected point mutations of oxacillinase enzyme
Variant name |
GenBank ID |
Point mutation |
Oxa-51 |
CAC83905.2 |
Wild type |
Oxa-132 |
EU547447.1 |
I263M |
Oxa-219 |
NG_049517.1 |
L167V |
Oxa-376 |
NG_049667.1 |
H93Y |
Oxa-377 |
NG_049668.1 |
T51K |
Oxa-411 |
NG_049699.1 |
A96T |
Oxa-530 |
KX599398.1 |
T50S |
Oxa-688 |
NG_062310.1 |
P194Q |
Oxa-693 |
NG_062315.1 |
A216T |
Oxa-702 |
NG_062324.1 |
L85I |
Oxa-880 |
KY126224.1 |
D68N |
3.3 Prediction of the functional and effects of point mutation
To distinguish between harmful and benign point mutations, nine computational techniques employing various machine learning algorithms were built. The outcomes of these techniques, which are shown in the Table 2, were used to group the effects of point mutation on beta-lactamases. On SIFT server, all the variants except Oxa-693 showed TOLERATED effect on protein function. On the second functional server, PolyPhen-2, variants (OXA-132, Oxa-219, Oxa-376, Oxa-530, Oxa-693, Oxa-702) showed the threshold score closer to 1.0, indicating the deleterious effect. On the other hand, Oxa-377, Oxa-411, and Oxa-880 variants were predicted to be benign. Prediction from PROVEAN showed that Variants Oxa-219 and Oxa-693 had deleterious effects on protein function. On PredyFlexy server, variants Oxa-376 and Oxa-530 presented rigid flexibility of beta-lactamases. However, variants OXA-132, Oxa-219, Oxa-376, Oxa-377, Oxa-411, Oxa-530, Oxa-688, Oxa-693, and Oxa-702 demonstrated decreased stability on I-Mutant3.0 server.
Table 2 Functional and structural impact analysis by SHIFT, PolyPhen-2, PROVEAN, I-mutant, and PredyFlexy
Variants |
Mutation |
SHIFT |
PolyPhen-2 |
PROVEAN |
I-mutant (stability-RI) |
PredyFlexy |
OXA-132 |
I263M |
TOLERATED 0.07 |
PROBABLY DAMAGING—0.998 (sensitivity: 0.27; specificity: 0.99) |
Neutral = −1.163 |
Decrease 9 |
1-INTERMEDIATE |
Oxa-219 |
L167V |
TOLERATED 0.07 |
PROBABLY DAMAGING—0.993 (sensitivity: 0.70; specificity: 0.97) |
Deleterious = −2.881 |
Decrease 8 |
1-INTERMEDIATE |
Oxa-376 |
H93Y |
TOLERATED 0.23 |
POSSIBLY DAMAGING—0.953 (sensitivity: 0.79; specificity: 0.95) |
Neutral = −1.558 |
Decrease 7 |
2-FLEXIBLE |
Oxa-377 |
T51K |
TOLERATED 0.89 |
BENIGN—0.014 (sensitivity: 0.96; specificity: 0.79) |
Neutral = 0.221 |
Decrease 7 |
2-FLEXIBLE |
Oxa-411 |
A96T |
TOLERATED 0.27 |
BENIGN—0.145 (sensitivity: 0.92; specificity: 0.86) |
Neutral = −0.621 |
Decrease 2 |
1-INTERMEDIATE |
Oxa-530 |
T50S |
TOLERATED 0.11 |
POSSIBLY DAMAGING—0.851 (sensitivity: 0.59; specificity: 0.75) |
Neutral = −2.190 |
Decrease 7 |
2-FLEXIBLE |
Oxa-688 |
P194Q |
TOLERATED 0.41 |
BENIGN—0.087 (sensitivity: 0.93; specificity: 0.85) |
Neutral = 0.904 |
Decrease 8 |
2-FLEXIBLE |
Oxa-693 |
A216T |
AFFECT PROTEIN FUNCTION 0.03 |
PROBABLY DAMAGING—0.998 (sensitivity: 0.27; specificity: 0.99) |
Deleterious = −2.808 |
Decrease 4 |
1-INTERMEDIATE |
Oxa-702 |
L85I |
TOLERATED 0.59 |
POSSIBLY DAMAGING—0.902 (sensitivity: 0.82; specificity: 0.94) |
Neutral = −0.625 |
Decrease 8 |
0-RIGID |
Oxa-880 |
D68N |
TOLERATED 0.98 |
BENIGN—0.015 (sensitivity: 0.96; specificity: 0.79) |
Neutral = −0.907 |
Decrease 5 |
2-FLEXIBLE |
3.4 Comparative prediction of 3d structure of native and mutant proteins
Three-dimensional models were generated using point mutations predicted by the study. The tertiary model of proteins determines their ability to execute different functionality or connect with other compounds. In this project, we utilized the RaptorX web service to construct 11 homology models of native and variants. This web server incorporates a set of CASP13 and CAMEO protocols to develop 3D protein models. It used three template structures (4ZDX, 5KZH, and 5L2F) to generate the protein structures. Our wild-type and mutants exhibited TM values higher than 0.5.
3.5 Prediction of refined and validated protein models
The proposed models of beta-lactamase were uploaded to GalaxyRefine and SAVES webserver for structure refining and energy minimizing. It restored the disordered architectures of the protein models. Inside the SAVES system, the polished structures acquired via GalaxyRefine were checked to utilize PROCHECK, VERIFY3D, and ERRAT utility. The quality of the structures was predicted using the percentile score from Verify3D, the Quality factor from ERRAT, and the RAMACHANDRAN graph from PROCHECK. Three of them had a percentile score of more than 80%, a Quality factor of more than 90%, and amino acids in the most preferred areas of more than 90% (RAMACHANDRAN analysis) (Table 3) (ESI, Fig. 1–3†).
Table 3 Validation results of OXA-51, OXA-376, and OXA-530 variants using Verify3D, ERRAT, and PROCHECK service of SAVES server
Models |
Verify3D |
Errat |
PROCHECK |
Residues with 3D-1D score > = 0.2 |
Quality factor |
Most favoured regions |
Additional allowed regions |
Most favoured region + additional allowed region |
OXA-51 |
80.66% |
95.4887 |
92.1% |
7.1% |
99.2% |
OXA-376 |
97.19% |
95.279 |
90.3% |
9.7% |
100.0% |
OXA-530 |
85.54% |
95.671 |
93.1% |
5.5% |
98.6% |
3.6 Molecular docking by Autodock-vina
Molecular docking was performed in this study to determine the binding affinity and interaction between established beta-lactam antibiotics and beta-lactamase enzymes. For this study, 7 beta-lactam antibiotics of different genre such as Ampicillin, Cefaclor, Imipenem, aztreonam, Cefotaxime, Doripenem and a non-beta lactam inhibitor 7-(2-Amino-2-Phenyl-Acetylamino)-3-Chloro-8-Oxo-1-Aza-Bicyclo [4.2.0] Oct-2-Ene-2-Carboxylic Acid (CHEMBL3545874/DB04293) were chosen. As receptors, 10 variants of beta-lactamases have been screened which contain point mutation variants of OXA-51. As we are comparing the antibiotic resisting ability of the variants, OXA-51 has been chosen as the control. The strategy of this study was to compare the enzymatic activity of OXA-51 variants against these antibiotic drugs. Docking scores of the variants had been compared with the native, OXA-51 to identify the binding energy. Moreover, hydrogen bonds, bonding orders was identified while exporting docking output. The higher the binding energy is the more stable and stronger the binding of the ligand-receptor. Again, the more stable the ligand-receptor binding, the greater the enzymatic activity of Beta-lactamases. The binding affinity of Ampicillin, Cefaclor, Imipenem, aztreonam, DB04293, Cefotaxime, and Doripenem against native beta-lactamase enzyme (Oxa-51) was −6.5, −6.3, −5.5, −6.7, −6.4, −6.0, −6.4 kcal mol−1 respectively (Table 4). However, against the Oxa-376 and Oxa-530 variants, the binding affinity and nonbonded interaction of these antibiotics increased. For variant (Oxa-376), binding affinity of Ampicillin, Cefaclor, Imipenem, aztreonam, DB04293, Cefotaxime, and Doripenem maintained −7.1, −6.9, −6.5, −7.5, −7.6, −7.1, −7.2 kcal mol−1 respectively and for variant (Oxa-530), it remained −7, −6.7, −6, −6.6, −6.1, −6.6, −7.5 kcal mol−1 respectively (Tables 5 and 6). Against variant Oxa-880, antibiotics showed the lowest binding affinity and hydrogen bonds. The binding affinity of Ampicillin, Cefaclor, Imipenem, aztreonam, DB04293, Cefotaxime, and Doripenem decreased to −5.8, −5.3, −4.6, −7.8, −5.6, −5.7, −6.1 kcal mol−1 respectively. Docking results of other variants (Oxa-132, Oxa-219, Oxa-377, Oxa-411, Oxa-688, Oxa-693, Oxa-702) were provided in ESI (ESI, Tables 1–8†). Binding interactions of these protein-ligand complexes were provided in ESI, Fig. 4–21.† Doripenem showed the highest binding affinity and non-bonded interaction for Oxa-51, Oxa-376, and Oxa-530 variants. For Oxa-51, we found 11 (SER218, SER257, SER257, ARG260, LYS125, LYS125, ALA216, MET124, LYS125, SER127, SER218, SER218, ALA126, GLU208) hydrogen bonds and no hydrophobic bonds. On the other hand, Doripenem forms 11 hydrogen bonds (SER102, TRP195, SER232, ARG235, ARG235, LYS236, ALA101, ALA101, GLU182, SER102, SER193) and 2 hydrophobic bonds (PHE86, TRP195). Similarly, Doripenem forms 11 hydrogen bonds (LYS58, LYS58, SER193, TRP195, SER232, LYS236, LYS100, GLU182, GLU88, TRP195, SER102) and 3 hydrophobic bonds (TRP195, PHE86, TRP195).
Table 4 Binding affinity and nonbonded interaction between variant (Oxa-51) of oxacillinase enzyme and established antibiotics
Drugs |
Binding affinity (kcal mol−1) |
Number of H bond |
Interacting H bond with receptor (H-bond lowest distance) |
Ampicillin |
−6.5 |
8 |
VAL143, VAL143, LEU142 (2.14178), SER218,TRP220 (2.0013), GLY144, LYS83, LYS83 |
Cefaclor |
−6.3 |
7 |
LYS103 (1.85809), ASP134, ASP134, ARG137, ARG137, ARG137, ARG138 |
Imipenem |
−5.7 |
14 |
SER218, SER257, SER257, ARG260, LYS125, LYS125 (1.86524), ALA216, MET124, LYS125, SER127, SER218, SER218, ALA126, GLU208 |
Aztreonam |
−6.7 |
3 |
SER257 (2.37575), ARG260, ARG260 |
DB04293 |
−6.4 |
7 |
LYS83, TRP220, ARG260, ARG260, LYS125 (1.90849), SER218 |
Cefotaxime |
−6.0 |
8 |
LYS83, LYS83, SER218, SER257 (2.4812), ARG260, ARG260, SER257, LYS261 |
Doripenem |
−6.4 |
11 |
SER218, ARG260, LYS125 (1.86524), ALA216, MET124, LYS125, SER127, SER218, SER218, ALA126, GLU208 |
Table 5 Binding affinity and nonbonded interaction between variant (Oxa-376) of oxacillinase enzyme and established antibiotics
Drugs |
Binding affinity (kcal mol−1) |
Number of H bond |
Interacting H bond with receptor (H-bond lowest distance) |
Ampicillin |
−7.1 |
5 |
LYS58, LYS58, ARG235 (1.96773), ARG235, PRO87 |
Cefaclor |
−6.9 |
4 |
LYS58, ARG235 (1.96773), PRO87, ALA101 |
Imipenem |
−6.5 |
11 |
ILE104 (1.94426), SER193, SER232, ARG235, ARG235, ALA191, LYS100, GLU182, SER193, SER102, SER102 |
Aztreonam |
−6.5 |
5 |
SER232, SER232,ARG235 (2.03315), ARG235 |
DB04293 |
−7.6 |
4 |
LYS58, SER193, SER102 (2.22038), SER102 |
Cefotaxime |
−7.1 |
8 |
SER193 (2.01357), TRP195, SER232, ARG235, ARG235, LYS236, LYS236, GLU182 |
Doripenem |
−7.2 |
11 |
SER102, TRP195, SER232, ARG235, ARG235, LYS236, ALA101 (1.92811), ALA101, GLU182, SER102, SER193 |
Table 6 Binding affinity and nonbonded interaction between variant (Oxa-530) of oxacillinase enzyme and established antibiotics
Drugs |
Binding affinity (kcal mol−1) |
Number of H bond |
Interacting H bond with receptor (H-bond lowest distance) |
Ampicillin |
−7 |
5 |
LYS58, SER193, SER232 (2.14911), ARG235, LYS236 |
Cefaclor |
−6.7 |
7 |
LYS58, SER193, TRP195, ARG235 (1.93373), ARG235,SER193, LYS236 |
Imipenem |
−6 |
9 |
LYS58, LYS58, TRP195, ARG235, ARG235 (1.9691), LYS100, GLU182, SER193 |
Aztreonam |
−6.5 |
13 |
TRP195, SER232, SER232, ARG235, ARG235, ARG235, ARG235, SER102 (2.07985), LYS100, GLU182, SER193, SER193, SER193 |
DB04293 |
−6.1 |
4 |
SER193, ARG235 (2.03406), ARG235, SER193 |
Cefotaxime |
−6.6 |
11 |
LYS58, TRP197, SER232, ARG235, ARG235 (1.99628), ARG235, SER102, LYS100, GLU182, GLY194, TRP195 |
Doripenem |
−7.5 |
11 |
LYS58, LYS58, SER193, TRP195, SER232, LYS236 (1.99728), LYS100, GLU182, GLU88, TRP195, SER102 |
3.7 Evaluation of Glide and MM-GBSA scores
To validate the autodock-vina binding results of the Oxa-51, Oxa-376 and Oxa-530, we evaluated the glide and MM-GBSA scores and non-bonded interactions (Tables 7–10). The glide docking values of Aztreonam, Cefaclor, Doripenem, Ampicillin, DB04293, Imipenem, Cefotaxime against the native Oxa-51 was −3.878, −3.833, −3.8, −3.585, −3.244, −2.15, and −1.686 kcal mol−1. The docking score among the drugs and Oxa-376 and Oxa-530 variants increased same as Autodock-vina. For variant (Oxa-376), the glide values of Doripenem, Ampicillin, Imipenem, Cefotaxime, Cefaclor, Aztreonam, DB04293 were −6.132, −5.467, −5.021, −4.756, −4.426, −4.258, and −4.098 kcal mol−1. Again. for variant (Oxa-530), the glide values of Cefotaxime, Imipenem, Cefaclor, Doripenem, Aztreonam, Ampicillin, DB04293 were −5.666, −5.555, −4.784, −4.419, −4.259, −4.208, and −4.094 kcal mol−1. Following the glide score, we computed the MM-GBSA energies. The binding free energy of Aztreonam, Cefaclor, Doripenem, Ampicillin, DB04293, Imipenem, Cefotaxime against the native Oxa-51 was −36.4,9-26.13, −38.53, −29.98, −49.76, −12.29, and −14.4 kcal mol−1. For variant (Oxa-376), the binding free energy of Doripenem, Ampicillin, Imipenem, Cefotaxime, Cefaclor, Aztreonam, DB04293 were −44.12, −30.47, −37.65, −36.9, −39.58, −34.9, and −37.32 kcal mol−1. Again, for variant (Oxa-530), the binding free energy of Cefotaxime, Imipenem, Cefaclor, Doripenem, Aztreonam, Ampicillin, DB04293 was −41.48, −40.06, −46.64, −43.26, −44.02, −31.6, and −29 kcal mol−1. The non-bonded interaction between Doripenem and Oxa-51, Oxa-376, and Oxa-530 variants shows the maximum number of bonds. For Oxa-51, Doripenem interacts by 3 hydrogen bonds (GLU113, LYS125, GLU208) and 6 hydrophobic bonds (ALA126, ALA128, ILE129, PRO112, ILE206, TRP220). For Oxa-376, it forms 5 hydrogen bonds (ARG235, LYS236, LYS100, ALA101, SER102) and 7 hydrophobic bonds (PHE86, TRP79, TRP195, ALA101, ALA103, ILE104, PRO105). For Oxa-530, Doripenem forms 5 hydrogen bonds (ARG235, LYS236, SER102, LYS184, SER193) and 6 hydrophobic bonds (ILE189, ALA191, TRP195, ALA101, ALA103, ILE104) (Fig. 5–7).
Table 7 XP gscore and MM-GBSA scores between variant (Oxa-51) protein and established antibiotics
Drugs |
XP gscore (kcal mol−1) |
MM-GBSA scores (kcal mol−1) |
Aztreonam |
−3.878 |
−36.49 |
Cefaclor |
−3.833 |
−26.13 |
Doripenem |
−3.8 |
−38.53 |
Ampicillin |
−3.585 |
−29.98 |
DB04293 |
−3.244 |
−49.76 |
Imipenem |
−2.15 |
−12.29 |
Cefotaxime |
−1.686 |
−14.4 |
Table 8 XP gscore and MM-GBSA scores between variant (Oxa-376) protein and established antibiotics
Drug |
XP gscore (kcal mol−1) |
MM-GBSA scores (kcal mol−1) |
Doripenem |
−6.132 |
−44.12 |
Ampicillin |
−5.467 |
−30.47 |
Imipenem |
−5.021 |
−37.65 |
Cefotaxime |
−4.756 |
−36.9 |
Cefaclor |
−4.426 |
−39.58 |
Aztreonam |
−4.258 |
−34.9 |
DB04293 |
−4.098 |
−37.32 |
Table 9 XP gscore and MM-GBSA scores between variant (Oxa-530) protein and established antibiotics
Drug |
XP gscore (kcal mol−1) |
MM-GBSA scores (kcal mol−1) |
Cefotaxime |
−5.666 |
−41.48 |
Imipenem |
−5.555 |
−40.06 |
Cefaclor |
−4.784 |
−46.64 |
Doripenem |
−4.419 |
−43.26 |
Aztreonam |
−4.259 |
−44.02 |
Ampicillin |
−4.208 |
−31.6 |
DB04293 |
−4.094 |
−29.0 |
Table 10 Post dynamic MM-GBSA based binding free evaluation
Name of complex |
MM-GBSA (kcal mol−1) |
ΔGbind |
ΔGbind range |
Oxa-51_Doripenem |
−33.50 ± 7.50 |
−41.68 to −26.11 |
Oxa-376_Doripenem |
−41.22 ± 17.809 |
−59.038 to −23.42 |
Oxa-530_Doripenem |
−38.775 ± 6.085 |
−44.86 to −32.69 |
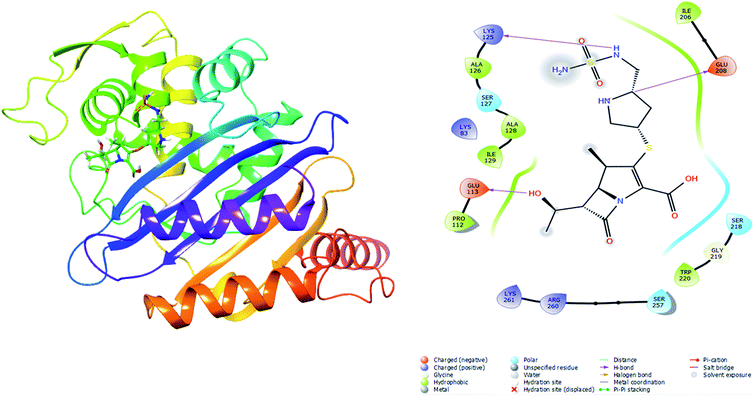 |
| Fig. 5 Illustration of Oxa-51_Doripenem complex from Schrodinger. | |
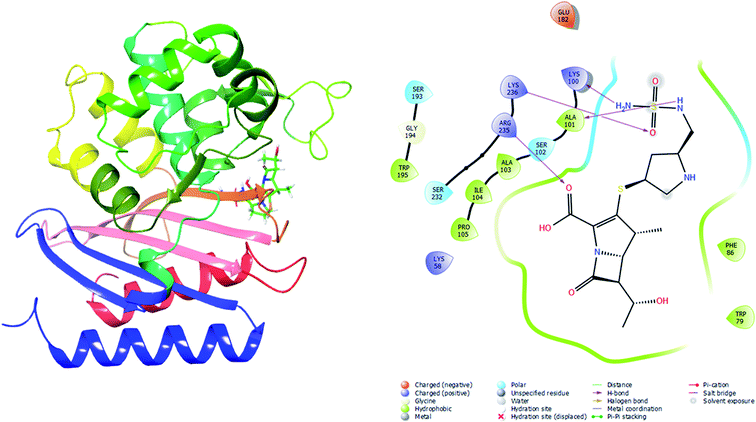 |
| Fig. 6 Illustration of Oxa-376_Doripenem complex from Schrodinger. | |
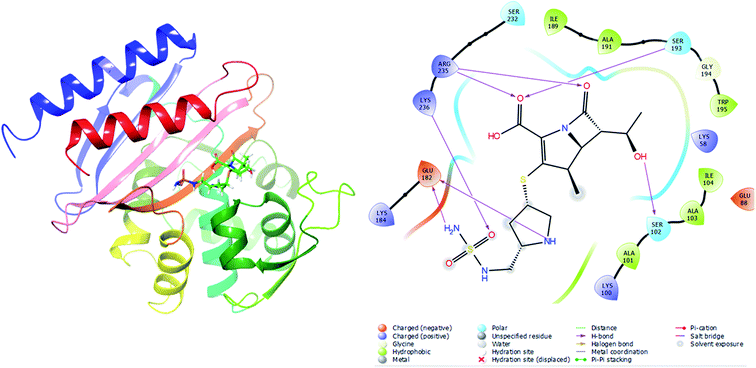 |
| Fig. 7 Illustration of Oxa-376_Doripenem complex from Schrodinger. | |
3.8 Molecular dynamics simulation of proteins by GROMACS
A molecular dynamics simulation of 100 ns was conducted to examine the stability and deviation of native and point mutated oxacillinase structures. Oxa-51, Oxa-376, and Oxa-530 variants had an average RMSD values of 0.36211013, 0.392086723, and 0.458128343 nm, respectively. Oxa-51 fluctuated between 0.15 to 0.4 nm. Oxa-51 exhibited the lowest RMSD, indicating that its conformation is more stable than Oxa-376 and Oxa-530 variants. Oxa-376 remained steady, though the fluctuation exceeded 0.4 nm after 75 ns, indicating destabilization. Oxa-530, on the other hand, demonstrated the largest destabilization, with a variation of more than 0.4 nm. Oxa-51 displayed a steady structure in the context of RMSF, except for the loop portions, where the fluctuation reached 0.6 nm. Oxa-51, Oxa-376, and Oxa-530 variants reported RMSF values ranging from 0.05 to 0.6 nm. From 214 to 222 amino acids, higher peaks were seen in the larger loop area. However, Oxa-376 and Oxa-530 mutants also oscillated above 0.4 nm other than the loop zone. Similarly, during the projected period, the average Rg values of the Oxa-376 and Oxa-530, Oxa-376 mutants were 1.68033965, 1.73529984, and 1.750554955 nm, respectively, indicating higher fluctuation in Oxa-376 and Oxa-530 structures. It suggested destabilization in mutant protein structures. In contrast to Oxa-51, the protein compactness may be disturbed in the Oxa-376 and Oxa-530 variants. According to H-bond analyses, the average number of H-bonds in native and mutant structures (Oxa-51, Oxa-376, and Oxa-530) were 183.0, 185.0, and 184.0, respectively. The difference in H-bond between the native and mutant structures suggested that these mutations could impair the native protein stability. An additional study in MDS was solvent accessible surface area (SASA), which revealed alteration of the surface area of proteins. The average SASA values of Oxa-51, Oxa-376, and Oxa-530 were 103.0, 113.0, and 111.0 nm2. So compared to Oxa-51, SASA studies revealed that following the point mutations (H93Y, T50S) in Oxa-376 and Oxa-530 variants might destabilize the native protein structures (Fig. 8).
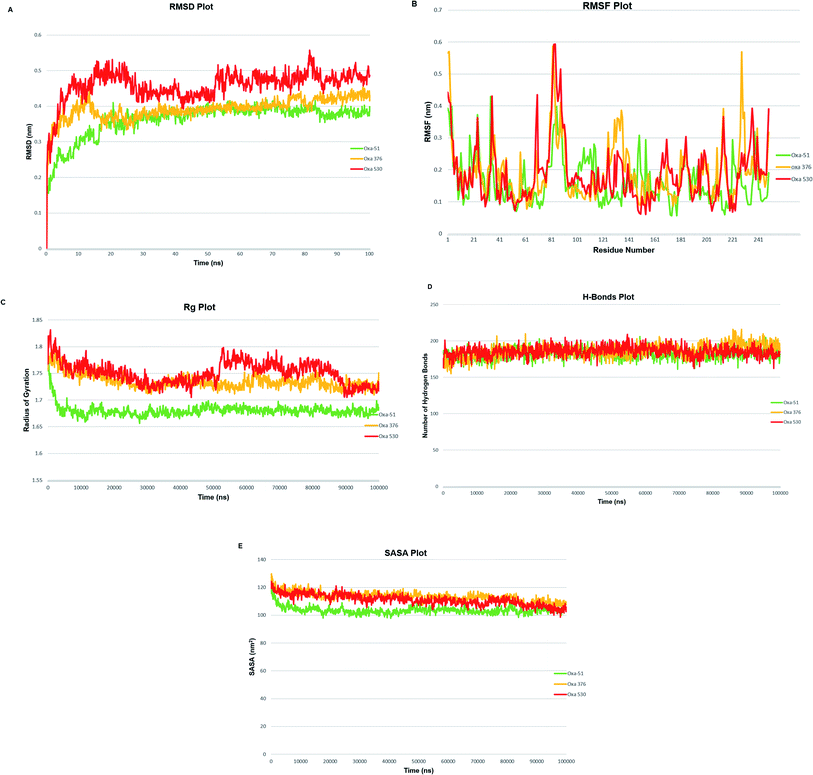 |
| Fig. 8 (A) Schematic presentation of Root Mean Square Deviation (RMSD) plot of Oxa-51 (Green), Oxa-376 (Yellow) and Oxa-530 (Red). (B) Schematic presentation of Root Mean Square Fluctuation (RMSF) plot of Oxa-51 (Green), Oxa-376 (Yellow) and Oxa-530 (Red). (C) Schematic presentation of Radius of Gyration (Rg) plot of Oxa-51 (Green), Oxa-376 (Yellow) and Oxa-530 (Red). (D) Schematic presentation of Hydrogen bonds (H-bonds) plot of Oxa-51 (Green), Oxa-376 (Yellow) and Oxa-530 (Red). (E) Schematic presentation of plot for Solvent Accessible Surface Area (SASA) of Oxa-51 (Green), Oxa-376 (Yellow) and Oxa-530 (Red). | |
3.9 Molecular dynamics simulation and post-simulation MM-GBSA analysis of protein–ligand complexes
Following molecular docking and MM-GBSA evaluation, we utilized the output protein–ligand complexes for MDS analysis. From the MD trajectory, we extracted RMSD, RMSF, ligand properties and protein–ligand contact mapping. Statistical results from RMSD graph depicts the stability of the protein–ligand complexes. The average RMSD values of Oxa-51, Oxa-376 and Oxa-530 were 6.319569431, 3.947480519, and 3.711052947 Å while the Doripenem had an average RMSD values of 17.40784416, 2.624325674, and 1.773090909 Å. After 2 ns, both Oxa-51 and Doripenem fluctuated above 4 Å, indicating that they were less stable during the 100 ns timescale. On the other hand, Doripenem and their complex with Oxa-376 and Oxa-530 remained under 4 Å. It revealed that Doripenem might be able to bind more firmly with the Oxa-376 and Oxa-530 enzymes. The RMSF data show the flexibility of protein structures. As presented in Fig. 9, the RMSF values of Oxa-376 and Oxa-530 did not vary much throughout the 100 ns timeframe except for the loop region, where the peak reached 4 Å and N-terminal area. However, Oxa-51 enzyme showed two large peaks near loop regions from 20 to 30 and 105 to 115 amino acids. It indicated that upon ligand binding, Oxa-51 enzyme demonstrated more fluctuation than its counterparts. The contacts between the Oxa-51_Doripenem, Oxa-376_Doripenem, and Oxa-530_Doripenem complexes were also observed during the 100 ns run. Hydrophobic, Hydrogen Bonds, Water Bridges and Ionic bonds were used to identify those interactions, as shown in Fig. 10. Doripenem produces LYS58 (Hydrogen Bonds, Water Bridges, and Ionic bonds), TRP89 (Hydrophobic, Hydrogen Bonds, and Water Bridges), lYS100 (Hydrogen Bonds and Water Bridges), ALA101 (Hydrogen Bonds and Water Bridges), SER193 (Hydrogen Bonds, Water Bridges, and Ionic bonds), TRP195 (Hydrophobic, Hydrogen Bonds, and Water Bridges), TRP197 (Hydrogen Bonds and Water Bridges), RG235 (Hydrogen Bonds and Water Bridges) bonds with Oxa-376 for 38%, 36%, 37%, 100%, 38%, 40%, 40%, and 35% of the simulation time (Fig. 10B). Similarly, Doripenem generates LYS58 (Hydrogen Bonds and Water Bridges), GLU88 (Hydrogen Bonds and Water Bridges), LYS100 (Hydrogen Bonds and Water Bridges), ALA101 (Hydrogen Bonds and Water Bridges), SER102 (Hydrogen Bonds and Water Bridges), SER193 (Hydrogen Bonds and Water Bridges), TRP195 (Hydrophobic, Hydrogen Bonds, and Water Bridges), TRP197 (Hydrophobic, Hydrogen Bonds, and Water Bridges), and GLN202 (Hydrogen Bonds and Water Bridges) bonds with Oxa-530 for 40%, 40%, 20%, 22%, 41%, 38%, >100%, 24%, and 79% of the simulation time (Fig. 10C). However, Doripenem had reduced interaction creating GLY106 (Hydrogen Bonds and Water Bridges), PHE111 (Hydrophobic, Hydrogen Bonds, and Water Bridges), PRO112 (Hydrogen Bonds and Water Bridges), TRP114 (Hydrophobic, Hydrogen Bonds, and Water Bridges) bonds with Oxa-51 for 25%, >100%, 37%, and 60% of the simulation time (Fig. 10C). Protein contacts with Doripenem demonstrated that Oxa-376_Doripenem, and Oxa-530_Doripenem complexes were stable forming plethora of non-bonded interaction, whereas Oxa-51_Doripenem might be less stable creating less bonds. The average free binding energy (ΔG) of Oxa-51_Doripenem, Oxa-376_Doripenem, and Oxa-530_Doripenem is −33.50 ± 7.50, −41.22 ± 17.809, and −38.775 ± 6.085 kcal mol−1. When compared to post dynamic MM-GBSA estimations, the pre dynamic MM-GBSA evaluation yielded an identical binding free energy value.
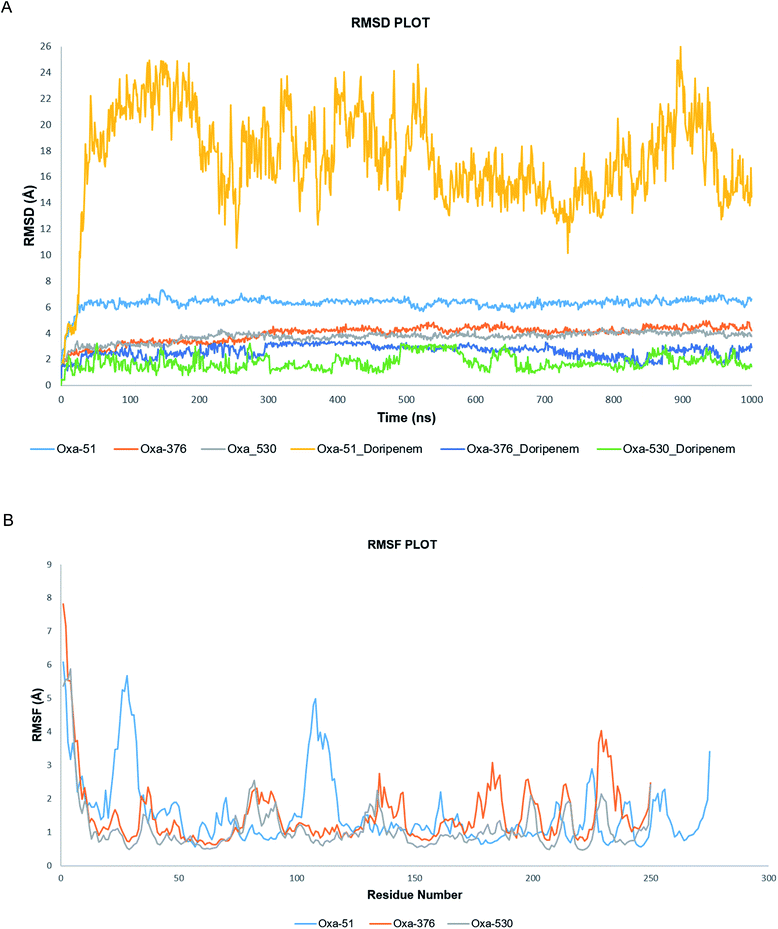 |
| Fig. 9 (A) Simulation plot of Root Mean Square Deviation (RMSD) showing Oxa-51 (Light Blue), Oxa-376 (Orange) and Oxa-530 (Grey), Oxa-51_Doripenam (Yellow), Oxa-51_Doripenam (Dark Blue), Oxa-51_Doripenam (Green). (B) Simulation plot of outcomes as Root Mean Square Fluctuation (RMSF) showing Oxa-51 (Light Blue), Oxa-376 (Orange) and Oxa-530 (Grey). | |
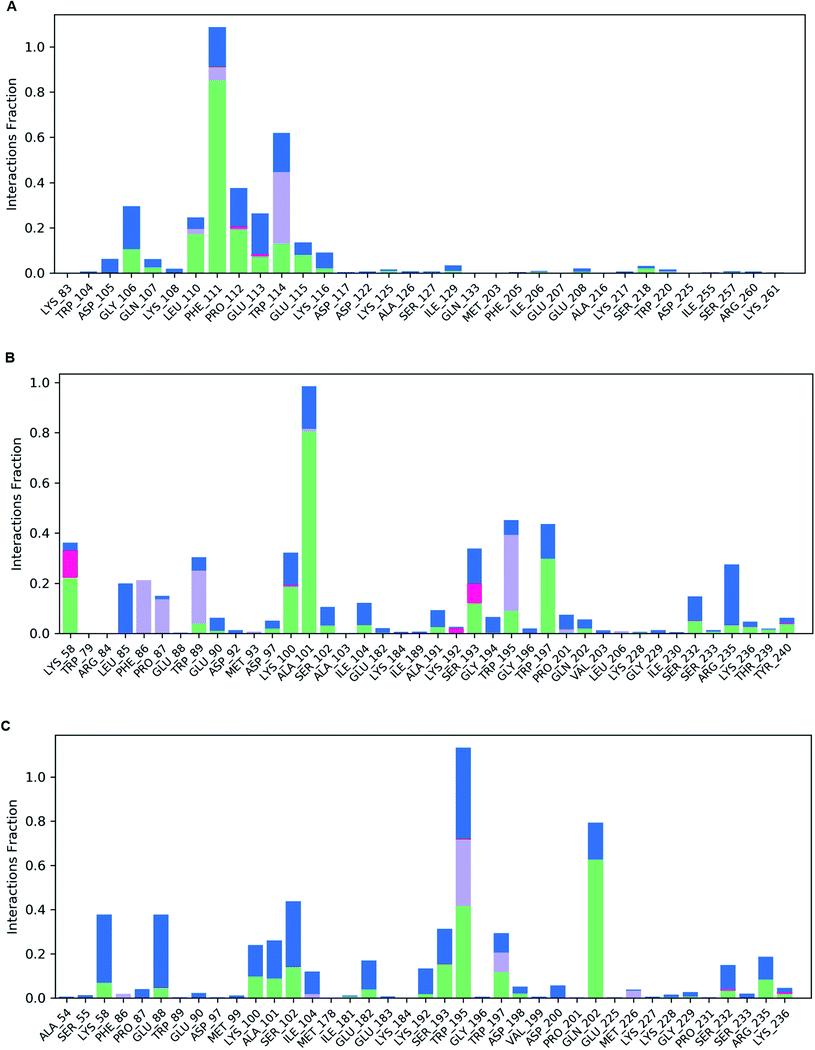 |
| Fig. 10 (A) Illustration of protein–ligand contact map of Oxa-51_Doripenem complex. (B) Illustration of protein–ligand contact map of Oxa-376_Doripenem complex. (C) Illustration of protein–ligand contact map of Oxa-530_Doripenem complex. | |
3.10 Molecular dynamics simulation analysis of Apo proteins by iMODS server
The MD simulation evaluation by the imods server was performed to compare the motions of wild-type and mutant protein structures (Fig. 11A–C). The deformability graph depicts the deformability scores, with greater deformability evident mostly in flexible hinge areas. The deformability graph showed that Oxa-376 had the highest fluctuation in deformability index with two peaks above 1. The B-factor evaluation typically yields an overall RMS score. Oxa-51 displayed a consistent B-factor score, but Oxa-376 and Oxa-530 fluctuated more and each had a large peak. The Eigen values describe the movement rigidity, that is directly connected to the energy it takes to flex the protein. The protein structure will deform most effectively at a shorter range of energies. The Eigen value and the variance map are inversely related. Here, the Oxa-51 had the lowest eigen score of 3.6388540406 × 10−5 compared to the eigen score of 3.0890868041 × 10−4 and 4.5085523818 × 10−4 of Oxa-376 and Oxa-530. The Covariance matrix between paired atoms and the Elastic network model represents the molecular correlation and attachment of atoms with spring. There is a gradual decrease in red color in the graphs of Oxa-376 and Oxa-530. It indicates that the correlation among the paired residues is reduced in the mutant structures.
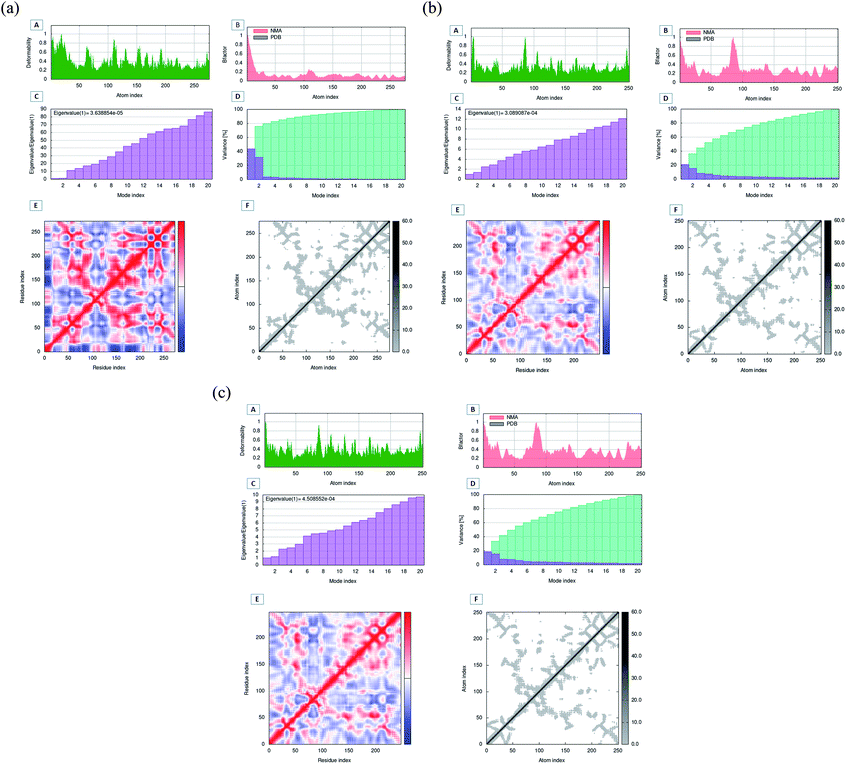 |
| Fig. 11 (A) Molecular dynamics simulation analysis of Oxa-51 protein structures of native and mutant models. (B) Schematic presentation of molecular dynamics simulation analysis of Oxa-376 protein structures of native and mutant models. (C) Molecular dynamics simulation analysis of Oxa-530 protein structures of native and mutant models. | |
4 Discussion
Oxacillinases (beta-lactamases) are known as one of the most important beta-lactam antibiotic degrading enzymes causing life-threatening problems in the vulnerable parts of the world. The presence of oxacillinases in bacteria damages the activity and structure of medications. It is also fundamental for causing antibiotic resistance. The negative impact of A. baumannii generating oxacillinases in illnesses has been studied using several methods.51 We devised our in silico study to figure out which Oxa-51 variants were the most harmful and how they contributed to the development of multidrug resistance.
Several disease-related computational techniques were utilized to distinguish between harmful and benign variants. A thorough examination of pBLAST database yielded the identification of several Oxa-51 variants. About 348 variants of Oxa-51 have been identified using the pBLAST program, and this data provided a total of 10 variants (Oxa-688, Oxa-376, Oxa-377, Oxa-880, Oxa-219, Oxa-411, Oxa-132, Oxa-693, Oxa-530, Oxa-702) containing single mutations at P194Q, H93Y, T51K, D68N, L167V, A96T, I263M, A216T, T50S, L85I, respectively. The newfound enzymes are OXA-51 variants that have developed multidrug resistance due to a single-point mutation. As a result, it implies that point mutation is the key determinant for drug resistance with a broad spectrum.52
Utilizing combination of disease and function-related bioinformatics strategies, we separated the dangerous point mutation types from tolerated or neutral ones. To ascertain whether the detected mutations were detrimental or benign, a total of five functional and structural tools (SIFT, PROVEAN, PolyPhen-2, I-Mutant3.0 and PredyFlexy) were used. Out of the 10 variants, expression of the Oxa-376 and Oxa-530 variants had a negative impact on the native protein's structure and function. However, all of the variations had either a functional or structural effect on the protein. For protein modeling, we chose all of the variants.
After filtering point mutations, we have used RaptorX, GalaxyRefine to build and refine the selected mutants. Then, through SAVES server, we utilized Verify3D, PROCHECK, and ERRAT modules to verify the protein models. Ramachandran plot, Quality Factor, and VERIFY3D score were evaluated to confirm the structural accuracy of the modeled proteins. These models provided the template to assess the hydrolytic capability of beta-lactamase against the established antibiotics.
Protein stability affects the affinity of ligands for proteins, therefore maintaining protein stability is essential for a protein's function and activity.53 As a result, molecular docking was used to evaluate the hypothesis regarding ligand inhibition of the target by calculating ligand-receptor binding energy, which is extremely significant in drug design.54 In the presence of antibiotics, many studies used molecular docking to test the hydrolytic activity of beta lactamase enzymes.55 To determine the extent of affinity between 7 antibiotics (Ampicillin, Aztreonam, Cefaclor, Cefotaxime, Doripenem, Imipenem, and DB04293 (CHEMBL3545874)) and oxa-51 variants, molecular docking was done using Autodock-vina and Galaxy Europe server (for grid box generation). Among the variants, Oxa-376 and Oxa-530 showed the highest affinity for all antibiotics out of 10 mutants, indicating the highest hydrolyzing capability of these variants. In this study, we compared the variants with the wild type protein by calculating binding affinity and the free energy contributed by H-bonds. The hydrogen bonding process involves the creation of hydrogen bonds formed by the proteins with water and the formation of new ones with the ligand in the protein–ligand complex.56 So, the higher the number of H-bonds, the better a protein's ability to create stronger ligand binding. This increased association indicates that beta-lactamase enzymes are breaking down the β-Lactam antibiotics more potently. One of our targeted mutants Oxa-376 and Oxa-530 showed higher binding affinity and H-bond with antibiotics than the Oxa-51 variant. Against Ampicillin, Cefaclor, Imipenem, Aztreonam, DB04293, Cefotaxime, and Doripenem antibiotics, Oxa-376 produced 4, 5, 11, 5, 4, 8, 11 and Oxa-530 produced 5, 7, 9, 13, 4, 11, 11 hydrogen bonds, respectively. As a result of the increased affinity and H-bonds created by Oxa-376 and Oxa-530, these point mutations could alter of the structure of the Oxa-51 enzymes and escalation of hydrolytic activity. Previous study of the effect of antibiotics Imipenem and Doripenem on variants of oxacillinases showed that Imipenem and Doripenem had a MIC (Minimum inhibitory concentration) value of 2 and 8 μg mL−1. This revealed that Imipenem and Doripenem, at small concentrations, had the ability to destroy A. baumannii more effectively.55 According to our findings, binding affinity of Imipenem and Doripenem against the variants (Oxa-376, Oxa-530) increased (Fig. 2–4). This supports the hypothesis that the variants (Oxa-376, Oxa-530) were the most alarming of the ones studied. However, other variants (Oxa-132, Oxa-219, Oxa-376, Oxa-377, Oxa-411, Oxa-530, Oxa-688, Oxa-693, Oxa-702, Oxa-880) did not show change of binding affinity. Other antibiotics (Ampicillin, Cefaclor, aztreonam, DB04293, and Cefotaxime) also presented an increased binding affinity towards the most damaging variants (Oxa-376, Oxa-530). These results conclude that mutants (Oxa-376, Oxa-530) could be the variants of concern. Apart from these mutations, variant Oxa-132 had the closest affinity for all antibiotics studied. With antibiotics Ampicillin, Cefaclor, Imipenem, aztreonam, DB04293, Cefotaxime, and Doripenem, variant Oxa-880 showed the lowest binding affinity of all, with reduced hydrogen bonds of 3, 4, 1, 4, 4, 4, 4, 10, respectively. On the other hand, variants (Oxa-219, Oxa-376, Oxa-377, Oxa-411, Oxa-530, Oxa-688) exhibited no change in interaction when compared to Oxa-51. It corroborates our theory that mutations in the 93rd (Oxa-376) and 50th (Oxa-530) locations of beta-lactamase might dramatically increase hydrolyzing activity.
To validate our earlier results, we used separate docking suite, Schrodinger software to obtain the Glide docking and MM-GBSA scores. Previously, we found increased affinity for Oxa-376, Oxa-530 variants against established antibiotics. In case of Oxa-376, Oxa-530 variants the glide XP score range was −6.132 to −4.098 kcal mol−1 and −5.666 to −4.094 kcal mol−1. But the glide XP results decreased between −3.878 to −1.686 for Oxa-51. The free binding energy (MM-GBSA score) were equivalent to glide XP score. We selected Oxa-51_Doripenem, Oxa-376_Doripenem and Oxa-530_Doripenem complexes for MD simulation by mimicking physiological state of human body to further support our findings. The atoms in a biomolecule are continuously moving, and molecular dynamic simulation has an impact on the dynamic nature of the biomolecule. It influences the target's structural and functional relationships along with protein–ligand interactions that are crucial for therapeutic research.57 Throughout the MD simulation, five parameters have been analyzed to measure the robustness of the protein structures. These parameters are the root-mean-square deviation (RMSD) of enzyme and the root-mean-square fluctuation (RMSF), Rg analysis, the H-bond analysis, Solvent accessible surface area (SASA) analysis.46 To compare macromolecular structure and dynamics, RMSD is a well-known option of determining the equilibration period and stability of the protein by comparing it with wild-type protein structure in MD simulations.42 As per The RMSD graph, Oxa-376 and Oxa-530 showed less stability when compared with the wild-type. Oxa-51 fluctuated below 0.4 ns during the 100 ns period of simulation where the two variants Oxa-376 and Oxa-530 fluctuated above 0.4 ns. As the higher RMSD value indicates the less stable structure, Oxa-376, and Oxa-530 are both unstable, with the latter being the more unstable. Previous simulation study on RMSD value showed stability of the Oxa-51 protein structure.55 RMSF is measured similarly to RMSD, which accounts for flexibility for every individual amino acid. When compared to Oxa-51, the variants Oxa-376 and Oxa-530 oscillated more than the wild type. Their fluctuation exceeding 0.4 nm indicated that the protein structure was extensively destabilized by the point mutations (H93Y, T50S). Another simulation parameter is Rg or Radius of gyration analysis. By comparing the radius of gyration throughout the Md simulation, we can assess the degree of compactness in the folding and unfolding of the protein.58 Rg value in our study revealed that point mutation modified the protein as Oxa-376 and Oxa-530 were more destabilizing than Oxa-51. Oxa-376 and Oxa-530 variants might lose compactness through these point mutations (H93Y, T50S). Again, the fourth parameter in the simulation process was H-bond. An essential property of protein is its H-bonding, which is responsible for its binding interaction and conformation. H-bonds can form large networks in the internal structure of proteins, connecting different sections.59 As a result, the stronger the affinity between protein and ligand, the more hydrogen bonds must be present. However, the average values of hydrogen bonds did not change for the protein structures (Oxa-51, Oxa-376 and Oxa-530). Another parameter, SASA, also revealed equivalent results in the present study. When studying the dynamic nature of the protein, the solvation free energy changes due to interaction must be taken into consideration.60 Throughout the denaturation step, the protein unfolds, exposing non-polar hydrophobic contacts to the aquatic environment. It destabilizes the protein's structure. The computational analysis of SASA shows the level of protein solvent accessibility. SASA values of this study highlighted that Oxa-376 and Oxa-530 variants fluctuated more than the native protein. This indicated that the mutations (H93Y, T50S) might destabilize the wild protein. As a result, we inferred from the simulation study that the Oxa-376 and Oxa-530 proteins have a deleterious effect on the Oxa-51 protein's structure and function. So, the plots of the MD simulation confirmed that the prediction of point mutations could destabilize the native structure of beta-lactamase. Simulation results from imods webserver also suggest Oxa-376 and Oxa-530 are more destabilizing than the Oxa-51. There is less deformability in the Oxa-51 structure. Similarly, the B-factor score reveals that Oxa-376 and Oxa-530 are more destabilizing. The protein Oxa-51 exhibited the lowest Eigen score, indicating motion stiffness in the structure and lower energy requirement for protein structure deformation.
Validation of the complex structures showed similar results as the protein structures (Oxa-51, Oxa-376 and Oxa-530) in Desmond. The RMSD plot from Desmond displayed that Oxa-376 and Oxa-530 had higher stability than the native structure upon binding with Doripenem. The RMSF results indicated that Oxa-51 had higher fluctuation compared to the other variants. On the other hand, Oxa-51 might have higher binding affinity for Doripenem. Protein–ligand contact map presented a clear binding scenario where the number of Hydrophobic, Hydrogen Bonds, Water Bridges, ionic bonds were higher in Oxa-376 and Oxa-530 variants compared to Oxa-51. LYS58, TRP89, lYS100, ALA101, SER193, TRP195, TRP197, ARG235 contributed to the highest percentage of simulation time for Oxa-376. LYS58, GLU88, LYS100, ALA101, SER102, SER193, TRP195, TRP197, GLN202 amino acids remained associated with Oxa-530. However, the number reduced to GLY106, PHE111, PRO112, TRP114 amino acids for Oxa-51. It implies that Oxa-376 and Oxa-530 binds more firmly with Doripenem. In the post-simulation part, MM-GBSA was vital to for flexible protein–ligand binding. Table 10 showed that Post dynamic MM-GBSA of Oxa-376_Doripenem and Oxa-530_Doripenem was −41.22 ± 17.809 and −38.775 ± 6.085 kcal mol−1 which decreased to −33.50 ± 7.50 kcal mol−1 for Oxa-51. Therefore, the MD simulation of protein–ligand complexes again verifies that our hypothetical findings of mutations might cause antibiotic resistance.
To determine the impact of a point mutation on the configuration of the oxacillinase enzyme, we used a series of computational tools. Recently, many drug design-related studies are using similar methodologies to uncover significant variants and thus disease-related information at the molecular level. Identification of point mutations (H93Y, T50S) in Oxa-376 and Oxa-530 mutants might reveal the most significant variant of beta-lactamase. As a result, this study is expected to make a substantial contribution to finding the most damaging mutations of beta-lactamases in the Acinetobacter family that might cause antibiotic resistance. Additionally, it would aid in multidrug resistance therapeutic study.
5 Conclusion
The current work focuses on antibiotic resistance traits in A. baumannii based on the hydrolyzing framework. The research draws the correlation between the binding strength of the antibiotics with beta-lactamase variants (Oxa-132, Oxa-219, Oxa-376, Oxa-377, Oxa-411, Oxa-530, Oxa-688, Oxa-693, Oxa-702, Oxa-880). Molecular docking results of wild-type and mutants with antibiotics suggested that Oxa-376 and Oxa-530 variants might increase the hydrolytic activity of beta-lactamase against the antibiotics. Subsequent evaluation using MD simulation revealed that point mutations (H93Y, T50S) on the native protein might disrupt the structural and functional conformation of beta-lactamase. Additionally, the study demonstrates that traditional beta-lactam antibiotics are losing their ability to cleave these beta-lactamase variants. Sufficient in vitro and in vivo investigations are recommended to validate our results and determine the complex mechanisms of antibiotic resistance on Oxa-376 and Oxa-530 variants of beta-lactamase. Nevertheless, our findings could provide the groundwork for future therapeutic development against A. baumannii multidrug-resistant strains.
Data availability
The datasets supporting the conclusions of this study are included within the article (and its additional files).
Author contributions
Conceptualization: Sajal Kumar Halder, Mahbubul Kabir Himel, Dr Aparna Shil. Investigation: Sajal Kumar Halder, Maria Mulla Mim, Aparna Shil, Mahbubul Kabir Himel. Methodology: Sajal Kumar Halder, Maria Mulla Mim, Md. Meharab Hassan Alif, Jannatul fardous shathi, Mahbubul Kabir Himel. Project administration: Mahbubul Kabir Himel, Dr Aparna Shil. Resources: Mahbubul Kabir Himel. Software: Sajal Kumar Halder, Maria Mulla Mim, Md. Meharab Hassan Alif, Jannatul fardous shathi. Supervision: Mahbubul Kabir Himel, Dr Aparna Shil. Writing—original draft: Sajal Kumar Halder, Maria Mulla Mim, Dr Aparna Shil.
Conflicts of interest
There are no conflicts of interest to declare.
References
- L. L. Maragakis and T. M. Perl, Clin. Infect. Dis., 2008, 46, 1254–1263 CrossRef CAS PubMed.
- A. Y. Peleg, H. Seifert and D. L. Paterson, Clin. Microbiol. Rev., 2008, 21, 538–582 CrossRef CAS PubMed.
- F. Perez, A. M. Hujer, K. M. Hujer, B. K. Decker, P. N. Rather and R. A. Bonomo, Antimicrob. Agents Chemother., 2007, 51, 3471–3484 CrossRef CAS PubMed.
- S. B. Almasaudi, Saudi J. Biol. Sci., 2018, 25, 586–596 CrossRef PubMed.
- L. Dijkshoorn, A. Nemec and H. Seifert, Nat. Rev. Microbiol., 2007, 5, 939–951 CrossRef CAS PubMed.
- G. M. Cerqueira and A. Y. Peleg, IUBMB Life, 2011, 63, 1055–1060 CrossRef CAS PubMed.
- M.-F. Lin and C.-Y. Lan, World J. Clin. Cases, 2014, 2, 787–814 CrossRef PubMed.
- S. Bagheri Josheghani, R. Moniri, F. Firoozeh, M. Sehat and Y. Dasteh Goli, J. Pathog., 2015, 2015, 957259 Search PubMed.
- D. Aubert, L. Poirel, J. Chevalier, S. Leotard, J. M. Pages and P. Nordmann, Antimicrob. Agents Chemother., 2001, 45, 1615–1620 CrossRef CAS PubMed.
- S. Brown and S. Amyes, J. Antimicrob. Chemother., 2006, 57, 1–3 CrossRef CAS PubMed.
- S. Brown, H. K. Young and S. G. B. Amyes, Clin. Microbiol. Infect., 2005, 11, 15–23 CrossRef CAS PubMed.
- V. Tiwari, I. Nagpal, N. Subbarao and R. R. Moganty, J. Mol. Model., 2012, 18, 3351–3361 CrossRef CAS PubMed.
- N. Pandey and M. Cascella, in StatPearls, StatPearls Publishing, Treasure Island (FL), 2022 Search PubMed.
- W. A. Agger, S. M. Callister and D. A. Jobe, Antimicrob. Agents Chemother., 1992, 36, 1788–1790 CrossRef CAS PubMed.
- S. Shaikh, J. Fatima, S. Shakil, S. M. D. Rizvi and M. A. Kamal, Saudi J. Biol. Sci., 2015, 22, 90–101 CrossRef CAS PubMed.
- E. E. Gill, O. L. Franco and R. E. W. Hancock, Chem. Biol. Drug Des., 2015, 85, 56–78 CrossRef CAS PubMed.
- A. Howard, M. O'Donoghue, A. Feeney and R. D. Sleator, Virulence, 2012, 3, 243–250 CrossRef PubMed.
- V. Manchanda, S. Sanchaita and N. Singh, J. Global Infect. Dis., 2010, 2, 291–304 CrossRef PubMed.
- M. L. Beeton, V. J. Chalker, N. C. Maxwell, S. Kotecha and O. B. Spiller, Antimicrob. Agents Chemother., 2009, 53, 2020–2027 CrossRef CAS PubMed.
- J. Blazquez, M. I. Morosini, M. C. Negri, M. Gonzalez-Leiza and F. Baquero, Antimicrob. Agents Chemother., 1995, 39, 145–149 CrossRef CAS PubMed.
- E. Temkin and Y. Carmeli, Clin. Infect. Dis., 2019, 69, 2029–2034 CrossRef PubMed.
- C. L. Ventola, Pharm. Ther., 2015, 40(4), 277–283 Search PubMed.
- J. Sgrignani, G. Grazioso and M. De Amici, Biochemistry, 2016, 55, 5191–5200 CrossRef CAS PubMed.
- D. Wheeler and M. Bhagwat, Methods Mol. Biol., 2007, 395, 149–176 CrossRef CAS PubMed.
- P. C. Ng and S. Henikoff, Nucleic Acids Res., 2003, 31, 3812–3814 CrossRef CAS PubMed.
- P. Kumar, S. Henikoff and P. C. Ng, Nat. Protoc., 2009, 4, 1073–1081 CrossRef CAS PubMed.
- I. A. Adzhubei, S. Schmidt, L. Peshkin, V. E. Ramensky, A. Gerasimova, P. Bork, A. S. Kondrashov and S. R. Sunyaev, Nat. Methods, 2010, 7, 248–249 CrossRef CAS PubMed.
- E. Capriotti, R. Calabrese and R. Casadio, Bioinformatics, 2006, 22, 2729–2734 CrossRef CAS PubMed.
- Y. Choi, G. E. Sims, S. Murphy, J. R. Miller and A. P. Chan, PLoS One, 2012, 7, e46688 CrossRef CAS PubMed.
- S. Kato, S.-Y. Han, W. Liu, K. Otsuka, H. Shibata, R. Kanamaru and C. Ishioka, Proc. Natl. Acad. Sci. U. S. A., 2003, 100, 8424–8429 CrossRef CAS PubMed.
- E. Capriotti, P. Fariselli and R. Casadio, Nucleic Acids Res., 2005, 33, W306–W310 CrossRef CAS PubMed.
- C. Benros, A. G. de Brevern, C. Etchebest and S. Hazout, Proteins, 2006, 62, 865–880 CrossRef CAS PubMed.
- A. Bornot, C. Etchebest and A. G. de Brevern, Proteins, 2009, 76, 570–587 CrossRef CAS PubMed.
- J. Peng and J. Xu, Proteins, 2011, 79(Suppl. 10), 161–171 CrossRef CAS PubMed.
- L. Heo, H. Park and C. Seok, Nucleic Acids Res., 2013, 41, W384–W388 CrossRef PubMed.
- F. Carrascoza, S. Zaric and R. Silaghi-Dumitrescu, J. Mol. Graphics Modell., 2014, 50, 125–133 CrossRef CAS PubMed.
- D. Seeliger and B. L. de Groot, J. Comput.-Aided Mol. Des., 2010, 24, 417–422 CrossRef CAS PubMed.
- O. Trott and A. J. Olson, J. Comput. Chem., 2010, 31, 455–461 CAS.
- V. Le Guilloux, P. Schmidtke and P. Tuffery, BMC Bioinf., 2009, 10, 168 CrossRef PubMed.
- R. A. Friesner, R. B. Murphy, M. P. Repasky, L. L. Frye, J. R. Greenwood, T. A. Halgren, P. C. Sanschagrin and D. T. Mainz, J. Med. Chem., 2006, 49, 6177–6196 CrossRef CAS PubMed.
- W. L. Jorgensen, D. S. Maxwell and J. Tirado-Rives, J. Am. Chem. Soc., 1996, 118, 11225–11236 CrossRef CAS.
- M. M. A. K. Shawan, S. K. Halder and M. A. Hasan, Bull. Natl. Res. Cent., 2021, 45, 27 CrossRef PubMed.
- J. J. Sahayarayan, K. S. Rajan, R. Vidhyavathi, M. Nachiappan, D. Prabhu, S. Alfarraj, S. Arokiyaraj and A. N. Daniel, Saudi J. Biol. Sci., 2021, 28, 400–407 CrossRef CAS PubMed.
- T. Hansson, C. Oostenbrink and W. van Gunsteren, Curr. Opin. Struct. Biol., 2002, 12, 190–196 CrossRef CAS PubMed.
- S. Pronk, S. Páll, R. Schulz, P. Larsson, P. Bjelkmar, R. Apostolov, M. R. Shirts, J. C. Smith, P. M. Kasson, D. van der Spoel, B. Hess and E. Lindahl, Bioinformatics, 2013, 29, 845–854 CrossRef CAS PubMed.
- S. K. Halder and F. Elma, J. Clin. Tuberc. Other Mycobact. Dis., 2021, 24, 100246 CrossRef PubMed.
- K. Bowers, E. Chow, H. Xu, R. Dror, M. Eastwood, B. Gregersen, J. Klepeis, I. Kolossvary, M. Moraes, F. Sacerdoti, J. Salmon, Y. Shan, and D. Shaw, in ACM/IEEE SC 2006 Conference (SC’06), IEEE, 2006, pp. 43 Search PubMed.
- M. Masetti, F. Falchi, D. Gioia, M. Recanatini, S. Ciurli and F. Musiani, Molecules, 2020, 25, 391–411 CrossRef PubMed.
- J. R. Lopéz-Blanco, J. I. Garzón and P. Chacón, Bioinformatics, 2011, 27, 2843–2850 CrossRef PubMed.
- J. R. López-Blanco, J. I. Aliaga, E. S. Quintana-Ortí and P. Chacón, Nucleic Acids Res., 2014, 42, W271–W276 CrossRef PubMed.
- A. Vahhabi, A. Hasani, M. A. Rezaee, B. Baradaran, A. Hasani, H. Samadi Kafil, F. Abbaszadeh and L. Dehghani, J. Chemother., 2021, 33, 137–155 CrossRef CAS PubMed.
- B. A. Evans and S. G. B. Amyes, Clin. Microbiol. Rev., 2014, 27, 241–263 CrossRef PubMed.
- M. C. Deller, L. Kong and B. Rupp, Acta Crystallogr., Sect. F: Struct. Biol. Commun., 2016, 72, 72–95 CrossRef CAS PubMed.
- G. M. Morris and M. Lim-Wilby, Methods Mol. Biol., 2008, 443, 365–382 CrossRef CAS PubMed.
- B. Ramachandran, J. Jeyakanthan and B. S. Lopes, J. Med. Microbiol., 2020, 69, 1062–1078 CrossRef CAS PubMed.
- H. Zhao and D. Huang, PLoS One, 2011, 6, e19923 CrossRef CAS PubMed.
- S. K. Halder, M. O. Rafi, E. B. Shahriar, S. Albogami, A. M. El-Shehawi, S. M. M. U. Daullah, M. K. Himel and T. B. Emran, Gene, 2022, 819, 146206 CrossRef CAS PubMed.
- S. Falsafi-Zadeh, Z. Karimi and H. Galehdari, Bioinformation, 2012, 8, 341–343 CrossRef PubMed.
- M. Siemers, M. Lazaratos, K. Karathanou, F. Guerra, L. S. Brown and A.-N. Bondar, J. Chem. Theory Comput., 2019, 15, 6781–6798 CrossRef CAS PubMed.
- H. Huang and C. Simmerling, J. Chem. Theory Comput., 2018, 14, 5797–5814 CrossRef CAS PubMed.
|
This journal is © The Royal Society of Chemistry 2022 |
Click here to see how this site uses Cookies. View our privacy policy here.