DOI:
10.1039/D2RA02637F
(Paper)
RSC Adv., 2022,
12, 16663-16676
Theoretical exploration of the binding selectivity of inhibitors to BRD7 and BRD9 with multiple short molecular dynamics simulations†
Received
25th April 2022
, Accepted 29th May 2022
First published on 6th June 2022
Abstract
Bromodomain-containing proteins 7 and 9 (BRD7 and BRD9) have been considered as potential targets of clinical drug design toward treatment of human cancers and other diseases. Multiple short molecular dynamics simulations and binding free energy predictions were carried out to decipher the binding selectivity of three inhibitors 4L2, 5U6, and 6KT toward BRD7 and BRD9. The results show that 4L2 has more favorable binding ability to BRD7 over BRD9 compared to 5U6 and 6KT, while 5U6 and 6KT possess more favorable associations with BRD9 than BRD7. Furthermore, estimations of residue-based free energy decompositions further identify that four common residue pairs, including (F155, F44), (V160, V49), (Y168, Y57) and (Y217, Y106) in (BRD7, BRD9) generate obvious binding differences with 4L2, 5U6, and 6KT, which mostly drives the binding selectivity of 4L2, 5U6, and 6KT to BRD7 and BRD9. Dynamic information arising from trajectory analysis also suggests that inhibitor bindings affect structural flexibility and motion modes, which is responsible for the partial selectivity of 4L2, 5U6, and 6KT toward BRD7 and BRD9. As per our expectation, this study theoretically provides useful hints for design of dual inhibitors with high selectivity on BRD7 and BRD9.
1. Introduction
Bromodomains (BRDs) are structurally conserved epigenetic reader modules observed in numerous chromatin- and transcription-associated proteins that have a capability to identify acetylated lysine residues.1 The human genome encodes 61 diverse BRDs that can be clustered into 8 families according to structure or sequence similarity.2,3 Functionally, BRD-containing proteins are relating to different disease processes, containing inflammation, oncology and viral replication, hence they are promising targets of drug design.4 Furthermore, in recent years several small-molecule inhibitors of BRDs have been employed in clinical tests for multiple disease such as cancers.5,6
BRD7 and BRD9 are two members of BRD subfamily IV and have an important role in treatment of multiple types of human diseases.7,8 Although BRD7 and BRD9 have differences in residue sequence, they share a highly similar topology structure shaped by four representative α helices, namely αZ, αA, αB and αC. The αZ and αA are linked by the ZA_loop while the αB and αC are connected by the BC_loop, and these secondary structures form a hydrophobic binding pocket of acetylated lysine. BRD7 was first identified as a drug targets to design tumor inhibitor in nasopharyngeal carcinoma (NPC) by Zhou group in 2010.9 Then extensive researches demonstrated that BRD7 is involved in development and progression of different types of cancer.10,11 Meanwhile, several researches suggested that BRD9 function12 as a key regulator of androgen receptor signaling and prostate cancer,13 ovarian cancer,14 hepatocellular carcinoma,15 and squamous cell lung cancer.16 Therefore, inhibiting activity of BRD9 through small molecules has been a novel and reliable pathway of tumor treatment.17 BRD7 and BRD9 play a critical role in controlling gene expression and regulating cell cycle,18,19 apart from an empirical correlation with multiple human cancers.20,21 Despite appearance of considerable differences in the ZA loop of BRD7 and BRD9, several potential hydrogen bonding interactions in their binding site seem to affect biological functions and therapeutic potential,22 which enhances selective capability of inhibitors toward these two bromodomains23 Notably, Karim et al. applied a multifaceted method to characterize feature of different small-molecule inhibitors with devious degrees of potency and selectivity for BRD7 and BRD9.24 The group of Brennan develop chemical probes to investigate binding preference of inhibitors to BRD7 and BRD9.23 Despite extensive experimental researches on interaction mechanism of inhibitors with BRD7 and BRD9 in different works, decoding the conformational changes of these two proteins at atom levels because of inhibitor associations are still highly essential.25,26
With fast development of calculation approach and simulation technology,27–34 various molecular dynamics (MD) methods, including conventional MD,35–39 multiple molecular dynamics (multiple short molecular dynamics),40–42 accelerated MD (aMD) simulations,34,43–51 have been widely utilized to perform conformational evolution of targets. Different methods of binding free energy prediction, involved molecular mechanics-Poisson Boltzmann surface area (MM-PBSA),52–57 thermodynamics integration (TI),58,59 free energy perturbation (FEP)60,61 and solvated interaction energy (SIE)62–64 methods, are extensively applied to evaluate binding ability of ligands to targets. Machine learning and deep learning methods are also introduced to successfully investigate ligand–target binding mechanism and unveil molecular basis of ligand–target associations.65,66 Furthermore, these simulation methods have been involved in successful insights into inhibitor–BRD binding mechanism. Xing et al. adopted machine-learning-assisted approach to find new inhibitors that efficiently inhibit the activity of BRD4.67 Su et al. employed MD simulations and MM-PBSA calculation to clarify interaction mechanisms of inhibitors with BRD2 and reveal binding selectivity of small molecules on different BRDs.68,69
Currently, many works on design of small molecules impeding the activity of BRDs are still on going. Recently, a small molecule 5U6 (5MQ1) is designed to inhibit the activity of BRD7, while two inhibitors 4L2(5JI8)70 and 6KT(4Z6H)71 are developed to suppress the activity of BRD9. Three small molecules also inhibit the activity of partial BRDs and they possess different levels of binding affinity to BRDs. Further exploring binding difference of 4L2, 5U6 and 6KT to BRD7 and BRD9 can provide vital molecular mechanism for development of small molecules targeting BRDs. Based on this aim 4L2, 5U6 and 6KT are picked for the current study. The conformation and binding pocket inhibitor–BRD compounds are exhibited in Fig. 1A and B, respectively, while the structures of 4L2, 5U6 and 6KT are depicted in Fig. 1C–E, individually. In this research, multiple short molecular dynamics simulations are utilized to enhance conformational sampling of inhibitor–BRD complexes, molecular mechanics-generalized Born surface area (MM-GBSA) approach is wielded to access binding ability of 4L2, 5U6 and 6KT to BRDs, calculations of cross-correlation map (CCM)72,73 is employed to clarify internal dynamics of inhibitor-bound BRDs and calculations of residue-based free energy decomposition are applied to identify interaction networks of 4L2, 5U6 and 6KT with BRD7 and BRD9. This research is anticipated to provide vital molecular mechanisms on associating difference of inhibitors with BRD7 over BRD9 for development of highly selective inhibitors holding back the activity of BRD7 and BRD9.
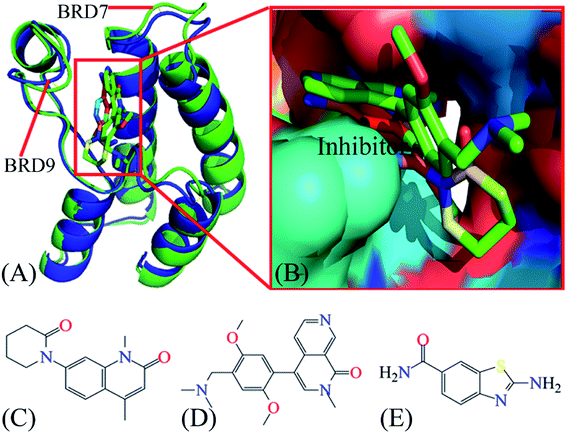 |
| Fig. 1 Molecular structures: (A) structural superimposition of inhibitor–BRD7 complex with inhibitor–BRD9 one, in which BRD7 is displayed in green and BRD9 in blue; (B) binding pocket of two different inhibitors in BRD7 and BRD9, among which inhibitors are shown in stick modes and BRD7 and BRD9 in surface modes; (C), (D) and (E) corresponding to structures of 4L2, 5U6 and 6KT, respectively. The blue and red letters suggest the polar atoms possibly forming hydrogen bonding interactions and electrostatic interactions with BRD7 and BRD9. BRD7 and BRD9 are displayed in cartoon modes, while inhibitors displayed in stick or line modes. In this figure, the crystal structures, ID code 5MQ1 and 4Z6H, are used to respectively display the structures of the inhibitor–BRD7 and inhibitor–BRD9 complexes. | |
2. Theory and methods
2.1 Simulated system setup
The initial configurations of BRD7 complexed with 5U6 and BRD9 complexed with 4L2 and 6KT are withdrew from the protein data bank (https://www.rcsb.org): the entry ID 5MQ1 for the 5U6–BRD7 complex and 5JI8 and 4Z6H for the 4L2– and 6KT–BRD9 ones, respectively.71 Since the crystal structures of the 4L2–BRD7 and 6KT–BRD7 compounds are unavailable, the crystal structures 4Z6H and 5JI8 are superimposed with 5MQ1 to separately produce the structures of the 4L2– and 6KT–BRD7 complexes by removing 5U6 and BRD9 by means of the PyMol software (https://www.pymol.org). Similar treatment to the above description is adopted to obtain the 5U6–BRD9 structure missed in the PDB. Both crystal water and non-inhibitor molecules are removed from the initialized systems, while all missing hydrogen atoms are added to their heavy atoms by using the Leap tool in Amber 20. The ff14SB force field74,75 and TIP3P model76 are employed to assign the simulated parameters to two proteins BRD7 and BRD9 as well as water molecules, separately. The configuration of inhibitors 4L2, 5U6, and 6KT are optimized with the semiempirical AM1 method, and afterwards, atomic BCC charges are given to 4L2, 5U6, and 6KT by using the Antechamber tool in Amber 20.77,78 The force field parameters of L2, 5U6, and 6KT are yielded with the general amber force field (GAFF).79 Five and seven chloridion ions (Cl−) are respectively placed around the inhibitor-bound BRD7 and BRD9 to generate six neutral simulation systems. In addition, octahedral periodic boxes with 12.0 Å buffer along each dimension, composed of TIP3P water model, are utilized to solve the inhibitor–BRD7 or BRD9 complexes. The initial conformation and nine replica taken from the later 100 ns MD are randomly assign initial velocities to run ten separate replica MD simulation of 100 ns, which is described in multiple short molecular dynamics simulation.
2.2 Multiple short molecular dynamics simulations
Before implementing multiple short molecular dynamics simulations, the initial system is subject to an optimized progress, consisting of steepest descent minimization of 2500 steps and conjugate gradient one of another 2500 steps, to relieve unfavorable atomic contacts and bond orientations. Then, a moderate process of 2 ns is wielded to elevate the temperature of the system from 0 to 300 K and then the system is further optimized for another 2 ns at the level of 300 K. A cMD simulation without restriction is executed for 100 ns at the levels of 300 K temperature and 1 bar pressure by taking advantage of periodic boundary conditions (PBCs) together with particle mesh Ewald (PME) approach.80 Nine new replicas are randomly taken from the above mentioned 100 ns cMD trajectory and initial velocities of each atom are assigned to these nine structures by means of Maxwell distribution to restart nine new cMD simulations. Finally, the balanced parts extract from the aforementioned 10 independent trajectories are linked into a single trajectory (ST) to run the post-processing analysis. The hydrogen-heavy atoms' chemical bonds are constrained through the SHAKE approach so that 2 fs time step is adopted during the system evolution.81 A rational cutoff value of 10 Å is utilized to compute electrostatic interactions and van der Waals ones, from which the long range electrostatic interactions is estimated with the particle mesh Ewald (PME) method.80,82 In the present study, the multiple short molecular dynamics simulations are carried out with the CUDA enabled NVIDIA graphics processing units (GPUs) inlayed at the Amber 20.83,84
2.3 MM-GBSA calculations
Empirical equation-based MM-PBSA and MM-GBSA methods are more popular approaches for calculations of binding free energies since they are more accurate than numerous scoring functions of molecular docking and less computationally requirement than alchemical free energy approaches.38,52,85,86 The Hou's team access the performance of MM-GBSA and MM-PBSA approaches by computing protein–ligand binding affinities with various simulation protocols and their information indicates that MM-GBSA method can produce rational results in drug design and related research fields.87–89 Hence, in the present work, the MM-GBSA approach, as listed in the formula (1), was implemented to calculate binding free energies of 4L2, 5U6, and 6KT to BRD7 and BRD9. |
ΔGbind = Gcomp − Gpro − Ginh = ΔEele + ΔEvdW + ΔGgb + ΔGnonpol − TΔS
| (1) |
in which Gcomp, Gpro, and Ginh represent free energies of the inhibitor–BRD complex, BRD7/BRD9 and inhibitors, individually. In addition, the terms ΔEele and ΔEvdW indicate electrostatic interactions (EIs) and van der Waals interactions (vDWIs) of 4L2, 5U6, and 6KT with BRD7 and BRD9, which can be calculated based on molecular mechanics theory. The components ΔGgb and ΔGnonpol represent polar solvation free energies (PSFEs) and nonpolar solvation free energies (NPSFEs), independently. Polar solvation free energies are solved through the Generalized Born (GB) model from Onufriev et al.90 Nonpolar solvation free energies are computed with an empirical formula ΔGnonpol = γ × ΔSASA + β, where the parameters γ and β (0.0072 kcal mol−1 Å−2 and 0.0 kcal mol−1) are withdrew from the work of Gohlke et al.,91 and ΔSASA arises from the alterations in solvent accessible surface area (SASA) due to inhibitor bindings. The last term −TΔS indicates the entropy differences caused by the presence of inhibitors, which is evaluated by means of the mmpbsa_py_nabnmode program stemming from Amber 20.92,93
2.4. Principal components analysis methodology
Principal component analysis (PCA) is a significant tool for screening large concerted movements from an ensemble of conformational structures emerging form the molecular simulations or experiments. This methodology has been extensively employed to investigate conformational changes of receptors with regard to its function.94,95 PCA can be calculated by implementing the diagonalization on a covariance matrix constructed with the atomic coordinates recorded in multiple short molecular dynamics simulations, which will yield a set of eigenvectors and eigenvalues. The eigenvectors reflect the motional directions in conformational space of protein domains, while the eigenvalues represent mean square fluctuations of the movement along the corresponding eigenvectors. The first several eigenvectors with large eigenvalues are notable for demonstrating the whole motions of proteins. In the present work, the CPPTARJ module96 embodied in Amber was employed to implement PCA on the joined multiple short molecular dynamics trajectory. The software Pymol and VMD were wielded to draw pictures.
3. Results and discussion
3.1. Structural features
In order to get reasonable conformation samplings of BRD7 and BRD9, multiple short molecular dynamics simulations, composed of 100 ns cMD simulations of 10 replicas, are implemented on the BRD7 and BRD9 systems bound by 4L2, 5U6 and 6KT, respectively. Root-mean-square-deviations (RMSDs) of backbone atoms from BRD7 and BRD9 relative to their initial optimized configuration are computed to check the structure fluctuation through multiple short molecular dynamics simulations (Fig. S1†). The information of RMSDs reveal that all replicas are tending towards the stability after 40 ns of simulations, indicating that the equilibrium of all simulated systems are fundamentally reached. Hence, the equilibrated sections (40–60 ns) from 10-replica simulations are integrated to form a single combined trajectory (SCT) of 600 ns for each complex. This SCT is used to execute all calculations and extract the useful conformational information.
To check local structural fluctuations of BRD7 and BRD9 induced by the presence of 4L2, 5U6 and 6KT, the Cα atomic coordinates saved at the SCT are employed to estimate root-mean-square fluctuations (RMSFs) of BRD7 and BRD9 (Fig. 2). The RMSF distribution of BRD7 is similar to the one of BRD9, demonstrating that both BRD7 and BRD9 possess similar rigid and flexible domains. Apparent structural differences primarily take place in four regions, consisting of L1 (residues 149–157 for BRD7 and 37–45 for BRD9), L2 (residues 161–172 for BRD7 and 49–60 for BRD9), L3 (residues 187–196 for BRD7 and 75–84 for BRD9) and L4 (residues 212–223 for BRD7 and 100–111 for BRD9). These results imply that some residues stemming from the above four regions are certainly situate at hot spots of inhibitor–BRD associations. The RMSF values of BRD7 and BRD9 in the bound state of 6KT are bigger than that in binding of 4K2 and 5U6, especially for the regions L1 and L2, indicating that binding of 4K2 and 5U6 yields stronger restriction on the regions L1 and L2 of BRD7 and BRD9 than binding of 6KT. Structurally, the regions L1 and L2 are located near binding sites of BRD7 and BRD9, which signifies that certain residues in L1 and L2 mainly drive binding selectivity of 4L2, 5U6 and 6KT toward BRD9 and BRD7. Although two secondary structures L3 and L4 are not in the vicinity of binding sites from BRD7 and BRD9, the varieties in structural flexibility of L3 and L4 can exert vital impacts on binding of 4L2, 5U6 and 6KT to BRD7 and BRD9.
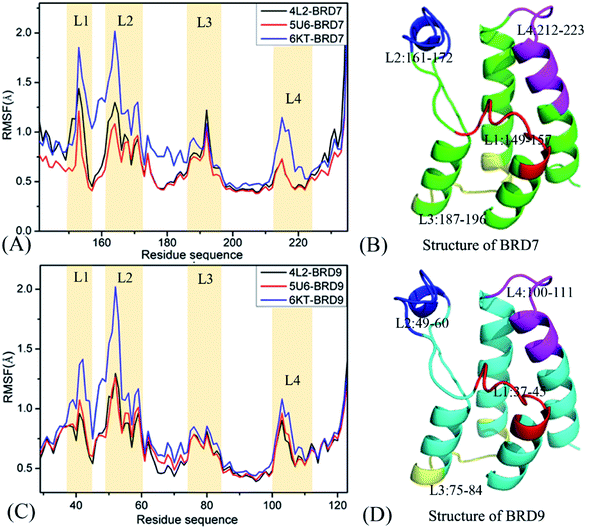 |
| Fig. 2 Root-mean-square fluctuations (RMSFs) of the Cα atoms in BRD7 and BRD9: (A) RMSFs for BRD7 complexed with inhibitors 4L2, 5U6 and 6KT, (B) the structure of BD7, (C) RMSFs for BRD9 complexed with inhibitors 4L2, 5U6 and 6KT, (D) the structure of BRD9. | |
3.2 Dynamics behavior changes of BRD7 and BRD9
To uncover differences in dynamics behavior of BRD7 and BRD9, CCMs are computed by utilizing the Cα atomic coordinates kept at the SCT (Fig. 3), in which the dark blue and blue describe sturdily anticorrelated (AC) motions while the yellow and red are the indicators of sturdily positive correlated (PC) movements. A correlated motion of a certain domain relative to itself is embodied by the diagonal parts while that between different domains are reflected by the off-diagonal regions. As exhibited in Fig. 3, binding of 4L2, 5U6 and 6KT exerts evident influences on dynamics behavior of BRD7 and BRD9.
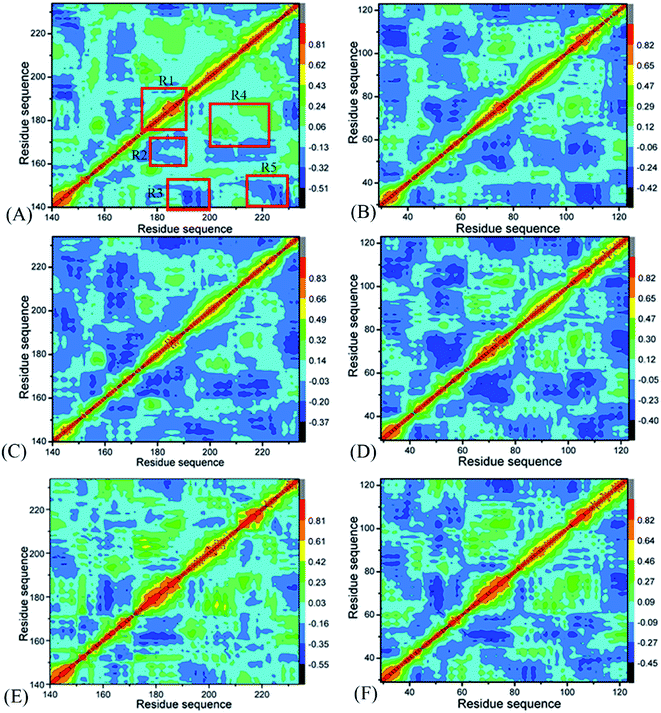 |
| Fig. 3 Cross-correlation maps computed by utilizing the coordinates of Cα atoms around their mean positions recorded at the single joined multiple short molecular dynamics trajectory: (A), (C), and (E) corresponding to BRD7 complexed with 4L2, 5U6 and 6KT, separately; (B), (D), and (F) corresponding to BRD9 complexed with 4L2, 5U6 and 6KT, respectively. | |
For BRD7 bound by 4L2, 5U6 and 6KT (Fig. 3A, C and E), a PC movement (yellow and red) is observed, while obvious AC motions (blue and dark blue) appear at three regions R2, R4, and R5. By referencing the 4L2-bound BRD7, the presence of 4L2 in BRD9 not only slightly weaken the PC motion in the R1 but also weakens the AC movement in the R3 (Fig. 3B). In addition, binding of 4L2 to BRD9 also strengthens the AC motions in R2 and R5 relative to the 4L2-bound BRD7 (Fig. 3B). Binding of 5U6 to BRD9 hardly affects the AC movements occurred at R2 and R5 relative to the 5U6-bound BRD7, but slightly weakens the AC motions in R3 from BRD9 (Fig. 3D). By comparison with the 6KT-bound BRD7, although association of 6KT with BRD9 hardly alters motion modes in R1 and R4 from BRD9, it evidently strengthens the AC movements in R2, R3, and R5 from BRD9. Based on the above discussion, associations of identical inhibitors lead to motion mode difference between BRD7 and BRD9, which demonstrates that some residues situated in R1–R5 of BRD7 and BRD9 may be involved in hot binding spots of 4L2, 5U6 and 6KT to BRD7 and BRD9.
3.3 Conformational changes of BRD7 and BRD9 caused by inhibitor bindings
Principal component analysis (PCA) is extensively employed to investigate concerted motions of structural domains from proteins.97 This approach can filter significantly concerted movements from structural ensembles arising from experimental or simulation works. In the present work, PCA is employed to decode molecular mechanism of binding selectivity of 4L2, 5U6 and 6KT to BRD7 and BRD9. PCA can be realized through a diagonalization on a covariance matrix built with the Cα atomics coordinates extracted from the SCT. As displayed in Fig. 4, the first six principal components of BRD7 bound by 4L2, 5U6 and 6KT, describing significantly collected movements, occupy 59.90%, 48.95% and 64.49% of the observed movements in multiple short molecular dynamics simulations, separately, while that of BRD9 bound by 4L2, 5U6 and 6KT account for 52.24%, 55.15%, and 60.93% of the total motions from the multiple short molecular dynamics simulations, independently. Compared with the 4L2- and 6KT-bound BRD7, the first few eigenvalues of the 4L2- and 6KT-bound BRD9 are extremely abated by inhibitor associations, while the eigenvalues of the 5U6–BRD9 are evidently increased because of inhibitor associations, indicating that the presence of 4L2, 5U6 and 6KT in the binding pocket of BRD7 and BRD9 produce significant influences on the total motion strength of these two BRDs.
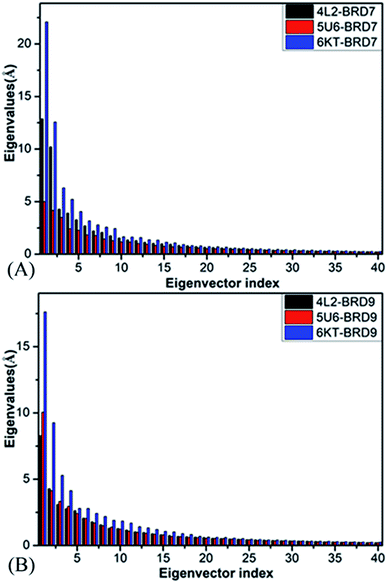 |
| Fig. 4 The function of eigenvalues versus eigenvector index stemming from PCA based on the single joined multiple short molecular dynamics trajectory: (A) BRD7 and (B) BRD9 complexed with three inhibitors 4L2, 5U6, and 6KT, respectively. | |
Motion directions of BRD7 along the first eigenvector stemming from PCA are depicted in Fig. S2.† Fig. S2A, C and E† displays the concerted motions of the 4L2-, 5U6- and 6KT-associated BRD7, separately, while Fig. S2B, D and F† shows that of the 4L2-, 5U6- and 6KT-bound BRD9, individually. In contrast with the 4L2-, 5U6- and 6KT-bound BRD7, associations of these inhibitors not only alter movement direction of the ZA and BC loops in BRD9, but also change motion strengthen of these two loops. In addition, the αZ helix in the 4L2-bound BRD7 moves toward the right (Fig. S2A†), while that in the 4L2-associated BRD9 is changed upwards and inwards (Fig. S2B†). The αZ helix in the 5U6-bound BRD7 moves toward the left and up (Fig. S2C†), but that in the 5U6-associate BRD9 is transformed toward the right and up (Fig. S2D†). The αA helix in the 6KT-bound BRD7 moves toward the right and up, on the contrary that of the 6KT-associated BRD9 is changed toward the right and down (Fig. S2F†). These results indicate that the changes in essential dynamics behavior detected by multiple short molecular dynamics simulations may be responsible for binding selectivity of 4L2, 5U6 and 6KT on BRD7 and BRD9.
3.4 Binding affinity of inhibitors to BRD7 and BRD9
To decipher binding preference of 4L2, 5U6 and 6KT to BRD7 and BRD9, MM-GBSA approach is employed to calculate binding free energies of three inhibitors to BRD7 and BRD9 by using 300 structural frames withdrawn from the 600 ns SCTs with a time interval of 2 ns. Since the computing time of entropy is highly expensive, only 100 snapshots taken from the above 300 structural frames are applied to compute entropy contributions to binding of 4L2, 5U6 and 6KT. All energetic data arising from MM-GBSA calculation are exhibited in Table 1.
Table 1 Binding affinities of inhibitors to BRD7 and BRD9 computed with MM-GBSA approacha
Terms |
4L2–BRD7 |
4L2–BRD9 |
5U6–BRD7 |
5U6–BRD9 |
6KT–BRD7 |
6KT–BRD9 |
Mean |
Semb |
Mean |
Semb |
Mean |
Semb |
Mean |
Semb |
Mean |
Semb |
Mean |
Semb |
All components of free energies are in kcal mol−1. Standard errors of means. ΔGele+gb = ΔEele + ΔGgb. ΔGvdW+nonpol = ΔEvdW + ΔGnonpol. |
ΔEele |
−26.38 |
0.17 |
−28.66 |
0.21 |
−19.03 |
0.17 |
−19.65 |
0.19 |
−15.71 |
0.52 |
−18.66 |
0.39 |
ΔEvdW |
−34.71 |
0.15 |
−34.61 |
0.16 |
−40.35 |
0.15 |
−41.93 |
0.15 |
−19.60 |
0.44 |
−23.5 |
0.28 |
ΔGgb |
36.08 |
0.15 |
38.49 |
0.01 |
32.78 |
0.14 |
33.93 |
0.16 |
22.38 |
0.57 |
26.0 |
0.35 |
ΔGnonpol |
−2.89 |
0.01 |
−2.89 |
0.01 |
−3.33 |
0.01 |
−3.43 |
0.01 |
−1.92 |
0.04 |
−2.25 |
0.02 |
ΔGele+gbc |
9.7 |
0.16 |
9.83 |
0.11 |
13.75 |
0.16 |
14.28 |
0.17 |
6.67 |
0.55 |
7.34 |
0.37 |
ΔGvdW+nonpold |
−37.6 |
0.08 |
−37.5 |
0.18 |
−43.68 |
0.08 |
−45.36 |
0.08 |
−21.52 |
0.24 |
−25.75 |
0.15 |
ΔH |
−27.9 |
0.18 |
−27.67 |
0.44 |
−29.93 |
0.14 |
−31.08 |
0.15 |
−14.85 |
0.39 |
−18.41 |
0.32 |
TΔS |
16.41 |
0.65 |
16.74 |
0.64 |
18.28 |
0.74 |
18.78 |
0.77 |
13.19 |
0.69 |
15.42 |
0.70 |
ΔGbind |
−11.49 |
|
−10.93 |
|
−11.65 |
|
−12.30 |
|
−1.66 |
|
−2.99 |
|
For BRD7 and BRD9 bound by 4L2, 5U6 and 6KT, favorable EIs (ΔEele) in the gas phase are entirely screened by unfavorable PSFEs (ΔGgb) to provide unfavorable contributions (ΔGele+gb) for bindings of 4L2, 5U6 and 6KT to BRD7 and BRD9. The variations of entropies (−TΔS) also impair associations of 4L2, 5U6 and 6KT with BRD7 and BRD9. Conversely, vdWIs (ΔEvdW) and NPSFEs (ΔGnonpol) contribute favorable forces (ΔGvdW+nonpol) to associations of 4L2, 5U6 and 6KT with BRD7 and BRD9. According to Table 1, although the values of ΔGele+gb for the 4L2-bound BRD9 are increased by 0.13 kcal mol−1 relative to the 4L2-bound BRD7, the favorable forces (ΔGvdW+nonpol) of the 4L2-bound BRD9 are decreased by 0.1 kcal mol−1 compared with the 4L2-bound BRD7, which results in a decrease of 0.23 kcal mol−1 in the binding enthalpy (ΔH) of 4L2 to BRD9 relative to the 4L2-bound BRD7. On the contrary, the −TΔS of the 4L2-bound BRD9 is increased by 0.33 kcal mol−1 by referencing the 4L2-associated BRD7. As a whole, binding affinity of 4L2 to BRD9 is reduced by 0.56 kcal mol−1 relative to that of 4L2 to BRD7, demonstrating that 4L2 has better selectivity toward BRD7 than BRD9. As displayed in Table 1, the ΔGele+gb of the 5U6-bound BRD9 is increased by 0.53 kcal mol−1 compared to that of the 5U6-associated BRD9, and the ΔGvdW+nonpol of the 5U6-bound BRD9 is improved by 1.68 kcal mol−1 relative to that of the 5U6-associated BRD7, causing an enhancement of 1.15 kcal mol−1 in binding enthalpy of the 5U6-associated BRD9 compared to that of the 5U6-bound BRD7. Meanwhile, the −TΔS of the 5U6-bound BRD9 is increased by 0.5 kcal mol−1 relative to that of the 5U6-associated BRD7. Thus, binding free energy of 5U6 to BRD9 is strengthened by 0.65 kcal mol−1 by referencing the 5U6–BRD7 complex, suggesting that 5U6 has a binding preference to BRD9 over BRD7. On the basis of Table 1, the interactions ΔGele+gb and ΔGvdW+nonpol of 6KT with BRD9 are elevated by 0.67 and 4.23 kcal mol−1 compared to that of 6KT with BRD7, separately, causing an enhancement of 3.56 kcal mol−1 in binding enthalpy of the 6KT–BRD9 compound relative to the 6KT–BRD7 one. Meanwhile, the −TΔS of the 6KT-bound BRD9 is 2.23 kcal mol−1 stronger than that of the 6KT-associated BRD7, which totally leads to an increase of 1.33 kcal mol−1 in binding strength of 6KT to BRD9 by likening to that of 6KT to BRD7. Therefore, 6KT has a binding preference toward BRD9 over BRD7.
Based on the above analyses, although the entropy contribution of 4L2, 5U6, and 6KT to BRD9 is apparently enhanced relative to that of three inhibitors to BRD7, enthalpy alterations compensate this unfavorable effect. It is found that 4L2 has better selectivity toward BRD7 over BRD9, while 5U6 and 6KT can more favorably bind to BRD9 than BRD7. Finally, a conclusion is drawn that enthalpy effects play pivotal roles in selective recognition of 4L2, 5U6, and 6KT on BRD7 and BRD9.
3.5 Bing selectivity uncovered by inhibitor–residue interactions
To clarify binding selectivity of 4L2, 5U6, and 6KT to BRD7 versus BRD9, the details concerning interactions of inhibitors with separate residues are provided through MM-GBSA method. Table 2 displayed the decomposition of ΔGligand–residue values into contributions from sidechain and backbone of key residues in BRD7 and BRD9 bound by 4L2, 5U6, and 6KT. It is found that energy contributions from sidechain of residues play major roles in inhibitor–residue interactions. Key residues of BRD7 and BRD9 that form important inhibitor–residue interactions with energies stronger than 0.8 kcal mol−1 are depicted in Fig. 4 and 5. Moreover, a CPPTRAJ tool in Amber 20 is applied to discern hydrogen bonding interactions (HBIs) of 4L2, 5U6, and 6KT with BRD7 and BRD9 (Table 3). Hydrogen bonds (HBs) and the corresponding radial distribution function (RDF) of H–O distance of 4L2, 5U6, and 6KT away from key residues in BRD7 and BRD9 are exhibited in Fig. 5 and S6.†
Table 2 Interactions between key residues of BRD7/BRD9 and three inhibitors (all values are in kcal mol−1)
BRDs |
Key residues |
Inhibitors |
4L2 |
5U6 |
6KT |
Sidechain |
Backbone |
Total |
Sidechain |
Backbone |
Total |
Sidechain |
Backbone |
Total |
BRD7 |
A154 |
−0.25 |
−0.17 |
−0.42 |
−0.33 |
−0.29 |
−0.62 |
−0.02 |
−0.02 |
−0.04 |
F155 |
−1.40 |
−0.23 |
−1.63 |
−2.07 |
−0.01 |
−2.08 |
−0.62 |
−0.12 |
−0.74 |
F156 |
−0.46 |
−0.15 |
−0.61 |
−0.64 |
−0.16 |
−0.81 |
−0.48 |
−0.10 |
−0.58 |
F158 |
−0.51 |
0.03 |
−0.48 |
−0.44 |
0.15 |
−0.29 |
−0.03 |
0.03 |
−0.01 |
V160 |
−1.89 |
−0.24 |
−2.13 |
−1.88 |
−0.42 |
−2.30 |
−0.78 |
−0.04 |
−0.82 |
I164 |
−1.18 |
−0.10 |
−1.28 |
−2.18 |
−0.23 |
−2.41 |
−0.61 |
−0.14 |
−0.75 |
Y168 |
−0.31 |
−0.01 |
−0.33 |
−0.29 |
−0.02 |
−0.31 |
−0.34 |
−0.01 |
−0.35 |
N211 |
−1.86 |
0.02 |
−1.84 |
−1.86 |
0.02 |
−1.84 |
−1.34 |
0.01 |
−1.33 |
Y217 |
−2.41 |
−0.14 |
−2.55 |
−2.63 |
−0.15 |
−2.78 |
−1.33 |
−0.09 |
−1.42 |
BRD9 |
G43 |
−0.11 |
−0.07 |
−0.18 |
−0.15 |
−0.37 |
−0.52 |
−0.01 |
−0.05 |
−0.06 |
F44 |
−1.51 |
−0.30 |
−1.81 |
−2.09 |
−0.21 |
−2.31 |
−1.17 |
−0.19 |
−1.37 |
F45 |
−0.40 |
−0.13 |
−0.53 |
−0.58 |
−0.16 |
−0.74 |
−0.64 |
−0.11 |
−0.74 |
F47 |
−0.57 |
0.06 |
−0.51 |
−0.57 |
0.24 |
−0.34 |
−0.04 |
0.04 |
0.00 |
V49 |
−1.93 |
−0.25 |
−2.19 |
−2.02 |
−0.46 |
−2.48 |
−1.04 |
−0.05 |
−1.09 |
I53 |
−1.24 |
−0.09 |
−1.33 |
−2.24 |
−0.20 |
−2.44 |
−0.67 |
−0.14 |
−0.81 |
A54 |
−0.47 |
−0.08 |
−0.54 |
−0.60 |
−0.20 |
−0.81 |
−0.43 |
−0.06 |
−0.49 |
Y57 |
−0.28 |
−0.01 |
−0.30 |
−0.29 |
−0.01 |
−0.31 |
−0.94 |
−0.01 |
−0.96 |
A96 |
−0.33 |
−0.31 |
−0.64 |
−0.35 |
−0.22 |
−0.57 |
−0.45 |
−0.35 |
−0.80 |
Y99 |
−0.48 |
−0.02 |
−0.50 |
−0.51 |
−0.02 |
−0.52 |
−0.49 |
−0.05 |
−0.54 |
N100 |
−1.80 |
0.01 |
−1.79 |
−1.82 |
0.01 |
−1.81 |
−1.32 |
−0.00 |
−1.32 |
Y106 |
−2.37 |
−0.14 |
−2.51 |
−2.62 |
−0.13 |
−2.76 |
−1.46 |
−0.11 |
−1.57 |
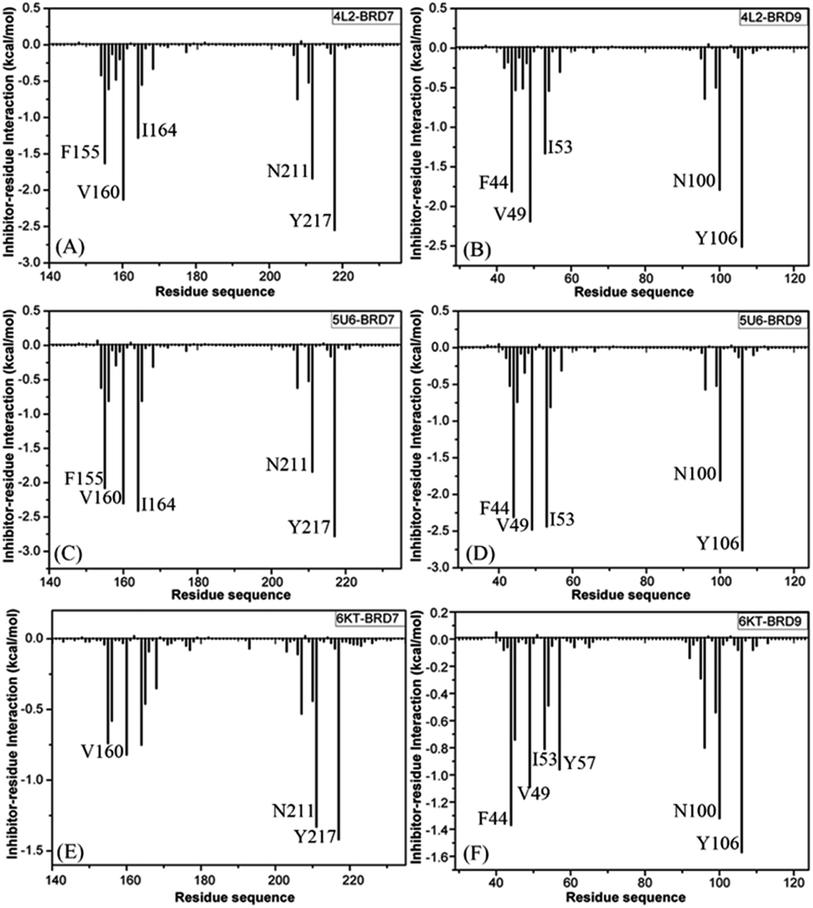 |
| Fig. 5 Inhibitor–residue interactions calculated by using residue-based free energy decomposition approach, only residues stronger than 0.8 kcal mol−1 are listed: (A) the 4L2–BRD7 complex, (B) the 4L2–BRD9 complex, (C) the 5U6–BRD7 complex, (D) the 5U6–BRD9 complex, (E) the 6KT–BRD7 complex and (F) the 6KT–BRD9 complex. | |
Table 3 Hydrogen bonding interactions between inhibitors and BRD7/9 calculated by the program of CPPTRAJ
Complexes |
Hydrogen bonds |
Distancea (Å) |
Anglea (°) |
Occupancyb (%) |
Hydrogen bonds are determined by the acceptor–donor atom distance of <3.5 Å and acceptor–H–donor angle of >120°. Occupancy (%) is defined as the percentage of simulation time that a specific hydrogen bond exists. The full lines represent chemical bonds, and the dotted lines indicate hydrogen bonding interactions. |
4L2–BRD7 |
Asn211–ND2–HD21⋯4L2–OAD |
2.90 |
159.66 |
99.91 |
4L2–BRD9 |
Asn100–ND2–HD21⋯4L2–OAD |
2.91 |
159.38 |
99.83 |
5U6–BRD7 |
Asn211–ND2–HD21⋯5U6–O11 |
2.93 |
161.64 |
99.74 |
5U6–BRD9 |
Asn100–ND2–HD21⋯5U6–O11 |
2.92 |
161.56 |
99.80 |
6KT–BRD7 |
Asn211–ND2–HD21⋯6KT-O11 |
2.95 |
154.41 |
47.97 |
Phe155–O⋯6KT–N13–H7 |
3.11 |
149.26 |
35.17 |
6KT–BRD9 |
Asn100–ND2–HD21⋯6KT–O11 |
2.93 |
152.86 |
53.52 |
Phe44–O⋯6KT–N13–H19 |
3.13 |
146.01 |
44.82 |
Tyr57–OH–HH⋯6KT–O11 |
2.76 |
160.57 |
31.48 |
For the 4L2-bound BRD7 and BRD9, 4L2 produces favorable interactions with F155, V160, I164, N211 and Y217 in BRD7 and all of them are stronger than 0.8 kcal mol−1 (Fig. 5A). The interaction strengths of 4L2 with F155, V160, I164, N211 and Y217 are respectively scaled by −1.63, −2.13, −1.28, −1.84, and −2.55 kcal mol−1 and they are in structural consistence with the π–π interactions of the hydrophobic rings from F155 and Y217 with that from 4L2 and the CH–π interactions of the alkyls in V160, I164 and N211 with the hydrophobic ring from 4L2 (Fig. 5A). In addition, a HBI (Asn211–ND2–HD21⋯4L2–OAD) with the 99.91% occupancy is detected between 4L2 and BRD7 (Table 3). In the 4L2–BRD7 complex, the nitrogen atom ND2 of Asn211 provide a hydrogen atom HD21 to form a HB with an oxygen atom OAD donated by 4L2. Fig. 7A depicts the geometrical positions of the HBs, and the HB distances and their frequency distributions are plotted in Fig. 7B. In comparison with the 4L2-bound BRD7, interaction modes of 4L2 with BRD9 are extremely similar to that of 4L2 with BRD7 (Fig. 5B, 6B and S3A and B†). It is discovered that interactions difference of 4L2 with residues (V160, V49), (I164, I53), (N211, N100), and (Y217, Y106) corresponding to (BRD7, BRD9) is less than 0.06 kcal mol−1, suggesting that these residues do not play critical roles in the binding selectivity of 4L2 toward BRD7 and BRD9. The interaction energy of 4L2 with F44 in BRD9 is strengthened by 0.18 kcal mol−1 relative to that of 4L2 with the corresponding residue F155 in BRD7, which indicates that residues (F155, F44) provide main contributions for the selectivity of 4L2 on BRD7 over BRD9.
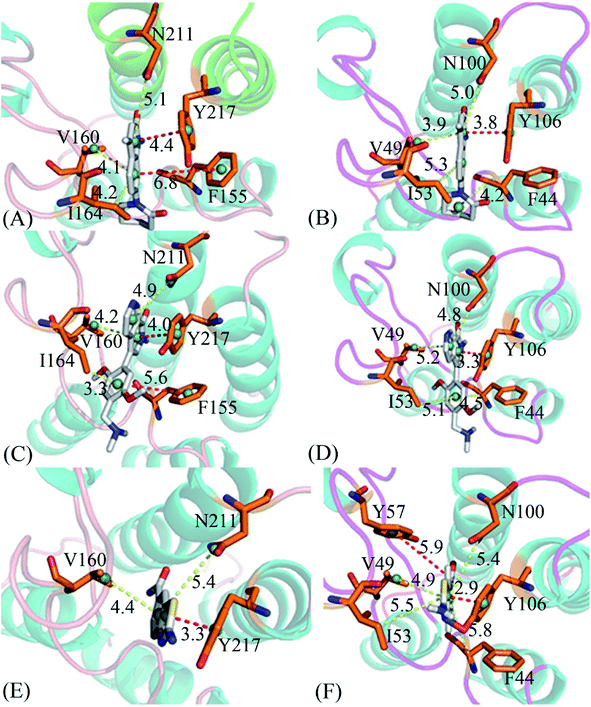 |
| Fig. 6 Geometric position of three inhibitors relative to key residues involved in important hydrophobic interaction stronger than 0.8 kcal mol−1: (A) the 4L2–BRD7 complex, (B) the 4L2–BRD9 complex, (C) the 5U6–BRD7 complex, (D) the 5U6–BRD9 complex, (E) the 6KT–BRD7 complex and (F) the 6KT–BRD9 complex. The yellow lines indicate the CH–π interactions and the red dash ones mean the π–π interactions. | |
As for the 5U6-bound BRD7 over BRD9, favorable interactions stronger than −0.8 kcal mol−1 are detected between 5U6 and five residues F155, V160, I164, N211 and Y217 in BRD7 (Fig. 5C). The interaction energies of F155 and Y217 in BRD7 with 5U6 are −2.08 and −2.78 kcal mol−1, which respectively agree with the π–π interactions between hydrophobic ring of 5U6 and the ones of F155 and Y127 (Fig. 5C and 6C). As exhibited in geometric positions (Fig. 6C), the CH groups of V160, I164, and N211 from BRD7 are situated near the ring of 5U8, thus these three residues in BRD7 prefer to yield the CH–π interactions with 5U6, and the 5U6–V160, I164 and N211 interaction energies are respectively scaled by −2.30, −2.41, and −1.84 kcal mol−1. According to Fig. 5D, 6D, S3C† and Table 3, binding mode of 5U6 to BRD9, including hydrophobic interactions and HBIs, is similar to that of 5U6 to BRD7. The interaction energies of F44 and V49 in BRD9 are respectively strengthened by 0.23 and 0.18 kcal mol−1 relative to residues F155 and V160 in BRD7, indicating that these two residues contribute key forces to binding selectivity of 5U6 on BRD7 and BRD9.
With regard to the 6KT-bound BRD7 and BRD9, three residues V160, N211, and Y217 in BRD7 are involved interactions stronger −0.82, −1.33, and −1.42 kcal mol−1 with 6KT (Fig. 6E and 7E). Moreover, 6KT forms two HBIs with BRD7 (Asn211–ND2–HD21⋯6KT–O11 and Phe155–O⋯6KT–N13–H7) with the occupancies are 47.97% and 35.17%, respectively (Table 3 and Fig. 7E). According to Fig. 5F, interactions of 6K6 with F44, V49, I53, Y57, N100 and Y106 in BRD9 are −1.37, −1.09, −0.81, −0.96, −1.32, and −1.57 kcal mol−1, respectively, and they structurally arise from the π–π interactions of the hydrophobic rings from Y57 and Y106 with that from 6KT and the CH–π interactions of the alkyls in F44, V49, I53, and N100 with the hydrophobic ring in 6KT (Fig. 6F). In addition, 6KT forms three HBs with BRD9, including Asn100–ND2–HD21⋯6KT–O11, Phe44–O⋯6KT–N13–H19, and Tyr57–OH–HH⋯6KT–O11 with the occupancies are 53.52%, 44.82%, and 31.48%, respectively (Table 3, and Fig. S3E†). The interactions of 6KT with residues (I164, I53) and (N211, N100) in (BRD7, BRD9) are almost same, demonstrating that these residues hardly contribute forces to binding selectivity of 6KT on BRD7 and BRD9. The interactions of 6KT with F44, V49, Y57, and Y106 in BRD9 are strengthened by 0.63, 0.27, 0.61, and 0.15 kcal mol−1 compared with that of 6KT with F155, V160, Y168 and Y217 in BRD7, which indicates that residues (F155, F44), (V160, V49), (Y168, Y57), and (Y217, Y106) in (BRD7, BRD9) are mainly responsible for binding selectivity of 6KT om BRD7 and BRD9.
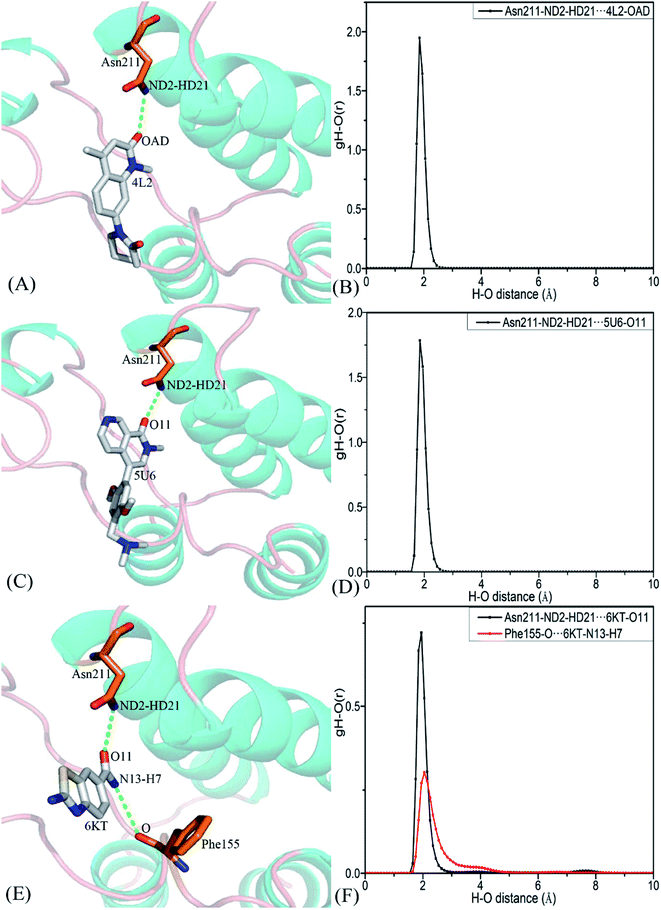 |
| Fig. 7 Hydrogen bonds and the corresponding radial distribution function (RDF) of H–O distance between three inhibitors and key residues of BRD7: (A) the 4L2–BRD7 complex, (B) RDF of H–O distance between 4L2–OAD and Asn211–ND2–HD21, (C) the 5U6–BRD7 complex, (D) RDF of H–O distance between 5U6–O11 and Asn211–ND2–HD21, (E) the 6KT–BRD7 complex and (F) RDF of H–O distances between 6KT–O11 and Asn211–ND2–HD21, and 6KT–N13–H7 and Phe155–O. | |
4. Conclusions
Based on the key roles of insights into binding selectivity of 4L2, 5U6, and 6KT on BRD7 and BRD9 in anti-cancer drug design, multiple short molecular dynamics simulations, composed of ten independent 100 ns MD ones, are carried out on six systems consisting of BRD7 and BRD9 bound by 4L2, 5U6, and 6KT so as to acquire reasonably conformational sampling on BRD7 and BRD9. Our studies demonstrate that the structural flexibility of two loops L1 and L3 in BRD7 is higher than that of BRD9, furthermore these two domains display various internal dynamics behavior. On the basis of MM-GBSA calculations, binding affinities of 4L2, 5U6, and 6KT to BRD9 is evidently enhanced compare to that of three inhibitors to BRD7, more interestingly, it is uncovered that the entropy changes play key roles in binding selectivity of 4L2, 5U6, and 6KT toward BRD7 and BRD9. In summary, 4L2 shows better selectivity toward BRD7 than BRD9, while 5U6 and 6KT possess a more favorable bind ability to BRD9 than BRD7. Besides, residue-based free energy decomposition calculations also identify that four common residues (F155, F44), (V160, V49), (Y168, Y57), and (Y217, Y106) pertaining to (BRD7, BRD9), produce significant binding difference of inhibitors to BRD7 and BRD9, suggesting that these residues are mainly responsible for the binding selectivity of inhibitors towards BRD7 and BRD9. This study is also expected to provide molecular mechanism and structure affinity relationship for design of highly selective inhibitors targeting BRD7 and BRD9.
Conflicts of interest
No potential conflict of interest was reported by the authors.
Acknowledgements
This work is supported by the National Natural Science Foundation of China (No. 21863003 and 61762048), the Jiangxi Provincial Natural Science Foundation (No. 20202BABL203015), and the Shandong Provincial Natural Science Foundation (No. ZR2021MA069). The authors sincerely thank Prof. Jianzhong Chen (School of Science, Shandong Jiaotong University, Jinan 250357, China) for useful discussions and invaluable comments.
References
- S. G. Smith and M. M. Zhou, ACS Chem. Biol., 2016, 11, 598–608 CrossRef CAS PubMed
. - P. Filippakopoulos, S. Picaud, M. Mangos, T. Keates, J.-P. Lambert, D. Barsyte-Lovejoy, I. Felletar, R. Volkmer, S. Müller, T. Pawson, A.-C. Gingras, C. H. Arrowsmith and S. Knapp, Cell, 2012, 149, 214–231 CrossRef CAS PubMed
. - R. Sanchez, J. Meslamani and M.-M. Zhou, Biochim. Biophys. Acta, Gene Regul. Mech., 2014, 1839, 676–685 CrossRef CAS PubMed
. - M. Wanior, A. Krämer, S. Knapp and A. C. Joerger, Oncogene, 2021, 40, 3637–3654 CrossRef CAS PubMed
. - C. H. Arrowsmith, C. Bountra, P. V. Fish, K. Lee and M. Schapira, Nat. Rev. Drug Discovery, 2012, 11, 384–400 CrossRef CAS PubMed
. - O. M. H. Salo-Ahen, I. Alanko, R. Bhadane, A. M. J. J. Bonvin, R. V. Honorato, S. Hossain, A. H. Juffer, A. Kabedev, M. Lahtela-Kakkonen, A. S. Larsen, E. Lescrinier, P. Marimuthu, M. U. Mirza, G. Mustafa, A. Nunes-Alves, T. Pantsar, A. Saadabadi, K. Singaravelu and M. Vanmeert, Processes, 2021, 9, 71 CrossRef CAS
. - X. Zhu, Y. Liao and L. Tang, OncoTargets Ther., 2020, 13, 13191–13200 CrossRef CAS
. - V. Zoppi, S. J. Hughes, C. Maniaci, A. Testa, T. Gmaschitz, C. Wieshofer, M. Koegl, K. M. Riching, D. L. Daniels, A. Spallarossa and A. Ciulli, J. Med. Chem., 2019, 62, 699–726 CrossRef CAS PubMed
. - W. Niu, Y. Luo, X. Wang, Y. Zhou, H. Li, H. Wang, Y. Fu, S. Liu, S. Yin, J. Li, R. Zhao, Y. Liu, S. Fan, Z. Li, W. Xiong, X. Li, G. Li, C. Ren, M. Tan and M. Zhou, Cell Death Dis., 2018, 9, 519 CrossRef PubMed
. - X. Yu, Z. Li and J. Shen, Am. J. Transl. Res., 2016, 8, 742–748 CAS
. - R. Zhao, Y. Liu, C. Wu, M. Li, Y. Wei, W. Niu, J. Yang, S. Fan, Y. Xie, H. Li, W. Wang, Z. Zeng, W. Xiong, X. Li, G. Li and M. Zhou, Front. Cell Dev. Biol., 2021, 9, 659392 CrossRef PubMed
. - C. M. Bell, P. Raffeiner, J. R. Hart and P. K. Vogt, Cancers, 2019, 11, 1634 CrossRef CAS PubMed
. - A. Alpsoy, S. M. Utturkar, B. C. Carter, A. Dhiman, S. E. Torregrosa-Allen, M. P. Currie, B. D. Elzey and E. C. Dykhuizen, Cancer Res., 2021, 81, 820–833 CrossRef CAS PubMed
. - Q. Zhou, J. Huang, C. Zhang, F. Zhao, W. Kim, X. Tu, Y. Zhang, S. Nowsheen, Q. Zhu, M. Deng, Y. Chen, B. Qin, K. Luo, B. Liu, Z. Lou, R. W. Mutter and J. Yuan, Nat. Commun., 2020, 11, 2639 CrossRef CAS PubMed
. - D. Fang, M.-R. Wang, J.-L. Guan, Y.-Y. Han, J.-Q. Sheng, D.-A. Tian and P.-Y. Li, Exp. Cell Res., 2021, 406, 112727 CrossRef CAS PubMed
. - H. Huang, Y. Wang, Q. Li, X. Fei, H. Ma and R. Hu, Cancer Lett., 2019, 446, 81–89 CrossRef CAS PubMed
. - J. Su, X. Liu, S. Zhang, F. Yan, Q. Zhang and J. Chen, Chem. Biol. Drug Des., 2019, 93, 163–176 CrossRef CAS PubMed
. - A. E. Burrows, A. Smogorzewska and S. J. Elledge, Proc. Natl. Acad. Sci. U. S. A., 2010, 107, 14280 CrossRef CAS
. - J. Drost, F. Mantovani, F. Tocco, R. Elkon, A. Comel, H. Holstege, R. Kerkhoven, J. Jonkers, P. M. Voorhoeve, R. Agami and G. Del Sal, Nat. Cell Biol., 2010, 12, 380–389 CrossRef CAS PubMed
. - H.-T. Huang, S.-M. Chen, L.-B. Pan, J. Yao and H.-T. Ma, Oncol. Rep., 2015, 33, 283–291 CrossRef CAS PubMed
. - W.-J. Wu, K.-S. Hu, D.-L. Chen, Z.-L. Zeng, H.-Y. Luo, F. Wang, D.-S. Wang, Z.-Q. Wang, F. He and R.-H. Xu, Eur. J. Clin. Invest., 2013, 43, 131–140 CrossRef CAS PubMed
. - Y. Hu, C. Jiang, B. Li, L. Zhou, R. Xu, Y. Guo, Y. Cao, G. Cao and S. Lu, CrystEngComm, 2020, 22, 5841–5853 RSC
. - P. G. K. Clark, D. J. Dixon and P. E. Brennan, Drug Discov. Today, 2016, 19, 73–80 CrossRef PubMed
. - R. M. Karim, A. Chan, J.-Y. Zhu and E. Schönbrunn, J. Med. Chem., 2020, 63, 3227–3237 CrossRef CAS PubMed
. - M. A. Clegg, P. Bamborough, C.-w. Chung, P. D. Craggs, L. Gordon, P. Grandi, M. Leveridge, M. Lindon, G. M. Liwicki, A.-M. Michon, J. Molnar, I. Rioja, P. E. Soden, N. H. Theodoulou, T. Werner, N. C. O. Tomkinson, R. K. Prinjha and P. G. Humphreys, J. Med. Chem., 2020, 63, 5816–5840 CrossRef CAS PubMed
. - S. W. Park and J. M. Lee, Int. J. Mol. Sci., 2020, 21, 7127 CrossRef CAS PubMed
. - F. D. Prieto-Martínez and J. L. Medina-Franco, Chapter Five – Current advances on the development of BET inhibitors: insights from computational methods, in Advances in Protein Chemistry and Structural Biology, ed. T. Karabencheva-Christova and C. Christov, Academic Press, 2020, vol. 122, pp. 127–180 Search PubMed
. - L. Duan, S. Dong, K. Huang, Y. Cong, S. Luo and J. Z. H. Zhang, Phys. Chem. Chem. Phys., 2021, 23, 2025–2037 RSC
. - Y. Miao, W. Sinko, L. Pierce, D. Bucher, R. C. Walker and J. A. McCammon, J. Chem. Theory Comput., 2014, 10, 2677–2689 CrossRef CAS PubMed
. - J. Chen, S. Zhang, W. Wang, L. Pang, Q. Zhang and X. Liu, J. Chem. Inf. Model., 2021, 61, 1954–1969 CrossRef CAS PubMed
. - S. S. Liang, X. G. Liu, Y. X. Cui, S. L. Zhang, Q. G. Zhang and J. Z. Chen, SAR QSAR Environ. Res., 2021, 32, 573–594 CrossRef CAS PubMed
. - S. Liang, X. Liu, S. Zhang, M. Li, Q. Zhang and J. Chen, Phys. Chem. Chem. Phys., 2022, 24, 1743–1759 RSC
. - J. Wang, A. Alekseenko, D. Kozakov and Y. Miao, Front. Mol. Biosci., 2019, 6, 112 CrossRef CAS PubMed
. - J. Wang and Y. Miao, J. Phys. Chem. B, 2019, 123, 6462–6473 CrossRef CAS PubMed
. - S. L. Wu, J. Zhao, H. B. Sun, H. Y. Li, Y. Y. Yin and L. L. Zhang, SAR QSAR Environ. Res., 2021, 32, 221–246 CrossRef CAS PubMed
. - L. Wang, Y. Wang, H. Sun, J. Zhao and Q. Wang, Chem. Phys. Lett., 2019, 736, 136785 CrossRef CAS
. - M.-J. Yang, X.-Q. Pang, X. Zhang and K.-L. Han, J. Struct. Biol., 2011, 173, 57–66 CrossRef CAS PubMed
. - G. Hu, H. Li, S. Xu and J. Wang, Int. J. Mol. Sci., 2020, 21, 1926 CrossRef CAS
. - W. Xue, F. Yang, P. Wang, G. Zheng, Y. Chen, X. Yao and F. Zhu, ACS Chem. Neurosci., 2018, 9, 1128–1140 CrossRef CAS PubMed
. - Y. Wang, S. Wu, L. Wang, Z. Yang, J. Zhao and L. Zhang, RSC Adv., 2021, 11, 745–759 RSC
. - J. Chen, J. Wang, B. Yin, L. Pang, W. Wang and W. Zhu, ACS Chem. Neurosci., 2019, 10, 4303–4318 CrossRef CAS PubMed
. - J. Chen, X. Liu, S. Zhang, J. Chen, H. Sun, L. Zhang and Q. Zhang, Phys. Chem. Chem. Phys., 2020, 22, 2262–2275 RSC
. - L. Duan, X. Guo, Y. Cong, G. Feng, Y. Li and J. Z. H. Zhang, Front. Chem., 2019, 7, 540 CrossRef CAS PubMed
. - J. Chen, L. Wang, W. Wang, H. Sun, L. Pang and H. Bao, Comput. Biol. Med., 2021, 135, 104639 CrossRef CAS PubMed
. - L. C. T. Pierce, R. Salomon-Ferrer, C. A. F. de Oliveira, J. A. McCammon and R. C. Walker, J. Chem. Theory Comput., 2012, 8, 2997–3002 CrossRef CAS PubMed
. - Y. Miao, V. A. Feher and J. A. McCammon, J. Chem. Theory Comput., 2015, 11, 3584–3595 CrossRef CAS PubMed
. - J. Chen, B. Yin, W. Wang and H. Sun, ACS Chem. Neurosci., 2020, 11, 1811–1826 CrossRef CAS PubMed
. - J. Wang, P. R. Arantes, A. Bhattarai, R. V. Hsu, S. Pawnikar, Y.-m. M. Huang, G. Palermo and Y. Miao, Wiley Interdiscip. Rev.: Comput. Mol. Sci., 2021, 11, e1521 CAS
. - J. Wang and Y. Miao, J. Chem. Phys., 2020, 153, 154109 CrossRef CAS PubMed
. - J. Chen, W. Wang, L. Pang and W. Zhu, Phys. Chem. Chem. Phys., 2020, 22, 21238–21250 RSC
. - J. Chen, Q. Zeng, W. Wang, Q. Hu and H. Bao, RSC Adv., 2022, 12, 1742–1757 RSC
. - W. Wang and P. A. Kollman, Proc. Natl. Acad. Sci. U. S. A., 2001, 98, 14937–14942 CrossRef CAS
. - J. Wang, P. Morin, W. Wang and P. A. Kollman, J. Am. Chem. Soc., 2001, 123, 5221–5230 CrossRef CAS PubMed
. - W. Wang and P. A. Kollman, J. Mol. Biol., 2000, 303, 567–582 CrossRef CAS PubMed
. - G. Hu and J. Wang, Eur. J. Med. Chem., 2014, 74, 726–735 CrossRef CAS PubMed
. - W. Xue, P. Wang, G. Tu, F. Yang, G. Zheng, X. Li, X. Li, Y. Chen, X. Yao and F. Zhu, Phys. Chem. Chem. Phys., 2018, 20, 6606–6616 RSC
. - J. Chen, W. Wang, H. Sun, L. Pang and H. Bao, Comput. Biol. Med., 2021, 134, 104485 CrossRef CAS PubMed
. - J. Chen, X. Wang, T. Zhu, Q. Zhang and J. Z. H. Zhang, J. Chem. Inf. Model., 2015, 55, 1903–1913 CrossRef CAS PubMed
. - H. Tzoupis, G. Leonis, T. Mavromoustakos and M. G. Papadopoulos, J. Chem. Theory Comput., 2013, 9, 1754–1764 CrossRef CAS PubMed
. - J. Chen, X. Wang, L. Pang, J. Z. H. Zhang and T. Zhu, Nucleic Acids Res., 2019, 47, 6618–6631 CrossRef CAS PubMed
. - M. Aldeghi, A. Heifetz, M. J. Bodkin, S. Knapp and P. C. Biggin, J. Am. Chem. Soc., 2017, 139, 946–957 CrossRef CAS PubMed
. - M. Naïm, S. Bhat, K. N. Rankin, S. Dennis, S. F. Chowdhury, I. Siddiqi, P. Drabik, T. Sulea, C. I. Bayly, A. Jakalian and E. O. Purisima, J. Chem. Inf. Model., 2007, 47, 122–133 CrossRef PubMed
. - Y. Gao, T. Zhu and J. Chen, Chem. Phys. Lett., 2018, 706, 400–408 CrossRef CAS
. - F. Yan, X. Liu, S. Zhang, Q. Zhang and J. Chen, Chem. Biol. Drug Des., 2020, 95, 87–103 CrossRef CAS PubMed
. - P. Gainza, F. Sverrisson, F. Monti, E. Rodolà, D. Boscaini, M. M. Bronstein and B. E. Correia, Nat. Methods, 2020, 17, 184–192 CrossRef CAS PubMed
. - W. F. D. Bennett, S. He, C. L. Bilodeau, D. Jones, D. Sun, H. Kim, J. E. Allen, F. C. Lightstone and H. I. Ingólfsson, J. Chem. Inf. Model., 2020, 60, 5375–5381 CrossRef CAS PubMed
. - J. Xing, W. Lu, R. Liu, Y. Wang, Y. Xie, H. Zhang, Z. Shi, H. Jiang, Y.-C. Liu, K. Chen, H. Jiang, C. Luo and M. Zheng, J. Chem. Inf. Model., 2017, 57, 1677–1690 CrossRef CAS PubMed
. - J. Su, X. Liu, S. Zhang, F. Yan, Q. Zhang and J. Chen, J. Biomol. Struct. Dyn., 2018, 36, 1212–1224 CrossRef CAS PubMed
. - J. Su, X. Liu, S. Zhang, F. Yan, Q. Zhang and J. Chen, Chem. Biol. Drug Des., 2018, 91, 828–840 CrossRef CAS PubMed
. - P. G. K. Clark, L. C. C. Vieira, C. Tallant, O. Fedorov, D. C. Singleton, C. M. Rogers, O. P. Monteiro, J. M. Bennett, R. Baronio, S. Müller, D. L. Daniels, J. Méndez, S. Knapp, P. E. Brennan and D. J. Dixon, Angew. Chem. Int. Ed., 2015, 54, 6217–6221 CrossRef CAS PubMed
. - N. Wang, F. Li, H. Bao, J. Li, J. Wu and K. Ruan, ChemBioChem, 2016, 17, 1456–1463 CrossRef CAS PubMed
. - T. Ichiye and M. Karplus, Proteins, 1991, 11, 205–217 CrossRef CAS
. - J. Chen, S. Zhang, W. Wang, H. Sun, Q. Zhang and X. Liu, ACS Chem. Neurosci., 2021, 12, 2591–2607 CrossRef CAS PubMed
. - D. Song, R. Luo and H.-F. Chen, J. Chem. Inf. Model., 2017, 57, 1166–1178 CrossRef CAS PubMed
. - J. A. Maier, C. Martinez, K. Kasavajhala, L. Wickstrom, K. E. Hauser and C. Simmerling, J. Chem. Theor. Comput., 2015, 11, 3696–3713 CrossRef CAS PubMed
. - W. L. Jorgensen, J. Chandrasekhar, J. D. Madura, R. W. Impey and M. L. Klein, J. Chem. Phys., 1983, 79, 926–935 CrossRef CAS
. - A. Jakalian, B. L. Bush, D. B. Jack and C. I. Bayly, J. Comput. Chem., 2000, 21, 132–146 CrossRef CAS
. - A. Jakalian, D. B. Jack and C. I. Bayly, J. Comput. Chem., 2002, 23, 1623–1641 CrossRef CAS PubMed
. - J. Wang, R. M. Wolf, J. W. Caldwell, P. A. Kollman and D. A. Case, J. Comput. Chem., 2004, 25, 1157–1174 CrossRef CAS PubMed
. - U. Essmann, L. Perera, M. L. Berkowitz, T. Darden, H. Lee and L. G. Pedersen, J. Chem. Phys., 1995, 103, 8577–8593 CrossRef CAS
. - J.-P. Ryckaert, G. Ciccotti and H. J. C. Berendsen, J. Comput. Phys., 1977, 23, 327–341 CrossRef CAS
. - T. Darden, D. York and L. Pedersen, J. Chem. Phys., 1993, 98, 10089–10092 CrossRef CAS
. - A. W. Götz, M. J. Williamson, D. Xu, D. Poole, S. Le Grand and R. C. Walker, J. Chem. Theor. Comput., 2012, 8, 1542–1555 CrossRef PubMed
. - R. Salomon-Ferrer, A. W. Götz, D. Poole, S. Le Grand and R. C. Walker, J. Chem. Theory Comput., 2013, 9, 3878–3888 CrossRef CAS PubMed
. - R. M. Crean, C. R. Pudney, D. K. Cole and M. W. van der Kamp, J. Chem. Inf. Model., 2022, 62, 577–590 CrossRef CAS PubMed
. - C. Wang, D. A. Greene, L. Xiao, R. Qi and R. Luo, Front. Mol. Biosci., 2018, 4, 87 CrossRef PubMed
. - E. Wang, H. Sun, J. Wang, Z. Wang, H. Liu, J. Z. H. Zhang and T. Hou, Chem. Rev., 2019, 119, 9478–9508 CrossRef CAS PubMed
. - H. Sun, Y. Li, M. Shen, S. Tian, L. Xu, P. Pan, Y. Guan and T. Hou, Phys. Chem. Chem. Phys., 2014, 16, 22035–22045 RSC
. - H. Sun, Y. Li, S. Tian, L. Xu and T. Hou, Phys. Chem. Chem. Phys., 2014, 16, 16719–16729 RSC
. - A. Onufriev, D. Bashford and D. A. Case, Proteins, 2004, 55, 383–394 CrossRef CAS PubMed
. - H. Gohlke, C. Kiel and D. A. Case, J. Mol. Biol., 2003, 330, 891–913 CrossRef CAS PubMed
. - B. R. Miller, T. D. McGee, J. M. Swails, N. Homeyer, H. Gohlke and A. E. Roitberg, J. Chem. Theory Comput., 2012, 8, 3314–3321 CrossRef CAS PubMed
. - B. Xu, H. Shen, X. Zhu and G. Li, J. Comput. Chem., 2011, 32, 3188–3193 CrossRef CAS PubMed
. - J. Chen, S. Zhang, Q. Zeng, W. Wang, Q. Zhang and X. Liu, Front. Mol. Biosci., 2022, 9, 912518 CrossRef PubMed
. - L.-Q. Yang, P. Sang, R.-P. Zhang and S.-Q. Liu, RSC Adv., 2017, 7, 42094–42104 RSC
. - D. R. Roe and T. E. Cheatham, J. Chem. Theory Comput., 2013, 9, 3084–3095 CrossRef CAS PubMed
. - F. Yan, X. Liu, S. Zhang, J. Su, Q. Zhang and J. Chen, Int. J. Mol. Sci., 2018, 19, 2496 CrossRef PubMed
.
|
This journal is © The Royal Society of Chemistry 2022 |
Click here to see how this site uses Cookies. View our privacy policy here.