DOI:
10.1039/D2RA02022J
(Paper)
RSC Adv., 2022,
12, 14716-14723
New strategy for clinical etiologic diagnosis of acute ischemic stroke and blood biomarker discovery based on machine learning†
Received
29th March 2022
, Accepted 9th May 2022
First published on 16th May 2022
Abstract
Acute ischemic stroke (AIS) is a syndrome characterized by high morbidity, prevalence, mortality, recurrence and disability. The longer the delay before proper treatment of a stroke, the greater the likelihood of brain damage and disability. Computed tomography and nuclear magnetic resonance are the primary choices for fast diagnosis of AIS in the early stage, which can provide certain information about infarction location and degree, and even the vascular distribution of lesions responsible for strokes. However, this is quite difficult to achieve in small clinics or at-home diagnoses. Hematology tests could quickly obtain a large number of pathology-related indicators, and offer an effective method for rapid AIS diagnosis when combined with the machine learning technique. To explore a reliable, predictable method for early clinical etiologic diagnosis of AIS, a retrospective study was deployed on 456 AIS patients at the early stage and 28 reference subjects without the symptoms of AIS, by means of the selected significant traits amongst 64 clinical and blood traits in conjunction with powerful machine learning strategies. Five representative biomarkers were closely related to cardioembolic (CE), 22 to large artery atherosclerosis (LAA), and 15 to small vessel occlusion (SVO) strokes, respectively. With these biomarkers, different etiologic subtypes of stroke patients were determined with high accuracy of >0.73, sensitivity of >0.73, and specificity of >0.70, which was comparable to the accuracy obtained in the emergency department by clinical diagnosis. The proposed method may offer an alternative strategy for the etiologic diagnosis of AIS at the early stage when integrating significant blood traits into machine learning.
1. Introduction
Strokes are a leading cause of death and disability worldwide from the viewpoint of clinical practice.1,2 They often give rise to a serious economic burden on patients and even society. Cerebral ischemia caused by atherosclerosis and/or vascular embolism is the most common factor, which may result in ischemic stroke. To date, more attention has been paid to ischemic stroke due to the high incidence, high prevalence, high mortality, high recurrence rate, and high disability rate. Acute ischemic stroke (AIS) is a syndrome related to several distinct pathologies. In general, it includes five subtypes, such as large artery atherosclerosis (LAA), small vessel occlusion (SVO), cardioembolic (CE) stroke, stroke of other determined etiology (OC), and stroke of undetermined etiology (UND), according to the Trial of ORG 10172 in Acute Stroke Treatment (TOAST) criteria.3 Treatment and prognosis outcomes for different subtypes are quite variable. Specifically, SVO stroke has shown the most favorable prognosis, whereas CE stroke manifested the poorest. Antiplatelet polytherapy was associated with a better prognosis than monotherapy in LAA stroke, and intensive antithrombotic strategies were better than antiplatelet monotherapy in CE subtype. Also, the risk of death was higher with anticoagulant therapy in patients with SVO subtype.4 Platelet activation and coagulation play an important role in CE and LAA, but much less in SVO. Antiplatelet therapy does not have a significant effect on SVO.5 Besides, clinical outcomes and stroke severity may differ in different stroke subtypes. Kim et al. reported that the difference between the previous antiplatelet users and nonusers was significant only in patients with LAA, yet not in those CE and SVO.6 Therefore, understanding AIS in terms of the subtypes and making an accurate prediction at early stage is valuable for appropriate prognosis and management plans.
At present, computed tomography (CT) and nuclear magnetic resonance (NMR) are the main means for clinical diagnosis of AIS in the early stage, which provide rich information not only for the detection of infarction, but also for the determination of the location and degree, or the vascular distribution of the lesions responsible for the stroke.7 For the AIS patients, it is important to quickly determine the subtypes, and then receive an effective thrombolytic therapy in time because such a thrombolytic therapy must be rapidly initiated within a narrow time window of a few hours. Studies have shown that the thrombolytic time window should be within 4.5 hours of the onset of stroke symptoms in patients.8 A comprehensive examination, e.g., CT or NMR, could lead to an accurate diagnosis of stroke, yet is costly and time-consuming. Most importantly, it may result in delays in timely treatment. Therefore, there is a strong need to explore new rapid and effective alternative methods for facilitating the easy diagnosis of strokes. By the methods, the stroke can be essentially ascertained, so that appropriate treatment can be timely conducted, and prognosis outcome forecast.
Hematology testing is one of the fast, convenient, economical methods to understand the overall health status of patients, and can be simply completed in nearly all primary hospitals within half an hour. It has been proved that the hematology traits discover very important information about how the stroke progresses.9 For example, blood cell characteristics, coagulation factors, platelet activation and aggregation pathways are related to thrombosis. These biomarkers play an important role in revealing the pathological mechanism of the occurrence and development of stroke.10 However, the hematological traits are probably influenced by many factors, such as infection, inflammation, blood-clotting disorders, leukemia and the body's response to chemotherapy treatments. Hence, it is still challenging to diagnose stroke only by blood traits or identify specific biomarkers in blood traits through conventional statistical methods.
Machine learning (ML) is a powerful tool and commonly described as a strategy or programme to relate multiple features of the objectives under investigation. ML has been widely applied in many scientific fields, such as chemistry,11,12 biology,13 medicine,14 and so on. ML models were established to predict the composition of complex systems by using molecular spectroscopy,15–18 or to explore any quantitative structure–activity relationship through designing a large number of active molecules for disease treatment.19 Recently, ML models were yielded to address the problems in the subtypes of AIS,20 salvageable tissue lesion21,22 and outcomes,22 etc. The most model outputs were likely desirable, however, failed to substantially shorten the time from symptom onset to treatment, since the models were commonly built upon neuroimaging data, which were relatively time-consuming to acquire.23,24 To date, there is a lack of well-validated ML models for ischemic classification based on blood traits.
In this work, AIS was investigated concerning its subtypes by means of hematology traits and ML methods. For the clinical etiologic diagnosis of CE, LAA, and SVO strokes at early stage, several machine learning models were built with typical blood features. The results showed that different subtypes of stroke patients were etiologically determined with high accuracy of >0.73, sensitivity of >0.73, and specificity of >0.70. To our knowledge, the study could be the first report on well-validated diagnosis models for AIS. The hematology biomarkers might imply new root causes relating to the different subtypes of AIS. Machine learning methods were suited to handle the imbalance and missing values in clinical hematology trait data which could probably hinder the proper stroke diagnosis.
2. Materials and methods
2.1 Patient
This retrospective study involved a total of 476 patients hospitalized in the Department of Neurology, the Affiliated Hospital of Guizhou Medical University, China from July 2018 to January 2020. The study was reviewed and approved by the Ethic Committee of the Affiliated Hospital of Guizhou Medical University (Approval number: 2020104K). All the patients signed off a necessarily informed consent form. The patients included a total of 456 AISs at early stage and another 28 reference subjects. For AIS, the inclusion criteria were that the patients must meet the Diagnosis of the Chinese Guidelines for the Diagnosis and Treatment of Acute Ischemic Stroke 2018. The subtypes of AIS were determined by a medical professional according to clinical diagnosis and neuroimaging data.
2.2 Clinical chemistry data
According to the TOAST criteria, 456 AISs were clinically diagnosed by comprehensive imaging evaluation and divided into five subtypes, i.e., 65 patients in CE, 157 in LAA, 165 in SVO, 44 in OC, and 19 in UND. Notably, two patients were specifically determined within two subtypes of CE and LAA strokes. Only the three subtypes, i.e., CE, LAA, and SVO strokes, were significantly considered for diagnosis modelling. There were 64 clinical and blood features/variables collected for the individual patients, including 10 general information, 3 blood routine indicators, 5 blood coagulation factors, 42 biochemical indicators, 3 myocardial markers, and one immune indicator (see details in the given Table S1† in the ESI). The general information was generated by a face-to-face survey. The blood routine indicators were measured on an XN2000 automatic blood routine analyzer (Sysmex, Japan). The blood coagulation factors were conducted on an automatic coagulation analyzer (STA-R-EVOLUTION, French). The biochemical indicators were produced on an E602 automatic biochemical analyzer (Cobas, Switzerland). The myocardial markers were acquired on an E702 automatic biochemical analyzer (Cobas, Switzerland). The immune indicator was obtained by using an IMMAGE800 automatic specific protein analyzer (Beckman Coulter, American).
Close inspection of the data indicated that only 36 features of the total 64 ones were complete and the remaining 28 features contained many missing values up to a pretty large percentage of 20.94%. Fig. 1a shows the distribution of missing values in the data along with the columns in clinical features, of which the corresponding values were scaled to the range of 0–1 for clarification. One can observe that the most missing value occurred in the personal general information, α-HBDH, TG, TC, HDL-C, LDL-C, RC, LP(a), Hcy, ApoA1, ApoB, ApoA1/ApoB, hs-CTnT, NT-proBNP, Mb and RF, respectively. As is known, all these features were relevant to AIS to a certain extent. Therefore, the missing values should be carefully treated in the data analysis.
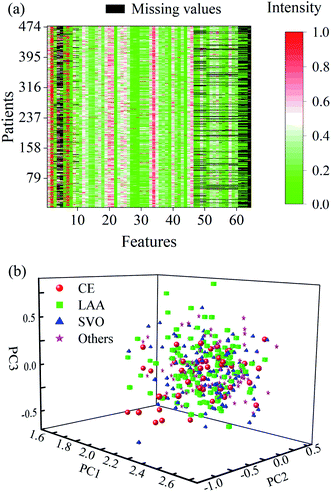 |
| Fig. 1 (a) Clinical data with missing values, (b) distribution of PCA scores obtained from the 36 complete features of all the patients. | |
The clinical data comprised of only 36 complete features of all the patients were initially run with the principal component analysis (PCA), and three principal components (PCs) were used. As a consequence, a random normal distribution can be seen from the resultant PC scores (Fig. 1b). No obvious difference appeared in these scattering scores among the subtypes. It may suggest that the blood routine indicators contain poor etiologic information about AIS, and it is difficult to achieve a reliable diagnostic model of AIS only by a single routine blood trait combined with conventional statistical methods.
2.3 Machine learning
Herein, ML method may be efficient for diagnosing AISs because it could deal with multiple features, rather than relying on just one or two factors, to make judgments in traditional clinical practice.
2.3.1 Data cleaning and missing value imputation. Missing values are common in a retrospective study due to uncertain diagnosis or irresistible causes. Sometimes, data cleaning with respect to outlier detection also results in some missing values. The data far away from the mean value were considered as outliers and had to be removed. However, missing values are challenging to most ML methods which cannot directly handle the data unless the values can be reasonably compensated. Trimmed scores regression (TSR)25 has been regarded as a powerful tool for missing value imputation. Based on the prediction from the known values, missing values can be calculated iteratively. Herein, TSR was used to impute the missing values caused by the uncertain diagnosis and data cleaning.
2.3.2 Feature selection. The fact that practical data are generally redundant or there exist a lot of uninformative features in the data often makes a multivariate calibration model fail to predict new objects. Feature (or variable) selection has been proved critical to enhancing the model prediction performance in our previous studies.16–18 In this study, the rank feature for classification using minimum redundancy maximum relevance (MRMR)26 was employed to spot the most representative blood features and meanwhile reduce the redundancy of data. The algorithm was performed by compensating the redundancy and relevance goals with specified parameters.
2.3.3 Multivariate calibration. Decision tree was employed for building the diagnosis model in the study. In a decision tree, a hierarchical tree structure was simulated, of which the leaves represent class labels and branches symbolize the conjunctions of features linking to those class labels. However, there was a strong class imbalance in the real clinical data, and a regular decision tree showed a poor performance in prediction. Thus, we resorted to the hybrid data sampling/boosting (RUSBoost) algorithm,27 which is an efficient algorithm for dealing with data with a certain imbalance dataset. RUSBoost was executed in a random under-sampling (RUS) and boosting procedure with the weighted average of multiple weak learners generated by the decision tree. That is, using RUS on the original data with imbalance class in calculation, many sub-data can be hence produced with balance class, and weak learners generated by the decision tree. Then, based on these weak learners an ensemble model can be averaged with the weight determined by maximization prediction accuracy. The number of weak learners is the key parameter for trading off the efficiency and overfitting of the assembled model. This decision tree could largely enhance the performance of machine learning model in prediction.
2.3.4 Figure of merits. Herein, the figure of merits (FOMs) in terms of accuracy, sensitivity and specificity28 were adopted for evaluating the performance of the model. The FOMs are commonly defined as follows:
where TP, TN, FP and FN represent the number of true positive, true negative, false positive and false negative patient samples predicted by the model, respectively. A large accuracy value suggests a satisfactory prediction for all the patients, while large sensitivity and specificity mean a promising prediction for positive and negative samples, respectively.Receiver operating characteristic (ROC) curve has been also commonly used to validate the efficiency of classification models. ROC curve was created by plotting the true positive rate (TPR) against false positive rate (FPR) at various thresholds. The area under ROC curves (AUC) provided a measure of the model efficiency, ranging from 0 to 1. The larger the AUC is, the closer the model to a perfect classifier.
Before calibration, the patients were randomly divided into a calibration set and a validation set with a split ratio of 4
:
1. Consequently, 381 patient samples in the calibration set were used for establishing the machine learning models, and the validation set was comprised of the remaining 95 patient samples, and utilized for validating the prediction efficacy of the calibration models. The AIS subtypes, CE, LAA and SVO were mainly considered for modelling, whereas SUD and OC were ignored because of the lack of diagnosis patients. Fig. 2 represents the schematic diagram illustrating the strategy for clinical etiologic diagnosis of AIS and blood biomarker discovery.
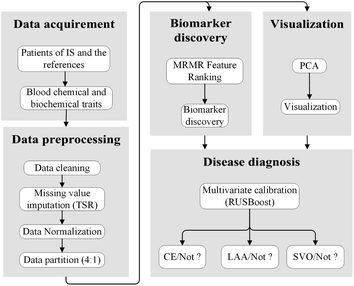 |
| Fig. 2 Schematic diagram of strategy for clinical etiologic diagnosis of AIS and blood biomarker discovery. | |
3. Results and discussion
3.1 Data processing
Data cleaning was first conducted along with the columns for cleaning the unreliable records or measurements from the clinical variables. As a result, 323 outlying records or measurements were detected and replaced with missing values. These outlying records or measurements had values that exceeded the mean absolute deviation away from the mean value.
TSR was then implemented to complete the imputation of missing values among the clinical data. Note that the key parameter of iteration number was set to an empirical value of 2000 in request. PCA was also run in the regression steps to reduce the data redundancy, with only two PCs. Fig. 3a delineates the clinical data with all the missing values imputed. Particular attention should be given to the 11–46 columns on account of the replacement of outlying values. Compared with the original data in Fig. 1a, one can see that the gaps caused by missing values were now filled with proper figures in the range of 0 up to 1.
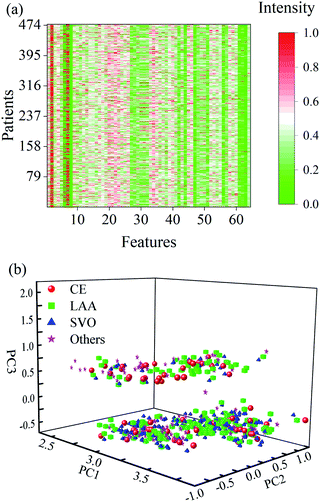 |
| Fig. 3 (a) Clinical data with missing values imputation, (b) distribution of PCA scores obtained from 64 traits of all the patients after data imputation. | |
Afterwards, PCA was rerun on these data after the missing value imputation, with three specified PCs. The distribution of the resulting PC scores of 64 features of all the patients clearly shows two distinct clusters along with the 3rd principal component (Fig. 3b). The correlation coefficients between the scores and subtypes of AISs were also calculated, and a slight increase can be observed after missing value imputation (see details in the given Table S2† in the ESI). This implies that additional information with respect to the etiologic diagnosis of stroke among the patients was discovered and the data imputation of missing values was necessary.
3.2 Blood biomarker discovery
MRMR was carried out to identify significant clinical features that were desired to enable to discriminate of the subtypes of CE, LAA, and SVO of strokes. For this purpose, a new strategy was taken in a Monte Carlo sampling protocol.29 With a stringent 80% sampling ratio, the entire calibration set was randomly split into a subset that comprised 305 patients. The feature selection was subsequently implemented on such a calibration subset, and feature scores were calculated for the use of measuring the importance and uncertainty of each feature. This procedure was repeated in 100 runs. Consequently, 100 independent calibration subsets were individually produced. The mean value and standard deviation of all the feature scores were computed for each of the 64 clinical and blood features using the 100 individual runs, say mean ± std, n = 100. Fig. 4a displays the obtained feature scores in descending order for diagnosis of CE strokes. One can observe that at the beginning both the mean value and standard deviation of the scores were pretty large. As the feature number increased, there appeared a sharp drop. From the 5th feature onwards, the decreasing trend became slow and down to a flat. Therefore, the first five features were determined as the significant ones which could discriminate the CE from other strokes. These five significant clinical features pointed to NT-proBNP, BUN, PLT, GLB and PA in order (Table 1). The first important feature, N-terminal B-type natriuretic peptide precursor (NT-proBNP) is a neurohormonal peptide secreted by atrial and ventricular myocytes. The level of serum NT-proBNP level is a critical indicator useful for assessing the risk of stroke or death in patients with atrial fibrillation receiving anticoagulant therapy.30 The determination of serum NT-proBNP level during hospitalization can predict the prognostic outcome of patients with heart failure.31 The two features of BUN32 and PA33 are both related to myocardial infarction. PLT plays an important role in the process of thrombosis.34 CE is a mural thrombus in the heart that enters the cerebral artery with the blood flow and blocks the blood vessel, thus is an important complication of heart disease. It is primarily associated with atrial fibrillation regarding left atrial thrombosis, heart valve disease, artificial heart valve, cardiomyopathy, and heart failure. Moreover, CE is closely related to left ventricular thrombosis of myocardial infarction.35 Therefore, the joint detection of these indicators can help identify the subtype of CE on its occasions.
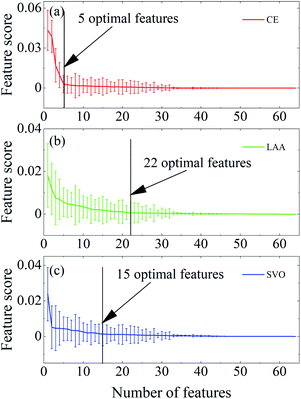 |
| Fig. 4 Feature selection for calculating the importance of 64 clinical and blood traits for the subtypes of (a) CE, (b) LAA and (c) SVO. Error bars represent the standard deviation in n = 100 sampling runs. | |
Table 1 Significant clinical and blood traits identified for diagnosing the CE, LAA and SVO strokes
Subtypes of AIS |
Identified traits |
CE |
NT-proBNP, BUN, PLT, GLB and PA |
LAA |
Hypertension, PT, ApoA1/ApoB, TC, HDL-C, diabetes, age, INR, TT, APTT, sex, Hcy, cTNT, DB, TBA, γ-GGT, DBP, TP, Cys-C, ALB, UA, and α-HBDH |
SVO |
Sex, BUN/Cr, ALB, AST, TBA, NT-proBNP, α-HBDH, CK-MB, DB, Na+, RBC, HCO3–, LDH, BUN, and PA |
As for the subtype of LAA, the significance of 64 features, in terms of feature scores obtained during the feature selection implementation, was presented in Fig. 4b. The descending curve suggested that the first 22 features were important for classifying the LAA subtype. They were in order from the 1st hypertension up to the 22nd α-HBDH (Table 1). It was found in clinical studies that the development of atherosclerosis is ascribed to several classical risk factors including age, gender, hypertension, dyslipidemia (e.g., either the TC and LDL-C increase or the HDL-C decrease) and diabetes.36 Besides, high homocysteine,37 low bile acid,38 high cystatin C,39 low albumin,40 high uric acid41,42 and low bilirubin43 in their levels are all relevant symptoms of the development of atherosclerosis. ApoA1 has an anti-atherosclerotic effect, and ApoB has the opposite effect.44 Therefore, the decrease in the ratio of ApoA1/ApoB is related to the formation of atherosclerosis. Atherosclerosis caused by intracranial and extracranial arteries or their cortical branches to cause obvious vascular stenosis (>50%) or vulnerable plaque is an important mechanism of LAA.45,46 The factors that generally promote the development of atherosclerosis may play a considerable part in the development of LAA. For example, cTNT and α-HBDH in the endogenous coagulation pathway are significantly related to the occurrence and development of LAA,5 though the mechanism is unclear. Hence, they may become a new biomarker of LAA formation.
Likewise, 15 traits were found to be dedicated to the diagnosis of SVO, as shown in Fig. 4c. Table 1 listed these traits in order. About a quarter of arteriolar occlusive strokes have been caused by small vessel disease, including hyalinosis of small arteries at the end of the perforator and atherosclerosis of the main perforator, supported by a history of hypertension and diabetes. The factors leading to atherosclerosis, hypertension, and diabetes may be important to the occurrence and development of SVO. This result indicated that sex is another important feature for SVO. The possible reason may be that estradiol has a specific neuroprotective effect on young females.47 Furthermore, the increased ratio of blood urea nitrogen-to-creatinine in patients with AIS is associated with venous thromboembolism.48 Serum creatine kinase can promote the development of arterial hypertension to a certain extent.49 The increase of RBCs, adenosine diphosphate released by RBC, blood viscosity and the slowdown of blood fluidity can cause and/or increase the aggregation of PLT and thrombosis.49 AST, LDH, CK, CK-MB and α-HBDH are the myocardial zymograms for detecting/testing cardiac function. They are widely used as indicators of myocardial damage caused by SVO. However, the role of the Na+, HCO3−, BUN and PA in the occurrence and development of SVO is unclear. These blood features may be new indicators for the occurrence and development of SVO. The blood features ascertained in this study apart from classical ones could be inspiring indicators for helping discriminate AISs, and may provide a new insight into the disease from a viewpoint of chemical analysis.
3.3 Etiologic diagnosis of strokes
With the significant blood features ascertained in the feature selection step, RUSBoost was undertaken to establish the diagnosis models of strokes by using the calibration set. The optimal number of weak learners was determined for the individual CE, LAA, and SVO strokes by carrying out a 10-fold cross-validation. Meanwhile, the FOMs values were calculated for model evaluation. The details of the resultant models together with the FOMs values were summarized in Table 2.
Table 2 Model performance for diagnosing the CE, LAA and SVO strokes by use of significant traits
|
No. of learners |
Accuracy |
Sensitivity |
Specificity |
CE |
Calibration |
62 |
0.99 |
0.99 |
0.99 |
Validation |
|
0.86 |
0.73 |
0.88 |
![[thin space (1/6-em)]](https://www.rsc.org/images/entities/char_2009.gif) |
LAA |
Calibration |
79 |
0.93 |
0.93 |
0.92 |
Validation |
|
0.77 |
0.76 |
0.77 |
![[thin space (1/6-em)]](https://www.rsc.org/images/entities/char_2009.gif) |
SVO |
Calibration |
60 |
0.94 |
0.95 |
0.94 |
Validation |
|
0.73 |
0.77 |
0.70 |
From the table, one could observe that for the diagnosis of CE strokes, 62 weak learners were trained and assembled into a final calibration model. The model showed pretty good accuracy, sensitivity, and specificity with all their FOM values equal to 0.99, which approached a best maximum limit of 1.00. At the same time, high FOM values were obtained for the validation stroke patients, as were 0.86, 0.73, and 0.88, respectively. This result was very comparable to the literature studies with genetic biomarkers.50 It implied that such a model with the easily acquired blood traits could provide another tool for accurate etiologic determination.
With 79 weak learners, a second model was established for the LAA stroke diagnosis. All three FOM values were larger than 0.92 for the calibration of 128 patients. While those obtained from 29 LAA stroke patients in the validation set were at least greater than 0.76. This seemed fairly satisfactory in that to our knowledge there was no similar validating work undertaken in previous stroke studies, which mostly focused on calibration modelling either due to the lack of representative patients or the overfitting problem of the models.
For the 130 SVO strokes, 60 weak learners could lead to an acceptable diagnosis model. The resulting FOMs were well acceptable, above 0.94 for the calibration patients and 0.70 for the validation comprised of 35 SVO stroke patients, respectively. The overfitting might account for this slight difference between them, and this issue could be solved through a possible enlargement of SVO stroke patients.
Fig. 5 showed the ROC curves of the models obtained with the validation set. One can observe that the results seemed acceptable, as the three curves were far from the diagonal line. The models of CE and LAA were slightly superior to that of SVO on account of larger AUC, but for SVO the AUC also reached a value of 0.78, which is comparable to the accuracy in the emergency department by clinical diagnosis.51 This demonstrated that the models were acceptable for the prediction of unknown patients in practical applications. A significant difference of the present work to those published lied in that: (1) the calibration data were mainly comprised of 64 clinical traits collected from 456 AIS patients, and this was quite easy and fast to acquire; (2) the diagnosis models were developed for three individual subtypes of AIS, and the performance seemed acceptable; and (3) the models were well-validated by independent data.
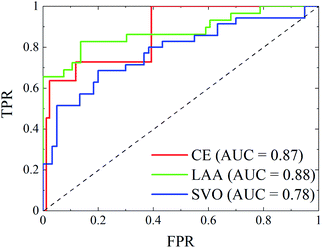 |
| Fig. 5 ROC curves of external validation for clinical etiologic diagnosis of AIS subtypes. | |
4. Conclusions
This study demonstrated that using the hematology traits of patients in conjunction with powerful machine learning methods, one could generate effective diagnosis models for easily discriminating AISs either in LAA, SVO, or CE subtypes, and discover blood biomarker amongst the 64 clinical and blood features. The hematology traits proved to be highly informative and useful for this purpose once significant traits were picked up from 64 features. It offered a new strategy for the etiologic diagnosis of AISs illustrated with the schematic diagram and was completely different from the neuroimaging test.
Author contributions
Jin Zhang: conceptualization, methodology, writing – original draft. Ting Yuan: data curation, writing – original draft. Sixi Wei: writing – original draft. Zhanhui Feng: writing – original draft. Boyan Li: writing and review, methodology, editing. Hai Huang: writing – review & editing.
Conflicts of interest
There are no conflicts to declare.
Acknowledgements
This work was funded by the National Natural Science Foundation of China (Grant No. 22004022, 21864008 and 82060442), Guizhou Provincial Science and Technology Projects (Grant No. ZK[2021]045 and [2018]1130), the Department of Education of Guizhou Province, China (Grant No. KY[2021]163), Guizhou Provincial Innovative Talents Team to H. Huang (Grant No. 2019-5610), Excellent Young Talents Plan of Guizhou Medical University ([2021]104), Guizhou Medical University Foundation (Grant No. 19NSP067 and [2019]002).
Notes and references
- C. O. Johnson, M. Nguyen, G. A. Roth, E. Nichols, T. Alam, D. Abate, F. Abd-Allah, A. Abdelalim, H. N. Abraha, N. M. E. Abu-Rmeileh, O. M. Adebayo, A. M. Adeoye, G. Agarwal, S. Agrawal, A. N. Aichour, I. Aichour, M. T. E. Aichour, F. Alahdab, R. Ali, N. Alvis-Guzman, N. H. Anber, M. Anjomshoa, J. Arabloo, A. Arauz, J. Ärnlöv, A. Arora, A. Awasthi, M. Banach, M. A. Barboza, S. L. Barker-Collo, T. W. Bärnighausen, S. Basu, A. B. Belachew, Y. M. Belayneh, D. A. Bennett, I. M. Bensenor, K. Bhattacharyya, B. Biadgo, A. Bijani, B. Bikbov, M. S. Bin Sayeed, Z. A. Butt, L. Cahuana-Hurtado, J. J. Carrero, F. Carvalho, C. A. Castañeda-Orjuela, F. Castro, F. Catalá-López, Y. Chaiah, P. P.-C. Chiang, J.-Y. J. Choi, H. Christensen, D.-T. Chu, M. Cortinovis, A. A. M. Damasceno, L. Dandona, R. Dandona, A. Daryani, K. Davletov, B. de Courten, V. De la Cruz-Góngora, M. G. Degefa, S. D. Dharmaratne, D. Diaz, M. Dubey, E. E. Duken, D. Edessa, M. Endres, E. J. A. Faraon, F. Farzadfar, E. Fernandes, F. Fischer, L. S. Flor, M. Ganji, A. K. Gebre, T. G. Gebremichael, B. Geta, K. E. Gezae, P. S. Gill, E. V. Gnedovskaya, H. Gómez-Dantés, A. C. Goulart, G. Grosso, Y. Guo, R. Gupta, A. Haj-Mirzaian, A. Haj-Mirzaian, S. Hamidi, G. J. Hankey, H. Y. Hassen, S. I. Hay, M. I. Hegazy, B. Heidari, N. A. Herial, M. A. Hosseini, S. Hostiuc, S. S. N. Irvani, S. M. S. Islam, N. Jahanmehr, M. Javanbakht, R. P. Jha, J. B. Jonas, J. J. Jozwiak, M. Jürisson, A. Kahsay, R. Kalani, Y. Kalkonde, T. A. Kamil, T. Kanchan, A. Karch, N. Karimi, H. Karimi-Sari, A. Kasaeian, T. D. Kassa, H. Kazemeini, A. T. Kefale, Y. S. Khader, I. A. Khalil, E. A. Khan, Y.-H. Khang, J. Khubchandani, D. Kim, Y. J. Kim, A. Kisa, M. Kivimäki, A. Koyanagi, R. K. Krishnamurthi, G. A. Kumar, A. Lafranconi, S. Lewington, S. Li, W. D. Lo, A. D. Lopez, S. Lorkowski, P. A. Lotufo, M. T. Mackay, M. Majdan, R. Majdzadeh, A. Majeed, R. Malekzadeh, N. Manafi, M. A. Mansournia, M. M. Mehndiratta, V. Mehta, G. Mengistu, A. Meretoja, T. J. Meretoja, B. Miazgowski, T. Miazgowski, T. R. Miller, E. M. Mirrakhimov, B. Mohajer, Y. Mohammad, M. Mohammadoo-khorasani, S. Mohammed, F. Mohebi, A. H. Mokdad, Y. Mokhayeri, G. Moradi, L. Morawska, I. Moreno Velásquez, S. M. Mousavi, O. S. S. Muhammed, W. Muruet, M. Naderi, M. Naghavi, G. Naik, B. R. Nascimento, R. I. Negoi, C. T. Nguyen, L. H. Nguyen, Y. L. Nirayo, B. Norrving, J. J. Noubiap, R. Ofori-Asenso, F. A. Ogbo, A. T. Olagunju, T. O. Olagunju, M. O. Owolabi, J. D. Pandian, S. Patel, N. Perico, M. A. Piradov, S. Polinder, M. J. Postma, H. Poustchi, V. Prakash, M. Qorbani, A. Rafiei, F. Rahim, K. Rahimi, V. Rahimi-Movaghar, M. Rahman, M. A. Rahman, C. Reis, G. Remuzzi, A. M. N. Renzaho, S. Ricci, N. L. S. Roberts, S. R. Robinson, L. Roever, G. Roshandel, P. Sabbagh, H. Safari, S. Safari, S. Safiri, A. Sahebkar, S. Salehi Zahabi, A. M. Samy, P. Santalucia, I. S. Santos, J. V. Santos, M. M. Santric Milicevic, B. Sartorius, A. R. Sawant, A. E. Schutte, S. G. Sepanlou, A. Shafieesabet, M. A. Shaikh, M. Shams-Beyranvand, A. Sheikh, K. N. Sheth, K. Shibuya, M. Shigematsu, M.-J. Shin, I. Shiue, S. Siabani, B. H. Sobaih, L. A. Sposato, I. Sutradhar, P. N. Sylaja, C. E. I. Szoeke, B. J. Te Ao, M.-H. Temsah, O. Temsah, A. G. Thrift, M. Tonelli, R. Topor-Madry, B. X. Tran, K. B. Tran, T. C. Truelsen, A. G. Tsadik, I. Ullah, O. A. Uthman, M. Vaduganathan, P. R. Valdez, T. J. Vasankari, R. Vasanthan, N. Venketasubramanian, K. Vosoughi, G. T. Vu, Y. Waheed, E. Weiderpass, K. G. Weldegwergs, R. Westerman, C. D. A. Wolfe, D. Z. Wondafrash, G. Xu, A. Yadollahpour, T. Yamada, H. Yatsuya, E. M. Yimer, N. Yonemoto, M. Yousefifard, C. Yu, Z. Zaidi, M. Zamani, A. Zarghi, Y. Zhang, S. Zodpey, V. L. Feigin, T. Vos and C. J. L. Murray, Lancet Neurol., 2019, 18, 439–458 CrossRef.
- P. B. Gorelick, Lancet Neurol., 2019, 18, 417–418 CrossRef.
- H. P. Adams, B. H. Bendixen, L. J. Kappelle, J. Biller, B. B. Love, D. L. Gordon and E. E. Marsh, Stroke, 1993, 24, 35–41 CrossRef PubMed.
- D. Kim, S.-H. Lee, B. Joon Kim, K.-H. Jung, K.-H. Yu, B.-C. Lee and J.-K. Roh, Eur. Heart J., 2013, 34, 2760–2767 CrossRef CAS PubMed.
- E. L. Harshfield, M. C. Sims, M. Traylor, W. H. Ouwehand and H. S. Markus, Brain, 2020, 143, 210–221 CrossRef PubMed.
- K. Wook Joo, K. Youngchai, Y. Mi Hwa, I. Sun Hye, P. Jung Hyun, L. JiSung, L. Juneyoung, H. Moon Ku and B. Hee Joon, Stroke, 2010, 41, 1200–1204 CrossRef PubMed.
- A. K. Saenger and R. H. Christenson, Clin. Chem., 2010, 56, 21–33 CrossRef CAS PubMed.
- A. Montaño, I. Staff, L. D. McCullough and G. Fortunato, Am. J. Emerg. Med., 2013, 31, 1707–1709 CrossRef.
- G. C. Jickling and F. R. Sharp, Neurotherapeutics, 2011, 8, 349–360 CrossRef CAS PubMed.
- D. Gregg and P. J. Goldschmidt-Clermont, Circulation, 2003, 108, e88–90 CrossRef CAS PubMed.
- N. Artrith, K. T. Butler, F.-X. Coudert, S. Han, O. Isayev, A. Jain and A. Walsh, Nat. Chem., 2021, 13, 505–508 CrossRef CAS PubMed.
- J. Zhang, C. Guo, W. S. Cai and X. G. Shao, Chemom. Intell. Lab. Syst., 2021, 210, 104244 CrossRef CAS.
- P. Villoutreix, Development, 2021, 148(1), dev188474 CrossRef CAS.
- A. L. Beam and I. S. Kohane, JAMA, 2018, 319, 1317–1318 CrossRef PubMed.
- J. Zhang, B. Y. Li, Y. Hu, L. X. Zhou, G. Z. Wang, G. Guo, Q. H. Zhang, S. C. Lei and A. H. Zhang, Anal. Chim. Acta, 2021, 1142, 169–178 CrossRef CAS.
- J. Zhang, C. Guo, X. Y. Cui, W. S. Cai and X. G. Shao, Anal. Chim. Acta, 2019, 1050, 25–31 CrossRef CAS PubMed.
- J. Zhang, X. Y. Cui, W. S. Cai and X. G. Shao, Sci. China: Chem., 2019, 62, 271–279 CrossRef CAS.
- J. Zhang, X. Y. Cui, W. S. Cai and X. G. Shao, J. Chemometr., 2018, 32, e2971 CrossRef.
- J. Zhang, L. B. Yang, Z. Q. Tian, W. J. Zhao, C. Q. Sun, L. J. Zhu, M. J. Huang, G. Guo and G. Y. Liang, ACS Med. Chem. Lett., 2022, 13, 99–104 CrossRef CAS PubMed.
- N. M. Murray, M. Unberath, G. D. Hager and F. K. Hui, J. Neurointerventional Surg., 2020, 12, 156–164 CrossRef PubMed.
- H. Kamal, V. Lopez and S. A. Sheth, Front. Neurol., 2018, 9, 945 CrossRef PubMed.
- Y. Xie, B. Jiang, E. Gong, Y. Li, G. Zhu, P. Michel, M. Wintermark and G. Zaharchuk, AJR, Am. J. Roentgenol., 2019, 212, 44–51 CrossRef PubMed.
- A. Sahu, P. M. Okin, R. B. Devereux, J. W. Weinsaft, I. Diaz, S. S. Omran, A. Gupta, B. B. Navi, C. Iadecola and H. Kamel, Stroke, 2019, 50, A121 Search PubMed.
- J. Heo, G. Yoon Jihoon, H. Park, D. Kim Young, S. Nam Hyo and H. Heo Ji, Stroke, 2019, 50, 1263–1265 CrossRef.
- A. Folch-Fortuny, F. Arteaga and A. Ferrer, Chemom. Intell. Lab. Syst., 2016, 154, 93–100 CrossRef CAS.
- H. Peng, F. Long and C. Ding, IEEE Trans. Pattern Anal. Mach. Intell., 2005, 27, 1226–1238 Search PubMed.
- C. Seiffert, T. M. Khoshgoftaar, J. Van Hulse and A. Napolitano, IEEE Trans. Syst. Man Cybern. Syst. Hum., 2010, 40, 185–197 Search PubMed.
- A. C. Olivieri, N. M. Faber, J. Ferré, R. Boqué, J. H. Kalivas and H. Mark, Pure Appl. Chem., 2006, 78, 633–661 CrossRef CAS.
- Q. S. Xu and Y. Z. Liang, Chemom. Intell. Lab. Syst., 2001, 56, 1–11 CrossRef CAS.
- K. Kuronuma, Y. Okumura, T. Morikawa, K. Yokoyama, N. Matsumoto, E. Tachibana, K. Oiwa, M. Matsumoto, T. Kojima, H. Haruta, K. Nomoto, K. Sonoda, K. Arima, R. Kogawa, F. Takahashi, T. Kotani, K. Ohkubo, S. Fukushima, S. Itou, K. Kondo, M. Chiku, Y. Ohno, M. Onikura and A. Hirayama, Int. Heart J., 2020, 61, 492–502 CrossRef CAS.
- P. Bettencourt, A. Azevedo, J. Pimenta, F. Friões, S. Ferreira and A. Ferreira, Circulation, 2004, 110, 2168–2174 CrossRef CAS PubMed.
- T. Kiris, E. Avci and A. Celik, Int. Urol. Nephrol., 2019, 51, 475–481 CrossRef.
- B. Zhang, C. Gao, Q. Hou, J. Yin, L. Xie, S. Pu, Y. Yi and Q. Gao, J. Neurol., 2012, 259, 1420–1425 CrossRef CAS.
- A. Yuri Gasparyan, L. Ayvazyan, D. P. Mikhailidis and G. D. Kitas, Curr. Pharm. Des., 2011, 17, 47–58 CrossRef.
- H. Markus, Medicine, 2016, 44, 515–520 CrossRef.
- T. Farkhondeh, R. Afshari, O. Mehrpour and S. Samarghandian, Biol. Trace Elem. Res., 2020, 196, 27–36 CrossRef CAS PubMed.
- B. Balint, V. K. Jepchumba, J. L. Gueant and R. M. Gueant-Rodriguez, Biochimie, 2020, 173, 100–106 CrossRef CAS PubMed.
- T. Q. de Aguiar Vallim, E. J. Tarling and P. A. Edwards, Cell Metab., 2013, 17, 657–669 CrossRef CAS.
- T. Umemura, T. Kawamura, S. Mashita, T. Kameyama and G. Sobue, Cerebrovasc. Dis. Extra, 2016, 6, 1–11 CrossRef PubMed.
- M. S. Babu, S. Kaul, S. Dadheech, K. Rajeshwar, A. Jyothy and A. Munshi, Nutrition, 2013, 29, 872–875 CrossRef CAS PubMed.
- L. Qin, Z. Yang, H. Gu, S. Lu, Q. Shi, Y. Xing, X. Li, R. Li, G. Ning and Q. Su, BMC Cardiovasc. Disord., 2014, 14, 26 CrossRef PubMed.
- B. Gryszczyńska, M. Budzyń, D. Formanowicz, M. Wanic-Kossowska, P. Formanowicz, W. Majewski, M. Iskra and M. P. Kasprzak, J. Clin. Med., 2020, 9, 1416 CrossRef PubMed.
- P. Novák, A. O. Jackson, G.-J. Zhao and K. Yin, Life Sci., 2020, 257, 118032 CrossRef PubMed.
- G. Walldius and I. Jungner, J. Intern. Med., 2006, 259, 493–519 CrossRef CAS PubMed.
- C. P. Derdeyn, Neuroimaging Clin. N. Am., 2007, 17, 303–311 CrossRef PubMed.
- E. Marulanda-Londono and S. Chaturvedi, Neurol. Clin. Pract., 2016, 6, 252–258 CrossRef PubMed.
- F. Medlin, M. Amiguet, A. Eskandari and P. Michel, Eur. J. Neurol., 2020, 27, 1680–1688 CrossRef CAS PubMed.
- H. Kim, K. Lee, H. A. Choi, S. Samuel, J. H. Park and K. W. Jo, J. Korean Neurosurg. Soc., 2017, 60, 620–626 CrossRef PubMed.
- L. M. Brewster, J. F. Clark and G. A. van Montfrans, J. Hypertens., 2000, 18, 1537–1544 CrossRef CAS PubMed.
- G. C. Jickling and F. R. Sharp, Stroke, 2015, 46, 915–920 CrossRef PubMed.
- E. Arch Allison, C. Weisman David, S. Coca, V. Nystrom Karin, R. Wira Charles and L. Schindler Joseph, Stroke, 2016, 47, 668–673 CrossRef CAS PubMed.
|
This journal is © The Royal Society of Chemistry 2022 |
Click here to see how this site uses Cookies. View our privacy policy here.