DOI:
10.1039/D2RA01187E
(Paper)
RSC Adv., 2022,
12, 12258-12271
Time-dependent multivariate and spectroscopic characterisation of oil residue in Niger Delta soil†
Received
22nd February 2022
, Accepted 14th April 2022
First published on 22nd April 2022
Abstract
In this paper, we present a detailed evaluation of changes in the oil residue in soil following a spill using weathering indices obtained from analytical instruments such as UV, IR, GC, and 1H NMR, and chemometrics based on the time of spill in the Niger Delta region of Nigeria. UV, IR and 1H NMR spectra of eight (8) oil residue samples were analyzed. The PCA of the UV and IR spectrometric index showed that the first two PCs accounted for 87 and 71% of the variance of the index, respectively. The detailed results suggested that the absorption ratios A225/256 and A248/278 from UV were good estimators for petroleum of different weathering profiles and the presence of different types of di- and poly-aromatics, nitrogen, sulphur, and oxygen (NSO) containing compounds. Similarly, sulphoxide, aromatic, and carbonyl index obtained from IR would be more valuable in evaluating changes in oil residue over time. An 84% PC obtained for NMR indicators described for weathered crude oil was the best at explaining structural changes compared to the region defined for fresh heavy crude oil. These models showed good predictive ability for the crude-oil residue composition and could be used to provide a rapid assessment of compositional differences in crude-oil residue following a spill.
1 Introduction
The realisation that crude oil contamination of the environment is extensive and significant has emerged largely as a result of increased awareness with improved evaluation methods. Statistics showed that there is a gradual decrease in the number of occurrence of oil spills, however, medium and large-scale spills persist globally.1 The oil-fed economic and industrial growth that has occurred in Nigeria has caused environmental damage.2 Studies have shown that the quantity of oil spilled over 50 years was put at 9–13 million barrels, an equivalent of 50 Exxon Valdez spills.3 Knowledge of the distribution of the major structural classes of hydrocarbons present in crude oil residue is therefore needed for effective implementation of remediation strategies for crude oil spill polluted sites. Once entering the environment, an oil spill is subject to a variety of weathering processes such as evaporation, dissolution, dispersion, flushing owing to wave energy, emulsification, photochemical oxidation, microbial biodegradation, adsorption to suspended matter, and deposition onto surfaces.4–7 Thus, characterizations of the individual molecular components of the degraded crude oil are quite challenging due to the complex composition of crude oils.
Researchers have used analytical and physical chemistry techniques to monitor the degradation and fate of crude oil in the environment. Techniques such as gas chromatography (GC), gas chromatography-mass spectrometry (GC-MS)8 high-performance liquid chromatography (HPLC), thin layer chromatography (TLC),9 and ultraviolet-visible spectroscopy (UV)10 have been employed in the analysis of spilled oil. Other studies have combined these analytical techniques with pattern recognition methods such as principal component analysis (PCA) and hierarchical cluster analysis (HCA) to classify weathered oil.11–13 This has driven studies of aged polluted systems to evaluate the compositional changes of the oil residue following a spill.4,14 As a spectroscopic tool, UV-visible measurement is useful for many pure chemicals. However, complex mixtures like crude oils with high absorption coefficients are also amenable to UV application.15 This technique is increasingly being employed for in-field applications for laboratory studies of crude oils and petroleum asphaltenes.16–19 Direct quantification of functional groups in asphaltenes in heavy crude oil and the effect of dispersants and additives on their blends have also been studied using UV spectroscopy.20–23 UV studies have shown that the aromatic moiety through π–π* and dipole interactions, is one of the dominant contributors to asphaltene self-association24 and have been used to match weathered oil with source of spill25 as well as determine compositional changes in persistent and photochemically transformed water-soluble constituents of industrial crude oil and natural seep oil in seawater.26 Absorption maxima and absorption ratio derived from UV measurements have been used27–30 to differentiate between SARA fractions, fresh oil, and degraded samples as well as determine the probable type of hydrocarbon in seawater. These ratios have been used successfully to differentiate the classes of aromatic (low molecular hydrocarbon with nearly identical aromatic values, mono-aromatic hydrocarbon, and di-and polyaromatic hydrocarbons) found in the samples. Fourier Transform Infrared spectroscopy (FTIR) presents another unique approach to determining the changes in composition and distribution of certain chemical functionalities or classes of compounds in crude oil.31,32 It requires minimum sample treatment and the acquisition of spectra takes a few minutes and offers acceptable repeatability in liquid samples.33,34 IR spectroscopy has been applied in agriculture,35 fuels and energy,36,37 and petroleum geochemistry.13,32,38–42 Weathering indices calculated from aliphatic and aromatic biomarkers derived from the oil residue were used to ascertain the sources of hydrocarbon contamination and the extent of degradation of the spilled oils.43 These biomarkers are highly reliable because of their specificity, hydrophobic nature, and long residence time in the environment.44,45 Gas chromatography (GC) or gas chromatography-mass spectroscopy (GC-MS) sometimes gives poor resolution due to severe overlaps of the numerous peaks.46 Unaltered constituents of crude oil cannot be characterized by direct GC analysis, hence the derivatization or other treatments are usually needed. The need for high accuracy, precision, quality assurance/quality control measures required in the use of chromatographic technique often makes the use of GC tasking. NMR spectroscopy has been proposed as a powerful and highly useful technique for the structural elucidation of various crude oil residues and their fractions following spillages.47 Although NMR spectroscopy is well known in organic chemistry, it has only been applied to the study of crude oil fractions in the last few decades.47–50 It is explicit in describing structural parameters of crude oil and its fractions.51–55 Multidimensional NMR experiments such as correlation spectroscopy (COSY), heteronuclear correlation (HETCOR), and heteronuclear single quantum coherence (HSQC) spectroscopy have yielded important information about 1H–1H and 1H–13C connectivity in petroleum molecules as well as the chemistry of crude oil with respect to functional groups present.55,56 As a result of the variations in environmental conditions and time, true composition of oil residue varies as it ages in soil. Therefore, there is a need to measure field samples as it is impossible to reproduce similar conditions in the laboratory. The Niger Delta region provides an excellent background for such study especially for clean-up and remediation work.57–59 Thus, this research aims to (a) determine the level of degradation using weathering index for oil residue obtained from UV, IR, NMR spectroscopic tools, and gas chromatography, and (b) develop a model that will give an instantaneous view of the structural composition and changes in crude oil residues following a spill by using principal component analysis.
2 Materials and methods
2.1 Study area and sample collection
Niger Delta region in Nigeria is the delta of the Niger River that sits directly on the Gulf of Guinea on the Atlantic Ocean. This study was carried out in Edo, Delta, and Rivers States of the Niger Delta region of Nigeria. Fig. 1 shows the map of the Niger Delta region and sampling points. The sampling was done in May 2015. The study involved eight (8) notable oil spilled sampling sites for the survey while fresh crude oil was used as the control. The choice of sampling was based on a site with a history and time of oil spill. Soil samples (S1– S6) were obtained from the oil spilled sites, while samples coded B1 and B2 were obtained by biostimulation; a simulated process involving spiking of unpolluted soil samples with a combination of fresh crude oil and cow dung acting as the natural fertilizer. The cow dung contained petroleum utilizing bacteria to improve the degradation of the oil residue overtime. The fresh crude oil (FC) used as the control sample was also subjected to the same procedure as described for the oil residue samples.
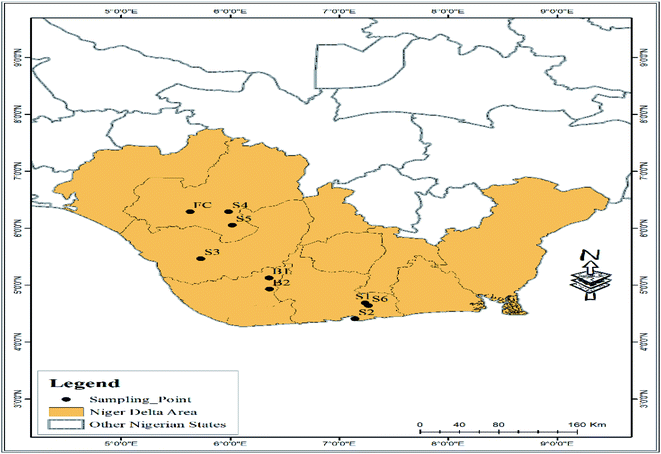 |
| Fig. 1 Map of the Niger Delta region of Nigeria showing sampling points. | |
2.2 Extraction and fractionation of oil residue
The extraction and fractionation of the oil residue from soil have been described elsewhere.60 The extracted oil and fractions obtained were used for subsequent gas chromatographic (GC), ultraviolet-visible spectroscopy (UV), infrared spectroscopy (IR), and nuclear magnetic resonance (NMR) analyses.
2.3 Ultraviolet analysis
The absorbance of the oil residue and fresh crude oil in hexane (20 mg L−1) was measured on a UV-2600 spectrophotometer (Shimadzu, Japan). Selected absorption maxima and absorption ratios that defined hydrocarbon content and type were used to determine the variation of oil residue composition with the age of spill. The selected absorption ratios used were A205/A215, A228/A256, A248/A267, and A248/A278.61,62 Standard solutions of 10 mg L−1 crude oil in hexane were prepared and successively analysed in duplicate on a UV-vis spectrophotometer in the range of 200–450 nm in order to assess the correlation between compositional changes and time of spills. The different absorbance signals (A) depicting naphthenic and aromatic compounds in the resulting sample spectra were plotted against the corresponding wavelengths (λ) of 230 nm and 260 nm, respectively.63 A linear regression curve of variations in absorbance at specific wavelength versus time was obtained; and the line equation and the coefficient of determination (R2) were evaluated.
2.4 Infrared spectroscopic analysis
Attenuated Total Reflectance Infra-red (ATR-IR) analyses were carried out for oil residue and fresh crude oil using a Bruker-Alpha FTIR Spectrophotometer (Bruker Optics GmbH Ettlinger, Germany). Data were recorded in the MIR spectral range of 400–4000 cm−1 at a spectral resolution of 2 cm−1. Twenty-four (24) scans were averaged for both the backgrounds and the sample spectra. All experiments were done at ambient conditions (T = 302 K). The functional and structural band areas were used to generate the IR spectrometric index based on the methods of Pieri et al.64 and Ganz and Kalkreuth.65 The average area in the defined region was used to calculate the spectrometric index, and the standard deviation of all measurements was found to be within the acceptable limit. The main areas used have been described by Permanyer et al.66 and are given in Table S1.†
2.5 Gas chromatographic-flame ionization detector (GC-FID) analysis
The saturate fraction of the oil residues was analysed for total petroleum hydrocarbon (TPH) using the method previously described by Udoetok and Osuji.67 Individual n-alkanes were identified based on the retention times of the standards of n-alkane n-C8 to n-C40 (Sigma Aldrich). The concentrations of each n-alkane in the samples were subsequently determined from the calibration curves of individual n-alkanes.
2.6 1H-NMR measurement
The 1H-spectra were obtained using a 5% (w/w) oil residue in CDCl3 (99.8%, Aldrich) using a 5 mm probe at a spinning rate of 10 Hz and a temperature of 301.15 K. The spectrum was recorded on a 500 MHz Bruker Avance NMR spectrometer while 30° pulses (Bruker zg30 pulse sequence) were used with a delay time of 2 s (sweep width 3900.25 Hz). Sixteen scans were averaged for each spectrum. The automated phase and baseline corrections were applied to get reproducible integral values. The spectra were integrated three times and the mean values were used in the calculations. To assess the repeatability of 1H chemical shift at 500 MHz, some samples were independently determined in triplicate and a standard deviation of 0.003 ppm was obtained. The defined regions in the NMR spectra obtained were then integrated as presented in Table 1 and were used to obtain the percentage areas of each region. Table 2 describes the assignments of all the regions integrated.
Table 1 1H NMR integral region and assignment
Parameter |
Nudelmann et al.68 |
Rios et al.69 |
Mohammad and Azeredo70 |
Poveda and Molina71 |
H1 |
0.5–1.0 |
0.5–1.0 |
0.5–1.0 |
0.1–1.0 |
H2 |
1.0–1.5 |
1.0–1.5 |
1.0–1.7 |
1.0–1.5 |
H3 |
1.5–2.0 |
1.5–2.0 |
1.7–1.9 |
1.5–2.0 |
H4 |
2.0–3.0 |
— |
|
2.0–4.5 |
H5 |
— |
— |
— |
4.5–6 |
H6 |
— |
— |
— |
6.0–7.2 |
H7 |
— |
— |
— |
7.2–9.2 |
H8 |
— |
— |
6.0–9.3 |
9.0–12.0 |
HA |
6.6–8.5 |
— |
— |
— |
HA1 |
— |
6.0–7.5 |
— |
— |
HA2 |
— |
7.5–9.0 |
— |
— |
HOH |
3.0–5.0 |
— |
— |
— |
HCOOH |
10.0–11.0 |
— |
— |
— |
Table 2 Description for assignmenta
Parameter |
Structural assignment |
Ref. 68–71. |
H1 |
Aliphatic hydrogens in methyl or methylene γ or further from an aromatic ring |
H2 |
Aliphatic hydrogens in methyl or methylene β or further from an aromatic ring |
H3 |
Alicyclic hydrogens in position β to two aromatic rings (naphthenic rings and methylenes) |
H4 |
Aliphatic hydrogens in methyl or methylene γ to an aromatic ring, which can be attached in a γ position to another or the same ring |
H5 |
Olefinic hydrogen |
H6 |
H∝ to aromatic rings CH2 and CH |
H7 |
Hydrogens in polyaromatic rings |
H8 |
Aldehydic and carboxylic hydrogen |
HA |
Aromatic hydrogen linked to monoaromatic rings |
HA1 |
Some tri- and tetra-aromatic |
HA2 |
Aromatic hydrogen linked to aromatic carbons in di- or polyaromatic rings |
HOH |
Protons of phenolic and n-alcoholic compounds |
HCOOH |
Aldehydic and carboxylic hydrogen |
2.7 Principal component analysis
Owing to the variations in the chemical compositions of the oil residue over time, the predefined data set consisting of variables obtained from UV absorbance ratio, infrared spectrometric index, GC weathering index, and NMR region areas integrated for the samples were utilized for the PCA analysis. Principal component analysis (PCA) is a combination of variables describing the major relationship in a set of data. It helps to give a projection of the most important information contained in a set of data that do not appear ordinarily. Data from the study was analyzed using OriginPro2019b Statistics software and visualized using SIMCA 17.0 (Umetrics). Principal component analysis (PCA) was computed to identify significant principal components in the data as well as possible loadings and similarities between the oil residue and the time of the spill. The PCA was carried out by the varimax normalized rotation method. A scree plot and Kaiser criterion with an eigenvalue greater than 1 was performed to determine the number of important components present in the data, to provide a visual interrelationship, detect and interpret sample patterns, similarities, and disparities.72,73
3 Results and discussions
3.1 Spectra features and absorbance ratio determined from UV-vis spectroscopy
Fig. 2 shows the UV-vis absorption spectra of the oil residue in hexane and the distinguished absorption maxima bands at various wavelengths. All samples have a basic feature with shoulders between 228 and 230 nm (λ1) as well as between 256 and 274 nm (λ2). This would suggest that a complex mixture of compounds such as saturates, aromatics, resins, and asphaltene were responsible for these absorptions.74 Since it is difficult to assign absorption maxima to a single compound in an oil mixture, we, therefore, took advantage of the similarity in the chemical nature of fractions. For example, an absorption band owing to the differences in the aromatic composition of the sample would arise as a result of the ability of substituents present in the aromatic moiety to go into electronic interactions such as conjugation or hyperconjugation (in the case of alkyl substituent) with the π-bond system of the ring leading to a shift to a longer or shorter wavelength. Also, degradations often lead to the formation of auxochromes or groups (polar/resin in nature) in the molecules that create an additional 2 to 5 nm to a wavelength of major absorption bands.75
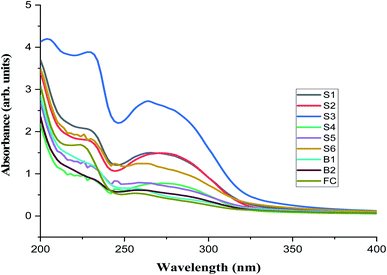 |
| Fig. 2 The UV-visible spectrum of oil residues from samples S1–B2 and FC. | |
On a sample basis, younger residues B1, B2, S5, and S6 (Table S1†) show absorption maxima between 226–230 nm and 254–262 nm. This suggests the presence of unsubstituted polyaromatic compounds such as pyrene (245 nm), anthracene (255 nm), and phenanthrene (255 nm) as the major constituents.76 Older residues such as samples S1, S2, and S3 have absorption maxima at 278, 274, and 264 nm, respectively compared to fresh crude oil with absorption maxima at 256 nm. It could be that these samples contained alkylated polyaromatic compounds with contributions from three and four aromatic systems that often give rise to absorption at a longer wavelength.77 In this study, four absorption ratios (Table S2†) calculated from the UV visible spectra were used to evaluate the compositional changes in time as a result of weathering processes. The absorption ratio A205/215 was between 1.213 and 1.525; older samples (S1, S2, and S3) had relatively constant values while the values for younger residue (<5 years) were slightly higher. These may be attributed to the presence of soluble low molecular weight hydrocarbon and aromatic compounds such as benzene, xylene, and toluene.30 The higher values of A205/215 obtained for fresh crude oil supported this evidence. The values calculated for A228/256 ratio were higher than A205/215.
The ratio A228/256 has been described as a good estimator for petroleum input and may be constant for oil from the same source and different for oil of other origin or weathering profile.78 In our case, this ratio increased with the time of the spill and may be as a result of an increase in long-chain carbon or branched chain hydrocarbon attached to aromatic moieties. The third and fourth ratios defined as A248/267 and A248/278 supported by the R2 value of 0.9117 in Fig. 3b were indicators of the presence of different types of di- and polyaromatics62 and compounds containing nitrogen, sulphur, and oxygen (NSO) atoms that could cause the bathochromic shift. Fig. 3a and b show the linear relationships of absorbance of oil residue with the time of spill at 230 nm (benzenic compounds) and 262 nm (naphthenic compounds), respectively. An optimum concentration of 20 mg L−1 of the oil residue was used for the absorbance measurement because above this concentration, the oil residues began to aggregate and the maximum absorbance was disrupted as a result of noise74 and low concentrations below 10 mg L−1 could not be used because the technique could not detect species below this concentration.79 The absorbance values of samples S2, S3, S4, S5, B1, and B2 gave fairly acceptable and positive regression values (R2) of 0.8463 and 0.9117 at 230 nm and 262 nm, respectively. In assessing the validity of the linear regression obtained, the absorbance value of the fresh crude oil gave a time scale of less than one year at 230 nm. This unexpected value gives credence to the linear relationship between the absorbance at the selected wavelength and the time of spill. The absorbance values of samples S1 and S6 (omitted in the plot) which were higher than expected might have been strongly influenced by their compositions that caused the overlapping of the absorbances of the different constituents in them.
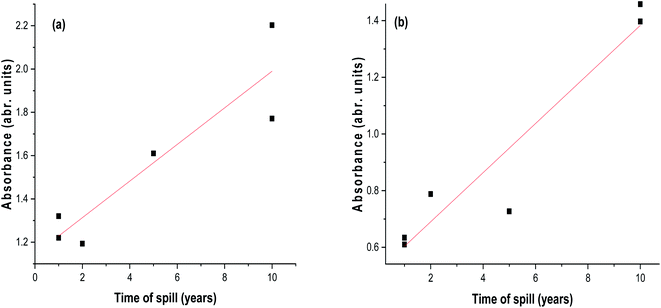 |
| Fig. 3 A plot of the absorbance of oil residue samples in hexane as a function of time of spill at (a) 230 nm. (b) 262 nm. | |
3.2 Spectra features and spectrometric index determined from infra-red spectroscopy
The use of ATR to simplify and evaluate mid-infrared spectroscopy (MIR) is well documented.80 The ATR is noted for accuracy because, in MIR, the fundamental bands are specific, sharp, and sensitive to include the absorption band of aliphatic C–H bands and additional bands originating from groups containing oxygen, sulphur, and nitrogen. Table S3† shows the assignment of the bands observed in a typical crude oil IR assay while the results of the IR spectrometric index calculated are shown in Table S4.†
Differences in the structural composition were not obvious by mere visual inspection as observed in the IR spectra (Fig. 4a) of whole S1-FC oil residue samples. In order to illustrate the differences in the fractions obtained, a representative spectrum obtained from saturates, aromatics, and polar fractions of sample S1 is shown in Fig. 4b. Appearance and absence of bands at the region that defines each fraction showed the presence of functional groups in the oil residues. Generally, saturates show characteristic bands at 2962 to 2872 cm−1 as a result of the C–H stretching vibration from CH3 groups while bending vibrations of CH3 appear between 1475 and 1375 cm−1.81 The presence of straight-chain alkane is also confirmed by the band at 721 cm−1. Evidence of the aromaticity of the sample is supported by the presence of bands at 900 to 675 cm−1 and 1605 cm−1. A representative spectrum (Fig. 4c) of the resin fraction of sample B1 shows broad and strong bands between 1330 and 1100 cm−1 assigned to the S
O functional group82 and 1030 cm−1 which is typical of the aromatic ethers and sulphoxide groups.32 The resin fraction of sample B1 that contains phenols and organic acids shows bands for O–H and C
O at 3100–3600 cm−1 and 1710–1680 cm−1, respectively. The application of the spectrometric index obtained from the IR region to PCA, yielded useful information on the pattern of distribution of NSO species in samples with the time of spill.
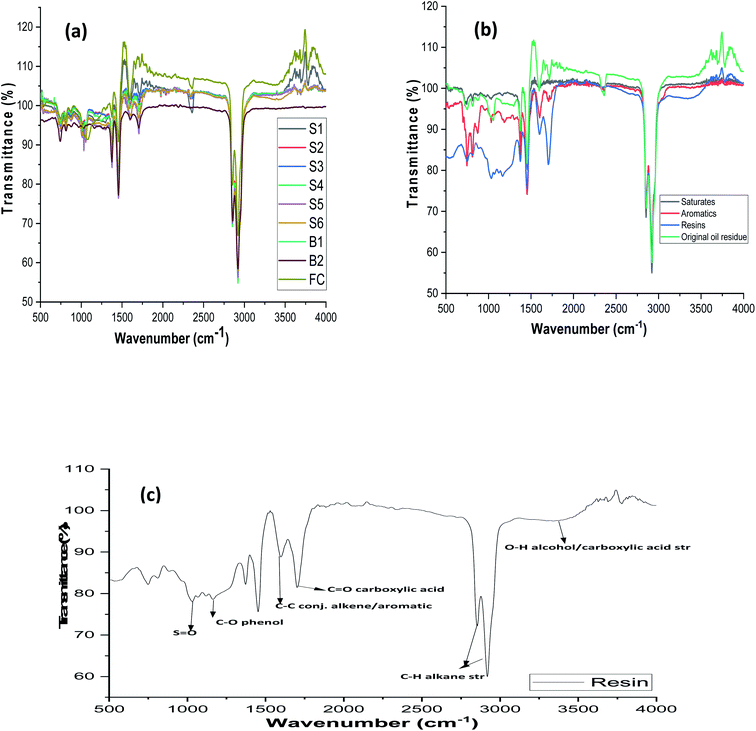 |
| Fig. 4 (a) Overlaid spectra of oil residue (S1-FC) samples (b) spectra of saturates, aromatics, and resin fractions of sample S1. (c) Enhanced infrared spectrum of polar/resin fraction of sample B1. | |
3.3 Gas chromatography (n-alkane distribution)
Normal-alkanes give useful information about the extent of weathering or freshness of crude oil following a spill. Samples with different spill histories can be compared by using the distribution of the n-alkanes biomarkers (pristane and phytane) and the total concentrations of the individual alkane.83 Table S5† shows the GC weathering indices of the saturate fractions (F1) of the oil residue obtained from different locations. Evaporative losses removed the low molecular weight alkanes for all the samples and then biodegradation and photo-oxidation started to affect the nature and distribution of the classes of biomarkers, complex alkanes, and naphthenic compounds present in the oil residues.83 Compared to the oil residues, the fresh crude oil is characterized by alkanes from C11–C40 (S1) while there are no n-alkanes below C14 in samples S1–B2. Pristane (Pr) and phytane (Ph) are ubiquitous in crude oil and are resistant to weathering84 and have been used as indicators of petroleum contamination.85 Pristine/phytane (Pr/Ph) ratio ranged from 0.74–1.56, indicating petroleum origin source.86 According to Didyk et al.,87 a high concentration of phytane could also be explained by low microbial activities in a reducing condition as is the case of older residues with Pr/Py values less than 1 except for S1F1 and values greater than 1, for younger residue. The values of Pr/n-C17 and Ph/n-C18 ranged from 2.7–6.6 and 2.46–6.76, respectively. This result is in agreement with the report by Faboya et al.88 and is an indication of the loss of n-C17 and n-C18 relative to pristane and phytane. The carbon preference index (CPI) values calculated from the n-alkane distribution in the present study ranged from 1.29–1.42. CPI values of n-alkanes close to 1 are considered to emanate from petroleum sources.89 There was no observable or drastic change in the ratio (Pr + n-C17)/(Ph + n-C18) for the oil residue and as such may not be useful in spotting the obvious differences in the structural compositions of the oil residue.
3.4 Principal component analysis of UV, IR, and GC index
Weathering Indices obtained from UV (Table S2†), IR (Table S4†), and GC (Table S5†) measurements relevant to the elucidation of structural and composition changes in oil residue following a spill were used in the PCA analysis. Table 3 shows the first two PCs obtained from the spectroscopic and GC weathering index. The selection of two PC scores was appropriate considering the percent variance in each of the PCs. For the absorbance ratio, PC 1 and PC 2 accounted for 62 and 25% of the total variance, respectively. Its PC 1 was influenced by positive values of A248/A278. (62%), A248/A267 (59%) and A228/A256 (45%) in that order, and represented the younger residue and biostimulated samples. PC 2 has a high correlation (87%) with A205/A215 suggesting a high concentration of aliphatic compounds.68 This shows that the oil residue and the fresh crude oil are characterized by the saturate fraction and gives reliable information on the extent of weathering, irrespective of the degradation process involved. The pattern in the biplot (Fig. 5a) clearly shows that oil residues S1, S2, and S3 are very similar in composition and are different from the younger residues such as S4, S6, and S5. These results agree with our hypothesis since they cluster together in the PCA biplot. Similarly, Fig. 5b indicates a good evolution of the sample with time in an anti-clockwise manner.
Table 3 Component factor and total variance explained from UV, IR and GCa
|
Absorbance ratio |
Spectrometric index |
GC weathering index |
PC1 |
PC2 |
|
PC1 |
PC2 |
|
PC1 |
PC2 |
AI = aromatic index, Alip I = aliphatic index, BI = branched index, LCI = long chain index CI = carbonyl index, SI = sulfoxide index, odd/even = (Pr + n-C17)/(Ph + n-C18). |
Age |
−0.47 |
0.37 |
Age |
−0.23 |
0.577 |
Pr/Ph |
−0.363 |
0.53 |
A205/A215 |
0.22 |
0.87 |
AI |
−0.52 |
−0.29 |
Pr/n-C17 |
0.340 |
0.77 |
A228/A256 |
0.45 |
−0.472 |
Alip I |
0.50 |
0.09 |
Ph/n-C18 |
0.748 |
−0.13 |
A248/A267 |
0.59 |
0.07 |
BI |
0.30 |
0.51 |
CPI |
−0.729 |
−0.31 |
A248/A278 |
0.62 |
−0.04 |
LCI |
0.37 |
0.11 |
Odd/even |
−0.383 |
0.31 |
|
|
CI |
0.26 |
−0.64 |
|
|
|
|
|
SI |
−0.67 |
0.30 |
|
|
|
Eigenvalue |
2.487 |
1.028 |
Eigenvalue |
3.463 |
1.518 |
Eigenvalue |
4.293 |
0.86 |
% |
62.18 |
25.71 |
% |
49.48 |
21.70 |
% |
71.55 |
14.35 |
% cumm |
62.18 |
87.89 |
% cumm |
49.48 |
71.18 |
% cumm |
71.55 |
85.91 |
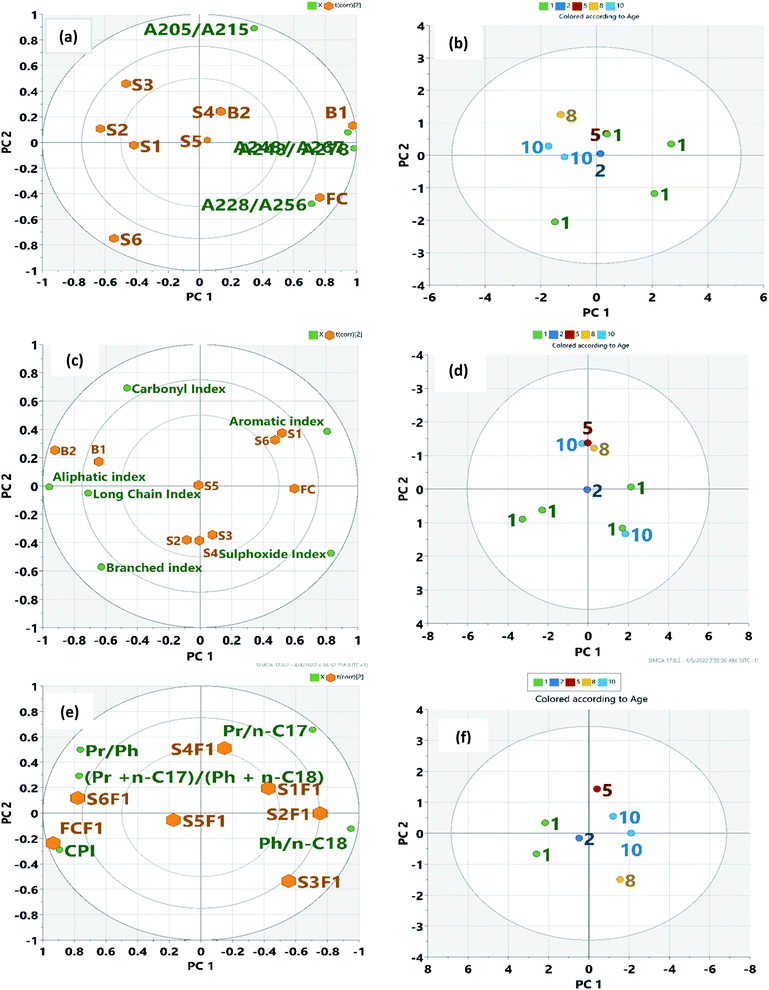 |
| Fig. 5 Biplot showing weathering index and samples: (a) absorption ratio and samples (c) spectrometric index and samples (e) GC weathering index and saturates of the samples. Green dots represent variables, corresponding to the points of loading plot. Brown dots represent the sample points. Also, Fig. 5(b), (d), and (f) show the score plot of age of spill. | |
Other studies have reported the property of crude oil either by using the SARA fractions or the whole crude.41,80 The relationship between the IR spectrometric index and the time of spill was made obvious by the PCA biplot. From our results, biostimulated samples B1 and B2 and older oil residues (S1, S2, and S3) were more of carboxylic and aliphatic acids. Samples B1 and B2 had lower ∑A values compared to other non-assisted oil residues (S1–S6). This decrease is consistent with the loss of more degradable hydrocarbon as microorganisms prefer small molecules.68 In the PCA determination, no pre-classification of the oil residue was performed. The first and second PCs represented 49.5 and 22% of the total variance, respectively. The Euclidean distance between the samples in the plot represented real differences in the IR structural index. The first PC was largely influenced by aromatic index (52%), aliphatic index (50%), sulphoxide index (46%), and long-chain index (37%) in that order. The second PC was influenced by the branched index (51%) and carbonyl index (44%). Fig. 5c also shows that the most positive values were represented by older oil residues (S2, S3, and S4) with the sulphoxide content while B1 and B2 had a good correlation with carbonyl index suggesting biodegradation. The younger residues correlated with aromatic index for sample S6 suggesting the transformation of aromatic compounds. However, the unlikely correlation of sample S1 (10 years) may suggest an increase in polyaromatic hydrocarbon. This distribution into groups based on the time of spill (Fig. 5d) and a 71% obtained for the total variance suggests that IR spectrometric index (carbonyl, sulphoxide, and aromatic index) would be a better indicator to account for structural changes in crude oil following a spill. It is usually assumed that the major transformation occurs in the first year of the environmental exposure but the biplot shows that recent spills may have similar characteristics as the aged oil residue. PCA analysis of the GC weathering index gave an 85% total variance explained by the first two PCs (Table 3). The first PC (71%) represented the younger residues and fresh crude oil. The biplot (Fig. 5a) shows how the parameters are influenced by the samples with high Pr/Ph, low Pr/C17, and Phy/C18) suggesting high concentration of aliphatic compound.68 The second PC (14%) was associated with older oil residues influenced by CPI and (Pr + n-C17)/(Ph + n-C18). This suggests the transformation of aliphatic compounds into a polar compound and a possible increase in the aromatic component. Fig. 6f shows the distribution of the time of spill. Transformation occurs in the first few years of environmental exposure.68
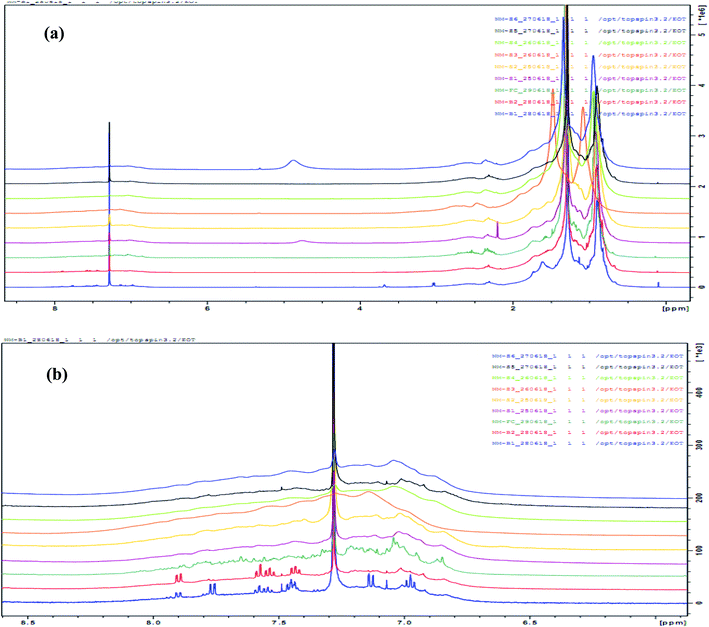 |
| Fig. 6 Overlaid NMR spectrum of samples (a) full-spectrum (b) aromatic region (6.5–8.00 ppm). | |
3.5 Nuclear magnetic resonance
Marshall and Rodgers90 have described crude oil as the most complex compositional organic mixture. In this work, we exploited the limitation of NMR as a tool for the structural elucidation of a single-component system and applied it to the determination of the composition of a multi-component system such as oil residue. As expected, the NMR spectrum showed an overlapping profile (Fig. 6a and b) making it difficult to differentiate between samples. Fig. 7 shows a representative integrated NMR spectrum (sample S1) using the areas described by Poveda and Molina.71
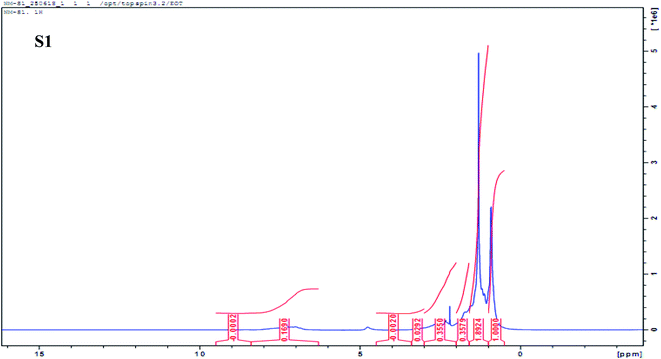 |
| Fig. 7 Representative 1H NMR spectrum (500 MHz) of sample S1 in CDCl3 showing the integrated region based on Poveda and Molina (2012) NMR region. | |
3.6 Principal component analysis of NMR index
PCA was conducted on all of the four NMR predefined regions with the anticipation that the oil residues would show compositional differences. NMR region described by Nudelmann et al.68 and Rios et al.69 was used for weathered crude oil while the region described by Poveda and Molina, (2012)71 and Mohammad and Azeredo, (2014)70 for heavy crude oil was used for the PCA. The PCA results in Table 4 indicate that the first two PCs from Nudelmann et al. (2008)68 and Rios et al. (2014)69 explained 65.75% and 84.18% of the total variance, respectively. Similarly, the first two PCs of the data from Poveda and Molina (2012)71 and Mohammad and Azeredo (2014)70 NMR region explained 64.66% and 61.75% of the total variance, respectively. This clearly shows that NMR indicators described for weathered crude could best explain compositional differences than the region defined for fresh heavy crude oil. The first PC for Nudelmann et al. (2008)68 was largely influenced by H1, H3, H4, HOH, and HCOOH while the second PC was influenced by H2. In the Rios et al. (2014)69 NMR area, PC 1 was influenced by positive H2 and A2 and a negative H3 and HA1 while its PC 2 was influenced by positive H1 and HA2, and negatively by H2 loading. PC 1 from Poveda and Molina (2012)71 NMR region was influenced by H2 and H4 while PC 2 was influenced by positive loading of H6 and a negative loading of H1 and H3. Finally, in the NMR region described by Mohammad and Azeredo (2014),70 PC1 was influenced by negative loading of H1 and H3 and positive for H7 and H8. The PC 2 was influenced by a positive and negative loading of H5 and H2, respectively. Samples exhibiting similar characteristics will become closer to each other in the biplot as given in the figures. Fig. 8a shows the PCA biplot of the sample distribution with NMR parameters described by Nudelmann et al.68 In this case, there was a clear pattern recognition between the oil residue and the NMR structural features. Older residues (S1, S2, S3, and possibly S4) were characterized by H2, H4, and HCOOH. Apart from the HCOOH, there are aliphatic hydrogens in methyl or methylene in β or γ position to an aromatic ring and are fairly resistant to degradation.91 Free fatty acids with an alkane chain lengths of C14–C26 range have been reported in petroleum sand samples and the typical acids found were alkanoic, mono- and di-unsaturated C18-alkenoic acids.92 Acid components present in the polar fractions could be carboxylic acids for species with two or more oxygen atoms, phenols, and also NSO species, such as pyrroles, indoles, and thiols,93 as well as esters.94 The formation of carboxylic acids and hydroxyl functionality is also as a result of microbial degradation of petroleum hydrocarbons following several oxidative processes.95,96 Samples B1 and B2 hold interesting features in that they are characterized mainly by H1 and H3 (Fig. 8a). This indicates that the already identified microbial consortium in the cow dung such as Acinetobacter spp., Bacillus spp., Pseudomonas spp., Alcaligenes spp., and Serratia spp.97 that are heterotrophic and petroleum-utilizing in nature might have degraded the aromatics (benzenoid) and phenolic component into nutrient, biomass, and CO2 via biodegradation and through their intermediates.98,99 The gas chromatography-mass spectrometry and IR analyses of the polar fraction of the oil residues show that samples S1, S2, and S3, B1, and B2 contained carboxylic acids and alcohols.100 Younger oil residues (S5 and S6) are characterized by HA, H2, and H4. These samples may contain long side-chain n-alkyl aromatic with side carbon atoms ranging from C7–C27.49 Fig. 8b shows the time evolution of the oil residues with NMR parameters. The older residues (>5 years) clustered and younger residues (<5 years) evolved in a clockwise direction, suggesting that time evolution and environmental conditions could affect degradation in a predictable pattern. Reports by Zhou et al.101 shows that data from both chromatographic and NMR parameters agree well with the level of biodegradation of crude oil sample from different sources. Fig. 8c shows the biplot from Rios et al.69 NMR parameters. It appeared that older residues (S1, S2, and S3), as well as residues that might have undergone enhanced degradation (B1 and B2), were characterized by HA1 and H3. It is clear that samples S6 and FC described by H2 only did not show any proximity to samples and so might not be a structural feature to consider when evaluating older residues. Fig. 8d shows the time evolution of the sample with NMR parameters from Rios et al.69 In this spatial distribution of time, the degraded oil residues with a time of spill greater than 5 years and biostimulated samples (B1 and B2) were grouped. The maximum variance obtained from this biplot might probably be as a result of fewer NMR parameter terms used. Biplots (Fig. 8e) obtained from PCA analysis of NMR parameters defined by Mohammed and Azeredo70 showed that samples S1 and S2 were characterized by H2 and H8 while samples S4 and S5 were characterized by H4, H5, H6, and H7. Also, B1, B2, and FC were characterized by H1. The biplot obtained from PCA analysis using Poveda and Molina (2012)71 NMR parameters (Fig. 8g) did not show any clear pattern. This was the same for the time evolution (Fig. 8h) and so was not discussed further. It has been suggested that the environmental evolution of the oil spill samples when reported may not reflect the true nature of samples in the field, but would give an instantaneous view on the level of weathering in the area under study.6
Table 4 Component factor and total variance explained from NMR region
Parameter |
Nudelmann et al.68 |
Rios et al.69 |
Poveda and Molina71 |
Mohammad and Azeredo70 |
PC1 |
PC 2 |
PC 1 |
PC 2 |
PC 1 |
PC 2 |
PC 1 |
PC 2 |
H1 |
0.56 |
0.05 |
0.38 |
0.52 |
0.06 |
−0.54 |
−0.44 |
0.28 |
H2 |
0.32 |
0.44 |
0.76 |
−0.67 |
0.42 |
0.28 |
0.29 |
−0.57 |
H3 |
0.48 |
— |
−0.56 |
0.15 |
0.28 |
−0.40 |
−0.44 |
0.22 |
H4 |
0.45 |
— |
— |
— |
0.42 |
0.04 |
0.22 |
0.27 |
H5 |
— |
— |
— |
— |
0.30 |
0.18 |
0.20 |
0.48 |
H6 |
— |
— |
— |
— |
0.39 |
0.45 |
0.32 |
0.27 |
H7 |
— |
— |
— |
— |
00.25 |
0.30 |
0.40 |
0.38 |
H8 |
— |
— |
— |
— |
−0.27 |
0.33 |
0.40 |
−0.04 |
HA |
0.28 |
— |
— |
— |
— |
— |
— |
— |
HA1 |
— |
— |
−0.47 |
0.28 |
— |
— |
— |
— |
HA2 |
— |
— |
0.48 |
0.40 |
— |
— |
— |
— |
HOH |
0.60 |
— |
— |
— |
— |
— |
— |
— |
HCOOH |
0.51 |
— |
— |
— |
— |
— |
— |
— |
Eigenvalue |
3.08 |
1.51 |
2.90 |
1.30 |
2.72 |
1.12 |
2.99 |
1.94 |
% |
44.0 |
21.0 |
58.06 |
26.11 |
40.42 |
24.24 |
37.48 |
24.27 |
Commulative |
44.0 |
65.0 |
58.06 |
84.18 |
40.42 |
64.66 |
37.48 |
61.75 |
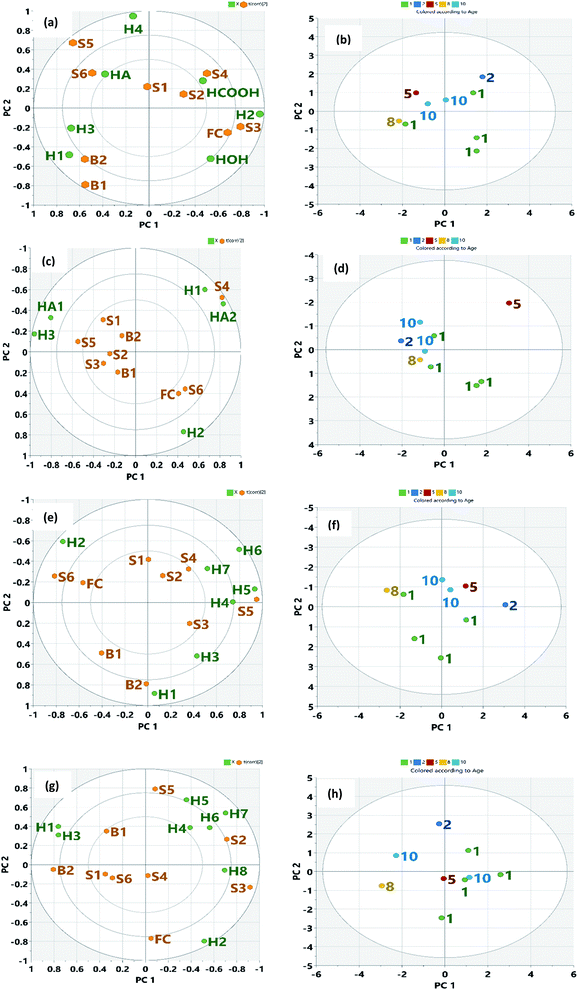 |
| Fig. 8 Biplot for the first two PCs for NMR parameters with (a) NMR parameters with samples using Nudelmann et al.68 (2008) (c) NMR parameters with samples using Rios et al.69 (2014) (e) NMR parameters with samples using Mohammed and Azeredo70 (2012) (f) NMR parameters with samples using Poveda and Molina71 (2012). Green dots represent variables, corresponding to the points of loading plot. Brown dots represent the sample points. Fig. 8(b), (d), (f) and (h) show the score plot of age of spill. | |
4 Conclusions
This work has shown that compositional changes in crude oil following a spill at different times can be modelled using GC weathering index, UV absorption ratios, IR spectrometric indices, NMR spectroscopy and Chemometrics to give a good prediction on the functional groups in each of the oil residue irrespective of the environmental exposure or conditions. Pr/Ph ratio and CPI values close to unity from the GC index showed that the oil residues were all derived from petroleum sources. Results also suggested that the absorption ratios A225/256 and A248/278 from UV were good estimators for petroleum of different weathering profiles while sulphoxide, aromatic and carbonyl index obtained from IR would be more valuable in evaluating changes in oil residues over time. For both the UV and IR spectroscopic measurements, two PCs were captured that explained 87% and 71% of the total variance, respectively. NMR indicated that β and γ alkyl-substituted aromatic, aliphatic, and –OH and COOH type compounds provided the structural difference in oil residues with the time of the spill. The Niger Delta region provided an excellent point for this study as a result of its notable and continual oil spill. The NMR region described for weathered oil residue could be applied to obtain structural differences. PCA indicated that the time evolution of the sample followed a predictable pattern and might be useful when the age of a spill is known.
Author contributions
Nnamdi Menkiti: methodology, formal analysis, investigation & writing original draft. Chukwuemeka Isanbor: conceptualization, supervision, review & editing. Olusegun Ayejuyo: supervision, review & editing. Doamekpor Louis: supervision, NMR analysis and interpretation. Twum Emmanuel: supervision, NMR analysis and interpretation.
Conflicts of interest
There are no conflicts to declare.
Acknowledgements
We thank TWAS/UNESCO for the research grant for equipment and chemicals to C. I research group.
References
- International Tanker Owners Pollution Federation Limited (ITOPF), Oil tanker spill statistics, 2021, https://www.itopf.org/knowlegde-resources/data-statistics/statistics, accessed online 20th, January, 2022 Search PubMed.
- G. O. Anoliefo, O. Isikhuemhen and E. Ohimain, Nigeria. J. Soils Sediments., 2006, 6, 30–36 CrossRef.
- Federal Ministry of Environment Abuja, Nigerian Conservation Foundation Lagos, WWF UK, and CEESP-IUCN Commission on Environmental, Economic, and Social Policy, May 31, (2006), Niger Delta Resource Damage Assessment and Restoration Project.
- U. Yim, H. Ha, S. Y. An, J. G. Won, J. H. Han, G. M. Hong, S. H. Kim, J. H. Jung and W. J. Shim, J. Hazard. Mater., 2011, 197, 60–69 CrossRef CAS PubMed.
- R. M. Garrett, I. J. Pickering, C. E. Haith and R. C. Prince, Environ. Sci. Technol., 1998, 32, 3719–3723 CrossRef CAS.
- M. D'Auria, L. Emanuele, R. Racioppi and V. Velluzzi, J. Hazard. Mater., 2009, 164, 32–38 CrossRef PubMed.
- B. C. Linares, O. L. Fuentealba, S. M. Mudge and L. R. Sepulveda, Fuel, 2013, 103, 876–883 CrossRef.
- Z. Wang, M. Fingas and D. S. Page, J. Chromatogr. A, 1999, 843, 369–411 CrossRef CAS.
- I. R. Kaplan and Y. Galperon, in Ground Water and Soil Contamination: Technical Preparation and Litigation Management, ed. T. J. Bois II and B. J. Luther, Wiley, New York. 1996 Search PubMed.
- Z. D. Wang and M. F. Fingas, Mar. Pollut. Bull., 2003, 47, 423–452 CrossRef CAS PubMed.
- F. R. Varela, S. D. Rodríguez, G. M. P Carracedo, J. M. Andrade, E. Fernández, S. Muniategui and D. Prada, Talanta, 2005, 68, 116–125 CrossRef PubMed.
- A. M. Fonseca, J. L. Biscaya, J. Aires-de Sousa and A. M. Lobo, Anal. Chim. Acta, 2006, 556, 374–382 CrossRef CAS.
- F. R. Varela, G. M. P. Carracedo, F. P. Rivera, J. M. Andrade, S. Muniategui and D. Prada, Talanta, 2006, 69, 409–417 CrossRef PubMed.
- J. Wang, X. Zhang and G. Li, Chemosphere, 2011, 85, 609–615 CrossRef CAS PubMed.
- A. Barbaro, G. Cecchi and P. Mazzinghi, Appl. Opt., 1991, 30, 852–857 CrossRef CAS PubMed.
- O. C. Mullins, Optical Interrogation of Aromatic Moieties in Crude Oils and Asphaltenes in Structures and Dynamics of Asphaltenes, ed. O. C. Mullins and E. Y. Sheu, Springer, New York, 1999, ch. II, pp.21–78 Search PubMed.
- I. N. Evdokimov, N. Y. Eliseev and B. R. Akhmetov, J. Pet. Sci. Eng., 2003, 37, 145–152 CrossRef CAS.
- I. N. Evdokimov, N. Y. Eliseev and B. R. Akhmetov, J. Pet. Sci. Eng., 2004, 37, 135–143 CrossRef.
- B. Agyei-Tuffour, S. Gbogbo, D. Dodoo-Arhin, L. N. W. Damoah, J. K. Efavi, A. Yaya and E. Nyankson, Cogent Engineering, 2020, 7, 174494 CrossRef.
- H. Groenzin and O. C. Mullins, Energy Fuels, 2000, 14, 677–684 CrossRef CAS.
- L. Gmachowski and M. Paczuski, Colloids Surf., A, 2015, 484, 402–407 CrossRef CAS.
- V. Jozsa and A. Kun-Balog, Fuel Process. Technol., 2015, 139, 61–66 CrossRef CAS.
- E. E. Banda, S. I. Padrón, N. V. Gallardo, J. L. Rivera, U. Páramo, N. P. Díaz and A. M. Mendoza, Pet. Sci. Technol., 2016, 34, 732–738 CrossRef.
- N. E. Rezaee, F. Heidarizadeh, S. Sajjadifar and Z. Abbasi, J. Pet. Eng., 2013, 1–5 Search PubMed.
- Z. Zhou, L. Guo, A. M. Shiller, S. E. Lohrenz, V. L. Asper and C. L. Osburn, Mar. Chem., 2013, 148, 10–21 CrossRef CAS.
- K. Snyder, N. Mladenov, W. Richardot, N. Dodder, A. Nour, C. Campbell and H. Eunha, Mar. Pollut. Bull., 2021, 165, 112049 CrossRef CAS PubMed.
- S. Yoon, S. D. Bhatt, W. Lee, H. Y. Lee, S. Y. Jeong, J. Baeg and C. W. Lee, Korean J. Chem. Eng., 2009, 26, 64–71 CrossRef CAS.
- K. Baginska and I. Gawel, Fuel Process. Technol., 2004, 85, 1453–1462 CrossRef CAS.
- H. F. Henning, Original Research Article, 1979, 234–237 Search PubMed.
- E. Omayma, A. Ahmed, S. Mahmoud and M. M. Abd El Rahman, Egypt. Int J Environ. Res., 2015, 4, 70–86 Search PubMed.
- N. Aske, H. Kallevik and J. Sjoblom, Energy Fuels, 2001, 15, 1304–1312 CrossRef CAS.
- A. Hannisdal, P. V. Hemmingsen and J. Sjoblom, Ind. Eng. Chem. Res., 2005, 44, 1349–1357 CrossRef CAS.
- H. Chung, M. Ku and J. S. Lee, Appl. Spectrosc., 2000, 54, 239–245 CrossRef CAS.
- J. A. Orrego-Ruiz, A. Guzmán, D. Molina and E. Mejía-Ospino, Energy Fuels, 2011, 25, 3678–3686 CrossRef CAS.
- K. M. Hosamani and S. S. Ganjihal, Ind. Crops Prod., 2003, 18, 111–116 CrossRef CAS.
- R. M. Roehner and F. V. Hanson, Energy Fuels, 2001, 15, 756–763 CrossRef CAS.
- G. Dutra, C. Martelli, M. José Da Silva, R. L. Patyk and R. E. M. Morales, Sensors, 2017, 17, 1278–1288 CrossRef PubMed.
- J. Sjoblom, N. Aske, I. Harald Auflem, O. Brandal, T. Erik Havre, O. Saether, A. Westvik, E. Eng Johnsen and H. Kallevik, Adv. Colloid Interface Sci., 2003, 100–102, 399–473 CrossRef CAS.
- F. M. Adebiyi and V. Thoss, Fuels, 2014, 118, 426–431 CrossRef CAS.
- T. A. Adedosu and O. O. Sonibare, J. Appl. Sci., 2005, 5, 906–909 CrossRef.
- I. Abdulkadir, S. A. Uba, A. Salihu and M. N. Almustapha, Nig. J. of Basic and Appl. Sci., 2016, 24, 47–55 CrossRef.
- B. Gawel, M. Eftekhardadkhah and G. Oye, Energy Fuels, 2014, 28, 997–1003 CrossRef CAS.
- T. A. Adedosu, H. O. Adedosu, O. S. Sojinu and A. A. Olajire, Environ. Earth Sci., 2013, 68, 2139–2144 CrossRef CAS.
- C. G. Seguel, S. M. Mudge, C. Salgado and M. Toledo, Water Res., 2001, 17, 4166–4174 CrossRef.
- M. M. Mudge and C. E. Duce, Environ. Pollut., 2005, 136, 209–220 CrossRef PubMed.
- G. S. Kapur and S. Berger, Fuel, 2002, 81, 883–892 CrossRef CAS.
- S. M. Rıos and N. S. Nudelmann, J. Dispersion Sci. Technol., 2005, 26, 1–8 CrossRef.
- J. G. Speight, The Chemistry and Technology of Petroleum, Marcel Dekker, New York, 1991 Search PubMed.
- T. K. Dutta and S. Harayama, Environ. Sci. Technol., 2000, 34, 1500–1505 CrossRef CAS.
- F. Sanchez-Minero, J. Ancheyta, G. Silva-Oliver and S. Flores-Valle, Fuel, 2013, 110, 318–321 CrossRef CAS.
- Y. Yang, B. Liu, H. Xi, X. Sun and T. Zhang, Fuel, 2003, 82, 721–727 CrossRef CAS.
- M. Dutta Majumdar, R. Gerken, R. Mikula and P. Hazendonk, Energy Fuels, 2013, 27, 6528–6537 CrossRef.
- K. A. Thorn and L. G. Cox, PLoS One, 2015, 10(11), e0142452 CrossRef PubMed.
- Y. Li, Y. Liu, D. Jiang, J. Xu, X. Zhao and Y. Hou, Mar. Pollut. Bull., 2018, 133, 852–860 CrossRef CAS PubMed.
- G. Gao, J. Cao, T. Xu, H. Zhang, Y. Zhang and K. Hua, Fuel, 2020, 271, 117622 CrossRef CAS.
- C. Zheng, M. Zhu, W. Zhou and D. Zhang, J. Energy Resour. Technol., 2017, 139, 1–9 Search PubMed.
- G. Ji, C. Zhou and G. Zhou, Ultrason. Sonochem., 2011, 18, 506–512 CrossRef CAS PubMed.
- X. Li, Y. Du, G. Wu, Z. Li, H. Li and H. Sui, Chemosphere, 2012, 88, 245–249 CrossRef CAS PubMed.
- J. Wang, X. Zhang and G. Li, J. Soils Sediments, 2012, 12, 117–127 CrossRef CAS.
- N. Menkiti, C. Isanbor and O. Ayejuyo, Chem. Pap., 2019, 73, 1743–1752 CrossRef CAS.
- M. Ehrhardt and G. Petrick, Mar. Pollut. Bull., 1989, 20, 560–565 CrossRef CAS.
- M. S. Maged, E. A. Omayma, A. F. Nazik and M. M. Abd El Rahman, Egypt. J. Pet., 1999, 8, 77–85 Search PubMed.
- E. E. Banda-Cruz, N. V. Gallardo-Rivas, R. D. Martínez-Orozco, U. Páramo-García and A. M. Mendoza-Martínez, J. Appl. Spectrosc., 2021, 87, 1157–1162 CrossRef CAS.
- N. Pieri, J. Planche and J. Kister, Analusis, 1996, 24, 113–122 CAS.
- H. H. Ganz and W. Kalkreuth, Fuel, 1987, 66, 708–711 CrossRef CAS.
- A. Permanyer, L. Douifi, A. Lahcini, J. Lamontagne and J. Kister, Fuel, 2002, 81, 861–866 CrossRef CAS.
- I. A. Udoetok and L. C. Osuji, Environ. Monit. Assess., 2008, 141, 359–364 CrossRef CAS PubMed.
- N. S. Nudelmann, S. M. Rĺos and O. Katusich, J. Phys. Org. Chem., 2008, 21, 329–337 CrossRef.
- S. M. Rios, M. Barquín and N. S. Nudelmann, J. Phys. Org. Chem., 2014, 27, 352–357 CrossRef CAS.
- A. Mohammad and R. B. V. Azeredo, Fuel, 2012, 130, 126–134 CrossRef.
- J. C. Poveda and D. R. Molina, J. Pet. Sci. Eng., 2012, 84–85, 1–7 CrossRef CAS.
- S. Dragovic' and A. Onjia, J. Environ. Radioact., 2006, 89, 150–158 CrossRef PubMed.
- I. U. Etukudo, I. J. Okop and C. O. Obadimu, Int. J. Eng. Sci., 2014, 3, 06–17 Search PubMed.
- E. Banda, S. Padrón, N. Gallardo, U. Páramo, N. Díaz and A. Melo, J. Eng. Technol., 2017, 6, 49–58 Search PubMed.
- E. O. Odebunmi and S. A. Adeniyi, Bull. Chem. Soc. Ethiop., 2007, 21, 135–140 CrossRef CAS.
- R. A. Friedel and M. Orchin, Ultraviolet Spectra of Aromatic Compounds, Wiley Interscience, New York, 1951, p. 16 Search PubMed.
- W. Giger and M. Blumer, Anal. Chem., 1974, 46, 1663–1671 CrossRef CAS PubMed.
- E. M. Levy, Mar. Pollut. Bull., 1980, 11, 51–56 CrossRef CAS.
- M. C. Merola, C. Carotenuto, V. Gargiulo, F. Stanzione, A. Ciajolo and M. Minale, Fuel Process. Technol., 2016, 148, 236–247 CrossRef CAS.
- W. Liang, C. Ying, L. Dane, D. Raja, C. Sreenivasulu and N. Ravi, Spectrochim. Acta, Part A, 2019, 207, 183–188 CrossRef PubMed.
- R. M. Silverstein, Spectroscopic Identification of Organic Compounds, John Wiley & Sons, New York, 6th edn, 1998, p. 43 Search PubMed.
- J. G. Speight, The Chemistry and Technology of Petroleum, Marcel Dekker, New York, 3rd edn, 1998, p. 67 Search PubMed.
- C. Wang, B. Chen, B. Zhang, P. Guo and M. Zhao, Environ. Sci.: Processes Impacts, 2014, 16, 2408–2414 RSC.
- J. K. Volkman, R. Alexander, R. I. Kagi, S. J. Rowland and P. N. Sheppard, Org. Geochem., 1984, 6, 619–632 CrossRef CAS.
- S. C. Snedaker, P. W. Glynn, D. G. Rumbold and E. F. Corcoran, Mar. Pollut. Bull., 1995, 30, 83–89 CrossRef CAS.
- S. O. Sojinu, O. O. Sonibare, O. Ekundayo and E. Y. Zeng, Sci. Total Environ., 2012, 441, 89–96 CrossRef CAS PubMed.
- B. Didyk, B. Simoneit, S. Brassell and G. Eglinton, Nature, 1978, 272, 216–222 CrossRef CAS.
- O. L. Faboya, S. O. Sojinu, O. O. Sonibare, O. T. Falodun and Z. Liao, Environ. Forensics, 2016, 17, 27–35 CrossRef CAS.
- P. E. Kolattukudy, Chemistry and biochemistry of natural waxes, Elsevier, Amsterdam, The Netherlands, 1976 Search PubMed.
- A. G. Marshall and R. P. Rodgers, Acc. Chem. Res., 2004, 37, 53–69 CrossRef CAS PubMed.
- T. L. Schaeffer, S. G. Cantwell, J. L. Brown, D. S. Watt and R. R. Fall, Appl. Environ. Microbiol., 1979, 38, 742–746 CrossRef CAS PubMed.
- J. S. Watson, D. M. Jones, R. P. J. Swannell and A. C. T. van Guin, Org. Geochem., 2002, 33, 1153–1169 CrossRef CAS.
- N. A. Tomczyk and R. E. Winans, Energy Fuels, 2001, 15, 1498–1504 CrossRef CAS.
- D. Mao, R. Lookman, H. Van De Weghe, R. Weltens, G. Vanermen, N. De Brucker and L. Diels, Chemosphere, 2009, 77, 1508–1513 CrossRef CAS PubMed.
- H. Maki, T. Sasaki and S. Harayama, Chemosphere, 2001, 44, 1145 CrossRef CAS PubMed.
- Y. Dong, Z. Lang, X. Kong, D. Lu and Z. Liu, Environ. Sci.: Processes Impacts, 2015, 17, 763–774 RSC.
- S. B. Akinde and O. Obire, World J. Microbiol. Biotechnol., 2008, 24, 1999–2002 CrossRef.
- D. Singh and M. H. Fulekar, Innovative Rom. Food Biotechnol., 2007, 1, 31–36 Search PubMed.
- D. Singh and M. H. Fulekar, J. Hazard. Mater., 2008, 175, 336–343 CrossRef PubMed.
- N. Esquinas, E. Rodríguez-Valdés, G. Márquez and J. L. R. Gallego, Chemosphere, 2017, 184, 1089–1098 CrossRef CAS PubMed.
- Z. Zhou, J. Lu, H. Wu and L. Yang, Pet. Sci. Technol., 2021, 39, 441–449 CrossRef CAS.
|
This journal is © The Royal Society of Chemistry 2022 |