DOI:
10.1039/D2RA00226D
(Paper)
RSC Adv., 2022,
12, 5501-5508
A theoretical study on the formation mechanism of carboxylic sulfuric anhydride and its potential role in new particle formation†
Received
12th January 2022
, Accepted 6th February 2022
First published on 16th February 2022
Abstract
New particle formation (NPF) is the major source of atmospheric aerosol particles. However, the chemical species involved and the exact mechanism are still unclear. Cycloaddition reaction of SO3 to carboxylic acids bas been identified as a possible formation mechanism of carboxylic sulfuric anhydrides which may be involved in NPF. Herein, energy profiles for forming diaterpenylic acetate sulfuric anhydride (DTASA) through cycloaddition of SO3 to diaterpenylic acid acetate (DTAA) and the potential role of DTASA in NPF were studied through computational methods combined with atmospheric cluster dynamics code (ACDC). Gas phase reaction barriers for the two carboxyl groups of DTAA are 0.4 and 0.6 kcal mol−1, respectively, illustrating a feasible formation mechanism for DTASA. According to thermodynamical analysis and dynamical simulations, atmospheric clusters containing DTASA and atmospheric nucleation precursors sulfuric acid (SA), ammonia (NH3) and dimethylamine (DMA) possess both thermodynamically and dynamically higher stabilities than those of DTAA-contained clusters. Furthermore, DTASA–NH3 and DTASA–DMA are more stable than SA–NH3 and SA–DMA, enabling DTASA, even carboxylic sulfuric anhydrides, to become potential participants in the atmospheric NPF process which may hence promote a better understanding of NPF.
1. Introduction
Aerosol particles are an important part of air pollution, which can influence human health, global climate and the environment in direct or indirect ways.1 A mass of studies have shown that new particle formation (NPF) through gas-to-particle conversion is the main source of atmospheric aerosol particles and the leading uncertainties in global climate modeling and forecasting.2–4
Sulfuric acid (SA) has been proved to be essential to initiate new particle formation in the atmosphere2,5–8 through clustering with various nucleation precursors such as water (H2O),9 ammonia (NH3),10–12 dimethylamine (DMA),13,14 methylamine (MA),15 monoethanolamine (MEA),16 piperazine (PZ),17 methanesulfonic acid,18,19 nitric acid20 and iodic acid.21,22 However, it is not efficient enough to explain the particle formation rates observed in the atmosphere, suggesting that other compounds may participate in the nucleation process. Low molecular weight organic acids such as glycolic acid,23 malonic acid,24 lactic acid25 and glyoxylic acid26 were identified to promote SA–NH3 or SA–DMA cluster formation efficiently through an analogous “catalytic” mechanism where the organic acid participated in cluster formation and finally evaporated out from the clusters due to their high volatilities. That is, they can not form stable enough clusters in the atmospheric nucleation process which is the initial step of new particle formation. Highly oxygenated molecules (HOMs) have been subsequently identified to be comparable to SA27 and form stable clusters with atmospheric nucleation precursors in the atmospheric nucleation process. As a second-generation oxidation product of α-pinene in the atmosphere, diaterpenylic acid acetate (DTAA) is a representative HOMs with the high O/C ratio of 6/10 and two carboxyl groups (C10H16O6). Hence it is likely to be involved in the atmospheric new particle formation.28 Furthermore, recent studies have shown that except the dominant reaction of sulfur trioxide (SO3) with water vapor in the troposphere,29 cycloaddition reaction of SO3 to carboxylic acids is a possible formation mechanism of carboxylic sulfuric anhydrides which would have lower vapor pressure than the corresponding carboxylic acids and have more inter-molecular interaction sites.30–32 It is hence speculated that the contribution of DTAA to new particle formation may be improved by the reaction with SO3 to generate diaterpenylic acetate sulfuric anhydride (DTASA, C10H16O9S).
In the present study, reaction mechanism as well as energy profile for the cycloaddition reaction of SO3 to DTAA was first calculated to generate DTASA. Potential ability of DTAA and DTASA in the initial step of atmospheric NPF were further investigated and compared both in the thermodynamic and dynamic aspects to illustrate the importance of cycloaddition reaction of SO3 to carboxylic acids and carboxylic sulfuric anhydride in NPF.
2. Computational methods
2.1 Computational details
All the geometry optimizations and vibrational frequency calculations with density functional theory (DFT) were performed in Gaussian 09.33 M06-2X functional was used with 6-311++G(3df,3pd) basis set for the stationary points calculation of the gas phase reaction of SO3 to DTAA molecules at 298 K since M06-2X/6-311++G(3df,3pd) has been proved to show good performance in thermochemistry and free energies calculations for atmospheric relevant reactions and clusters formation.34–39 The vibration frequency of the transition state was calculated to ensure that there was only one imaginary frequency. In order to ensure the proper reactant and product sides, intrinsic reaction coordinate (IRC) calculations were further performed.40–42 For a more accurate evaluation, single point calculations at the DLPNO-CCSD(T)/aug-cc-pVTZ level of theory43 were applied to all optimized stationary points with the ORCA program44 for the fact that the DLPNO-CCSD(T)/aug-cc-pVTZ results have been proved to be consistent with that of CCSD(T)-F12a/VDZ-F12, and yield a small mean absolute error.45 In this case, for reactions of SO3 to DTAA, the corrected Gibbs free energies were obtained by combining the single point energies (ΔEDLPNO) at the DLPNO-CCSD(T)/aug-cc-pVTZ level with the thermal contribution to the Gibbs free energy (ΔGTherm) at the M06-2X/6-311++G(3df,3pd) level as shown in eqn (1). |
ΔGDLPNO = ΔEDLPNO + ΔGTherm
| (1) |
2.2 Cluster structure sampling
As both DTAA, DTASA possess multi-functional groups which could form inter-molecular interactions with atmospheric precursors through different configurations, a global minima sampling process (Fig. S1†) which was employed in our former studies concerning atmospheric cluster formation was used to search the global minimum structure. Firstly, 1000 initial lower-energy structures were generated automatically with ABCluster (a software that utilizes artificial bee colony algorithm to build clusters configurations)46 and then optimized with the PM7 semi-empirical method in Mopac.47 After that, 100 structures with lower energies were obtained and re-optimized at the M06-2X/6-31+G* level of theory. Finally, 30 optimal guessed structures as well as a large number of manual sampling for each cluster formation considering the complexity of cluster isomers were optimized at the M06-2X/6-311++G(3df,3pd) level of theory and the global minimum structure was ultimately obtained. It should be noticed that two conformers of sulfuric acid (trans-SA and cis-SA) were taken into account in the first automatical clusters configurations building with ABCluster software and manual configurations building to make sure a comprehensive sampling.
2.3 Wavefunction analysis
Electrostatic potential (ESP) on the surface of molecular plays an important role in predicting reactive sites and inter-molecular interaction sites. The site with more positive ESP is more likely to react with the sites which possesses more negative ESP,48 forming reactant complex or stable clusters. Based on the outputs of Multiwfn,49,50 ESP-mapped van der Waals (vdW) surface were visualized with VMD program.51 Localized orbital locator (LOL) is one of the real space functions with the ability to reveal the molecular electronic structure, hence the color-filled LOL map of atmospheric cluster was analyzed with Multiwfn, where the minimum and maximum localization electron density are represented by blue and red area, respectively, and the redder area indicates a stronger interaction between two nuclei. Based on the electron density ρ which is closely related to bonding strength,52 the topological characteristics of bond critical points (BCPs) were further analyzed so as to investigate the strength of molecular interactions (hydrogen bond and proton transfer) in the cluster. Hydrogen bond energy (EH) could be calculated approximately by the potential energy density V(rbcp) at corresponding BCP according to Espinosa et al.53 shown in eqn (2).
In addition, to evaluate bond strength of the newly formed covalent bond through proton transfer, Laplacian bond order (LBO) which is positively correlated with bonding strength52 was calculated using Multiwfn.54
2.4 Kinetic simulation
Kinetic simulation was carried out to determine evaporation coefficient of the monomer from corresponding cluster as well as the dynamical stability of cluster with Atmospheric Cluster Dynamics Code (ACDC) in MATLAB-R2013a program.55,56 The evaporation coefficient were calculated with eqn (3). |
 | (3) |
where βij is the collision coefficient between cluster i and j, cei is the equilibrium concentration of cluster i, ΔGi is the Gibbs free energy for forming cluster i, and cref is the monomer concentration of the reference vapor corresponding to a pressure of 1 atm where the Gibbs free energies were calculated.
3. Results and discussion
Reaction sites between DTAA and SO3 was first predicted through the electrostatic potential (ESP), the reaction mechanism as well as the energy profile was subsequently calculated. And finally, potential role of DTAA and DTASA in the nucleation process were identified and compared. The Gibbs free energies (GDFT) and Gibbs free energy correction terms (GTherm) at M06-2X/6-311++G(3df,3pd) level, single point energies (EDLPNO) at DLPNO-CCSD(T)/aug-cc-pVTZ level, the corrected Gibbs free energies (GDLPNO), the imaginary frequency of transition state structures were collected at Tables S1 and S2 in the ESI.† Topological data of the atmospheric clusters (Tables S4–S7†) and coordinates of all stationary points (Tables S8–S27†) were reported in the ESI.†
3.1 Prediction of the cycloaddition reaction sites between DTAA and SO3
The electrostatic potential (ESP) on the van der Waals (vdW) surface of DTAA and SO3 molecules was used to predict reaction sites where the site with more positive ESP is more likely to react with the site with more negative ESP.48 As clearly shown in Fig. 1, the hydrogen atoms in the carboxyl groups (–COOH) of DTAA have more positive ESP, which makes it easy to interact with the oxygen atoms with more negative ESP in SO3 molecule. Meanwhile, the sulfur atom in SO3 possesses more positive ESP, hence is more likely to interact with the oxygen atoms possessing more negative ESP in –COOH of DTAA. Therefore, ideal reaction sites for the cycloaddition reaction of SO3 to DTAA was presented in Fig. 1(c), which is consistent with the mechanism proposed in recent studies.30,31
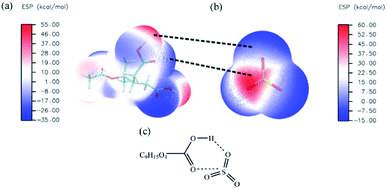 |
| Fig. 1 ESP on molecular vdW surface of (a) DTAA molecule (b) SO3 molecule. The unit is kcal mol−1. The white, green, red and yellow sticks represent H, C, O and S atoms, respectively. The black dotted lines between the positive and negative ESP represent the tendency of the weak inter-molecular interaction. (c) Possible reactant complex of SO3 and DTAA. | |
3.2 Gas phase cycloaddition reaction of SO3 to DTAA
According to the predicted reaction complex of SO3 and DTAA, reaction mechanism as well as the energy profile were further calculated. There are two different carboxyl groups (–COOH) in DTAA molecule shown in Fig. 2(a) for which the energy barriers may be different. The reactant state (Reactn), transition state (TSn) and the generated product (Prodn) were optimized and shown in Fig. 2(b) where n represents the position of the –COOH functional group and the corresponding carbon atom located. It is clear to see that two –COOH functional groups in DTAA could both react with SO3 to generate DTASA-1 and DTASA-2 through a reaction barrier of 0.4 and 0.6 kcal mol−1, respectively, relative to the reactant complex React1 and React2. Even though the reaction barrier of –COOH group containing C5 is slightly higher (0.6 kcal mol−1) than that of C1 (0.4 kcal mol−1), it is still competitive and enable the reaction feasible in the gas phase. Meanwhile, the generated DTASA-1 and DTASA-2 are 4.3 and 5.0 kcal mol−1 lower than that of the reactant monomers (DTAA + SO3). For a clear comparison, the most stable configurations of DTAA, DTASA-1 and DTASA-2 have been shown in Fig. S2.†
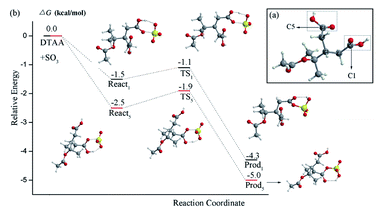 |
| Fig. 2 (a) The most stable molecular structure of DTAA monomer. (b) Reaction energy profiles (kcal mol−1) for the cycloaddition reaction of SO3 to DTAA at DLPNO-CCSD(T)/aug-cc-pVTZ//M06-2X/6-311++G(3df,3pd) level of theory. The H, C, O and S atoms are represented as white, gray, red and yellow spheres, respectively. | |
DTASA-1 and DTASA-2 have been shown in Fig. S2.† In general, DTASA which is stable in the gas phase can be easily generated through cycloaddition reaction of SO3 to DTAA molecule with low reaction barriers. For the advantages that –HSO4 functional group in DTASA possesses more inter-molecular interaction sites than the –COOH functional group in DTAA, DTASA may play a potentially important role in atmospheric new particle formation. Herein, the potential role of DTAA as well as DTASA in participating gas phase nucleation process was further investigated and compared by topological analysis and dynamic simulations.
3.3 The potential role of DTAA and DTASA in the initial new particle formation
In this section, the possibilities of DTAA and DTASA to participate in NPF were compared through the thermodynamical and dynamical stabilities of corresponding clusters containing common atmospheric nucleation precursors – water molecule (H2O), ammonia (NH3), dimethylamine (DMA) and sulfuric acid (SA). The Gibbs free energy for formation of these clusters (ΔG), potential energy density V(r) at corresponding BCPs and hydrogen bond energy (EH)53 were collected in Tables S5–S8 in the ESI.†
3.3.1 Comparison of DTAA, DTASA and SA in NPF containing H2O. Ubiquitous water (H2O) molecule is abundant in the atmosphere and involved in new particle formation process,57 clusters containing H2O (DTAA–H2O, DTASA–H2O and SA–H2O) were firstly calculated and compared. Fig. 3 presents the most stable structures and corresponding color-filled LOL maps which show the presence of hydrogen bonds. And clearly, clusters DTAA–H2O, DTASA–H2O and SA–H2O each contain two hydrogen bonds and the hydrogen bond energies (EH) in cluster DTASA–H2O is the largest (−27.01 and −5.86 kcal mol−1) as collected in Table S4.† However, the Gibbs free energy for forming DTASA–H2O is −2.76 kcal mol−1, slightly larger than that of SA–H2O (−2.93 kcal mol−1). Moreover, DTAA has low hygroscopicity with the reaction free energy of 0.21 kcal mol−1 for forming DTAA–H2O. Combining EH of the hydrogen bonds and Gibbs free energies of formation, thermodynamical stabilities for clusters are in the order of SA–H2O > DTASA–H2O > DTAA–H2O. Even the cluster SA–H2O is slightly more stable than DTASA–H2O, but DTASA is still competitive in the initial new particle formation due to its stronger interaction with water.
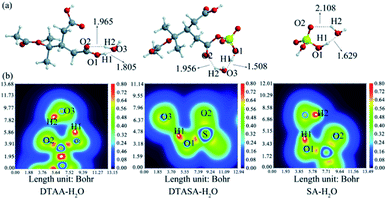 |
| Fig. 3 (a) The most stable configurations at M06-2X/6-311++G(3df,3pd) level of theory and (b) corresponding color-filled LOL map of clusters DTAA–H2O, DTASA–H2O and SA–H2O. Dashed lines represent the hydrogen bonds, and all distances are in angstroms. The H, C, O and S atoms are represented as white, gray, red and yellow spheres, respectively. | |
3.3.2 Comparison of DTAA, DTASA and SA in NPF containing base species (NH3 and DMA). Base species such as ammonia (NH3) and dimethylamine (DMA) are considered to stabilize embryonic sulfuric acid clusters against evaporation through forming acid–base pairs and then enhance sulfate aerosol formation.58 In the formation of acid–base pairs, molecular interactions transformed from hydrogen-bonding interactions to electrostatic interactions through the transfer of a proton from acid molecule to base molecule. This could enhance molecular interactions in acid–base cluster and further improve cluster stability.59 In this section, the most stable configurations of clusters DTAA–NH3, DTASA–NH3 and SA–NH3 were calculated and presented in Fig. 4(a) along with corresponding color-filled map of LOL confirming the existence of proton transfer. Apparently, there are no proton transfer in clusters DTAA–NH3 and SA–NH3, both of which contain two hydrogen bonds. The EH of the major hydrogen bond (O1–H1⋯N) in DTAA–NH3 (−14.44 kcal mol−1) is higher than that in SA–NH3 (−24.96 kcal mol−1) and the EH difference between the other hydrogen bonds in clusters DTAA–NH3 and SA–NH3 is only −0.07 kcal mol−1. Hence the molecular interactions in cluster SA–NH3 are stronger than those in cluster DTAA–NH3. In the case of the LOL map of DTASA–NH3, the green area of O1⋯H1 and the red area of H1–N indicate that the proton transfer of H1 atom in DTASA molecule to NH3 molecule has completed, and the stability of cluster was improved due to proton transfer and ion-pair formation. In addition, cluster DTASA–NH3 contains three hydrogen bonds with the EH of −27.01, −5.52 and −4.19 kcal mol−1. Combining EH with the Gibbs free energies for formation of DTAA–NH3, DTASA–NH3 and SA–NH3 (−0.71, −8.34 and −7.33 kcal mol−1), clusters thermodynamical stabilities are in the order of DTASA–NH3 > SA–NH3 > DTAA–NH3.
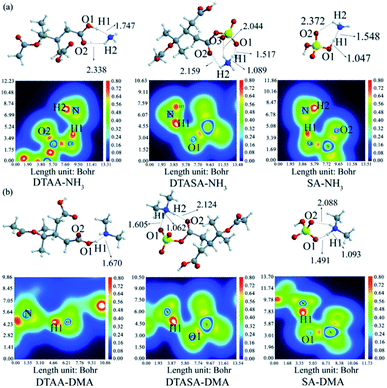 |
| Fig. 4 The most stable configurations at M06-2X/6-311++G(3df,3pd) level of theory and corresponding color-filled LOL map of clusters (a) DTAA–NH3, DTASA–NH3 and SA–NH3. (b) DTAA–DMA, DTASA–DMA and SA–DMA. Dashed lines represent the hydrogen bonds, and all distances are in angstroms. The H, C, N, O and S atoms are represented as white, gray, blue, red and yellow spheres, respectively. | |
For clusters containing DMA which is highly alkaline than NH3 as shown in Fig. 4(b), there is still no proton transfer in cluster DTAA–DMA which contains only one hydrogen bond with the EH of −18.81 kcal mol−1 and it is the least stable. Compared with clusters DTAA–DMA, a complete proton transfer from SA or DTASA to DMA molecule has taken place, resulting that both clusters SA–DMA and DTASA–DMA contain a newly formed covalent bond N–H1 through proton transfer and two hydrogen bonds. Meanwhile, the Laplacian bond order (LBO) of N–H1 in cluster DTASA–DMA is 0.59, which is larger than that of SA–DMA (0.48), indicating that cluster DTASA–DMA is more stable. Moreover, the Gibbs free energies for formation of DTAA–DMA, DTASA–DMA and SA–DMA are −1.90, −15.53 and −11.25 kcal mol−1, respectively. Therefore, clusters thermodynamical stabilities are in the order of DTASA–DMA > SA–DMA > DTAA–DMA. In this case, DTASA presents a considerable advantage on forming stable clusters with base molecules even than SA. For the area where concentration of base molecules is relatively higher, DTASA is speculated to play a more important role in NPF process.
3.3.3 Comparison of DTAA, DTASA and SA in NPF containing SA. Considering the essential role of sulfuric acid (SA) in aerosol particles formation,2,60 the thermodynamical stabilities of clusters containing SA molecule were studied and compared. As the most stable configurations of clusters DTAA–SA, DTASA–SA and SA–SA shown in Fig. 5, both DTAA–SA and SA–SA contain two hydrogen bonds. The EH are −27.40, −12.90 kcal mol−1 for DTAA–SA and −16.82, −16.83 kcal mol−1 for SA–SA. As to DTASA–SA, there are three hydrogen bonds with the EH of −19.70, −16.57 and −8.35 kcal mol−1. In the case of the Gibbs free energies for formation, DTASA–SA (−7.99 kcal mol−1) and DTAA–SA (−7.88 kcal mol−1) are both slightly larger than that of SA–SA (−8.42 kcal mol−1), with the differences of 0.43 and 0.54 kcal mol−1, respectively. Based on the EH and Gibbs free energies of formation, the thermodynamical stabilities of clusters containing SA are in the order of SA–SA > DTASA–SA > DTAA–SA. In summary, clusters containing DTAA are the least thermodynamically stable compared with the clusters containing SA or DTASA, while organic sulfuric anhydride DTASA produced by the cycloaddition reaction of SO3 to DTAA has a stronger interaction with atmospheric nucleation precursors due to the advantage of –SO4H functional group. Therefore, it is speculated that DTASA possesses potential to participate in atmospheric new particle formation.
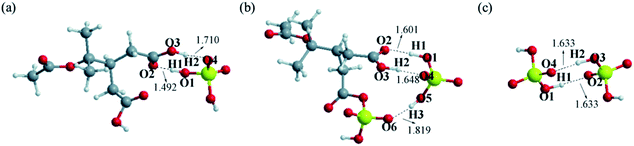 |
| Fig. 5 The most stable configurations at M06-2X/6-311++G(3df,3pd) level of theory of clusters (a) DTAA–SA (b) DTASA–SA (c) SA–SA. Dashed lines represent the hydrogen bonds, and all distances are in angstroms. The H, C, O and S atoms are represented as white, gray, red and yellow spheres, respectively. | |
3.4 Comparison of DTASA, DTAA and SA in NPF through kinetic simulations
Thermodynamic stabilities of clusters were systematic compared based on the thermodynamic and topological analysis. It was found that DTASA has stronger interactions with nucleation precursors compared with DTAA, and, even has the strongest interactions with base molecules (NH3 and DMA) when compared with SA. Atmospheric Cluster Dynamics Code (ACDC) simulations were further performed to study the evaporation coefficients of different monomers from corresponding clusters and the dynamical stabilities of clusters.55,59 A higher evaporation coefficient of monomer (H2O, NH3, DMA and SA) from clusters means a weak inter-molecular interactions and a higher probability to evaporate out from the clusters, that is, the clusters is less dynamic stable.
As clearly presented in Fig. 6, for clusters containing H2O, the evaporation coefficient of monomers in the DTAA–H2O is 2 and 3 orders of magnitude higher than those of DTASA–H2O and SA–H2O, respectively, due to the weak inter-molecular interactions and the high Gibbs free energy for formation. Therefore, the dynamical stabilities for clusters containing H2O are in the order of SA–H2O > DTASA–H2O > DTAA–H2O. As for clusters containing base species (NH3 and DMA), the dynamical stabilities for the clusters are both in the order of DTASA–base > SA–base > DTAA–base. It is worth mentioning that for DMA-contained clusters, the evaporation coefficient of monomers in the DTASA–DMA is 10 and 2 orders of magnitude lower than those in DTAA–DMA and SA–DMA, respectively, indicating that cluster DTASA–DMA is relatively more stable and potentially involved in the atmospheric nucleation process. For the clusters containing SA, the dynamical stabilities are in the order of SA–SA > DTASA–SA > DTAA–SA, but there is less difference of evaporation coefficients among three clusters. All the dynamical stabilities order are totally in consistent with the thermodynamical stabilities order drawn in section 2.3.1–2.3.3. In general, clusters containing DTAA are the least stable, and its potential role in NPF could be improved by the cycloaddition reaction with SO3 to generate DTASA. DTASA could form more stable clusters with base species (NH3 and DMA) than SA and DTAA, indicating that DTASA has potential to participate in atmospheric NPF where the concentration of base molecules is relatively higher.
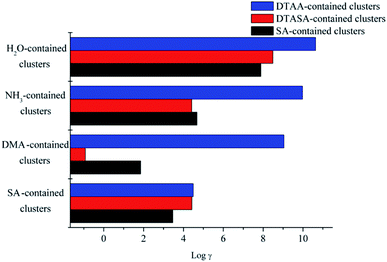 |
| Fig. 6 The logarithms of evaporation coefficient γ(1/s) of the monomers in the corresponding clusters at 298 K. | |
4. Conclusions
Carboxylic sulfuric anhydrides formed through cycloaddition reaction of SO3 to carboxylic acids are considered to have the potential to participate in atmospheric new particle formation. Reaction mechanism as well as the energy profile for the cycloaddition reaction of SO3 to diaterpenylic acid acetate (DTAA) was studied at the DLPNO-CCSD(T)/aug-cc-pVTZ//M06-2X/6-311++G(3df,3pd) level of theory. The reaction barriers for the two carboxy groups of DTAA are 0.4 and 0.6 kcal mol−1, respectively, demonstrating that the reaction are feasible to take place in the gas phase to generate diaterpenylic acetate sulfuric anhydride (DTASA) which possesses the –SO4H functional group. For the aim of identifying the potential role of DTASA, thermodynamical and dynamical stabilities of clusters containing DTASA and common atmospheric nucleation precursors were subsequently calculated and compare with DTAA and SA. According to topological analysis and Atmospheric Cluster Dynamics Code (ACDC) simulations, the inter-molecular interactions in DTASA-contained clusters are stronger than those of DTAA-contained clusters, presenting higher thermodynamic and dynamic stabilities. Moreover, DTASA could form more stable clusters with base species (NH3 and DMA) even than SA, indicating that DTASA may play a potential role in the initial new particle formation where the concentration of base molecules is relatively higher.
In conclusion, the dominant sink of SO3 is its reaction with water vapor (H2O) in the troposphere to the significant formation of sulfuric acid and thus promotes sub-2.5 nm particle formation. Considering the aggregate concentration and variety of organic acids in the atmosphere, especially the area where the H2O concentration is relatively lower and organic acid concentration is relatively higher, cycloaddition reaction of SO3 to organic acids could improve the nucleation ability of organic acids. That is, the generated carboxylic sulfuric anhydrides may play a potential role in atmospheric chemistry and hence should be cautiously valued. Furthermore, this may promote a better understanding of NPF and contribute to a better science-based solutions in the resolving of heavy haze problems.
Author contributions
Conceptualization, Haijie Zhang; methodology, software, investigation, Haijie Zhang and Rui Gao; data curation, Wei Wang; writing—original draft preparation, Wei Wang and Haijie Zhang; writing—review and editing, Haijie Zhang, Yisheng Xu and Hong Li; supervision, Yisheng Xu and Hong Li.
Conflicts of interest
There are no conflicts of interest to declare.
Acknowledgements
This research was funded by the China Postdoctoral Science Foundation (Grant No. 2020M680636), the National Natural Science Foundation of China (No. 41375133), the Science Foundation of Chinese Research Academy of Environmental Sciences (No. JY-41375133), the Budget Surplus of Central Financial Science and Technology Plan (No. 2021-JY-14), the Beijing Municipal Science & Technology Commission (Grant No. Z181100005418015) and National Key Research and Development Program of China (Grant No. 2019YFC0214800).
References
- R. Zhang, I. Suh, J. Zhao, D. Zhang, E. C. Fortner, X. Tie, L. T. Molina and M. J. Molina, Science, 2004, 304, 1487–1490 CrossRef CAS PubMed.
- M. Kulmala, J. Kontkanen, H. Junninen, K. Lehtipalo, H. E. Manninen, T. Nieminen, T. Petaja, M. Sipila, S. Schobesberger, P. Rantala, A. Franchin, T. Jokinen, E. Jarvinen, M. Aijala, J. Kangasluoma, J. Hakala, P. P. Aalto, P. Paasonen, J. Mikkila, J. Vanhanen, J. Aalto, H. Hakola, U. Makkonen, T. Ruuskanen, R. L. Mauldin 3rd, J. Duplissy, H. Vehkamaki, J. Back, A. Kortelainen, I. Riipinen, T. Kurten, M. V. Johnston, J. N. Smith, M. Ehn, T. F. Mentel, K. E. Lehtinen, A. Laaksonen, V. M. Kerminen and D. R. Worsnop, Science, 2013, 339, 943–946 CrossRef CAS PubMed.
- M. Ehn, J. A. Thornton, E. Kleist, M. Sipila, H. Junninen, I. Pullinen, M. Springer, F. Rubach, R. Tillmann, B. Lee, F. Lopez-Hilfiker, S. Andres, I. H. Acir, M. Rissanen, T. Jokinen, S. Schobesberger, J. Kangasluoma, J. Kontkanen, T. Nieminen, T. Kurten, L. B. Nielsen, S. Jorgensen, H. G. Kjaergaard, M. Canagaratna, M. D. Maso, T. Berndt, T. Petaja, A. Wahner, V. M. Kerminen, M. Kulmala, D. R. Worsnop, J. Wildt and T. F. Mentel, Nature, 2014, 506, 476–479 CrossRef CAS PubMed.
- G. Zhao, Y. Zhu, Z. Wu, T. Zong, J. Chen, T. Tan, H. Wang, X. Fang, K. Lu, C. Zhao and M. Hu, Atmos. Chem. Phys., 2021, 21, 9995–10004 CrossRef CAS.
- C. Kuang, P. H. McMurry, A. V. McCormick and F. L. Eisele, J. Geophys. Res., 2008, 113, D10209 CrossRef.
- L. Yao, X. Fan, C. Yan, T. Kurtén, K. R. Daellenbach, C. Li, Y. Wang, Y. Guo, L. Dada, M. P. Rissanen, J. Cai, Y. J. Tham, Q. Zha, S. Zhang, W. Du, M. Yu, F. Zheng, Y. Zhou, J. Kontkanen, T. Chan, J. Shen, J. T. Kujansuu, J. Kangasluoma, J. Jiang, L. Wang, D. R. Worsnop, T. Petäjä, V.-M. Kerminen, Y. Liu, B. Chu, H. He, M. Kulmala and F. Bianchi, Environ. Sci. Technol. Lett., 2020, 7, 809–818 CrossRef CAS PubMed.
- C. Yan, R. Yin, Y. Lu, L. Dada, D. Yang, Y. Fu, J. Kontkanen, C. Deng, O. Garmash, J. Ruan, R. Baalbaki, M. Schervish, R. Cai, M. Bloss, T. Chan, T. Chen, Q. Chen, X. Chen, Y. Chen, B. Chu, K. Dällenbach, B. Foreback, X. He, L. Heikkinen, T. Jokinen, H. Junninen, J. Kangasluoma, T. Kokkonen, M. Kurppa, K. Lehtipalo, H. Li, H. Li, X. Li, Y. Liu, Q. Ma, P. Paasonen, P. Rantala, R. E. Pileci, A. Rusanen, N. Sarnela, P. Simonen, S. Wang, W. Wang, Y. Wang, M. Xue, G. Yang, L. Yao, Y. Zhou, J. Kujansuu, T. Petäjä, W. Nie, Y. Ma, M. Ge, H. He, N. M. Donahue, D. R. Worsnop, V.-M. Kerminen, L. Wang, Y. Liu, J. Zheng, M. Kulmala, J. Jiang and F. Bianchi, Geophys. Res. Lett., 2021, 48, e2020GL091944 CAS.
- X. Zhao, Y. Li, C. Zuo, Y. Sun, F. Xu, A. B. Nadykto, L. Du, Y. Xu, Q. Zhang and W. Wang, RSC Adv., 2021, 11, 493–500 RSC.
- V. Loukonen, T. Kurtén, I. K. Ortega, H. Vehkamäki, A. A. H. Pádua, K. Sellegri and M. Kulmala, Atmos. Chem. Phys., 2010, 10, 4961–4974 CrossRef CAS.
- D. J. Coffman and D. A. Hegg, J. Geophys. Res., 1995, 100, 7147–7160 CrossRef CAS.
- D. Chen, W. Wang, D. Li and W. Wang, RSC Adv., 2020, 10, 5173–5182 RSC.
- S.-K. Miao, S. Jiang, X.-Q. Peng, Y.-R. Liu, Y.-J. Feng, Y.-B. Wang, F. Zhao, T. Huang and W. Huang, RSC Adv., 2018, 8, 3250–3263 RSC.
- C. N. Jen, P. H. McMurry and D. R. Hanson, J. Geophys. Res., 2014, 119, 7502–7514 CrossRef CAS.
- A. Kürten, T. Jokinen, M. Simon, M. Sipilä, N. Sarnela, H. Junninen, A. Adamov, J. Almeida, A. Amorim, F. Bianchi, M. Breitenlechner, J. Dommen, N. M. Donahue, J. Duplissy, S. Ehrhart, R. C. Flagan, A. Franchin, J. Hakala, A. Hansel, M. Heinritzi, M. Hutterli, J. Kangasluoma, J. Kirkby, A. Laaksonen, K. Lehtipalo, M. Leiminger, V. Makhmutov, S. Mathot, A. Onnela, T. Petäjä, A. P. Praplan, F. Riccobono, M. P. Rissanen, L. Rondo, S. Schobesberger, J. H. Seinfeld, G. Steiner, A. Tomé, J. Tröstl, P. M. Winkler, C. Williamson, D. Wimmer, P. Ye, U. Baltensperger, K. S. Carslaw, M. Kulmala, D. R. Worsnop and J. Curtius, Proc. Natl. Acad. Sci. U. S. A., 2014, 111, 15019–15024 CrossRef PubMed.
- J. Shen, J. Elm, H. B. Xie, J. Chen, J. Niu and H. Vehkamaki, Environ. Sci. Technol., 2020, 54, 13498–13508 CrossRef CAS PubMed.
- J. Shen, H. B. Xie, J. Elm, F. Ma, J. Chen and H. Vehkamaki, Environ. Sci. Technol., 2019, 53, 14387–14397 CrossRef CAS PubMed.
- F. Ma, H. B. Xie, J. Elm, J. Shen, J. Chen and H. Vehkamaki, Environ. Sci. Technol., 2019, 53, 8785–8795 CrossRef CAS PubMed.
- H. Zhao, X. Jiang and L. Du, Chemosphere, 2017, 174, 689–699 CrossRef CAS PubMed.
- C. Zuo, X. Zhao, H. Wang, X. Ma, S. Zheng, F. Xu and Q. Zhang, J. Environ. Sci., 2021, 100, 328–339 CrossRef PubMed.
- L. Liu, F. Yu, L. Du, Z. Yang, J. S. Francisco and X. Zhang, Proc. Natl. Acad. Sci. U. S. A., 2021, 118, e2108384118 CrossRef CAS PubMed.
- M. Sipilä, N. Sarnela, T. Jokinen, H. Henschel, H. Junninen, J. Kontkanen, S. Richters, J. Kangasluoma, A. Franchin, O. Peräkylä, M. P. Rissanen, M. Ehn, H. Vehkamäki, T. Kurten, T. Berndt, T. Petäjä, D. Worsnop, D. Ceburnis, V.-M. Kerminen, M. Kulmala and C. O'Dowd, Nature, 2016, 537, 532–534 CrossRef PubMed.
- A. Ning, L. Liu, L. Ji and X. Zhang, Atmos. Chem. Phys. Discuss., 2021, 2021, 1–20 Search PubMed.
- M. J. Perri, S. Seitzinger and B. J. Turpin, Atmos. Environ., 2009, 43, 1487–1497 CrossRef CAS.
- H. Zhang, H. Li, L. Liu, Y. Zhang, X. Zhang and Z. Li, Chemosphere, 2018, 203, 26–33 CrossRef CAS PubMed.
- H. Li, O. Kupiainen-Maatta, H. J. Zhang, X. H. Zhang and M. F. Ge, Atmos. Environ., 2017, 166, 479–487 CrossRef CAS.
- L. Liu, X. Zhang, Z. Li, Y. Zhang and M. Ge, Chemosphere, 2017, 186, 430–437 CrossRef CAS PubMed.
- J. Kirkby, J. Duplissy, K. Sengupta, C. Frege, H. Gordon, C. Williamson, M. Heinritzi, M. Simon, C. Yan, J. Almeida, J. Trostl, T. Nieminen, I. K. Ortega, R. Wagner, A. Adamov, A. Amorim, A. K. Bernhammer, F. Bianchi, M. Breitenlechner, S. Brilke, X. Chen, J. Craven, A. Dias, S. Ehrhart, R. C. Flagan, A. Franchin, C. Fuchs, R. Guida, J. Hakala, C. R. Hoyle, T. Jokinen, H. Junninen, J. Kangasluoma, J. Kim, M. Krapf, A. Kurten, A. Laaksonen, K. Lehtipalo, V. Makhmutov, S. Mathot, U. Molteni, A. Onnela, O. Perakyla, F. Piel, T. Petaja, A. P. Praplan, K. Pringle, A. Rap, N. A. Richards, I. Riipinen, M. P. Rissanen, L. Rondo, N. Sarnela, S. Schobesberger, C. E. Scott, J. H. Seinfeld, M. Sipila, G. Steiner, Y. Stozhkov, F. Stratmann, A. Tome, A. Virtanen, A. L. Vogel, A. C. Wagner, P. E. Wagner, E. Weingartner, D. Wimmer, P. M. Winkler, P. Ye, X. Zhang, A. Hansel, J. Dommen, N. M. Donahue, D. R. Worsnop, U. Baltensperger, M. Kulmala, K. S. Carslaw and J. Curtius, Nature, 2016, 533, 521–526 CrossRef CAS PubMed.
- K. Kristensen, K. L. Enggrob, S. M. King, D. R. Worton, S. M. Platt, R. Mortensen, T. Rosenoern, J. D. Surratt, M. Bilde, A. H. Goldstein and M. Glasius, Atmos. Chem. Phys., 2013, 13, 3763–3776 CrossRef.
- J. Carmona-García, T. Trabelsi, A. Francés-Monerris, C. A. Cuevas, A. Saiz-Lopez, D. Roca-Sanjuán and J. S. Francisco, J. Am. Chem. Soc., 2021, 143, 18794–18802 CrossRef PubMed.
- R. B. Mackenzie, C. T. Dewberry and K. R. Leopold, Science, 2015, 349, 58–61 CrossRef CAS PubMed.
- A. K. Huff, R. B. Mackenzie, C. J. Smith and K. R. Leopold, J. Phys. Chem. A, 2017, 121, 5659–5664 CrossRef CAS PubMed.
- J. Zhong, H. Li, M. Kumar, J. Liu, L. Liu, X. Zhang, X. C. Zeng and J. S. Francisco, Angew. Chem., Int. Ed., 2019, 58, 8351–8355 CrossRef CAS PubMed.
- M. J. T. G. Frisch, G. B. V. M. Scalmani, X. H. H. P. Li, M. E. M. T. Hada, Y. K. O. N. Honda, F. B. M. H. Ogliaro, R. N. J. R. Kobayashi, S. S. T. J. Iyengar, J. B. B. V. Cross, O. A. A. J. Yazyev, K. Z. V. G. Morokuma, S. D. A. D. Dapprich and D. J. Fox, Gaussian Inc, Wallingford CT, 2009.
- Y. Zhao and D. G. Truhlar, Theor. Chem. Acc., 2008, 120, 215–241 Search PubMed.
- J. Elm, M. Bilde and K. V. Mikkelsen, J. Chem. Theory Comput., 2012, 8, 2071–2077 CrossRef CAS PubMed.
- N. Mardirossian and M. Head-Gordon, J. Chem. Theory Comput., 2016, 12, 4303–4325 CrossRef CAS PubMed.
- Z. Wang, C. Zhang, G. Lv, X. Sun, N. Wang and Z. Li, Int. J. Mol. Sci., 2019, 20, 3746 CrossRef CAS PubMed.
- H. Li, J. Zhong, H. Vehkamäki, T. Kurtén, W. Wang, M. Ge, S. Zhang, Z. Li, X. Zhang, J. S. Francisco and X. C. Zeng, J. Am. Chem. Soc., 2018, 140, 11020–11028 CrossRef CAS PubMed.
- M. A. Ali, Phys. Chem. Chem. Phys., 2019, 21, 19242–19251 RSC.
- X. Sun, C. Zhang, Y. Zhao, J. Bai, Q. Zhang and W. Wang, Environ. Sci. Technol., 2012, 46, 8148–8155 CrossRef CAS PubMed.
- F. Xu, H. Wang, Q. Zhang, R. Zhang, X. Qu and W. Wang, Environ. Sci. Technol., 2010, 44, 1399–1404 CrossRef CAS PubMed.
- Q.-D. Wang, M.-M. Sun and J.-H. Liang, Int. J. Mol. Sci., 2019, 20, 1275 CrossRef PubMed.
- C. Riplinger, B. Sandhoefer, A. Hansen and F. Neese, J. Chem. Phys., 2013, 139, 134101 CrossRef PubMed.
- F. Neese, Wiley Interdiscip. Rev.: Comput. Mol. Sci., 2012, 2, 73–78 CAS.
- J. Elm, N. Myllys and T. Kurten, J. Phys. Chem. A, 2017, 121, 4578–4587 CrossRef CAS PubMed.
- J. Zhang and M. Dolg, Phys. Chem. Chem. Phys., 2015, 17, 24173–24181 RSC.
- J. J. P. Stewart, Stewart Computational Chemistry, Colorado Springs, CO, USA, 2016 Search PubMed.
- S. Manzetti and T. Lu, J. Phys. Org. Chem., 2013, 26, 473–483 CrossRef CAS.
- T. Lu and F. Chen, J. Comput. Chem., 2012, 33, 580–592 CrossRef CAS PubMed.
- T. Lu and F. Chen, J. Mol. Graphics Modell., 2012, 38, 314–323 CrossRef CAS PubMed.
- W. Humphrey, A. Dalke and K. Schulten, J. Mol. Graphics, 1996, 14(33–38), 27–38 Search PubMed.
- J. R. Lane, J. Contreras-Garcia, J. P. Piquemal, B. J. Miller and H. G. Kjaergaard, J. Chem. Theory Comput., 2013, 9, 3263–3266 CrossRef CAS PubMed.
- E. Espinosa, E. Molins and C. Lecomte, Chem. Phys. Lett., 1998, 285, 170–173 CrossRef CAS.
- T. Lu and F. Chen, J. Phys. Chem. A, 2013, 117, 3100–3108 CrossRef CAS PubMed.
- M. J. McGrath, T. Olenius, I. K. Ortega, V. Loukonen, P. Paasonen, T. Kurten, M. Kulmala and H. Vehkamaki, Atmos. Chem. Phys., 2012, 12, 2345–2355 CrossRef CAS.
- L. F. Shampine and M. W. Reichelt, SIAM J. Sci. Comput., 1997, 18, 1–22 CrossRef.
- E. R. Lovejoy, J. Curtius and K. D. Froyd, J. Geophys. Res., 2004, 109, D08204 CrossRef.
- J. Almeida, S. Schobesberger, A. Kürten, I. K. Ortega, O. Kupiainen-Määttä, A. P. Praplan, A. Adamov, A. Amorim, F. Bianchi, M. Breitenlechner, A. David, J. Dommen, N. M. Donahue, A. Downard, E. Dunne, J. Duplissy, S. Ehrhart, R. C. Flagan, A. Franchin, R. Guida, J. Hakala, A. Hansel, M. Heinritzi, H. Henschel, T. Jokinen, H. Junninen, M. Kajos, J. Kangasluoma, H. Keskinen, A. Kupc, T. Kurtén, A. N. Kvashin, A. Laaksonen, K. Lehtipalo, M. Leiminger, J. Leppä, V. Loukonen, V. Makhmutov, S. Mathot, M. J. McGrath, T. Nieminen, T. Olenius, A. Onnela, T. Petäjä, F. Riccobono, I. Riipinen, M. Rissanen, L. Rondo, T. Ruuskanen, F. D. Santos, N. Sarnela, S. Schallhart, R. Schnitzhofer, J. H. Seinfeld, M. Simon, M. Sipilä, Y. Stozhkov, F. Stratmann, A. Tomé, J. Tröstl, G. Tsagkogeorgas, P. Vaattovaara, Y. Viisanen, A. Virtanen, A. Vrtala, P. E. Wagner, E. Weingartner, H. Wex, C. Williamson, D. Wimmer, P. Ye, T. Yli-Juuti, K. S. Carslaw, M. Kulmala, J. Curtius, U. Baltensperger, D. R. Worsnop, H. Vehkamäki and J. Kirkby, Nature, 2013, 502, 359–363 CrossRef CAS PubMed.
- J. Elm, M. Passananti, T. Kurten and H. Vehkamaki, J. Phys. Chem. A, 2017, 121, 6155–6164 CrossRef CAS PubMed.
- M. Sipila, T. Berndt, T. Petaja, D. Brus, J. Vanhanen, F. Stratmann, J. Patokoski, R. L. Mauldin 3rd, A. P. Hyvarinen, H. Lihavainen and M. Kulmala, Science, 2010, 327, 1243–1246 CrossRef PubMed.
Footnotes |
† Electronic supplementary information (ESI) available. See DOI: 10.1039/d2ra00226d |
‡ These authors contributed equally to this work. |
|
This journal is © The Royal Society of Chemistry 2022 |
Click here to see how this site uses Cookies. View our privacy policy here.