DOI:
10.1039/D1RA07328A
(Paper)
RSC Adv., 2022,
12, 1194-1207
Investigating natural compounds against oncogenic RET tyrosine kinase using pharmacoinformatic approaches for cancer therapeutics†
Received
1st October 2021
, Accepted 30th November 2021
First published on 5th January 2022
Abstract
Rearranged during transfection (RET) tyrosine kinase is a transmembrane receptor tyrosine kinase regulating vital aspects of cellular proliferation, differentiation, and survival. An outstanding challenge in designing protein kinase inhibitors is due to the development of drug resistance. The “gain of function” mutations in the RET gate-keeper residue, Val804, confers resistance to the majority of known RET inhibitors, including vandetanib. To curtail this resistance, researchers developed selpercatinib (LOXO-292) against the RET gate-keeper mutant forms – V804M and V804L. In the present in silico investigation, a receptor–ligand pharmacophore model was generated to identify small molecule inhibitors effective for wild-type (WT) as well as mutant RET kinase variants. The generated model was employed to screen 144
766 natural products (NPs) available in the ZINC database and the retrieved NPs were filtered for their drug-likeness. The resulting 2696 drug-like NPs were subjected to molecular docking with the RET WT kinase domain and a total of 27 molecules displayed better dock scores than the reference inhibitors – vandetanib and selpercatinib. From 27 NPs, an aggregate of 12 compounds demonstrated better binding free energy (BFE) scores than the reference inhibitors, towards RET. Thus, the 12 NPs were also subjected to docking, simulation, and BFE estimation towards the constructed gate-keeper RET mutant structures. The BFE calculations revealed 3 hits with better BFE scores than the reference inhibitors towards WT, V804M, and V804L RET variants. Thus, the scaffolds of hit compounds presented in this study could act as potent RET inhibitors and further provide insights for drug optimization targeting aberrant activation of RET signaling, specifically the mutation of gate-keeper residue – Val804.
1. Introduction
The RET proto-oncogene was originally discovered in NIH3T3 cells when Takahashi et al. in 1985 exogenously transfected a DNA sequence from human lymphoma cells into murine NIH3T3 fibroblasts.1 The gene was found to be rearranged during this process, which led to its name ‘REarranged during Transfection’, abbreviated as RET.2 The human RET gene was subsequently mapped to chromosome 10 (10q11.2) by Ishizaka et al.3 The encoded RET glycoprotein is a receptor tyrosine kinase (RTK) comprising three domains including the extracellular domain, the hydrophobic transmembrane domain and the intracellular tyrosine kinase domain.4 The extracellular RET domain consists of three parts encompassing the four cadherin-like domains (CLDs) of 110 residues each, one Ca2+ binding site between CLD2 and CLD3 and a cysteine-rich domain of 120 residues connecting the transmembrane domain.2,4 The intracellular RET section encompasses the juxtamembrane segment of 50 residues, the tyrosine kinase domain and a carboxy (C)-terminal tail of 100 residues long. The ‘long’ RET isoform comprises of 51 unique C-terminal residues (RET51), while the ‘short’ RET isoform consists of 9 C-terminal residues (RET9). The RTK RET plays an essential role in signaling pathways for the survival, development and maintenance of neuronal populations present in the kidney, and sympathetic, parasympathetic and enteric nervous systems.5,6 Ligands of the glial-derived neurotrophic factor (GDNF) family activates RET kinase in unification with the GDNF family receptor α1–4 (GFRα1–4) by the trans-phosphorylation phenomenon.7 The stability, maturation and translocation at the plasma membrane of RET protein is disrupted by mutations within the extracellular RET domain.8,9 Correspondingly, loss of kinase activity is associated with mutations within the intracellular kinase domain leading to aberrant kinase signaling.7 Oncogenic activation of RET can also occur via chromosomal rearrangements producing hybrid proteins that combines RET kinase domain with another protein containing a dimerization domain.10
The abnormal expression of RET has been implicated in several human disorders including Hirschsprung's disease,11–13 multiple endocrine neoplasias type 2A (MEN2A) and 2B (MEN2B),12,14,15 non-small cell lung cancer (NSCLC) as well as papillary (PTC)12,16 and medullary thyroid cancers (MTC).12,17 Oncogenic RET activity is also observed in numerous cancer types such as prostate,18 colorectal,19 pancreatic,20 breast21 and glioblastomas.22 Therefore, RET RTK is considered as a quintessential therapeutic target for the development of inhibitors directed towards cancerous ailments. The FDA (Food and Drug Administration) and EMA (European Medicines Agency) has granted approval for the multikinase inhibitors – vandetanib and cabozantinib for the treatment of RET-positive MTC. Vandetanib works by blocking the aberrant activity of multiple kinases including RET, VEGFRs and EGFR, while cabozantinib causes the concurrent blockade of VEGFRs, RET, MET and c-KIT.6 Additionally, lenvatinib and sorafenib were also granted FDA approval for differentiated thyroid cancers.23 However, each of these drugs are multikinase inhibitors and are not selective towards RET-driven cancers. Moreover, the use of multikinase inhibitors is linked with mild to heavy toxicities resulting from inhibition of other kinases.24,25 Besides the adverse effects arising from modulation of other target proteins, several inhibitors have demonstrated to be ineffective against mutations in the gate-keeper residues. The mutation in the gate-keeper residue Val804 (V804) of RET, particularly the V804M/L mutations, confers resistance to several inhibitors. Hence, there is a need for identification of novel and selective RET inhibitors targeting the aforementioned mutations. Recently, LOXO-292 (RETEVMO, selpercatinib), developed by Loxo oncology was granted approval by FDA in May, 2020 for the treatment of RET fusion-positive thyroid cancer, RET fusion positive NSCLC and RET-mutant MTC.26 Selpercatinib demonstrated potency against both wild-type (WT) RET as well as RET V804M/L mutations in cellular assays.27 Furthermore, LOXO-292 is highly selective against RET-rearranged tumors.25 Therefore, the development of selective RET inhibitors targeting the gate-keeper mutations is a major research goal.
The above-mentioned objectives prompted us to identify selective RET inhibitors targeted towards the WT and RET gate-keeper mutations, V804M and V804L, similar to selpercatinib. Accordingly, we have performed an in silico investigation via developing a receptor-based pharmacophore model by adopting the RET interaction with a pyrazolopyrimidine inhibitor PP1, as a template structure. The developed model was then employed to virtually screen the natural compounds embedded in the ZINC database. Subsequently, the mapped natural compounds were filtered via Lipinski's, Veber's and pharmacokinetic properties for their drug-likeness. The drug-like natural molecules thereby arising from the above step were subjected to molecular docking with the RET protein kinase domain and the compounds demonstrating higher docking scores than selpercatinib were chosen. The selected natural molecules along with selpercatinib were checked for their stability within the RET kinase domain catalytic pocket for a period of 20 ns via molecular dynamics simulations. Additionally, the compounds were also evaluated on the basis of their binding affinities towards the RET RTK. The compounds exhibiting affinities similar or better to that seen for selpercatinib–RET interaction were further subjected to docking with the RET mutant structures – V804M and V804L. The compounds demonstrating binding affinities with WT as well as mutant structures were thus considered as hits and their interactions within the RET catalytic pocket were observed and reported in our study. The confirmed final hits were regarded as potential RET kinase inhibitors possessing potent scaffolds targeting the WT as well as gate-keeper mutations.
2. Materials and methods
2.1 Generation of receptor–ligand pharmacophore model
The generation of a receptor–ligand (structure-based) pharmacophore model relies on the catalytically active site of a protein and its interaction with the bound co-crystallized ligand.28 The knowledge acquired from the model aids in identification of essential pharmacophoric features required for a protein's inhibition.29 The crystal structure of RET tyrosine kinase complexed with a pyrazolopyrimidine inhibitor, PP1 (1-tert-butyl-3-p-tolyl-1H-pyrazolo[3,4-d]pyrimidin-4-ylamine) (PDB ID: 2IVV) was downloaded from Protein Data Bank (PDB) repository for pharmacophore generation.30 Subsequently, the protein structure was prepared utilizing the Clean Protein module within Discovery Studio (DS) v.2018, following which the Receptor–Ligand Pharmacophore Generation tool was engaged for model generation. By employing the flexible fitting method, the module generates an ideal hypothesis with variable features on the basis of six pre-defined feature collection set (hydrogen bond acceptor (HBA), hydrogen bond donor (HBD), positive ionizable (PI), ring aromatic (RA), negative ionizable (NI) and hydrophobic (Hy)).31 Following feature identification, the model is ranked and estimated on the basis of genetic function approximation (GFA) method and its selectivity score is determined.32 The pharmacophore model generated from the above step was escalated for consequent validation.
2.2 Decoy set validation of generated model
The pharmacophore validation step is carried out to evaluate the capability of the generated model in differentiating between active and decoy compounds. Accordingly, our generated pharmacophore model was assessed by a well-established method known as Güner–Henry (GH) approach, which is also named as decoy set method. A dataset consisting of 330 compounds was prepared which comprised of 24 active RET inhibitors reported in literature. The remaining compounds were considered as inactive for RET or decoys. Following dataset preparation, the Ligand Pharmacophore Mapping module in DS was employed where the decoy set was screened by the generated model. The GH approach thereby generates a goodness of fit (GF) score which ranges between 0 (null model) and 1 (ideal model).28,33,34 The GF score is calculated on the basis of below equation, where D stands for the dataset encompassing active as well as inactive compounds, A represents the active compounds within D, Ht denotes the hit molecules which are retrieved by the generated pharmacophore model and Ha represents the total number of active hit compounds acquired by the model. The validation of the model was also checked via the Receiver Operating Characteristic (ROC) curve and the results were assessed on the basis of the area under the curve (AUC). The value of AUC ranges from 0.0 to 1.0, where AUC value 0 to 0.5 denotes random chance of discrimination, 0.51 to 0.7 represents acceptable value range, 0.71 to 0.8 suggests a good model, and AUC value 0.81 to 1.0 represents an excellent model.
2.3 Virtual screening and drug-likeness filtering for ZINC natural product (ZINC NP) database compounds
The three-dimensional (3D) database of ZINC natural products (NP) was downloaded and prepared in DS, consisting of 144
766 compounds. The Ligand Pharmacophore Mapping module employed for validation of the generated model was also used to screen the natural compounds available in ZINC database. The compounds which mapped the features of our model were retained for further analysis. The mapped compounds acquired from the screening process were filtered by three consecutive filtering criterions incorporating the Lipinski's rule of five (Ro5),35 Veber's36 and ADMET (Absorption, Distribution, Metabolism, Excretion, and Toxicity) rules to obtain drug-like natural molecules. The Veber's and Ro5 rules collectively suggest that a potential drug-like molecule should demonstrate a total of ≤10 rotatable bonds, lipophilicity (log
P) ≤5, molecular weight of ≤500 kDa, hydrogen bond donor groups ≤5 and hydrogen bond acceptor groups ≤10. The above filtering steps were carried out by the Filter by Lipinski and Veber Rules module within DS. An additional filtering was also done by the ADMET Descriptors tool in DS which oversees that the potential drug-like compound will/will not cross blood–brain barrier and does not demonstrate CYP2D6 inhibition and hepatotoxicity. Additionally, the compounds should demonstrate good intestinal absorption and solubility. Thus, these acquired drug-like natural molecules which mapped the features of our generated pharmacophore model were taken forward for molecular docking.
2.4 Molecular docking of drug-like natural products with RET tyrosine kinase
The process of molecular docking is performed to decipher the bioactive binding poses and essential interaction of a given drug-like molecule within the catalytic pocket of a macromolecular protein target. The drug-like natural compounds assimilated from the above-mentioned virtual screening process were employed to dock with the RET kinase domain catalytic pocket (PDB ID: 2IVV). The GOLD (Genetic Optimisation for Ligand Docking) automated docking software with its default scoring system (GoldScore and ChemScore) was used for our docking study.37,38 The fitness scoring function, GoldScore, is a force field based scoring function, operating on four components including protein–ligand hydrogen bond energy, protein–ligand van der Waals energy, ligand internal van der Waals energy, and ligand intramolecular hydrogen bonding energy. The ChemScore is an empirical scoring function and estimates the free energy of ligand binding to macromolecular protein target. It incorporates simple contact terms for estimating the free energy change, using lipophilic, metal–ligand binding contributions, ligand flexibility, and hydrogen bonding interactions. Accordingly, the crystal structure downloaded from PDB was prepared and the binding site was defined as a sphere of 8 Å using the Define and Edit Binding Site module in DS. In order to check the reliability of our docking software, the co-crystallized ligand (PP1) in PDB ID: 2IVV was re-docked by providing the X, Y, and Z co-ordinates as 25.08, 7.02, and 9.83, respectively. Following this reliability check, the drug-like natural compounds were prepared with the Minimize Ligands module in DS and consequently employed for docking. The docking of drug-like natural molecules with the RET tyrosine kinase domain was carried out with the same parameters as that employed for docking of PP1. A total of 10 conformers were generated per compound and the RET kinase inhibitors – vandetanib and selpercatinib were considered as reference inhibitors. The compounds which demonstrated higher GoldScores and lower ChemScores than reference inhibitors were considered for further analysis and their molecular interactions within the RET kinase domain pocket were evaluated. The interactions of obtained compounds were assessed with residues Leu730, Gly731, Val738, Ala756, Lys758, Ile788, Leu802, Ile803, Val804, Glu805, Tyr806, Ala807, Gly810, Leu881, Ser891, and Asp892 enclosing the binding pocket of RET kinase domain. The chosen compounds which demonstrated better dock scores than reference inhibitors and key interactions with RET binding pocket residues were refined under physiological conditions in the next step.
2.5 Molecular dynamics simulation of RET–ligand docking interactions
The major limitation of molecular docking studies is that they fail to consider the dynamics of protein–ligand interaction in real-time. Additionally, the full consideration of target flexibility is lacking, leading to miss some molecules active for a specific target.39 As compared to docking, molecular dynamics (MD) simulations consider the flexibility of protein targets in real-time and are being widely used to refine the results obtained from docking studies.40,41 In addition, simulation studies aid in understanding the interactions of a particular ligand within the binding pocket at the atomic level. The complexes of ligands with RET, acquired from the above docking process were regarded as initial structures for MD simulations in GROningen MAchine for Chemical Simulations (GROMACS) v2018.42 The RET protein structure was parametrized by CHARMm27 forcefield,43 while the parameters for docked ligands were obtained from SwissParam tool.44 A three-point solvent model (TIP3P) was used to immerse all simulation systems and neutralized by addition of chloride ions. Prior to equilibrating all systems, energy minimization by steepest descent algorithm was carried out for 50
000 steps.45 Following energy minimization, equilibration in NVT (constant number of particles, volume, and temperature) ensemble and NPT (constant number of particles, pressure, and temperature) ensemble was performed, for 1000 ps each. The NVT ensemble equilibrates the system using a V-rescale thermostat,46 while maintaining the system temperature at 300 K. The NPT ensemble maintains the system pressure at 1.0 bar by using the Parrinello–Rahman barostat.47 After equilibration by NVT and NPT ensembles, MD simulation of 20 ns was performed for all systems. The PME (Particle Mesh Ewald) method handles the long-range electrostatic interactions,48 while the bond constrains are monitored by LINCS (LINear Constraint Solver) algorithm.49 The VMD (visual molecular dynamics) molecular graphics program was utilized for visualizing simulation results.50 The MD results for all systems were plotted in terms of trajectory plots and interaction of ligands with RET kinase domain was visualized in DS.
2.6 Binding free energy calculations of RET–ligand interactions
The molecular docking approach predicts the favorable binding disposition of a particular ligand within the protein's catalytic pocket and is considered as gold standard in virtual screening studies for identification of novel hits. However, docking studies apply simple scoring functions for estimating the protein–ligand binding affinities, thus leading to varying results.51 Therefore, a more profitable method for carrying out reliable estimation of protein–ligand binding affinities is commonly used for evaluating the MD trajectories. The popular approach, namely, molecular mechanics Poisson–Boltzmann surface area (MM/PBSA) combines the molecular mechanics energies with surface area continuum solvation and computes the free energy of binding of ligands with macromolecular protein targets.52,53 The MM/PBSA binding affinity calculations were used in previous studies with varying success to rationalize/reproduce experimental findings and also improve the results obtained from virtual screening/docking strategies.52 Thus, the g_mmpbsa plugin tool embedded within GROMACS was utilized to compute the binding affinities of compounds with RET kinase.54 A total of 50 frames for RET–ligand MD complexes were selected evenly for entire 20 ns and free energy ΔGbind was computed according to the below equation. The term Gcomplex represents the total free energy of protein–ligand complex, while Gprotein and Gligand denotes the free energies of individual components in the solvent when unbound.
ΔGbind = Gcomplex − (Gprotein + Gligand) |
2.7 Molecular docking, MD simulations, and binding affinity evaluation of natural products with RET mutant protein structures
The Val804 in the RET kinase domain confers resistance to the established RET inhibitors, PP1 and vandetanib.55 In recent years, a new generation of selective RET inhibitors were identified and selpercatinib was approved by US FDA in 2020.25,26 Selpercatinib is effective for WT as well as for gate-keeper resistance Val804 mutations (V804M/L).56 With the objective of identifying similar compounds as selpercatinib which demonstrate effectiveness for WT and gate-keeper mutations, the hits acquired from MD simulation were subjected to molecular docking with the gatekeeper mutant structures. Accordingly, the Val804 residue was modelled via the Build and Edit Protein tool to methionine and leucine for formation of V804M and V804L mutant structures. Molecular docking and dynamics simulation of hits was performed with the build mutants and the generated trajectories were evaluated via MM/PBSA analysis. The hits which showed similar or better binding free energies than selpercatinib were retained and regarded as being effective towards WT and gate-keeper mutations.
3. Results and discussion
Numerous computational techniques were applied for the identification of potential RET tyrosine kinase inhibitors, the schematic overview of our in silico investigation is summarized (Fig. 1).
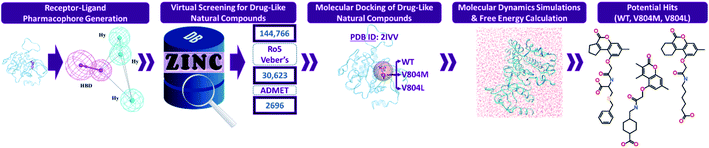 |
| Fig. 1 The workflow followed in the present in silico investigation for identification of potential RET inhibitors. | |
3.1 Receptor–ligand (structure-based) pharmacophore model
A receptor–ligand pharmacophore model was generated from the crystallographic structure of RET tyrosine kinase domain complexed with a pyrazolopyrimidine inhibitor, PP1 (PDB ID: 2IVV). The model explored the key features of PP1 binding with RET, which resulted in four pharmacophoric features, resulting in one HBD and three Hy features with the selectivity score of 6.9375 (Table 1 and Fig. 2). The pharmacophore model indicated that PP1 interacts with Glu805 and Ala807 via its HBD feature, thereby forming hydrogen bonds. PP1 also interacts with various surrounding residues including Leu730, Val738, Ala756, Lys758, Val804, and Leu881 via the three generated Hy features, thus forming hydrophobic bonds (Fig. 2B). Previous literature by Kuei-Chung Shih et al. also reported four features (HBA, Hy, HBA, and RA) essential for RET inhibition, developed via ligand-based pharmacophore modeling.57 Therefore, the generated model was taken forward to the next step of validation, to check its reliability.
Table 1 Structure-based pharmacophore model summary with its generated features
Pharmacophore model |
Number of features |
Feature seta |
Selectivity score |
HBD: hydrogen bond donor; Hy: hydrophobic. |
Pharmacophore_01 |
4 |
HBD, Hy, Hy, Hy |
6.9375 |
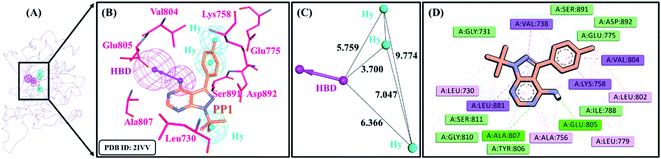 |
| Fig. 2 Receptor–ligand pharmacophore model. (A) Pharmacophore model generated at the catalytic site of RET tyrosine kinase with co-crystallized ligand, PP1 (PDB ID: 2IVV). (B) RET inhibitor, PP1 mapping with essential residues of RET tyrosine kinase via key pharmacophoric features – HBD and Hy. (C) Interfeature distance between the features of the pharmacophore. HBD (hydrogen bond donor) and Hy (hydrophobic), and (D) interactions of PP1 with key residues of the RET binding pocket. | |
3.2 Decoy set model validation
The pharmacophore model generated in the above step was validated by a well-known method known as decoy set method. In this method, an external dataset is screened, consisting of active and decoy compounds, resulting in GF score ranging from 0 to 1. Accordingly, from a dataset (D) of 330 compounds, 34 hits (Ht) were retrieved. Out of the total hits acquired, 24 (Ha) inhibitors active for RET kinase, were obtained. The GF score was thus calculated as 0.75, which is near the range value for an ideal model (Table 2). From the ROC curve analysis, it was observed that the model revealed reasonable quality with AUC value of 0.804 and was demonstrated to be a good model (Fig. S2†). This confirmed the robustness of our model in predicting active RET compounds from an external database.
Table 2 Decoy set validation of generated pharmacophore model with an external dataset composed of active RET inhibitors and decoy set molecules
Sr. no. |
Parameters |
Values |
1 |
Total number of compounds in the external dataset (D) |
330 |
2 |
Total number of active compounds in the dataset (A) |
24 |
3 |
Total number of hits retrieved by the model from the dataset (Ht) |
34 |
4 |
Total number of active compounds in the hits retrieved (Ha) |
24 |
5 |
% yield of active ((Ha/Ht) × 100) |
70.58 |
6 |
% ratio of actives ((Ha/A) × 100) |
100 |
7 |
False negatives (A − Ha) |
0 |
8 |
False positives (Ht − Ha) |
10 |
9 |
Goodness of fit score (GF) |
0.75 |
3.3 Drug-like natural compounds derived by virtual screening
After validation, the model was considered for virtual screening of ZINC NP database comprising 144
766 compounds of natural origin. Mapping of ZINC database resulted in 58
538 compounds which demonstrated the four features of our pharmacophore model. These large number was further reduced by applying three filtering rules as mentioned above. The application of Lipinski's Ro5 and Veber's filter further decreased the number to a total of 30
623 compounds. Subsequent application of ADMET filter resulted in 2696 natural compounds. These 2696 natural products were escalated for docking with the RET tyrosine kinase domain (Fig. 1).
3.4 Molecular docking of retrieved natural compounds with RET tyrosine kinase domain
The drug-like natural (2696) compounds attained from the virtual screening step were subjected to molecular docking with the crystallographic structure of RET tyrosine kinase domain (PDB ID: 2IVV). It was reported by Pietra et al. that the PP1 inhibitor in PDB ID: 2IVV enters a small cavity with its methyl-phenyl moiety, thereby imposing the long Lys758 side chain to shift its orientation.58 Thus, 2IVV is able to allocate larger inhibitors like vandetanib and selpercatinib. Therefore, the aforementioned crystallographic structure was taken into consideration for our docking analysis. The robustness of GOLD in re-docking the co-crystallized PP1 was evaluated, resulting in root mean square deviation (RMSD) of 1.07 Å. Moreover, the docked pose of PP1 overlaid perfectly with its crystal structure conformation (Fig. S1†). As the RMSD was observed to be in an acceptable range (<2 Å), the minimized structures of 2696 ligands were further docked. In our docking analysis, vandetanib and selpercatinib were regarded as reference inhibitors and compounds demonstrating better dock scores than them were selected. Vandetanib demonstrated a GoldScore of 55.87 and a ChemScore of −28.27, while selpercatinib displayed a GoldScore of 57.56 and a ChemScore of −28.58. Using these scores as cut-off, a total of 39 natural compounds showed better dock scores. The 39 natural molecules were further scrutinized on the basis of their molecular interactions with the catalytic residues in RET kinase domain. Thus, an aggregate of 27 compounds from ZINC NP database displayed better docking scores as well as favorable molecular interactions with the residues contained within RET binding pocket (Table S1†). The 27 compounds complexed with RET were assessed via MD simulations.
3.5 Molecular dynamics simulation and free energy analysis
The 27 docked complexes chosen from docking analysis along with the two complexes of reference inhibitors with RET were taken as initial co-ordinates for MD simulations. In total, 29 systems were subjected to a production run of 20 ns each. Following simulations, the binding affinity of each compound towards RET was evaluated in terms of binding free energy (BFE) values (Table S1†). The MM/PBSA calculation revealed a BFE value of −115.97 kJ mol−1 for vandetanib, while selpercatinib demonstrated a BFE value of −95.76 kJ mol−1. The BFE values of reference inhibitors were taken as cut-off and a total of 12 ZINC NPs out of 27 NPs exhibited better BFE values (Table 3). Hence, their stability was assessed for the entire simulation period.
Table 3 The distribution of total binding free energy scores for reference inhibitors and potential natural products (NPs) from ZINC NP database with wild-type (WT) RET tyrosine kinase
Compounds (ZINC ID) |
van der Waals (kJ mol−1) |
Electrostatic (kJ mol−1) |
Polar solvation (kJ mol−1) |
SASA energy (kJ mol−1) |
Binding energy ΔGbind (kJ mol−1) |
NP1 (ZINC85879094) |
−176.08 ± 11.448 |
−244.29 ± 38.875 |
227.95 ± 60.658 |
−19.96 ± 0.992 |
−212.39 ± 30.783 |
NP2 (ZINC02113839) |
−170.07 ± 17.995 |
−391.46 ± 22.996 |
385.77 ± 26.354 |
−21.78 ± 1.016 |
−197.55 ± 21.070 |
NP3 (ZINC04030012) |
−179.09 ± 16.288 |
−384.79 ± 30.802 |
396.57 ± 28.457 |
−22.38 ± 0.825 |
−189.68 ± 23.350 |
NP4 (ZINC08764543) |
−179.57 ± 13.056 |
−222.18 ± 20.335 |
235.07 ± 20.435 |
−19.26 ± 0.802 |
−185.95 ± 20.154 |
NP5 (ZINC72325379) |
−123.55 ± 12.977 |
−206.21 ± 26.680 |
163.98 ± 35.327 |
−16.22 ± 1.218 |
−182.00 ± 33.852 |
NP6 (ZINC12885019) |
−176.32 ± 13.027 |
−277.69 ± 27.172 |
306.81 ± 29.105 |
−21.13 ± 1.284 |
−168.33 ± 28.872 |
NP7 (ZINC02123418) |
−204.19 ± 20.333 |
−319.22 ± 17.691 |
390.01 ± 18.150 |
−24.00 ± 1.304 |
−157.41 ± 20.002 |
NP8 (ZINC02125740) |
−181.45 ± 16.820 |
−258.52 ± 42.232 |
305.56 ± 52.888 |
−21.55 ± 1.367 |
−155.95 ± 33.849 |
NP9 (ZINC98364168) |
−130.86 ± 19.920 |
−414.19 ± 59.594 |
408.01 ± 29.077 |
−17.47 ± 0.857 |
−154.52 ± 36.901 |
NP10 (ZINC02121773) |
−161.41 ± 18.329 |
−318.35 ± 48.122 |
366.09 ± 41.275 |
−20.94 ± 0.932 |
−134.62 ± 27.939 |
NP11 (ZINC04030018) |
−165.05 ± 14.246 |
−273.40 ± 25.813 |
330.53 ± 32.449 |
−21.10 ± 1.228 |
−129.02 ± 20.563 |
NP12 (ZINC02112951) |
−165.94 ± 21.037 |
−330.54 ± 28.769 |
392.17 ± 25.582 |
−21.26 ± 1.052 |
−125.58 ± 33.252 |
Vandetanib |
−202.52 ± 9.323 |
−43.11 ± 8.860 |
149.45 ± 14.798 |
−19.79 ± 0.820 |
−115.97 ± 17.341 |
Selpercatinib |
−259.28 ± 12.103 |
−51.79 ± 12.308 |
240.69 ± 28.916 |
−25.38 ± 1.125 |
−95.76 ± 21.511 |
3.6 Affinity analysis of NPs with the gate-keeper mutations (V804M/L) in RET kinase domain via molecular docking and dynamics simulations
The 12 NPs from ZINC database were also employed to dock with the mutated kinase domains. Moreover, their BFE values towards the gate-keeper mutations were further computed by MM/PBSA methodology. The calculation revealed a BFE value of −82.01 kJ mol−1 for vandetanib and −103.53 kJ mol−1 for selpercatinib towards the V804M gate-keeper mutant. Accordingly, 6 NPs from the above 12 NPs demonstrated better docking scores as well as BFE values than reference inhibitors (Tables S2† and 4). The MM/PBSA analysis revealed a BFE value of −93.36 kJ mol−1 for vandetanib and −105.83 kJ mol−1 for selpercatinib towards the V804L gate-keeper mutant protein. Accordingly, 3 NPs from the above 12 NPs exhibited better docking scores as well as BFE values than reference inhibitors (Tables S3† and 5). Thus, a total of 3 NPs were considered as hits inhibiting the WT as well as gate-keeper mutations – V804M and V804L (Table 5).
Table 4 The distribution of total binding free energy scores for reference inhibitors and potential natural products (NPs) from ZINC NP database with RET gate-keeper mutant (V804M) structure
Compounds (ZINC ID) |
van der Waals (kJ mol−1) |
Electrostatic (kJ mol−1) |
Polar solvation (kJ mol−1) |
SASA energy (kJ mol−1) |
Binding energy ΔGbind (kJ mol−1) |
NP7 (ZINC02123418) |
−164.02 ± 28.023 |
−205.75 ± 25.985 |
155.52 ± 48.195 |
−18.19 ± 2.448 |
−232.44 ± 31.621 |
NP11 (ZINC04030018) |
−176.69 ± 15.573 |
−205.64 ± 25.947 |
173.60 ± 48.316 |
−19.65 ± 1.865 |
−228.39 ± 29.790 |
NP3 (ZINC04030012) |
−172.86 ± 12.306 |
−295.81 ± 47.410 |
268.00 ± 65.907 |
−21.49 ± 1.279 |
−222.16 ± 48.294 |
NP6 (ZINC12885019) |
−144.38 ± 18.061 |
−284.10 ± 56.409 |
257.10 ± 53.858 |
−18.94 ± 1.496 |
−190.33 ± 32.931 |
NP2 (ZINC02113839) |
−189.69 ± 17.155 |
−392.85 ± 42.492 |
427.15 ± 42.676 |
−22.28 ± 0.653 |
−177.67 ± 25.168 |
NP10 (ZINC02121773) |
−173.72 ± 13.818 |
−204.05 ± 37.850 |
228.05 ± 53.142 |
−20.46 ± 1.170 |
−170.17 ± 31.811 |
Vandetanib |
−173.02 ± 26.439 |
−6.61 ± 8.749 |
116.97 ± 26.423 |
−19.34 ± 2.967 |
−82.01 ± 17.181 |
Selpercatinib |
−265.56 ± 10.514 |
−40.55 ± 10.291 |
228.01 ± 25.624 |
−25.44 ± 0.955 |
−103.53 ± 28.664 |
Table 5 The distribution of total binding free energy scores for reference inhibitors and potential hits from ZINC natural product (NP) database with RET gate-keeper mutant (V804L) structure
Compounds/hits (ZINC ID) |
van der Waals (kJ mol−1) |
Electrostatic (kJ mol−1) |
Polar solvation (kJ mol−1) |
SASA energy (kJ mol−1) |
Binding energy ΔGbind (kJ mol−1) |
NP7/Hit1 (ZINC02123418) |
−201.80 ± 12.964 |
−211.89 ± 30.665 |
243.09 ± 47.563 |
−23.71 ± 1.356 |
−194.31 ± 32.373 |
NP2/Hit2 (ZINC02113839) |
−166.66 ± 14.315 |
−211.89 ± 27.958 |
225.66 ± 43.924 |
−19.08 ± 1.013 |
−171.98 ± 27.358 |
NP3/Hit3 (ZINC04030012) |
−175.59 ± 11.659 |
−307.58 ± 34.392 |
358.97 ± 32.033 |
−22.20 ± 0.841 |
−146.40 ± 19.385 |
Vandetanib |
−168.00 ± 20.959 |
−17.96 ± 16.256 |
111.07 ± 41.212 |
−18.46 ± 2.340 |
−93.36 ± 22.040 |
Selpercatinib |
−258.30 ± 11.203 |
−37.23 ± 12.001 |
214.54 ± 31.239 |
−24.83 ± 0.895 |
−105.83 ± 25.589 |
3.7 Stability and interaction analysis of hits with wild-type (WT) and mutated RET kinase domains
The backbone RMSD of complexes was used as the criteria to assess the stability of all systems for the entire simulation period of 20 ns. The simulated systems (WT, V804M, and V804L) demonstrated steady-state stability for complete production run (Fig. S3–S5†). The average RMSD for the WT systems was observed to be in the range of 0.25 to 0.34 nm, while the average for both mutant systems was in the range of 0.28 to 0.36 nm (Table S4†). Therefore, the representative structure was extracted from the last 1 ns of stable MD trajectories in order to comprehend the binding mode of all NPs with WT and mutated RET kinase domains.
The hydrogen bonds from the equilibrium trajectory of vandetanib's and selpercatinib's interaction with WT and mutated RET kinase domain was analyzed. It was observed that vandetanib and selpercatinib displayed hydrogen bonding interaction with key residue Ala807 of the kinase domain. The mutation in residue Val804 disrupts the hydrogen bonding of vandetanib and Ala807, as a result, vandetanib forms hydrogen bonds with other residues of the domain. The V804M and V804L mutation does not disrupt the hydrogen bonding of selpercatinib and Ala807, thus suggesting a strong interaction. This signifies that the mutation in the gate-keeper residue does not lead to steric hindrance between selpercatinib and RET protein. Similarly, the NPs also displayed interactions with residues of RET kinase domain including Leu730, Glu732, Glu734, Phe735, Gly736, Lys758, Tyr806, Ala807, Ser811, Arg878, Asn879, Lys889, Ser891, Asp892, Arg912 via hydrogen bonds (Fig. 3–5). Interactions with the aforementioned residues were also reported in previous studies.58–62 Furthermore, hydrophobic and van der Waals interactions were observed with various residues of RET kinase domain, as seen from their 2D interaction plots (Fig. S6A, B and S7A–L†).
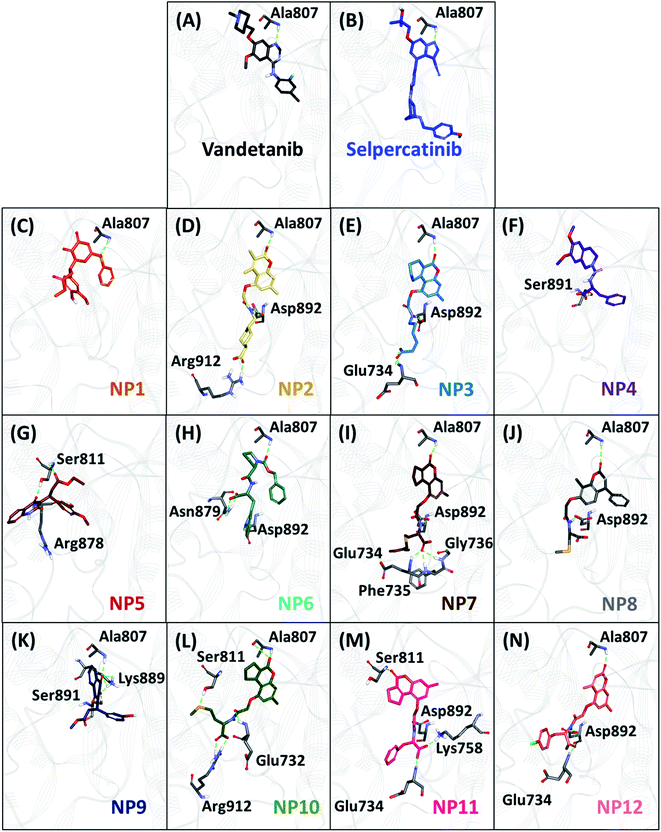 |
| Fig. 3 The binding mode of reference inhibitors (vandetanib and selpercatinib) and 12 acquired natural products (NPs) with wild-type (WT) RET kinase domain and molecular interactions with key residues. Hydrogen bonds are shown as green dashed lines. | |
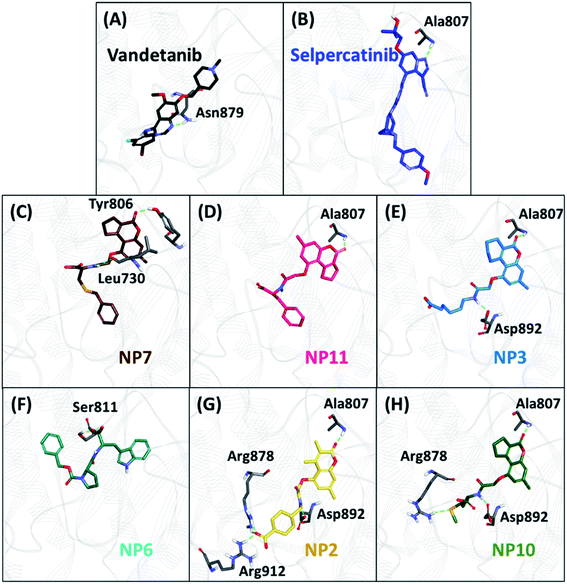 |
| Fig. 4 The binding mode of 6 acquired natural products (NPs) with gate-keeper (V804M) mutant RET kinase domain and molecular interactions with key residues. Hydrogen bonds are shown as green dashed lines. | |
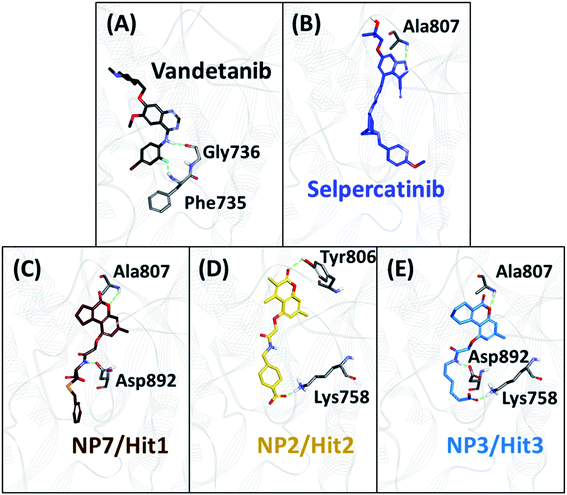 |
| Fig. 5 The binding mode of 3 acquired hits with gate-keeper (V804L) mutant RET kinase domain and molecular interactions with key residues. Hydrogen bonds are shown as green dashed lines. | |
As perceived from 2D molecular interactions, our MD analysis confirmed that vandetanib was not observed to form any interaction with Met804 and Leu804 residue (Fig. S6C and E†). This analysis was consistent with previously published study by George Priya Doss et al.59 On the other hand, selpercatinib demonstrates van der Waals interaction with Met804 and Leu804 residues (Fig. S6D and F†). The molecular interactions of NPs with mutated kinase domains suggested no steric clashes with the Val804 residue when mutated to methionine (Fig. S8†) or leucine (Fig. S9†). Instead, interactions of our acquired NPs with Met804 and Leu804 residues were observed via van der Waals or hydrophobic π-bonds (Fig. S8 and S9†). From the above-mentioned scrupulous analysis, 3 hits were observed to demonstrate better docking scores as well as BFE values than the reference inhibitors for WT and mutated RET proteins (Tables S1–S3†). Hence, ZINC02123418 (Hit1), ZINC02113839 (Hit2), and ZINC04030012 (Hit3) were considered as the most potent compounds capable of inhibiting the WT as well as the RET gate-keeper mutant forms.
A comparison of their backbone root mean square fluctuation (RMSF) was further performed for assessing the flexibility of RET WT and mutated forms. Accordingly, the backbone RMSF was measured for reference inhibitors and 3 hits obtained from the above analyses. Previous RMSF study by George Priya Doss et al. suggested that V804M mutation affects the binding of vandetanib with RET and thus makes the backbone more flexible.59 Similar RMSF pattern was observed in our study for vandetanib. When Val804 is mutated to methionine or leucine, the residue backbone adopts a higher fluctuation as compared to WT for vandetanib (Fig. 6A). On the other hand, the residue backbone for selpercatinib does not adopt fluctuation as compared to WT, during the course of the simulation (Fig. 6B). This report suggests that V804M/L mutation does not affect the binding of selpercatinib with RET. As seen from the RMSF plots of 3 hits, the RMSF pattern of Hit2 was observed to similar to the pattern of vandetanib, thus showing larger fluctuations (Fig. 6D). This suggests that even though Hit2 demonstrates significant BFE towards RET WT and mutated forms, its binding is affected by the Val804 mutation. However, the RMSF pattern for Hit1 and Hit3 was observed to be similar to that seen for selpercatinib (Fig. 6C and E). Thus, it can be postulated that the V804M/L mutation does not affect the binding of Hit1 and Hit3. Therefore, from the above overall analyses, we anticipate that our hits can be deemed fit for RET WT and gate-keeper mutant inhibition.
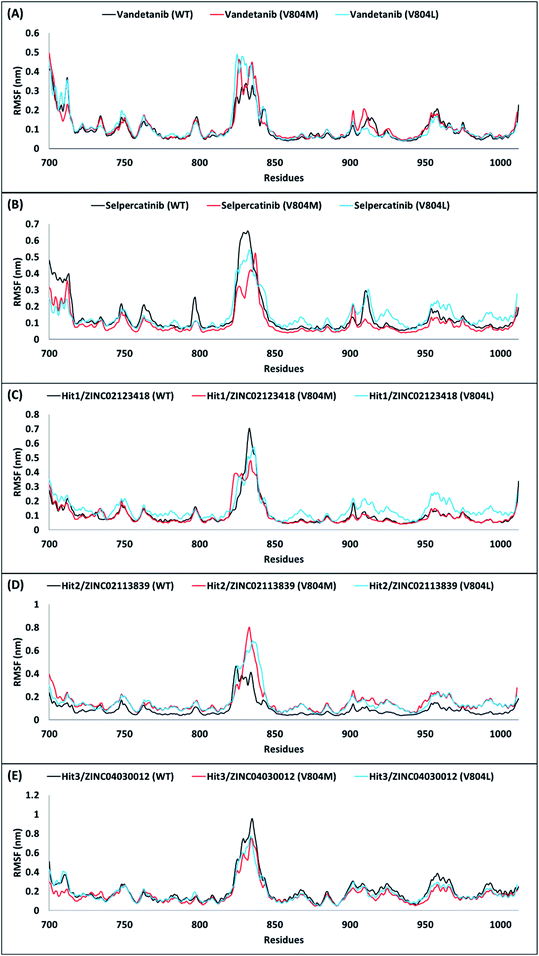 |
| Fig. 6 Backbone RMSFs are shown as a function of time for wild-type (WT), V804M, and V804L RET protein with the reference inhibitors, (A) vandetanib, (B) selpercatinib, and acquired hits, (C) Hit1, (D) Hit2, and (E) Hit3. | |
The BFE calculations via MM/PBSA enables the decomposition of ΔGbind values into identifiable contributions.52 As perceived from the free energy analysis, the van der Waals interaction provided the highest driving force for the binding of vandetanib and selpercatinib (Tables 3–5). On the other hand, the driving force for the binding of hits was dominated by the electrostatic as well as the van der Waals interaction contributing to their total BFE values (Tables 3–5). Furthermore, the individual residues contributing to the total free energy of binding for reference inhibitors and hits were probed. It was observed that residues Val738, Lys758, Arg878, and Asn879 contributed to the total BFE of vandetanib, while residues Val738 and Leu881 dominated the total BFE for selpercatinib (Fig. 7). Additionally, the highest entropic contribution for binding of hits was provided by Lys758 and Arg878 along with other residues of the binding pocket (Fig. 8).
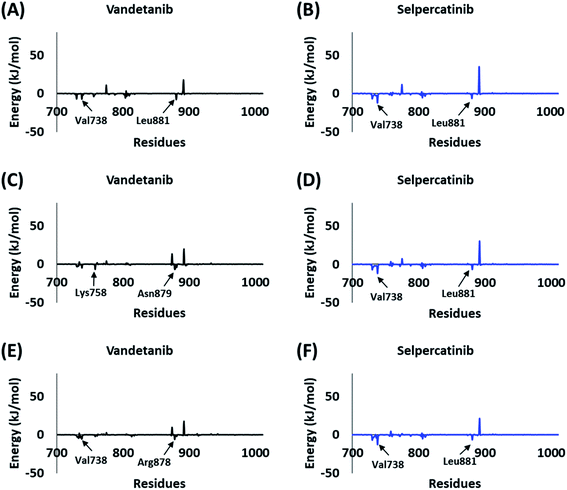 |
| Fig. 7 Energy decomposition of individual residues via MM/PBSA contributing to the total binding free energy of reference inhibitors – vandetanib (A, C, and E) and selpercatinib (B, D, and F) with wild-type (WT), V804M, and V804L RET protein structures. | |
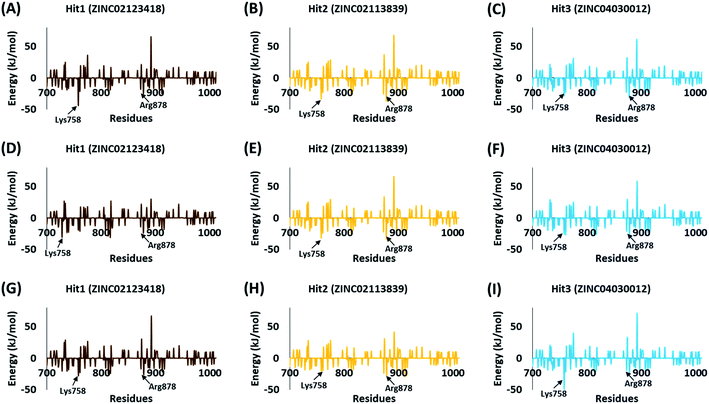 |
| Fig. 8 Energy decomposition of individual residues via MM/PBSA contributing to the total binding free energy of 3 hits – Hit1 (A, D, and G), Hit2 (B, E, and H), and Hit3 (C, F, and I) with wild-type (WT), V804M, and V804L RET protein structures. | |
The 3 identified hits were searched in the PubChem chemistry database (accessed on 10th September 2021, https://pubchem.ncbi.nlm.nih.gov/)63 to check if their activity is reported in the literature for RET RTK inhibition. Consequently, their SMILES (simplified molecular-input line-entry system) IDs were entered in the PubChem search bar. The search analysis suggested that the activity of our acquired hits has not been assessed for RET inhibition. Furthermore, BIOVIA Draw 2020 was used to generate the 2D structures of reference inhibitors and our obtained hits. The chemical structures along with their IUPAC names were presented as below (Table 6). The reference inhibitor, vandetanib is an anilinoquinazoline, while selpercatinib is a carbonitrile. Compared to the reference inhibitors, our obtained hits are propanoic, carboxylic, and hexanoic acids. The inhibitors of aforementioned origins have not been reported in literature for RET inhibition till date and also represent the features of the generated pharmacophore model (Fig. S10†). Thus, we envision that these hits maybe effective drug candidates against RET and can be recommended as potent therapeutics for WT as well as gate-keeper RET mutant forms.
Table 6 The chemical structures and IUPAC names of reference inhibitors (vandetanib and selpercatinib) and acquired hits from ZINC natural product database
Compound name |
IUPAC name |
Molecular structure |
Reference inhibitors |
Vandetanib |
N-(4-Bromo-2-fluorophenyl)-6-methoxy-7-[(1-methylpiperidin-4-yl)methoxy]quinazolin-4-amine |
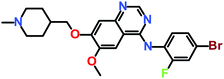 |
Selpercatinib |
6-(2-Hydroxy-2-methylpropoxy)-4-[6-[6-[(6-methoxypyridin-3-yl)methyl]-3,6-diazabicyclo[3.1.1]heptan-3-yl]pyridin-3-yl]pyrazolo[1,5-a]pyridine-3-carbonitrile |
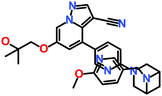 |
![[thin space (1/6-em)]](https://www.rsc.org/images/entities/char_2009.gif) |
ZINC natural product database hits |
Hit1 (ZINC02123418) |
3-Benzylsulfanyl-2-[[2-[(7-methyl-4-oxo-2,3-dihydro-1H-cyclopenta[c]chromen-9-yl)oxy]acetyl]amino]propanoic acid |
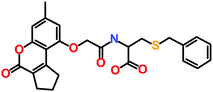 |
Hit2 (ZINC02113839) |
4-[[[2-(3,4,7-Trimethyl-2-oxochromen-5-yl)oxyacetyl]amino]methyl]cyclohexane-1-carboxylic acid |
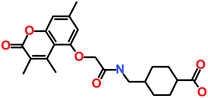 |
Hit3 (ZINC04030012) |
6-[[2-[(3-Methyl-6-oxo-7,8,9,10-tetrahydrobenzo[c]chromen-1-yl)oxy]acetyl]amino]hexanoic acid |
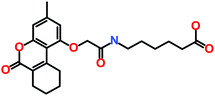 |
4. Conclusion
A receptor–ligand pharmacophore model exploiting the crystal structure of RET tyrosine kinase with its co-crystallized inhibitor, PP1, was generated and employed for virtual screening of ZINC natural products (NPs) database. The obtained NPs were probed for their drug-likeness properties and the acquired drug-like NPs were subjected to molecular docking with RET wild-type (WT) kinase domain. A total of 27 NPs demonstrated better docking scores than the reference inhibitors, vandetanib and selpercatinib. Subsequent molecular simulations in combination with binding free energy (BFE) calculations of 27 NPs resulted in 12 NPs exhibiting better BFE values than reference inhibitors. The docking, simulations, and BFE computation of 12 NPs with RET gate-keeper residue (V804) mutant forms was further performed, resulting in 3 NPs indicating significantly better dock scores and BFE scores than reference inhibitors. Thus, the 3 NPs were deemed as hits for WT, V804M, and V804L RET protein structures. The obtained hits did not demonstrate steric hindrance with the mutated gate-keeper residue. Moreover, the highest contribution for the total BFE of hits with RET WT and mutated forms was provided by residues – Lys758 and Arg878. Additionally, the scaffolds of hit molecules have not been reported hitherto for RET inhibition and hence, can be considered as novel therapeutics for developing effective RET inhibitors, specifically targeting the gate-keeper mutations.
Author contributions
Conceptualization, S. P.; methodology, S. P.; software, S. P.; validation, S. P.; formal analysis, S. P. and V. K.; investigation, S. P.; resources, K. W. L.; data curation, S. P.; writing—original draft preparation, S. P.; writing—review and editing, S. P.; visualization, S. P.; supervision, K. W. L. and J. C. H.; project administration, K. W. L. and J. C. H.; funding acquisition, J. C. H. All authors have read and agreed to the published version of the manuscript.
Conflicts of interest
The authors declare no conflict of interest.
Acknowledgements
This research was supported by Basic Science Research Program through the National Research Foundation of Korea (NRF) funded by the Ministry of Education (2020R1A6A1A03044344).
References
- M. Takahashi, J. Ritz and G. M. Cooper, Cell, 1985, 42, 581–588 CrossRef CAS.
- C. C. Jia, W. Chen, Z. L. Feng and Z. P. Liu, Future Med. Chem., 2021, 13, 45–62 CrossRef CAS PubMed.
- Y. Ishizaka, F. Itoh, T. Tahira, I. Ikeda, T. Sugimura, J. Tucker, A. Fertitta, A. V. Carrano and M. Nagao, Oncogene, 1989, 4(12), 1519–1521 CAS.
- C. F. Ibáñez, Cold Spring Harbor Perspect. Biol., 2013, 5(2), a009134 Search PubMed.
- P. Durbec, C. V. Marcos.-Gutierrez, C. Kilkenny, M. Grigoriou, K. Wartiowaara, P. Suvanto, D. Smith, B. Ponder, F. Costantini and M. Saarma, Nature, 1996, 381(6585), 789–793 CrossRef CAS PubMed.
- A. Drilon, Z. I. Hu, G. G. Y. Lai and D. S. W. Tan, Nat. Rev. Clin. Oncol., 2018, 15(3), 151–167 CrossRef CAS PubMed.
- I. Plaza-Menacho, L. Mologni and N. Q. McDonald, Cell. Signalling, 2014, 26, 1743–1752 CrossRef CAS.
- A. Pelet, O. Geneste, P. Edery, A. Pasini, S. Chappuis, T. Atti, A. Munnich, G. Lenoir, S. Lyonnet and M. Billaud, J. Clin. Invest., 1998, 101, 1415 CrossRef CAS PubMed.
- S. Kjær and C. F. Ibáñez, Hum. Mol. Genet., 2003, 12, 2133–2144 CrossRef PubMed.
- A. K. Mahato and Y. A. Sidorova, Int. J. Mol. Sci., 2020, 21, 1–21 Search PubMed.
- B. D. Hyndman, T. S. Gujral, J. R. Krieger, J. G. Cockburn and L. M. Mulligan, Hum. Mutat., 2013, 34(1), 132–142 CrossRef CAS PubMed.
- A. Y. Li, M. G. McCusker, A. Russo, K. A. Scilla, A. Gittens, K. Arensmeyer, R. Mehra, V. Adamo and C. Rolfo, Cancer Treat. Rev., 2019, 81, 101911 CrossRef.
- N. Nagy, R. A. Guyer, R. Hotta, D. Zhang, D. F. Newgreen, V. Halasy, T. Kovacs and A. M. Goldstein, Development, 2020, 147(21), dev190900 CrossRef.
- G. Martucciello, M. Lerone, L. Bricco, G. P. Tonini, L. Lombardi, C. G. D. Rossi and S. Bernasconi, Ital. J. Pediatr., 2012, 38, 9 CrossRef CAS PubMed.
- I. Plaza.-Menacho, Endocr.-Relat. Cancer, 2018, 25, T79–T90 CAS.
- A. Krishnan, J. Berthelet, E. Renaud, S. Rosigkeit, U. Distler, E. Stawiski, J. Wang, Z. Modrusan, M. Fiedler, M. Bienz, S. Tenzer, A. Schad, W. Roth, B. Thiede, S. Seshagiri, T. J. Musholt and K. Rajalingam, Nat. Commun., 2020, 11, 2056 CrossRef CAS PubMed.
- V. Larouche, A. Akirov, C. M. Thomas, M. K. Krzyzanowska and S. Ezzat, Curr. Oncol., 2019, 26, 389–394 CrossRef CAS PubMed.
- H. R. VanDeusen, J. R. Ramroop, K. L. Morel, S. Y. Bae, A. V. Sheahan, Z. Sychev, N. A. Lau, L. C. Cheng, V. M. Tan, Z. Li, A. Petersen, J. K. Lee, J. W. Park, R. Yang, J. H. Hwang, I. Coleman, O. N. Witte, C. Morrissey, E. Corey, P. S. Nelson, L. Ellis and J. M. Drake, Mol. Cancer Res., 2020, 18, 1176 CrossRef CAS PubMed.
- C. Santos, R. Sanz-Pamplona and R. Salazar, Ann. Oncol., 2018, 29, 1340–1343 CrossRef CAS.
- M. Amit, S. Na'ara, E. Fridman, E. Vladovski, T. Wasserman, N. Milman and Z. Gil, Int. J. Cancer, 2019, 144, 3014–3022 CrossRef CAS PubMed.
- B. S. Paratala, J. H. Chung, C. B. Williams, B. Yilmazel, W. Petrosky, K. Williams, A. B. Schrock, L. M. Gay, E. Lee, S. C. Dolfi, K. Pham, S. Lin, M. Yao, A. Kulkarni, F. DiClemente, C. Liu, L. Rodriguez-Rodriguez, S. Ganesan, J. S. Ross, S. M. Ali, B. Leyland-Jones and K. M. Hirshfield, Nat. Commun., 2018, 9(1), 4821 CrossRef PubMed.
- H. Y. Woo, K. Na, J. Yoo, J. H. Chang, Y. N. Park, H. S. Shim and S. H. Kim, Brain Tumor Pathol., 2020, 37(4), 136–144 CrossRef CAS PubMed.
- R. Roskoski and A. Sadeghi-Nejad, Pharmacol. Res., 2018, 128, 1–17 CrossRef CAS PubMed.
- S. Giunti, A. Antonelli, A. Amorosi and L. Santarpia, Int. J. Endocrinol., 2013, 2013, 803171 Search PubMed.
- C. J. Ackermann, G. Stock, R. Tay, M. Dawod, F. Gomes and R. Califano, OncoTargets Ther., 2019, 12, 7857 CrossRef CAS.
- A. Markham, Drugs, 2020, 80, 1119–1124 CrossRef CAS.
- I. Dagogo-Jack, S. E. Stevens, J. J. Lin, R. Nagy, L. Ferris, A. T. Shaw and J. F. Gainor, J. Thorac. Oncol., 2018, 13, e226–e227 CrossRef.
- S. Parate, V. Kumar, G. Lee, S. Rampogu, J. C. Hong and K. W. Lee, Pharmaceuticals, 2021, 14, 282 CrossRef CAS PubMed.
- J. Meslamani, J. Li, J. Sutter, A. Stevens, H.-O. Bertrand and D. Rognan, J. Chem. Inf. Model., 2012, 52, 943–955 CrossRef CAS.
- P. P. Knowles, J. Murray-Rust, S. Kjær, R. P. Scott, S. Hanrahan, M. Santoro, C. F. Ibáñez and N. Q. McDonald, J. Biol. Chem., 2006, 281, 33577–33587 CrossRef CAS.
- J. Sutter, J. Li, A. J. Maynard, A. Goupil, T. Luu and K. Nadassy, Curr. Comput.-Aided Drug Des., 2011, 7, 173–180 CrossRef CAS.
- S. Khedkar, A. Malde, E. Coutinho and S. Srivastava, Med. Chem., 2007, 3, 187–197 CrossRef CAS.
- S. Parate, V. Kumar, Danishuddin, J. C. Hong and K. W. Lee, Int. J. Mol. Sci., 2021, 22, 5311 CrossRef CAS PubMed.
- S. Parate, V. Kumar, J. C. Hong and K. W. Lee, Molecules, 2021, 26, 2114 CrossRef CAS PubMed.
- C. A. Lipinski, Drug Discovery Today: Technol., 2004, 1, 337–341 CrossRef CAS PubMed.
- D. F. Veber, S. R. Johnson, H.-Y. Cheng, B. R. Smith, K. W. Ward and K. D. Kopple, J. Med. Chem., 2002, 45, 2615–2623 CrossRef CAS PubMed.
- G. Jones, P. Willett, R. C. Glen, A. R. Leach and R. Taylor, J. Mol. Biol., 1997, 267, 727–748 CrossRef CAS.
- M. L. Verdonk, J. C. Cole, M. J. Hartshorn, C. W. Murray and R. D. Taylor, Proteins: Struct., Funct., Bioinf., 2003, 52, 609–623 CrossRef CAS.
- X. Liu, D. Shi, S. Zhou, H. Liu, H. Liu and X. Yao, Expert Opin. Drug Discovery, 2018, 13(1), 23–37 CrossRef CAS.
- P. Śledź and A. Caflisch, Curr. Opin. Struct. Biol., 2018, 48, 93–102 CrossRef PubMed.
- J. Zhao, Y. Cao and L. Zhang, Comput. Struct. Biotechnol. J., 2020, 18, 417 CrossRef CAS PubMed.
- M. J. Abraham, T. Murtola, R. Schulz, S. Páll, J. C. Smith, B. Hess and E. Lindah, SoftwareX, 2015, 1–2, 19–25 CrossRef.
- X. Zhu, P. E. M. Lopes and A. D. MacKerell, Wiley Interdiscip. Rev.: Comput. Mol. Sci., 2012, 2, 167–185 CAS.
- V. Zoete, M. A. Cuendet, A. Grosdidier and O. Michielin, J. Comput. Chem., 2011, 32, 2359–2368 CrossRef CAS PubMed.
- B. J. Jaidhan, P. Srinivasa Rao and A. Apparao, Int. J. Comput. Sci. Inf. Technol., 2014, 3525–3528 Search PubMed.
- G. Bussi, D. Donadio and M. Parrinello, J. Chem. Phys., 2007, 126, 014101 CrossRef PubMed.
- M. Parrinello and A. Rahman, J. Appl. Phys., 1998, 52, 7182 CrossRef.
- T. Darden, D. York and L. Pedersen, J. Chem. Phys., 1998, 98, 10089 CrossRef.
- B. Hess, J. Comput. Chem., 1997, 18(12), 1463–1472 CrossRef CAS.
- W. Humphrey, A. Dalke and K. Schulten, J. Mol. Graphics, 1996, 14, 33–38 CrossRef CAS PubMed.
- M. Berry, B. Fielding and J. Gamieldien, Emerging Trends in Computational Biology, Bioinformatics, and Systems Biology, 2015, 487 Search PubMed.
- S. Genheden and U. Ryde, Expert Opin. Drug Discovery, 2015, 10, 449 CrossRef CAS PubMed.
- G. Poli, C. Granchi, F. Rizzolio and T. Tuccinardi, Molecules, 2020, 25(8), 1971 CrossRef CAS PubMed.
- R. Kumari, R. Kumar, O. S. D. D. Consortium and A. Lynn, J. Chem. Inf. Model., 2014, 54, 1951–1962 CrossRef CAS PubMed.
- F. Carlomagno, T. Guida, S. Anaganti, G. Vecchio, A. Fusco, A. J. Ryan, M. Billaud and M. Santoro, Oncogene, 2004, 23(36), 6056–6063 CrossRef CAS PubMed.
- V. Subbiah, V. Velcheti, B. B. Tuch, K. Ebata, N. L. Busaidy, M. E. Cabanillas, L. J. Wirth, S. Stock, S. Smith, V. Lauriault, S. Corsi-Travali, D. Henry, M. Burkard, R. Hamor, K. Bouhana, S. Winski, R. D. Wallace, D. Hartley, S. Rhodes, M. Reddy, B. J. Brandhuber, S. Andrews, S. M. Rothenberg and A. Drilon, Ann. Oncol., 2018, 29, 1869 CrossRef CAS PubMed.
- K. C. Shih, C. W. Shiau, T. S. Chen, C. H. Ko, C. L. Lin, C. Y. Lin, C. S. Hwang, C. Y. Tang, W. R. Chen and J. W. Huang, Bioorg. Med. Chem. Lett., 2011, 21, 4490–4497 CrossRef CAS PubMed.
- V. La Pietra, S. Sartini, L. Botta, A. Antonelli, S. M. Ferrari, P. Fallahi, A. Moriconi, V. Coviello, L. Quattrini, Y. Y. Ke, H. Hsing-Pang, F. Da Settimo, E. Novellino, C. La Motta and L. Marinelli, Eur. J. Med. Chem., 2018, 150, 491–505 CrossRef CAS PubMed.
- C. George Priya Doss, B. Rajith, C. Chakraboty, V. Balaji, R. Magesh, B. Gowthami, S. Menon, M. Swati, M. Trivedi, J. Paul, R. Vasan and M. Das, Mol. BioSyst., 2014, 10, 421–436 RSC.
- S. P. Bhujbal, P. K. Balasubramanian and S. Joo Cho, Med. Chem. Res., 2017, 26, 3228–3239 CrossRef CAS.
- S. P. Bhujbal, P. K. Balasubramanian, S. Keretsu and S. J. Cho, Bull. Korean Chem. Soc., 2019, 40, 207–213 CrossRef CAS.
- S. P. Bhujbal, S. Keretsu and S. J. Cho, Molecules, 2021, 26(3), 691 CrossRef CAS PubMed.
- S. Kim, J. Chen, T. Cheng, A. Gindulyte, J. He, S. He, Q. Li, B. A. Shoemaker, P. A. Thiessen, B. Yu, L. Zaslavsky, J. Zhang and E. E. Bolton, Nucleic Acids Res., 2021, 49, D1388–D1395 CrossRef CAS PubMed.
Footnote |
† Electronic supplementary information (ESI) available. See DOI: 10.1039/d1ra07328a |
|
This journal is © The Royal Society of Chemistry 2022 |
Click here to see how this site uses Cookies. View our privacy policy here.