Multiplexed structured illumination super-resolution imaging with lifetime-engineered upconversion nanoparticles†
Received
23rd October 2021
, Accepted 2nd November 2021
First published on 2nd November 2021
Abstract
The emerging optical multiplexing within nanoscale shows super-capacity in encoding information by using lifetime fingerprints from luminescent nanoparticles. However, the optical diffraction limit compromises the decoding accuracy and throughput of the nanoparticles during conventional widefield imaging. This, in turn, challenges the quality of nanoparticles to afford the modulated excitation condition and further retain the multiplexed optical fingerprints for super-resolution multiplexing. Here we report a tailor-made multiplexed super-resolution imaging method using the lifetime-engineered upconversion nanoparticles. We demonstrate that the nanoparticles are bright, uniform, and stable under structured illumination, which supports a lateral resolution of 185 nm, less than 1/4th of the excitation wavelength. We further develop a deep learning algorithm to coordinate with super-resolution images for more accurate decoding compared to a numeric algorithm. We demonstrate a three-channel super-resolution imaging based optical multiplexing with decoding accuracies above 93% for each channel and larger than 60% accuracy for potential seven-channel multiplexing. The improved resolution provides high throughput by resolving the particles within the diffraction-limited spots, which enables higher multiplexing capacity in space. This lifetime multiplexing super-resolution method opens a new horizon for handling the growing amount of information content, disease source, and security risk in modern society.
1. Introduction
Optical multiplexing is an easily implemented solution that combines many signals onto an ensemble of optical carriers for information transmission,1,2 data storage,3,4 and security.5 The relatively mature technique of wavelength or colour division multiplexing, however, has shown limitations in the requirement of multi-lasers for excitation and spectral cross-talk. As an orthogonal dimension to colour, the lifetime can be used to boost the super capacity of multiplexing. The lifetime multiplexing method has been demonstrated by using microcarriers, which were tagged with nanoparticles that have separate lifetime identities under single laser excitation.6 However, the nanoparticles have not fully exerted their advantages on the smaller size as nanoscale carriers due to the restriction of optical diffraction limit during imaging. The diffraction limit will reduce the throughput of effective pixels within an imaging area. In particular, the spatial resolution further hampers the single nanocarriers for future digital assays in detecting low concentration analytes.
Towards the super-resolution multiplexing, organic fluorophores such as proteins and cyanine dyes have been explored for multi-colour or multi-lifetime super-resolution imaging through stochastic optical reconstruction microscopy (STORM),7 stimulated emission depletion (STED),8,9 and DNA point accumulation in nanoscale topography.10 However, the existent issues from the colour channel multiplexing remain in these experiments, e.g., the simultaneous employment of multi-colour laser to match for different dyes and the limited channel numbers by spectrum width to avoid cross-talks. For lifetime multiplexed imaging, a library of nanoprobes, which are bright, stable, and with different lifetimes under a single laser excitation, would simplify the experimental setup of multiplexed super-resolution imaging.
In search of the potential probe candidates, most of the nanoparticles such as quantum dots,11–13 carbon dots,14,15 and molecular probes16–18 show short lifetimes in nanoseconds, limiting the channels for lifetime multiplexing. In contrast, lanthanide-doped upconversion nanoparticles (UCNPs) have attracted significant attention due to the stable anti-Stokes shift emission19–22 and the tunable lifetime from microsecond to millisecond.6,23–25 Moreover, the lifetime can be tuned by changing the doping concentration of rare-earth ions, changing various kinds of emitter ions, or using different exciting lasers.
Here, we demonstrate that a library of UCNPs with tailored lifetime profiles shows sufficient brightness and long-term photostability for lifetime multiplexing super-resolution microscopy. Based on the lifetime profiles of UCNPs, we establish time-resolved structured illumination microscopy (TR-SIM), enabling high-throughput multiplexing super-resolved imaging through a single 808 nm excitation laser. We demonstrate the three-channel super-resolution optical multiplexing with decoding accuracy above 93% and potential seven-channel multiplexing with more than 60% recognition accuracy by a deep learning based algorithm.
2. Results and discussion
2.1. Experimental setup
The experimental setup for TR-SIM and time-resolved widefield microscope (TR-WF) is sketched in Fig. 1. It is built on the modification of a conventional widefield fluorescence microscopy system. A single-mode diode-pumped solid-state laser beam (LU0808M250, Lumics Inc., 250 mW C. W., the excitation power density of 3.23 kW cm−2 in spot size) illuminates the digital mirror device (DMD, DLP 4100, Texas Instruments Inc., USA) after beam collimation and expansion with apochromatic lenses. The DMD is controlled and synchronized using a multifunction data acquisition device (USB-6343, National Instruments) and a purpose-built LabVIEW program. The laser light is modulated by the DMD binary patterns and passes through the demagnified optical system, consisting of the objective lens to produce the sinusoidal fringe illumination patterns on the sample plane. For widefield imaging, the laser light can be switched directly to the objective lens via the flexible mirrors to bypass the DMD (the pink beams in Fig. 1). A short-pass dichroic mirror (DM, ZT785spxxr-UF1, Chroma Inc.) and a short pass filter (SPF, ET750sp-2p8, Chroma Inc.) are used to separate the excitation light and the fluorescence light. The detector is a time-resolved sCMOS camera (iStar sCMOS, 16 bits gray depth, Andor Inc.). The camera also functions as a time-gate modulator of exciting laser light via a BNC cable. The time-resolved ability is based on the gating method for fluorescence-lifetime imaging microscopy.26,27 The camera gate is 100 μs under the laser excitation of 0–200 μs. The received fluorescence intensity signal is integrated 7500 times (3000 for TR-WF), resulting in an exposure time of 100 μs × 7500 = 0.75 seconds for each image (0.3 s for TR-WF). The camera gates with different temporal delays from 0 μs to 3000 μs provide the image at different time regions within the lifetime curves, making it possible to acquire the 30-frame images from 0 μs to 3000 μs along with lifetime curves. The details are shown in Fig. S5 in ESI.† In addition, by switching a flip mirror located between the tube lens and the camera, the emission fluorescence light could be coupled to a multi-mode fiber (MMF, M24L02, Thorlabs Inc.), which is linked with a miniature monochromator (iHR550, Horiba Inc.) to detect the spectral range from 400 to 740 nm.
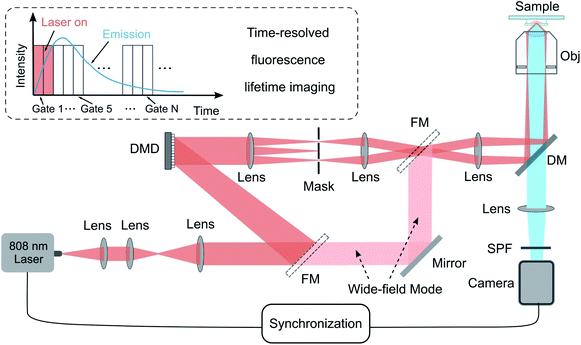 |
| Fig. 1 Schematic diagram of the TR-SIM & TR-WF microscope. DMD, digital micromirror device; FM, flexible mirror; Obj, objective lens; DM, dichroic mirror; SPF: short pass filter. The inset (top left) illustrates the time-resolved fluorescence lifetime imaging from 0 μs to 3000 μs with a time-gate of 100 μs and a gated excitation laser from 0 μs to 200 μs. | |
2.2. Nanoparticles with lifetime tunability
We synthesized a library of UCNPs with a well-defined Nd–Yb–Er core–shell–shell–shell (active core@energy migration shell@sensitization shell@inert shell) structure, as illustrated in Fig. 2(a). More details and the transmission electron microscopy (TEM) images are provided in the ESI (Fig. S1–S4).† Under 808 nm excitation, the Nd3+ sensitizers confined in the second outer layer harvest the excitation photons and transfer the energy to the Yb3+ in the same layer via cross-relaxation. Subsequently, the energy transfers via energy migration among the Yb3+ ions until reaching the core layer. Finally, the Er3+ emitters entrap the energy to generate luminescence (Fig. 2(b)). This upconverting energy transferring provides large anti-stokes emission and the resultant background-free imaging.28 This design used a core–multi-shell system based on previous works of tunable lifetimes.6,23,29 The structure with an additional migration layer (NaYF4:Yb3+) can significantly slow down the interfacial energy migration process from Nd3+ to the Yb3+ in the core layer, leading to a time-delayed up-rising time and longer lifetime (named as τ2-dots).
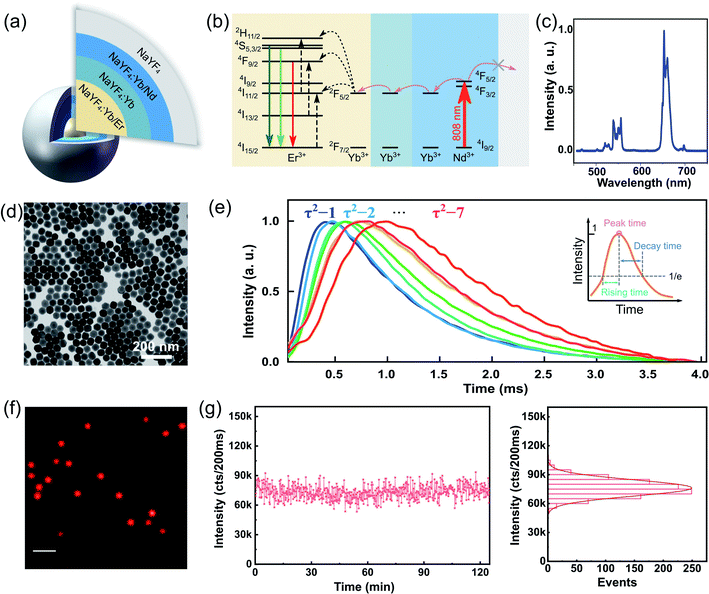 |
| Fig. 2 Photostable uniform nanoprobes with lifetime tunability. (a) Schematic representation of the core–multi-shell τ2-dots using NaYF4 as host nanocrystals: the core is co-doped by Yb3+ sensitizer and Er3+ emitter, the first layer of shell serves as the energy migration layer, doped with 5% Yb3+ ions, the second shell sensitizes 808 nm photons by 20% Nd3+ ions with the role of Yb3+ ions as the secondary sensitizers, and the outer layer of pure NaYF4 as optically inert shell passivates each single nanoparticle from surface quenchers. (b) Energy level diagrams of the core–multi-shell τ2-dots. (c) The typical upconversion emission spectrum of τ2-dots. (d) TEM characterization of the typical morphology of uniform nanocrystals (Yb3+–Nd3+–Er3+ core–multi shell nanoparticles) with an average size of 59 nm. An arbitrary batch (τ2-5) of τ2-dot used here was chosen from a library of core–multi-shell nanoparticles. (e) The experimentally averaged lifetime curves of τ2-x dots (x = 1, 2, …, 7) from 20 single dots for each type. (f) Widefield microscopic imaging of τ2-2 under 808 nm excitation. Scale bar: 2 μm. (g) Photostability of a single dot under 808 nm excitation in widefield microscopy (the intensity is the sum of covered pixels by a single dot). | |
Herein, we prepared seven types of τ2-dots. Fig. 2(c) shows its typical upconversion emission spectra. The TEM image in Fig. 2(d) shows typical NaYF4 UCNPs with uniform morphology, where the architectures of each particle indicate a hexagonal crystal phase and an average size of about 60 nm in diameter. Fig. 2(e) depicts the averaged lifetime fingerprints of τ2-1 to τ2-7 from experimentally collected curves of single dots for each type. For further clarification about the lifetime tunability, we define three parameters: rising time, peak time, and decay time to quantize the difference between the different types of τ2-dots. The peak time is defined by the time point at maximum intensity; the rising time and decay time are defined by the time between the peak time point and the time point with 1/e of the maximum intensity during the rise and decays, respectively (Fig. 2(e)). The peak time shifts from 400.1 μs to 982.1 μs, while the rising time and decay time prolong from 272.0 μs to 589.3 μs, and 942.4 μs to 1332.9 μs, respectively (see Fig. S6 and S7† for more details).
Under the widefield excitation of 808 nm laser, Fig. 2(f) shows an optical image of uniform and bright τ2-2 dots. This high brightness allows for TR-SIM to see single UCNPs. The fluorescence intensity from a single nanoparticle can retain for 120 min, verifying the strong fluorescence stability of τ2 dots (Fig. 2(g)). The photostability measurement of single τ2 dots (τ2-2 dots) is measured by a sum of covered pixels of this dot in TR-WF imaging mode under continuously gated excitation. The time gate here is 50 μs, which is shorter than the one used in TR-SIM, with an integration of 4000 times (an exposure time of 200 ms) in TR-WF mode. The duration of a single cycle is 3 ms. Thus, an on-going time of 12 s is spent for a single point measurement. A quantity of more than 600 time points is shown here to support the evidence of photostability in 120 min. Such photostability is highly desired for super-resolution imaging when it requires long-term and continuous recording.
2.3. Fluorescence time-resolved structured illumination microscopy
Taking advantage of the τ2-dots in brightness, photostability, and microsecond scale lifetime, we developed a time-resolved super-resolution imaging method based on structured illumination microscopy.30–36 Compared to traditional TR-WF, the TR-SIM has superior decoding accuracy and better efficiency on high-throughput optical multiplexing imaging, benefiting from its ability to resolve the aggregated particles within diffraction limitation (Fig. 3(a)). Fig. 3(a) is the diagram of TR-WF and TR-SIM. In TR-WF, under a widefield (WF) Gaussian beam excitation, the time-resolved camera collects WF images at different time points. The evolution of the integrated intensity shows the lifetime profile of a cluster of UCNPs (Y-axis is the summed intensity of its covered pixels). With improved resolution in structured illumination microscopy (SIM) mode, as a contrast, the cluster can be resolved into single UCNPs, with their lifetime profiles. Typically, nine excitation patterns are required to achieve linear SIM,30,32,35 as shown in Fig. 3(b). Here we use the DMD to generate the nine sinusoidal illumination patterns along with three angles (θ = 0°, 60°, and 120°) and three phase steps (φ = 0, 2π/3, and 4π/3) per angle for linear SIM. For each time point, the nine raw images are processed by the Fourier-based image reconstruction algorithm to obtain the super-resolution image. We use an open-source SIM reconstruction software fairSIM (free analysis and interactive reconstruction SIM)33 for both parameter estimation and reconstruction process. Furthermore, in order to acquire the 30-frame TR-SIM image series, nine groups of time-resolved frames are captured with the time-resolved camera under the nine excitation patterns, respectively. Fig. 3(c) shows the typical TR-SIM images of τ2-2 dots at the time points of 300 μs, 600 μs, and 900 μs with a time gate of 100 μs. The UCNPs can be clearly resolved by TR-SIM (Fig. 3(d)), compared with the TR-WF image (Fig. 3(e)). The line profile of a single UCNP (Fig. 3(f)) indicates a lateral resolution of 185.5 nm.
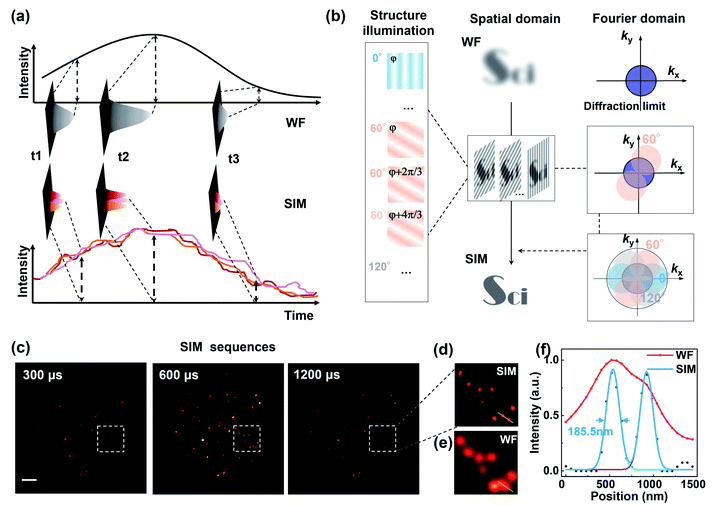 |
| Fig. 3 Time-resolved structured illumination microscopy (TR-SIM) for sub-diffraction imaging. (a) Illustration of the comparison of fluorescence lifetime imaging in widefield mode and SIM mode. The cluster in WF mode can be resolved into single UCNPs in SIM mode due to the improved resolution. The individual lifetime curves of single UCNPs are then obtained for the particle classification (Y-axis is the summed intensity of its covered pixels). (b) Principle of the SIM method. Typically, 9 sinusoidal excitation patterns with differences in the orientations and phases are used in SIM. By exciting the sample with illumination patterns, the high-frequency super-resolution information can be shifted into the diffraction-limited detection passband. Thus, super-resolution images can be reconstructed with an extended spatial frequency spectrum. (c) Example of super-resolution imaging series in TR-SIM at 300 μs, 600 μs, and 1200 μs, which are selected from a detection range from 0 μs to 3000 μs with a time gate of 100 μs, under the laser excitation patterns of 0–200 μs. Scale bar: 2 μm. (d) Enlarged imaging area under TR-SIM and corresponding area imaged by widefield microscopy (e). (f) Line profiles of the selected areas in (d) and (e). | |
Fig. 4 shows the TR-SIM and widefield images from samples τ2-1 to τ2-7, respectively. Here the super-resolution images are acquired from the time sequence where the intensities achieve the maximum in the lifetime profiles (range from 400 μs to 1000 μs) for a better signal-to-noise ratio. The line profiles of the arrow-pointed particles are shown in the third column. These line profiles are fitted with the Gaussian function to find their full width at half maxima (FWHM), ranging from 172.9 nm to 192.0 nm. The line profiles of single UCNPs indicate a lateral resolution of 184.8 ± 6.2 nm, with an improvement factor of 1.85 compared to the FWHM of 341.7 ± 19.6 nm in widefield. The ability to resolve individual nanoparticles with improved resolution allows TR-SIM to detect more single nanoparticles and their lifetime profiles than TR-WF, as different nanoparticles and their lifetime profiles are overlapped within the diffraction-limited spots in widefield imaging. The slight distortion of nanoparticles' images results from the limited time-resolved detecting efficiency, which can be improved by using a more sensitive camera, a more stable stage, or a higher excitation power in the future.
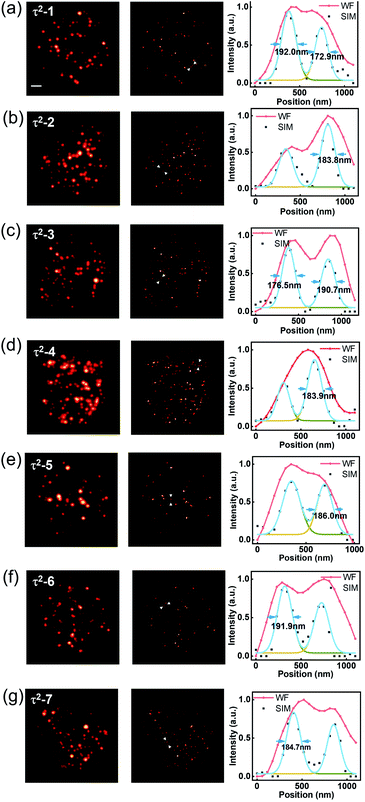 |
| Fig. 4 Comparison of TR-WF and TR-SIM with seven kinds of τ2 dots. For each group, TR-WF and TR-SIM and the line profiles of selected areas (indicated by the arrows in TR-SIM images) are shown from left to right. Scale bar: 2 μm. | |
2.4. Decoding in multiplexed super-resolution imaging
Next, we need to identify the spatially separated nanoparticles according to their lifetime fingerprints. This classification is based on recognizing the lifetime feature rather than absolute intensity. Hence, the time-invariant background light will not affect the classification accuracy. In Fig. 5, we developed a straightforward maximum correlation with the mean lifetime curve (MC-MLC) method to decode the multiplexing of different channels with different kinds of τ2-dots. The working scheme of MC-MLC is shown in Fig. 5(a)–(c). The statistic lifetime profiles (Fig. 5(b)) are averaged from many (typical >200) of single UCNP lifetime profiles (Fig. 5(a)). With the database of averaged lifetime profiles for all seven types of dots, we can identify any unknown single dot profile by pointwise intensity comparison, in which the quantitative indicators (the sum of squares of deviations:
, x = 1, 2, …, 7) of each round comparison determines the category of the single dot (Fig. 5(c)). Here, k represents the different time points, I is the lifetime profile from the unknown dot, and Ī is the averaged lifetime profile from the known dots.
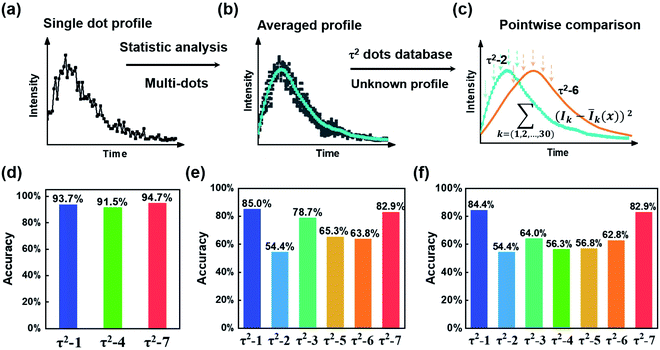 |
| Fig. 5 Recognition of multi-channel single τ2-dots in SIM mode with MC-MLC method. (a–c) Principle of MC-MLC method. The lifetime curve of a single dot (a) is compared with the mean curves of measured multiple τ2-dots (b). The least sum of squares of these deviations gives the classification result (c). (d–f) Classification accuracies of 3, 6, and 7 types of τ2-dots by MC-MLC method. | |
Fig. 5(d) shows the recognition results for τ2-1, τ2-4, and τ2-7 dots, in which the accuracies are higher than 90%, due to the well separated lifetime curves of these three types. The accuracy is estimated by taking the ratio of the classified number of particles with the total number of particles. For instance, during the three-channel accuracy estimating experiment (Fig. 5(d)), 1419 particles are classified into three types (334, 552, and 533 for τ2-1, τ2-4, and τ2-7, respectively) according to their lifetime profiles. The 313 particles within all 334 τ2-1 dots are classified into τ2-1, which indicates an accuracy of 93.7%. This validates our MC-MLC method. However, when we increase the categories of τ2-dots to six or seven types of dots, the accuracies of every dot decrease with the lowest accuracy of 54.4% for τ2-2 (Fig. 5(e) and (f)).
The bowl-shaped recognition accuracies in Fig. 5(f) indicate the challenge to identify the very similar lifetime profiles. The overlapping sections in lifetime curves result in difficulty in distinguishing UCNPs. The overlapping is from two factors: (a) how close the averaged curves are, (b) how much the variation for a single dot curve is. For the central types, both the similarly averaged curves and the dot-to-dot variations would make it very hard to distinguish the central types. According to the MC-MLC method, it only counts for the difference in the same time point, while the curve feature's information might be hidden inside the cross-analysis between different time points. Besides, the weight of the curve at different time points should be different with different intensity amplitudes and signal-to-noise ratios. Therefore, it is challenging to find a simple mathematical expression to include the dependent factors, while the deep learning algorithms provide a straightforward way to take all lifetime signatures, including cross-correlation between different time points and signal-to-noise ratios.
We further developed a deep learning algorithm to improve the multichannel recognition rates, as shown in Fig. 6(a). The algorithm is based on the convolutional neural networks (CNN) architecture, which contains one convolutional layer and a fully connected network with two layers. The CNN includes 80 channels on the convolutional layer, 80 neurons on the first fully connected layer, and 50 neurons on the second connected layer. The CNN is trained using a variant of the stochastic gradient descent (SGD) algorithm, “Adam”. All layers use the same global learning rate of 0.005. We use Pytorch deep learning platform to train, validate, and test our network. We first apply the super-resolution image series of the seven types of τ2-dots with a quantity of about 200 to 500 dots for training. To select single dots, we use the watershed segmentation based on the OTSU threshold to obtain the pixel area of each dot, in which the image frames at the peak time points are chosen to use for better signal to noise ratios. The lifetime curve of each dot is generated from the sum of all pixels contained in the area. The artificial neural networks are trained by τ2-dots' lifetime curves that are separately measured for each type. Then, the network could extract the fingerprint features of each type of τ2-dots and provide the optimized CNN and classification boundaries by getting feedback from accuracies. After the trained network was optimized, we randomly selected a set of untrained image series for each type of dots (about 50 single dots for each type) to test their classification accuracies. To eliminate the random effect, we repeated the validation experiment 50 times to produce the mean classification accuracies for each type of dots, in which 50 dots for each type are randomly selected for testing, and the others are used to train the CNN. For three-channel super-resolution multiplexing of τ2-1, 4, and 7, the mean classification accuracies of the lifetime profiles from selected single nanoparticles are further enhanced to 93%, 93%, and 96%, respectively (Fig. 6(b)). As shown in the top error bars, the false recognition parts of the dots are classified into their neighbors. To demonstrate the power of the super-resolved optical multiplexing, we compared the recognition possibility of TR-SIM and TR-WF on the mixed samples of τ2-1, 4, and 7 in Fig. 6(c). The aggregation under the WF mode dramatically affects the lifetime curves and the sample recognition. For example, an aggregation area of different kinds of τ2-dots would generate a mixed lifetime curve and lead to a wrong recognition. TR-SIM helps to address this aggregation-induced artificial effect by separating single dots from the cluster (Fig. 6(c)ii), benefiting from its higher lateral resolution (Fig. 6(c)i). Moreover, the improved lateral resolution also helps identify more particles (Fig. 6(c)iii).
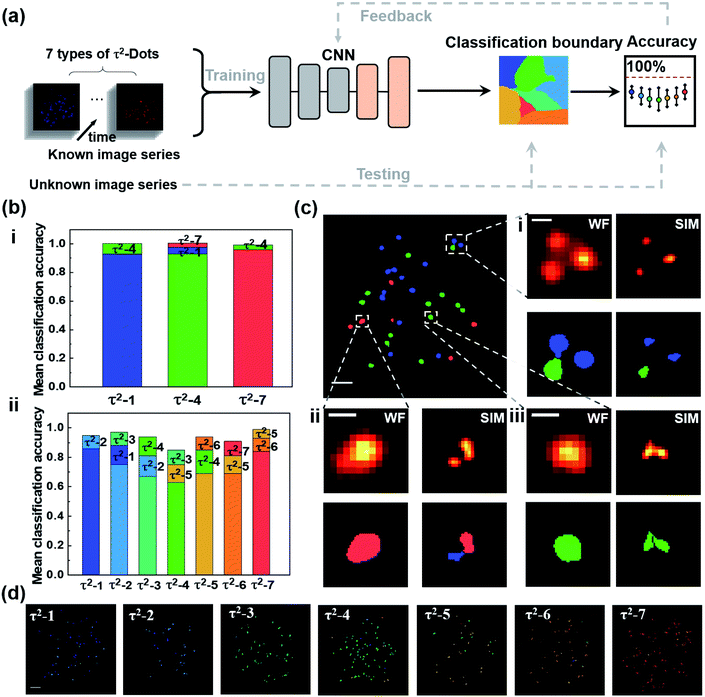 |
| Fig. 6 Deep learning assisted decoding of multi-channel single τ2-dots in super-resolution mode. (a) Illustration of the flow path of deep learning aided decoding process. The time-resolved image series of seven types of single τ2-dot are input to convolutional neural networks (CNN) which contains a convolutional network and a fully connected network with two layers to define the feature coverage for each type of τ2-dots (the classification boundaries). Another randomly untrained seven sets of images are used as ‘unknown dots’ for the classification with acquired boundaries. Recognition accuracies are obtained by comparing recognition results and true categories of tested dots, based on 50 times random validation test for each type of dots. (b) Mean classification accuracies of 3 and 7 types of τ2-dots with deep learning algorithms. The error bars at the top show the false recognitions. (c) Example of classification results for a mixture of three types of τ2-dots (τ2-1, 4, and 7 dots, as shown in blue, green and red color same to (b)) in widefield mode (upper left). Scale bar: 2 μm. (i–iii) Imaging results (upper) and classification results (bottom) of the selected small areas in WF mode and SIM mode show the precisely decoding and increased quantity of single dots due to the extended sub-diffraction limited resolution. Scale bar: 500 nm. (d) Classification result for the well-trained CNN to recognize the arbitrary seven sets of ‘unknown’ τ2-dots (for visualization purpose, pseudo-colour is used to represent each type of single dots corresponding to (b)). Scale bar: 2 μm. | |
For the potential seven-channel super-resolution multiplexing, all the accuracies are higher than 60%, with a range from 63% to 86%, showing the better performance of the deep learning algorithm than the MC-MLC method, with a typical graphic classification result shown in Fig. 6(b) and (d). By further assessing the unsuccessfully identified territories, we understand that the bias is mainly caused by the profile similarity from neighbouring types of dots. To improve the decoding accuracies, a further study could focus on enhancing the emission intensities of single dots for better signal-to-noise ratios of the raw images. The aggregated particles unresolved by SIM lead to mixed lifetime curves of single nanoparticles, which restricts classifying them by the current deep learning model. It is possible to recognize the single nanoparticles from these aggregates by further involving both the luminescence intensity (Fig. S6†) and lifetime curves into the neural network in the future since both the lifetime curves and emission intensities of aggregates are the linear recombination of the contained nanoparticles.
3. Conclusions
In conclusion, we have developed a super-resolution multiplexing imaging method with photostable lifetime-engineered nanoprobes: a library of Yb3+–Nd3+–Er3+ core–multi shell UCNPs with tailored lifetime profiles. Rather than implementing multiple excitation lasers or separated detection, a simple optical setup containing a single excitation laser and a single camera is applied here for multichannel recognition of the probes. We demonstrate the three-channel super-resolution optical multiplexing with decoding accuracies above 93% and potential seven-channel multiplexing with a 185 nm lateral resolution. Since this work is based on linear SIM, the resolution can be further improved by using the nonlinear response of upconversion emission to introduce high-frequency harmonics into the Fourier domain and to achieve the upconversion nonlinear SIM (U-NSIM)36 with more illumination patterns. The imaging speed can be improved by further developing the nanomaterials. For instance, a shorter lifetime of nanoparticles results in a shorter frame interval time, and a brighter emission offers a smaller integration number. Comparing with the previous work of confocal-based lifetime multiplexing of UCNPs-decorated microspheres6 and WF decoding of τ2-dots,38 this work enhanced the lateral resolution for nanoscale multiplexing of single particles towards higher multiplexing capacity in space. For example, this super-resolution lifetime multiplexing method will benefit the photonic data storage,6 as the data density increases with the improved reading out resolution. This method could also improve the efficiency of single-molecule (digital) upconversion-linked immunosorbent assay (digital ULISA),37 as higher resolution provides more accurate counting result of the number of single nanoparticles, and the time domain dimension offers more capacity for the multiplexing detection. Considering the various excitation power densities at different depths of biological samples, the CNN-based deep learning algorithm needs to be retrained with the input of lifetime curves of all the samples collected under similar conditions during real applications. The features of the dots in other domains, such as fluorescence intensity, emission spectrum, or excitation laser wavelength, could also be considered in the decoding algorithm to improve its performances further. The high resolution of the method brings the security pattern's resolving power from micro-scale to nanoscale. We believe that TR-SIM with lifetime-engineered nanoprobes will provide new insights into the multiplexed super-resolution imaging methods in the time dimension.
Conflicts of interest
The authors declare no competing financial interests.
Acknowledgements
This research was financially supported by the Australian Research Council Discovery Early Career Researcher Award Scheme (J. Z., DE180100669, F. W., DE200100074), and the Australian Research Council Discovery Project (DP190101058 − F. W.). The authors thank the financial support from China Scholarship Council Scholarships (B. L.: 201706020170, J. L.: 201508530231, L. D.: 201809370076).
References
- X. Zhang, S. Yang, H. Zhou, J. Liang, H. Liu, H. Xia, X. Zhu, Y. Jiang, Q. Zhang and W. Hu,
et al., Perovskite-Erbium Silicate Nanosheet Hybrid Waveguide Photodetectors at the Near-Infrared Telecommunication Band, Adv. Mater., 2017, 29, 1604431 CrossRef.
- J. Zhou, J. L. Leaño Jr, Z. Liu, D. Jin, K.-L. Wong, R.-S. Liu and J.-C. G. Bünzli, Impact of lanthanide nanomaterials on photonic devices and smart applications, Small, 2018, 14, 1801882 CrossRef.
- G. Lin, M. A. Baker, M. Hong and D. Jin, The quest for optical multiplexing in bio-discoveries, Chem, 2018, 4, 997–1021 CAS.
- Q. Zhang, Z. Xia, Y.-B. Cheng and M. Gu, High-capacity optical long data memory based on enhanced Young’s modulus in nanoplasmonic hybrid glass composites, Nat. Commun., 2018, 9, 1183 CrossRef.
- J. Zhao, D. Jin, E. P. Schartner, Y. Lu, Y. Liu, A. V. Zvyagin, L. Zhang, J. M. Dawes, P. Xi and J. A. Piper,
et al., Single-nanocrystal sensitivity achieved by enhanced upconversion luminescence, Nat. Nanotechnol., 2013, 8, 729–734 CrossRef CAS PubMed.
- Y. Lu, J. Zhao, R. Zhang, Y. Liu, D. Liu, E. M. Goldys, X. Yang, P. Xi, A. Sunna and J. Lu,
et al., Tunable lifetime multiplexing using luminescent nanocrystals, Nat. Photonics, 2014, 8, 32–36 CrossRef CAS.
- M. Bates, G. T. Dempsey, K. H. Chen and X. Zhuang, Multicolor super-resolution fluorescence imaging via multi-parameter fluorophore detection, ChemPhysChem, 2012, 13, 99–107 CrossRef CAS PubMed.
- J. Bückers, D. Wildanger, G. Vicidomini, L. Kastrup and S. W. Hell, Simultaneous multilifetime multi-color STED imaging for colocalization analyses, Opt. Express, 2011, 19, 3130–3143 CrossRef.
- T. Niehörster, A. Löschberger, I. Gregor, B. Krämer, H.-J. Rahn, M. Patting, F. Koberling, J. Enderlein and M. Sauer, Multi-target spectrally resolved fluorescence lifetime imaging microscopy, Nat. Methods, 2016, 13, 257–262 CrossRef.
- P. A. Gómez-García, E. T. Garbacik, J. J. Otterstrom, M. F. Garcia-Parajo and M. Lakadamyali, Excitation-multiplexed multicolor superresolution imaging with fm-STORM and fm-DNA-PAINT, Proc. Natl. Acad. Sci. U. S. A., 2018, 115, 12991–12996 CrossRef.
- M. Han, X. Gao, J. Z. Su and S. Nie, Quantum-dot-tagged microbeads for multiplexed optical coding of biomolecules, Nat. Nanotechnol., 2001, 19, 631–635 CAS.
- J. Yang, S. R. Dave and X. Gao, Quantum dot nanobarcodes: epitaxial assembly of nanoparticle-polymer complexes in homogeneous solution, J. Am. Chem. Soc., 2008, 130, 5286–5292 CrossRef CAS.
- Y. Zhao, H. C. Shum, H. Chen, L. L. Adams, Z. Gu and D. A. Weitz, Microfluidic generation of multifunctional quantum dot barcode particles, J. Am. Chem. Soc., 2011, 133, 8790–8793 CrossRef CAS.
- K. Jiang, S. Sun, L. Zhang, Y. Lu, A. Wu, C. Cai and H. Lin, Red, green, and blue luminescence by carbon dots: full-color emission tuning and multicolor cellular imaging, Angew. Chem., Int. Ed., 2015, 54, 5360–5363 CrossRef CAS.
- Z. Tian, X. Zhang, D. Li, D. Zhou, P. Jing, D. Shen, S. Qu, R. Zboril and A. L. Rogach, Full-color inorganic carbon dot
phosphors for white-light-emitting diodes, Adv. Opt. Mater., 2017, 5, 1700416 CrossRef.
- Y. Li, Y. T. H. Cu and D. Luo, Multiplexed detection of pathogen DNA with DNA-based fluorescence nanobarcodes, Nat. Biotechnol., 2005, 23, 885–889 CrossRef CAS PubMed.
- S. H. Um, J. B. Lee, S. Y. Kwon, Y. Li and D. Luo, Dendrimer-like DNA-based fluorescence nanobarcodes, Nat. Protoc., 2006, 1, 995–1000 CrossRef CAS PubMed.
- W. Zhou, D. Li, C. Xiong, R. Yuan and Y. Xiang, Multicolor-encoded reconfigurable DNA nanostructures enable multiplexed sensing of intracellular microRNAs in living cells, ACS Appl. Mater. Interfaces, 2016, 8, 13303–13308 CrossRef CAS PubMed.
- H. He, B. Liu, S. Wen, J. Liao, G. Lin, J. Zhou and D. Jin, Quantitative lateral flow strip sensor using highly doped upconversion nanoparticles, Anal. Chem., 2018, 90, 12356–12360 CrossRef CAS PubMed.
- C. Chen, F. Wang, S. Wen, Q. P. Su, M. C. Wu, Y. Liu, B. Wang, D. Li, X. Shan and M. Kianinia,
et al., Multi-photon near-infrared emission saturation nanoscopy using upconversion nanoparticles, Nat. Commun., 2018, 9, 3290 CrossRef.
- L. Gao, X. Shan, X. Xu, Y. Liu, B. Liu, S. Li, S. Wen, C. Ma, D. Jin and F. Wang, Video-rate upconversion display from optimized lanthanide ion doped upconversion nanoparticles, Nanoscale, 2020, 12, 18595–18599 RSC.
- C. Chen, B. Liu, Y. Liu, J. Liao, X. Shan, F. Wang and D. Jin, Heterochromatic Nonlinear Optical Responses in Upconversion Nanoparticles for Super-Resolution Nanoscopy, Adv. Mater., 2021, 33, 2008847 CrossRef CAS.
- F. Wang, R. Deng, J. Wang, Q. Wang, Y. Han, H. Zhu, X. Chen and X. Liu, Tuning upconversion through energy migration in core-shell nanoparticles, Nat. Mater., 2011, 10, 968–973 CrossRef CAS PubMed.
- Y. Fan, P. Wang, Y. Lu, R. Wang, L. Zhou, X. Zheng, X. Li, J. A. Piper and F. Zhang, Lifetime-engineered NIR-II nanoparticles unlock multiplexed in vivo imaging, Nat. Nanotechnol., 2018, 13, 941–946 CrossRef CAS.
- J. Liao, J. Zhou, Y. Song, B. Liu, Y. Chen, F. Wang, C. Chen, J. Lin, X. Chen, J. Lu and D. Jin, Preselectable Optical Fingerprints of Heterogeneous Upconversion Nanoparticles, Nano Lett., 2021, 21, 7659–7668 CrossRef CAS PubMed.
- L.-C. Chen, W. R. Lloyd III, C.-W. Chang, D. Sud and M.-A. Mycek, Fluorescence lifetime imaging microscopy for quantitative biological imaging, Methods Cell Biol., 2013, 114, 457–488 Search PubMed.
- L. Scipioni, A. Rossetta, G. Tedeschi and E. Gratton, Phasor S-FLIM: a new paradigm for fast and robust spectral fluorescence lifetime imaging, Nat. Methods, 2021, 18, 542–550 CrossRef CAS.
- Z. Song, Y. G. Anissimov, J. Zhao, A. V. Nechaev, A. Nadort, D. Jin, T. W. Prow, M. S. Roberts and A. V. Zvyagin, Background free imaging of upconversion nanoparticle distribution in human skin, J. Biomed. Opt., 2012, 18, 061215 CrossRef.
- J. Zuo, D. Sun, L. Tu, Y. Wu, Y. Cao, B. Xue, Y. Zhang, Y. Chang, X. Liu and X. Kong,
et al., Precisely tailoring upconversion dynamics via energy migration in core-shell nanostructures, Angew. Chem., Int. Ed., 2018, 57, 3054–3058 CrossRef CAS PubMed.
- M. G. Gustafsson, Surpassing the lateral resolution limit by a factor of two using structured illumination microscopy, J. Microsc., 2000, 198, 82–87 CrossRef CAS PubMed.
- L. Schermelleh, P. M. Carlton, S. Haase, L. Shao, L. Winoto, P. Kner, B. Burke, M. C. Cardoso, D. A. Agard and M. G. Gustafsson,
et al., Subdiffraction multicolor imaging of the nuclear periphery with 3D structured illumination microscopy, Science, 2008, 320, 1332–1336 CrossRef CAS.
- P. Kner, B. B. Chhun, E. R. Griffis, L. Winoto and M. G. Gustafsson, Super-resolution video microscopy of live cells by structured illumination, Nat. Methods, 2009, 6, 339–342 CrossRef CAS PubMed.
- M. Müller, V. Mönkemöller, S. Hennig, W. Hübner and T. Huser, Open-source image reconstruction of super-resolution structured illumination microscopy data in ImageJ, Nat. Commun., 2016, 7, 10980 CrossRef.
- D. Li, L. Shao, B.-C. Chen, X. Zhang, M. Zhang, B. Moses, D. E. Milkie, J. R. Beach, J. A. Hammer and M. Pasham,
et al., Extended-resolution structured illumination imaging of endocytic and cytoskeletal dynamics, Science, 2015, 349, 6251 CrossRef.
- R. Heintzmann and T. Huser, Super-resolution structured illumination microscopy, Chem. Rev., 2017, 117, 13890–13908 CrossRef CAS.
- B. Liu, C. Chen, X. Di, J. Liao, S. Wen, Q. P. Su, X. Shan, Z.-Q. Xu, L. A. Ju and C. Mi,
et al., Upconversion nonlinear structured illumination microscopy, Nano Lett., 2020, 20, 4775–4781 CrossRef CAS PubMed.
- Z. Farka, M. J. Mickert, A. Hlavacek, P. Skládal and H. H. Gorris, Single molecule upconversion-linked immunosorbent assay with extended dynamic range for the sensitive detection of diagnostic biomarkers, Anal. Chem., 2017, 89, 11825–11830 CrossRef CAS PubMed.
- J. Liao, J. Zhou, Y. Song, B. Liu, J. Lu and D. Jin, Optical Fingerprint Classification of Single Upconversion Nanoparticles by Deep Learning, J. Phys. Chem. Lett., 2021, 12, 10242–10248 CrossRef CAS PubMed.
Footnote |
† Electronic supplementary information (ESI) available. See DOI: 10.1039/d1na00765c |
|
This journal is © The Royal Society of Chemistry 2022 |
Click here to see how this site uses Cookies. View our privacy policy here.