DOI:
10.1039/D1MD00331C
(Research Article)
RSC Med. Chem., 2022,
13, 183-195
Inhibition of N-type calcium ion channels by tricyclic antidepressants – experimental and theoretical justification for their use for neuropathic pain†
Received
13th October 2021
, Accepted 20th December 2021
First published on 21st December 2021
Abstract
A number of tricyclic antidepressants (TCAs) are commonly prescribed off-label for the treatment of neuropathic pain. The blockade of neuronal calcium ion channels is often invoked to partially explain the analgesic activity of TCAs, but there has been very limited experimental or theoretical evidence reported to support this assertion. The N-type calcium ion channel (CaV2.2) is a well-established target for the treatment of neuropathic pain and in this study a series of eleven TCAs and two closely related drugs were shown to be moderately effective inhibitors of this channel when endogenously expressed in the SH-SY5Y neuroblastoma cell line. A homology model of the channel, which matches closely a recently reported Cryo-EM structure, was used to investigate via docking and molecular dynamics experiments the possible mode of inhibition of CaV2.2 channels by TCAs. Two closely related binding modes, that occur in the channel cavity that exists between the selectivity filter and the internal gate, were identified. The TCAs are predicted to position themselves such that their ammonium side chains interfere with the selectivity filter, with some, such as amitriptyline, also appearing to hinder the channel's ability to open. This study provides the most comprehensive evidence to date that supports the notion that the blockade of neuronal calcium ion channels by TCAs is at least partially responsible for their analgesic effect.
Introduction
The first indication that tricyclic antidepressants (TCAs) might have the potential to ameliorate neuropathic pain came from a 1960 study that found that patients treated with imipramine experienced pain relief.1 By 1999, the off-label prescription of TCAs had become a mainstay in the treatment of neuropathic pain.2 An analysis of placebo-controlled trials published at the time found that, with a number of conditions, including diabetic neuropathy, postherpetic neuralgia, peripheral nerve injury and central pain, numbers needed to treat (NNT) were lowest with TCAs, when compared to other drugs including the selective serotonin reuptake inhibitors, carbamazepine, gabapentin, dextromethorphan, tramadol, oxycodone and capsaicin.2 Subsequently, a treatment algorithm developed at the University of Manitoba recommend four TCAs, amitriptyline, nortriptyline, imipramine and desipramine, as first line treatments for neuropathic pain.3 Studies and reviews that support the prescription of TCAs for a range of chronic pain conditions, including neuropathic pain, have continued to be published over the intervening years.4–6
Several authors have highlighted the need for strong scientific evidence to support the prescription of TCAs for neuropathic pain.7,8 Tricyclic antidepressants are known to be relatively unselective drugs that can simultaneously hit multiple pharmacological targets.7,9 Their analgesic effects have been partly attributed to the inhibition of noradrenaline and serotonin reuptake,10 adenosine uptake,11 and voltage gated sodium ion channels,12 and the modulation of NMDA receptors.13 A preliminary report in 1990 also revealed that TCAs have the potential to block voltage gated calcium channels,14 and this conclusion was later supported by the results of an investigation of the effect of TCAs on the calcium-induced contraction of guinea pig ileum.15 A series of TCAs were also used to test a recombinant model for the N-type calcium ion channel (CaV2.2) expressed in a HEK 293 cell line.16 Nonetheless, while it is often stated that the blockade of neuronal calcium ion channels may at least partially account for the analgesic activity of TCAs,4,9,10,17 this property has been the subject of only very limited scientific investigation.
The N-type calcium ion channel is an established target for the treatment of neuropathic pain.18 The channel consists of a unique α1 pore-forming subunit and auxiliary α2-δ and β subunits. The general structure of the α1 subunit is similar to that of other voltage-gated ion channels, comprising a single protein chain arranging itself into four domains (I–IV), each consisting of six transmembrane segments S1–S6 (Fig. 1). Three main features can be identified:
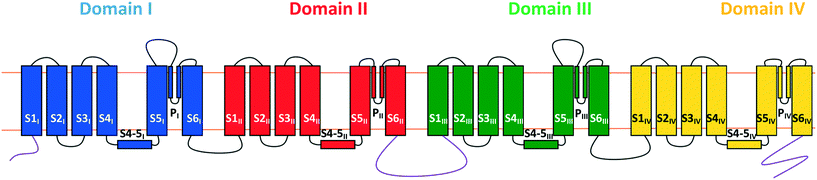 |
| Fig. 1 Representation of the α1 subunit of the CaV2.2 channel oriented with the extracellular region at the top. Shown are the abbreviations used for each segment (SXn) and the colour coding of the four domains (domain I, blue; domain II, red; domain II, green; domain IV, yellow). The selectivity filter is formed from the PI–IV loops and the internal gate is formed from the S6 segments. | |
a) The P-loops between the S5 and S6 segments of each domain form the channel's selectivity filter. Single glutamate residues on each of the four loops combine to form a binding site capable of accommodating one or two calcium ions and allows the channel to select for calcium over other ions;19
b) The S6 segments from each domain combine to form the internal gate; and
c) The S4 segments act as the voltage sensor for the channel. As a result of the multiple positively charged residues (arginine and lysine) present on these segments, negative potentials across the cell membrane attract them down towards the inside of the cell, in turn constraining the internal gate segments and locking the channel shut. Conversely, depolarisation allows the S4 segments to slide upwards and the S6 segments to move apart, thus opening the gate.20
The inhibition of the CaV2.2 channel is responsible for the pain-blocking activity of the intrathecally-delivered peptidic drug ziconotide or Prialt®, which is a synthetic form of the cone snail venom peptide, ω-conotoxin MVIIA.21 The ability of this cystine knot peptide, and the closely related ω-conotoxin GVIA, to potently block CaV2.2 channels stimulated research into the development of small molecule mimics of these venom components.22 Work over a number of years led to range of lower-molecular weight inhibitors that incorporated more drug-like features as the research progressed.23,24 Some of the most recently developed inhibitors bear a strong molecular similarity to TCAs,25 as do others patented by NeuroMed Technologies.26 This prompted the study described here, in which the N-type channel-blocking ability of a set of thirteen drugs (Fig. 2) was examined using endogenously expressed CaV2.2 channel in the SH-SY5Y neuroblastoma cell line. The test drugs included a series of commonly prescribed TCAs and a small number of drugs that had a close molecular similarity to TCAs. Further, since until recently,27 a crystal structure of the human CaV2.2 channel did not exist, an in silico model for this channel was developed which makes use of molecular dynamics (MD) simulations. This has allowed an intimate, theoretical investigation of the TCAs' mechanism of action at this channel.
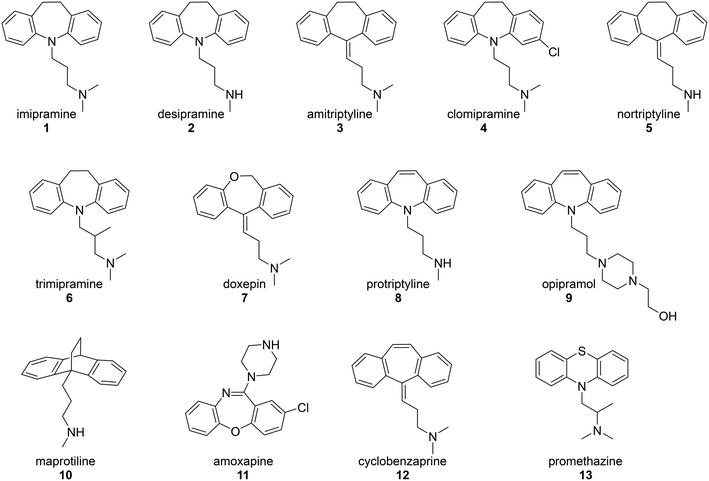 |
| Fig. 2 Chemical structures of the test drugs employed in this study. | |
Results and discussion
Biological assays
In the original study by Lavoie, Beauchamp and Elie,14 the ability of four TCAs, imipramine (1), desipramine (2), amitriptyline (3) and clomipramine (4), to inhibit voltage dependent calcium ion channels was measured through their effect on potassium-induced 45Ca uptake in synaptosomes isolated from rat brain cortex. These TCAs were found to inhibit 45Ca uptake with IC50 values in the range 26–31 μM. The current study used a modern calcium flux assay, which involved evaluation of the inhibition of the human CaV2.2 channel endogenously expressed in the neuroblastoma cell line, SH-SY5Y. This calcium flux imaging assay employed the FLIPRTETRA platform and included the CaV1 blocker nifedipine. This is a well-established tool for determining inhibition of the human CaV2.2 channel by low molecular weight compounds and peptides, and the inclusion of nifedipine ensures that the vast majority of observed calcium responses are due to the CaV2.2 channel.28,29 Cilnidipine was used as a positive control.30
The test compounds (1–13) were chosen based on a number of criteria. Firstly, the TCAs examined by Lavoie et al. (1–4) were included. Secondly, the results of the study by Wong and co-workers8 were taken into account. They reported that, of the TCAs commonly prescribed off-label, amitriptyline (3) was the one most likely to be prescribed for pain, followed by nortriptyline (5), trimipramine (6) and desipramine (2). Hence nortriptyline (5) and trimipramine (6) were included. Five other TCAs, doxepin (7), protriptyline (8), opipramol (9), maprotiline (10) and amoxapine (11), were chosen as these drugs are commonly prescribed tricyclic antidepressants in Australia31 and the United States32 and/or Europe. Finally, two structurally-related drugs, the muscle relaxant cyclobenzaprine (12) and the sedating antihistamine promethazine (13) were also tested. The purpose of choosing a wide set of TCAs for study was to gauge the generality of the CaV2.2 blockade by this class of drug and to look for any obvious structure–activity relationships. The calculated dose response curves obtained with these compounds are shown in Fig. 3 and IC50 values are presented in Table 1.
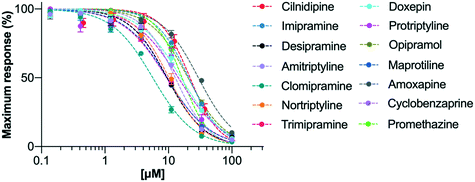 |
| Fig. 3 Activities of the test drugs in hCaV2.2 channels, determined by calcium influx fluorescence-imaging assays. Concentration–response curves for inhibition of hCaV2.2 under application of serial diluted concentrations of drugs 1–13 and the CaV2.2 control, cilnidipine. Data are presented as mean ± SEM from n = 3–5 independent experiments. The calculated IC50 values are shown in Table 1. | |
Table 1 Functional inhibition of the calcium channels hCaV2.2 by test drugs 1–13 and positive control cilnidipine. Data are presented as mean ± SEM and 95% CI from n = 3–5 independent experiments
Drug |
IC50 (μM) |
SEM |
95% CI (μM) |
Cilnidipine |
26 |
4 |
9–43 |
Imipramine (1) |
22 |
5 |
7–36 |
Desipramine (2) |
11 |
2 |
5–16 |
Amitriptyline (3) |
12 |
3 |
5–20 |
Clomipramine (4) |
9 |
4 |
1–19 |
Nortriptyline (5) |
10 |
1 |
7–12 |
Trimipramine (6) |
11 |
2 |
5–16 |
Doxepin (7) |
18 |
4 |
7–29 |
Protriptyline (8) |
14 |
3 |
6–23 |
Opipramol (9) |
24 |
7 |
6–42 |
Maprotiline (10) |
10 |
2 |
5–14 |
Amoxapine (11) |
27 |
4 |
15–39 |
Cyclobenzaprine (12) |
10 |
2 |
5–16 |
Promethazine (13) |
18 |
3 |
9–28 |
It can be seen that all of the chosen drugs were found to inhibit calcium influx through hCaV2.2 channels at equivalent or better potency to the positive control, cilnidipine. Further, tricyclic structures predominantly consisting of a carbon framework and bearing a flexible amine sidechain tended to show slightly stronger inhibition (see data for 2–6, 8, 10 and 12), and secondary amines were preferred over tertiary (for example see data for 1versus2). It can also be seen that halogen substitution appeared to improve potency (see data for 1versus4). All of these findings provide valuable insights into how more effective CaV2.2 inhibitors based on the TCA structure might be developed.
In silico studies
As indicated above, until recently there was no resolved structure for CaV2.2 available, however Martinez-Ortiz and Cardozo had published a homology model of the pore-forming α1 subunit of the CaV2.2 channel,33 constructed from a rabbit CaV1.1 single-particle Cryo-EM map (PDB: 5GJV).34 These researchers paid special attention to accurately modelling the S1 to S4 segments containing the channel's voltage-sensing domains33 (Fig. 1 and 4) which are of particular importance to its gating dynamics. In the present study, Martinez-Ortiz and Cardozo's static homology model was used as a basis for a theoretical construct which could be employed to probe the mechanism of inhibition of human CaV2.2 channel by TCAs. This involved embedding the homology model into a simulated cell membrane, incorporating a simulated salt solution and making use of MD to model channel behaviour. The theoretical model developed in this way was also compared to the recently published human CaV2.2 structure developed through the use of Cryo-EM.27
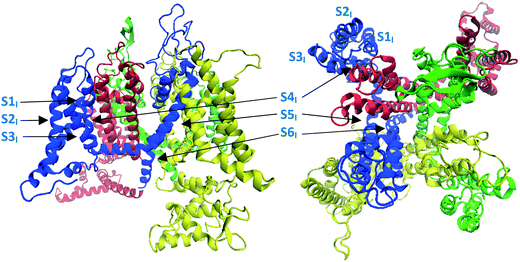 |
| Fig. 4 Image showing the structure of the Martinez-Ortiz and Cardozo homology model of the α1 subunit of the CaV2.2 channel, which was constructed from a rabbit CaV1.1 single-particle Cryo-EM map.33 Left: side view, right: top (extracellular) view. Domains I to IV are coloured blue, red, green and yellow respectively, consistent with colour coding defined in Fig. 1. | |
Protein equilibration.
The CaV2.2 homology model was first subjected to all-atom MD simulations to assess its stability and further refine it. Using the online server CHARMM-GUI,35 the CaV2.2 structure was embedded in a membrane of 85% 1-palmitoyl-2-oleoylphosphatidylcholine (POPC)/15% cholesterol,36,37 then the system was solvated in 0.15 M sodium chloride and a 36 ns simulation was launched using the NAMD 2.13 package.
The root-mean-square deviation (RMSD) of the non-hydrogen atoms and protein backbone started to plateau around 15 ns at 5.1 Å, which suggested that a local equilibrium had been reached (Fig. S1, ESI†). The transmembrane domains were quite stable with an average backbone RMSD of only 2.6 Å. To equilibrate the structure with calcium ions, the water and sodium chloride were then removed, and an aqueous 0.15 M calcium chloride solution was introduced, and a further 60 ns simulation was run. In this case, the RMSD of the non-hydrogen atoms started to plateau at around 20 ns of simulation time (Fig. S2, ESI†) at a value of 3.9 Å, with the transmembrane backbone deviating by only 2.2 Å. The structure obtained at the end of the simulation was then extracted and used in subsequent experiments.
It was found in the second simulation that as expected, calcium ions were quickly attracted towards the channel by the multiple acidic residues on the S5-P1 and P2-S6 loops. After approximately 3 ns of simulation time the selectivity filter was populated by two calcium ions (Fig. 5). Spontaneous calcium permeation through the channel was not observed as the intracellular gate remained closed. As our model was constructed with periodic boundary conditions, polarisation across the membrane was absent, thus there was no voltage potential to drive the structural shifts required for channel opening to occur. This was also observed in a study of the CaV1.2 channel, where the introduction of an external electric field and a steering force were needed to push a calcium ion into the channel, ultimately resulting in its passage through the channel.38
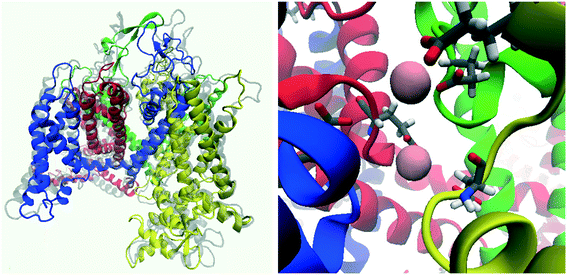 |
| Fig. 5 Snapshot of the CaV2.2 channel following MD simulations with NaCl then CaCl2 solutions. Left: Side view of the channel (initial state of the homology model is overlayed in transparent grey). Right: Selectivity filter and associated calcium ions (pink spheres). | |
Comparison between equilibrated homology model and the Cryo-EM structure.
Using the Schrödinger Maestro package, the equilibrated homology model was aligned with the recently released Cryo-EM structure of the N-type calcium channel (PDB: 7MIY)27 and a backbone RMSD of 3.9 Å was found. The main deviation between the two structures appears to come from the extra- and intracellular loops that occur between the transmembrane segments, and the intracellular S6–S1 segments between each domain. Given that these regions of the protein are more free-flowing during the MD simulation and expected to be more mobile in vivo, a backbone RMSD of almost 4 Å is reasonable. The core structure of the pore formed from the S4–S5, S5, P1 and S6 segments, where the TCAs were found to dock (vide infra), were however very similar between the three structures (Fig. 6). The RMSD for this part of the structure was found to be only 1.9 Å. This value is well within the range of those observed during the MD simulations and suggests that either structure could be used to model TCA binding.
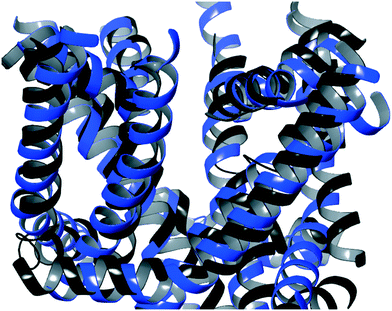 |
| Fig. 6 An overlay of the side view of the identified TCA binding site for the post-equilibration homology model (blue) and the Cryo-EM structure (black).27 | |
Docking.
Having obtained an equilibrated CaV2.2 model embedded in a simulated lipid bilayer and surrounded by a calcium chloride solution, attention was then turned to docking the TCAs into the channel to investigate possible binding modes and positions. The TCA structures were first imported into Schrödinger Maestro, and Glide was used to dock rigidly the compounds onto the model. As starting points, the binding of the TCAs on either side of selectivity filter were considered. The relevant region above the selectivity filter is represented on the refined CaV2.2 structure in Fig. 7 by a purple rectangle, whereas the region below the selectivity filter is represented by a black rectangle. These regions encompass the locations of several inhibitors in CaV-ligand and NaV-ligand Cryo-EM structures available in the Protein Data Bank: Three CaV1.1 blocking drugs, diltiazem (PDB: 6JPB), nifedipine (PDB: 6JP5) and verapamil (PDB: 6JPA), were found to bind in the CaV1.1 channel in the internal cavity below the selectivity filter and above the internal gate.39 The synthetic CaV3.1 inhibitor Z944 binds to the CaV3.1 channel in a similar manner (PDB: 6KZP).40 By contrast, the recently published Cryo-EM study of the CaV2.2 channel, ω-conotoxin MVIIA (ziconotide) was shown to bind just above the selectivity filter, sterically blocking access to it through strong interactions with the P2II segments, as well as the S5-P1III and S5-P1IV loops (7MIX).27 Consistent with this finding, ω-conotoxin GVIA, a peptide that also selectively blocks the CaV2.2 channel, was predicted by Chen and Chung to dock in a similar manner during MD simulations on a homology model of the CaV2.2 selectivity filter based on bacterial NaVAb channel.41 Two other natural marine toxins, tetrodotoxin (PDB: 6J8I, 6J8J) and saxitoxin (PDB: 6J8G, 6J8H), block the more distantly related NaV1.7 channel by obstruction of the NaV1.7 selectivity filter itself, on the extracellular side, at the convergence of the four P2I–IV segments that together form the filter.42
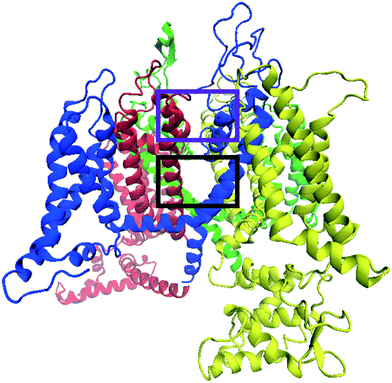 |
| Fig. 7 Side view of the homology model of the CaV2.2 channel, which has been refined using MD simulations, showing potential inhibitor binding sites. The purple rectangle encompasses the location of inhibitors that interfere with the selectivity filter and the black rectangle highlights a binding region consisting of an internal cavity between the selectivity filter and the internal gate. | |
Taking into account the two main reported voltage-gated ion channel inhibitor binding positions mentioned above, a very large box with dimensions 26 × 26 × 26 Å, centered on the selectivity filter glutamate residues, was used to investigate possible TCA binding sites in the CaV2.2 channel. The box included the lipophilic pockets on both the intra- and extra-cellular sides of the selectivity filter. From docking experiments performed within this region, it was found that almost all TCAs localised in the same general region, in the central cavity between the selectivity filter and internal gate, encompassed by the black rectangle shown in Fig. 7. Within these, several docking modes were observed, as detailed below.
Binding mode 1: amitriptyline/maprotiline-like.
A first cluster of compounds – imipramine (1), amitriptyline (3), nortriptyline (5), protriptyline (8), maprotiline (10) and promethazine (13) – were observed to dock under the P1III segment of the selectivity filter and between the S6III, S5III and S6IV segments, as represented in Fig. 8 and 9. This is analogous to where nifedipine was shown to bind in the CaV1.1 channel.39
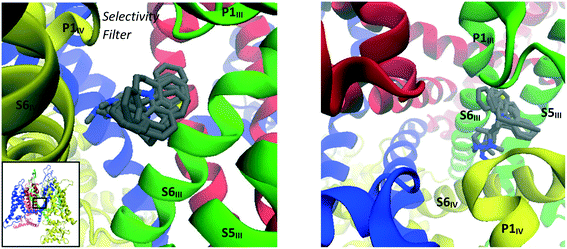 |
| Fig. 8 Images showing binding mode 1 with overlays of binding poses of imipramine (1), amitriptyline (3), nortriptyline (5), protriptyline (8), maprotiline (10) and promethazine (13) docked into the refined CaV2.2 structure; side (left) and top (right) views. | |
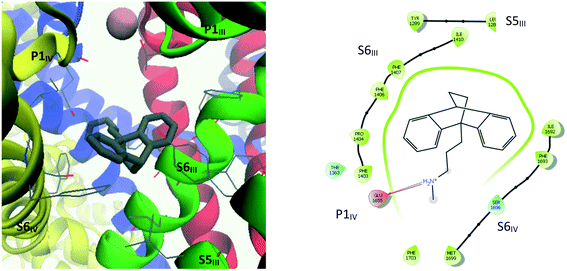 |
| Fig. 9 Left: 3D representation showing amino acid residues within 4 Å of maprotiline (10). Right: Ligand interaction diagram (within 4 Å) for maprotiline docked into the refined CaV2.2 structure. | |
The interaction diagram for maprotiline (10, Fig. 9) reveals that the lipophilic head group is in close contact with hydrophobic residues on all three segments – S6III, S5III and S6IV. Despite the close proximity of aromatic residues such as Tyr1289, Phe1407 and Phe1603, no π–π interactions could be detected, but it seems likely that they could occur if the TCA–protein complex was allowed to relax. Amitriptyline (3, Fig. 10) on the other hand was found to form one such π–π interaction between one of its aromatic rings and Phe1403, with the ammonium group forming a cation–π interaction with the side chain of Phe1407. The other coordinating residues are almost identical to maprotiline's.
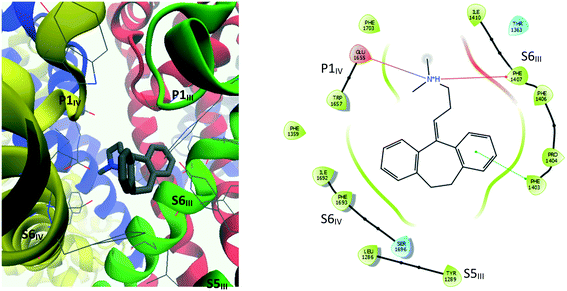 |
| Fig. 10 Left: 3D representation showing amino acid residues within 4 Å of amitriptyline (3). Right: Ligand interaction diagram (within 4 Å) for amitriptyline docked into the refined CaV2.2 structure. | |
By analogy with the mechanism of inhibition proposed for nifedipine,39 it is reasonable to expect that by associating with both S6III and S6IV, the inhibitors that bind in this region have a mode of action that acts to constrain the two segments from moving apart, and thus limiting the internal gate's ability to open. Maprotiline's propylammonium sidechain extends to make a hydrogen bond with one of the selectivity filter glutamate residues, Glu1655, whose sidechain had been pulled down under the pressure of calcium ions in the MD equilibration simulation. While this may be a less representative conformation for this amino acid, it seems likely that the positively charged ammonium group would remain drawn to the negative residues of the selectivity filter, and thus also obstruct calcium ions from passing through.
Binding mode 2: desipramine-like.
Desipramine (2) and opipramol (9) were found to bind in the cavity between the selectivity filter and the internal gate of CaV2.2 (Fig. 11), in a similar position to where diltiazem was shown to bind in CaV1.1.39 The tricyclic heads of desipramine (2) and opipramol (9) were found to associate primarily with residues from the S6I and S6II segments, as well as Phe1703 on S6IV and Phe1407 and Phe1411 on S6III. The ammonium sidechain of desipramine was predicted to coordinate with Glu1655, while opipramol's piperazinylethanol sidechain appeared to associate with the four segments forming the selectivity filter, P1I, P1II, P1III and P1IV.
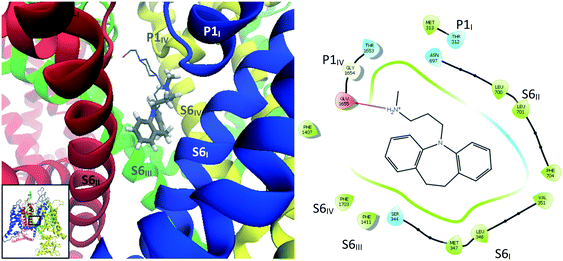 |
| Fig. 11 Images showing binding mode 2 with overlays of binding poses of desipramine (2, tubular representation) and opipramol (9, wire representation) docked into the refined CaV2.2 structure. Left: Side view of docking site. Right: Interaction diagram for desipramine (2). | |
Alternative binding modes.
Trimipramine (6) and cyclobenzaprine (12) (Fig. 12A) were found to localise in a similar region as the amitriptyline/maprotiline cluster, between S6III, S5III and S6IV, but their orientations were reversed, with their ammonium sidechains pointing between S6IV and S5III, making a hydrogen bond with Ser1696 in an otherwise largely hydrophobic environment. This binding mode is expected to be unstable, however, as it seems more plausible that in vivo, cyclobenzaprine in particular would ultimately reorient and bind in a similar way to its closely related analogues imipramine (1), amitriptyline (3) and nortriptyline (5). Doxepin (7) and amoxapine (11) (Fig. 12B) were found to bind in a similar way to 6 and 12. Their ammonium groups were found to make hydrogen bonds with the carbonyl oxygen of Phe1400, while the tricyclic system was seen to be coordinated by residues on S6III and the edges of P1II and P1III, leaving a high degree of exposure to water.
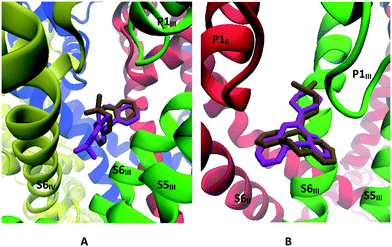 |
| Fig. 12 A: Docking mode of trimipramine (magenta) and cyclobenzaprine (ochre). B: Docking mode of amoxapine (magenta) and doxepin (ochre). | |
Finally, clomipramine (4) (Fig. 13) was found to dock between P1III and P2III, on the extracellular side of the channel. For these last three compounds (4, 7 and 11), it is not clear why they would be active if the identified docking sites were their actual primary in vivo binding sites. Given their close structural similarity with the other TCAs, they might be expected to dock in one of the other sites discussed previously; although it should be noted that doxepin (7) and amoxapine (11) differ from other TCAs by having an oxygen atom in the tricyclic structure and clomipramine (4) is the only halogenated TCA considered in this study. Nonetheless, this highlights that non-inhibiting docking sites for TCAs probably exists, which may contribute to their moderate (double digit micromolar) activities and relatively small differences between measured IC50 values.
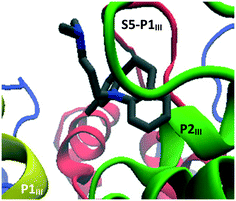 |
| Fig. 13 Docking mode of clomipramine. | |
Molecular dynamics simulations of docked structures.
In order to further refine the structures obtained from the rigid docking experiments, MD simulations were run with five representative CaV2.2–TCA complexes. Structures in which amitriptyline (3), nortriptyline (5) and maprotiline (10) had been docked were taken as representative examples for binding mode 1, and the structure docked with desipramine (2) as representative of binding mode 2. Of the alternative binding modes, the structure docked with trimipramine (6) was considered, as in this case the TCA appeared to be in an unstable pose, and MD simulations were expected to allow it to relax into a more realistic orientation. In each case, the docking pose was copied onto the base model and 150 ns simulations were then carried out. A blank simulation with no ligand was also performed as a reference. No significant conformational shifts of the protein were observed throughout these simulations. In particular, the internal gate remained closed and the backbone RMSD of the transmembrane domain hovered around 2.5 Å, in line with what was observed during the initial equilibration phase described above (Fig. S3, ESI†).
Maprotiline's tetracyclic ring system was found to be very stable in its initially docked position (binding mode 1, Fig. 14), with an average RMSD of 2.27 Å. The main interactions predicted by Glide – Glu1655, Phe1407, Thr1363, Tyr1289, Ser1696, Phe1403, Ile1692 – persisted throughout the simulation. The ammonium group, however, was observed to move closer to the selectivity filter, with the methyl substituent becoming positioned just under the calcium ion.
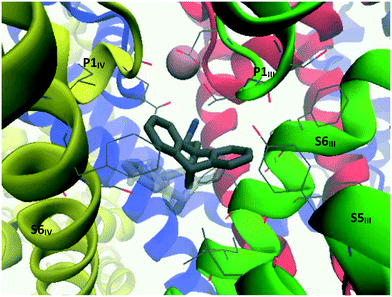 |
| Fig. 14 Image showing the side view of the structure resulting from the MD simulation run on the CaV2.2-maprotiline docked complex. Transparent: maprotiline's starting position predicted through Glide docking; solid: final position. | |
Amitriptyline's tricyclic system was found to rotate by around 60 degrees from its initial docking pose while the ammonium group moved slightly closer to the selectivity filter – as was observed for maprotiline – within the first nanosecond (Fig. 15). From this point onwards it remained in place, with an average RMSD of 2.18 Å. The interactions with Leu1286, Tyr1289 (S5III); Phe1359, Thr1363 (P1III); Phe1403, Phe1406, Phe1407 (S6III); Glu1655 (P1IV); Ile1692, Phe1693 and Ser1696 (S6IV) predicted by the rigid docking also persisted throughout the simulation.
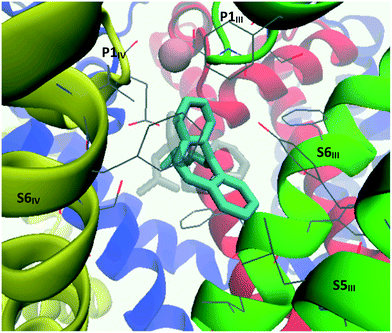 |
| Fig. 15 Image showing the side view of the structure resulting from the MD simulation run on the CaV2.2-amitriptyline docked complex. Transparent: amitriptyline's starting position predicted through Glide docking; cyan: averaged position from the main conformational cluster. | |
When the CaV2.2-desipramine docked complex (binding mode 2) was employed as the starting point for the MD simulation, the TCA was observed to move around the surface of the internal gate; however, cluster analysis – using a RMSD cutoff of 2.0 Å – found a major cluster (magenta, Fig. 16) encompassing most of the conformations observed during 30 to 129 ns of the simulation. In this position, the ligand is coordinated by residues on the four segments forming the internal gate: Met347 (S6I), Leu700, Phe704 (S6II), Phe1407, Val1408, Phe1411 (S6III) and Phe1703 (S6IV). The ammonium tail appeared to help keep the Glu1655 (P1IV) sidechain in its “down” conformation, indicating that it could most likely disrupt the selectivity filter.
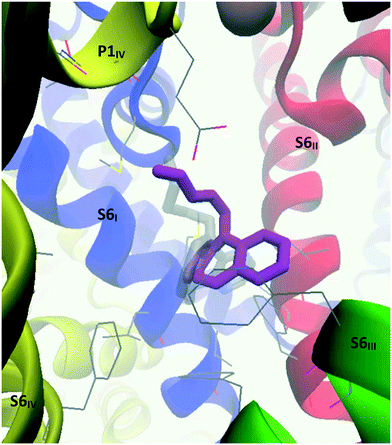 |
| Fig. 16 Image showing the side view of the structure resulting from the MD simulation run on the CaV2.2-desipramine docked complex. Transparent: desipramine's starting position predicted through Glide docking; magenta: desipramine's averaged position from the main conformational cluster identified during 30 to 129 ns of the simulation. | |
Interestingly, while nortriptyline rigidly docked in the same mode as its methylated analogue amitriptyline (binding mode 1), this TCA was observed to drift from the pocket between S6III and S6IV within the initial nanoseconds of the simulation, and settled between S6III and S6II, under P1II (Fig. 17), which is the analogous docking site of verapamil in CaV1.1.39 There nortriptyline markedly obstructs the selectivity filter, with the tricyclic head leaning on hydrophobic residues on these three segments, while its ammonium tail extended to coordinate Met313 on P1I and/or Glu1655 on P1IV. More precisely, cluster analysis using a RMSD cutoff of 2.0 Å revealed three poses; in each case, interactions with the following residues were observed: Pro1404, Phe1407, (S6III), Thr1363 (P1II), Leu700 (S6II), Met313 (P1I), Glu1655 (P1IV). Further details can be found in Table S1 (ESI†).
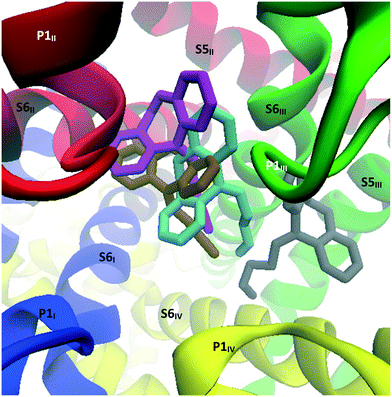 |
| Fig. 17 Image showing the top view of the structures resulting from the MD simulation run on the CaV2.2-nortriptyline docked complex. Grey: nortriptyline's starting position predicted through Glide docking; cyan, ochre and magenta: nortriptyline's main poses observed during MD calculations. These poses are presented separately in the ESI.† | |
When MD calculations were performed with the CaV2.2-trimipramine docked complex (initially considered to be an alternative binding mode), the TCA was observed to flip around within the first 15 ns and adopt a pose more in line with the amitriptyline–maprotiline docking mode (binding mode 1). In this adjusted pose, the ammonium tail is facing the P-loop of the selectivity filter. Three related poses were identified by cluster analysis, as represented in Fig. 18; cluster 1 (cyan) in particular – accounting for approximately 80 ns of the 150 ns simulation – closely aligns with the amitriptyline simulation. The coordinating residues, described in Table S2 (ESI†), are almost identical in each cluster. Interestingly, the cluster 1 and cluster 2 conformations alternated at around 97 ns then 119 ns of simulation time.
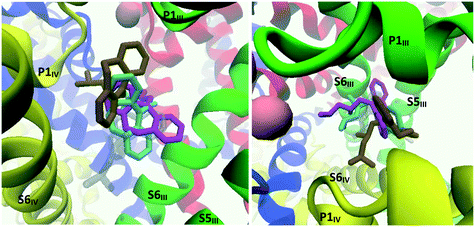 |
| Fig. 18 Image showing the structures resulting from the MD simulation run on the CaV2.2-trimipramine docked complex. Left: Side view; right: top view. Transparent grey: trimipramine's starting position predicted through Glide docking; cyan, ochre and magenta: trimipramine's main poses observed during MD calculations. These poses are presented separately in the ESI.† | |
Conclusions
While it is often stated that the inhibition of neuronal calcium ion channels may be partially responsible for the effectiveness of TCAs in the treatment of neuropathic pain, until now, there has been very little scientific evidence to support this assertion. In the current study, a set of eleven TCAs and two related drugs were evaluated for their ability to inhibit the human N-type or CaV2.2 channel endogenously expressed in the neuroblastoma cell line, SH-SY5Y. The CaV2.2 channel was chosen because, amongst the human neuronal calcium ion channels, the CaV2.2 channel is one of the most well validated targets for neuropathic pain drug development. It was found that all of the test drugs showed moderate inhibition of the hCaV2.2 channel, with those predominantly consisting of a carbon framework and bearing a flexible amine sidechain (2–6, 8, 10 and 12) eliciting slightly stronger responses. These compounds were found to be more than two-fold stronger inhibitors of the hCaV2.2 channel than the positive control, cilnidipine, a N-type channel blocker that has been shown to effectively suppress nociception in vivo.43 Other structure–activity relationships were also revealed and together may provide useful guidance in the development of more effective drugs for neuropathic pain based on the TCA core structure. Importantly, for the purposes the current study, the inhibition results provide further validation for the off-label prescription of TCAs for neuropathic pain. The magnitude of the obtained IC50s, bordering on single digit micromolar activity, while generally superior to that produced by cilnidipine, does however support the notion that the observed therapeutic effect of certain TCAs in the treatment of neuropathic pain does not solely result from their ability to block the CaV2.2 channel. Indeed, TCAs are known to be relatively promiscuous drugs and are thought to elicit their pain killing effect through a range of channels and receptors. While, in the presence of nifedipine, the vast majority of calcium responses in the SHSY5Y cell line have been shown to be due to CaV2.2 channel activity, a residual signal for T-type calcium channels has been identified.29 The complete block of calcium channel activity at high TCA concentrations (data not shown) therefore points to likely T-type inhibition by these drugs. This property of TCAs, as well as potential state-dependent effects and ion channel subtype selectivities, are currently being investigated in detail.
In order to gain insights into the mechanism of action of TCAs on the CaV2.2 channel, an in silico model of the pore-forming α1 subunit of the CaV2.2 channel, previously constructed from a static rabbit CaV1.1 single-particle Cryo-EM map, was used as a starting point. The homology model was embedded into a simulated cell membrane, and MD simulations, which incorporated a simulated calcium chloride solution, were used to model channel behaviour. The transmembrane component of the model thus obtained compared favourably with a recently reported Cryo-EM structure of the human CaV2.2 channel. Rigid docking of the selected TCAs, followed by further MD simulations, revealed two plausible binding sites for TCAs in the human CaV2.2 channel. These correspond to identified binding sites for known blockers of other calcium channels, CaV1.1 and CaV3.1, revealed in recent Cryo-EM studies. The two binding sites in the CaV2.2 channel found with desipramine and maprotiline are illustrated in Fig. 19.
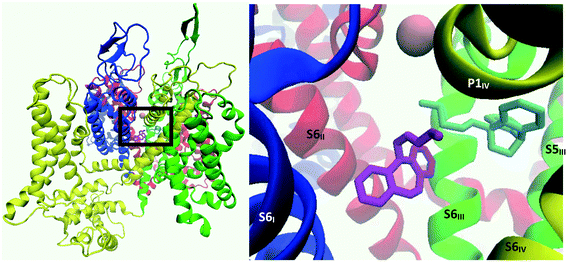 |
| Fig. 19 Structure of the CaV2.2 channel at 150 ns simulation time. Left: The black rectangle indicates the general region of the CaV2.2 channel where the TCAs were predicted to bind. Right: Maprotiline (cyan) and the representative desipramine cluster (magenta), highlighting the two main TCA binding modes found in this study. | |
Desipramine and opipramol prefer to bind between the four S6 segments. Like diltiazem in CaV1.1 and Z944 in CaV3.1,40 desipramine appears to inhibit the channel by physically obstructing the pore, preventing calcium ions from passing through. Maprotiline and most other TCAs were predicted to settle in a pocket formed between S5III, S6III and S6IV, in a similar manner to nifedipine in CaV1.1.39 Dihydropyridines like nifedipine are state-dependent allosteric inhibitors of L-type calcium channel (CaV1) with higher affinity for the inactivated states in which the internal gate remains closed.44 By interacting with two of the S6 segments forming the internal gate, these compounds are thought to prevent the necessary twisting and bending of the S6 segments to widen the pore,45 therefore keeping the internal gate locked and preventing the calcium ions from passing through. Presumably maprotiline and the other TCAs predicted to bind in this site act in a similar way.
Comparison of the refined homology model described here with the recently resolved Cryo-EM structure of CaV2.2 revealed a high degree of similarity, supporting the validity of the modelling undertaken. Calcium ion channel homology models built from closely related calcium channels, and for which there are no experimentally-obtained structures, can therefore be expected to provide highly accurate structures useful for docking and MD studies, as the examples here demonstrate.
Experimental section
Biological evaluation
All of the test drugs were obtained from Sigma-Aldrich Pty Ltd except desipramine (2), which was obtained from Toronto Research Chemicals. All were hydrochloride salts except trimipramine (6), which was a maleate salt, and amoxapine (11) which was unprotonated. The bioactivity of the test drugs and inhibition control cilnidipine (Alomone, Jerusalem, Israel) were evaluated by calcium influx assays using the Fluorescence-Imaging Plate Reader Tetra (FLIPRTETRA, Molecular Devices, CA, USA). Calcium influx measures were used for the evaluation of CaV2.2 channels endogenously expressed in the neuroblastoma SH-SY5Y. Briefly, neuroblastoma SH-SY5Y cells were seeded at 40
000 and 10
000 cells per well in 384 well flat clear-bottom black plates (Corning, NY, USA) and cultured at 37 °C in a humidified 5% CO2 incubator 48 h before assay. Cells were loaded with 20 μL per well of Calcium 4 dye (Molecular Devices) reconstituted in assay buffer containing (in mM) 140 NaCl, 11.5 glucose, 5.9 KCl, 1.4 MgCl2, 1.2 NaH2PO4, 5 NaHCO3, 1.8 CaCl2,10 HEPES pH 7.4 and 0.1% bovine serum albumin (BSA, Sigma), and incubated for 30 min at 37 °C in a humidified 5% CO2 incubator. Nifedipine 10 μM (CaV1 blocker) was added to the dye solution. The Ca2+ fluorescence responses were recorded at excitation 470–495 nm and emission 515–575 nm for 10 s to set the baseline, 300 s after addition of compound and for further 300 s after channel activation induced by the addition of 90 mM KCl and 5 mM CaCl2. Compound stock solutions were prepared at 100 mM in 100% DMSO and diluted further in the assay buffer to 1% DMSO, for the highest tested concentration of 100 μM of compound, and serial-diluted 3-fold in assay buffer.
In silico studies
Model construction.
The CaV2.2 homology model created by Martinez-Ortiz and Cardozo33 was obtained from their public GitHub repository.46 The structure was then reoriented using the Position of Proteins in Membranes (PPM) server47 to place the centre of the protein at the origin and the membrane normal parallel to the Z axis. The CHARMM-GUI server35 was then used to generate a membrane containing approximately 15% cholesterol and 85% POPC and solvate the system in a solution of 0.15 M sodium chloride, using the TIP3P water model. A total of 168 cholesterol molecules, 952 POPC molecules, 181
681 water molecules (TIP3P), 507 sodium ions and 507 chloride ions were added in an orthorhombic cell of dimensions 201.6 × 201.6 × 185.4 Å3.
All following MD simulations were then performed employing the NAMD 2.13 MD package with the CHARMM36 force field, under NPT conditions in a periodic box, with the temperature (310 K) and pressure (1.01325 bar) maintained using Langevin dynamics. A cut-off distance for interactions was set at 12 Å and a timestep of 2 fs.48 Calculations were performed on the M3G partition of the MASSIVE M3 cluster at Monash University using 12 cores of an Intel Xeon Gold 6150 processor and one Nvidia Tesla V100 GPU.49 Post-simulation analysis and further refining of the model was carried out using the VMD package.50
After a preliminary 1000 step minimisation, a 18
000
000-step simulation (36 ns) with no constraints was then launched. After extracting the state of the system at the end of this first simulation, the water and ions were removed, and a 0.15 M solution of calcium chloride was regenerated at a 4 Å distance from the protein and membrane molecules. A total of 165
658 water molecules, 469 calcium ions and 938 chloride ions were thus added in an orthorhombic cell of dimensions 200 × 200 × 180 Å3. A second simulation was then set to run for 30
000
000 steps (60 ns).
Docking.
The protein and TCA structures were imported in the Schrödinger Maestro suite,51 and a receptor grid of 26 × 26 × 26 Å3 centred on the selectivity filter residues Glu314, Glu663, Glu1365 and Glu1665 was generated. The ligands were then docked using the Glide subprogram.52
Molecular dynamics.
The docked conformations of maprotiline, amitriptyline, desipramine, nortriptyline and (R)-trimipramine were extracted and the SWISS-Param53 online server was used to generate parameters for the following MD simulations. The system size was reduced to comprise 376 POPC molecules, 59 cholesterol molecules, 41
489 water molecules, 11 calcium ions, 117 sodium ions, 140 chlorine ions and the ligand, in a cell of dimensions 140 × 140 × 120 Å3. Simulations of 150 ns for each CaV2.2–TCA complex (as well as a control simulation with no ligand) were then launched in parallel using the same parameters as in the previous section. Cluster analysis of the TCAs over the course of the simulation was carried out using the Clustering VMD plugin,54 using an RMSD cutoff of 2 Å.
Conflicts of interest
The authors declare no competing financial interest.
Acknowledgements
Monash University and CSIRO are acknowledged for funding. This work was also supported by a NHMRC Program Grant (APP1072113, RJL) and NHMRC Fellowship (1119056, RJL). We gratefully acknowledge Dr David Chalmers (MIPS, Monash University, Australia) for providing access to Schrödinger software. This research was also undertaken with the assistance of resources and services from Multi-modal Australian ScienceS Imaging and Visualisation Environment (MASSIVE), the National Computational Infrastructure (NCI) and CSIRO's High Performance Computing, which are all supported by the Australian Government. The School of Chemistry, Monash University is acknowledged from providing MS with a Faculty of Science Dean's Postgraduate Research Scholarship.
Notes and references
- F. Paoli, G. Darcourt and P. Cossa, Rev. Neurol., 1960, 102, 503–504 CAS.
- S. H. Sindrup and T. S. Jensen, Pain, 1999, 83, 389–400 CrossRef CAS.
- M. Namaka, C. R. Gramlich, D. Ruhlan, M. Melanson, I. Sutton and J. Major, Clin Ther, 2004, 26, 951–979 CrossRef.
- B. Verdu, I. Decosterd, T. Buclin, F. Stiefel and A. Berney, Drugs, 2008, 68, 2611–2632 CrossRef CAS.
- C. S. Zin, L. M. Nissen, M. T. Smith, J. P. O'Callaghan and B. J. Moore, CNS Drugs, 2008, 22, 417–442 CrossRef CAS.
- N. B. Finnerup, N. Attal, S. Haroutounian, E. McNicol, R. Baron, R. H. Dworkin, I. Gilron, M. Haanpää, P. Hansson, T. S. Jensen, P. R. Kamerman, K. Lund, A. Moore, S. N. Raja, A. S. C. Rice, M. Rowbotham, E. Sena, P. Siddall, B. H. Smith and M. Wallace, Lancet Neurol., 2015, 14, 162–173 CrossRef CAS.
- D. Fornasari, Pain Ther., 2017, 6(Suppl 1), S25–S33 CrossRef.
- J. Wong, A. Motulsky, M. Abrahamowicz, T. Eguale, D. L. Buckeridge and R. Tamblyn, BMJ, 2017, 356, j603 CrossRef.
- S. Sindrup, M. Otto, N. B. Finnerup and T. S. Jensen, Basic Clin. Pharmacol. Toxicol., 2005, 96, 399–409 CrossRef CAS.
- F. Coluzzi and C. Mattia, Curr. Pharm. Des., 2005, 11, 2945–2960 CrossRef CAS.
- J. W. Phillis and P. H. Wu, Comp. Biochem. Physiol., C: Comp. Pharmacol., 1982, 72, 179–187 CrossRef CAS.
- J.-H. Song, S.-S. Ham, Y.-K. Shin and C.-S. Lee, Eur. J. Pharmacol., 2000, 401, 297–305 CrossRef CAS.
- Y. D. Stepanenko, S. I. Boikov, D. A. Sibarov, P. A. Abushik, N. P. Vanchakova, D. Belinskaia, N. N. Shestakova and S. M. Antonov, Sci. Rep., 2019, 9, 19454 CrossRef.
- P.-A. Lavoie, G. Beauchamp and R. Elie, Can. J. Physiol. Pharmacol., 1990, 8, 1414–1418 CrossRef.
- C. Boselli, M. S. Barbone and A. Lucchelli, Can. J. Physiol. Pharmacol., 2007, 85, 1004–1011 CrossRef CAS.
- E. R. Benjamin, F. Pruthi, S. Olanrewaju, S. Shan, D. Hanway, X. Liu, R. Cerne, D. Lavery, K. J. Valenzano, R. M. Woodward and V. I. Ilyin, Biochem. Pharmacol., 2006, 72, 770–782 CrossRef CAS.
- B. Colombo, P. O. L. Annovazzi and G. Comi, Neurol. Sci., 2006, 27, S183–S189 CrossRef.
-
P. Beswick, 7.03 – Progress in the Discovery of Ca Channel Blockers for the Treatment of Pain A2 – Chackalamannil, Samuel, in Comprehensive Medicinal Chemistry III, ed. D. Rotella and S. E. Ward, Elsevier, Oxford, 2017, pp. 65–130 Search PubMed.
- J. Yang, P. T. Elllnor, W. A. Sather, J.-F. Zhang and R. W. Tsien, Nature, 1993, 366, 158–161 CrossRef CAS.
- S. Hering, E.-M. Zangerl-Plessl, S. Beyl, A. Hohaus, S. Andranovits and E. N. Timin, Pflugers Arch., 2018, 470, 1291–1309 CrossRef CAS.
- H. Safavi-Hemami, S. E. Brogan and B. M. Olivera, J. Proteomics, 2019, 190, 12–20 CrossRef CAS.
- P. J. Duggan and K. L. Tuck, Toxins, 2015, 7, 4175–4198 CrossRef CAS.
- J. R. McArthur, L. Motin, E. C. Gleeson, S. Spiller, R. J. Lewis, P. J. Duggan, K. L. Tuck and D. J. Adams, Br. J. Pharmacol., 2018, 175, 2284–2295 CrossRef CAS.
- A. Sairaman, F. C. Cardoso, A. Bispat, R. J. Lewis, P. J. Duggan and K. L. Tuck, Bioorg. Med. Chem., 2018, 26, 3046–3059 CrossRef CAS.
- F. C. Cardoso, M.-A. Marliac, C. Geoffroy, M. Schmit, A. Bispat, R. J. Lewis, K. L. Tuck and P. J. Duggan, Bioorg. Med. Chem., 2020, 28, 115655 CrossRef CAS.
-
T. Snutch, US Pat., 6310059B1, NeuroMed Technologies, Inc., 2001 Search PubMed.
- S. Gao, X. Yao and N. Yan, Nature, 2021, 596, 143–147 CrossRef CAS.
- I. Vetter and R. J. Lewis, Biochem. Pharmacol., 2010, 79, 908–920 CrossRef CAS.
- S. R. Sousa, I. Vetter, L. Ragnarsson and R. J. Lewis, PLoS One, 2013, 8, e59293 CrossRef CAS.
- T. Yamamoto, S. Ohno, S. Niwa, M. Tokumasu, M. Hagihara, H. Koganei, S.-I. Fujita, T. Takeda, Y. Saitou, S. Iwayama, A. Takahara, S. Iwata and M. Shoji, Bioorg. Med. Chem. Lett., 2011, 21, 3317–3319 CrossRef CAS.
-
https://www.pbs.gov.au/pbs/home
.
-
https://www.accessdata.fda.gov/scripts/cder/ob/index.cfm
.
- W. Martinez-Ortiz and T. J. Cardozo, Cell Rep., 2018, 23, 1399–1408 CrossRef CAS.
- J. Wu, Z. Yan, Z. Li, X. Qian, S. Lu, M. Dong, Q. Zhou and N. Yan, Nature, 2016, 537, 191–196 CrossRef CAS.
- S. Jo, T. Kim, V. G. Iyer and W. Im, J. Comput. Chem., 2008, 29, 1859–1865 CrossRef CAS.
- E. L. Wu, X. Cheng, S. Jo, H. Rui, K. C. Song, E. M. Dávila-Contreras, Y. Qi, J. Lee, V. Monje-Galvan, R. M. Venable, J. B. Klauda and W. Im, J. Comput. Chem., 2014, 35, 1997–2004 CrossRef CAS.
- H. Martinez-Seara, T. Róg, M. Karttunen, I. Vattulainen and R. Reigada, PLoS One, 2010, 5, e11162 CrossRef.
- T. Feng, S. Kalyaanamoorthy, A. Ganesan and K. Barakat, Biochim. Biophys. Acta, Gen. Subj., 2019, 1863, 1116–1126 CrossRef CAS.
- Y. Zhao, G. Huang, J. Wu, Q. Wu, S. Gao, Z. Yan, J. Lei and N. Yan, Cell, 2019, 177, 1495–1506.e12 CrossRef CAS.
- Y. Zhao, G. Huang, Q. Wu, K. Wu, R. Li, J. Lei, X. Pan and N. Yan, Nature, 2019, 576, 492–497 CrossRef CAS.
- R. Chen and S.-H. Chung, Biochemistry, 2013, 52, 3765–3772 CrossRef CAS.
- H. Shen, D. Liu, K. Wu, J. Lei and N. Yan, Science, 2019, 363, 1303–1308 CrossRef CAS.
- H. Koganei, M. Shoji and S. Iwata, Biol. Pharm. Bull., 2009, 32, 1695–1700 CrossRef CAS.
- M. C. Sanguinetti and R. S. Kass, Circ. Res., 1984, 55, 336–348 CrossRef CAS.
- M. J. Lenaeus, T. M. Gamal El-Din, C. Ing, K. Ramanadane, R. Pomès, N. Zheng and W. A. Catterall, Proc. Natl. Acad. Sci. U. S. A., 2017, 114, E3051–E3060 CrossRef CAS.
-
https://github.com/CardozoLab/Voltage-Gated-Calcium-Channels-/blob/master/README.md
.
- M. Lomize, I. D. Pogozheva, H. Joo, H. I. Mosberg and A. L. Lomize, Nucleic Acids Res., 2012, 40, D370–D376 CrossRef CAS.
- J. C. Phillips, D. J. Hardy, J. D. C. Maia, J. E. Stone, J. V. Ribeiro, R. C. Bernardi, R. Buch, G. Fiorin, J. Henin, W. Jiang, R. McGreevy, M. C. R. Melo, B. K. Radak, R. D. Skeel, A. Singharoy, Y. Wang, B. Roux, A. Aksimentiev, Z. Luthey-Schulten, L. V. Kale, K. Schulten, C. Chipot and E. Tajkhorshid, J. Chem. Phys., 2020, 153, 044130 CrossRef CAS.
- W. J. Goscinski, P. McIntosh, U. Felzmann, A. Maksimenko, C. J. Hall, T. Gureyev, D. Thompson, A. Janke, G. Galloway, N. E. Killeen, P. Raniga, O. Kaluza, A. Ng, G. Poudel, D. G. Barnes, T. Nguyen, P. Bonnington and G. F. Egan, Front. Neuroinform., 2014, 8, 30 Search PubMed.
- W. Humphrey, A. Dalke and K. Schulten, J. Mol. Graphics, 1996, 14, 33–38 CrossRef CAS.
- Schrödinger Release 2020–3: Maestro, Schrödinger, LLC, New York, NY, 2021.
- R. A. Friesner, J. L. Banks, R. B. Murphy, T. A. Halgren, J. J. Klicic, D. T. Mainz, M. P. Repasky, E. H. Knoll, M. Shelley, J. K. Perry, D. E. Shaw, P. Francis and P. S. Shenkin, J. Med. Chem., 2004, 47, 1739–1749 CrossRef CAS.
- V. Zoete, M. A. Cuendet, A. Grosdidier and O. Michielin, J. Comput. Chem., 2011, 32, 2359–2568 CrossRef CAS.
-
https://github.com/luisico/clustering
.
Footnotes |
† Electronic supplementary information (ESI) available. See DOI: 10.1039/d1md00331c |
‡ FCC and MS contributed equally to this work. |
|
This journal is © The Royal Society of Chemistry 2022 |