DOI:
10.1039/D2MA00044J
(Paper)
Mater. Adv., 2022,
3, 5532-5539
Computational understanding role of vacancies and distortions in wurtzite ferroelectric memory materials: implications for device miniaturization†
Received
14th January 2022
, Accepted 27th May 2022
First published on 10th June 2022
Abstract
The ever-increasing importance of applications based on machine-learning has driven the need to develop delicate energy-efficient electronic hardware. Compared to traditional von-Neumann architectures, which contain separate main-memory and storage units, nonvolatile edge computing utilizes the same device structure for data storage and rapid operations, therefore promising to decrease the energy costs of data-centered computing significantly. Although various research has focused on exploration of new device architectures, engineering material systems suitable for these device designs remains challenging. Ferroelectric materials (FEM) are leading contenders for these systems because of their excellent materials and electronic properties. Here, we demonstrate how the role of composition and distortion in structural signatures of FEM can be studied using density functional theory (DFT). The previously unreported origins of vacancies and distortions are revealed, and we show that the stable AlScN model with wurtzite structure is described by small/negligible vacancy concentrations and minimal localized distortions. Additionally, the low vacancy populations formed can be explained by the need to remove energetically-unfavorable anti-bonding Al–N and Sc–N interactions near the Fermi level. Theoretical studies elucidate a vacancy-facilitated decrease in leakage currents. These results provide deeper insights that can help to rationalize the design of next-generation low-power electronic systems.
Introduction
The ever-increasing demand for more data intensive applications in areas such as machine-learning and internet-of-things requires energy-efficient hardware for tasks including speech recognition, autonomous driving and image processing.1–3 As these applications need energy-efficient and high-performance computations, the memory and power limitations imposed by conventional von-Neumann computers, using separate main-memory and storage units, limit the ability of traditional processors to meet optimized requirements for these applications.4 Thus, the development of next-generation hardware has been an important area of research.5–7 Among them, edge computing, utilizing the same device structure for rapid operations and data storage, is presenting itself as an excellent hardware design to tackle portable, data intensive applications.8 The success of this approach depends on finding an excellent material platform capable of utilizing the full potential of this design.
Ferroelectric materials (FEM) are considered as a candidate material platform for achieving next-generation edge computing thanks to their minimized thickness and enhanced electrical control. FEM are also promising candidates for important applications, such as three-dimensional (3D) cross-point memories,9,10 hardware-based neural networks,11 in-memory computing,12,13 as well as optical and optoelectronic devices, including optical waveguides14 and piezoelectric sensors.15 Ferroelectric (FE) memory operations, based on the reversible switching between one orientation state to another in a nitride/oxide-based material, showing marked contrast in electrical resistivity, generally requires less energy and offers shorter switching times as well as larger maximum switching endurance.16 However, a technological challenge arises from decreasing the leakage current (weak depolarization process for long-term data retention), and at the time, minimizing the thin-film thickness (thin active layer for facilitating device downsizing).17 This has prevented widespread FEM commercialization.
Experiments have demonstrated that reactively sputtered FE thin films grow with microscopic inhomogeneities and defects such as vacancies.18,19 Moreover, microscopic models of FEM, e.g., AlScN-based systems, have recently been achieved by simulations.20–23 Additionally, calculations have demonstrated that AlN models with nitrogen vacancy at the interior/subsurface locations showed larger formation energies than AlN models with nitrogen vacancy at the surface location, because the formation of nitrogen vacancy at the surface location involves the breaking of a smaller number of Al–N bonds than that for the formation of nitrogen vacancy at interior or subsurface locations.24 However, very little is known about the role of distortions (e.g., the effects of structural relaxation on bonding/formation-energy properties) and vacancies in FE thin films. Besides, there could be a connection between the leakage current and change in bonding/electronic character for different defect concentrations. Herein, we examine the role of composition and distortion in structural signatures of FEM through computational strategy, i.e., via examining models with different concentrations of Al, Sc and N vacancies and with distortions, and characterizing their electronic/bonding characters using ab initio density functional theory (DFT). The models can elucidate previously unrevealed origin of the vacancies and distortions, and they demonstrate that the stable AlScN models with wurtzite structure is characterized by small/negligible concentrations of vacancies and minimal localized distortions. In addition, the low vacancy populations formed can be explained by the need to remove energetically-unfavorable anti-bonding Al–N and Sc–N interactions near the Fermi level. These calculations are also extended to a previously unconsidered one-hundred-and-twenty-eight-atom AlScN model to enhance the quality of the findings on atomistic nature of vacancies. Furthermore, theoretical studies reveal a vacancy-facilitated decrease in leakage currents, providing deeper insights to further enhance the performance of low-energy systems using FEM.
Method
DFT calculations
The first-principles calculations were performed using the pw.x code of Quantum Espresso software.25 The Perdew–Burke–Ernzerhof (PBE) exchange-correlation functionals were utilized.26 Projector augmented wave method (PAW)-type pseudopotentials for Al, N and Sc atoms were used. The crystal model was built by the special quasi-random structure (SQS) method,27,28 using the mcsqs code implemented in the alloy theoretic automated toolkit (ATAT) package.29 Brillouin-zone integrals for the 32-atom models were evaluated on a Monkhorst–Pack mesh of 6 × 6 × 4 k-points with a Gaussian smearing of 0.01 Ry. For calculations of total energies, a finer 8 × 8 × 5 k-point grid was employed. In defect-formation energy (Eform) calculations, the convergence threshold was set to 10−6 Ry. The cutoff energy of the plane-wave basis set was chosen to be 40 Ry. The 128-atom models were calculated using a Monkhorst–Pack mesh of 2 × 4 × 2 k-points for the relaxed processes. For calculations of the total energies and partial density of states (PDOS), a 3 × 6 × 2 k-point grid was harnessed. The structures were relaxed with the Broyden–Fletcher–Goldfarb–Shanno algorithm. Pair-correlation-function (PCF) analysis of structural features of the AlScN models were generated using an open visualization tool (OVITO).30 The models with N vacancies were created via removing N atoms from different interior locations (sites surrounded by 3 Sc and 1 Al atoms, 2 Sc and 2 Al atoms, or 1 Sc and 3 Al atoms) (Fig. S1 and S2, ESI†). Similar formation energies and electronic structures were disclosed by models with a specified N vacancy concentration. The model with a targeted N vacancy concentration showing the lowest formation energy was utilized for detailed discussion.
COHP analysis and electronic-structure calculations
The crystal-orbital-Hamilton-population (COHP) was computed using the local-orbital-basis-suite-towards-electronic-structure-reconstruction (LOBSTER) software.31,32 The COHP data were obtained from PAW functions via analytical projection onto an atomic-like basis sets utilized in the software. The software subsequently rebuilds the entire PAW function through the linear combination of atomic orbitals methods. Bunge basis sets33 (Al, 3s and 3p; Sc, 4s, 3p and 3d; N, 2s and 2p) were utilized. The electronic-structure computations were performed by non-spin-polarized techniques with more than 8 × 8 × 5 k-points in the irreducible Brillouin zones of the model. Visualizations of the model structures were performed using the Xcrysden34 and VESTA software.35
Results
Model structure and validation of AlScN system
The AlScN has been considered as a contender material platform for next-generation FE hardware. AlScN materials have been utilized for developing nonvolatile FE memory devices and frequency-tunable acoustic resonators.36 Recently, experiments have demonstrated, for the first time, ferroelectricity in AlScN thin films, which show larger remanent polarizations compared to perovskite/fluorite-based FEM.18 Moreover, increasing Sc alloying levels lead to ferroelectric switching observed under small electric fields.37 Additionally, experiments have focused on improving piezoelectric properties of aluminum-nitride through incorporating different concentrations of Sc atoms.38
We have examined the role of vacancies in AlxSc0.2–0.3Ny. Studies have demonstrated that Al0.78Sc0.22N exhibits a N vacancy concentration of ∼3.3–3.5 × 1012 vacancies per cubic centimeters/20–25% N vacancies.39 Based on these results and to understand structural and electronic signatures that may be associated with small leakage currents, we performed simulations of AlScN models with 6.3–25% N vacancies. To perform this, we have utilized a pristine Al0.75Sc0.25N model with a wurtzite structure and using 32 atoms (12 atoms of Al, 4 atoms of Sc and 16 atoms of N) in the computational model (Fig. 1). The lattice parameter a of the model was calculated based on a statistical distribution of Al and Sc atoms on one sub-lattice of the wurtzite system, where the second sub-lattice contained the N atoms. This results in the lattice parameters, i.e., a = 3.22 Å and c = 5.07 Å, which agrees well with the experimental data and theoretical calculations.40,41 Moreover, the Sc concentration dependent lattice parameters of the model agree well with the experimental data and theoretical calculations (Fig. S3, ESI†).
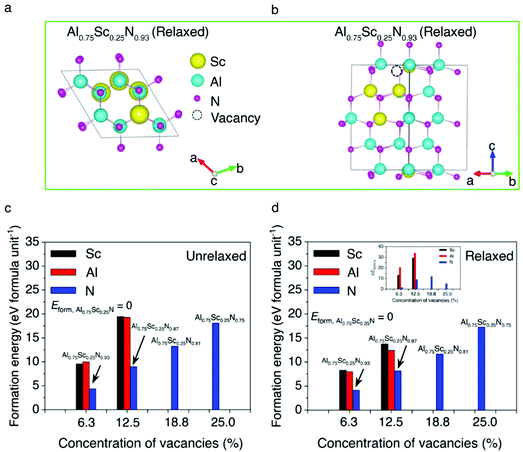 |
| Fig. 1 Wurtzite structure and defect formation energies of the AlScN systems with vacancies. (a) Top and (b) side-view snapshots of the AlScN system. Sublattice A contains N atoms (magenta), and sublattice B comprises Sc atoms (yellow) and Al atoms (cyan). (c and d) Formation energies for different concentrations of Al, Sc and N vacancies for the (c) unrelaxed and (d) relaxed models. Inset in (d) shows the plot of percentage decrease in defect formation energy as a function of vacancy concentrations. There is no data for the models with Al and Sc vacancy concentrations between 18.8% and 25.0% because these models can show large structural changes. | |
Defect formation energy of AlScN models with vacancies
Experiments and theoretical studies have demonstrated that the wurtzite AlScN structure is characterized by distortions around the ideal atomic positions of wurtzite system in Al0.5Sc0.5N.39 Nevertheless, in this work, all of the energies were compared with the energy of wurtzite structure of Al0.75Sc0.25N. In the computations, an increased number of Al, Sc or N atoms were removed from the Al0.75Sc0.25N supercell. The formation energies of the resultant compositions were computed via |  | (1) |
where Etot(Al0.75Sc0.25N) and Etot(x) denote the total energies of the models with the pristine composition Al0.75Sc0.25N and of the composition that results from the removal of Al, Sc or N atoms. Moreover, μi is the energy of the respective crystalline reservoir of Al, Sc or N, and ni denotes the number of vacancies generated upon the removal of atoms. Therefore, niμi is the energy of ni atoms in the elemental, crystalline reservoir.42
Fig. 1c and d shows the results of the Eform upon the removal of Al, Sc and N atoms for the structurally unrelaxed and relaxed (distorted) wurtzite AlScN structures. We find that the Eform of Al0.75Sc0.25N models increases after removing N atoms from Al0.75Sc0.25N models, such that the removal of N atoms from Al0.75Sc0.25N systems is not energetically favorable. Additionally, Fig. 1c and d discloses that it is unfavorable to remove multiple N atoms from Al0.75Sc0.25N models. Another point from Fig. 1 is that lattice distortions play a role (see change in Eform as the Al0.75Sc0.25N crystal is changed from the unrelaxed state to relaxed state). They also lead to a decrease in Eform. Moreover, the removal of N atoms is favored over removing the Al/Sc atoms. In subsequent computations, we have calculated the change in Eform upon the removal of Al atoms. Similar to the case of N removal, the Eform of Al0.75Sc0.25N models is increased by the removal of Al atoms. The Eform also decreases upon structural relaxation. Furthermore, removal of Sc atoms increases the Eform of Al0.75Sc0.25N models.
Thus, the analysis of Al0.75Sc0.25N reveals that the Eform of AlScN structures can be controlled by (1) removal of N, Al or Sc atoms and (2) localized distortions of the wurtzite system, which increases and decreases the Eform, respectively. Motivated by the finding that Al0.75Sc0.25N models with N vacancies show a small Eform, we will discuss the findings in Fig. 1 and role of these phenomena for the Al0.75Sc0.25N0.93–Al0.75Sc0.25N0.75 series. This could be understood in a conceptual framework based on bonding/anti-bonding states and their related energies.
COHP bonding analysis of AlScN models with N vacancies
To investigate the bonding character of AlScN systems, quantum-chemical computations were utilized. Studies have shown that phase-change-materials (PCM-) based models, e.g., GeTe-, InTe- and Sb2Te3-based systems, demonstrate strong antibonding states at the Fermi level for different bonds via COHP analyses.43–45 Additionally, decreasing antibonding states close to the Fermi level with an increase in vacancy concentration is disclosed by GeSbTe-based models.46Fig. 2 shows the COHP curves of the Sc–N and Al–N interactions in the systems of Al0.75Sc0.25N0.93, Al0.75Sc0.25N0.81 and Al0.75Sc0.25N0.75 under relaxed state. The model Al0.75Sc0.25N0.93 is characterized by negligible/low antibonding Sc–N and Al–N interactions near the Fermi level Ef (shaded region just below the horizontal line E = 0 eV), which indicates a negligible/small concentration of free carriers/valence electrons.47 Valence electron concentrations increases with a decrease in material stability,47 for instance, upon the removal of N atoms. In Al0.75Sc0.25N0.81, the population of anti-bonding states is similar/increased, whereas for Al0.75Sc0.25N0.75, it increases further such that the COHP shows a large population of antibonding states in the vicinity of Fermi level.
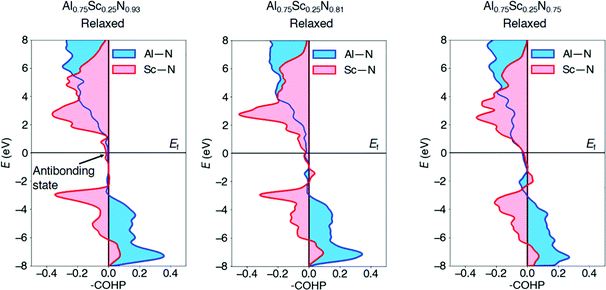 |
| Fig. 2 COHP analysis of AlScN models with N vacancies. The COHP of average values of Al—N (blue regions) and Sc—N (red regions) interactions in the Al0.75Sc0.25N0.93, Al0.75Sc0.25N0.81 and Al0.75Sc0.25N0.75 for the relaxed structures. The Fermi levels Ef were to set to zero energy. The right and left sides of the plot show bonding and anti-bonding interactions, respectively. | |
We further examined the bonding character of AlScN systems using the integrated-crystal-orbital-Hamilton-population (ICOHP). The numerical bond-strength ICOHP analysis yields that, for the relaxed structures, in going from original Al0.75Sc0.25N0.93 to Al0.75Sc0.25N0.75, the Sc–N bonds weakens on average by ∼11.27%. The Al–N bonds also weakens on average by ∼8.69% as the structure is changed from Al0.75Sc0.25N0.93 to Al0.75Sc0.25N0.75 (Tables S1 and S3, ESI†). This is because antibonding states are occupied.48 Thus, the energetic gain by occupying antibonding states results in a N-poor composition such as Al0.75Sc0.25N0.75. Moreover, after structural relaxation, the Sc–N bonds strengthens on average by ∼0.014% and 1.61% for the compositions Al0.75Sc0.25N0.93 and Al0.75Sc0.25N0.75, respectively. In the structures of Al0.75Sc0.25N0.93 and Al0.75Sc0.25N0.75, the Al–N bonds also strengthens on average by ∼1.40% and 0.067% (Tables S1–S5, ESI†). This may be due to the emptying of antibonding-states.48
Local structural features of AlScN models with vacancies
In the following, we discuss the phenomenon that drives further changes in bonding characters of the AlScN system for different concentrations of vacancies. To understand its behavior, Fig. 3 and Fig. S5 (ESI†) disclose the PCF for N–N, Al–Al, Sc–N, Sc–Sc and Al–N bonds for two different AlScN models (Al0.75Sc0.25N0.93, Al0.75Sc0.25N0.75) in the relaxed state. The models exhibit bonding character changes with composition for N–N bond, which results in the splitting into shorter and longer N–N bonds (the first peak for N–N bond broadens as the model is changed from Al0.75Sc0.25N0.93 to Al0.75Sc0.25N0.75). A similar finding is observed for the Al–Al, Sc–N, Sc–Sc and Al–N bonds, although with different degrees of splitting into shorter/longer bonds. These results indicate an interplay between vacancies and bonding-character-changes in AlScN models.
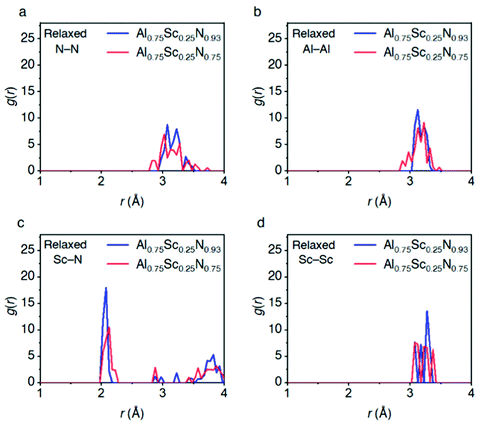 |
| Fig. 3 PCF analysis of structural features of the AlScN models with N vacancies. (a–d) PCF analyses for the (a) N–N, (b) Al–Al, (c) Sc–N and (d) Sc–Sc bonds for the systems with different concentration of N vacancies. The systems were structurally relaxed. | |
Theoretical studies
A binding model can be utilized to describe the origin of bonding-character-change for AlScN systems. Sc is a group-III transition element and show an oxidation state of +3. The Sc is similar to Al, i.e., it is isovalent. Thus, replacing the Al atoms with Sc would maintain the insulating behavior of AlN. The outermost electrons of Sc, which are in d-orbitals rather than the s and p-orbitals of Al, could lead to a difference when it is incorporated in the crystals. Since there are 5d-orbitals: dxy, dyz, dzx, dx2 and dx2−y2, the crystal field can split the energy levels of d-orbitals of Sc atom into t2g and e2g orbitals. Therefore, the stable structure of ScN is cubic-rocksalt, and not wurtzite. Since the crystal structure of ScN is cubic, it is expected that the effects of incorporation of Sc into AlN would be to distort the lattice structure from wurtzite system (unrelaxed state) towards cubic structure (relaxed state). A qualitative conclusion could also be achieved without electronic structure computation: the low-coordination number of elements in the models under relaxed/distorted state leads to non-bonding electronic states.46 Scandium, for example, has 1 valence 3d electron, an indication of instability of the wurtzite structure. Thus, 1 d-orbital can be represented as a non-bonding orbital occupied by 1 electron. N has 3 p-orbitals. This is because its 2p electron fill 1 p-orbital each based on the Hund's rule. In a possible interpretation, adding N to Sc-based system can reduce the population of non-bonding states (as N has 3 p-orbitals in 2p subshell) because the bonding between the one Sc orbital and three N orbitals leads to an energetically favorable configuration compared with a model that shows non-bonding states. Therefore, adding N to Al0.75Sc0.25N0.75 system is energetically favorable, as it decreases the population of non-bonding Sc states.
The energetic course seen in Fig. 1c and d for AlScN structures occurs because of driving forces: (1) the model needs to decrease its overall electron concentration to remove anti-bonding Sc–N/Al–N states, for instance, by adding N atoms, which favors a more N-rich composition, such as Al0.75Sc0.25N0.93 and (2) at the same time another energetic loss (decrease in Eform) is obtained by a distortion of the system, and the local source being the Sc atom. These phenomena are related because the Sc atom influences both of them.
Modeling a 128-atom AlScN model with N vacancies and its partial-density of states
The Eform of the 128-atom AlScN system (Fig. 4a and b) for different vacancy concentrations, shown in Fig. S7 (ESI†), reveals an associated trend with a close resemblance to that shown in 32-atom model. It can be observed that the Eform also increases and decreases with increasing concentration of vacancies and after structural relaxation, respectively. Thus, the Eform calculations of 128-atom wurtzite models support the findings of 32-atom Al0.75Sc0.25N models, since from them, similar changes in Eform were observed for different vacancy and structural conditions. Moreover, the number of atoms utilized for the model (128-atom system) can be larger than the average of ∼40 atoms used for current AlScN models under relaxed conditions (Fig. S8, ESI†).
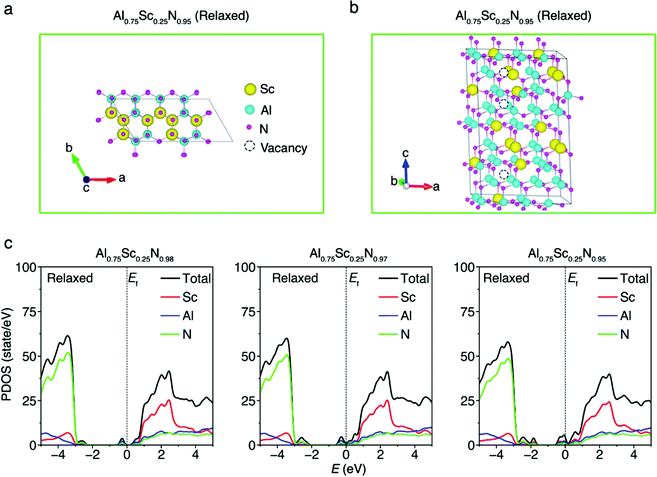 |
| Fig. 4 Wurtzite structure and defect formation energies of the 128-atom AlScN model with N vacancies. (a) Top and (b) side-view snapshots of the AlScN-based system. Sublattice A comprises N atoms (magenta), and sublattice B comprises Sc atoms (yellow) and Al atoms (cyan). (c) Partial-density of states (PDOS) of AlScN models with different concentrations of N vacancies in the relaxed state. The Fermi levels Ef were to set to zero energy. | |
Until now, the leakage current passing through the ferroelectric AlScN films is large and the physical reason is not well understood. Experiments have demonstrated an increase in leakage currents because of microstructural changes in high-temperature grown AlScN films.49 Moreover, a high leakage current is also observed for low-temperature-deposited AlScN films.50 The large leakage current causes problems in the ferroelectric extraction/measurement methodologies, such as the two-frequency technique and pulse-up/pulse-down strategy, which are harnessed to minimize the leakage currents.51,52 Moreover, the trade-off between decreasing leakage current, and simultaneously, minimizing thin-film thickness represents an impediment to fulfilling the promise of FEM.53 Hence, clarification of possible origin of the high leakage current is needed. Possible solution to this problem is shown in Fig. 1d, which discloses that Al0.75Sc0.25N models have a more stable wurtzite state with decreasing concentration of N vacancies. Fig. 4c shows the partial density of states (PDOS) of the AlScN models for different N vacancy concentrations. When the number of N vacancies decreases, the bandgap of the Al0.75Sc0.25N system becomes larger (Fig. 4c). This indicates that the Al0.75Sc0.25N crystal has a weaker electrical conduction with a decreased amount of N vacancies, which should lead to a smaller leakage current.
Discussion
Currently, applications such as energy-efficient system design through FEM is challenging because of several requirements: (1) excellent understanding of origin of vacancies and distortions, and (2) small leakage current. Currently, a limited number of FEM fulfill these requirements. The examples shown in this work indicate that existing state of the AlScN model can offer a guide to achieve these requirements with sufficient accuracy in terms of atomistic modeling. The key enhancement in AlScN systems to enable these applications is the investigation of models with different concentrations of vacancies and with distortions, and characterization of their bonding/electronic characters, achieved by ab initio DFT calculations. As a result, the previously unknown origins of vacancies/distortions are revealed, as well as relation between the leakage current and change in electronic/bonding characters for different defect concentrations. These provide insights to avoid the previous impasse between small leakage current and minimized thin-film thickness for facilitating long-term data retention, and at the same time, maintaining excellent device downscaling. Moreover, utilization of the one-hundred-and-twenty-eight-atom model enables a more accurate computation of the electronic characters for enhancing modeling accuracy.
Conclusion
In conclusion, understanding structural origin of vacancies and distortions in FEM is of vital importance for the development of delicate energy-efficient hardware. The atomistic modeling presented in this work enables a detailed characterization of the bonding/electronic characters in AlScN systems, e.g., the delicate interplay between anti-bonding states, p/d electron-band filling and distortions. These findings can provide significant insight, as well as a prospect of mitigating leakage currents in FE memories by suitable materials engineering to control the concentrations of vacancies in AlScN materials with wurtzite structure, thereby facilitating the achievement of energy-efficient hardware based on FEM for internet-of-things, machine-learning and edge computing.
Author contributions
Q. W. designed and performed the simulations and collected the data. S. X. G., C. L., M. L., Y. Z., L. L., T. H. L. and D. K. L. analyzed and discussed the data. D. K. L. conceived the project. All authors discussed the results and contributed to the writing of the manuscript.
Data availability
The data sets generated during and/or analyzed during the current study available from the corresponding author on reasonable request.
Conflicts of interest
The authors declare no competing interests.
Acknowledgements
We thank T. C. Chong (Singapore University of Technology and Design), F. A. Spaepen (Harvard University) and R. Zhao (Tsinghua University) for support, and N. Bajalovic, K. G. Lim, L. T. Ng for important discussions. The authors acknowledge support from the Singapore University of Technology and Design (SUTDT12017003), Changi General Hospital (Singapore) (CGH-SUTD-HTIF2019-001), Ministry of Education (Singapore) (MOE-T2EP50220-0022f), SUTD-Zhejiang-University (SUTD-ZJU (VP) 201903) grant programs. This work was supported by the Science and Engineering Research Council of A*STAR (Agency for Science, Technology and Research) Singapore, under Grant No. A20G9b0135. D. K. L. acknowledges support from the Massachusetts Institute of Technology–SUTD International Design Centre and National Supercomputing Centre, Singapore (15001618). S. X. G. acknowledges support from the SUTD President Graduate Scholarship.
References
- R. R. Murphy, Sci. Rob., 2020, 5, eaax1737 CrossRef PubMed.
- S. Feng, X. Yan, H. Sun, Y. Feng and H. X. Liu, Nat. Commun., 2021, 12, 748 CrossRef CAS PubMed.
- W. Rawat and Z. Wang, Neural Comput., 2017, 29, 2352–2449 CrossRef PubMed.
- D. K. Loke, G. J. Clausen, J. F. Ohmura, T. C. Chong and A. M. Belcher, ACS Appl. Nano Mater., 2018, 1, 6556 CrossRef CAS.
- M. Xu, X. Mai, J. Lin, W. Zhang, Y. Li, Y. He, H. Tong, X. Hou, P. Zhou and X. Miao, Adv. Funct. Mater., 2020, 30, 2003419 CrossRef CAS.
- M. Xu, M. Xu and X. Miao, InfoMat, 2022, e12315 CAS.
- Z. Yang, B. Li, J.-J. Wang, X.-D. Wang, M. Xu, H. Tong, X. Cheng, L. Lu, C. Jia, M. Xu, X. Miao, W. Zhang and E. Ma, Adv. Sci., 2022, 9, 2103478 CrossRef PubMed.
- T. Rausch, A. Rashed and S. Dustdar, Future Gener. Comput. Syst., 2021, 114, 259–271 CrossRef.
- K. Asadi, M. Li, P. W. Blom, M. Kemerink and D. M. De Leeuw, Mater. Today, 2011, 14, 592–599 CrossRef CAS.
- L. Li, A. M. Belcher and D. K. Loke, Nanoscale, 2020, 12, 24214 RSC.
- H. Y. Yoong, H. Wu, J. Zhao, H. Wang, R. Guo, J. Xiao, B. Zhang, P. Yang, S. J. Pennycook and N. Deng, Adv. Funct. Mater., 2018, 28, 1806037 CrossRef.
- F. Xue, X. He, Z. Wang, J. R.-D. Retamal, Z. Chai, L. Jing, C. Zhang, H. Fang, Y. Chai and T. Jiang, Adv. Mater., 2021, 33, 2008709 CrossRef CAS PubMed.
- D. Ielmini and H.-S. P. Wong, Nat. Electron., 2018, 1, 333–343 CrossRef.
- T. Jin and P. T. Lin, IEEE J. Sel. Top. Quantum Electron., 2020, 26, 1–7 Search PubMed.
- M. Dai, Z. Wang, F. Wang, Y. Qiu, J. Zhang, C.-Y. Xu, T. Zhai, W. Cao, Y. Fu, D. Jia, Y. Zhou and P.-A. Hu, Nano Lett., 2019, 19, 5410–5416 CrossRef CAS PubMed.
-
R. H. Olsson, Z. Tang and M. D. Agati, presented in part at the IEEE Custom Integrated Circuits Conference (CICC), 2020.
- T. Mikolajick, S. Slesazeck, H. Mulaosmanovic, M. H. Park, S. Fichtner, P. D. Lomenzo, M. Hoffmann and U. Schroeder, J. Appl. Phys., 2021, 129, 100901 CrossRef CAS.
- S. Fichtner, N. Wolff, F. Lofink, L. Kienle and B. Wagner, J. Appl. Phys., 2019, 125, 114103 CrossRef.
-
M. Clement, V. F. J. Olivares, T. Mirea, J. Olivares and E. Iborra, presented in part at the IEEE International Ultrasonics Symposium (IUS), 22–25 Oct. 2018, 2018.
- S. Clima, C. Pashartis, J. Bizindavyi, S. R.-C. McMitchell, M. Houssa, J. V. Houdt and G. Pourtois, Appl. Phys. Lett., 2021, 119, 172905 CrossRef CAS.
- A. Krishnamoorthy, S. C. Tiwari, A. Nakano, R. K. Kalia and P. Vashishta, Nanotechnology, 2021, 32, 49LT02 CrossRef CAS PubMed.
- K. H. Ye, G. Han, I. W. Yeu, C. S. Hwang and J.-H. Choi, Phys. Status Solidi RRL, 2021, 15, 2100009 CrossRef CAS.
- M. Noor-A-Alam, O. Z. Olszewski, H. Campanella and M. Nolan, ACS Appl. Mater. Interfaces, 2021, 13, 944–954 CrossRef CAS PubMed.
- Z.-J. Qiao, G.-D. Chen, H.-G. Ye, Y.-L. Wu, H.-B. Niu and Y.-Z. Zhu, Chin. Phys. B, 2012, 21, 087101 CrossRef.
- P. Giannozzi, S. Baroni, N. Bonini, M. Calandra, R. Car, C. Cavazzoni, D. Ceresoli, G. L. Chiarotti, M. Cococcioni and I. Dabo, J. Phys.: Condens. Matter, 2009, 21, 395502 CrossRef PubMed.
- J. P. Perdew, K. Burke and M. Ernzerhof, Phys. Rev. Lett., 1996, 77, 3865 CrossRef CAS PubMed.
- A. Zunger, S. H. Wei, L. G. Ferreira and J. E. Bernard, Phys. Rev. Lett., 1990, 65, 353–356 CrossRef CAS PubMed.
- S. H. Wei, L. G. Ferreira, J. E. Bernard and A. Zunger, Phys. Rev. B: Condens. Matter Mater. Phys., 1990, 42, 9622–9649 CrossRef CAS PubMed.
- A. van de Walle, M. Asta and G. Ceder, Calphad, 2002, 26, 539–553 CrossRef CAS.
- A. Stukowski, Modell. Simul. Mater. Sci. Eng., 2009, 18, 015012 CrossRef.
- R. Dronskowski and P. E. Bloechl, J. Phys. Chem., 1993, 97, 8617–8624 CrossRef CAS.
- S. Maintz, M. Esser and R. Dronskowski, Acta Phys. Pol., B, 2016, 47, 1165–1175 Search PubMed.
- C. F. Bunge, J. A. Barrientos and A. V. Bunge, At. Data Nucl. Data Tables, 1993, 53, 113–162 CrossRef CAS.
- A. Kokalj, J. Mol. Graphics Modell., 1999, 17, 176–179 CrossRef CAS PubMed.
- K. Momma and F. Izumi, J. Appl. Crystallogr., 2008, 41, 653–658 CrossRef CAS.
- J. Wang, M. Park, S. Mertin, T. Pensala, F. Ayazi and A. Ansari, J. Microelectromech. Syst., 2020, 29, 741–747 CAS.
- S. Zhang, D. Holec, W. Y. Fu, C. J. Humphreys and M. A. Moram, J. Appl. Phys., 2013, 114, 133510 CrossRef.
- S. Fichtner, N. Wolff, G. Krishnamurthy, A. Petraru, S. Bohse, F. Lofink, S. Chemnitz, H. Kohlstedt, L. Kienle and B. Wagner, J. Appl. Phys., 2017, 122, 035301 CrossRef.
- H. Momida, A. Teshigahara and T. Oguchi, AIP Adv., 2016, 6, 065006 CrossRef.
- C. Höglund, J. Birch, B. Alling, J. Bareño, Z. Czigány, P. O.-Å. Persson, G. Wingqvist, A. Zukauskaite and L. Hultman, J. Appl. Phys., 2010, 107, 123515 CrossRef.
- M. Akiyama, T. Kamohara, K. Kano, A. Teshigahara, Y. Takeuchi and N. Kawahara, Adv. Mater., 2009, 21, 593–596 CrossRef CAS PubMed.
- C. Walle and J. Neugebauer, J. Appl. Phys., 2004, 95, 3851–3879 CrossRef.
- U. V. Waghmare, N. A. Spaldin, H. C. Kandpal and R. Seshadri, Phys. Rev. B: Condens. Matter Mater. Phys., 2003, 67, 125111 CrossRef.
- V. L. Deringer, W. Zhang, P. Rausch, R. Mazzarello, R. Dronskowski and M. Wuttig, J. Mater. Chem. C, 2015, 3, 9519–9523 RSC.
- F. Rao, K. Ding, Y. Zhou, Y. Zheng, M. Xia, S. Lv, Z. Song, S. Feng, I. Ronneberger, R. Mazzarello, W. Zhang and E. Ma, Science, 2017, 358, 1423–1427 CrossRef CAS PubMed.
- M. Wuttig, D. Lüsebrink, D. Wamwangi, W. Wełnic, M. Gilleßen and R. Dronskowski, Nat. Mater., 2007, 6, 122–128 CrossRef CAS PubMed.
- P. M. Konze, R. Dronskowski and V. L. Deringer, Phys. Status Solidi RRL, 2019, 13, 1800579 CrossRef.
- S. Steinberg and R. Dronskowski, Crystals, 2018, 8, 225 CrossRef.
- A. Zukauskaite, G. Wingqvist, J. Palisaitis, J. Jensen, P. O.-Å. Persson, R. Matloub, P. Muralt, Y. Kim, J. Birch and L. Hultman, J. Appl. Phys., 2012, 111, 093527 CrossRef.
- D. Wang, J. Zheng, P. Musavigharavi, W. Zhu, A. C. Foucher, S. E. Trolier-McKinstry, E. A. Stach and R. H. Olsson, IEEE Electron Device Lett., 2020, 41, 1774–1777 CAS.
-
X. Liu, J. Zheng, D. Wang, P. Musavigharavi, E. A. Stach, R. Olsson III and D. Jariwala, 2020, arXiv preprint arXiv:2012.10019.
- X. Liu, D. Wang, K.-H. Kim, K. Katti, J. Zheng, P. Musavigharavi, J. Miao, E. A. Stach, R. H. Olsson and D. Jariwala, Nano Lett., 2021, 21, 3753–3761 CrossRef CAS PubMed.
- A. Sigov, Y. Podgorny, K. Vorotilov and A. Vishnevskiy, Phase Transitions, 2013, 86, 1141–1151 CrossRef CAS.
|
This journal is © The Royal Society of Chemistry 2022 |
Click here to see how this site uses Cookies. View our privacy policy here.