DOI:
10.1039/D2LC00641C
(Communication)
Lab Chip, 2022,
22, 3663-3667
A similarity scaling approach for organ-on-chip devices†
Received
12th July 2022
, Accepted 30th August 2022
First published on 31st August 2022
Abstract
Organ-on-chip devices (OoCs) provide more nuanced insights into (patho)physiological processes of the human body than static tissue models, and are currently the most promising approach to emulating human (patho)physiology in vitro. OoC designs vary greatly and questions remain as to how to maximize biomimicry and clinical translatability of the in vitro findings. Scaling is critical, yet has largely been ad hoc, consisting in matching one or a few variables between the OoC and the target organ. This has limited the predictive value of OoCs. Here, we propose a systematic approach based on the principle of similitude widely used in the physical sciences, and present three case studies from the recent literature to demonstrate how the approach works. A lung-on-a-chip and a liver-on-a-chip both satisfied important similarity criteria, and therefore yielded results that were in good agreement with clinical data. A gut–liver system failed to satisfy a key criterion of kinematic similarity, and yielded unphysiological pharmacokinetic responses in vitro. The similarity scaling approach promises to improve markedly the design and operation of organ- and human-on-chip devices.
Introduction
The past decade has seen intensifying efforts to develop complex in vitro models that closely replicate functions of human organs.1,2 These typically involve multiple cell types assembled in three dimensions to mimic the morphology and functionality of the target organs, and are popularly known as “organ-on-chip” (OoC) devices or microphysiological systems (MPS).3–5 Such OoCs can be assembled into a multi-organ-on-chip system to study inter-organ crosstalk.6–8 OoCs emulate organ-level (patho)physiology and are currently the most promising human-based approach in biomedical research. Much of this research has been motivated by the prospect of using OoCs to improve clinical translation and reduce attrition rate in the drug development process, potentially replacing animal testing.2,3,5,9–13
For a miniaturized on-chip culture to mimic a human organ, scaling is a central issue: how to design OoCs so that their performance in vitro can be extrapolated to the functions of organs in vivo? Existing approaches are mostly ad hoc, focusing on specific parameters or functions in a specific OoC. In particular, they do not account systematically for the many interlinked mechanisms and parameters in OoCs. For example, direct scaling14 and allometric scaling15 use the OoC-to-human size or mass ratio to determine the size of the organs on the chip. Such scalings do not involve any time scales, and cannot ensure proper scaling of rate parameters (e.g., perfusion and metabolic rates). In a gut–liver system, direct or allometric scaling would produce drug exposure times that are orders of magnitude below in vivo.16 Functional scaling strives for in vivo levels of key functions for each organ, e.g., metabolic rate for the liver or filtration rate for the kidney.17,18 But the difficulty lies in balancing the often conflicting needs of multiple functions, especially in multiple OoCs setups.6,16 From the earlier years of OoC development, scaling has been recognized as an outstanding problem.3,17,19,20 But a general framework for scaling remains elusive, and recent reviews have consistently listed scaling as an urgent problem to be tackled.1,8,21,22
We argue that the solution requires a shift in focus from the ad hoc needs of specific OoCs to a systematic view that accounts for the multiple factors involved. Here we propose one such framework by adapting the techniques of dimensional analysis and similarity, both classical tools in physics and engineering.
Similarity scaling
In any physical process, if the output P is determined by input quantities qi (i = 1,…, n), then these inputs must be algebraically linked in such a way as to yield the proper dimension of P. The pi theorem23 asserts that the relationship among P and qi can be reduced, without any loss of generality, to one among a smaller number of independent dimensionless Π groups, each formed by products of powers of P and qi. This relationship then forms the basis for scaling between a model and its prototype. The procedure is widely used in scale-up in physics and engineering,24,25 and the ESI† offers an example in aeronautics.
For an OoC, we first identify the key output P that is to be translated to in vivo. Then the Π group Π1 that represents P must be a function of the other Π groups, Π2,…, Πm,
with the total number
m <
n + 1. If we ensure that the inputs Π
2,…, Π
m for the OoC match those
in vivo, the output Π
1 must be matched as well. Thus, we have achieved
similarity between the OoC and its target organ, and the
in vitro measurement of
P can be translated to the
in vivo organ. Notably, this does not require knowledge of the function
f, which is almost always unknown in a complex system. The procedure can be extended to multiple output functions for a single OoC, or to multi-organ chips.
Similarity criteria
When scaling mechanical systems, one sometimes classifies the dimensionless groups according to geometric, kinematic and dynamic similarity.26,27 Geometric similarity governs the Π groups describing length, area and volume ratios, angles and shapes. Such ratios must be equal between the model and the prototype. Kinematic similarity requires equality of time-scale ratios in addition to length ratios. Thus, it concerns Π groups that involve velocity and other rate quantities. On the basis of these two, dynamic similarity further introduces mass ratios so that dynamic quantities such as pressure, shear stresses and forces, which typically constitute the output Π groups in a mechanical system, are scaled properly.
This scheme can be adapted and expanded for scaling OoCs. The Π groups about OoC size, shape and volume pertain to geometric similarity, those about residence time, perfusion rates and kinetic rates belong to kinematic similarity, and finally those involving forces and stresses fall under dynamic similarity. For OoCs, we need to add morphological similarity as a new criterion, which ensures the proper structure and morphology of heterotypic cell assemblies, e.g., to distinguish spheroids from dispersed cells, and predominantly 2D from 3D structures.19,28 Finally, metabolic outcomes such as concentration profiles are central to pharmacokinetic/pharmacodynamic (PKPD) studies using OoCs.16,29,30 Thus, we propose another new criterion called metabolic similarity that ensures proper scaling of dosage and concentrations.
It is also interesting to note that some of the ad hoc scaling schemes proposed in earlier work8,16,18,19 may be identified with the similarity criteria above. For example, requirements on chamber size ratio and cell number are for geometric similarity. Requirements on perfusion rates, metabolic rates, organ- or cell-to-liquid ratios and residence time concern kinematic similarity, and requirement on shear stress concerns dynamic similarity. Thus, in carrying out the proposed similarity scaling, one may also satisfy some such ad hoc criteria by accident.
Partial similarity
In principle, the pi theorem guarantees similarity. In the laboratory, however, complete similarity may not be attainable because of limitations on the materials available, fabrication techniques and accessible experimental conditions. In such cases, one strategically omits certain Π groups and strives for partial similarity.23 This is often necessary even for mechanical systems. In the OoC, we may have to determine which input Π's are more or less important for the phenomena of interest, and carry out scaling based on partial similarity. In multi-organ chips, the greater complexity implies a larger number of Π groups. Although the principle of similarity scaling applies to such systems, partial similarity may become unavoidable.
Case studies
Similarity scaling differs from prior scaling methods in that it accounts for all parameters and their interactions in a systematic way. Although OoCs are vastly more complex than mechanical systems, we show here that the success of similarity scaling in the latter can be reproduced in the former, provided that the OoC experiments are designed and executed properly. For this purpose, we have selected three studies from the literature based on the completeness of the reported parameters, operating conditions, and quantitative outputs for their respective OoCs. The availability of such data make them proper test cases for the similarity scaling approach.
Lung-on-a-chip (LOAC)
Huh et al.31,32 developed the LOAC as a mimic for an alveolus. It features an air–liquid interface that can be cyclically stretched to replicate the alveolar stretching during breathing. As a disease model for pulmonary edema due to cancer treatment by interleukin-2, the LOAC manifests a gradual loss of barrier function; the increase in permeability agrees well with ex vivo data from whole mouse lungs.32
To examine the success of LOAC from the angle of similarity scaling, we take the permeability to be the output that depends on a host of input variables and parameters, including the drug dosage, the frequency and amplitude of membrane stretching, and the medium perfusion velocity. Our dimensional analysis (see ESI† for details) produces 6 Π groups, with the output Π1 = k/D2 being the ratio of the membrane permeability to the chamber width squared. The authors have matched the Π groups between the LOAC and in vivo. In particular, the following similarity criteria are satisfied:
• Geometric similarity: the LOAC matches the chamber size and the air–liquid-interface thickness with the alveolus in vivo.
• Morphological similarity: the LOAC has confluent endothelial and epithelial monolayers apposed on either side of the membrane, approximating the air-blood boundary in vivo.
• Kinematic similarity: the membrane stretching amplitude, frequency and the medium perfusion velocity are all chosen to match the in vivo conditions.
• Metabolic similarity: the interleukin-2 dosage and application time are matched with in vivo conditions.
Thus, the LOAC and the operating conditions of Huh et al.32 have achieved similarity with the in vivo counterpart. This ensures that the output of the LOAC, the membrane permeability enhanced by interleukin-2 treatment, is translatable from the LOAC to the alveolus. Note that this is a somewhat special case of 1
:
1 size ratio between the model and the prototype. Moreover, all the Π groups are matched for a rare attainment of complete similarity.
More recently, second-generation LOACs have been developed that continue to maintain similarity scaling with a 1
:
1 size ratio, but with improved and refined features, including the use of primary alveolar epithelial cells instead of a cell line33 and the modeling of cytokine production and leukocyte recruitment following an influenza infection.34
Liver-on-a-chip
Ewart et al.35 studied drug-induced liver injury (DILI) on a Liver-Chip, an OoC that models a liver sinusoid. Judging by suppressed albumin production and other symptoms, the OoC distinguished toxic drugs from their non-toxic structural analogs, and correctly ordered the toxicity of drugs according to the Garside DILI rank. We will demonstrate below that this success is again rooted in satisfying similarity scaling to the in vivo organ, which is the human liver sinusoid in this case.
The details of the dimensional analysis can be found in the ESI,† and only a brief summary is given below. Without drug treatment, the Liver-Chip produces albumin in vitro in the physiologic in vivo range of 20–105 μg per 106 hepatocytes per day. In the DILI study, therefore, the main output is the albumin production as a fraction of the control without drug treatment: Π1 = ϕ. The input variables and parameters are listed in Table 1, along with the values of the input Π groups, Π2,…, Π5. Of these, Π2, Π3 and Π4 match reasonably well between in vitro and in vivo. Π5 differs considerably, but its small magnitudes suggest that permeation through the cell layers happens rapidly, and is not the rate-limiting step. Thus, we can disregard Π5 and claim partial similarity between the Liver-Chip and the liver sinusoid in vivo. This ensures translatability of albumin suppression Π1 = ϕ, and therefore the proper detection of DILI.
Table 1 Similarity scaling for the Liver-Chip in a DILI study.35 The input parameters are L: chip dimension, u: perfusion velocity, c: initial drug concentration, c50: required drug concentration to produce a 50% reduction in albumin, D: drug diffusivity in perfusate, P: permeability through the membrane, k: drug clearance rate. The various rates are for the drug diclofenac. See ESI† for details of dimensional analysis and the sources for the parameter values
Parameters |
In vitro values |
In vivo values |
L (μm) |
200 |
5 |
u (μm min−1) |
2500 |
6.67 × 104 |
k (μm3 min−1) |
1.67 × 109 |
2.93 × 107 |
D (μm2 min−1) |
4.50 × 104 |
4.50 × 104 |
P (μm min−1) |
6.53 × 106 |
6.53 × 106 |
c (μM) |
0.05 |
0.05 |
c
50 (μM) |
0.1 |
0.1 |
Π2 = c/c50 |
0.5 |
0.5 |
Π3 = uL2/k |
5.99 × 10−2 |
5.69 × 10−2 |
Π4 = uL/D |
11.1 |
7.41 |
Π5 = u/P |
3.83 × 10−4 |
1.02 × 10−2 |
Even though the Liver-Chip is much larger than the liver sinusoid in vivo, the perfusion and drug clearance rates also differ so as to compensate through the Π groups. This systematic treatment is the principal advantage of similarity scaling over prior scaling that focuses on matching individual parameters.
Gut–liver system
Cirit et al.16,36,37 linked a gut and a liver module into a multi-OoC system that captured the key functions of both organs—the permeation of orally administered drugs across the membrane in the gut and drug metabolism in the liver—as well as their crosstalk. The gut OoC has an apical chamber and a basolateral chamber, but the liver OoC has a single chamber. Both are connected to a mixing chamber that supplies the common perfusion. This system has been used to study drug metabolism in a multi-organ system.16,37
The system involves a larger number of parameters, and requires a lengthier dimensional analysis resulting in 12 Π groups (details in ESI†). For the present purpose, we need only discuss the parameters and Π groups relevant to kinematic similarity, more specifically the transport and kinetic rates. These are listed in Table 2 for the drug diclofenac. The dimensionless groups Π6 and Π7 indicate the gut and liver metabolic rates of the drug relative to its transport rate by perfusion. Π8 gives the ratio between the drug permeation and perfusion. The small values of Cg and Π6 indicate negligible drug metabolism in the gut. The most prominent discrepancy is in Π7 = Cl/Q, which is more than 10
000 times greater in vitro than in vivo. This severely violates the kinematic similarity.23 Π7 is also the ratio between the circulation time T0 = Vm/Q and the liver clearance time Tl = Vm/Cl. Not only is the circulation too slow in vitro, the clearance is also too fast. As a result, the time concentration profile fails to translate to the clinical data, with a much shorter time scale than in vivo (Fig. 1).16
Table 2 Similarity scaling for a gut–liver OoC system,16,37 with a partial list of the rate parameters: Cl and Cg: diclofenac clearance rates in liver and gut, P: membrane permeability, Q: perfusion rate, S: membrane area, Vm: volume of mixing chamber, T0: circulation time, Tl: liver clearance time. See ESI† for details of dimensional analysis and the sources for the parameter values
Parameters |
In vitro values |
In vivo values |
C
l (mL min−1) |
0.0102 |
0.0813 |
C
g (mL min−1) |
0.00029 |
0 |
P (cm min−1) |
8.83 × 10−4 |
1.08 × 10−3 |
Q (mL min−1) |
0.0104 |
1250 |
S (cm2) |
1.12 |
3 × 105 |
V
m (mL) |
1 |
1750 |
Π6 = Cg/Q |
0.0279 |
0 |
Π7 = Cl/Q |
0.980 |
6.50 × 10−5 |
Π8 = PS/Q |
0.0951 |
0.259 |
T
0 = Vm/Q (min) |
96.1 |
1.40 |
T
l = Vm/Cl (min) |
98.0 |
21 525 |
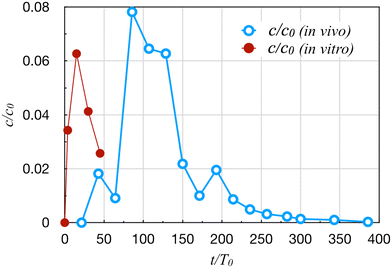 |
| Fig. 1 Comparison of the plasma diclofenac concentration profiles in vivo16 and in vitro.37 Time t is scaled by the circulation time T0, and concentration c by the initial concentration c0 in the dosing chamber. | |
Similarity scaling not only pinpoints the cause of the mis-scaling, but can also suggest ways to correct it. Since Cl depends on the metabolism of individual cells, it cannot be easily varied in vitro. Thus one can only raise the perfusion rate Q to lower Π7. To maintain the value of Π8, already roughly matched with in vivo, one must increase the gut epithelial area S in proportion to Q. This systematic approach to managing the parameters is the hallmark of similarity scaling.
Discussion
Similarity scaling offers a systematic scheme for matching OoCs and their target organs, as opposed to matching individual parameters. It is also mathematically guaranteed to work by the pi theorem. In practice, limitations in available materials and fabrication techniques often make complete similarity impossible. But even partial similarity can provide highly useful guidelines, as demonstrated in the above.
OoCs being biological systems puts special constraints on similarity scaling. An individual cell is in a sense the minimum unit and cannot be scaled further down. Thus, an OoC typically contains a smaller number of the same cells as in vivo, not the same number of “smaller cells” as perfect similarity would dictate. Moreover, certain parameters, e.g., cellular metabolic rates, will be more or less fixed at their in vivo values and not subject to large variations for scaling. Despite these limitations, we have shown the utility and potential of similarity scaling in the case studies. It will offer a general framework for designing the next generation of organ- and human-on-chip systems.
Materials and methods
The method of dimensional analysis is illustrated with examples in the ESI,† which also contains detailed analysis and all the data for the three case studies.
Author contributions
JJF and SH conceptualized the project, carried out the investigation, analyzed the data and wrote the paper.
Conflicts of interest
There are no conflicts to declare.
Acknowledgements
We acknowledge partial funding of this work from the Stiftung Charité (S. H.), the Natural Sciences and Engineering Research Council Canada (2020-04224 for S. H., 2019-04162 for J. J. F.), the German Research Foundation (DFG, SFB 1449-431232613, project A5, S. H.), and the John R. Evans Leaders Fund (S. H.). J. J. F. thanks Milica Radisic (University of Toronto) for suggesting the scaling problem to him. The image in the online “Table of Contents” entry was partly generated using Servier Medical Art, provided by Servier, licensed under a Creative Commons Attribution 3.0 unported license.
Notes and references
- G. Vunjak-Novakovic, K. Ronaldson-Bouchard and M. Radisic, Cell, 2021, 184, 4597–4611 CrossRef CAS PubMed.
- L. A. Low, C. Mummery, B. R. Berridge, C. P. Austin and D. A. Tagle, Nat. Rev. Drug Discovery, 2021, 20, 345–361 CrossRef CAS PubMed.
- K. Ronaldson-Bouchard and G. Vunjak-Novakovic, Cell Stem Cell, 2018, 22, 310–324 CrossRef CAS PubMed.
- S. E. Park, A. Georgescu and D. Huh, Science, 2019, 364, 960–965 CrossRef CAS PubMed.
- M. Weinhart, A. Hocke, S. Hippenstiel, J. Kurreck and S. Hedtrich, Pharmacol. Res., 2019, 139, 446–451 CrossRef CAS.
- J. H. Sung, Y. I. Wang, S. N. Narasimhan, M. Jackson, C. Long, J. J. Hickman and M. L. Shuler, Anal. Chem., 2019, 91, 330–351 CrossRef CAS PubMed.
- D. Park, J. Lee, J. J. Chung, Y. Jung and S. H. Kim, Trends Biotechnol., 2020, 38, 99–112 CrossRef CAS PubMed.
- M. Malik, Y. Yang, P. Fathi, G. J. Mahler and M. B. Esch, Front. Cell Dev. Biol., 2021, 9, 721338 CrossRef PubMed.
- N. T. Elliott and F. Yuan, J. Pharm. Sci., 2011, 100, 59–74 CrossRef CAS PubMed.
- U. Marx, T. Akabane, T. B. Andersson, E. Baker, M. Beilmann, S. Beken, S. Brendler-Schwaab, M. Cirit, R. David, E. M. Dehne, I. Durieux, L. Ewart, S. C. Fitzpatrick, O. Frey, F. Fuchs, L. G. Griffith, G. A. Hamilton, T. Hartung, J. Hoeng, H. Hogberg, D. J. Hughes, D. E. Ingber, A. Iskandar, T. Kanamori, H. Kojima, J. Kuehnl, M. Leist, B. Li, P. Loskill, D. L. Mendrick, T. Neumann, G. Pallocca, I. Rusyn, L. Smirnova, T. Steger-Hartmann, D. A. Tagle, A. Tonevitsky, S. Tsyb, M. Trapecar, B. Van de Water, J. Van den Eijnden-van Raaij, P. Vulto, K. Watanabe, A. Wolf, X. Zhou and A. Roth, ALTEX, 2020, 37, 365–394 Search PubMed.
- P. P. Adhikary, Q. U. Ain, A. C. Hocke and S. Hedtrich, Nat. Rev. Mater., 2021, 6, 374–376 CrossRef CAS PubMed.
- B. Cecen, C. Karavasili, M. Nazir, A. Bhusal, E. Dogan, F. Shahriyari, S. Tamburaci, M. Buyukoz, L. D. Kozaci and A. K. Miri, Pharmaceutics, 2021, 13, 1657 CrossRef CAS PubMed.
- K. Ronaldson-Bouchard, D. Teles, K. Yeager, D. N. Tavakol, Y. Zhao, A. Chramiec, S. Tagore, M. Summers, S. Stylianos, M. Tamargo, B. M. Lee, S. P. Halligan, E. H. Abaci, Z. Guo, J. Jacków, A. Pappalardo, J. Shih, R. K. Soni, S. Sonar, C. German, A. M. Christiano, A. Califano, K. K. Hirschi, C. S. Chen, A. Przekwas and G. Vunjak-Novakovic, Nat. Biomed. Eng., 2022, 6, 351–371 CrossRef CAS PubMed.
- I. Maschmeyer, A. K. Lorenz, K. Schimek, T. Hasenberg, A. P. Ramme, J. Hübner, M. Lindner, C. Drewell, S. Bauer, A. Thomas, N. S. Sambo, F. Sonntag, R. Lauster and U. Marx, Lab Chip, 2015, 15, 2688–2699 RSC.
- A. Ahluwalia, Sci. Rep., 2017, 7, 42113 CrossRef CAS PubMed.
- C. Maass, C. L. Stokes, L. G. Griffith and M. Cirit, Integr. Biol., 2017, 9, 290–302 CrossRef PubMed.
- J. P. Wikswo, E. L. Curtis, Z. E. Eagleton, B. C. Evans, A. Kole, L. H. Hofmeister and W. J. Matloff, Lab Chip, 2013, 13, 3496–3511 RSC.
- J. H. Sung, Y. Wang and M. L. Shuler, APL Bioeng., 2019, 3, 021501 CrossRef.
- C. Moraes, J. M. Labuz, B. M. Leung, M. Inoue, T.-H. Chun and S. Takayama, Integr. Biol., 2013, 5, 1149–1161 CrossRef CAS PubMed.
- C. L. Stokes, M. Cirit and D. A. Lauffenburger, CPT: Pharmacometrics Syst. Pharmacol., 2015, 4, 559–562 CAS.
- P. Sphabmixay, M. S. B. Raredon, A. J.-S. Wang, H. Lee, P. T. Hammond, N. X. Fang and L. G. Griffith, Biofabrication, 2021, 13, 045024 CrossRef CAS PubMed.
- D. E. Ingber, Nat. Rev. Genet., 2022, 23, 467–491 CrossRef CAS PubMed.
-
M. Zlokarnik, Scale-Up in Chemical Engineering, WILEY-VCH Verlag, 2nd edn, 2006 Search PubMed.
-
A. Bisio and R. L. Kabel, Scale-up of Chemical Processes: Conversion from Laboratory Scale Tests to Successful Commercial Size Design, Wiley-Interscience, 1985 Search PubMed.
-
J. M. Bonem, Chemical Projects Scale Up: How to Go from Laboratory to Commercial, Elsevier, 2018 Search PubMed.
-
J. R. Welty, C. E. Wicks, R. E. Wilson and G. L. Rorrer, Fundamentals of Momentum, Heat, and Mass Transfer, Wiley, 5th edn, 2008, pp. 125–136 Search PubMed.
-
F. M. White, Fluid Mechanics, McGraw-Hill, Seventh edn, 2011, pp. 293–345 Search PubMed.
- N. Ucciferri, T. Sbrana and A. Ahluwalia, Front. Bioeng. Biotechnol., 2014, 2, 74 Search PubMed.
- C. Ma, Y. Peng, H. Li and W. Chen, Trends Pharmacol. Sci., 2021, 42, 119–133 CrossRef CAS PubMed.
- Y. Yang, Y. Chen, L. Wang, S. Xu, G. Fang, X. Guo, Z. Chen and Z. Gu, Front. Bioeng. Biotechnol., 2022, 10, 900481 CrossRef PubMed.
- D. Huh, B. D. Matthews, A. Mammoto, M. Montoya-Zavala, H. Y. Hsin and D. E. Ingber, Science, 2010, 328, 1662–1668 CrossRef CAS PubMed.
- D. Huh, D. C. Leslie, B. D. Matthews, J. P. Fraser, S. Jurek, G. A. Hamilton, K. S. Thorneloe, M. A. McAlexander and D. E. Ingber, Sci. Transl. Med., 2012, 4, 159ra147 Search PubMed.
- P. Zamprogno, S. Wüthrich, S. Achenbach, G. Thoma, J. D. Stucki, N. Hobi, N. Schneider-Daum, C.-M. Lehr, H. Huwer, T. Geiser, R. A. Schmid and O. T. Guenat, Commun. Biol., 2021, 4, 168–423 CrossRef CAS PubMed.
- H. Bai, L. Si, A. Jiang, C. Belgur, R. Plebani, C. Oh, M. Rodas, A. Nurani, S. Gilpin, R. K. Powers, G. Goyal, R. P. Baun and D. E. Ingber, Nat. Commun., 2022, 13, 1928 CrossRef CAS PubMed.
-
L. Ewart, A. Apostolou, S. A. Briggs, C. V. Carman, J. T. Chaff, A. R. Heng, S. Jadalannagari, J. Janardhanan, K.-J. Jang, S. R. Joshipura, M. Kadam, M. Kanellias, V. J. Kujala, G. Kulkarni, C. Y. Le, C. Lucchesi, D. V. Manatakis, K. K. Maniar, M. E. Quinn, J. S. Ravan, A. C. Rizos, J. F. Sauld, J. Sliz, W. Tien-Street, D. Ramos Trinidad, J. Velez, M. Wendell, P. K. Mahalingaiah, D. E. Ingber and D. Levner, bioRxiv, 2021, preprint, DOI:10.1101/2021.12.14.472674.
- N. Tsamandouras, T. Kostrzewski, C. L. Stokes, L. G. Griffith, D. J. Hughes and M. Cirit, J. Pharmacol. Exp. Ther., 2017, 360, 95–105 CrossRef CAS PubMed.
- N. Tsamandouras, W. L. K. Chen, C. D. Edington, C. L. Stokes, L. G. Griffith and M. Cirit, AAPS J., 2017, 19, 1499–1512 CrossRef CAS PubMed.
|
This journal is © The Royal Society of Chemistry 2022 |
Click here to see how this site uses Cookies. View our privacy policy here.