DOI:
10.1039/D1FO02181H
(Paper)
Food Funct., 2022,
13, 356-374
The metabolic regulation of Fuzhuan brick tea in high-fat diet-induced obese mice and the potential contribution of gut microbiota†
Received
9th July 2021
, Accepted 2nd September 2021
First published on 14th September 2021
Abstract
This study investigated the metabolic effects of Fuzhuan brick tea (FBT) in high-fat diet (HFD)-induced obese mice and the potential contribution of gut microbiota. The results showed that FBT ameliorated the HFD-induced glycerophospholipid metabolic aberrance, specifically increased the serum levels of phosphatidylcholines (PCs), lysophosphatidylcholines (LysoPCs), and the ratio of PC to phosphatidylethanolamines (PE). Besides, FBT increased the serum level of gut microbiota-derived aryl hydrocarbon receptor (AhR) ligand, 3-indole propionic acid, as well as the relative abundance of intestinal AhR-ligand producing bacteria such as Clostridiaceae, Bacteroidales_S24-7_group, and Lactobacillaceae. However, the metabolic benefits of FBT were weakened when the gut microbiota were depleted by antibiotic treatment, thereby suggesting that gut microbiota was required for FBT to regulate glycerophospholipid metabolism. Indeed, the metabolites regulated by FBT were significantly correlated with the AhR-ligand producing bacteria. The KEGG pathway enrichment analysis and expressions of AhR target genes indicated that FBT would improve the glycerophospholipid metabolism via the AhR-Pemt signal axis, in which the gut microbiota and their metabolites played pivotal mediators. Overall, FBT could be a functional beverage to improve HFD-induced metabolic disorders in a gut microbiota dependent manner.
Introduction
Lipid metabolic disorder induced by a high-fat diet (HFD) has become a public health problem of global concern.1,2 Fatty acid, choline, and glycerophospholipid metabolism disorders are of great concern in the pathogenesis, diagnosis, and therapeutic target research.2,3 Several research studies have shown that HFD causes glycerophospholipid metabolic aberrance. Abnormal levels of serum phosphatidylcholines (PCs) and/or lower ratio of PCs to phosphatidylethanolamines (PEs) (PC/PE) have been regarded as major risk factors for obese, non-alcoholic fatty liver, and metabolic syndrome diseases.4–8 Adverse PC synthesis or inhibition of PE to PC conversion, catalyzed by phosphatidylethanolamine N-methyltransferase (Pemt), may be the primary reasons for these metabolic disorders.4,9–11Pemt is transcriptionally regulated by aryl hydrocarbon receptor (AhR), a nuclear receptor activated by ligands. Notably, 3-indole propionic acid, indole, indole acetic acid, and 3-methylindole indole, and other tryptophan metabolites are vitally endogenic AhR ligands primarily produced by gut microbiota.12–16 Therefore, gut microbiota and their metabolites might play pivotal roles in regulating glycerophospholipid metabolism.
The microbiota-host co-evolution and co-metabolites are responsible for their biological functions in the host physiology.17–20 Among the co-metabolites, short-chain fatty acids, bile acids, and tryptophan-indole derivates act as mediators in the promiscuous receptor–ligand interactions for metabolic regulation via the liver-gut axis.15,17,21,22 HFD has been proved as a major pejorative approach for changing the intestinal AhR-ligand producing microbes such as Lactobacillus, Bifidobacteriaceae, and Akkermansia muciniphila. As a result, declined microbiota-derived tryptophan-indole derivates might eventually induce AhR-dependent lipid metabolic disorder and obesity.12,16,17,23,24 Some obese patients also showed lower concentrations of AhR ligands compared to healthy individuals.17,25 Nowadays, AhR activation or AhR ligand supplementation have been effectively used to improve the lipid metabolism and hepatic steatosis in rodents.17,26,27
Many phytochemicals, such as polyphenols and polysaccharides in Oolong, Black, Pu-erh, Fuzhuan brick tea (FBT), and other plants, are shown to be potent in regulating glycerophospholipid metabolism.14,28–33 Our previous study revealed that FBT had exerted potent anti-obesity effects in HFD-fed mice via modulating gut microbiota.34 However, the metabolic role of FBT and the potential contribution of gut microbiota in HFD-induced obese mice remained unclear, and the underlying mechanisms of action are yet to be elucidated.
Herein, we aimed at investigating the role of FBT in regulating the serum metabolism and involved genes of HFD-induced obese mice. Also, the metabolomic responses of HFD and FBT on the antibiotic-induced microbiota-depleted mice and the relationship between the FBT-regulated metabolites and gut microbiota were analyzed. Ultimately, the potential contribution of gut microbiota and the corresponding mechanisms in the metabolic regulation of FBT would be revealed.
Materials and methods
Chemicals and reagents
All high-performance liquid chromatography (HPLC) grade reagents, including methanol, acetonitrile, formic acid, water, and isopropanol, were purchased from Fisher (Shanghai, China). Nonabsorbable broad-spectrum antibiotics were purchased from Sangon Biotech (Shanghai, China).
Preparation of Fuzhuan brick tea
FBT used in the present study were the same samples as in our previous study, which were obtained and extracted with boiling water.34 Briefly, FBT was extracted by boiling water twice, then the filtrate was concentrated at 58 °C under vacuum. The FBT extract was finally obtained after lyophilizing, containing soluble sugars of 22.28 ± 1.44%, polyphenols of 24.65 ± 0.24%, free amino acids of 3.19 ± 0.02%, and flavonoids of 2.19 ± 0.06%. The contents of epicatechin (EC), epigallocatechin gallate (EGCG), epigallocatechin gallate (GCG), and theanine were 0.67 ± 0.04%, 1.842 ± 0.16%, 0.20 ± 0.01%, and 0.536 ± 0.03% respectively.
Animals and experimental design
All animals used in this study were the same batch of animals as in our previous study, in which the obese mice model was designed and evaluated by the criteria, including serum lipid parameters (total cholesterol (TC), triglyceride (TG), high-density lipoprotein cholesterol (HDL-C), low-density lipoprotein cholesterol (LDL-C)), hepatic TC, TG levels, hepatic steatosis, adipocyte hypertrophy, and blood glucose homeostasis.34 Briefly, 35 male C57BL/6 mice (age: 5 weeks, body weight: 20.58 ± 0.89 g, no. 43004700044926) were randomly divided into the microbiota-depleted group (Ab) and healthy group (WT). The mice in Ab group were treated with nonabsorbable broad-spectrum antibiotics every other day to deplete the gut microbiota. Briefly, the mice were gavaged with a solution of mixed antibiotics at a dose of 25 μg per g bw neomycin, 12.5 μg per g bw vancomycin, 12.5 μg per g·bw imipenem, 25 μg per g·bw metronidazole, 12.5 μg per g·bw streptomycin, and 25 U per g·bw penicillin (Sangon Biotech, Shanghai, China) during the first 30 days. Over a longer treatment period, the solution of antibiotics was further supplemented with streptomycin of 50 μg per g·bw, gentamycin of 42.5 μg per g·bw, ciprofloxacin of 36.25 μg per g·bw, and bacitracin of 50 μg per g·bw, as per the method described in our previous study.34 For mice in the WT group, equivalent volumes of sterile water were used instead of antibiotics. After 2 weeks of antibiotic treatment, the Ab mice were further randomly assigned into normal chow diet (ND, 10% calories from fat, H10010) group (AbND), HFD (60% calories from fat, H10060) group (AbHFD), and HFD supplemented with 400 mg per kg per day FBT via intragastric gavage group (AbHFDH), with 5–6 mice in each group. Similarly, the WT mice were further assigned into ND group (WTND), HFD group (WTHFD), and HFD supplemented with FBT group (WTHFDH), with 6 mice in each group. All mice were given the corresponding treatments for 8 weeks. At the end of the experiment, the feces of mice were collected, immediately frozen in liquid nitrogen, and stored at −80 °C until further analysis of gut microbiota. Then, the mice were anesthetized and sacrificed after 5 h of fasting. The blood of mice was subsequently collected and centrifuged at 3000 rpm for 15 min. Then, the serum supernatant was withdrawn, immediately frozen in liquid nitrogen, and stored at −80 °C until further metabolomic analyses.
Metabolomic profiling of serum samples
The serum samples, stored at −80 °C, were thawed on ice. Then, 100 μL of serum sample was added into a tube containing 400 μL of ice-cold methanol/acetonitrile (1
:
1), vortexed for 30 s, and ultrasonically extracted in ice for 30 min. Subsequently, samples were placed at −20 °C for 30 min before centrifuging at 13
000g and 4 °C for 15 min. The supernatant was vacuum-dried and dissolved in 100 μL of methanol/water (1
:
1) for metabolic profiling. The quality control (QC) sample was pooled from the representative serum samples of mice from every group and analyzed similarly to the experiment samples in each batch.
The metabolic profiling was performed using the UPLC-Triple-TOF/MS system (AB Sciex, Pudong new area, Shanghai, China) with column BEH C18 (100 mm × 2.1 mm i.d., 1.7 μm, Waters, USA). The mobile phase A was 0.1% formic acid in water, and B was acetonitrile/isopropanol (1
:
1) containing 0.1% formic acid. The linear gradient was set as follows: 0–3 min: 0–20% B, 3–9 min: 20% B to 60% B, 9–11 min: 60% B to 100% B, 11–13.5 min: 100% B, 13.5–13.6 min: 100% B to 0% B, 13.6–16 min: 0% B. The acquisition rate was 0.4 mL min−1, and the column temperature was maintained at 40 °C. The TOF mass range was m/z 50–1000 in the positive and negative ion scanning modes. The MS data acquisition parameters were set as follows: ion source temperature, 120 °C; desolvation temperature, 500 °C; sheath gas flow, 15 L min−1; capillary voltage, 3000 V; electrospray capillary voltage, 10 kV; injection voltage, 40 V; collision voltage, 60 V.
Metabolomic profiling was carried out following the method of Yan Liu.14 Briefly, the raw data was transferred to Progenesis QI software (Waters Co., Milford, MA, USA) for baseline filtering, peak identification, integration, retention time correction, and peak alignment, and subsequently, a data matrix of retention time, mass-to-charge ratio, and peak intensity was obtained. Then, the metabolites were identified by Progenesis QI according to mass fragmentation (MS/MS analysis) and appropriate standards from the available online database, including HMDB (http://www.hmdb.ca) and METLIN (https://metlin.scripps.edu). The final matrix was further reduced after removing the peaks with the missing values in more than 60% of samples. Unsupervised principal component analysis (PCA) and orthogonal partial least squares-discriminant analysis (OPLS-DA) were subsequently used to evaluate the overall difference between groups. The metabolic pathway was analyzed using the Kyoto Encyclopedia of Genes and Genomes (KEGG) database resource (https://www.kegg/jp).
Analysis of gut microbiota
Gut microbiota analyzed in this study were the same as our previous study, which were carried out by 16S rRNA approach, and the data are accessible in the European Nucleotide Archive (ENA) database under accession ID PRJEB33981.34 In brief, microbial DNA was extracted from fecal samples using the FastDNA Spin Kit for Soil (Mpbio, Santa Ana, CA). The V3–V4 hypervariable region of the bacteria 16S rRNA gene was amplified with primers 338F (5′-ACTCCTACGGGAGGCAGCAG-3′) and 806R (5′-GGACTACHVGGGTWTCTAAT-3′) by a thermocycler PCR system (GeneAmp 9700, ABI). The purified amplicons were pooled in equimolar and paired-end sequenced (2 × 300) on an Illumina MiSeq platform (Illumina, San Diego, USA) by Majorbio Bio-Pharm Technology Co. Ltd (Shanghai, China). The raw reads from each sample were quality-filtered, trimmed, de-noised, merged, and then the chimeric sequences were identified and removed using the QIIME2 dada2 plugin to obtain the feature table of amplicon sequence variant (ASV) (Callahan et al. 2016). The remaining unique reads were clustered into operational taxonomic units (OTUs) based on the Silva database (SILVA) by UPARSE with a 97% similarity cutoff and generate the taxonomy table. Diversity metrics, including alpha diversity indices and beta diversity distance measurements, were calculated using the core-diversity plugin by QIIME2 (version 1.7.0) and R software (version 2.15.3). Appropriate methods include unsupervised principal component analysis (PCA), orthogonal partial least squares-discriminant analysis (OPLS-DA), and Wilcoxon rank-sum test were subsequently used to evaluate the bacterial difference among the samples and groups. Co-occurrence analysis was performed by calculating Spearman's rank correlations between the predominant bacteria and serum metabolites to display the association and the potential contributions of gut microbiota on metabolites.
RNA preparation and qRT-PCR analysis
To measure the expression of AhR and its target genes in liver samples, total RNA was extracted by Omega Total RNA Kit (Omega Bio. Inc., Changsha, China). RNA was reverse transcribed using PrimeScript RT Master Mix (TaKaRa Bio. Inc., Takara, Japan). Quantitative real-time reverse-transcription PCR (qRT-PCR) was performed using SYBR Green Master Mix and QuantStudio 3 Flex (Thermo Fisher Scientific Inc., Shanghai, Beijing) according to the manufacturer's guidelines. Experiments were performed in triplicate in a single plate, and the relative quantification was calculated using the 2−ΔΔCt method. Primer sequences for the targeted mouse genes, including AhR, Cyp1a1, Pemt, and Fasn are listed in the supplementary materials (Table S6†).
Statistical analysis
The differences in the relative serum metabolite levels and the relative abundance of gut microbiota (OTUs) in each group were both calculated using the Kruskal–Wallis test, and post hoc analysis was done with Bonferroni's corrected for multiple comparisons by SPSS 22 software (IBM) [Tables S2–S4 (ESI)†]. The differences in the PC/PE ratios between the groups were analyzed using Student's t-tests [Tables S3 and S4 (ESI)†]. The levels of metabolites including PC, LysoPC, and PE, which were annotated in glycerophospholipid metabolism pathway using KEGG database resource, were expressed as the mean ± standard error of the mean (S.E.M.), and they were analyzed by one-way ANOVA followed by Dunnett's test and graphically presented using Graph Pad Prism software 6.0 (La Jolla, CA) in Table S5 (ESI).† The correlation between the relative abundance of OTUs and metabolites was analyzed by Spearman's correlation (R software version 2.15.3). The difference between the means was considered statistically significant at *P ≤ 0.05, **P ≤ 0.01, and ***P ≤ 0.001.
Results
FBT ameliorated the serum metabolomic disorders induced by HFD
To investigate the metabolic effects of FBT in obese mice, HFD-fed male C57BL/6J mice were supplemented with FBT at the dose of 400 mg per kg per day for 8 consecutive weeks, and their serum samples were analyzed using UPLC-Triple TOF-MS in positive and negative modes. After peak alignment, 9394 effective peaks were observed in all groups, in which 438 metabolites were identified. PCA and OPLS-DA models were subsequently applied to correctly discriminate variable metabolites among the low-fat diet group (WTND) and the FBT supplemented group (WTHFDH) compared with the high-fat diet group (WTHFD). The variables, with VIP cut-off values of 1.0 and p-values of 0.05, were used to select the significant discriminant metabolites associated with the HFD approach and FBT supplementation. In PCA plots, a clear separation between samples in WTHFD and WTND was observed, and the WTHFDH group significantly diverged from the WTHFD group (Fig. S1†). The OPLS-DA score plot showed clear discrimination between each group, with score plots of (R2Y = 0.989, Q2 = 0.881) for WTND vs. WTHFD and (R2Y = 0.989, Q2 = 0.900) for WTHFDH vs. WTHFD, indicating that the model has good discrimination and prediction degree (Fig. 1A and C; Table S1†). Forty-one (9.36%) of 438 metabolites differed between the WTND and WTHFD groups, and forty-three (9.82%) of 438 metabolites in the WTHFDH group differed from the WTHFD group significantly (Fig. 1B and D). Among these differential metabolites, 24 metabolites were repetitive between these two comparative groups. Thus, 36 differential metabolites were identified as targeted metabolites to display differences across all groups (Fig. 2).
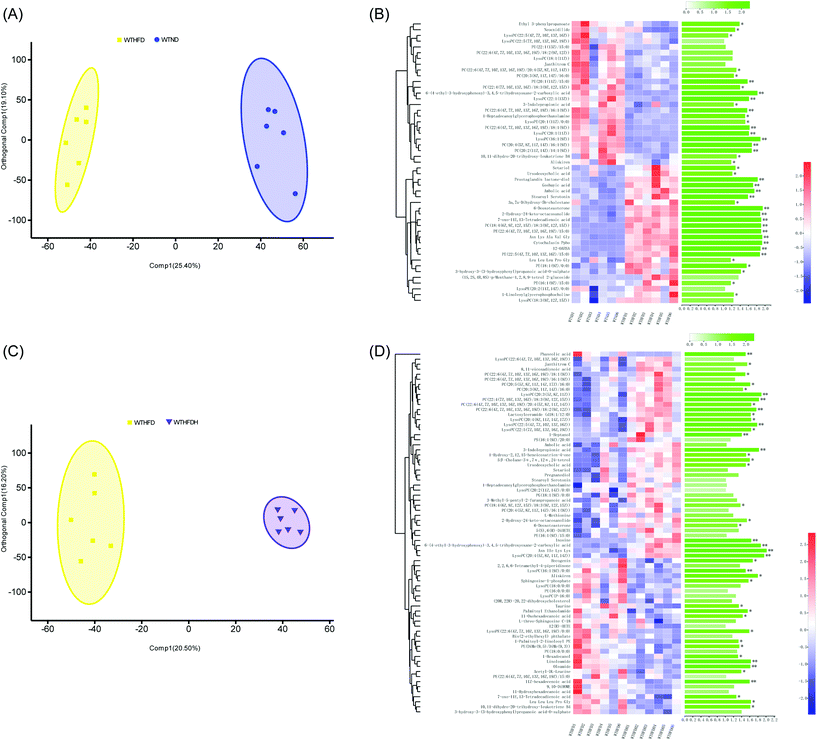 |
| Fig. 1 Effects of Fuzhuan brick tea on the serum metabolic profiles in high-fat diet-induced obese mice in healthy groups. (A) Orthogonal partial least squares-discriminant analysis (OPLS-DA) plots of serum metabolome show discrimination between the high-fat diet fed group (WTHFD) and the low-fat diet fed group (WTND); (B) heatmap with hierarchical clustering of individual metabolites, the most relevant for discriminating the variable importance in the projection (VIP) in OPLS-DA models and VIP scores between the high-fat diet fed group (WTHFD) and the low-fat diet fed group (WTND); (C) orthogonal partial least squares-discriminant analysis (OPLS-DA) plots of serum metabolome show discrimination between the Fuzhuan brick tea supplemented group (WTHFDH) and the high-fat diet fed group (WTHFD); (D) heatmap with hierarchical clustering of individual metabolites, relevant for discriminating the variable importance in the projection (VIP) in OPLS-DA models and VIP scores between the Fuzhuan brick tea supplemented group (WTHFDH) and the high-fat diet fed group (WTHFD). The green bars indicate the P values, adjusted from Wilcoxon rank-sum test (corrected for multiple hypothesis testing with the Benjamini–Hochberg method), *P < 0.05, **P < 0.01, ***P < 0.001. | |
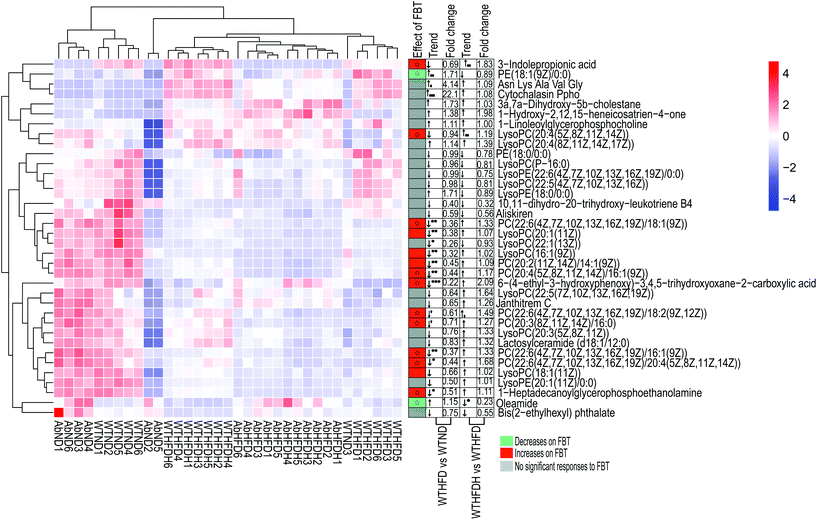 |
| Fig. 2 Heatmap of the 36 significantly differential metabolites, showing beneficial effects of Fuzhuan brick tea on serum metabolism in healthy mice and gut microbiota-depleted mice. The metabolites values are indicated by the colors of the squares, and the similarity among metabolites values or samples is presented by hierarchical clustering. The changes in the direction and folds of differential metabolites induced by high-fat diet (HFD) or Fuzhuan brick tea (FBT) supplementation, shown in Table S3 (ESI),† are represented by arrows (↑ or ↓). The arrows, (↑) and (↓), represented more and fewer values of differential metabolites in the HFD fed group (WTHFD) compared to the low-fat diet fed group (WTND), or the Fuzhuan brick tea supplemented group (WTHFDH) compared to the HFD fed group (WTHFD) in healthy mice. The asterisk (*, **, and ***) indicate significant differences between the means (P < 0.05, P < 0.01, and P < 0.001, respectively). Metabolites significantly increased and decreased by FBT, are shown in red and green, respectively. Other metabolites, not significantly influenced by FBT, are shown in gray. The star (☆) indicates metabolites with an adverse change in direction after HFD treatment alone and supplied with FBT, in which significant adverse change was marked with a solid star (★). See also Table S3 (ESI).† | |
Eleven phospholipids (30.56%) of the 36 differential metabolites in the WTHFD group were significantly reduced in contrast to the WTND group (P < 0.05) while reversed by FBT supplementation, implying that FBT improved the HFD-induced metabolic disturbances. Specifically, FBT significantly multiplied 1.49, 1.19, and 1.83 times over the levels of PC (22:6(4Z,7Z,10Z,13Z,16Z,19Z)/18:2(9Z,12Z)), LysoPC (20:4(5Z,8Z,11Z,14Z)), and 3-indole propionic acid in the WHHFDH group compared to the WTHFD group. However, FBT restrained the significant increase of PE (18:1(9Z)/0:0) in the WTHFD group compared to the WTND group. The increased level of oleamide under HFD was also significantly normalized after FBT supplementation. Interestingly, FBT supplementation restored the HFD-induced decrease in 7 PCs (PC (22:6(4Z,7Z,10Z,13Z,16Z,19Z)/18:2(9Z,12Z)), PC (20:3(8Z,11Z,14Z)/16:0), PC (22:6(4Z,7Z,10Z,13Z,16Z,19Z)/20:4(5Z,8Z,11Z, 14Z))), PC (20:2(11Z,14Z)/14:1(9Z)), PC(20:4(5Z,8Z,11Z,14Z)/16:1(9Z)), PC (22:6(4Z,7Z,10Z,13Z,16Z,19Z)/16:1(9Z)), and PC (22:6(4Z,7Z,10Z,13Z,16Z,19Z)/18:1(9Z)) and 3 LysoPCs (LysoPC (16:1(9Z)), LysoPC (20:1(11Z)), and LysoPC (20:4(5Z,8Z,11Z,14Z)), while decreased the PE level (PE (18:1(9Z)/0:0). The serum samples in the WTHFDH group contained higher ratios of PC (sum of the 7 key PCs) to PE (PC/PE = 0.54 versus 0.39, P > 0.05; Fig. 2; Table S3†) than those in the WTHFD group. It was reported that HFD could affect the PC synthesis or the conversion of PE to PC through decreasing the expression of genes, namely choline-phosphate cytidylyltransferase A (Pcyt1a) and Pemt, which subsequently caused an increase in the PC/PE ratio.4,8,9,35 These results suggested that serum metabolites responded to both HFD and FBT, and FBT effectively improved HFD-induced metabolic aberrations hallmarked by the low ratio of PC to PE.
The metabolic regulations of FBT in HFD-induced obese mice were less effective when gut microbiota was depleted
To address the metabolic effects of microbiota depletion caused by antibiotic treatment, the serum metabolic profiles of all antibiotics-treated mice were observed. PCA and OPLS-DA were also performed for obtaining the comparative interpretations and investigating the variations among the low-fat diet group (AbND) and the FBT supplemented group (AbHFDH) groups compared to the high-fat diet group (AbHFD) group. In PCA plots, the AbHFD and AbHFDH groups were clearly distinguished from the AbND group, while the AbHFDH group did not differ from the AbHFD group completely, suggesting that the consumption of FBT could not normalize the HFD-induced disordered metabolism (Fig. S1†). Meanwhile, the corresponding OPLS-DA loading plots identified 45 and 41 differential metabolites (VIP > 1.0 and P < 0.05), responsible for discriminating AbND vs. AbHFD and AbHFDH vs. AbHFD, respectively. Among them, 21 differential metabolites were similar to those in the healthy mice, but most of them showed no significant difference in distribution between each group in the microbiota-depleted mice (Fig. 2 and 3; Table S4†). The metabolic responses of FBT in HFD-fed healthy mice, such as increase of PC, LysoPC, 3-indole propionic acid, and decreased oleamide and PE (18:1(9Z)/0:0), vanished definitely in the microbiota-depleted mice. Contrarily, the significant increase of serum cytochalasin Ppho and 3a,7a-dihydroxy-5b-cholestane induced by HFD were reversed after FBT supplementation in the microbiota-depleted mice. Moreover, a reverse trend for the ratios of 7 PCs (increased by FBT in healthy mice) to PE (18:1(9Z)/0:0) was observed in the AbHFDH and AbHFD groups (PC/PE = 0.35 versus 0.62, P > 0.05; Fig. 2; Table S4†) compared to the WTHFDH and WTHFD groups (PC/PE = 0.54 versus 0.39, P > 0.05; Fig. 2; Table S3†). These results indicated that the metabolic responses of FBT in HFD-fed mice were much different after antibiotic treatment.
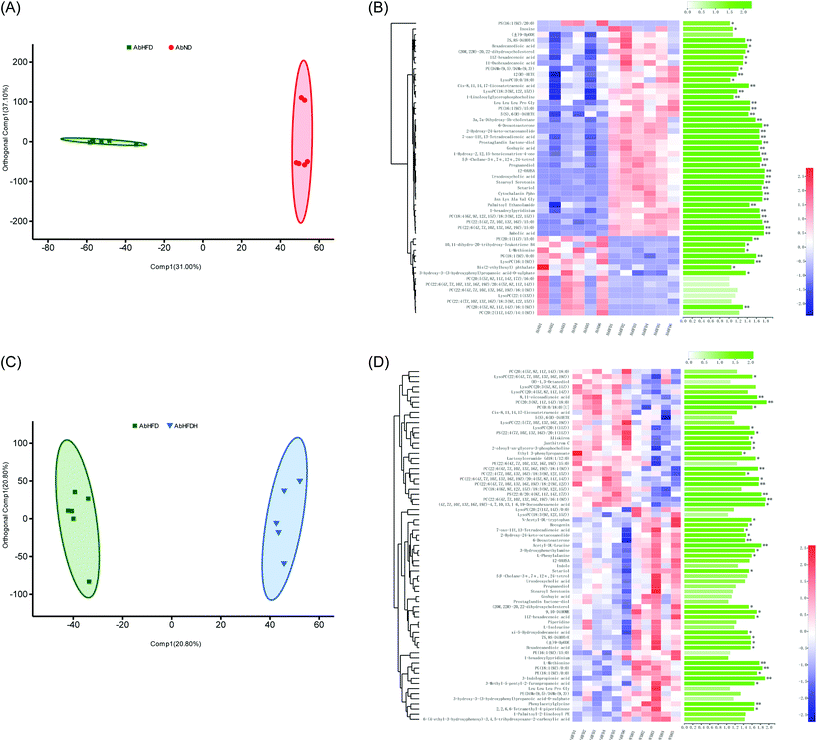 |
| Fig. 3 Effects of Fuzhuan brick tea on serum metabolic profiles in high-fat diet-induced obese mice in gut microbiota-depleted groups. (A) Orthogonal partial least squares-discriminant analysis (OPLS-DA) plots of serum metabolome show discrimination between the high-fat diet fed group (AbHFD) and the low-fat diet fed group (AbND); (B) heatmap with hierarchical clustering of individual metabolites, the most relevant for discriminating the variable importance in the projection (VIP) in OPLS-DA models and VIP scores between the high-fat diet fed group (AbHFD) and the low-fat diet fed group (AbND); (C) orthogonal partial least squares-discriminant analysis (OPLS-DA) plots of serum metabolome show discrimination between the Fuzhuan brick tea supplemented group (AbHFDH) and the high-fat diet fed group (AbHFD); (D) heatmap with hierarchical clustering of individual metabolites, the most relevant for discriminating the variable importance in the projection (VIP) in OPLS-DA models and VIP scores between the Fuzhuan brick tea supplemented group (AbHFDH) and the high-fat diet fed group (AbHFD). The green bars indicate the p values, adjusted from Wilcoxon rank-sum test (corrected for multiple hypothesis testing with the Benjamini–Hochberg method), * P < 0.05, ** P < 0.01, *** P < 0.001. | |
To understand the effect of antibiotic treatment on the metabolism of mice, OPLS-DA analysis was also performed for distinguishing the difference in metabolic profiles between the antibiotic-treated groups and the untreated groups under the same diet or FBT intervention. The corresponding OPLS-DA loading plots showed that 32 metabolites, including 3 PEs, 3 PCs, 3 LysoPCs, and 3-indole propionic acid, differed significantly between the microbiota-depleted mice and their corresponding healthy groups (AbND vs. WTD, AbHFD vs. WTHFD, and AbHFDH vs. WTHFDH) (Fig. 4). The results suggested that antibiotic treatment would have consistent impacts on the metabolism of mice, though it was unclear whether the metabolic consequences of antibiotic treatment were due to the depletion of gut microbiota or antibiotics themselves, or a combination thereof. In the 16 metabolites that FBT regulated significantly in healthy mice, the serum levels of 7 metabolites including PC(20:2(11Z,14Z)/14:1(9Z)), PC(20:4(5Z,8Z,11Z,14Z)/16:1(9Z)), LysoPC(16:1(9Z)), LysoPC(20:1(11Z)), LysoPE(20:1(11Z)/0:0), 1-heptadecanoylglycerophosphoethanolamine, and 3-indole propionic acid in microbiota-depleted mice were significantly lower than those of healthy mice. Notably, 3-indolepropionic acid was decreased by 94% in the gut microbiota-depleted mice compared to their respective healthy counterparts (Fig. 2; Table S4†). These results indicated that antibiotic treatment partly abolished the protective effects of FBT on the HFD-induced metabolic aberrance.
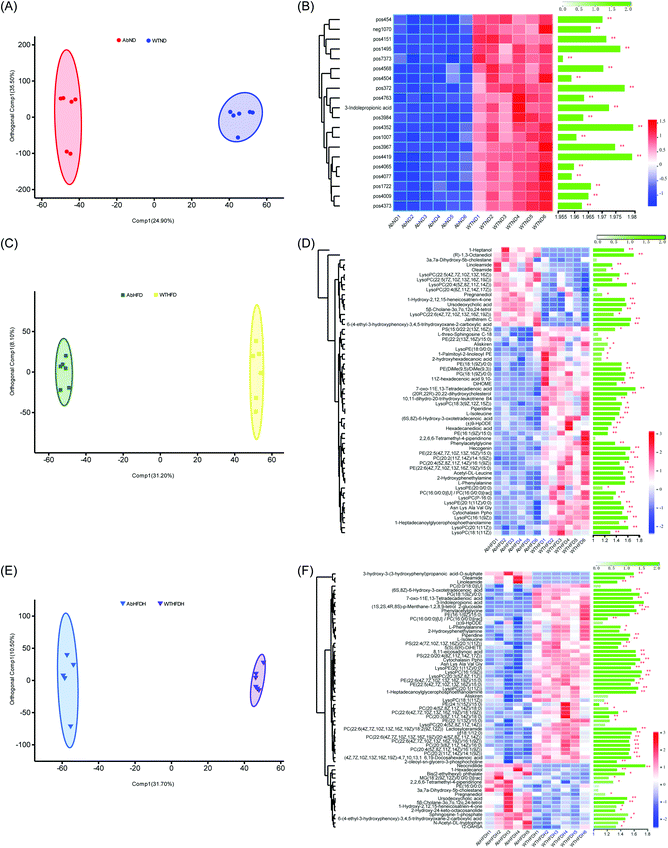 |
| Fig. 4 Effects of antibiotic-induced microbiota-depletion on serum metabolic profiles in high-fat diet-induced obese mice. (A) Orthogonal partial least squares-discriminant analysis (OPLS-DA) plots of serum metabolome show discrimination between the microbiota-depleted mice fed with low-fat diet (AbND) and their respective healthy counterparts (WTND); (B) heatmap with hierarchical clustering of individual metabolites, the most relevant for discriminating the variable importance in the projection (VIP) in OPLS-DA models and VIP scores between the microbiota-depleted mice fed with low-fat diet (AbND) and their respective healthy counterparts (WTND); (C) orthogonal partial least squares-discriminant analysis (OPLS-DA) plots of serum metabolome show discrimination between the microbiota-depleted mice fed with high-fat diet (AbHFD) and their respective healthy counterparts (WTHFD); (D) heatmap with hierarchical clustering of individual metabolites, the most relevant for discriminating the variable importance in the projection (VIP) in OPLS-DA models and VIP scores between the microbiota-depleted mice fed with high-fat diet (AbHFD) and their respective healthy counterparts (WTHFD); (E) orthogonal partial least squares-discriminant analysis (OPLS-DA) plots of serum metabolome show discrimination between the microbiota-depleted mice supplemented with Fuzhuan brick tea and fed with high-fat diet (AbHFDH) and their respective healthy counterparts (WTHFDH); (F) heatmap with hierarchical clustering of individual metabolites, the most relevant for discriminating the variable importance in the projection (VIP) in OPLS-DA models and VIP scores between the microbiota-depleted mice supplemented with Fuzhuan brick tea and fed with high-fat diet (AbHFDH) and their respective healthy counterparts (WTHFDH). The green bars indicate the P values, adjusted from Wilcoxon rank-sum test (corrected for multiple hypothesis testing with the Benjamini–Hochberg method), *P < 0.05, ** P < 0.01, *** P < 0.001. | |
The comparative study of metabolic profiles in microbiota-depleted and healthy mice revealed that the metabolic effects of antibiotics could not be completely ruled out. The direct effects of antibiotics themselves and indirect effects induced by microbiota depletion should be considered since antibiotics would affect metabolic gene expressions directly and microbiota and their metabolites could mediate external administrations and host metabolism.36,37 However, most of the regulatory mechanisms of metabolic genes by antibiotics were unknown and whether the regulations were related to microbiota remained unclear. Therefore, the indirect effects of antibiotics through gut microbiota depletion were of greater concern. Numerous studies have shown that antibiotic exposure resulted in lipid, glucose metabolism disorders and liver function abnormality. Accompanying the metabolic changes are a series of genes and intestinal flora alterations.38–40 Gut microbiota depletion and microbial metabolites have been proved to be closely related to antibiotic-caused metabolic changes.37,39 Many microbiome-related metabolic pathways underlying metabolic diseases after antibiotic treatment, such as bile acids metabolism, tryptophan metabolism, branched-chain amino acids metabolism, and shot-chain fatty acids metabolism, have been successively found. Almost without exception, the altered intestinal barrier and ecological disorders induced by antibiotics played important roles in host metabolism.37,41 This study indeed demonstrated that gut microbiota acted as key mediators for FBT in regulating metabolism. Once these mediators disappeared, FBT lost its metabolic effects. It was likely that the invalid metabolic regulation of FBT in microbiota-depleted mice could be explained by microbiota absence. All these findings indicated that gut microbiota was necessary for FBT to regulate the metabolism in obese mice.
Correlations between gut microbiota and serum metabolites regulated by FBT in the healthy mice
FBT treatment significantly improved the HFD induced metabolic disorders in healthy mice but not in the microbiota-depleted mice, thereby strongly indicating that the metabolic benefits of FBT were influenced by the gut microbiota. Though the moderating effect of FBT on mice gut microbiota has been reported in our previous study, the possible biological relevance of the gut microbiota and metabolic variation regulated by FBT were still unclear.34 Thus, the spearman's rank correlations between the relative abundance of dominant gut microbiota (38 OTUs) and 36 key metabolites regulated by FBT were investigated in the healthy mice.
Strong correlations were observed in 403 OTU-metabolite pairs (r > 0.5 or r < −0.5, P < 0.05; Fig. 5). The gut microbiota was significantly down-regulated by FBT, including OTU415 and OTU391 in the family Lachnospiraceae, OTU270, OTU443, OTU307, OTU442, and OTU494 in the family Ruminococcaceae, OTU435 in the family Lactobacillaceae, and OTU401 in the family Family_XIII, were significantly and negatively correlated with 7 serum PCs and 3 LysoPCs that were upregulated by FBT (as discussed above). Contrarily, these 9 OTUs were significantly and positively correlated with PE (18:1(9Z)/0:0) that were significantly down-regulated by FBT. Besides, OTU416 was significantly and positively correlated with oleamide, another metabolite significantly downregulated by FBT. Moreover, the gut microbiota significantly upregulated by FBT, including OTU12 in the family Porphyromonadaceae, OTU593 and OTU594 in the family Bacteroidales_S24-7_group, were significantly and positively correlated with the seven PCs and three LysoPCs that were upregulated by FBT. However, these gut microbiota were significantly and negatively correlated with PE(18:1(9Z)/0:0). Other metabolites that were significantly upregulated by FBT, including 3-indole propionic acid and LysoPC(20:4(5Z,8Z,11Z,14Z)), were significantly and positively correlated with the gut microbiota that were remarkably upregulated by FBT, such as OUT320, OUT389, OUT323, OUT454, OUT578, and OUT121. Notably, OTU600 in the family Lactobacillaceae upregulated by FBT, was significantly and positively correlated with the FBT-upregulated PCs (PC(22:6(4Z,7Z,10Z,13Z,16Z,19Z)/16:1(9Z)), PC(22:6(4Z,7Z,10Z,13Z,16Z,19Z)/18:1(9Z)), PC(22:6(4Z,7Z,10Z,13Z,16Z,19Z)/18:2(9Z,12Z)), PC(20:3(8Z,11Z,14Z)/16:0), and PC(22:6(4Z,7Z,10Z,13Z,16Z,19Z)/20:4(5Z,8Z,11Z,14Z)). Contrarily, OTU600 showed a significant and negative correlation with the FBT-downregulated PE (18:1(9Z)/0:0). Taken together, significant negative correlations were observed between the downregulated OTUs and upregulated PCs, LysoPCs, and 3-indole propionic acid, or conversely, between the upregulated OTUs and the downregulated PE(18:1(9Z)/0:0) after FBT supplementation. Contrarily, the upregulated OTUs were significantly and positively correlated with the upregulated metabolites after FBT supplementation and vice versa. Given the significant correlations between the dominant OTUs and metabolites and the abolished metabolic regulation of FBT in the microbiota-depleted mice, gut microbiota orchestrated the metabolic regulation of FBT in HFD-fed mice.
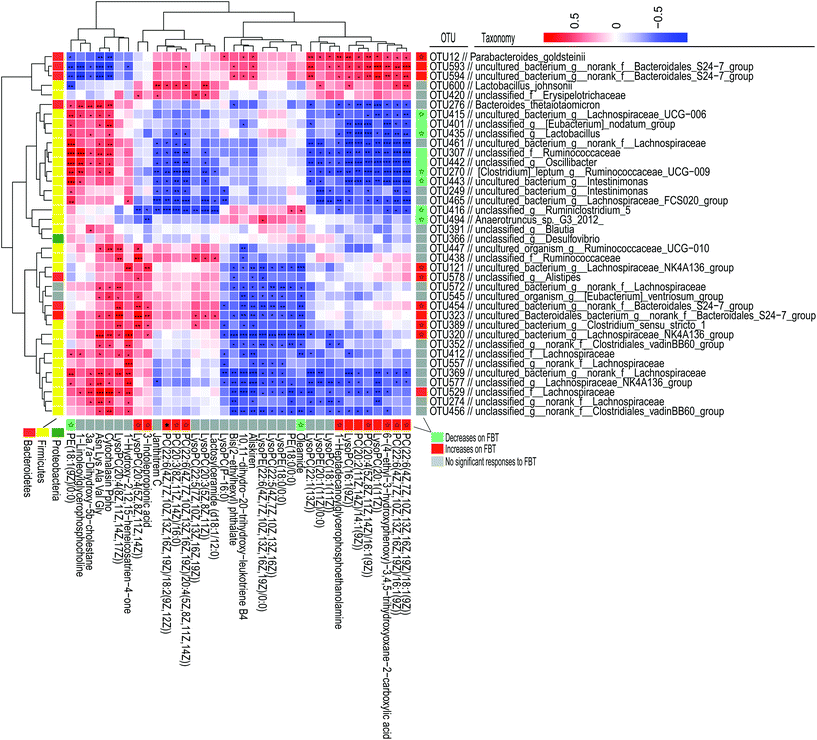 |
| Fig. 5 Spearman's correlations between the significantly altered intestinal microbiota and significantly altered serum metabolites by Fuzhuan brick tea in healthy mice. The heatmap shows Spearman's rank correlations between significantly altered serum metabolites by Fuzhuan brick tea and the relative abundance of selected operational taxonomic units (OTUs), as reported in our previous study.34 The asterisk (*, **, and ***) indicate the association significance (P < 0.05, P < 0.01, and P < 0.001, respectively). Hierarchical clustering is presented for both OTUs and metabolites, based on the Euclidean distances between their presented Spearman's rank correlations. The color code, used in the column on the right and under the heatmap, indicates OTUs and metabolites, significantly influenced by high-fat diet (HFD) and Fuzhuan brick tea (FBT). OTUs or metabolites significantly increased and decreased by FBT, are shown in red and green, respectively. Other OTUs and metabolites, not significantly influenced by FBT, are shown in gray. The star (☆) indicates OTUs or metabolites, showing an adverse change in direction for HFD alone treated animals and FBT supplemented mice, in which a significant adverse change was denoted with a solid star (★). See also Tables S2 and S3 (ESI).† | |
The potential regulation of FBT on metabolic pathways perturbed by HFD
To further investigate the mechanism of FBT for regulating HFD-induced metabolic aberrations by altering gut microbiota, the metabolic pathway analysis based on the differential metabolites identified in healthy mice and microbiota-depleted mice was conducted by KEGG topology (Fig. 6). Three metabolic pathways were significantly enriched between the WTND and WTHFD groups (P < 0.05), including glycerophospholipid metabolism (impact value = 0.6000), choline metabolism in cancer (impact value = 0.4000), and retrograde endocannabinoid signaling (impact value = 0.4000). Glycerophospholipid metabolism (impact value = 0. 8571) and choline metabolism in cancer (impact value = 0.5714) were also the most enriched metabolic pathways between the WTHFDH and WTHFD groups (though not significant; p > 0.05). For the microbiota-depleted mice, glycerophospholipid metabolism (impact value = 0.8571), retrograde endocannabinoid signaling (impact value = 0.2857) and arachidonic acid metabolism (impact value = 0.2857) were significantly matched between the AbND and AbHFD groups (P < 0.05). Glycerophospholipid metabolism (impact value = 0.3333) and choline metabolism in cancer (impact value = 0.3333) were the most enriched metabolic pathways between the AbHFDH and AbHFD groups (though not significant; p > 0.05). To evaluate the metabolic pathways associated with antibiotic treatment, KEGG enrichment analyses were also performed in the microbiota-depleted mice compared to their respective healthy counterparts depending on their differential metabolites. The results showed that only retrograde endocannabinoid signaling (impact value = 0.2000, 0.1818) was identified as a significantly perturbed metabolic pathway for AbHFD vs. WTHFD and AbHFDH vs. WTHFDH (P < 0.05), suggesting that retrograde endocannabinoid signaling was associated with antibiotic treatment. Thus, glycerophospholipid metabolism and choline metabolism in cancer were determined as the two most important metabolic pathways for FBT to ameliorate the HFD-induced metabolic disturbances and the schematic of metabolic changes is illustrated in Fig. 7. Specifically, 31 metabolites, including 13 LysoPCs, 12 PCs, and 6 PEs, were figured out to be closely involved in glycerophospholipid metabolism. The 13 LysoPCs and 12 PCs were also involved in choline metabolism in cancer, and 13 LysoPCs and 6 PEs were also involved in retrograde endocannabinoid signaling (Table S5†). Most strikingly, the levels of PCs and LysoPCs in the WTHFD were extensively higher than those in the WTND group, while the levels of PEs turned out to be the opposite. Fortunately, the reduced levels of PCs along with the elevation of PEs were further reversed by FBT supplementation (Fig. 7; Table S5†).
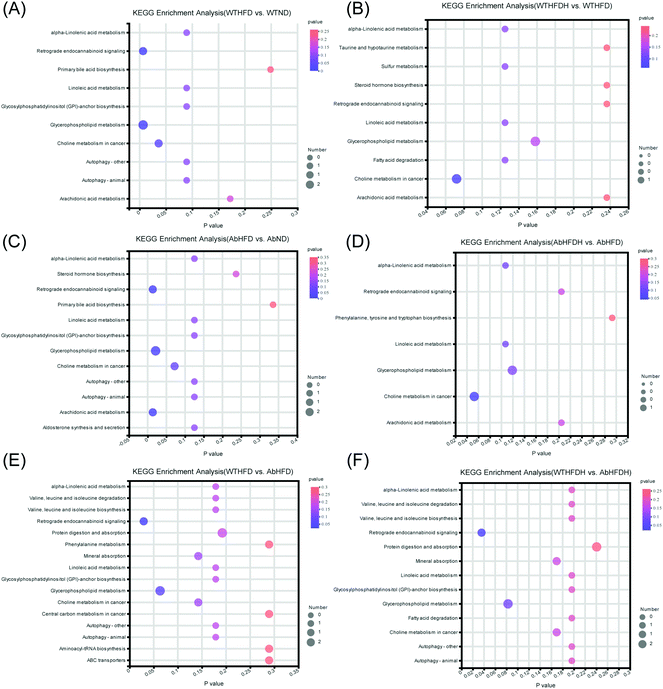 |
| Fig. 6 Bubble plots of altered metabolic pathways in serum of mice based on significantly differential metabolites are visualized by bubble plots. The altered metabolic pathways were filtered out by KEGG enrichment analysis based on the significantly differential metabolites in each comparison. Bubble area is proportional to the impact of each pathway, with the colour denoting the significance from highest (in orange) to lowest (in purple). (A) Metabolism pathways show a comparison between the high-fat diet fed group (WTHFD) and the low-fat diet fed group (WTND) in healthy mice; (B) metabolism pathways show a comparison between the Fuzhuan brick tea supplemented group (WTHFDH) and the high-fat diet fed group (WTHFD) in healthy mice; (C) metabolism pathways show a comparison between the high-fat diet fed group (AbHFD) and the low-fat diet fed group (AbND) in microbiota-depleted mice; (D) metabolism pathways show a comparison between the Fuzhuan brick tea supplemented group (AbHFDH) and the high-fat diet fed group (AbHFD) in microbiota-depleted mice; (E) metabolism pathways show comparison between the microbiota-depleted mice fed with high-fat diet (AbHFD) and their respective healthy counterparts (WTHFD); (F) metabolism pathways show comparison between the microbiota-depleted mice supplemented with Fuzhuan brick tea and fed with high-fat diet (AbHFDH) and their respective healthy counterparts (WTHFDH). | |
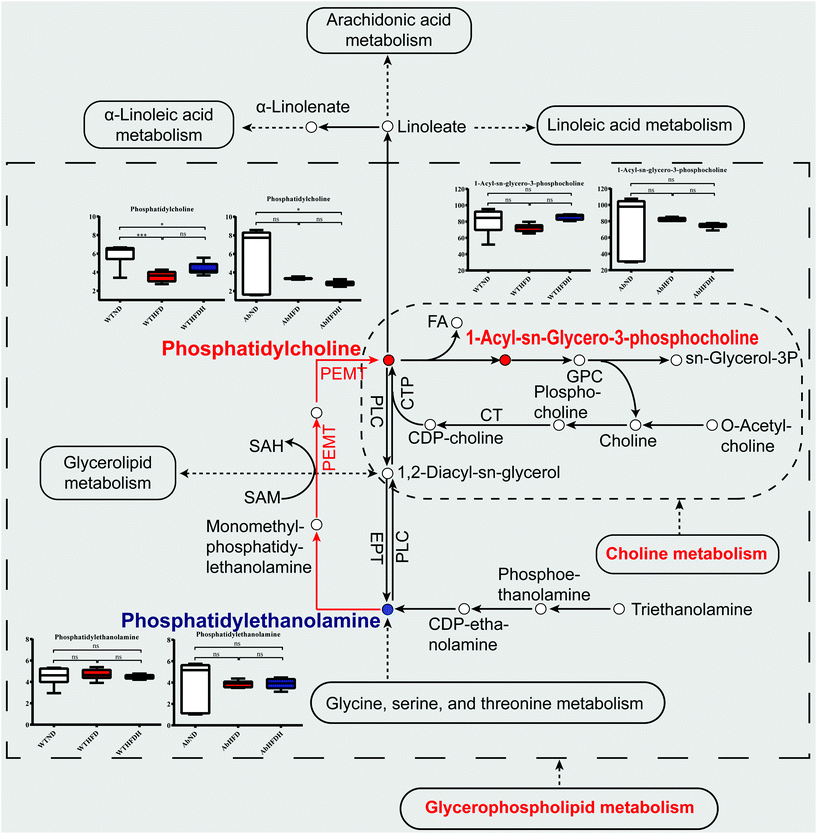 |
| Fig. 7 Simplified view of the major metabolic pathways significantly altered by Fuzhuan brick tea and involved metabolite values in healthy mice and microbiota-depleted mice. The metabolic pathways visualized glycerophospholipid metabolism and choline metabolism in cancer that were significantly altered by Fuzhuan brick tea in healthy mice. The solid spots in red or blue indicate that the metabolites were increased or decreased after Fuzhuan brick tea supplementation in healthy mice, respectively. The red arrows indicated the upregulated phosphatidylcholines (PCs) to phosphatidylethanolamines (PEs) conversion catalyzed by phosphatidylethanolamine N-methyltransferase (Pemt). The serum metabolites values were expressed as the mean ± standard error of the mean (S.E.M.). See also Table S5 (ESI).† | |
To verify whether the elevated serum PCs, LysoPCs, and the reduced PEs after FBT supplementation were attributed to signals associated with glycerophospholipid metabolism, the mRNA expressions of Pemt and its transcription regulator AhR in liver were investigated in healthy mice. As a result, both mRNA expressions of AhR and Pemt were significantly decreased in WTHFD and WTHFDH groups relative to the WTND group, and Pemt mRNA was notably increased after FBT supplementation (Fig. 8A and C). Besides, the expression of AhR typical target gene Cyp1a1 in the liver was significantly upregulated by FBT supplementation, indicating that the AhR activity was activated by FBT, subsequently being responsible for the high expression of Pemt and the promotion of PEs to PCs conversion (Fig. 8B). Moreover, the mRNA level of Fasn showed similar tendencies of AhR and Pemt expressions in the liver. These results indicated that FBT would reverse the inhibition effects of HFD on the PE to PC conversion in liver through the transcription of Pemt depending on AhR, as well as hepatic lipogenesis. However, the disparate changes of PCs, LysoPCs, and PEs observed in gut microbiota-depleted mice suggested that gut microbiota were necessary for FBT to regulate the disorder of glycerophospholipid metabolism and choline metabolism in cancer induced by HFD. We, therefore, hypothesized that FBT could enhance PC synthesis or the PE to PC conversion by shaping gut microbes.
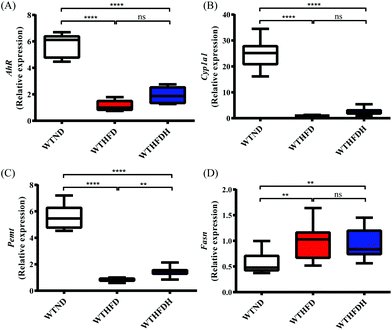 |
| Fig. 8 Effects of Fuzhuan brick tea on AhR and its target genes in the liver of healthy mice. (A) Gene expression of AhR; (B) gene expression of Cyp1a1; (C) gene expression of Pemt; (D) gene expression of Fasn. | |
Discussion
The continuous spread of metabolic abnormalities induced by HFD has presented a global health problem. Gut microbiota and its metabolites often play crucial roles in diet-induced metabolic disorders.3,8,12,13,18,42 Our previous study has elucidated the impact of FBT on gut microbiota and exhibited that FBT supplementation can subsequently reduce HFD-induced obesity.34 However, the metabolic effects of FBT on HFD-induced obese mice and the association of the metabolic response of FBT with gut microbiota are yet to be elucidated. In this study, we aimed at elucidating the metabolic regulation of FBT in HFD-induced obese mice and the underlying mechanisms. The results showed that FBT could improve HFD-induced serum metabolic aberrance in healthy mice but not in microbiota-depleted mice treated with antibiotics. Also, the serum metabolites significantly regulated by FBT were highly correlated to the FBT-shaped gut microbiota. As shown in metabolic pathway analyses, FBT may improve the HFD-induced metabolic aberrance via regulating glycerophospholipid metabolism and choline metabolism in cancer partially depending on gut microbiota.
Glycerophospholipid metabolism has been concerned with various metabolic disorders, such as obesity, atherosclerosis, insulin resistance, and nonalcoholic fatty liver disease.6–8,17,43–46 Several adiponutrin or patatin-like phospholipase, including lysophospholipase-like 1, lipase 3, PLA2G6, and iPLA2β, have been identified as genome-wide significant loci associated with obesity traits by genome-wide association study (GWAS) in humans and mice.47,48 PC and PE, the major glycerophospholipids in mammalian biological membranes, play critical roles in regulating lipid, lipoprotein, and whole-body energy metabolism.6–8 Abnormally low PC/PE ratio can broadly impact obesity and liver disease progressions. Firstly, excessive food intake could inevitably stimulate lipogenesis and accumulation of neutral lipids, including triglyceride, for energy storage in lipid droplets surrounded by the phospholipid monolayer. The relative abundance of PC and PE on the surface of lipid droplets impacts their dynamics and the size of lipid droplets.5,49,50 Therefore, lower PC and/or PE content in HFD or excess-fed animals would promote TG storage and increase the size of the lipid droplets.50,51 Secondly, impaired synthesis of PC and PE or inhibited conversion of PE to PC could lead to a compensatory upregulation of cholesterol regulatory element-binding proteins (SREBPs), which in turn could trigger de novo lipogenesis and would eventually contribute to an elevation of hepatic TG and hence steatosis, obesity and metabolic syndrome.4,5,16,49 Thirdly, depletion of PCs will block the protein trafficking from the endoplasmic reticulum (ER)-to-Golgi, which induce TG accumulation and subsequently decrease lipoprotein secretion, and ultimately deteriorate hepatic steatosis.4,5,52 Moreover, some PCs and LysoPCs are peroxisome proliferator-activated receptor-alpha (PPARα) ligands, which activate PPARα and subsequently improve fatty acid oxidation and affect lipid content in the tissues.53 It is well established that the usual composition and proportion of hepatic and circulation glycerophospholipids constitute a major risk factor for obesity and NAFLD.53,54 The levels of hepatic and circulating PCs, LysoPCs, and the PE/PE ratio in obese or NAFLD patients were generally lower than healthy individuals.55–57 Importantly, these glycerophospholipid metabolic aberrances were considered highly associated with the gut microbiota dysbiosis induced by HFD in rodent models.14,58 Concurring with these findings, our study found that the serum levels of PCs, LysoPCs, and the PC/PE ratio were apparently decreased by HFD compared to the LFD-fed mice. These metabolic phenomena were exactly consistent with the higher hepatic and serum TG levels, bigger lipid droplets in the liver, and epididymal adipose tissues in our previous study.34 Intriguingly, FBT showed significant improvement in the glycerophospholipid metabolic aberrances induced by HFD.
PCs are supplied through diet, or they can be synthesized via the 1,2-diacylglycerol cholinephosphotransferase (CDP-choline) and the Pemt pathway in the liver.5 In rodents, Pemt, a liver enriched enzyme, is responsible for approximately one-third of hepatic PCs biosynthesis.5 Nevertheless, the Pemt pathway, converting PE to PC via three sequential methylation reactions, plays critical physiological roles under certain circumstances, such as starvation, cold, pregnancy, etc.4,5,9 In our study, the decreased PCs or PC/PE ratio in HFD-fed mice was achieved either by a blockage in PCs synthesis or more efficient recycling of PCs in the liver. Though choline content, the raw material for the synthesis of PC via CDP-choline pathway, was much higher in HFD than in LFD (2.58 vs. 1.90 g kg−1), the HFD-fed mice demonstrated a lower abundance of PCs in serum. Hence, the lower serum levels of PCs in HFD-fed mice could not be interpreted as the depressed PC synthesis via the CDP-choline pathway, but it was more likely blocked by the Pemt pathway. Besides, the contents of LysoPCs, the decomposition products of PCs, were less in HFD-fed mice than those in the LFD group. Therefore, the decreased level of PCs in HFD-fed mice could be interpreted as increased utilization of PCs. HFD can disrupt calcium homeostasis and cause chronic liver ER stress in a metabolic context.51 As Pemt was localized to the ER and mitochondria-associated membranes (MAM), the calcium transport defects and hepatic ER stress might affect ER folding capacity and suppress the Pemt expression and activation, leading to a decline in the PC/PE ratio.4,9,51 However, aberrant ER phospholipids may inhibit sarco/endoplasmic reticulum calcium ATPase (SERCA) activity and ER stress, subsequently reducing the secretion of excessive lipids from the liver, and thus inducing hepatosteatosis and ER stress.51 Therefore, the HFD-induced aberrant phospholipid metabolism may disrupt calcium homeostasis, causing liver ER stress, depressing the Pemt pathway, and ultimately resulting in the ‘HFD–ER stress–Pemt depression-decline of PC/PE – ER-stress’ vicious cycle. FBT may depend on breaking this vicious cycle and restoring the PE to PC conversion and ultimately raise the PC/PE ratio.
Besides depending on the ‘HFD–ER stress–Pemt depression-decline of PC/PE – ER-stress’ vicious cycle, HFD may decrease the Pemt activity and decline the PC/PE ratio through various gut microbiota and its metabolites. Specifically, gut microbiota can metabolize tryptophan to produce specific Trp-indole derivates, such as 3-indole propionic acid, indole, indole acetic acid, and 3-methylindole indole.12,13,17 These tryptophan metabolites are endogenous ligands for AhR, a ligand-activated nuclear receptor regulating the immune response at barrier sites and lipid metabolism through AhR signaling.12,15,17,22–24,59–61 After binding with these endogenous ligands, AhR is translocated into the nucleus and binds to dioxin response elements to induce the transcription of genes involved in xenobiotic metabolism and glycerophospholipid metabolism, including Cyp1a1 and Pemt.15,21,62 Thus, gut microbiota-derived Trp-metabolites act as signal molecules to indirectly participate in the regulation of glycerophospholipid metabolism of hosts by interfering with the AhR receptor, and subsequently transcriptionally regulating target genes such as Pemt. Moreover, the key proteins regulating cholesterol and fatty acid metabolites such as fatty acid synthase (Fasn) and cholesterol regulatory element-binding protein 1c (SREBP-1c) can be negatively regulated by AhR, subsequently affecting lipidmetabolism.13,16,17,63,64 Previous studies indicated that gut microbiota-derived indole-3-acetic acid increased the AhR activity and effectively reversed the adipogenesis and liver steatosis in HFD-fed mice.13,15 Besides, the ethanol-induced liver injury could be attenuated by indole-3-acetic acid supplementation.65 Therefore, the microbiota-derived Trp-metabolites might play key roles in glycerophospholipid metabolism via the AhR-Pemt signal axis.
It is important to note that the 3-indole propionic acid, a microbiota-specific AhR ligand, was remarkably decreased in HFD-fed mice concurring with the decreased PC, LysoPC contents, and PC/PE ratio. Fortunately, these metabolic disturbances were remarkably reversed by FBT supplementation in healthy mice. Besides, the mRNA expression of genes involved in the AhR-Pemt signal axis, as well as Cyp1a1, were remarkably elevated by FBT in healthy mice. These results indicated that FBT would improve the HFD-induced glycerophospholipid metabolic disorders by regulating genes, Pemt and Fasn in a manner consistent with 3-indole propionic acid activation of AhR in the liver. However, when the gut microbiota was depleted by antibiotic treatment, the serum levels of 7 metabolites, including 3-indole propionic acid absolutely declined, and an opposite PC/PE ratio was observed after FBT supplementation in the antibiotic-treated mice compared to their respective healthy counterparts. It seemed that the beneficial effects of FBT on glycerophospholipid metabolism were invalid in the absence of gut bacteria after antibiotic treatment. Though there was no report of glycerophospholipid metabolic effects in antibiotic studies, the antibiotics-induced depression in aromatic amino acid metabolism occurring due to gut microbiota depletion has been proved in the microbiota-host co-metabolism by germ-free and microbiome depletion models.36,43,66–68 The metabolic consequences of antibiotic treatment would probably be due to the depletion of gut microbiota or antibiotics themselves, or a combination of both. It is certain that the major metabolic effects of antibiotics were achieved through gut microbiota depletion since only 10% of all metabolites observed in conventionally raised animals were shown in their germ-free counterparts, though gut signaling and colonic metabolism have been proved to be disturbed by antibiotics.43,68,69 These microbiome-related metabolic responses for antibiotics were confirmed again by the nearly perished 3-indole propionic acid in gut microbiota-depleted mice (94% lower than their respective healthy counterparts) in this study. Therefore, the invalid regulation of FBT on HFD-induced metabolic aberration in antibiotic-treated mice may be mainly attributed to its indirect effects on gut microbiota depletion and the absence of the AhR-Pemt signal axis. In other words, gut microbiota and its metabolites such as 3-indole propionic acid could be responsible for FBT to regulate glycerophospholipid metabolism.
Several studies have shown that gastrointestinal AhR-ligand producing bacteria would be altered by HFD, and ultimately lead to metabolic aberrance and associated phenotypic changes.21,22 Some typical gut microbiota, including Bacteroidetes, Lactobacillus spp., Clostridium sporogenes, Peptostreptococcus species, Allobaculum, and Actinobacteria can decompose tryptophan into AhR ligands and subsequently participate in metabolic regulation and obesity control.66,70–75 Several active bacteria in the animal intestine, specifically Lactobacillus rhamnosus GG, Lactobacillus reuteri, and Lactobacillus helveticus, have been proved to ameliorate ethanol-induced liver disease and metabolic syndrome studies.17 In line with the declined AhR-ligand producing bacteria, their tryptophan metabolites, such as indole-3-acetate and 3-indole propionic acid, were definitely depleted when animals were fed with HFD.13,76 By co-analyzing the differences in the metabolite profiles and gut microbiota, Yunci Ma et al. found that the decreased abundance of Bacteroidetes was responsible for the lower content of plasma indole-3-acetic acid and the lower expression of hepatic AhR in HFD-fed mice.16 Moreover, significantly positive correlations between Bifidobacterium and Allobaculum with the fecal levels of indole acrylic acid and indolelatic acid were identified in a metabolic disorder model induced by chronic alcohol consumption.14
Consistent with these researches, our previous study found that various AhR-ligand producing bacteria, including Clostridiaceae, Bacteroidales_S24-7_group, and Lactobacillaceae, were significantly upregulated by FBT in HFD-fed mice.34 By further analysis of metabolism for the same mice in this study, we found that the majority of these AhR-ligand producing bacteria were significantly positively correlated with serum PCs, LysoPCs, and 3-indole propionic acid in healthy mice. However, along with the absence of these bacteria, the key tryptophan metabolite (3-indole propionic acid) nearly disappeared, and FBT lost its improvement on the HFD-induced glycerophospholipid metabolism in the gut microbiota-depleted mice. All these results consistently suggested that FBT may improve the glycerophospholipid metabolism of HFD-fed mice in a microbiota-derived AhR-dependent manner, in which the key tryptophan metabolite (3-indole propionic acid) produced by AhR-ligand producing bacteria played as a mediator in the AhR-Pemt signal axis.
Conclusions
In conclusion, it was found that FBT favorably exhibited improvement on the glycerophospholipid metabolism of HFD-fed mice, indicated by elevated PCs, LysoPCs, and the PC/PE ratio. These glycerophospholipids, as well as a key tryptophan metabolite (3-indole propionic acid), were significantly correlated with several AhR-ligand producing bacteria shaped by FBT in healthy mice. Besides, the invalid metabolic impacts of FBT in the gut microbiota-depleted mice suggested that the FBT-induced regulation of glycerophospholipid metabolism would highly depend upon the gut AhR-ligand producing bacteria. The expression changes of hepatic genes, including AhR, Pemt, Cyp1a1, and Fasn, after FBT supplementation indicated that the most probable mechanism for FBT producing these metabolic benefits involved the AhR-Pemt signal axis. Thereinto, the gut AhR-ligand producing bacteria, as well as their tryptophan metabolite (3-indole propionic acid), played crucial mediators between the AhR activity, Pemt-catalyzed conversion of PCs from PEs, and FBT complementation.
This study demonstrated the critical role of FBT in the metabolic improvement of HFD-fed mice and the potential contribution of gut microbiota. To explore a causal link between gut AhR-ligand producing bacteria and the metabolic protection of FBT, the fecal and hepatic metabolome targeted for glycerophospholipids, luminal tryptophanase along with trp-indole derivates, should be further studied.
Author contributions
Dongmin Liu designed the research and wrote the original manuscript. Jianhui Wang and Hongliang Zeng analyzed and visualized data. Fang Zhou, Beibei Wen, Xiangna Zhang, Yong Luo, and Wenliang Wu investigated the literature and contributed new ideas and analytical tools. Jianan Huang and Zhonghua Liu conceptualized, supervised, and acquired funding for the project and validated the original manuscript. All authors read and approved the manuscript.
Ethical approval
The animal protocol used in this study was approved by the animal care committee at the Animal Center of Hunan Provincial Center for New Drug Safety Evaluation and Research (Hunan, China) and performed in accordance with the guide for the care and use of laboratory animals published by the ministry of health, People's Republic of China.
Conflicts of interest
The authors declare no competing financial interest.
Acknowledgements
This work was supported by China Agriculture Research System of MOF and MARA (CARS-19-09B, CARS-23), National Natural Science Foundation of China (31471706), Hunan Provincial Natural Science Foundation of China (2020JJ5570), Research and Development Program in Key Areas of Hunan Province (2020NK2027), and Changsha Municipal Natural Science Foundation, (kq2007063). The authors wish to thank the Changsha University of Science & Technology, Key Laboratory of Ministry of Education for Tea Science in China, and the National Research Center of Engineering Technology for Utilization of Botanical Functional Ingredients in China for the financial support.
References
- A. J. Romain, J. Marleau and A. Baillot, Association between physical multimorbidity, body mass index and mental health/disorders in a representative sample of people with obesity, Br. J. Prev. Soc. Med., 2019, 73, 874–880 Search PubMed.
-
S. Ettinger, in Nutritional Pathophysiology of Obesity and its Comorbidities, 2017, pp. 1–26, DOI:10.1016/b978-0-12-803013-4.00001-6.
- P. Gerard, Gut microbiota and obesity, Cell. Mol. Life Sci., 2016, 73, 147–162 CrossRef CAS PubMed.
- D. E. Vance, Phospholipid methylation in mammals: from biochemistry to physiological function, Biochim. Biophys. Acta, 2014, 1838, 1477–1487 CrossRef CAS PubMed.
- J. N. van der Veen, J. P. Kennelly, S. Wan, J. E. Vance, D. E. Vance and R. L. Jacobs, The critical role of phosphatidylcholine and phosphatidylethanolamine metabolism in health and disease, Biochim. Biophys. Acta, Biomembr., 2017, 1859, 1558–1572 CrossRef CAS PubMed.
- H. M. Park, K. T. Park, E. C. Park, S. I. Kim, M. S. Choi, K. H. Liu and C. H. Lee, Mass Spectrometry-Based Metabolomic and Lipidomic Analyses of the Effects of Dietary Platycodon grandiflorum on Liver and Serum of Obese Mice under a High-Fat Diet, Nutrients, 2017, 9, 1–17 Search PubMed.
- J. Liu, S. J. Yue, Z. R. Yang, W. W. Feng, X. T. Meng, A. T. Wang, C. Peng, C. Y. Wang and D. Yan, Oral hydroxysafflor yellow A reduces obesity in mice by modulating the gut microbiota and serum metabolism, Pharmacol. Res., 2018, 134, 40–50 CrossRef CAS PubMed.
- S. Wan, F. Kuipers, R. Havinga, H. Ando, D. E. Vance, R. L. Jacobs and J. N. van der Veen, Impaired Hepatic Phosphatidylcholine Synthesis Leads to Cholestasis in Mice Challenged With a High-Fat Diet, Hepatol. Commun., 2019, 3, 262–276 CrossRef CAS PubMed.
- D. E. Vance, Physiological roles of phosphatidylethanolamine N-methyltransferase, Biochim. Biophys. Acta, Mol. Cell Biol. Lipids, 2013, 1831, 626–632 CrossRef CAS PubMed.
- X. Gao, J. N. van der Veen, M. Hermansson, M. Ordonez, A. Gomez-Munoz, D. E. Vance and R. L. Jacobs, Decreased lipogenesis in white adipose tissue contributes to the resistance to high fat diet-induced obesity in phosphatidylethanolamine N-methyltransferase-deficient mice, Biochim. Biophys. Acta, 2015, 1851, 152–162 CrossRef CAS PubMed.
- Y. Zia, A. Al Rajabi, S. Mi, T. T. Ju, K. A. Leonard, R. Nelson, A. Thiesen, B. P. Willing, C. J. Field, J. M. Curtis, J. N. van der Veen and R. L. Jacobs, Hepatic Expression of PEMT, but Not Dietary Choline Supplementation, Reverses the Protection against Atherosclerosis in Pemt(-/-)/Ldlr(-/-) Mice, J. Nutr., 2018, 148, 1513–1520 CrossRef PubMed.
- A. Agus, J. Planchais and H. Sokol, Gut Microbiota Regulation of Tryptophan Metabolism in Health and Disease, Cell Host Microbe, 2018, 23, 716–724 CrossRef CAS PubMed.
- S. Krishnan, Y. Ding, N. Saedi, M. Choi, G. V. Sridharan, D. H. Sherr, M. L. Yarmush, R. C. Alaniz, A. Jayaraman and K. Lee, Gut Microbiota-Derived Tryptophan Metabolites Modulate Inflammatory Response in Hepatocytes and Macrophages, Cell Rep., 2018, 23, 1099–1111 CrossRef CAS PubMed.
- Y. Liu, Y. Luo, X. Wang, L. Luo, K. Sun and L. Zeng, Gut Microbiome and Metabolome Response of Pu-erh Tea on Metabolism Disorder Induced by Chronic Alcohol Consumption, J. Agric. Food Chem., 2020, 68, 6615–6627 CrossRef CAS PubMed.
- Y. C. Kim, S. Seok, S. Byun, B. Kong, Y. Zhang, G. Guo, W. Xie, J. Ma, B. Kemper and J. K. Kemper, AhR and SHP regulate phosphatidylcholine and S-adenosylmethionine levels in the one-carbon cycle, Nat. Commun., 2018, 9, 540 CrossRef PubMed.
- Y. Ma, K. Chen, L. Lv, S. Wu and Z. Guo, Ferulic acid ameliorates nonalcoholic fatty liver disease and modulates the gut microbiota composition in high-fat diet fed ApoE(-/-) mice, Biomed. Pharmacother., 2019, 113, 108753–108763 CrossRef CAS PubMed.
- J. M. Natividad, A. Agus, J. Planchais, B. Lamas, A. C. Jarry, R. Martin, M. L. Michel, C. Chong-Nguyen, R. Roussel, M. Straube, S. Jegou, C. McQuitty, M. Le Gall, G. da Costa, E. Lecornet, C. Michaudel, M. Modoux, J. Glodt, C. Bridonneau, B. Sovran, L. Dupraz, A. Bado, M. L. Richard, P. Langella, B. Hansel, J. M. Launay, R. J. Xavier, H. Duboc and H. Sokol, Impaired Aryl Hydrocarbon Receptor Ligand Production by the Gut Microbiota Is a Key Factor in Metabolic Syndrome, Cell Metab., 2018, 28, 737–750 CrossRef CAS PubMed.
- Y. Heianza, D. J. Y. Sun, X. Li, J. A. DiDonato, G. A. Bray, F. M. Sacks and L. Qi, Gut microbiota metabolites, amino acid metabolites and improvements in insulin sensitivity and glucose metabolism: the POUNDS Lost trial, Gut, 2019, 68, 263–270 CrossRef CAS PubMed.
- Y. Sanz, A. Santacruz and P. Gauffin, Gut microbiota in obesity and metabolic disorders, Proc. Nutr. Soc., 2010, 69, 434–441 CrossRef CAS PubMed.
- R. Liu, J. Hong, X. Xu, Q. Feng, D. Zhang, Y. Gu, J. Shi, S. Zhao, W. Liu, X. Wang, H. Xia, Z. Liu, B. Cui, P. Liang, L. Xi, J. Jin, X. Ying, X. Wang, X. Zhao, W. Li, H. Jia, Z. Lan, F. Li, R. Wang, Y. Sun, M. Yang, Y. Shen, Z. Jie, J. Li, X. Chen, H. Zhong, H. Xie, Y. Zhang, W. Gu, X. Deng, B. Shen, X. Xu, H. Yang, G. Xu, Y. Bi, S. Lai, J. Wang, L. Qi, L. Madsen, J. Wang, G. Ning, K. Kristiansen and W. Wang, Gut microbiome and serum metabolome alterations in obesity and after weight-loss intervention, Nat. Med., 2017, 23, 859–868 CrossRef CAS PubMed.
- T. C. Fung, The microbiota-immune axis as a central mediator of gut-brain communication, Neurobiol. Dis., 2020, 136, 1–9 CrossRef PubMed.
- L. Zhang, R. G. Nichols and A. D. Patterson, The aryl hydrocarbon receptor as a moderator of host-microbiota communication, Curr. Opin. Toxicol., 2017, 2, 30–35 CrossRef PubMed.
- N. G. Girer, D. Carter, N. Bhattarai, M. Mustafa, L. Denner, C. Porter and C. J. Elferink, Inducible Loss of the Aryl Hydrocarbon Receptor Activates Perigonadal White Fat Respiration and Brown Fat Thermogenesis via Fibroblast Growth Factor 21, Int. J. Mol. Sci., 2019, 20, 950–969 CrossRef CAS PubMed.
- V. Osadchiy, J. S. Labus, A. Gupta, J. Jacobs, C. Ashe-McNalley, E. Y. Hsiao and E. A. Mayer, Correlation of tryptophan metabolites with connectivity of extended central reward network in healthy subjects, PLoS One, 2018, 13, e0201772–e0201788 CrossRef PubMed.
- Y. T. Chen, N. S. Yang, Y. C. Lin, S. T. Ho, K. Y. Li, J. S. Lin, J. R. Liu and M. J. Chen, A combination of Lactobacillus mali APS1 and dieting improved the efficacy of obesity treatment via manipulating gut microbiome in mice, Sci. Rep., 2018, 8, 1–11 Search PubMed.
- S. S. Iyer, T. Gensollen, A. Gandhi, S. F. Oh, J. F. Neves, F. Collin, R. Lavin, C. Serra, J. Glickman, P. S. A. de Silva, R. B. Sartor, G. Besra, R. Hauser, A. Maxwell, A. Llebaria and R. S. Blumberg, Dietary and Microbial Oxazoles Induce Intestinal Inflammation by Modulating Aryl Hydrocarbon Receptor Responses, Cell, 2018, 173, 1123–1134 CrossRef CAS PubMed.
- W. Ke, G. Bonilla-Rosso, P. Engel, P. Wang, F. Chen and X. Hu, Suppression of High-Fat Diet-Induced Obesity by Platycodon Grandiflorus in Mice Is Linked to Changes in the Gut Microbiota, J. Nutr., 2020, 150, 1–11 CrossRef PubMed.
- G. Chen, M. Xie, P. Wan, D. Chen, Z. Dai, H. Ye, B. Hu, X. Zeng and Z. Liu, Fuzhuan Brick Tea Polysaccharides Attenuate Metabolic Syndrome in High-Fat Diet Induced Mice in Association with Modulation in the Gut Microbiota, J. Agric. Food Chem., 2018, 66, 2783–2795 CrossRef CAS PubMed.
- T. Wu, J. Xu, Y. Chen, R. Liu and M. Zhang, Oolong tea polysaccharide and polyphenols prevent obesity development in Sprague-Dawley rats, Food Nutr. Res., 2018, 62, 1599–1607 CAS.
- T. Wu, Y. Guo, R. Liu, K. Wang and M. Zhang, Black tea polyphenols and polysaccharides improve body composition, increase fecal fatty acid, and regulate fat metabolism in high-fat diet-induced obese rats, Food Funct., 2016, 7, 2469–2478 RSC.
- T. Wu, L. Yang, X. Guo, M. Zhang, R. Liu and W. Sui, Raspberry anthocyanin consumption prevents diet-induced obesity by alleviating oxidative stress and modulating hepatic lipid metabolism, Food Funct., 2018, 9, 2112–2120 RSC.
- T. Wu, Y. Gao, X. Guo, M. Zhang and L. Gong, Blackberry and Blueberry Anthocyanin Supplementation Counteract High-Fat-Diet-Induced Obesity by Alleviating Oxidative Stress and Inflammation and Accelerating Energy Expenditure, Oxid. Med. Cell. Longevity, 2018, 2018, 4051232–4051241 Search PubMed.
- F. Huang, X. Zheng, X. Ma, R. Jiang, W. Zhou, S. Zhou, Y. Zhang, S. Lei, S. Wang, J. Kuang, X. Han, M. Wei, Y. You, M. Li, Y. Li, D. Liang, J. Liu, T. Chen, C. Yan, R. Wei, C. Rajani, C. Shen, G. Xie, Z. Bian, H. Li, A. Zhao and W. Jia, Theabrownin from Pu-erh tea attenuates hypercholesterolemia via modulation of gut microbiota and bile acid metabolism, Nat. Commun., 2019, 10, 4971–4988 CrossRef PubMed.
- D. Liu, J. Huang, Y. Luo, B. Wen, W. Wu, H. Zeng and L. Zhonghua, Fuzhuan Brick Tea Attenuates High-Fat Diet-Induced Obesity and Associated Metabolic Disorders by Shaping Gut Microbiota, J. Agric. Food Chem., 2019, 67, 13589–13604 CrossRef CAS PubMed.
- J. M. Natividad, A. Agus, J. Planchais, B. Lamas, A. C. Jarry, R. Martin, M. L. Michel, C. Chong-Nguyen, R. Roussel, M. Straube, S. Jegou, C. McQuitty, M. Le Gall, G. da Costa, E. Lecornet, C. Michaudel, M. Modoux, J. Glodt, C. Bridonneau, B. Sovran, L. Dupraz, A. Bado, M. L. Richard, P. Langella, B. Hansel, J. M. Launay, R. J. Xavier, H. Duboc and H. Sokol, Impaired Aryl Hydrocarbon Receptor Ligand Production by the Gut Microbiota
Is a Key Factor in Metabolic Syndrome, Cell Metab., 2018, 28, 737–749 CrossRef CAS PubMed.
- A. Zarrinpar, A. Chaix, Z. Z. Xu, M. W. Chang, C. A. Marotz, A. Saghatelian, R. Knight and S. Panda, Antibiotic-induced microbiome depletion alters metabolic homeostasis by affecting gut signaling and colonic metabolism, Nat. Commun., 2018, 9, 2872–2885 CrossRef PubMed.
- J. Aron-Wisnewsky, M. V. Warmbrunn, M. Nieuwdorp and K. Clement, Metabolism and Metabolic Disorders and the Microbiome: The Intestinal Microbiota Associated With Obesity, Lipid Metabolism, and Metabolic Health-Pathophysiology and Therapeutic Strategies, Gastroenterology, 2021, 160, 573–599 CrossRef CAS PubMed.
- X. Wang, Y. Zheng, Y. Ma, L. Du, F. Chu, H. Gu, R. A. Dahlgren, Y. Li and H. Wang, Lipid metabolism disorder induced by up-regulation of miR-125b and miR-144 following beta-diketone antibiotic exposure to F0-zebrafish (Danio rerio), Ecotoxicol. Environ. Saf., 2018, 164, 243–252 CrossRef CAS PubMed.
- T. Kuno, M. Hirayama-Kurogi, S. Ito and S. Ohtsuki, Reduction in hepatic secondary bile acids caused by short-term antibiotic-induced dysbiosis decreases mouse serum glucose and triglyceride levels, Sci. Rep., 2018, 8, 1253–1268 CrossRef PubMed.
- D. Reijnders, G. H. Goossens, G. D. Hermes, E. P. Neis, C. M. van der Beek, J. Most, J. J. Holst, K. Lenaerts, R. S. Kootte, M. Nieuwdorp, A. K. Groen, S. W. Olde Damink, M. V. Boekschoten, H. Smidt, E. G. Zoetendal, C. H. Dejong and E. E. Blaak, Effects of Gut Microbiota Manipulation by Antibiotics on Host Metabolism in Obese Humans: A Randomized Double-Blind Placebo-Controlled Trial, Cell Metab., 2016, 24, 63–74 CrossRef CAS PubMed.
- B. A. Kappel, L. De Angelis, M. Heiser, M. Ballanti, R. Stoehr, C. Goettsch, M. Mavilio, A. Artati, O. A. Paoluzi, J. Adamski, G. Mingrone, B. Staels, R. Burcelin, G. Monteleone, R. Menghini, N. Marx and M. Federici, Cross-omics analysis revealed gut microbiome-related metabolic pathways underlying atherosclerosis development after antibiotics treatment, Mol. Metab., 2020, 36, 100976–100994 CrossRef CAS PubMed.
- M. D. Pieczynska, Y. Yang, S. Petrykowski, O. K. Horbanczuk, A. G. Atanasov and J. O. Horbanczuk, Gut Microbiota and Its Metabolites in Atherosclerosis Development, Molecules, 2020, 25, 594–569 CrossRef CAS PubMed.
- A. Zarrinpar, A. Chaix, Z. J. Z. Xu, M. W. Chang, C. A. Marotz, A. Saghatelian, R. Knight and S. Panda, Antibiotic-induced microbiome depletion alters metabolic homeostasis by affecting gut signaling and colonic metabolism, Nat. Commun., 2018, 9, 2872–2885 CrossRef PubMed.
- M. Milard, F. Laugerette, A. Durand, C. Buisson, E. Meugnier, E. Loizon, C. Louche-Pelissier, V. Sauvinet, L. Garnier, S. Viel, K. Bertrand, F. Joffre, D. Cheillan, L. Humbert, D. Rainteau, P. Plaisancie, L. B. Bindels, A. M. Neyrinck, N. M. Delzenne and M. C. Michalski, Milk Polar Lipids in a High-Fat Diet Can Prevent Body Weight Gain: Modulated Abundance of Gut Bacteria in Relation with Fecal Loss of Specific Fatty Acids, Mol. Nutr. Food Res., 2019, 63, 1801078–1801091 CrossRef PubMed.
- G. M. Forster, J. Stockman, N. Noyes, A. L. Heuberger, C. D. Broeckling, C. M. Bantle and E. P. Ryan, A Comparative Study of Serum Biochemistry, Metabolome and Microbiome Parameters of Clinically Healthy, Normal Weight, Overweight, and Obese Companion Dogs, Top. Companion. Anim. Med., 2018, 33, 126–135 CrossRef PubMed.
- H. Feng, S. J. Zhang, J. M. F. Wan, L. F. Gui, M. C. Ruan, N. Li, H. Y. Zhang, Z. G. Liu and H. L. Wang, Polysaccharides extracted from Phellinus linteus ameliorate high-fat high-fructose diet induced insulin resistance in mice, Carbohydr. Polym., 2018, 200, 144–153 CrossRef CAS PubMed.
- B. W. Parks, E. Nam, E. Org, E. Kostem, F. Norheim, S. T. Hui, C. Pan, M. Civelek, C. D. Rau, B. J. Bennett, M. Mehrabian, L. K. Ursell, A. Q. He, L. W. Castellani, B. Zinker, M. Kirby, T. A. Drake, C. A. Drevon, R. Knight, P. Gargalovic, T. Kirchgessner, E. Eskin and A. J. Lusis, Genetic Control of Obesity and Gut Microbiota Composition in Response to High-Fat, High-Sucrose Diet in Mice, Cell Metab., 2013, 17, 141–152 CrossRef CAS PubMed.
- E. K. Speliotes, L. M. Yerges-Armstrong, J. Wu, R. Hernaez, L. J. Kim, C. D. Palmer, V. Gudnason, G. Eiriksdottir, M. E. Garcia, L. J. Launer, M. A. Nalls, J. M. Clark, B. D. Mitchell, A. R. Shuldiner, J. L. Butler, M. Tomas, U. Hoffmann, S. J. Hwang, J. M. Massaro, C. J. O'Donnell, D. V. Sahani, V. Salomaa, E. E. Schadt, S. M. Schwartz, D. S. Siscovick, C. R. N. Nash, G. Consortium, M. Investigators, B. F. Voight, J. J. Carr, M. F. Feitosa, T. B. Harris, C. S. Fox, A. V. Smith, W. H. Kao, J. N. Hirschhorn, I. B. Borecki and G. Consortium, Genome-wide association analysis identifies variants associated with nonalcoholic fatty liver disease that have distinct effects on metabolic traits, PLoS Genet., 2011, 7, e1001324–e1001338 CrossRef CAS PubMed.
- H. W. Lee and S. Pyo, Acrylamide induces adipocyte differentiation and obesity in mice, Chem.-Biol. Interact., 2019, 298, 24–34 CrossRef CAS PubMed.
- B. J. Barkla, A. Garibay-Hernandez, M. Melzer, T. W. T. Rupasinghe and U. Roessner, Single cell-type analysis of cellular lipid remodelling in response to salinity in the epidermal bladder cells of the model halophyte Mesembryanthemum crystallinum, Plant, Cell Environ., 2018, 41, 2390–2403 CrossRef CAS PubMed.
- S. Fu, L. Yang, P. Li, O. Hofmann, L. Dicker, W. Hide, X. Lin, S. M. Watkins, A. R. Ivanov and G. S. Hotamisligil, Aberrant lipid metabolism disrupts calcium homeostasis causing liver endoplasmic reticulum stress in obesity, Nature, 2011, 473, 528–531 CrossRef CAS PubMed.
- J. E. Nahon, M. Hoekstra, V. van Harmelen, P. C. N. Rensen, K. W. van Dijk, S. Kooijman and M. Van Eck, Proteoglycan 4 deficiency protects against glucose intolerance and fatty liver disease in diet-induced obese mice, Biochim. Biophys. Acta, Mol. Basis Dis., 2019, 1865, 494–501 CrossRef CAS PubMed.
- A. R. Hanning, X. Wang, Z. Hashemi, S. Wan, A. England, R. L. Jacobs and C. B. Chan, Both low- and regular-fat cheeses mediate improved insulin sensitivity and modulate serum phospholipid profiles in insulin-resistant rats, J. Nutr. Biochem., 2019, 64, 144–151 CrossRef CAS PubMed.
- C. Zeng, B. Wen, G. Hou, L. Lei, Z. Mei, X. Jia, X. Chen, W. Zhu, J. Li, Y. Kuang, W. Zeng, J. Su, S. Liu, C. Peng and X. Chen, Lipidomics profiling reveals the role of glycerophospholipid metabolism in psoriasis, Gigascience, 2017, 6, 1–11 CrossRef CAS PubMed.
- I. Moreno-Indias, L. Sanchez-Alcoholado, P. Perez-Martinez, C. Andres-Lacueva, F. Cardona, F. Tinahones and M. I. Queipo-Ortuno, Red wine polyphenols modulate fecal microbiota and reduce markers of the metabolic syndrome in obese patients, Food Funct., 2016, 7, 1775–1787 RSC.
- P. Samczuk, M. Luba, J. Godzien, A. Mastrangelo, H. R. Hady, J. Dadan, C. Barbas, M. Gorska, A. Kretowski and M. Ciborowski, “Gear mechanism” of bariatric interventions revealed by untargeted metabolomics, J. Pharm. Biomed. Anal., 2018, 151, 219–226 CrossRef CAS PubMed.
- M. Basaranoglu and N. Ormeci, Nonalcoholic fatty liver disease: Diagnosis, pathogenesis, and management, Turk. J. Gastroenterol., 2014, 25, 127–132 CrossRef PubMed.
- P. Li, J. Huang, N. Xiao, X. Cai, Y. Yang, J. Deng, L. H. Zhang and B. Du, Sacha inchi oil alleviates gut microbiota dysbiosis and improves hepatic lipid dysmetabolism in high-fat diet-fed rats, Food Funct., 2020, 11, 5827–5841 RSC.
- B. Gao, A. Emami, S. Nath and B. Schnabl, Microbial Products and Metabolites Contributing to Alcohol-Related Liver Disease, Mol. Nutr. Food Res., 2020, e2000023–e2000029, DOI:10.1002/mnfr.202000023.
- A. Tripathi, J. Debelius, D. A. Brenner, M. Karin, R. Loomba, B. Schnabl and R. Knight, The gut-liver axis and the intersection with the microbiome, Nat. Rev. Gastroenterol. Hepatol., 2018, 15, 397–411 CrossRef CAS PubMed.
- B. J. Moyer, I. Y. Rojas, J. S. Kerley-Hamilton, H. F. Hazlett, K. V. Nemani, H. W. Trask, R. J. West, L. E. Lupien, A. J. Collins, C. S. Ringelberg, B. Gimi, W. B. Kinlaw 3rd and C. R. Tomlinson, Inhibition of the aryl hydrocarbon receptor prevents Western diet-induced obesity. Model for AHR activation by kynurenine via oxidized-LDL, TLR2/4, TGFbeta, and IDO1, Toxicol. Appl. Pharmacol., 2016, 300, 13–24 CrossRef CAS PubMed.
- A. Kumar, K. Sundaram, J. Mu, G. W. Dryden, M. K. Sriwastva, C. Lei, L. Zhang, X. Qiu, F. Xu, J. Yan, X. Zhang, J. W. Park, M. L. Merchant, H. C. L. Bohler, B. Wang, S. Zhang, C. Qin, Z. Xu, X. Han, C. J. McClain, Y. Teng and H. G. Zhang, High-fat diet-induced upregulation of exosomal phosphatidylcholine contributes to insulin resistance, Nat. Commun., 2021, 12, 213–234 CrossRef CAS PubMed.
- M. Y. Zhang, W. L. Sun, M. H. Zhou and Y. Tang, MicroRNA-27a regulates hepatic lipid metabolism and alleviates NAFLD via repressing FAS and SCD1, Sci. Rep., 2017, 7, 14493–14503 CrossRef PubMed.
- H. F. Xu, J. Luo, W. S. Zhao, Y. C. Yang, H. B. Tian, H. B. Shi and M. Bionaz, Overexpression of SREBP1 (sterol regulatory element binding protein 1) promotes de novo fatty acid synthesis and triacylglycerol accumulation in goat mammary epithelial cells, J. Dairy Sci., 2016, 99, 783–795 CrossRef CAS PubMed.
- T. Hendrikx, Y. Duan, Y. H. Wang, J. H. Oh, L. M. Alexander, W. Huang, P. Starkel, S. B. Ho, B. Gao, O. Fiehn, P. Emond, H. Sokol, J. P. van Pijkeren and B. Schnabl, Bacteria engineered to produce IL-22 in intestine induce expression of REG3G to reduce ethanol-induced liver disease in mice, Gut, 2019, 68, 1504–1515 CrossRef CAS PubMed.
- D. Dodd, M. H. Spitzer, W. Van Treuren, B. D. Merrill, A. J. Hryckowian, S. K. Higginbottom, A. Le, T. M. Cowan, G. P. Nolan, M. A. Fischbach and J. L. Sonnenburg, A gut bacterial pathway metabolizes aromatic amino acids into nine circulating metabolites, Nature, 2017, 551, 648–652 CrossRef CAS PubMed.
- S. Wang, M. Q. Huang, X. You, J. Y. Zhao, L. L. Chen, L. Wang, Y. J. Luo and Y. Chen, Gut microbiota mediates the anti-obesity effect of calorie restriction in mice, Sci. Rep., 2018, 8, 13037–13051 CrossRef PubMed.
- W. R. Wikoff, A. T. Anfora, J. Liu, P. G. Schultz, S. A. Lesley, E. C. Peters and G. Siuzdak, Metabolomics analysis reveals large effects of gut microflora on mammalian blood metabolites, Proc. Natl. Acad. Sci. U. S. A., 2009, 106, 3698–3703 CrossRef CAS PubMed.
- A. Morgun, A. Dzutsev, X. X. Dong, R. L. Greer, D. J. Sexton, J. Ravel, M. Schuster, W. Hsiao, P. Matzinger and N. Shulzhenko, Uncovering effects of antibiotics on the host and microbiota using transkingdom gene networks, Gut, 2015, 64, 1732–1743 CrossRef CAS PubMed.
- T. Zelante, R. G. Iannitti, C. Cunha, A. De Luca, G. Giovannini, G. Pieraccini, R. Zecchi, C. D'Angelo, C. Massi-Benedetti, F. Fallarino, A. Carvalho, P. Puccetti and L. Romani, Tryptophan catabolites from microbiota engage aryl hydrocarbon receptor and balance mucosal reactivity via interleukin-22, Immunity, 2013, 39, 372–385 CrossRef CAS PubMed.
- H. M. Roager and T. R. Licht, Microbial tryptophan catabolites in health and disease, Nat. Commun., 2018, 9, 3294–3304 CrossRef PubMed.
- K. E. Mercer, L. Yeruva, L. Pack, J. L. Graham, K. L. Stanhope, S. V. Chintapalli and B. D. Piccolo, Xenometabolite signatures in the UC Davis type 2 diabetes mellitus rat model revealed using a metabolomics platform enriched with microbe-derived metabolites, Am. J. Physiol., 2020, 319, 1–43 CrossRef PubMed.
- M. Wlodarska, C. Luo, R. Kolde, E. d'Hennezel, J. W. Annand, C. E. Heim, P. Krastel, E. K. Schmitt, A. S. Omar, E. A. Creasey, A. L. Garner, S. Mohammadi, D. J. O'Connell, S. Abubucker, T. D. Arthur, E. A. Franzosa, C. Huttenhower, L. O. Murphy, H. J. Haiser, H. Vlamakis, J. A. Porter and R. J. Xavier, Indoleacrylic Acid Produced by Commensal Peptostreptococcus Species Suppresses Inflammation, Cell Host Microbe, 2017, 22, 25–37 CrossRef CAS PubMed.
- J. J. Galligan, Beneficial actions of microbiota-derived tryptophan metabolites, Neurogastroenterol. Motil., 2018, 30, e13283–e13287 CrossRef PubMed.
- B. Lamas, M. L. Richard, V. Leducq, H. P. Pham, M. L. Michel, G. Da Costa, C. Bridonneau, S. Jegou, T. W. Hoffmann, J. M. Natividad, L. Brot, S. Taleb, A. Couturier-Maillard, I. Nion-Larmurier, F. Merabtene, P. Seksik, A. Bourrier, J. Cosnes, B. Ryffel, L. Beaugerie, J. M. Launay, P. Langella, R. J. Xavier and H. Sokol, CARD9 impacts colitis by altering gut microbiota metabolism of tryptophan into aryl hydrocarbon receptor ligands, Nat. Med., 2016, 22, 598–605 CrossRef CAS PubMed.
- L. Zhang, R. G. Nichols, J. Correll, I. A. Murray, N. Tanaka, P. B. Smith, T. D. Hubbard, A. Sebastian, I. Albert, E. Hatzakis, F. J. Gonzalez, G. H. Perdew and A. D. Patterson, Persistent Organic Pollutants
Modify Gut Microbiota-Host Metabolic Homeostasis in Mice Through Aryl Hydrocarbon Receptor Activation, Environ. Health Perspect., 2015, 123, 679–688 CrossRef CAS PubMed.
Footnote |
† Electronic supplementary information (ESI) available: Fig. S1 exhibits principal component analysis (PCA) score plots of serum metabolome in healthy mice and microbiota-depleted mice (A, B). Tables show the model parameters of orthogonal partial least squares-discriminant analysis (OPLS-DA) in different comparative groups (Table S1), the relative abundance of OTUs showing differences among all groups of healthy mice (Table S2), the values of differential metabolites showing differences among all groups of healthy mice (Table S3), the values of differential metabolites among all groups of microbiota-depleted mice, and the differences in several metabolites in the microbiota-depleted mice compared to their respective healthy counterparts (Table S4), metabolites values involved in glycerophospholipid metabolism, showing differences between the HFD treated group alone, as well as FBT-treated healthy mice and microbiota-depleted mice (Table S5). See DOI: 10.1039/d1fo02181h |
|
This journal is © The Royal Society of Chemistry 2022 |
Click here to see how this site uses Cookies. View our privacy policy here.