Surveillance and seasonal correlation of rotavirus A with coliphages and coliforms in two sewage impacted lakes in highly urbanized regions of western India†
Received
23rd August 2021
, Accepted 10th November 2021
First published on 16th November 2021
Abstract
Group A rotavirus (RVA), an enteric virus, is a major cause of gastroenteric disease and it is known to cause mortality among children and infants. The applicability of coliforms and coliphages as indicators for RVA was evaluated in this study. The abundance of RVA, ‘SUSP2’, a novel ‘superspreader’ somatic coliphage, MS2, a male-specific coliphage, and coliforms were monitored in two lakes located in the neighboring cities of Mumbai and Thane, India. RVA was detected in 71% and 60% of the composite samples collected from Powai lake in Mumbai and Masunda lake in Thane, respectively. MS2 was detected in 81% and 79%, while SUSP2 was detected in 75% and 81% of the composite samples from Powai lake and Masunda lake, respectively. The maximum RVA, MS2, and SUSP2 concentrations in the composite samples from Powai lake were 5.47 × 105, 6.75 × 102, and 3.47 × 103 copies per 100 mL, respectively, while, the corresponding values for Masunda lake were 2.13 × 104, 2.61 × 104, and 2.21 × 104 copies per 100 mL, respectively. The viral counts were found to be lowest during the monsoon and highest during winter. For samples collected from these lakes, the seasonal correlation between RVA and the indicators was analyzed using Spearman's rank correlation. The coliphages showed a moderate to strong positive correlation with RVA particularly in winter and demonstrated a Spearman correlation coefficient (ρ) in the range of 0.59–0.87 (p < 0.05). However, in the summer and monsoon, a significant correlation between the coliphage, MS2, and RVA was observed only in Powai lake. Thus, coliphages are good indicators of RVA, especially in the winter months, when the occurrence of RVA in the lakes is highest. In the summer and monsoon, both coliphages and coliforms need to be monitored to predict the presence of RVA with a higher degree of confidence. Principal component analysis (PCA) and binary logistic regression (BLR) also confirmed MS2 as a better indicator of RVA. Pearson's correlation coefficient (r) ranged between 0.46–0.74 (p < 0.05). This is the first study reporting the viral load in surface water bodies in India across seasons and the correlation between RVA and coliphages.
Water impact
The MS2 coliphage was found to be a better indicator of rotavirus A (RVA) compared to coliforms. A strong correlation between coliphages and RVA was observed in winter when the RVA concentration was the highest. This study suggests that the surveillance of coliphages in surface water can better indicate the enteric virus concentration in surface water.
|
Introduction
Enteric viruses are water-borne pathogens that are known to persistently occur in surface water bodies due to increased anthropogenic activities.1,2 These viruses enter the water bodies either through point or non-point sources, agricultural runoffs, or leaking sewers.3,4 Among the 140 enteric viruses identified, rotaviruses and adenoviruses are the most commonly occurring enteric viruses found in water bodies.5,6 Moreover, these viruses are known to be pathogenic even when present at very low copy numbers (1 copy per mL).7
Several studies have suggested coliphages to be suitable indicators for fecal (enteric) viruses in water.5,8–10 Coliphages are typically categorized into two groups based on the receptor sites on the host as somatic coliphages and F-specific coliphages. Somatic coliphages are lytic viruses that bind to the cell wall of the bacterial host, while the F-specific coliphages bind to the sex pili of the host.11 Some researchers have reported that somatic coliphages are capable of multiplying in natural water bodies.12,13 Thus, they were found to outnumber the F-specific RNA coliphages in waterbodies by a factor of 5 and cytopathogenic human viruses by a factor of 500.14 However, some studies have suggested that somatic coliphage multiplication in the environment is highly improbable and that the influence of replication outside the gut can be considered insignificant compared to that contributed by feces.15,16 Bacterial viruses may serve as a potential indicator for enteric viruses due to their structural resemblance, resistance to environmental stress conditions, and also their comparable concentration in water bodies.17–20 However, some studies have suggested that the occurrence and abundance of coliphages do not correlate well with those of enteric viruses, such as, rotaviruses and adenoviruses.21,22 Water quality surveillance for virus contamination may be carried out using two approved methods, i.e., by monitoring specific enteric viruses or by monitoring bacteriophages as surrogates for viral water quality management. Undoubtedly, molecular-based methods have made the direct detection of enteric viruses more practical.23 However, monitoring each type of enteric virus is time-consuming and expensive, requires technical expertise, and demands the collection of a large volume of water samples for analysis. On the other hand, the detection of infectious bacteriophages can be conducted easily through a simpler and more cost-effective approach compared to that of enteric viruses. However, to date, no consensus has been reached regarding the use of coliphages as alternative indicators of enteric viruses during microbial water quality monitoring.5
Currently, limited studies have been reported in India for viral surveillance and correlation in recreational/drinking water sources. Recently, a study conducted by Zehra et al. (2020)24 suggested monitoring physicochemical water quality parameters together with fecal indicator bacteria and adenoviral markers for monitoring the water quality of surface water bodies. Only one study based in India has reported the seasonal variation of two viruses, i.e., hepatitis E virus (HEV) and RVA, in sewage samples collected from Vellore, India.25 RNA isolated from the fecal/plasma samples of patients suffering from sporadic hepatitis in a hospital was analyzed and sequenced and was matched with that obtained from sewage samples. The HEV strains isolated from the sporadic hepatitis cases showed 94–100% similarity with the HEV strains isolated from sewage.25 Previously, four virus types, i.e., pepper mild mottle virus (PMMoV), hepatitis A, Aichi virus, and noroviruses (NoVs), were monitored in the Brahmaputra River; however, the authors primarily focused on the increased antibiotic resistance among the bacterial community.26 None of the studies conducted in India have quantified the virus concentration in surface water bodies, for identifying potential indicators for rotavirus, although rotavirus is a major concern for India due to poor sanitation practices.27 Thus, to address the gaps, the present study was aimed to detect the presence of rotavirus A and coliphages in lakes in highly urbanized regions in and around Mumbai (India). Their abundance in the water bodies was monitored to obtain a correlation between the pathogens and their indicators. Sampling of the lakes was conducted monthly from January 2018 to January 2020. In composite samples collected from the lakes, RVA was monitored along with coliphages, MS2 (F specific coliphage), and a novel ‘superspreader’ somatic coliphage, SUSP2,28 coliforms (total and fecal), and chemical oxygen demand (COD). Identification of key microbial water quality parameters associated with RVA levels was performed by employing Pearson's correlation. The seasonal correlation between the indicators and RVA was determined using Spearman's rank correlation. Further, to identify the potential predictor for RVA, binary logistic regression (BLR) analysis was conducted individually with each predictor variable. Additionally, physicochemical analysis of water samples collected from multiple locations in each lake was also conducted to determine the spatial variability in pollutant load.
Materials and methods
2.1 Site description, sample collection, and water quality analysis
In total, 283 water samples were collected from two different lakes located in highly urbanized parts of Mumbai (19.12° N, 72.91° E) and Thane (19.19° N, 72.98° E). Powai lake (210 ha) located in Mumbai is a relatively larger lake compared to Masunda lake (7.2 ha) located in Thane (Fig. 1). The lakes are surrounded by commercial and residential areas and are thus impacted by anthropogenic activities. Apart from serving as a catchment to control floods, these lakes are used for recreational activities, such as angling and boating. Powai lake additionally is used for irrigation purposes.29 The sampling locations were selected close to the entry point of urban runoffs into the lakes. During any sampling event, 600 mL of a sample from each location was collected at a depth of 1 m from the surface for Powai lake (8 locations) and Masunda lake (5 locations). Several hotels, slums, residential areas, and an academic campus were located in the vicinity of Powai lake. Analysis of RVA and indicator organisms was only conducted using a spatially composite sample prepared by combining samples from all locations in equal proportion. Monthly sampling was conducted over a duration of two years, from January 2018 to January 2020. For each location, COD, turbidity, TSS, and total coliforms (TC) were analyzed as per standard methods.30 For each lake, the composite samples (n = 48) were analyzed for the presence of RVA and indicator organisms (SUSP2, MS2, total coliforms (TC), and fecal coliforms (FC)). COD was also estimated for the composite samples. The framework for sampling is shown in Fig. 2.
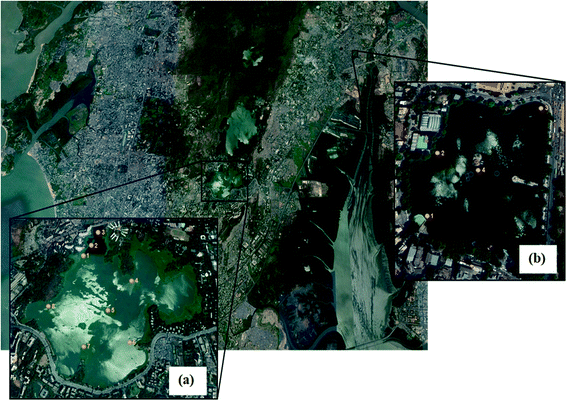 |
| Fig. 1 Sampling locations within the lakes: (a) Powai Lake and (b) Masunda Lake. | |
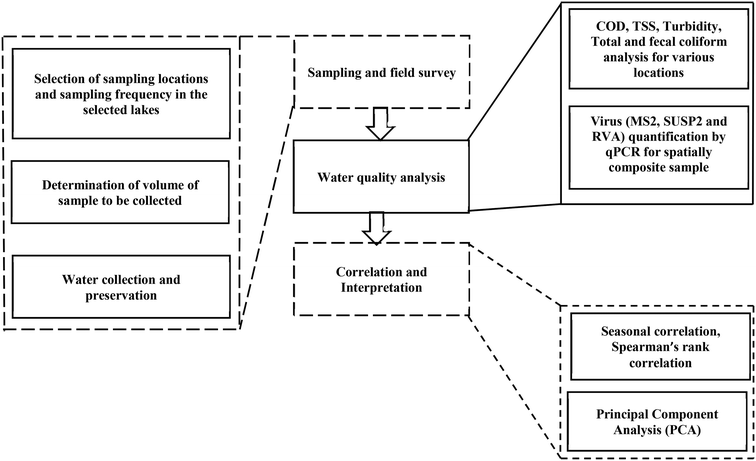 |
| Fig. 2 Framework of the study for correlating RVA with other pollution indicators in lakes. | |
2.2 Quantification of total coliforms and fecal coliforms
Detection and quantification of coliforms in the water samples were done using the membrane filtration method. The media used for enumeration of TC and FC were m-endo agar and MFC agar, respectively, and standard protocols were followed.30 Briefly, water samples (50–100 mL) were sequentially filtered through a glass fiber filter with a cutoff size of 2.7 μm (Whatman 1823-024, GE Healthcare and Lifesciences, UK) and a nylon membrane filter with a cutoff size of 0.45 μm (Pall Lifesciences, USA). The m-endo agar plates and MFC agar plates were incubated overnight at 37 °C and 45 °C, respectively, for the estimation of total coliforms and fecal coliforms. On m-endo agar, the total coliforms appeared as colonies with a green metallic sheen. Fecal coliforms formed light to dark blue colonies on MFC agar plates. The concentration of total coliforms and fecal coliforms was expressed as CFU per 100 mL.
2.3 Construction of standards for coliphages
The phage isolated from Powai lake (SUSP2) was identified as a novel somatic ‘superspreader’ coliphage containing double-stranded (ds) DNA.28 The protocol for isolation of the phage from the lake has been discussed in the ESI.† The phage DNA was isolated by the phenol–chloroform method as specified by Sambrook and Russell (2001).31 The concentration of isolated phage DNA was quantified by measuring the absorbance at 260 nm (8453 UV-visible, Agilent, USA). Thereafter, it was serially diluted (106 to 102 copies per mL) to construct a standard plot with primers specific to the tail region of SUSP2 (Table S2, ESI†). Construction of a standard plot for the MS2 phage was done by employing pure RNA (0.8 μg mL−1) of MS2 procured from Roche, (USA). The RNA was reverse transcribed to cDNA using a Hyperscript reverse transcription kit (GeneALL, Korea). Reverse transcription was performed as per the instructions in the kit. Briefly, 1 μL of the pure RNA was incubated with random primers and deoxynucleotide triphosphates (dNTPs) at 65 °C to remove the secondary structure in RNA. Subsequently, the samples were incubated with the reverse transcriptase enzyme at 55 °C for an hour. The first step of strand separation is necessary for annealing the random hexamers onto the RNA for successful reverse transcription. Finally, the reaction was terminated at 95 °C and the cDNA was stored at −20 °C until use. The standard curve was prepared using dilutions of MS2 cDNA (106 to 102 copy number per mL) with primers specific to the assembly protein region of MS2 as suggested by O'Connell et al. (2006)32 (Table S2 in the ESI†). The qPCR standards were run using a Brilliant III Ultra-Fast SYBR Green qPCR master mix (Agilent, USA). The qPCR mixtures for construction of standards contained 2 μL template DNA/cDNA or standard, 10 μL of 2× SYBR Green qPCR master mix, 1 μL (500 nM) of the forward primer, 1 μL (500 nM) of the reverse primer, and 6 μL of nuclease-free water for a 20 μL qPCR mixture. The thermal cycling protocol for qPCR was as follows: initial incubation at 95 °C for 3 min, and 40 cycles of 95 °C for 5 s and 60 °C for 10 s. All the qPCR reactions were performed on a CFX 96 real-time PCR detection system, Biorad, USA. The detection limit for the qPCR assay was 102 gene copies per mL. Melt curve analysis was performed with one cycle at 65 °C for 5 s, and the readings were recorded from 65 to 95 °C with an increment of 0.5 °C. The standards for SUSP2 and MS2 are provided in the ESI† (Table S3).
2.4 Construction of an external standard for rotavirus A (RVA)
The following procedure was used to construct an external standard for RVA. Virus concentration from domestic wastewater (500 mL) was performed using a protocol adapted from Clokie and Kropinski (2009)33 with minor modifications. Briefly, 500 mL of domestic wastewater was centrifuged at 6000g for 10 min, followed by sequential filtration to remove larger particles as well as bacteria and fungi through filters with cut-off sizes of 2.7 μm (Whatman 1823-024, GE Healthcare and Lifesciences, UK), 0.45 μm (Pall Lifesciences, USA), and 0.22 μm (Pall Lifesciences, USA). Thereafter, the filtrate was concentrated using an ultracentrifuge (Optima XPN-100, Beckman Coulter, USA) operated at 25
000 rpm for 2 h at 4 °C. The supernatant was decanted, and the pellet was suspended in 1 mL SM buffer (Table S1, ESI†). The nucleic acid of the concentrated sample was extracted using a GeneALL kit (GeneALL, Korea). The nucleic acid of these viruses was then reverse-transcribed using the Hyperscript GeneALL kit (GeneALL, Korea). Since RVA is a dsRNA virus, the initial RNA strand separation was performed at 95 °C for 5 min. The product, i.e., cDNA, obtained upon reverse transcription was further subjected to amplification using a primer targeting the NSP5 region of rotavirus (Table S2, ESI†). Amplification was done thrice (under the same conditions as those discussed in section 2.3) to obtain a sufficient concentration of the amplified product for subsequent ligation of the product into a vector. Thereafter, the qPCR product was run on an agarose gel (0.7%) in Tris-acetate-EDTA (TAE) buffer for 45–60 min. Subsequently, the product was purified using a HiPurA™ quick gel purification kit (Himedia, USA). The purified qPCR amplicon was cloned into the pMiniT vector using a NEB (New England Biolabs, USA) PCR cloning kit according to the manufacturer's protocol with some modifications. Briefly, the ligation mixture (10 μL) containing the PCR amplicon (insert) was incubated overnight at 18 °C followed by 5 h incubation at 22 °C. The ligation mixture typically contained the insert (PCR amplicon), pMiniT vector, T4 DNA ligase, ATP, end polishing components, and ligation enhancers. The end polishing components were employed to remove the single nucleotide overhangs generated during the PCR reaction so as to convert the insert to a blunt end, while DMSO or polyethylene glycol (PEG) was used as a ligation enhancer. Subsequently, the transformation of the ligation mixture into 100 μL of freshly prepared competent cells of E. coli DH5α was performed. The mixture was incubated on ice for 30 min. This was followed by heat shock at 42 °C for 90 s and subsequent incubation on ice for 5 min. Luria broth (LB, 900 μL) was added to the mixture and it was incubated in a shaker set at 37 °C for 1 h at 250 rpm. The suspension was subsequently centrifuged at 4000 rpm at 25 °C for 10 min. The supernatant (800 μL) was aspirated without disturbing the pellet. The pelleted cell suspension was plated on a LB agar plate containing 100 μg mL−1 ampicillin. The plates were incubated overnight at 37 °C to select ampicillin-resistant colonies. The white colonies were isolated and grown overnight in ampicillin LB broth, and the plasmid DNA was isolated using a Genejet Plasmid Miniprep kit (Thermo Scientific, USA). The concentration of the plasmid DNA was thereafter quantified by measuring the absorbance at 260 nm, and the copy number of the plasmid was determined as specified by Casali and Preston (2003).34 The presence of the insert was confirmed by qPCR with primers targeting the NSP5 region. The plasmid DNA was serially diluted to construct the external standard for RVA quantification (105–102 copy number per mL) using qPCR. The standards were analyzed in duplicate, along with non-template controls during each qPCR run. The cycle threshold (Ct) versus log(copy number) of the target virus was plotted and was thereafter used to quantify RVA in water samples. The qPCR standard equation and associated parameters, such as efficiency and R2, are shown in the ESI† (Table S3).
2.5 Detection of rotavirus and coliphages in environmental water samples
Water samples collected from both the lakes were transported to the laboratory in an icebox and thereafter stored at 4 °C. The water samples from each location were sequentially filtered through membranes with cut-off sizes of 2.7 μm (Whatman 1823-024, GE Healthcare and Lifesciences, UK), 0.45 μm (Pall Lifesciences, USA), and 0.22 μm (Pall Lifesciences, USA). For each lake, a composite sample was prepared by mixing an equal volume of samples from each of the locations. Thereafter, 4.8 L of composite sample for Powai lake and 3 L of composite sample for Masunda lake were ultracentrifuged (SW 32 rotor, Optima XPN-100, Beckman Coulter, USA) at 25
000 rpm at 4 °C for 2 h. The supernatant was discarded, and the pellet was then solubilized in SM buffer to obtain a final volume of 1 mL to achieve a 4800 and 3000-fold concentration for the Powai lake and Masunda lake samples, respectively. The phages and the human virus, RVA, were concentrated simultaneously employing ultracentrifugation and thereafter quantified using qPCR to overcome problems of the lack of correlation due to differences in the sampling and monitoring protocols. The genomic material of the virus concentrated from the lake water was extracted using a viral RNA/DNA extraction kit as per the manufacturer's instructions (GeneAll, Korea). Subsequently, reverse transcription was performed (since both MS2 and RVA contain RNA as their genomic material) employing a Hyperscript reverse transcription kit (GeneAll, Korea). The extracted DNA/cDNA was then subjected to qPCR with primers specific to each of the virus (Table S2 in the ESI†), and the copy numbers of the viruses SUSP2, MS2, and RVA in the composite lake samples were quantified using the corresponding standard curves (Table S3, ESI†). A non-template control and positive control (reverse transcribed MS2 RNA procured from Roche, USA) were included in each reaction. For both samples and standards, the qPCR conditions were maintained as suggested by the manufacturer (Brilliant III Ultra-Fast SYBR Green qPCR master mix, Agilent, USA).
2.6 Statistical analysis
Statistical tests and procedures were performed using Origin 2018 (OriginLab Corporation, Northampton, MA, USA) and Microsoft Excel (MS Office, Microsoft 2016). Two-way analysis of variance (ANOVA) with a cut-off p value of 0.05 was applied to compare the mean values of pollution indicators (total COD, total coliforms, TSS, and turbidity) over the locations and seasons. Furthermore, specific analyses of individual variables and the correlation between them were carried out by employing Pearson's correlation (r). The seasonal relationships between the various parameters measured for the composite samples, such as COD, total coliforms, fecal coliforms, SUSP2, and MS2, and the pathogen, RVA, were compared by determining the Spearman rank correlation coefficients (ρ). Spearman's rank method was chosen for analyzing the seasonal correlation as the method is capable of capturing even monotonic relationships along with linear relationships. A strong correlation for both Pearson and Spearman rank correlations is indicated by a correlation coefficient greater than 0.7, whereas a correlation coefficient in the range of 0.4 to 0.69 and less than 0.39 are indicative of moderate and weak correlations, respectively.35 A binary logistic regression (BLR) model was used to determine whether the concentration of each of the indicator organisms (MS2, SUSP2, total coliforms, and fecal coliforms) predicted the probability of occurrence of RVA in the lakes. For BLR analysis, the dependent variable, i.e., RVA, was treated as a binary variable, i.e., a score of 0 was assigned when RVA was not detected, and a score of 1 was assigned when RVA was detected. The independent variables, i.e., MS2, SUSP2, total coliforms, and fecal coliforms, were continuous variables, and for samples in which organisms were not detected, a value of 0.05 copies per 100 mL was assigned before data transformation.36 Principal component analysis (PCA) was also performed to determine the association between RVA and the indicators. For RVA, coliphages, and coliforms, statistical analysis was performed after the transformation of the data to ln
N, where N is the number concentration of bacteria or copy number of viruses per 100 mL.
Results
3.1 Water quality assessment of the lakes
A total of 283 water samples were collected from Powai lake (168) and Masunda lake (115). In Powai lake, COD, total coliforms (TC), TSS, and turbidity were found to range from 16–377 mg L−1, 0–4 × 104 CFU per 100 mL, 1–302.5 mg L−1, and 7.5–489 NTU, respectively. Two-way ANOVA suggested that the mean COD, TC, TSS, and turbidity levels across different seasons were significantly different for both the lakes (0.0001 < p < 0.05), as also evident from the box–whisker plots (Fig. 3 and 4). Previous studies have reported consistently high pollution levels in Powai lake.29 These values indicate possible sewage contamination of the lakes, with Powai lake being possibly affected by leaking sewers or direct discharge of sewage from nearby slums and establishments. Particularly, a higher pollutant load was observed at locations 2, 3, 6, and 7 (Fig. 1). In contrast, the relatively smaller Masunda lake exhibited a more homogeneous pollutant load (Fig. 2). The COD levels in Masunda lake ranged from 3–134 mg L−1, where the lowest level was reported in July which is also the month of highest precipitation in Thane, India. The total coliforms, TSS, and turbidity levels ranged from 0–3500 CFU per 100 mL, 2–148 mg L−1, and 5 NTU–79 NTU, respectively. The high values of the water quality parameters (Fig. 4) compared to the prescribed standards37 revealed sewage infiltration into the lakes. Based on analysis of physicochemical parameters, Singare et al. (2010)38 also reported a high pollution load in Masunda lake.
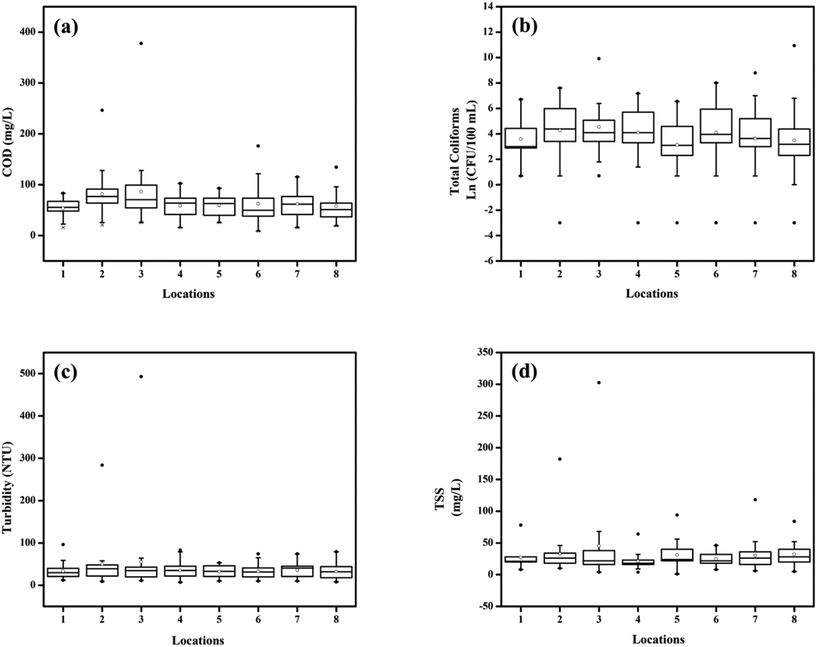 |
| Fig. 3 Box and whisker plots depicting the variation in water quality parameters at various locations in Powai lake: (a) COD (mg L−1), (b) total coliforms (CFU per 100 mL), (c) turbidity (NTU), and (d) TSS (mg L−1). The number of points at each location: (a) n = 42, (b) n = 41, (c) n = 42, and (d) n = 21; •: outliers; ∘: mean. | |
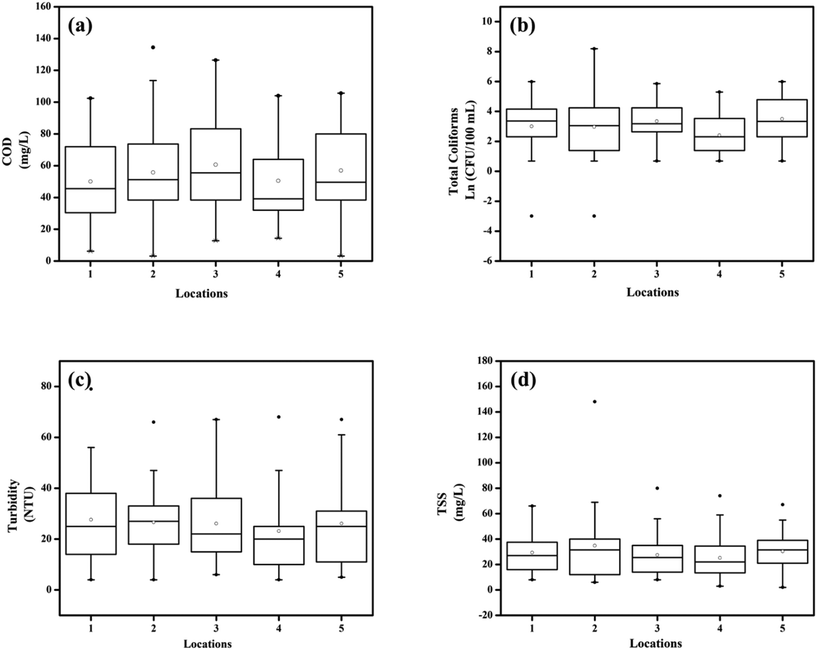 |
| Fig. 4 Box and whisker plots depicting the variation in water quality parameters at various locations in Masunda lake: (a) COD (mg L−1), (b) total coliforms (CFU per 100 mL), (c) turbidity (NTU), and (d) TSS (mg L−1). The number of points at each location: (a) n = 46, (b) n = 42, (c) n = 46, and (d) n = 24; •: outlier; ∘: mean. | |
3.2 Quantification of RVA and fecal indicators
The overall frequency of detection, the concentration range, and the average number of RVA and indicator organisms (SUSP2, MS2, total coliforms, and fecal coliforms) in composite samples from the two lakes are shown in Table 1 and Fig. 5. The prevalence of rotavirus A was found to be highest during the winter months. The RVA concentration was particularly high in February and March, with these months corresponding to the times when Mumbai and Thane record relatively lower temperatures. The concentration of viruses in the lakes across the months is shown in Table S3, ESI.† The RVA genome was detected in 34 and 29 of the 48 composite water samples collected from Powai lake and Masunda lake, respectively. Thus, the detection frequency of RVA was 71% and 60% for the two lakes. MS2 and SUSP2 were positively detected in 81% (39 out of 48 samples) and 75% (36 out of 48), of the composite water samples from Powai lake, respectively. For Masunda lake, MS2 and SUSP2 were detected in 79% (38 out of 48 samples) and 81% (39 out of 48 samples) of the composite water samples, respectively. Comparison of RVA and fecal indicators (MS2, SUSP2, total coliforms, and fecal coliforms) over the months showed that the concentrations of these viruses and coliforms were significantly different over time in both Powai lake (p = 2.68 × 10−5) and Masunda lake (p = 0.0006). In general, the virus concentration was found to be lowest in the monsoon and highest in winter. The possible reason for the low concentration of viruses and coliforms in the lake during the monsoon may be attributed to the dilution effect caused by heavy precipitation. It was observed that similar to the viruses, the water quality parameters, such as TSS, turbidity, and COD, were relatively high during the winter months, and they were lowest during the monsoon. The highest COD values were 118 mg L−1 in Powai lake and 102 mg L−1 in Masunda lake and these values were recorded in March 2018 and February 2018, respectively.
Table 1 Summary statistics for RVA and fecal indicators in Powai lake and Masunda lake
Microorganism |
No. of samples |
Range (copies/100 mL) |
% positive |
Mean ± SE |
Powai Lake |
RVA |
48 |
0 to 5.46 × 105 |
71 |
2.4 × 104 ± 1.46 × 104 |
SUSP2 |
48 |
0 to 3.47 × 103 |
75 |
542 ± 153 |
MS2 |
48 |
0 to 675 |
81 |
76 ± 22 |
Fecal coliform |
36 |
0 to 400 |
97 |
80 ± 15 |
Total coliform |
48 |
1 to 1111 |
100 |
388 ± 115 |
Masunda Lake |
RVA |
48 |
0 to 2.12 × 104 |
60 |
2009 ± 638 |
SUSP2 |
48 |
0 to 2.21 × 104 |
81 |
2074 ± 677 |
MS2 |
48 |
0 to 2.61 × 104 |
79 |
1234 ± 545 |
Fecal coliform |
36 |
0 to 2 × 103 |
98 |
198 ± 74 |
Total coliform |
48 |
0 to 2 × 103 |
88 |
274 ± 64 |
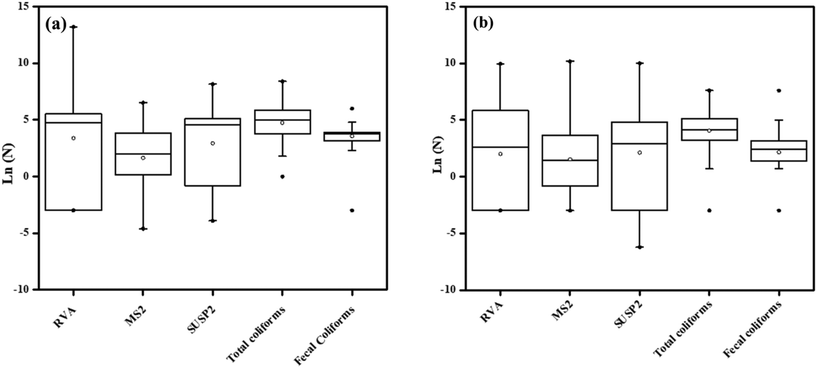 |
| Fig. 5 Box and whisker plots depicting the variation in virus concentration across two years in (a) Powai Lake and (b) Masunda Lake. Number of points, n = 48, N: copies per 100 mL, •: outlier, ∘: mean. | |
The ability of any pathogen to maintain its infectivity outside a host is dependent on various parameters, which include temperature, moisture, dehydration, and UV light. Temperature is the most important parameter impacting the survival of any pathogenic virus in water.39 The ambient temperature in both the cities was approximately 19–27 °C for the winter months as opposed to 26–32 °C in the monsoon and 30–35 °C in summer. A greater abundance of the viruses in the lakes in the winter months may be attributed to increased virus shedding by infected people, as an increasing trend of rotaviral diarrhoea cases was seen particularly during the winter months.40,41
3.3 Correlation of viruses and indicators across the years
Pearson's correlation matrix (Table 2) showed a strong correlation between MS2 and RVA (r = 0.74, p < 10−4) and a moderate correlation between SUSP2 and RVA for Powai lake (r = 0.46, p = 0.001). A similar trend was observed for Masunda lake, where MS2 showed a moderate correlation with RVA (r = 0.46, p = 0.005), while SUSP2 showed a poor correlation with RVA (r = 0.40, p = 0.004). A moderate correlation was obtained between TC and FC in both the lakes (r = 0.52–0.59, p < 0.05). Additionally, PCA (ESI†) of the variables, i.e., viruses (RVA, MS2, and SUSP2) and coliforms (total coliforms and fecal coliforms), over two years showed similar results. Smaller angles between the vector lines (Fig. S1, ESI†) corresponding to the coliphage, MS2, and RVA revealed that the presence of MS2 is closely related to that of RVA. Similarly, smaller angles between vector lines of TC and FC symbolize their close association with each other.
Table 2 Pearson's correlation coefficients for RVA and fecal indicators for Powai lake and Masunda lake
|
RVA |
MS2 |
SUSP2 |
TC |
FC |
COD |
Significant correlation values (p < 0.05) are represented in bold.
|
Powai Lake (n = 48) |
|
RVA |
1 |
|
|
|
|
|
MS2 |
0.74
|
1 |
|
|
|
|
SUSP2 |
0.46
|
0.52
|
1 |
|
|
|
TC |
0.11 |
0.27 |
−0.31 |
1 |
|
|
FC |
0.04 |
0.12 |
−0.17 |
0.52
|
1 |
|
COD |
0.19 |
−0.02 |
0.21 |
0.22 |
0.13 |
1 |
Masunda Lake (n = 48) |
|
RVA |
1 |
|
|
|
|
|
MS2 |
0.46
|
1 |
|
|
|
|
SUSP2 |
0.40
|
0.21 |
1 |
|
|
|
TC |
0.09 |
0.38 |
−0.08 |
1 |
|
|
FC |
0.02 |
0.23 |
−0.28 |
0.59
|
1 |
|
COD |
0.30 |
0.18 |
0.51 |
−0.08 |
0.26 |
1 |
3.4 Seasonal correlations between RVA and fecal indicators
Mumbai and Thane experience summer, winter, and monsoon in April–June, November–March, and July–September, respectively. In October, the temperature in the city increases again; hence, the virus concentration for October was grouped with that for the summer months. As the data were segregated into months corresponding to seasons, the data points were reduced. Thus, the data collected were not normally distributed. Hence, a non-parametric method, i.e., Spearman's rank method, was chosen to predict the seasonal correlation between the concentrations of the target virus (RVA), coliphages, and coliforms.
The Spearman correlation coefficients (ρ) between enteric virus, coliphages, and coliforms in environmental water samples (Powai lake and Masunda lake) are shown in Table 2. In winter, it was observed that coliphages MS2 and SUSP2 showed a moderate to strong correlation with RVA (ρ = 0.58–0.86, p < 0.0006) in both the lakes. It was also evident that for winter, SUSP2 showed a stronger correlation with RVA compared to MS2, and MS2 and SUSP2 correlation was observed only in Powai lake (ρ = 0.67, p = 0.001). No correlation between the coliforms and RVA was observed for winter for both lakes. However, total coliforms showed a strong correlation with MS2 (ρ = 075, p = 0.0001) and moderate correlation with fecal coliforms (ρ = 0.51, p = 0.02) in Masunda lake.
For summer, it was observed that in Powai lake, MS2 (ρ = 0.59) and TC (ρ = 0.47) showed a moderate correlation with RVA. Additionally, a strong correlation between fecal coliforms and total coliforms (ρ = 0.83, p = 0.00007) was observed in Powai lake. However, no association between RVA and the other variables (coliphages and coliforms) was observed in Masunda lake. Only SUSP2 showed a moderate correlation (ρ = −0.63, p = 0.009) with fecal coliforms in Masunda lake in summer. Typically, the virus concentration was lowest in the monsoon when MS2 (ρ = 0.56, p = 0.05) and total coliforms (ρ = −0.62, p = 0.03) showed a moderate correlation with RVA in Powai lake and Masunda lake, respectively. SUSP2 and FC showed no association with RVA in the monsoon in both the lakes. Additionally, MS2 in Masunda lake and TC in Powai lake showed no correlation with RVA during the monsoon. Also, a strong correlation between the coliphages, SUSP2 and MS2 (ρ = 0.80, p = 0.001) and a moderate correlation between coliforms, TC and FC (ρ = 0.66, p = 0.02) were observed in Masunda lake.
Thus, it can be inferred that coliphages are good indicators of RVA, especially in the winter months, when the occurrence of RVA is highest. In the summer and monsoon, both coliphages and coliforms need to be monitored to predict the presence of RVA with confidence. It was observed that similar to those of RVA, the SUSP2 and MS2 abundance and concentrations were highest in the winter months and lowest during the monsoon. In addition to temperature, turbidity also played an important role, as also discussed by Da Silva (2013).42 Lower turbidity and increased water flow have been reported to reduce the viral concentration. In the current study, the turbidity was highest during winter (489 NTU) while it was lowest during the monsoon (4.5 NTU). Excessive rainfall during the monsoon season caused the lake to overflow as the water level reached the maximum value. The poor correlation between RVA and coliphages in the monsoon may have resulted from lower quantification accuracy at lower concentration and reduced viral shedding of RVA during the monsoon. In India, it has been observed that RVA infections are highest during the winter months.40,41Table 3 provides a seasonal correlation between RVA and fecal indicators (MS2, SUSP2, and coliforms).
Table 3 Spearman's rank correlation coefficient for RVA and fecal indicators over the seasons
|
RVA |
MS2 |
SUSP2 |
TC |
FC |
Powai Lake (n = 20) winter |
RVA |
1 |
|
|
|
|
MS2 |
0.69
|
1 |
|
|
|
SUSP2 |
0.86
|
0.67
|
1 |
|
|
TC |
−0.19 |
0.36 |
−0.25 |
1 |
|
FC |
−0.17 |
−0.17 |
−0.22 |
0.07 |
1 |
Masunda Lake (n = 20) winter |
RVA |
1 |
|
|
|
|
MS2 |
0.59
|
1 |
|
|
|
SUSP2 |
0.87
|
0.36 |
1 |
|
|
TC |
0.17 |
0.75
|
−0.15 |
1 |
|
FC |
−0.04 |
0.10 |
−0.33 |
0.51
|
1 |
Powai Lake (n = 16) summer |
RVA |
1 |
|
|
|
|
MS2 |
0.59
|
1 |
|
|
|
SUSP2 |
0.43 |
0.76
|
1 |
|
|
TC |
0.47
|
0.29 |
0.04 |
1 |
|
FC |
0.26 |
0.23 |
0.18 |
0.83
|
1 |
Masunda Lake (n = 16) summer |
RVA |
1 |
|
|
|
|
MS2 |
0.16 |
1 |
|
|
|
SUSP2 |
−0.45 |
−0.37 |
1 |
|
|
TC |
0.39 |
0.26 |
−0.13 |
1 |
|
FC |
0.29 |
0.33 |
−0.63
|
0.39 |
1 |
Powai Lake (n = 12) monsoon |
RVA |
1 |
|
|
|
|
MS2 |
0.56
|
1 |
|
|
|
SUSP2 |
0.40 |
0.80
|
1 |
|
|
TC |
−0.12 |
−0.09 |
0.17 |
1 |
|
FC |
0.18 |
0.32 |
0.59
|
0.75
|
1 |
|
RVA |
MS2 |
SUSP2 |
TC |
FC |
Significant correlations (p < 0.05) are indicated in bold.
|
Masunda Lake (n = 12) monsoon |
RVA |
1 |
|
|
|
|
MS2 |
0.08 |
1 |
|
|
|
SUSP2 |
−0.15 |
0.17 |
1 |
|
|
TC |
−0.62
|
0.11 |
0.15 |
1 |
|
FC |
−0.48 |
0.31 |
0.26 |
0.66
|
1 |
3.5 Binary logistic regression (BLR) of RVA and indicators
BLR was conducted, wherein RVA was the response variable and the variables MS2, SUSP2, TC, and FC were individually analyzed as predictors. This was done so as to prevent the violation of the basic assumption of BLR, i.e., the predictor variables should not be interdependent. Table 4 provides the coefficients and the odds ratio for the BLR model and it can be observed that among the predictors, only MS2 consistently affected the presence of RVA in both the lakes (p < 0.01). The odds ratio (OR) greater than 1 suggested a positive correlation between the presence of MS2 and RVA. This implies that the higher the MS2 population, the higher will be the probability of RVA presence. In contrast, for SUSP2, TC, and FC, the significance level was lower (0.4 < p < 0.06.)
Table 4 Binary logistic regression (BLR) models for predicting the presence of RVA
Predictor |
Powai Lake |
Masunda Lake |
Coefficient |
Odds ratio |
p value |
Coefficient |
Odds ratio |
p value |
Significant correlations (p < 0.05) are indicated in bold.
|
MS2 |
0.64 ± 0.19
|
1.90
|
7.07 × 10
−4
|
0.27 ± 0.11
|
1.31
|
0.01
|
SUSP2 |
0.14 ± 0.08 |
1.15 |
0.09 |
0.05 ± 0.07 |
1.05 |
0.44 |
TC |
0.40 ± 0.21 |
1.49 |
0.06 |
0.19 ± 0.16 |
1.21 |
0.24 |
FC |
0.21 ± 0.21 |
1.24 |
0.32 |
0.11 ± 0.12 |
1.12 |
0.36 |
Discussion
With the development of specific molecular-based methods, such as qPCR, the occurrence of enteric viruses in surface and recreational water bodies has been monitored over the last few decades in various countries worldwide.5,21 Viruses being pathogens circulating in environmental matrices have attracted the interest of various regulatory authorities and researchers. In India, a large proportion of sewage goes untreated into lakes and rivers, and correspondingly, there is numerous incidences of viral outbreaks.43 Despite the high incidence of diarrhoeal outbreaks and other viral diseases, routine monitoring for enteric viruses is not being carried out in water bodies in India.43,44
Among the various enteric viruses, human adenoviruses are the most abundant.21,45 However, in India, a major concern among the enteric viruses is rotavirus.46 Rotavirus infection is known to cause diarrhoea among children and it is one of the major causes of death among children in India.27,47,48 A study conducted by Vivek et al. (2013)25 reported high levels of RVA in wastewater treatment plants, where 77% of the samples tested positive for RVA. A high frequency of occurrence of rotavirus has also been reported across the globe over the years.5,8,49,50 A relatively high prevalence of rotavirus in surface water bodies, such as lakes and rivers, justifies the importance of monitoring rotaviruses in recreational water bodies as well. In the current study, 60% and 71% of the samples from two sewage impacted lakes were found to be positive for RVA.
The physicochemical analysis conducted in various locations in the lakes provided evidence for sewage infiltration into the lakes. Powai lake (which eventually joins the Mithi River) is relatively large. Based on the monitoring conducted, the possible locations for point and non-point sources of sewage entering Powai lake were identified as locations 2, 3, 6, and 7. In location 3, the COD was found to be as high as 300 mg L−1 in certain months, and this high COD value may be attributed to leaking sewers or direct discharge of sewage from nearby slums and establishments. Coliform concentrations were also typically high for these locations (0–104 CFU per 100 mL). Significant temporal variation in pollutant load was observed for both Powai lake and Masunda lake. The COD value for Masunda lake in certain months was as high as 134 mg L−1 which also indicates possible infiltration of sewage into the lake.51 However, definite locations for sewage entry into Masunda lake could not be established. Masunda lake had a relatively homogeneous spatial distribution of pollutants. This may be attributed to its smaller catchment area. Microbial source tracking may be performed in subsequent studies to establish the source and location of fecal pollution in these lakes.
PCA further confirmed the possibility of employing the coliphage, MS2, as an indicator for RVA. RVA showed a moderate (r = 0.46) to strong (r = 0.74) correlation with MS2 in Powai lake and Masunda lake, respectively. In contrast, SUSP2 showed a moderate correlation with RVA only in Powai lake. However, no correlation was observed between RVA and total coliforms for both the lakes. Similar results have been reported in the literature, where enteric viruses, noroviruses GI, rotaviruses, and astroviruses, were found to have a significant correlation with coliphages, and no correlation was found with indicator bacteria.9 Another study conducted by Miao et al. (2018)51 suggested that enteroviruses can be predicted employing total coliforms as an indicator. However, the same study reported that none of the other viruses (rotaviruses, astroviruses, noroviruses GII, and adenoviruses) were correlated with total coliforms. A comparative study of enteric viruses, coliphages, and indicator bacteria for evaluating water quality suggested that coliphages can be used as potential indicators of fecal contamination in reused water.52 In contrast, yet other studies have reported no correlation between enteric viruses (such as rotaviruses and adenoviruses) and either coliphages or coliforms, as shown for Lake Pontchartrain in southeast Louisiana, USA.21 Thus, it can be stated that the correlation between an enteric virus and an indicator virus may vary depending on the climatic conditions and on the type of enteric virus under consideration. In the current study, a trend was consistently observed across the lakes where MS2 (r = 0.74–0.46, p < 0.001) is significantly correlated with RVA with a higher correlation coefficient value followed by SUSP2 (r = 0.46–0.40, p < 0.02).
Seasonal fluctuations in rotaviral infection are documented around the globe, and reports suggest an increased incidence of RVA infections under cold and dry weather conditions. This is particularly true for temperate regions as compared to tropical regions.51,53,54 It has been hypothesized that the survival of infective rotavirus is higher under cool and dry conditions. A relative drop in humidity and rainfall in combination with the drying of soils might increase the aerial transport of dried contaminated fecal material.55,56 Similar observations were recorded in this study, wherein the RVA load was found to be highest during the winter months (November–March). Among the coliphages, somatic coliphages and F-specific phages may be both associated with human or animal fecal contamination.9,57 In the current study, the frequency of detection of MS2 (79–81%) and SUSP2 (75–81%) was comparable. A significant and predominant correlation of RVA with coliphages was observed across the lakes particularly in winter. In contrast, MS2 showed association with RVA during the monsoon and summer months only in Powai lake. Moreover, TC showed a negative correlation with RVA in the monsoon (r = −0.62, p = 0.03). The lack of a positive correlation between human viruses and coliforms has been reported earlier.21,51 The lack of correlation of FC and a negative correlation with FC in Masunda lake was possibly caused by differential decay of human virus, RVA, and fecal indicator bacteria in the environment.58 BLR analysis conducted for RVA as response and indicator variables (MS2, SUSP2, TC, and FC) as predictors also reiterated MS2 as the only significant predictor for RVA presence. The reason could be the similar surface characteristics and also the survival rate of MS2 with enteric viruses.10
Conclusions
Water quality analysis conducted from January 2018 to January 2020 revealed that both lakes were heavily impacted by sewage and temporal variation was significant for both lakes, Powai lake and Masunda lake. In composite lake samples, quantification of RVA and indicators (SUSP2, MS2, total coliforms, fecal coliforms, and COD) suggested a strong positive correlation between coliphages and RVA, particularly in winter. In the summer and monsoon, both coliphages and coliforms may be used as indicators. PCA analysis and BLR further reiterated that MS2 is a better indicator of the enteric virus, RVA. This study thus supports the hypothesis that the coliphage, MS2, is a better indicator of RVA compared to coliforms. The results of this study can help policy makers to improve surface water quality evaluation by adding the surveillance of coliphages.
Conflicts of interest
There are no conflicts to declare.
Acknowledgements
The authors would like to thank Dr. Pramod Salaskar, Secretary, Naushad Ali Sarovar Samvardhini (NASS), for facilitating sampling in Powai lake and Masunda lake over the two years; the Thane Municipal Corporation for providing the necessary permission for sampling in Masunda lake; the Masunda lake boat club and the Maharashtra state angling association for providing the boats for sample collection from the lakes. Lakshmi Pisharody gratefully acknowledges the Shri Girish Vishnuprasad Desai Research Excellence award for partially funding this work. The authors would also like to thank the Tata Centre for Technology and Design (TCTD), IIT Bombay for funding the initial phase of this work.
References
- N. Nwachuku and C. P. Gerba, Rev. Environ. Contam. Toxicol., 2006, 186, 1–56 CrossRef.
- A. C. Schultz, S. Perelle, S. Di Pasquale, K. Kovac, D. De Medici, P. Fach, H. M. Sommer and J. Hoorfar, Int. J. Food Microbiol., 2011, 145, S158–S166 CrossRef.
- T. T. Fong and E. K. Lipp, Microbiol. Mol. Biol. Rev., 2005, 69, 357–371 CrossRef CAS PubMed.
- I. A. Hamza, L. Jurzik, K. Überla and M. Wilhelm, Int. J. Hyg. Environ. Health, 2011, 214, 424–436 CrossRef PubMed.
- S. Rezaeinejad, G. G. R. V. Vergara, C. H. Woo, T. T. Lim, M. D. Sobsey and K. Y. H. Gin, Water Res., 2014, 58, 122–131 CrossRef CAS PubMed.
- J. W. He and S. Jiang, Appl. Environ. Microbiol., 2005, 71, 2250–2255 CrossRef CAS PubMed.
- D. Y. Graham, G. R. Dufour and M. K. Estes, Arch. Virol., 1987, 92, 261–271 CrossRef CAS.
- I. A. Hamza, L. Jurzik, A. Stang, K. Sure, K. Überla and M. Wilhelm, Water Res., 2009, 43, 2657–2668 CrossRef CAS PubMed.
- L. Liang, S. G. Goh, G. G. R. V. Vergara, H. M. Fang, S. Rezaeinejad, S. Y. Chang, S. Bayen, W. A. Lee, M. D. Sobsey, J. B. Rose and K. Y. H. Gin, Appl. Environ. Microbiol., 2015, 81, 850–860 CrossRef CAS PubMed.
- B. R. McMinn, N. J. Ashbolt and A. Korajkic, Lett. Appl. Microbiol., 2017, 65, 11–26 CrossRef CAS PubMed.
-
C. P. Gerba, in Virus occurrence and survival in the environmental waters, Human viruses in water, ed. A. Bosch, Elsevier, Amsterdam, The Netherlands, 2007, pp. 91–108 Search PubMed.
-
N. J. Ashbolt, W. O. K. Grawbow and M. Snozzi, Water quality: Guidelines, standards, and health: Assessment of risk and risk management for water-related infectious disease, in Indicators of microbial water quality, ed. L. Fewtrell and J. Bartram, IWA Publishing, London, UK, 2001, pp. 289–316 Search PubMed.
- H. Leclerc, S. Edberg, V. Pierzo and J. M. Delattre, J. Appl. Microbiol., 2000, 88, 5–21 CrossRef CAS PubMed.
- W. O. K. Grabow, Water SA, 2001, 27, 251–268 Search PubMed.
- J. Jofre, J. Appl. Microbiol., 2009, 106, 1059–1069 CrossRef CAS PubMed.
- M. A. Woody and D. O. Cliver, J. Appl. Microbiol., 1997, 82, 431–440 CrossRef CAS.
- E. Dias, J. Ebdon and H. Taylor, Water Res., 2017, 129, 172–179 CrossRef.
- G. A. Shin and M. D. Sobsey, Water Res., 2008, 42, 4562–4568 CrossRef CAS.
- J. Cormier and M. A. Janes, J. Virol. Methods, 2014, 196, 86–92 CrossRef CAS PubMed.
- V. Tyagi and A. Chopra, Iran. J. Environ. Health Sci. Eng., 2006, 3, 205–216 CAS.
- E. M. Cooksey, G. Singh, L. C. Scott and T. G. Aw, Sci. Total Environ., 2019, 649, 1514–1521 CrossRef CAS PubMed.
- T. H. Jones, J. Brassard, E. Topp, G. Wilkes and D. R. Lapen, Appl. Environ. Microbiol., 2017, 83, 1–22 Search PubMed.
- B. Wangkahad, S. Mongkolsuk and K. Sirikanchana, Environ. Sci. Technol., 2017, 51, 2235–2245 CrossRef CAS.
- A. Zehra, S. Kaur, R. Singh and J. P. S. Gill, Water, Air, Soil Pollut., 2020, 231, 1–12 CrossRef.
- R. Vivek, U. G. Zachariah, J. Ramachandran, C. E. Eapen, D. P. Rajan and G. Kanga, Trans. R. Soc. Trop. Med. Hyg., 2013, 107, 363–367 CrossRef CAS PubMed.
- M. Kumar, B. Ram, R. Honda, C. Poopipattana, V. Duc, T. Chaminda and H. Furumai, Sci. Total Environ., 2019, 693, 133640 CrossRef CAS PubMed.
- J. E. Tate, A. H. Burton, C. Boschi-Pinto, A. D. Steele, J. Duque, and U. D. Parashar, Lancet Infect. Dis., 2012, 12, 136–141 CrossRef.
- E. C. Keen, V. V. Bliskovsky, F. Malagon, J. D. Baker, J. S. Prince, J. S. Klaus and S. L. Adhya, MBio, 2017, 8, e02115–e02116 CrossRef CAS.
- V. Rasal, U. Patil and M. Bhot, Journal of Aquatic Biology and Fisheries, 2019, 7, 110–114 Search PubMed.
-
APHA, Standard Methods for the Examination of water and wastewater, American Public Health Association, Washington, DC, USA, 18th edn, 2012 Search PubMed.
-
J. Sambrook and D. W. Russell, Molecular cloning laboratory manual, Cold spring harbour laboratory press, Cold Spring Harbour, New York, 2001, pp. 1–15 Search PubMed.
- K. P. O'Connell, J. R. Bucher, P. E. Anderson, C. J. Cao, A. S. Khan, M. V. Gostomski and J. J. Valdes, Appl. Environ. Microbiol., 2006, 72, 478–483 CrossRef.
-
K. E. Wommack, K. E. Williamson, R. R. Helton, S. R. Bench and D. M. Winget, Methods for the isolation of viruses from environmental samples, in Bacteriophages methods and protocols, Isolation, characterization, and interactions, ed. R. J. Clokie and A. M. Kropinski, Humana Press, New York, USA, 2009, pp. 3–15 Search PubMed.
-
S. Ehrt and D. Schnappinger, in Isolation of plasmids from E. coli by alkaline lysis, E. coli plasmid vectors, ed. N. Casali and A. Preston, Humana Press Inc., Totowa, NJ, 2003, pp. 75–78 Search PubMed.
- P. Schober and L. A. Schwarte, Anesth. Analg., 2018, 126, 1763–1768 CrossRef PubMed.
- L. E. Black, G. M. Brion and S. J. Freitas, Appl. Environ. Microbiol., 2007, 73, 3965–3974 CrossRef CAS PubMed.
-
B. Sengupta and S. K. Paliwal, Environmental Standards for Ambient Air, Automobiles, Fuels, Industries and Noise, Central Pollution Control Board (CPCB), 2000–2001 Search PubMed.
- P. U. Singare, R. S. Lokhande and K. U. Naik, Interdiscip. Environ. Rev., 2010, 11, 90 CrossRef.
- R. Sinclair, S. A. Boone, D. Greenberg, P. Keim and C. P. Gerba, Appl. Environ. Microbiol., 2008, 74, 555–563 CrossRef CAS.
- V. Ghoshal, M. K. Nayak, N. Misra, R. Kumar, N. S. Reddy and N. K. Mohakud, Indian J. Pediatr., 2021, 88, 41–46 CrossRef.
- A. K. Shrivastava, N. S. Reddy, S. Giri, P. S. Sahu, M. Das, N. K. Mohakud and R. R. Das, J. Global Infect. Dis., 2019, 11, 147–152 CrossRef PubMed.
-
D. T. G. Da Silva, Bacteriophages as indicators of human enteric viruses in Mussels (Mytilus Edulis), Ph.D. thesis, University of Brighton, 2013 Search PubMed.
- S. A. Majumdar, A. K. Nayak and S. N. Misra, Int. J. Civ. Eng. Technol., 2019, 10, 664–670 Search PubMed.
- A. Nair, Curr. Sci., 2019, 116, 1461–1462 CrossRef.
- X. Pang, Y. Qiu, T. Gao, R. Zurawell, N. F. Neumann, S. Craik and B. E. Lee, Water Res., 2019, 153, 349–356 CrossRef CAS PubMed.
- S. Chitambar, V. Gopalkrishna, P. Chhabra, P. Patil, H. Verma, A. Lahon, R. Arora, V. Tatte, S. Ranshing, G. Dhale, R. Kolhapure, S. Tikute, J. Kulkarni, R. Bhardwaj, S. Akarte and S. Pawar, Int. J. Environ. Res. Public Health, 2012, 9, 895–915 CrossRef PubMed.
- S. K. Morris, S. Awasthi, A. Khera, D. G. Bassani, G. Kang, U. D. Parashar, R. Kumar, A. Shet, R. I. Glass and P. Jha, Bull. W. H. O., 2012, 90, 720–727 CrossRef.
- T. Varghese, S. Alokit Khakha, S. Giri, N. P. Nair, M. Badur, G. Gathwala, S. Chaudhury, S. Kaushik, M. Dash, N. K. Mohakud, R. K. Ray, P. Mohanty, C. P. G. Kumar, S. Venkatasubramanian, R. Arora, V. E. Raghava Mohan, J. D. Tate, U. Parashar and G. Kang, Pathogens, 2021, 10, 416 CrossRef PubMed.
- B. Calgua, J. Rodriguez-Manzano, A. Hundesa, E. Suñen, M. Calvo, S. Bofill-Mas and R. Girones, J. Virol. Methods, 2013, 187, 215–221 CrossRef CAS PubMed.
- V. E. Prez, H. R. Poma, G. G. Giordano, M. Victoria, S. V. Nates, V. B. Rajal and P. A. Barril, J. Water Health, 2020, 18, 409–415 CrossRef PubMed.
- J. Miao, X. Guo, W. Liu, D. Yang, Z. Shen, Z. Qiu, X. Chen, K. Zhang, H. Hu, J. Yin, Z. Yang, J. Li and M. Jin, BMC Infect. Dis., 2018, 18, 1–9 CrossRef PubMed.
- A. C. Espinosa, C. F. Arias, S. Snchez-Colán and M. Mazari-Hiriart, Environ. Health, 2009, 8, 1–10 CrossRef.
- J. S. Jagai, R. Sarkar, D. Castronovo, D. Kattula, J. McEntee, H. Ward, G. Kang and E. N. Naumova, PLoS One, 2012, 7, 2–14 CrossRef PubMed.
- M. M. Patel, V. Pitzer, W. J. Alonso, D. Vera, B. Lopman, J. Tate, C. Viboud and U. D. Parashar, Pediatr. Infect. Dis. J., 2013, 32, e134–e147 CrossRef PubMed.
- S. A. Ansari, V. S. Springthorpe and S. A. Sattar, Rev. Infect. Dis., 1991, 13, 448–461 CrossRef CAS PubMed.
- K. Moe and J. A. Shirley, Arch. Virol., 1982, 72, 179–186 CrossRef CAS.
- N. A. Ballester, J. H. Fontaine and A. B. Margolin, J. Water Health, 2005, 3, 59–68 CrossRef CAS.
- S. C. Jiang, W. Chu and J. W. He, Appl. Environ. Microbiol., 2007, 73, 6468–6474 CrossRef CAS.
Footnote |
† Electronic supplementary information (ESI) available. See DOI: 10.1039/d1ew00604e |
|
This journal is © The Royal Society of Chemistry 2022 |