Dimethylamine in cloud water: a case study over the northwest Atlantic Ocean†
Received
2nd September 2022
, Accepted 5th October 2022
First published on 10th October 2022
Abstract
This study analyzes characteristics of an important alkyl amine species, dimethylamine (DMA), in cloud water over the northwest Atlantic. Data were gathered from the winter and summer 2020 deployments of the Aerosol Cloud meTeorology Interactions oVer the western ATlantic Experiment (ACTIVATE) on board the HU-25 Falcon. Thirty-eight out of 98 samples exhibited DMA above detection limits, with the overwhelming majority in the winter season (33, 52% of winter samples) compared to summer (5, 14% of summer samples). Higher levels of DMA were observed in the winter, especially during cold air outbreaks (CAOs), which was also the case for NO3−, NH4+, and non sea salt-SO42−. This is in part due to a combination of low temperatures and offshore flow enhanced with continental pollutants such as from agriculture, industry, urban activity, and biomass burning. Unlike the inorganic acidic anions, oxalate was significantly correlated to DMA in summer and the winter in both CAO and non-CAO conditions, with a presumed reason being biomass burning supported by the consistent correlation between DMA and nss-K+ in each season. ACTIVATE data are compared to a cloud water dataset from the northeast Pacific, with the latter exhibiting much higher DMA levels due possibly to more abundant ocean biological emissions. The seasonal differences and enhancement in DMA during CAO conditions relative to non-CAO winter days motivates continued research into the partitioning behavior of DMA and its sources as amines play an important role in carbon and nitrogen cycles in the marine environment.
Environmental significance
Amines are ubiquitous organic bases that play a crucial role in several atmospheric chemical processes, such as new particle formation, formation of low-volatility amine salts in aerosol particles, and aqueous chemistry. Their presence in particles can impact hygroscopic properties and lead to adverse health effects. While research into the nature of these species in the gas and aerosol phases has intensified in recent decades, much less is known about their nature in cloud water, which offers a large aqueous reservoir for dissolution of gaseous amines while also containing amines through activation of cloud condensation nuclei containing amines. This study exploits a unique airborne dataset over the northwest Atlantic across different seasons to examine the concentration characteristics of one of the most abundant amines, dimethylamine. The results show that this species is much more common in the winter relative to the summer and that conditions associated with cold air outbreaks lead to especially higher concentrations. This work provides additional support for how biomass burning may be an important source of amines in cloud water.
|
1 Introduction
Characterizing cloud water composition is insightful in providing clues about air mass types impacting clouds.1–4 Solute composition in cloud water impacts pH and thus processes dependent on acidity,5 such as aqueous phase reactions.6,7 Cloud water species can also impact gas partitioning8 and affect ecosystems due to wet deposition.9,10 A potentially important yet understudied portion of species in cloud water includes organonitrogen species such as amines.
Amines are ubiquitous organic bases that play a crucial role in several atmospheric chemical processes.11 They participate in new particle formation12–14 and growth processes,15,16 in addition to formation of low-volatility amine salts in aerosol particles.17–20 Their impact extends from altering hygroscopic properties21–23 to adverse health effects.24,25 While research into the gas-aerosol partitioning of amines, reactivity with existing inorganic salts,26,27 and their properties in the aerosol phase has intensified in recent decades,28,29 much less is known about their nature in cloud water, which offers a large aqueous reservoir for dissolution of gaseous amines in addition to the activation of cloud condensation nuclei (CCN) containing amines.30–32
Dimethylamine (DMA) is one of the more easily detected alkyl amines in aerosol and cloud water samples due to its solubility and abundance relative to many other amine species.28,32–35 DMA has a range of sources, including emissions from industry,35 biomass burning,36,37 vehicle emissions,38 animal husbandry,39,40 and the ocean.41 Past work focused on DMA and other alkyl amines in the gas and aerosol phases reported concentrations in different regions and examined interrelationships with the major competing base species (ammonium, NH4+) and acidic species that it can form salts with such as inorganic17,33,42–45 and organic acids.15,16,46,47 To our knowledge, one study focused on DMA specifically in cloud water with airborne measurements.32 This study shows that DMA concentrations are enhanced closest to the California coast (relative to farther offshore) potentially due to marine biogenic emissions and that its concentrations increased significantly when impacted by biomass burning due to presumed dissolution of DMA into cloud water. Stahl et al.48 analyzed cloud water collected during the Clouds, Aerosols and Monsoon Processes – Philippines Experiment (CAMP2Ex) airborne campaign and reported that DMA, on average, accounted for 1.7% of total organic carbon during flights around the Philippines. Crosbie et al.49 analyzed three case flights of developed maritime convection from that same campaign and reported no measurable DMA despite two cases being moderately polluted and the third being influenced by smoke. Others have examined DMA in cloud water using surface-based measurements, such as at mountain Schmücke in central Germany, showing enhanced DMA levels owing to its high solubility.50 DMA has been detected in cloud droplet residual particles, further confirming a role for it in clouds.51 Knowledge gaps pertaining to DMA include a full understanding of its partitioning behavior between gas, solid, and aqueous media and the dependence of these processes on factors such as pH, humidity, and temperature.11,28
We leverage a unique cloud water dataset collected in recent deployments of the Aerosol Cloud meTeorology Interactions oVer the western ATlantic Experiment (ACTIVATE)52 to study the seasonal variation of DMA levels in cloud water, relationships between DMA and other species in cloud water, and possible influential sources for DMA. Results from ACTIVATE are then directly compared to the Youn et al.32 study that investigated DMA in cloud water off the California coast. We focus on DMA as that was the only alkyl amine detected in ACTIVATE cloud water samples.
2 Data
2.1 ACTIVATE campaign description
The ACTIVATE campaign comprised two aircraft (HU-25 Falcon and King Air) flying in vertical coordination over the northwest Atlantic to investigate aerosol-cloud-meteorology interactions. In situ data were collected by the Falcon, which flew below, in, and just above boundary layer clouds. The King Air flew at ∼9 km and conducted remote sensing of the atmospheric column underneath the aircraft and launched dropsondes. Six deployments were conducted between 2020 and 2022, with separate winter and summer deployments each year. This study focuses on cloud water samples collected by the Falcon during the first two deployments in 2020: winter (14 February – 12 March 2020) and summer (13 August – 30 September 2020). We further divided the winter flights into two categories to isolate data on cold air outbreak (CAO) conditions, as recent work shows unique conditions in those flights affecting aerosol particles and clouds.53–56 We used the same methods in recent ACTIVATE studies53,57 to distinguish CAO days using a combination of Visible Infrared Imaging Radiometer Suite (VIIRS) imagery (NASA Worldview), flight reports, weather forecast data, and King Air dropsonde data.
The Falcon flight path typically constituted a series of legs below, in, and above clouds.58 The most relevant legs to this study are the “below cloud base (BCB)” legs from which we used meteorological data, and the “above cloud base (ACB)” and “below cloud top (BCT)” legs where cloud water samples were gathered. Cloud water samples might have also been collected during slant ascents and descents between the legs whenever the aircraft was in cloud.
2.2 Falcon data
2.2.1 Cloud water.
The Falcon collected cloud water samples using the Axial Cyclone Cloud-water Collector AC3.59 The AC3 inertially separates droplets from the air stream with a collection efficiency >60% above diameters of 20 μm. The median duration of collection per sample was 5.0 minutes (25th/75th percentile = 2.5/9.4 minutes). Samples collected in vials were analyzed post-flight via ion chromatography (IC) using documented methods.60,61 Each sample ran for 30 minutes, which included a 5 minute equilibrium time between samples at a flow rate of 0.4 mL min−1. Anion analysis relied on a multi-step gradient method and a Dionex IonPac ASH11-HC (2 mm × 250 mm) column, AERS 500e suppressor, and potassium hydroxide (KOH) as the eluent. For cation analysis, an isocratic method was used with methanesulfonic acid (MSA) as the eluent, a Dionex IonPac CS12A (2 mm × 250 mm) column, and a CERS 500e suppressor. The subset of detected species relevant to this study includes DMA, sodium (Na+), methanesulfonate (MSA), ammonium (NH4+), nitrate (NO3−), oxalate, and non-sea salt forms of sulfate (nss-SO42−), calcium (nss-Ca2+), and potassium (nss-K+). The nss parameters were calculated using knowledge of Na+ and concentrations in each sample of either SO42−, Ca2+, or K+ and the ratio of Na+ to each of those three species in natural sea water62 with the assumption that all Na+ is from sea salt. The limits of detection can be found in Table S1.† Air-equivalent solute concentrations are reported following the same method described in Gonzales et al.61 Briefly, aqueous concentrations from the IC are multiplied by the mean liquid water content (LWC) in clouds (i.e., when LWC ≥ 0.02 g m−3) during the period of sample collection divided by the density of liquid water. LWC data are used from the Fast Cloud Droplet Probe (FCDP; 3 μm–50 μm; Stratton Park Engineering Company Incorporated (SPEC Inc.)).63,64
Concentrations of a suite of elements were measured using an inductively coupled plasma mass spectrometer (ICP-MS; Agilent 7800 Series). Samples were analyzed in general purpose plasma mode (helium) with an integration time of 0.5 seconds for all elements. A semi-quantitative analysis was performed using a two-point calibration (calibration blank and 10 ppb semiquantitative standard). Internal standards of indium (I) and bismuth (Bi) were added to all standards and samples via on-line addition at a final concentration of 50 ppb. Elements discussed in this work include: Al, V, Fe, Ni, Cu, Zn, As, Cd, Pb. We additionally measured pH of the cloud water samples using an Orion Star™ A211 pH meter coupled to an Orion™ 8103BNUWP ROSS Ultra™ pH electrode (precision of 0.01). Two-point calibrations (pHs 4 and 7) were conducted for the pH measurements.
Of the 98 cloud water samples gathered during the 40 research flights in 2020, 38 exhibited DMA concentrations above the detection limit. The median cloud water sample volumes collected were 3.9 mL and 1.9 mL for the winter and summer, respectively. Although this might seem like a limited number of samples, there is significant difficulty in collecting such samples and DMA is generally at low concentration as compared to species more readily measured by IC such as Na+ and SO42−. The low DMA concentrations do not diminish the importance of characterizing this species as it is a marker representative of alkyl amines that are poorly understood in the context of aerosol–cloud interactions and general characterization of cloud water. There is significant scarcity in cloud water DMA concentrations reported in the literature motivating the importance of learning about this sample set.
2.2.2 Other Falcon data.
Meteorological data used in this study include static air temperature (Rosemount 102 sensor) and water vapor mixing ratio (WVMR) and relative humidity (RH) derived from the Diode Laser Hygrometer (DLH).65 Carbon monoxide (CO) data were obtained from a Picarro G2401 gas concentration analyzer.66 Submicrometer aerosol composition data were measured by an Aerodyne High-Resolution Time-of-Flight Aerosol Mass Spectrometer (AMS)67 with organic mass concentrations of most relevance to this study. Those data were collected in a 1 Hz fast-MS mode and averaged up to 25 second resolution. All other instrument data from this section are used at 1 second resolution.
2.3 Supporting datasets
Air mass transport pathways leading up to the point of cloud water samples were identified using the Hybrid Single-Particle Lagrangian Integrated Trajectory (HYSPLIT)68,69 model. Global Data Assimilation System (GDAS) meteorological data (0.5° × 0.5°) were used to acquire 72 and 240 hour back trajectories with 6 hour time resolution per trajectory. Speciated optical depth data over North America and the northwest Atlantic are used from the Navy Aerosol Analysis and Prediction System (NAAPS).70,71 We use NAAPS data at 1° × 1° spatial resolution and 6 hour temporal resolution with a focus on smoke optical depths.
To aid in aerosol type identification during one of the case flights presented in this work, we used data from the nadir-viewing high spectral resolution lidar (NASA Langley Research Center HSRL-2) installed on the King Air. We used these data in combination with in situ and model datasets to infer the presence of biomass burning aerosols above the planetary boundary layer. Readers are referred to Burton et al.72 for information about the HSRL-2 operational details.
To examine the potential role of ocean biogenic emissions as a source of DMA in cloud water,18 we obtained Level-3 (8 day average, 4 km resolution) MODIS-Aqua data for chlorophyll a (mg m−3) and the particulate backscattering coefficient (bbp; 443 nm; m−1) that encompassed the winter and summer 2020 ACTIVATE flight domains. The chlorophyll a product represents the concentration of the photosynthetic pigment in the upper ocean derived from passive remote sensing of ocean color.73 The particulate backscattering coefficient describes the radiant intensity scattered into the backwards direction by particles in the water column, which can be used as a proxy for the particulate organic carbon concentration in waters where scattering is dominated by organic particles (e.g., open ocean regions with low concentrations of inorganic particles).74 For two case studies presented in this work where high-level clouds prevented passive ocean color remote sensing retrievals, HSRL-2 retrievals of bbp (532 nm; 10 m depth) measured from the King Air were used to supplement the seasonal patterns highlighted in the passive imagery.75 We caution that chlorophyll a and bbp are not perfect proxies for upper ocean biomass or the amount of organic carbon enrichment in marine-emitted aerosol particles;76,77 however, in the face of these limitations there have been links shown between amine concentrations over the ocean and at least chlorophyll a.32,33,78
Lastly, to put the results from ACTIVATE in perspective, data are used from another airborne campaign with documented DMA concentrations in cloud water,32 which was the Nucleation in California Experiment (NiCE; July–August 2013) based in Marina, California with 23 flights over the northeast Pacific.79–81 The Center for Interdisciplinary Remotely-Piloted Aircraft Studies (CIRPAS) Twin Otter payload in that campaign is summarized elsewhere,82 and of most relevance here is the modified Mohnen slotted-rod collector83 which obtained 119 samples that were analyzed with the same IC system as the ACTIVATE samples.32 Past work has intercompared the AC3 and slotted-rod collector on the CIRPAS Twin Otter and showed that campaign-wide mean concentrations of trace species exhibited good agreement between the two collectors during the 2016 Fog and Stratocumulus Evolution (FASE) campaign over the northeast Pacific.59
3. Results and discussion
3.1 DMA seasonal concentrations
We take advantage of ACTIVATE's dataset for two different seasons to study interseasonal DMA concentration trends. Others have shown seasonal differences in amine behavior, such as how higher atmospheric water and particle acidity in the summer (versus fall) can enhance aminium salt formation in particles.46 Cloud water concentrations of DMA arise mainly from this species being associated with the CCN that nucleate into cloud drops or from dissolution of gaseous amines into cloud water. Furthermore, processes such as hydrolysis in the aqueous phase can convert amines into amides and vice versa.84 Past work over the northeast Pacific suggested that DMA levels were significantly larger in cloud water compared to aerosol measurements with a particle-into-liquid sampler around and in clouds, with the speculated reason being volatilization of the DMA upon droplet evaporation,32 much like what has been observed for nitrate.81 Amines were detected in higher relative amounts in cloud residues relative to outside of clouds in a different study with the reasoning being their high solubility.50
The number of samples with detectable DMA was distributed as follows relative to the total number of cloud water samples collected per category as a percentage (locations in Fig. 1): winter non-CAO = 16 out of 24 (66.7%); winter CAO = 17 out of 39 (43.6%); summer = 5 out of 35 (14.3%). The median/mean/standard deviation/25th percentile/75th percentile levels (ng m−3) of DMA in each category were as follows: winter non-CAO = 1.61/3.91/6.11/0.64/3.45; winter CAO = 4.97/7.39/7.86/1.51/7.79; summer = 0.25/0.63/0.69/0.25/0.34. These results indicate markedly less DMA abundance in cloud water in the summer. During the winter, DMA concentrations were considerably higher in CAO conditions (when comparing samples with detectable DMA), with over a factor of 3 enhancement in DMA's median concentration relative to non-CAO conditions, which exceeded enhancements of all other species when comparing those two categories (Table 1).
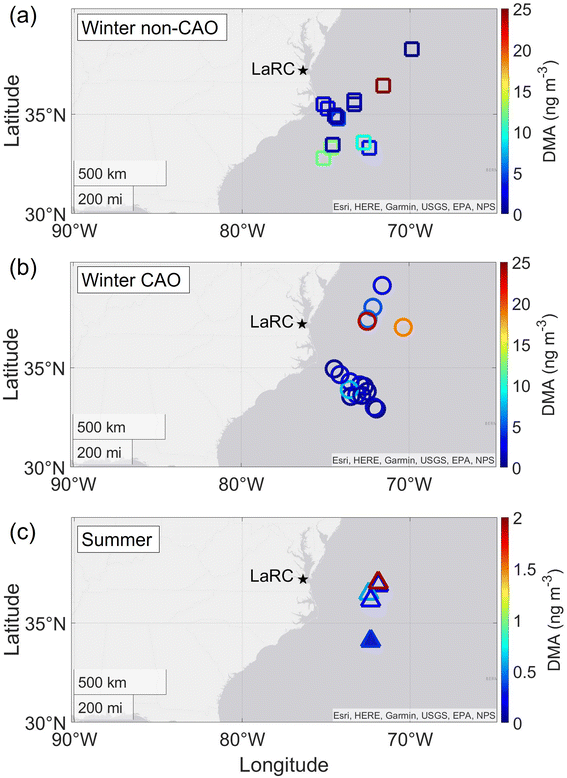 |
| Fig. 1 Cloud water DMA air-equivalent concentration (ng m−3) spatial maps for (a) winter non-CAO, (b) winter CAO, and (c) summer periods in 2020. The filled marker in panel (c) represents a sample influenced by biomass burning. | |
Table 1 Median values and sample numbers for the following parameters categorized into winter non-CAO, winter CAO, and summer conditions: cloud water pH and air-equivalent solute concentrations, cloud water sample altitudes, the cloud water DMA
:
NH4+ molar ratio, relevant meteorological variable values from below cloud base (BCB) legs, and ocean biological parameter values from MODIS-Aqua
|
Winter non-CAO |
Winter CAO |
Summer |
Median |
n
|
Median |
n
|
Median |
n
|
Sample altitude (m) |
1397 |
16 |
1403 |
17 |
1668 |
5 |
Cloud water pH |
4.54 |
11 |
5.21 |
16 |
5.91 |
6 |
NH4+ (ng m−3) |
146 |
24 |
318 |
39 |
29 |
29 |
DMA (ng m−3) |
1.61 |
16 |
4.97 |
17 |
0.25 |
5 |
NO3− (ng m−3) |
1813 |
24 |
2960 |
39 |
507 |
33 |
nss-SO42− (ng m−3) |
147 |
24 |
164 |
39 |
25 |
35 |
DMA : NH4+ (molar basis) |
0.003 |
16 |
0.002 |
17 |
0.001 |
5 |
BCB carbon monoxide (ppm) |
0.14 |
4015 |
0.14 |
4215 |
0.12 |
7041 |
BCB air temperature (°C) |
4.52 |
6521 |
1.00 |
7258 |
19.75 |
9737 |
BCB relative humidity (%) |
86 |
6521 |
84 |
7258 |
88 |
9737 |
BCB water vapor mixing ratio (ppmv) |
7834 |
6521 |
6151 |
7258 |
20 938 |
9737 |
Chlorophyll a (mg m−3) |
0.84 |
5 |
0.84 |
5 |
0.49 |
7 |
Particulate backscattering coefficient (bbp; 443 nm; m−1) |
0.003 |
5 |
0.003 |
5 |
0.002 |
7 |
For context, median cloud water DMA concentrations off the California coast32 were 5.8 ng m−3 (n = 42) and 10.1 ng m−3 (n = 11) for samples without and with biomass burning influence, respectively. In terms of aerosol concentrations over the North Atlantic (West Irish Coast), median submicrometer levels during periods of low (n = 7) and high (n = 5) biological activity were 1 and 10 ng m−3, respectively.18 Median DMA levels in the submicrometer range (0.14–0.42 μm) at Cape Verde were as follows: May 2007 = 0.21 ng m−3 (n = 11); June 2007 = 0.21 ng m−3 (n = 10); December 2007 = 0.54 ng m−3 (n = 24). Reported DMA concentrations over the northwest Atlantic during ACTIVATE flights and those archived from the eastern North Atlantic are considerably lower than those over the northeast Pacific.
3.2 Seasonal variability in related variables
Table 1 examines median values of different variables for comparison with DMA. The decreasing order of median DMA concentrations from winter CAO to winter non-CAO and summer is similar to the following three species among the various ions and elements examined: NH4+, NO3−, nss-SO42−. Factors affecting DMA concentration variability include air mass source, temperature, acidity, and RH.20,33,40,45,46 Although causal evidence is not provided, we compare seasonal trends in these factors versus trends in DMA concentrations. It is first important to note that there was not a significant difference (p > 0.05; Mann–Whitney test) between the altitude that cloud water samples were collected for each of the three categories based on Table 1 values.
3.2.1 Cloud water pH.
Cloud water was least acidic during the summer (5.91) as compared to winter (CAO = 5.21; non-CAO = 4.54), with the median values being statistically different (p < 0.05; Mann–Whitney test). Rehbein et al.85 showed that trimethylamine partitions better onto pre-existing particles during periods of acidic cloud processing. Total Henry's law constants of amine bases increase substantially with decreasing pH promoting greater uptake of amines from the gas phase.28 The trend of more acidic cloud water and higher DMA levels in the winter periods in the study region is consistent with elevated DMA levels at higher acidity in the literature.
3.2.2 Temperature and water vapor.
We next examined meteorological parameters measured in below cloud base (BCB) legs to assess why summertime concentrations of DMA, NH4+, NO3−, and nss-SO42− are lowest and why in winter, their concentrations are highest in CAO conditions (when detected). A statistically significant difference was observed between the medians (p < 0.05; Mann–Whitney test) of all three categories for all the variables in Table 1, with the only exceptions being: summer versus winter non-CAO for CO; sample altitude; DMA
:
NH4+ molar ratio between the two winter seasons; chlorophyll a and particulate backscattering coefficient between summer and winter due to insufficient sample size.
Higher air temperature and water vapor mixing ratio were observed during summer over the study area, while the lowest values were in winter CAO conditions. As one source of DMA in cloud water is activation of CCN containing DMA, it important to note that there is a thermodynamic preference amines (similar to NH4+, NO3−) have in the aerosol phase for lower temperatures owing to their semi-volatile nature.36 Moreover, lower temperatures help gaseous amines partition more effectively into the aqueous phase.28 While RH showed little difference between the three temporal categories owing to a large extent to its dependence on temperature, the water vapor mixing ratio was considerably lower in winter. It is unclear how important that factor was versus the lower ambient temperatures to lead to higher cloud water DMA concentrations. In contrast to ACTIVATE and other regions like the southeast U.S.,36 data from the semi-arid southwestern U.S. revealed higher particulate DMA levels with higher temperature and water vapor mixing ratio with the speculated cause being more sulfate available for aminium salt formation, more biogenic volatile organic compound emissions, and more aerosol-phase water to promote equilibrium partitioning.86 Certainly, the range of results among regions warrants continued research to understand the sensitivity of DMA partitioning behavior to both temperature and various proxies of humidity such as water vapor mixing ratio and RH. Temperature and humidity variables could potentially co-vary with other factors that may be influential for DMA concentrations, which is hard to decipher with the current dataset.
3.2.3 Atmospheric circulation and carbon monoxide.
Fig. 2 shows 72 hour HYSPLIT air mass trajectories leading up to where cloud water samples were detected. The range of DMA sources have been noted already, but here we list relevant sources in the specific study region. There is great potential for marine biogenic emissions as the flight data were over the northwest Atlantic. But there are also agricultural sources such as dairy farms and poultry operations in Pennsylvania, Delaware, Maryland, and Virginia that are major emitters of NH3
87 and presumably could be as well for amines. There are extensive industrial operations along the U.S. east coast along with major populated areas leading to a wide range of anthropogenic emissions.56 Biomass burning is common throughout the year, ranging from residential wood burning along the U.S. east coast, especially in winter88 to transported smoke plumes from wildfires and agricultural burning.89
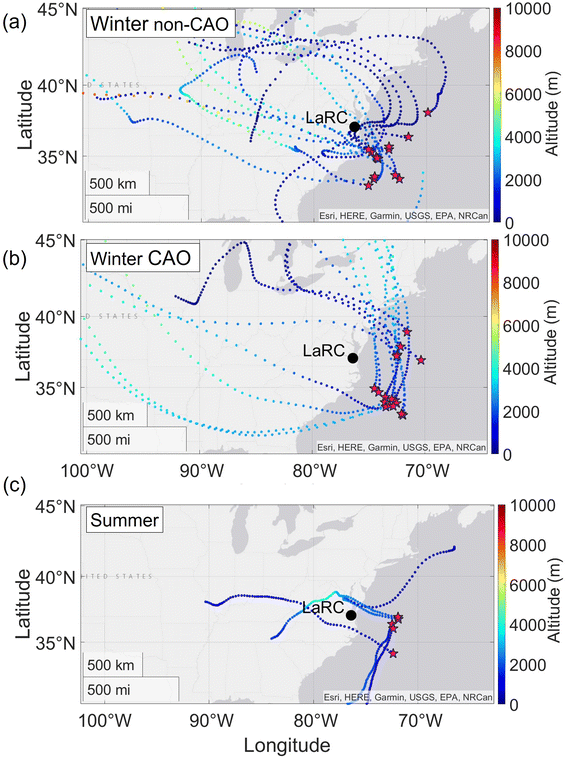 |
| Fig. 2 HYSPLIT 72 hour back trajectories corresponding to the cloud water samples marked by stars for (a) winter non-CAO, (b) winter CAO, and (c) summer. Color bar shows the altitude for each back trajectory every 6 hours. | |
As expected, trajectories were mainly from over the U.S. east coast for the two winter categories without much discernible difference. These air masses were thus significantly impacted by continental emissions, including those from anthropogenic sources, agricultural activity, and likely biomass burning.88,90 In summer, the trajectories were different (albeit based on five samples) and characteristic of the summertime atmospheric circulation with boundary layer winds moving north along the coast;91 thus, these air masses are presumed to have had less continental influence than the winter categories. A higher median altitude was calculated for the total 72 hour duration of back trajectories in winter CAO conditions (2951 m) as compared to winter non-CAO (1888 m), with the values being statistically different (p < 0.05; Mann–Whitney test). Higher altitudes coming from the north during the winter CAO are consistent with colder air, which as noted in Section 3.2.2 helps promote more partitioning of DMA into cloud water.
Carbon monoxide is a tracer for anthropogenic emissions. It has been shown to be higher in winter 2020 ACTIVATE flights compared to summer and thus possibly helpful in explaining why there is more prevalent nucleation (e.g., more precursor concentrations such as SO2).53Table 1 reinforces that CO is higher in winter, which is consistent with air mass trajectories being aligned with offshore flow. There was not a major difference in median CO levels between the two winter categories (∼0.14 ppm), even though statistically, they were different (winter non-CAO > winter CAO). Therefore, more continental influence in the winter likely contributed to higher DMA levels. However, the relative importance of air mass source origin versus other factors such as temperature cannot be teased out with this dataset.
A few noteworthy features exist when comparing Fig. 1 and 2. In winter non-CAO conditions, the samples with the highest DMA (>10 ng m−3) are linked to trajectories that traveled over the southeast U.S., which has extensive agricultural burning during the winter months, such as with burning related to the sugarcane pre-harvesting season.92–95 The samples with notably high DMA (>15 ng m−3) in winter CAO conditions near 37°N were closest to land based on trajectory paths (mainly northerly) and thus least impacted by processes reducing pollutant concentrations during transport such as dilution and wet scavenging that commonly occurs offshore during CAO events which have high cloud fraction.96,97 As will be shown later, one DMA sample in the summer (Fig. 1c) was linked to transported biomass burning.
3.2.4 Ocean biology.
There is mounting evidence that DMA production is influenced by ocean biological activity over the eastern North Atlantic18,33 and other regions such as the northeast Pacific Ocean.32 Periods of high ocean biological activity are linked to increased levels of organic nitrogen over the western North Pacific.98Table 1 shows the domain-wide mean values of chlorophyll a and particulate backscattering coefficient (ocean areas between 32–38°N, 69–77°W) based on the visual illustration in Fig. 3. There are higher chlorophyll a and particulate backscattering coefficient values near the coast. There were no major changes in either of the two parameters during the winter and summer 2020 campaigns, preventing the opportunity to compare periods of low and high biomass as in other studies.18 A cautionary note again in light of this discussion is that there is an important distinction between biomass and biological activity as high standing stock does not necessarily translate into high emissions rates. However, we find value in still examining DMA trends as a function of ocean biomass levels.
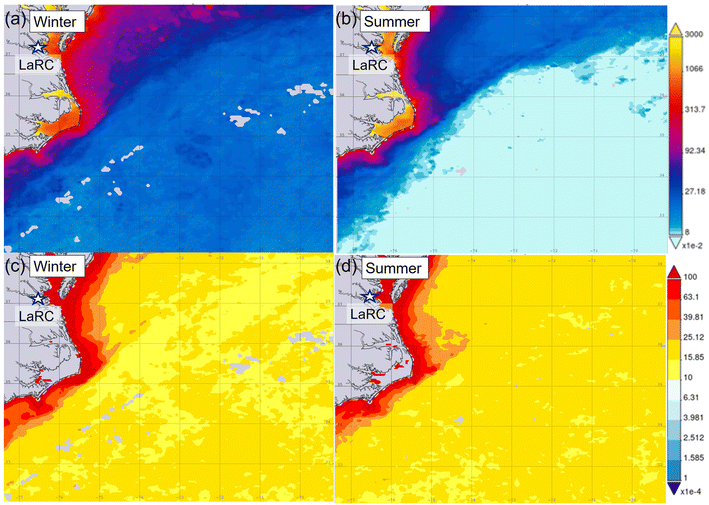 |
| Fig. 3 Averaged MODIS-Aqua satellite images for the ACTIVATE region (https://www.oceancolor.gsfc.nasa.gov/) coinciding with (a and c) winter (14 February 2020–12 March 2020) and (b and d) summer (13 August 2020–30 September 2020) deployment periods. Panels (a) and (b) show results for chlorophyll a (mg m−3) in winter and summer, respectively. Particulate backscattering coefficient (bbp; 443 nm; m−1) seasonal spatial maps are shown in panels (c) and (d). | |
While it is difficult to spatially link such values in these maps to actual cloud water samples owing to the range of limitations in making such a comparison, we focus on seasonal differences in ocean parameters and cloud water DMA. Both chlorophyll a and the particulate backscattering coefficient were larger in the winter, similar to DMA. Others have emphasized the limitation of these ocean biological parameters in terms of relationships with marine aerosol and cloud water organic enrichment,76,77 but seasonal differences in Table 1 are at least supportive of a relationship that requires more detailed research. What remains difficult to disentangle is the relative importance of ocean biogenic emissions versus continental sources and other factors in governing cloud water DMA levels.
The study of Youn et al.,32 which reported considerably higher cloud water DMA concentrations, was characterized by higher chlorophyll a values (∼2–6 mg m−3; see their Fig. 7) in the domain of the 72 hour back trajectories impacting their sampled air masses. Although impossible to prove here, those higher values are at least in support of a possible link between higher biogenic emissions and more cloud water DMA in that marine region compared to the northwest Atlantic. Comparing chlorophyll a values between studies is challenging as several factors impact how marine emissions affect clouds including the spatial domain over which these values are obtained.
3.3 Species interrelationships
Table S2† reports the interrelationships between various species of interest, broken down into the three temporal categories discussed already. The associated scatterplots are in Fig. S1.† We report Pearson's correlation coefficient (r) values only if they are statistically significant at a 95% confidence level (p < 0.05). The absolute r value categorizes the strength of the relationship as follows: r = 0–0.2: weak; r = 0.21–0.4: moderately weak; r = 0.41–0.6: moderate; r = 0.61–0.8: moderately strong; and r ≥ 0.81: strongly correlated.99 A variety of species are included in Table S2† to represent different types of sources with the caveat that many of these species have multiple sources:62,79,100–104 sea spray (Na+), crustal matter (Al, Fe, nss-Ca2+), marine biogenic emissions (MSA), combustion (As, Pb, Zn, Cu, V, Ni, nss-K+), waste processing (Cd), and secondary aerosol formation processes (NH4+, NO3−, nss-SO42−, oxalate). Relationships in Table S2† are insightful in providing hints about potential emissions sources and/or suggesting which acidic gases (e.g., sulfuric, nitric, organic acids) participate in salt formation with DMA.
The winter temporal categories have one common feature regarding DMA and that is the significant correlation between DMA and NH4+ (r = 0.97). This is likely due to ammonia and amines being co-emitted from various sources such as from industry,35 biomass burning,36 animal husbandry,40 and the ocean.41 In addition, NH4+ is a major base participating in salt formation with acids much like DMA does,18,105 which has been supported by similar mass size distributions between DMA and NH4+ in various regions.32,45 Other similarities between the winter categories for DMA include a moderate correlation with oxalate (r = 0.58), moderately strong and strong correlations with nss-Ca2+, nss-K+, and Al (r: 0.79–1.00).
Both nss-SO42− and NO3− are major inorganic anions participating in salt formation with amines, as evident from similar mass size distributions with DMA.32,45,106 It is somewhat surprising that DMA is only correlated to both acids in winter CAO conditions, with the correlation being slightly stronger for NO3− (r = 0.94) than nss-SO42− (r = 0.79). Oxalate is examined as there has been increased research investigating properties of alkylaminium carboxylate particles107 pointing to appreciable hygroscopicity depending on the amine and carboxylic acid participating in salt formation together.22 Oxalate was correlated to DMA in both winter seasons as already noted providing at least some support for a common formation mechanism and/or emissions source(s). Although not the only source, biomass burning is common in the wintertime along the U.S. east coast88 and is an important source for DMA, oxalate, and several other species in Table S2† such as MSA, nss-K+, and Al.32,36,106 We caution that some of the difference between winter categories can be due to the reduced sample sizes as is visually illustrated in Fig. S1† as a minority of points often can strongly influence a correlation coefficient. An important result is that the winter CAO conditions yield not only higher overall levels of cloud water DMA, NH4+, NO3−, and nss-SO42−, but also more statistically significant correlations with species in Table S2† presumably due to more polluted air masses from the continent enriched with most of the various species studied here.
As will be shown in a case study flight, the summertime correlation results are driven mainly by one sample that was influenced by biomass burning. This is the most plausible explanation for why DMA is strongly correlated with nss-K+, oxalate, nss-SO42−, and Na+ (r: 0.88–0.97), as these species have been shown to be enhanced in concentration during periods of biomass burning.104,106,108 Other strong correlations with DMA in summer include Cu, Zn, Cd, and Pb (r = 1.00), which suggests these species were also enhanced in the single sample with biomass burning influence.
MSA is of relevance to this work as it stems partly from marine biogenic emissions109 and because nucleation in the marine atmosphere can occur between mixtures of water, amines, and MSA.110 However, MSA was only significantly correlated to DMA in winter non-CAO conditions and strongly correlated with NH4+ and NO3− in that same category (r = 0.96 for both). This suggests it is not co-emitted with DMA to the extent that it is a strong predictor for DMA enhancement. Similar conclusions apply to the sea salt tracer species Na+, which did not show a consistently strong correlation with DMA in all three temporal categories.
We compared the correlation results to those from the NiCE cloud water dataset32 over the northeast Pacific (Table S3†). The NiCE results show that DMA was strongly correlated with NH4+ (r = 0.83), like ACTIVATE, but also with oxalate (r = 0.83). In fact, oxalate was more correlated with DMA than the major inorganic anions NO3− (r = 0.63) and nss-SO42− (r = 0.38). This reinforces the importance of continued research into alkylaminium carboxylate particles.22 Like ACTIVATE, there was no strong correlation throughout the NiCE campaign between DMA and either Na+ or MSA, indicating that these species commonly derived from different ocean emission processes are not good predictors for the presence of DMA in clouds.
Of interest is the relative abundance on a molar basis of DMA versus NH4+, as the latter is the primary base in salt formation. Other work has suggested that there is competitive uptake between DMA and NH3 on acidic particles in the marine atmosphere dependent on the DMA
:
NH3 molar ratio.111 One study showed that the ratio of particulate dimethylaminium
:
NH4+ in liquid particles (compared to dry particles) exceeded that of DMA
:
NH3, despite excess NH3 in the gas phase, demonstrating the important role of the aqueous phase;112 they defined dimethylaminium as nNH2(CH3)2+ + nNH(CH3)2. Table 1 shows that the median DMA
:
NH4+ molar ratio was higher in the two winter categories (0.002 = CAO, 0.003 = non CAO) than the summer (0.001). The DMA
:
NH4+ molar ratio is considerably higher in NiCE (0.018; n = 77) than ACTIVATE (0.002; n = 37) in the absence of biomass burning. When compared to those values, there was very little difference in the respective campaign-specific ratios in the presence of biomass burning for NiCE (0.019; n = 10) and ACTIVATE (0.001; n = 1). Note that this excluded classification of ACTIVATE data into the biomass burning category if there was residential wood burning, such as in the winter,88 which is harder to identify as compared to wildfire plumes. Molar ratios reported from aerosol measurements in southern Arizona and the NiCE region by the central California coast ranged from 0 to 0.04,32 whereas values in the fine mode ranged from 0.005–0.2 for rural and continental air by Toronto.45
Fig. 4 shows the relationship between NH4+ and DMA. The positive correlation is slightly higher for NiCE (R2 = 0.69) relative to ACTIVATE (R2 = 0.54). The separation between the data points per campaign points to some unique differences between the two regions. It is unclear if the separating factors are more related to pollution sources, transport behavior and photochemical aging differences between NH3/NH4+ and DMA, or meteorology, warranting analysis of data from other regions.
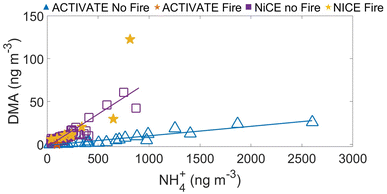 |
| Fig. 4 Relationship between DMA and NH4+ mass for ACTIVATE (R2 = 0.54; blue) and NiCE (R2 = 0.69; purple). Star markers represent those samples in each campaign that were influenced by biomass burning from summertime wildfires. Equations of the best fit lines for each campaign were as follows: NiCE = 0.08x − 2.89; ACTIVATE = 0.01x − 1.02. | |
3.4 Case studies
3.4.1 Winter flights.
Two case flights are summarized here that were selected based on having numerous cloud water samples. The first flight (Fig. 5a–c) on 14 February 2020 was in CAO conditions, unlike the second flight (Fig. 6a–c) on 6 March 2020. The flight on 14 February was in post-frontal conditions with high cloud fraction during most of the flight, with sampling in warm and mixed-phase clouds. The wind direction was northerly/northwesterly along most of the flight track, consistent with the organization of the cloud street features characteristic of CAO conditions over the northwest Atlantic. DMA concentrations in the four collected cloud water samples ranged from 5.63 to 24.78 ng m−3, which is on the high end of the concentrations during ACTIVATE flights (Table 1). These samples were collected at altitudes ranging between 837 and 1400 m.
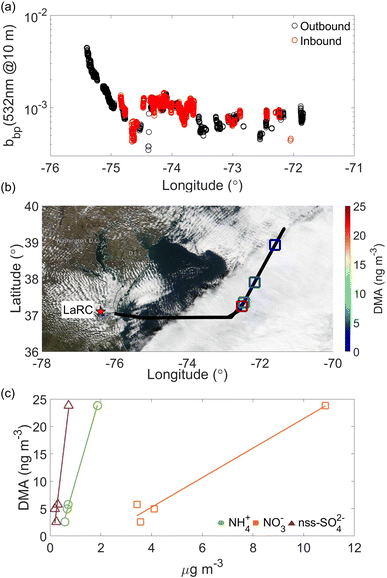 |
| Fig. 5 Summary of data from ACTIVATE research flight 1 on Friday 14 February 2020. (a) HSRL-2 particulate backscattering (bbp; 532 nm) coefficient near the ocean surface (depth of 10 m) as a function of longitude during the round flight. Red and black markers show data for the inbound and outbound tracks, respectively. (b) Spatial map of DMA concentrations overlaid on true-color imagery from the Visible Infrared Imaging Radiometer Suite (VIIRS; NASA Worldview). (c) Scatter plots of DMA versus inorganic species with linear best fits shown (R2: DMA–NH4+ = 0.99, DMA–NO3− = 0.98, DMA–nss-SO42− = 0.96). | |
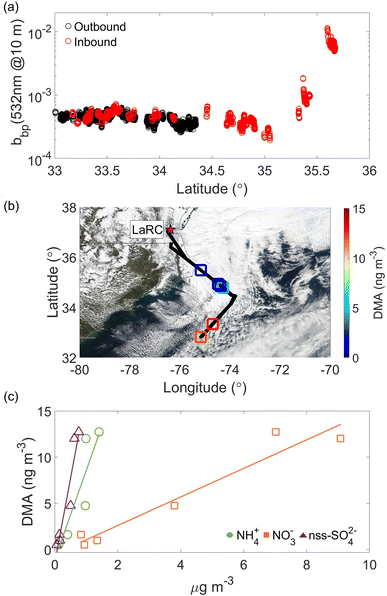 |
| Fig. 6 Summary of data from ACTIVATE research flight 16 on Friday 6 March 2020. (a) HSRL-2 particulate backscattering (bbp; 532 nm) coefficient near the ocean surface (depth of 10 m) as a function of latitude during the flight. Red and black markers show data for the inbound and outbound flights, respectively. (b) Spatial map of DMA concentrations overlaid on true-color imagery from the Visible Infrared Imaging Radiometer Suite (VIIRS; NASA Worldview). (c) Scatter plots of DMA versus inorganic species with linear best fits shown (R2: DMA–NH4+ = 0.82, DMA–NO3− = 0.94, DMA–nss-SO42− = 0.91). | |
DMA exhibited strong correlations with NH4+, nss-SO42−, NO3−, and nss-K+ (R2 ≥ 0.89). The strong relationship with nss-K+ in the wintertime could be due to residential wood burning emissions.88 The spatial distribution of concentrations shows one sample with especially high concentrations near the turn point of the aircraft. The results of this flight support continental emissions being an important driver of the high concentrations owing to the origins of these species from anthropogenic precursors. We speculate that low temperatures characteristic of CAOs may help facilitate the formation of ammonium- and aminium-based salts that can activate into drops, in addition to dissolution of gaseous amines into cloud water.28 It is hard to tease out the relative importance of both mechanisms with the current dataset.
As the ocean biological proxies from MODIS-Aqua have coarse temporal resolution, here we provide context during the flight by coloring the flight track with the HSRL-2 retrieval of the particulate backscattering coefficient. Further motivation for using the airborne HSRL-2 data is that ocean satellite data is obscured by clouds, including cirrus clouds. Linking the lidar data for upper ocean characteristics to cloud data is complex and needs to consider the actual amount of ocean biogenic emissions, transport path, and the possibility of wet scavenging of particles during transport offshore. However, this is a unique application of HSRL-2 data that we explore here and recommend leveraging in future studies of this nature. Fig. 5a reveals a longitudinal gradient with higher particulate backscattering coefficient values closer to the coast, suggesting that likely ocean emissions were not a strong driver of DMA in this flight based on correlations DMA exhibited with other species linked to anthropogenic activity.
The case flight on 6 March 2020 is summarized in Fig. 6 and was conducted on a day without CAO conditions. Winds were predominantly westerly with continental air mass influence. Six cloud water samples were collected at aircraft altitudes ranging between 618 and 2060 m. DMA concentrations ranged from 0.52 to 12.72 ng m−3, with strong correlations when compared to NH4+, NO3−, and nss-SO42− (R2 ≥ 0.82), similar to the 14 February flight. This flight had a weaker correlation between DMA and nss-K+ (R2 = 0.40). The HSRL-2 bbp data show expectedly higher concentrations near the coast (Fig. 6a). It is noteworthy to highlight the HSRL-2 bbp coefficient data reproducibility for both flights (Fig. 5a and 6a) for the inbound (red markers) and outbound (black markers) flights.
3.4.2 Summertime flight with fire influence.
Research flight 28 on 26 August 2020 was unique in that the Falcon sampled a cloud with biomass burning influence supported by various datasets. Summertime is typically marked by lower cloud fraction over the northwest Atlantic compared to wintertime, which is evident in Fig. 7. Winds were generally westerly on this flight. While the HSRL-2 often detected smoke during ACTIVATE flights, it was not always the case that there was clear evidence of it mixing with boundary layer clouds sampled by the Falcon. Research flight 28 was separately investigated by Mardi et al.89 who showed the mixing of smoke plumes with boundary layer clouds. That study showed enhanced aerosol extinction coefficient (532 nm) in the marine boundary layer and even higher values in the free troposphere (>2 km). Furthermore, flight scientist reports pointed to biomass burning influence, including support from in situ measurements of CO and AMS organic concentrations.113,114 Organic and CO concentrations along the flight track are clearly above the respective summer median values, including the time during the cloud water collection (Fig. 7a and b). NAAPS data for smoke and sulfate (Fig. 7c) and HYSPLIT 72 hour back trajectory data strongly suggest that the sampled smoke was influenced in part by air transported from western North America where there were active wildfires (Fig. 7d).
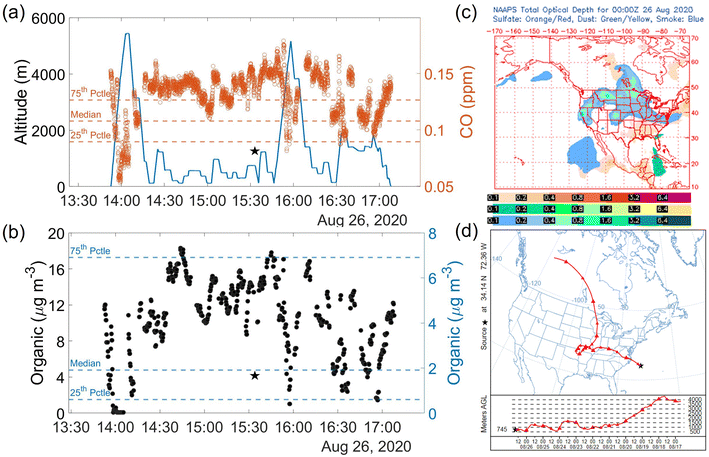 |
| Fig. 7 (a) Time series for in situ measurements of CO and flight altitude over the ACTIVATE region during RF28 (26 August 2020). (b) Time series for in situ AMS organic concentration in μg m−3. Dashed lines show the median, 25th percentile (pctle), and 75th pctle for the all the summer data. (c) Prognostic NAAPS map of smoke aerosol distribution. (d) HYSPLIT 240 hour back trajectories corresponding to the cloud water sample collected during RF28. The black star in (a and b) shows the collection time of the cloud water sample. | |
The concentration of DMA in this single sample was 0.38 ng m−3, slightly above the median in the summer of 0.25 ng m−3. Regarding other chemical measurements in cloud water, this sample's oxalate to sulfate ratio was the highest of the 2020 flights (0.24). Recent work has attributed such high ratios to biomass burning influence.115 Oxalate's concentration in this single sample was 130.76 ng m−3 in contrast to the summer median value of 92.77 ng m−3, indicating that it was higher than normal.
In contrast, we also examined the other two flights in the summer with detectable cloud water DMA levels (13 and 17 August), and none of them showed any evidence of smoke. A previous study found that amines (C1–C6) are detected during biomass burning plume events, suggesting that biomass burning can be a considerable source of amines in the southeastern U.S.36 As noted already, cloud water DMA levels were significantly higher during biomass burning conditions over the northeast Pacific.32
4. Conclusions
A unique airborne cloud water dataset is analyzed in this work with a focus on dimethylamine (DMA), which is a common alkyl amine observed in atmospheric gases, particles, and cloud water. This study adds important DMA data to a very limited archive of such data in cloud water based on airborne measurements, with the only other such studies to our knowledge being that of the NiCE dataset from the northeast Pacific and CAMP2Ex from southeast Asia.48,49
Notable results in this work are that concentrations of this species are markedly lower over the northwest Atlantic in ACTIVATE compared to the northeast Pacific in NiCE. One possible explanation is more ocean biological emissions in the latter region. When comparing ACTIVATE winter and summer flights, there were more relative samples with detectable DMA in the winter (67% of winter non-CAO samples; 44% of winter CAO samples) as compared to the summer (14%). The concentration trend of NO3−, NH4+, and nss-SO42− were uniquely similar to DMA among species examined in that their levels were highest in winter CAO conditions, followed by winter non-CAO conditions, and lastly summer. Analysis of other seasonally-dependent factors suggests that winter CAO levels are highest due to a presumed combination of low temperatures and offshore flow with anthropogenic pollutants, including biomass burning. This work adds to the growing evidence of how biomass burning is an important source of alkyl amines, such as DMA. This dataset motivates continued research into the partitioning behavior of amines between the gas, solid, and aqueous phases, in addition to examining the effect of cloud water acidity on partitioning. The winter samples in ACTIVATE were more acidic than summer, and it is uncertain how influential this was in promoting higher DMA levels.
As amines have been linked to new particle formation116–118 it is important to note that winter CAO conditions (lower temperature and humidity) were most conducive to such events during ACTIVATE,53 especially near clouds, with low temperatures and RH shown to be potentially contributing factors. Amines associated with nucleated particles can grow into sizes sufficiently large to activate into drops and thus be sampled as cloud water. Although outside the scope of this study, future research is encouraged to assess if these nucleation events identified around clouds in the northwest Atlantic are contributing appreciably to DMA solute concentrations in cloud water, which would have implications for other species participating in those nucleation events. Studying amines is important as they play an important role in the marine environment's carbon and nitrogen cycles.
Data availability
ACTIVATE airborne data can be accessed from http://doi.org/10.5067/ASDC/ACTIVATE_Aerosol_AircraftInSitu_Falcon_Data_1 and http://doi.org/10.5067/ASDC/ACTIVATE_AerosolCloud_AircraftRemoteSensing_KingAir_Data_1. ACTIVATE data can be accessed by registering for an Earthdata account/login: https://urs.earthdata.nasa.gov/. MONARC data can be accessed at: https://doi.org/10.6084/m9.figshare.5099983.v10.
Author contributions
AFC and AS conceived the study and drafted the manuscript. YC, BLC, EC, HD, JPD, GSD, MF, SK, RHM, JBN, MAS, CTS, TS, KLT, CV and LDZ contributed to data collection and archival from ACTIVATE. All authors contributed to editing the final version of the manuscript.
Conflicts of interest
The authors declare no conflict of interest.
Acknowledgements
This work was supported by NASA grant 80NSSC19K0442 in support of ACTIVATE, a NASA Earth Venture Suborbital-3 (EVS-3) investigation funded by NASA's Earth Science Division and managed through the Earth System Science Pathfinder Program Office. The CIRPAS Twin Otter measurements were funded by Office of Naval Research grant N00014-10-1-0811. C. V. and S. K. acknowledge funding by the DFG (German Research Foundation)–TRR301-Project-ID 428312742 and SPP1294 HALO under contract VO1504/7-1. B. L. C was supported by an appointment to the NASA Postdoctoral Program at NASA Langley Research Center, administered by Oak Ridge Associated Universities under contract with NASA. The authors gratefully acknowledge the NOAA Air Resources Laboratory for the HYSPLIT transport and dispersion model and the READY website (https://www.ready.arl.noaa.gov). We acknowledge the NASA Ocean Biology Processing Group (OBPG) for supporting access to the satellite ocean color data used here (https://www.oceancolor.gsfc.nasa.gov/). We recognize the use of imagery from the NASA Worldview application (https://www.worldview.earthdata.nasa.gov/), part of the NASA Earth Observing System Data and Information System. We thank the pilots and aircraft maintenance personnel of NASA Langley Research Services Directorate for their work in conducting the ACTIVATE flights.
References
- K. B. Benedict, T. Lee and J. L. Collett Jr, Cloud water composition over the southeastern Pacific Ocean during the VOCALS regional experiment, Atmos. Environ., 2012, 46, 104–114 CrossRef CAS.
- J. L. Collett Jr, A. Bator, D. E. Sherman, K. F. Moore, K. J. Hoag, B. B. Demoz, X. Rao and J. E. Reilly, The chemical composition of fogs and intercepted clouds in the United States, Atmos. Res., 2002, 64, 29–40 CrossRef.
- D. J. Straub, T. Lee and J. L. Collett Jr, Chemical composition of marine stratocumulus clouds over the eastern Pacific Ocean, J. Geophys. Res.: Atmos., 2007, 112, D04307 CrossRef.
- Z. Wang, A. Sorooshian, G. Prabhakar, M. M. Coggon and H. H. Jonsson, Impact of emissions from shipping, land, and the ocean on stratocumulus cloud water elemental composition during the 2011 E-PEACE field campaign, Atmos. Environ., 2014, 89, 570–580 CrossRef CAS.
- J. L. Collett Jr, A. Bator, X. Rao and B. B. Demoz, Acidity variations across the cloud drop size spectrum and their influence on rates of atmospheric sulfate production, Geophys. Res. Lett., 1994, 21, 2393–2396 CrossRef.
- J. D. Blando and B. J. Turpin, Secondary organic aerosol formation in cloud and fog droplets: a literature evaluation of plausibility, Atmos. Environ., 2000, 34, 1623–1632 CrossRef CAS.
- B. Ervens, B. Turpin and R. Weber, Secondary organic aerosol formation in cloud droplets and aqueous particles (aqSOA): a review of laboratory, field and model studies, Atmos. Chem. Phys., 2011, 11, 11069–11102 CrossRef CAS.
- H. O. T. Pye, A. Nenes, B. Alexander, A. P. Ault, M. C. Barth, S. L. Clegg, J. L. Collett Jr, K. M. Fahey, C. J. Hennigan, H. Herrmann, M. Kanakidou, J. T. Kelly, I. T. Ku, V. F. McNeill, N. Riemer, T. Schaefer, G. Shi, A. Tilgner, J. T. Walker, T. Wang, R. Weber, J. Xing, R. A. Zaveri and A. Zuend, The Acidity of Atmospheric Particles and Clouds, Atmos. Chem. Phys., 2020, 20, 4809–4888 CrossRef CAS PubMed.
- J. N. Galloway, F. J. Dentener, D. G. Capone, E. W. Boyer, R. W. Howarth, S. P. Seitzinger, G. P. Asner, C. C. Cleveland, P. Green and E. A. Holland, Nitrogen cycles: past, present, and future, Biogeochemistry, 2004, 70, 153–226 CrossRef CAS.
- K. G. McGregor and C. Anastasio, Chemistry of fog waters in California's Central Valley: 2. Photochemical transformations of amino acids and alkyl amines, Atmos. Environ., 2001, 35, 1091–1104 CrossRef CAS.
- X. Ge, A. S. Wexler and S. L. Clegg, Atmospheric amines – Part I. A review, Atmos. Environ., 2011, 45, 524–546 CrossRef CAS.
- L. Yao, O. Garmash, F. Bianchi, J. Zheng, C. Yan, J. Kontkanen, H. Junninen, S. B. Mazon, M. Ehn, P. Paasonen, M. Sipila, M. Wang, X. Wang, S. Xiao, H. Chen, Y. Lu, B. Zhang, D. Wang, Q. Fu, F. Geng, L. Li, H. Wang, L. Qiao, X. Yang, J. Chen, V. M. Kerminen, T. Petaja, D. R. Worsnop, M. Kulmala and L. Wang, Atmospheric new particle formation from sulfuric acid and amines in a Chinese megacity, Science, 2018, 361, 278–281 CrossRef CAS PubMed.
- A. Kürten, A. Bergen, M. Heinritzi, M. Leiminger, V. Lorenz, F. Piel, M. Simon, R. Sitals, A. C. Wagner and J. Curtius, Observation of new particle formation and measurement of sulfuric acid, ammonia, amines and highly oxidized organic molecules at a rural site in central Germany, Atmos. Chem. Phys., 2016, 16, 12793–12813 CrossRef.
- J. Almeida, S. Schobesberger, A. Kurten, I. K. Ortega, O. Kupiainen-Maatta, A. P. Praplan, A. Adamov, A. Amorim, F. Bianchi, M. Breitenlechner, A. David, J. Dommen, N. M. Donahue, A. Downard, E. Dunne, J. Duplissy, S. Ehrhart, R. C. Flagan, A. Franchin, R. Guida, J. Hakala, A. Hansel, M. Heinritzi, H. Henschel, T. Jokinen, H. Junninen, M. Kajos, J. Kangasluoma, H. Keskinen, A. Kupc, T. Kurten, A. N. Kvashin, A. Laaksonen, K. Lehtipalo, M. Leiminger, J. Leppa, V. Loukonen, V. Makhmutov, S. Mathot, M. J. McGrath, T. Nieminen, T. Olenius, A. Onnela, T. Petaja, F. Riccobono, I. Riipinen, M. Rissanen, L. Rondo, T. Ruuskanen, F. D. Santos, N. Sarnela, S. Schallhart, R. Schnitzhofer, J. H. Seinfeld, M. Simon, M. Sipila, Y. Stozhkov, F. Stratmann, A. Tome, J. Trostl, G. Tsagkogeorgas, P. Vaattovaara, Y. Viisanen, A. Virtanen, A. Vrtala, P. E. Wagner, E. Weingartner, H. Wex, C. Williamson, D. Wimmer, P. Ye, T. Yli-Juuti, K. S. Carslaw, M. Kulmala, J. Curtius, U. Baltensperger, D. R. Worsnop, H. Vehkamaki and J. Kirkby, Molecular understanding of sulphuric acid-amine particle nucleation in the atmosphere, Nature, 2013, 502, 359–363 CrossRef CAS PubMed.
- J. N. Smith, M. Dunn, T. VanReken, K. Iida, M. R. Stolzenburg, P. H. McMurry and L. Huey, Chemical composition of atmospheric nanoparticles formed from nucleation in Tecamac, Mexico: Evidence for an important role for organic species in nanoparticle growth, Geophys. Res. Lett., 2008, 35, D22S03 CrossRef.
- K. Barsanti, P. H. McMurry and J. Smith, The potential contribution of organic salts to new particle growth, Atmos. Chem. Phys., 2009, 9, 2949–2957 CrossRef CAS.
- S. Angelino, D. T. Suess and K. A. Prather, Formation of aerosol particles from reactions of secondary and tertiary alkylamines: characterization by aerosol time-of-flight mass spectrometry, Environ. Sci. Technol., 2001, 35, 3130–3138 CrossRef CAS PubMed.
- M. C. Facchini, S. Decesari, M. Rinaldi, C. Carbone, E. Finessi, M. Mircea, S. Fuzzi, F. Moretti, E. Tagliavini, D. Ceburnis and C. D. O'Dowd, Important source of marine secondary organic aerosol from biogenic amines, Environ. Sci. Technol., 2008, 42, 9116–9121 CrossRef CAS PubMed.
- S. Murphy, A. Sorooshian, J. Kroll, N. Ng, P. Chhabra, C. Tong, J. Surratt, E. Knipping, R. Flagan and J. Seinfeld, Secondary aerosol formation from atmospheric reactions of aliphatic amines, Atmos. Chem. Phys., 2007, 7, 2313–2337 CrossRef CAS.
- A. Sorooshian, S. Murphy, S. Hersey, H. Gates, L. Padro, A. Nenes, F. Brechtel, H. Jonsson, R. Flagan and J. Seinfeld, Comprehensive airborne characterization of aerosol from a major bovine source, Atmos. Chem. Phys., 2008, 8, 5489–5520 CrossRef CAS.
- C. Qiu and R. Zhang, Physiochemical properties of alkylaminium sulfates: hygroscopicity, thermostability, and density, Environ. Sci. Technol., 2012, 46, 4474–4480 CrossRef CAS PubMed.
- M. Gomez-Hernandez, M. McKeown, J. Secrest, W. Marrero-Ortiz, A. Lavi, Y. Rudich, D. R. Collins and R. Zhang, Hygroscopic Characteristics of Alkylaminium Carboxylate Aerosols, Environ. Sci. Technol., 2016, 50, 2292–2300 CrossRef CAS PubMed.
- Y. Chu, M. Sauerwein and C. K. Chan, Hygroscopic and phase transition properties of alkyl aminium sulfates at low relative humidities, Phys. Chem. Chem. Phys., 2015, 17, 19789–19796 RSC.
- H. Greim, D. Bury, H. J. Klimisch, M. Oeben-Negele and K. Ziegler-Skylakakis, Toxicity of aliphatic amines: structure-activity relationship, Chemosphere, 1998, 36, 271–295 CrossRef CAS PubMed.
- D. Lee and A. S. Wexler, Atmospheric amines – Part III: Photochemistry and toxicity, Atmos. Environ., 2013, 71, 95–103 CrossRef CAS.
- B. Bzdek, D. Ridge and M. Johnston, Amine exchange into ammonium bisulfate and ammonium nitrate nuclei, Atmos. Chem. Phys., 2010, 10, 3495–3503 CrossRef CAS.
- C. Qiu, L. Wang, V. Lal, A. F. Khalizov and R. Zhang, Heterogeneous reactions of alkylamines with ammonium sulfate and ammonium bisulfate, Environ. Sci. Technol., 2011, 45, 4748–4755 CrossRef CAS PubMed.
- X. Ge, A. S. Wexler and S. L. Clegg, Atmospheric amines – Part II. Thermodynamic properties and gas/particle partitioning, Atmos. Environ., 2011, 45, 561–577 CrossRef CAS.
- K. J. Zarzana, D. O. De Haan, M. A. Freedman, C. A. Hasenkopf and M. A. Tolbert, Optical properties of the products of alpha-dicarbonyl and amine reactions in simulated cloud droplets, Environ. Sci. Technol., 2012, 46, 4845–4851 CrossRef CAS PubMed.
- K. A. Hill, P. B. Shepson, E. S. Galbavy, C. Anastasio, P. S. Kourtev, A. Konopka and B. H. Stirm, Processing of atmospheric nitrogen by clouds above a forest environment, J. Geophys. Res.: Atmos., 2007, 112, D11301 CrossRef.
- J. W. Hutchings, B. Ervens, D. Straub and P. Herckes, N-nitrosodimethylamine occurrence, formation and cycling in clouds and fogs, Environ. Sci. Technol., 2010, 44, 8128–8133 CrossRef CAS PubMed.
- J.-S. Youn, E. Crosbie, L. Maudlin, Z. Wang and A. Sorooshian, Dimethylamine as a major alkyl amine species in particles and cloud water: Observations in semi-arid and coastal regions, Atmos. Environ., 2015, 122, 250–258 CrossRef CAS PubMed.
- C. Müller, Y. Iinuma, J. Karstensen, D. van Pinxteren, S. Lehmann, T. Gnauk and H. Herrmann, Seasonal variation of aliphatic amines in marine sub-micrometer particles at the Cape Verde islands, Atmos. Chem. Phys., 2009, 9, 9587–9597 CrossRef.
- C. Qiu and R. Zhang, Multiphase chemistry of atmospheric amines, Phys. Chem. Chem. Phys., 2013, 15, 5738–5752 RSC.
- J. Zheng, Y. Ma, M. Chen, Q. Zhang, L. Wang, A. F. Khalizov, L. Yao, Z. Wang, X. Wang and L. Chen, Measurement of atmospheric amines and ammonia using the high resolution time-of-flight chemical ionization mass spectrometry, Atmos. Environ., 2015, 102, 249–259 CrossRef CAS.
- Y. You, V. Kanawade, J. De Gouw, A. B. Guenther, S. Madronich, M. Sierra-Hernández, M. Lawler, J. N. Smith, S. Takahama and G. Ruggeri, Atmospheric amines and ammonia measured with a chemical ionization mass spectrometer (CIMS), Atmos. Chem. Phys., 2014, 14, 12181–12194 CrossRef.
-
J. M. Lobert, D. H. Scharffe, H. Weimin, T. A. Kuhlbusch, R. Seuwen, P. Warneck and P. J. Crutzen, in Global Biomass Burning. Atmospheric, Climatic, and Biospheric Implications, 1991 Search PubMed.
- D. Yang, S. Zhu, Y. Ma, L. Zhou, F. Zheng, L. Wang, J. Jiang and J. Zheng, Emissions of Ammonia and Other Nitrogen-Containing Volatile Organic Compounds from Motor Vehicles under Low-Speed Driving Conditions, Environ. Sci. Technol., 2022, 56, 5440–5447 CrossRef CAS PubMed.
- N. M. Ngwabie, G. W. Schade, T. G. Custer, S. Linke and T. Hinz, Abundances and flux estimates of volatile organic compounds from a dairy cowshed in Germany, J. Environ. Qual., 2008, 37, 565–573 CrossRef CAS PubMed.
- G. W. Schade and P. J. Crutzen, Emission of aliphatic amines from animal husbandry and their reactions: Potential source of N2O and HCN, J. Atmos. Chem., 1995, 22, 319–346 CrossRef CAS.
-
O. Hertel, S. Reis, C. A. Skjoth, A. Bleeker, R. Harrison, J. N. Cape, D. Fowler, U. Skiba, D. Simpson and T. Jickells, in The European Nitrogen Assessment: Sources, Effects and Policy Perspectives, Cambridge University Press, 2011, pp. 177–207 Search PubMed.
- H. Hellén, A. J. Kieloaho and H. Hakola, Gas-phase alkyl amines in urban air; comparison with a boreal forest site and importance for local atmospheric chemistry, Atmos. Environ., 2014, 94, 192–197 CrossRef.
- P. J. Silva, M. E. Erupe, D. Price, J. Elias, Q. GJ Malloy, Q. Li, B. Warren and D. R. Cocker III, Trimethylamine as precursor to secondary organic aerosol formation via nitrate radical reaction in the atmosphere, Environ. Sci. Technol., 2008, 42, 4689–4696 CrossRef CAS PubMed.
- T. VandenBoer, M. Markovic, A. Petroff, M. Czar, N. Borduas and J. Murphy, Ion chromatographic separation and quantitation of alkyl methylamines and ethylamines in atmospheric gas and particulate matter using preconcentration and suppressed conductivity detection, J. Chromatogr. A, 2012, 1252, 74–83 CrossRef CAS PubMed.
- T. VandenBoer, A. Petroff, M. Markovic and J. Murphy, Size distribution of alkyl amines in continental particulate matter and their online detection in the gas and particle phase, Atmos. Chem. Phys., 2011, 11, 4319–4332 CrossRef CAS.
- K. A. Pratt, L. E. Hatch and K. A. Prather, Seasonal volatility dependence of ambient particle phase amines, Environ. Sci. Technol., 2009, 43, 5276–5281 CrossRef CAS PubMed.
- B. J. Williams, A. H. Goldstein, N. M. Kreisberg, S. V. Hering, D. R. Worsnop, I. M. Ulbrich, K. S. Docherty and J. L. Jimenez, Major components of atmospheric organic aerosol in southern California as determined by hourly measurements of source marker compounds, Atmos. Chem. Phys., 2010, 10, 11577–11603 CrossRef CAS.
- C. Stahl, E. Crosbie, P. A. Bañaga, G. Betito, R. A. Braun, Z. M. Cainglet, M. O. Cambaliza, M. T. Cruz, J. M. Dado and M. R. A. Hilario, Total organic carbon and the contribution from speciated organics in cloud water: airborne data analysis from the CAMP 2 Ex field campaign, Atmos. Chem. Phys., 2021, 21, 14109–14129 CrossRef CAS.
- E. Crosbie, L. D. Ziemba, M. A. Shook, C. E. Robinson, E. L. Winstead, K. L. Thornhill, R. A. Braun, A. B. MacDonald, C. Stahl, A. Sorooshian, S. C. van den Heever, J. P. DiGangi, G. S. Diskin, S. Woods, P. Bañaga, M. D. Brown, F. Gallo, M. R. A. Hilario, C. E. Jordan, G. R. Leung, R. H. Moore, K. J. Sanchez, T. J. Shingler and E. B. Wiggins, Closure analysis of aerosol-cloud
composition in tropical maritime warm convection, EGUsphere, 2022, 2022, 1–55 Search PubMed.
- A. Roth, J. Schneider, T. Klimach, S. Mertes, D. Van Pinxteren, H. Herrmann and S. Borrmann, Aerosol properties, source identification, and cloud processing in orographic clouds measured by single particle mass spectrometry on a central European mountain site during HCCT-2010, Atmos. Chem. Phys., 2016, 16, 505–524 CrossRef CAS.
- Q. Lin, G. Zhang, L. Peng, X. Bi, X. Wang, F. J. Brechtel, M. Li, D. Chen, P. a. Peng and G. Sheng, In situ chemical composition measurement of individual cloud residue particles at a mountain site, southern China, Atmos. Chem. Phys., 2017, 17, 8473–8488 CrossRef CAS.
- A. Sorooshian, B. Anderson, S. E. Bauer, R. A. Braun, B. Cairns, E. Crosbie, H. Dadashazar, G. Diskin, R. Ferrare and R. C. Flagan, Aerosol–cloud–meteorology interaction airborne field investigations: Using lessons learned from the US West Coast in the design of ACTIVATE off the US East Coast, Bull. Am. Meteorol. Soc., 2019, 100, 1511–1528 Search PubMed.
- A. F. Corral, Y. Choi, E. Crosbie, H. Dadashazar, J. P. DiGangi, G. S. Diskin, M. Fenn, D. B. Harper, S. Kirschler, H. Liu, R. H. Moore, J. B. Nowak, A. J. Scarino, S. Seaman, T. Shingler, M. A. Shook, K. L. Thornhill, C. Voigt, B. Zhang, L. D. Ziemba and A. Sorooshian, Cold Air Outbreaks Promote New Particle Formation Off the U.S. East Coast, Geophys. Res. Lett., 2022, 49, e2021GL096073 CrossRef.
- F. Tornow, A. S. Ackerman, A. M. Fridlind, B. Cairns, E. C. Crosbie, S. Kirschler, R. H. Moore, D. Painemal, C. E. Robinson, C. Seethala, M. A. Shook, C. Voigt, E. L. Winstead, L. D. Ziemba, P. Zuidema and A. Sorooshian, Dilution of Boundary Layer Cloud Condensation Nucleus Concentrations by Free Tropospheric Entrainment During Marine Cold Air Outbreaks, Geophys. Res. Lett., 2022, 49, e2022GL098444 CrossRef CAS.
- X.-Y. Li, H. Wang, J. Chen, S. Endo, G. George, B. Cairns, S. Chellappan, X. Zeng, S. Kirschler and C. Voigt, Large-Eddy Simulations of Marine Boundary Layer Clouds Associated with Cold-Air Outbreaks during the ACTIVATE Campaign. Part I: Case Setup and Sensitivities to Large-Scale Forcings, J. Atmos. Sci., 2022, 79, 73–100 Search PubMed.
- A. Sorooshian, A. F. Corral, R. A. Braun, B. Cairns, E. Crosbie, R. Ferrare, J. Hair, M. M. Kleb, A. H. Mardi, H. Maring, A. McComiskey, R. Moore, D. Painemal, A. Jo Scarino, J. Schlosser, T. Shingler, M. Shook, H. Wang, X. Zeng, L. Ziemba and P. Zuidema, Atmospheric Research Over the Western North Atlantic Ocean Region and North American East Coast: A Review of Past Work and Challenges Ahead, J Geophys Res Atmos, 2020, 125, e2019JD031626 Search PubMed.
- C. Seethala, P. Zuidema, J. Edson, M. Brunke, G. Chen, X. Y. Li, D. Painemal, C. Robinson, T. Shingler, M. Shook, A. Sorooshian, L. Thornhill, F. Tornow, H. Wang, X. Zeng and L. Ziemba, On Assessing ERA5 and MERRA2 Representations of Cold-Air Outbreaks Across the Gulf Stream, Geophys. Res. Lett., 2021, 48, e2021GL094364 CrossRef PubMed.
- H. Dadashazar, E. Crosbie, Y. Choi, A. F. Corral, J. P. DiGangi, G. S. Diskin, S. Dmitrovic, S. Kirschler, K. McCauley, R. H. Moore, J. B. Nowak, C. E. Robinson, J. Schlosser, M. Shook, K. L. Thornhill, C. Voigt, E. L. Winstead, L. D. Ziemba and A. Sorooshian, Analysis of MONARC and ACTIVATE Airborne Aerosol Data for Aerosol-Cloud Interaction Investigations: Efficacy of Stairstepping Flight Legs for Airborne In Situ Sampling, Atmosphere, 2022, 13, 1242 CrossRef CAS.
- E. Crosbie, M. D. Brown, M. Shook, L. Ziemba, R. H. Moore, T. Shingler, E. Winstead, K. Lee Thornhill, C. Robinson, A. B. MacDonald, H. Dadashazar, A. Sorooshian, A. Beyersdorf, A. Eugene, J. Collett Jr, D. Straub and B. Anderson, Development and characterization of a high-efficiency, aircraft-based axial cyclone cloud water collector, Atmos. Meas. Tech., 2018, 11, 5025–5048 CrossRef CAS PubMed.
- M. AzadiAghdam, R. A. Braun, E.-L. Edwards, P. A. Bañaga, M. T. Cruz, G. Betito, M. O. Cambaliza, H. Dadashazar, G. R. Lorenzo, L. Ma, A. B. MacDonald, P. Nguyen, J. B. Simpas, C. Stahl and A. Sorooshian, On the nature of sea salt aerosol at a coastal megacity: Insights from Manila, Philippines in Southeast Asia, Atmos. Environ., 2019, 216, 116922 CrossRef CAS.
- M. E. Gonzalez, A. F. Corral, E. Crosbie, H. Dadashazar, G. S. Diskin, E.-L. Edwards, S. Kirschler, R. H. Moore, C. E. Robinson, J. S. Schlosser, M. Shook, C. Stahl, K. L. Thornhill, C. Voigt, E. Winstead, L. D. Ziemba and A. Sorooshian, Relationships between supermicrometer particle concentrations and cloud water sea salt and dust concentrations: analysis of MONARC and ACTIVATE data, Environ. Sci.: Atmos., 2022, 738–752 CAS.
-
J. H. P. S. N. Seinfeld, Atmospheric Chemistry and Physics : from Air Pollution to Climate Change, 2016 Search PubMed.
- I. Knop, S. E. Bansmer, V. Hahn and C. Voigt, Comparison of different droplet measurement techniques in the Braunschweig Icing Wind Tunnel, Atmos. Meas. Tech., 2021, 14, 1761–1781 CrossRef.
- S. Kirschler, C. Voigt, B. Anderson, R. Campos Braga, G. Chen, A. F. Corral, E. Crosbie, H. Dadashazar, R. F. Ferrare and V. Hahn, Seasonal updraft speeds change cloud droplet number concentrations in low level clouds over the Western North Atlantic, Atmospheric Chemistry and Physics Discussions, 2022, 1–32 Search PubMed.
-
G. S. Diskin, J. R. Podolske, G. W. Sachse and T. A. Slate, Open-path airborne tunable diode laser hygrometer, in Diode lasers and applications in atmospheric sensing, SPIE, 2002, vol. 4817, pp. 196–204 Search PubMed.
- J. P. DiGangi, Y. Choi, J. B. Nowak, H. S. Halliday, G. S. Diskin, S. Feng, Z. R. Barkley, T. Lauvaux, S. Pal and K. J. Davis, Seasonal Variability in Local Carbon Dioxide Combustion Sources over the Central and Eastern US using Airborne In-Situ Enhancement Ratios, Earth and Space Science Open Archive, 2021, 2–17 Search PubMed.
- P. DeCarlo, E. Dunlea, J. Kimmel, A. Aiken, D. Sueper, J. Crounse, P. Wennberg, L. Emmons, Y. Shinozuka and A. Clarke, Fast airborne aerosol size and chemistry measurements above Mexico City and Central Mexico during the MILAGRO campaign, Atmos. Chem. Phys., 2008, 8, 4027–4048 CrossRef CAS.
- G. Rolph, A. Stein and B. Stunder, Real-time Environmental Applications and Display sYstem: READY, Environ. Model. Softw., 2017, 210–228 CrossRef.
- A. Stein, R. R. Draxler, G. D. Rolph, B. J. Stunder, M. Cohen and F. Ngan, NOAA's HYSPLIT atmospheric transport and dispersion modeling system, Bull. Am. Meteorol. Soc., 2015, 96, 2059–2077 CrossRef.
- P. Lynch, J. S. Reid, D. L. Westphal, J. Zhang, T. F. Hogan, E. J. Hyer, C. A. Curtis, D. A. Hegg, Y. Shi and J. R. Campbell, An 11-year global gridded aerosol optical thickness reanalysis (v1. 0) for atmospheric and climate sciences, Geosci. Model Dev., 2016, 9, 1489–1522 CrossRef CAS.
-
NRL, Naval Research Laboratory Navy Aerosol Analysis and Prediction System, 2022, https://www.nrlmry.navy.mil/aerosol/ Search PubMed.
- S. Burton, C. Hostetler, A. Cook, J. Hair, S. Seaman, S. Scola, D. Harper, J. Smith, M. Fenn and R. A. Ferrare, Calibration of a high spectral resolution lidar using a Michelson interferometer, with data examples from ORACLES, Appl. Opt., 2018, 57, 6061–6075 CrossRef CAS PubMed.
- J. E. O'Reilly, S. Maritorena, B. G. Mitchell, D. A. Siegel, K. L. Carder, S. A. Garver, M. Kahru and C. McClain, Ocean color chlorophyll algorithms for SeaWiFS, J. Geophys. Res.: Oceans, 1998, 103, 24937–24953 CrossRef.
- D. Stramski, E. Boss, D. Bogucki and K. J. Voss, The role of seawater constituents in light backscattering in the ocean, Prog. Oceanogr., 2004, 61, 27–56 CrossRef.
- J. A. Schulien, M. J. Behrenfeld, J. W. Hair, C. A. Hostetler and M. S. Twardowski, Vertically- resolved phytoplankton carbon and net primary production from a high spectral resolution lidar, Opt. Express, 2017, 25, 13577–13587 CrossRef CAS PubMed.
- P. K. Quinn, T. S. Bates, K. S. Schulz, D. Coffman, A. Frossard, L. Russell, W. Keene and D. Kieber, Contribution of sea surface carbon pool to organic matter enrichment in sea spray aerosol, Nat. Geosci., 2014, 7, 228–232 CrossRef CAS.
- M. Rinaldi, S. Fuzzi, S. Decesari, S. Marullo, R. Santoleri, A. Provenzale, J. von Hardenberg, D. Ceburnis, A. Vaishya and C. D. O'Dowd, Is chlorophyll-a the best surrogate for organic matter enrichment in submicron primary marine aerosol?, J. Geophys. Res.: Atmos., 2013, 118, 4964–4973 CrossRef CAS.
- A. Sorooshian, L. T. Padró, A. Nenes, G. Feingold, A. McComiskey, S. P. Hersey, H. Gates, H. H. Jonsson, S. D. Miller and G. L. Stephens, On the link between ocean biota emissions, aerosol, and maritime clouds: Airborne, ground, and satellite measurements off the coast of California, Global Biogeochem. Cycles, 2009, 23, GB4007 CrossRef.
- A. Sorooshian, E. Crosbie, L. C. Maudlin, J. S. Youn, Z. Wang, T. Shingler, A. M. Ortega, S. Hersey and R. K. Woods, Surface and Airborne Measurements of Organosulfur and Methanesulfonate Over the Western United States and Coastal Areas, J. Geophys. Res.: Atmos., 2015, 120, 8535–8548 CrossRef CAS PubMed.
- M. M. Coggon, A. Sorooshian, Z. Wang, J. S. Craven, A. R. Metcalf, J. J. Lin, A. Nenes, H. H. Jonsson, R. C. Flagan and J. H. Seinfeld, Observations of continental biogenic impacts on marine aerosol and clouds off the coast of California, J. Geophys. Res.: Atmos., 2014, 119, 6724–6748 CrossRef CAS.
- G. Prabhakar, B. Ervens, Z. Wang, L. Maudlin, M. Coggon, H. Jonsson, J. Seinfeld and A. Sorooshian, Sources of nitrate in stratocumulus cloud water: Airborne measurements during the 2011 E-PEACE and 2013 NiCE studies, Atmos.
Environ., 2014, 97, 166–173 CrossRef CAS.
- A. Sorooshian, A. B. MacDonald, H. Dadashazar, K. H. Bates, M. M. Coggon, J. S. Craven, E. Crosbie, S. P. Hersey, N. Hodas, J. J. Lin, A. Negron Marty, L. C. Maudlin, A. R. Metcalf, S. M. Murphy, L. T. Padro, G. Prabhakar, T. A. Rissman, T. Shingler, V. Varutbangkul, Z. Wang, R. K. Woods, P. Y. Chuang, A. Nenes, H. H. Jonsson, R. C. Flagan and J. H. Seinfeld, A multi-year data set on aerosol-cloud-precipitation-meteorology interactions for marine stratocumulus clouds, Sci. Data, 2018, 5, 180026 CrossRef PubMed.
-
D. A. Hegg and P. V. Hobbs, Studies of the Mechanisms and Rates with Which Nitrogen Species Are Incorporated, 1987 Search PubMed.
- S.-H. Lee, Perspective on the Recent Measurements of Reduced Nitrogen Compounds in the Atmosphere, Front. Environ. Sci., 2022, 328 Search PubMed.
- P. J. Rehbein, C. H. Jeong, M. L. McGuire, X. Yao, J. C. Corbin and G. J. Evans, Cloud and fog processing enhanced gas-to-particle partitioning of trimethylamine, Environ. Sci. Technol., 2011, 45, 4346–4352 CrossRef CAS PubMed.
- J. S. Youn, Z. Wang, A. Wonaschutz, A. Arellano, E. A. Betterton and A. Sorooshian, Evidence of aqueous secondary organic aerosol formation from biogenic emissions in the North American Sonoran Desert, Geophys. Res. Lett., 2013, 40, 3468–3472 CrossRef CAS PubMed.
- N. Balasus, M. A. Battaglia Jr, K. Ball, V. Caicedo, R. Delgado, A. G. Carlton and C. J. Hennigan, Urban aerosol chemistry at a land–water transition site during summer–Part 1: Impact of agricultural and industrial ammonia emissions, Atmos. Chem. Phys., 2021, 21, 13051–13065 CrossRef CAS.
- A. Sullivan, H. Guo, J. Schroder, P. Campuzano-Jost, J. Jimenez, T. Campos, V. Shah, L. Jaeglé, B. Lee and F. Lopez-Hilfiker, Biomass burning markers and residential burning in the WINTER aircraft campaign, J. Geophys. Res.: Atmos., 2019, 124, 1846–1861 CrossRef CAS.
- A. H. Mardi, H. Dadashazar, D. Painemal, T. Shingler, S. T. Seaman, M. A. Fenn, C. A. Hostetler and A. Sorooshian, Biomass Burning Over the United States East Coast and Western North Atlantic Ocean: Implications for Clouds and Air Quality, J. Geophys. Res.: Atmos., 2021, 126, e2021JD034916 Search PubMed.
- A. F. Corral, R. A. Braun, B. Cairns, V. A. Gorooh, H. Liu, L. Ma, A. H. Mardi, D. Painemal, S. Stamnes, B. van Diedenhoven, H. Wang, Y. Yang, B. Zhang and A. Sorooshian, An Overview of Atmospheric Features Over the Western North Atlantic Ocean and North American East Coast - Part 1: Analysis of Aerosols, Gases, and Wet Deposition Chemistry, J. Geophys. Res.: Atmos., 2021, 126, e2020JD032592 Search PubMed.
- D. Painemal, A. F. Corral, A. Sorooshian, M. A. Brunke, S. Chellappan, V. Afzali Gorooh, S. H. Ham, L. O'Neill, W. L. Smith Jr and G. Tselioudis, An overview of atmospheric features over the Western North Atlantic Ocean and North American East Coast—Part 2: Circulation, boundary layer, and clouds, J. Geophys. Res.: Atmos., 2021, 126, e2020JD033423 Search PubMed.
- A. F. Corral, H. Dadashazar, C. Stahl, E.-L. Edwards, P. Zuidema and A. Sorooshian, Source Apportionment of Aerosol at a Coastal Site and Relationships with Precipitation Chemistry: A Case Study over the Southeast United States, Atmosphere, 2020, 11, 1212 CrossRef CAS PubMed.
- E. L. Edwards, A. F. Corral, H. Dadashazar, A. E. Barkley, C. J. Gaston, P. Zuidema and A. Sorooshian, Impact of various air mass types on cloud condensation nuclei concentrations along coastal southeast Florida, Atmos. Environ., 2021, 254, 118371 CrossRef CAS PubMed.
- J. S. Le Blond, S. Woskie, C. J. Horwell and B. J. Williamson, Particulate matter produced during commercial sugarcane harvesting and processing: A respiratory health hazard?, Atmos. Environ., 2017, 149, 34–46 CrossRef CAS.
- O. Sevimoğlu and W. F. Rogge, Seasonal variations of PM10—Trace elements, PAHs and Levoglucosan: Rural sugarcane growing area versus coastal urban area in Southeastern Florida, USA. Part II: Elemental concentrations, Particuology, 2019, 46, 99–108 CrossRef.
- H. Dadashazar, M. Alipanah, M. R. A. Hilario, E. Crosbie, S. Kirschler, H. Liu, R. H. Moore, A. J. Peters, A. J. Scarino, M. Shook, K. L. Thornhill, C. Voigt, H. Wang, E. Winstead, B. Zhang, L. Ziemba and A. Sorooshian, Aerosol responses to precipitation along North American air trajectories arriving at Bermuda, Atmos. Chem. Phys., 2021, 21, 16121–16141 CrossRef CAS PubMed.
- F. Tornow, A. S. Ackerman and A. M. Fridlind, Preconditioning of overcast-to-broken cloud transitions by riming in marine cold air outbreaks, Atmos. Chem. Phys., 2021, 21, 12049–12067 CrossRef CAS.
- Y. Miyazaki, K. Kawamura and M. Sawano, Size distributions of organic nitrogen and carbon in remote marine aerosols: Evidence of marine biological origin based on their isotopic ratios, Geophys. Res. Lett., 2010, 37, L06803 CrossRef.
-
R. W. Cooksey, in Illustrating Statistical Procedures: Finding Meaning in Quantitative Data, Springer, 2020, pp. 141–239 Search PubMed.
- R. Arimoto, R. Duce, D. Savoie and J. Prospero, Trace elements in aerosol particles from Bermuda and Barbados: Concentrations, sources and relationships to aerosol sulfate, J. Atmos. Chem., 1992, 14, 439–457 CrossRef CAS.
- L. Ma, H. Dadashazar, M. R. A. Hilario, M. O. Cambaliza, G. R. Lorenzo, J. B. Simpas, P. Nguyen and A. Sorooshian, Contrasting wet deposition composition between three diverse islands and coastal North American sites, Atmos. Environ., 2021, 244, 117919 CrossRef CAS PubMed.
- J. S. Reid, P. V. Hobbs, R. J. Ferek, D. R. Blake, J. V. Martins, M. R. Dunlap and C. Liousse, Physical, chemical, and optical properties of regional hazes dominated by smoke in Brazil, J. Geophys. Res.: Atmos., 1998, 103, 32059–32080 CrossRef CAS.
- M. Singh, P. A. Jaques and C. Sioutas, Size distribution and diurnal characteristics of particle-bound metals in source and receptor sites of the Los Angeles Basin, Atmos. Environ., 2002, 36, 1675–1689 CrossRef CAS.
- C. Stahl, M. T. Cruz, P. A. Bañaga, G. Betito, R. A. Braun, M. A. Aghdam, M. O. Cambaliza, G. R. Lorenzo, A. B. MacDonald and M. R. A. Hilario, Sources and characteristics of size-resolved particulate organic acids and methanesulfonate in a coastal megacity: Manila, Philippines, Atmos. Chem. Phys., 2020, 20, 15907–15935 CrossRef CAS.
- S. M. Murphy, A. Sorooshian, J. H. Kroll, N. L. Ng, P. Chhabra, C. Tong, J. D. Surratt, E. Knipping, R. C. Flagan and J. H. Seinfeld, Secondary aerosol formation from atmospheric reactions of aliphatic amines, Atmos. Chem. Phys., 2007, 7, 2313–2337 CrossRef CAS.
- L. C. Maudlin, Z. Wang, H. H. Jonsson and A. Sorooshian, Impact of wildfires on size-resolved aerosol composition at a coastal California site, Atmos. Environ., 2015, 119, 59–68 CrossRef CAS.
- A. Lavi, E. Segre, M. Gomez-Hernandez, R. Zhang and Y. Rudich, Volatility of atmospherically relevant alkylaminium carboxylate salts, J. Phys. Chem. A, 2015, 119, 4336–4346 CrossRef CAS PubMed.
- J. S. Schlosser, R. A. Braun, T. Bradley, H. Dadashazar, A. B. MacDonald, A. A. Aldhaif, M. A. Aghdam, A. H. Mardi, P. Xian and A. Sorooshian, Analysis of aerosol composition data for western United States wildfires between 2005 and 2015: Dust emissions, chloride depletion, and most enhanced aerosol constituents, J. Geophys. Res.: Atmos., 2017, 122, 8951–8966 CrossRef CAS PubMed.
- T. Bates, B. Lamb, A. Guenther, J. Dignon and R. Stoiber, Sulfur emissions to the atmosphere from natural sourees, J. Atmos. Chem., 1992, 14, 315–337 CrossRef CAS.
- M. L. Dawson, M. E. Varner, V. Perraud, M. J. Ezell, R. B. Gerber and B. J. Finlayson-Pitts, Simplified mechanism for new particle formation from methanesulfonic acid, amines, and water via experiments and ab initio calculations, Proc. Natl. Acad. Sci., 2012, 109, 18719–18724 CrossRef CAS PubMed.
- D. Chen, X. Yao, C. K. Chan, X. Tian, Y. Chu, S. L. Clegg, Y. Shen, Y. Gao and H. Gao, Competitive uptake of dimethylamine and trimethylamine against ammonia on acidic particles in marine atmospheres, Environ. Sci. Technol., 2022, 56, 5430–5439 CrossRef CAS PubMed.
- M. Sauerwein and C. K. Chan, Heterogeneous uptake of ammonia and dimethylamine into sulfuric and oxalic acid particles, Atmos. Chem. Phys., 2017, 17, 6323–6339 CrossRef CAS.
- J. Chen, C. Li, Z. Ristovski, A. Milic, Y. Gu, M. S. Islam, S. Wang, J. Hao, H. Zhang, C. He, H. Guo, H. Fu, B. Miljevic, L. Morawska, P. Thai, Y. F. Lam, G. Pereira, A. Ding, X. Huang and U. C. Dumka, A review of biomass burning: Emissions and impacts on air quality, health and climate in China, Sci. Total Environ., 2017, 579, 1000–1034 CrossRef CAS PubMed.
- P. J. Crutzen, L. E. Heidt, J. P. Krasnec, W. H. Pollock and W. Seiler, Biomass burning as a source of atmospheric gases CO, H2, N2O, NO, CH3Cl and COS, Nature, 1979, 282, 253–256 CrossRef CAS.
- M. R. A. Hilario, E. Crosbie, P. A. Bañaga, G. Betito, R. A. Braun, M. O. Cambaliza, A. F. Corral, M. T. Cruz, J. E. Dibb and G. R. Lorenzo, Particulate Oxalate-To-Sulfate Ratio as an Aqueous Processing Marker: Similarity Across Field Campaigns and Limitations, Geophys. Res. Lett., 2021, 48, e2021GL096520 CrossRef CAS PubMed.
- T. Berndt, F. Stratmann, M. Sipilä, J. Vanhanen, T. Petäjä, J. Mikkilä, A. Grüner, G. Spindler, L. Mauldin III and J. Curtius, Laboratory study on new particle formation from the reaction OH+ SO2: influence of experimental conditions, H 2 O vapour, NH 3 and the amine tert-butylamine on the overall process, Atmos. Chem. Phys., 2010, 10, 7101–7116 CrossRef CAS.
- M. Erupe, A. Viggiano and S.-H. Lee, The effect of trimethylamine on atmospheric nucleation involving H2SO4, Atmos. Chem. Phys., 2011, 11, 4767–4775 CrossRef CAS.
- T. Kurten, V. Loukonen, H. Vehkamäki and M. Kulmala, Amines are likely to enhance neutral and ion-induced sulfuric acid-water nucleation in the atmosphere more effectively than ammonia, Atmos. Chem. Phys., 2008, 8, 4095–4103 CrossRef CAS.
|
This journal is © The Royal Society of Chemistry 2022 |
Click here to see how this site uses Cookies. View our privacy policy here.