Graphene quantum dots obstruct the membrane axis of Alzheimer's amyloid beta†
Received
17th September 2021
, Accepted 23rd November 2021
First published on 23rd November 2021
Abstract
Alzheimer's disease (AD) is a primary form of dementia with debilitating consequences, but no effective cure is available. While the pathophysiology of AD remains multifactorial, the aggregation of amyloid beta (Aβ) mediated by the cell membrane is known to be the cause for the neurodegeneration associated with AD. Here we examined the effects of graphene quantum dots (GQDs) on the obstruction of the membrane axis of Aβ in its three representative forms of monomers (Aβ-m), oligomers (Aβ-o), and amyloid fibrils (Aβ-f). Specifically, we determined the membrane fluidity of neuroblastoma SH-SY5Y cells perturbed by the Aβ species, especially by the most toxic Aβ-o, and demonstrated their recovery by GQDs using confocal fluorescence microscopy. Our computational data through discrete molecular dynamics simulations further revealed energetically favorable association of the Aβ species with the GQDs in overcoming peptide–peptide aggregation. Overall, this study positively implicated GQDs as an effective agent in breaking down the membrane axis of Aβ, thereby circumventing adverse downstream events and offering a potential therapeutic solution for AD.
1. Introduction
Alzheimer's disease (AD) is a primary form of neurodegeneration manifested by a loss of memory, impaired cognitive function, and shortened lifespan.1 While the pathophysiology of AD remains controversial, it is generally accepted that the aggregation of amyloid beta (Aβ), a kinetic process of nucleation, elongation and saturation,2–4 is the cause of neuroinflammation, tauopathies, neuronal death, and the progression of AD.5,6
AD is often considered to be a membrane disorder, for a number of reasons.7–9 First of all, Aβ is a peptide cleaved off the transmembrane amyloid precursor protein by β and γ secretases in neurons and synapses. As a result, Aβ is relatively hydrophobic and possesses an inherent affinity for the cell membrane. Since Aβ40 is the most abundant while Aβ42 the most amyloidogenic among the Aβ isoforms, their structure and dynamics have been extensively studied in the literature.10 Second of all, while the mechanism of Aβ toxicity remains inconclusive, it is widely acknowledged that the cell membrane, together with other environmental factors such as pH, free lipids and physiological metals, can catalyze the structural transitions of Aβ from disordered monomers to soluble oligomers that are rich in α-helices and antiparallel β-sheets, and to amyloid fibrils that possess a ubiquitous cross-β backbone.11–13 Interactions of the peptide aggregates with the cell membrane trigger downstream events, such as membrane leakage, loss in membrane fluidity and homeostasis of physiological ions, production of reactive oxygen species (ROS), mitochondrial damage, and cell death.1 In particular, the oligomeric forms of amyloid aggregates have shown to be the most toxic and are a major contributor to the pathophysiologies of AD, Parkinson's disease (PD) and type 2 diabetes (T2D), among others.10,14,15
Much research over the past two decades has focused on understanding the amyloid aggregation of Aβ, employing cryo-electron microscopy (cryoEM), solid-state nuclear magnetic resonance (ssNMR) spectroscopy, X-ray crystallography, atomic force microscopy, size exclusion chromatography, Fourier transform infrared (FTIR) spectroscopy, and fluorescence microscopy/spectroscopy.10 The membrane axis of the AD pathogenesis has been established based on the carpet model, the poration model, the membrane receptor model, and the detergent model,11,16–18 involving membrane mimetics such as lipid bilayers, liposomes, large unilamellar vesicles and nanodiscs.19 In the development of AD therapeutics, peptidomimetics, small molecules, amyloid antibodies, and nanoparticles have been used as inhibitors to drive off pathway the self-aggregation of Aβ,20–39 or to shut down the peptide–membrane connection by promoting membrane–inhibitor and peptide–inhibitor interactions.40 Only a few of these efforts, however, have considered the interplay between cell membrane, Aβ, and the inhibitor within the same context.
In consideration of the central role of cell membrane in Aβ aggregation and the crucial need for developing nanomedicines for AD, here we examined the mitigation of membrane perturbation induced by Aβ with graphene quantum dots (GQDs). The neuroblastoma SH-SY5Y cell line is a common in vitro model for studying neuronal function and differentiation, offering opportunities to investigate the interaction of Aβ not only with phospholipids (as in lipid vesicles or bilayers) but also cholesterol- and monosialotetrahexosylganglioside-enriched lipid rafts, transmembrane proteins, and nicotinic acetylcholine receptors that are pertinent to the neuronal response to the peptide.41–44 GQDs, with surface modifications or in conjunction with other inhibitors, are biocompatible zero-dimensional nanostructures which have shown promising effects against the aggregation and toxicity of monomeric amyloid proteins associated with AD, T2D and PD.45–47 Specifically, in the current study we examined cell membrane fluidity using confocal fluorescence microscopy in conjunction with a lipophilic laurdan dye reporter. The mechanisms of GQDs binding with the three major forms of Aβ, i.e., monomers (Aβ-m), oligomers (Aβ-o) and amyloid fibrils (Aβ-f), were revealed using discrete molecular dynamics (DMD) simulations. This study offered first evidence on the recovery of membrane fluidity by GQDs, an Aβ aggregation inhibitor and a future AD nanomedicine.
2. Materials and methods
Materials
Hydroxylated graphene quantum dots (GQDs) (1 mg mL−1, purity >80%) were purchased from ACS Material. Lyophilized human beta-amyloid (1–42) (Aβ42 monomer, DAEFRHDSGYEVHHQKLVFFAEDVGSNKGAIIGLMVGGVVIA; HPLC purity ≥95%48) was purchased from AnaSpec. Oligomer A11 polyclonal antibody was obtained from Invitrogen. Donkey anti-rabbit Alex 594 secondary antibody and phalloidin-iFluor 488 were purchased from Abcam. An OxiSelect™ intracellular ROS detection kit was obtained from Cell Biolabs. All sample solutions were prepared in Milli-Q water and the solvents used were of analytical grade.
Attenuated total reflection-Fourier transform infrared (ATR-FTIR) spectroscopy
ATR-FTIR spectroscopy (4000–800 cm−1) was performed on an IRTracer-100 (Shimadzu) spectrometer, equipped with a He–Ne laser as well as an MCT detector (Hg–Cd–Te), which was constantly cooled with liquid nitrogen. As far as the sample preparation is concerned, 5 μL of the hydroxylated GQDs (1 mg mL−1) were added on top of the reservoir and were air-dried afterwards using a heat gun. Data acquisition included the transmittance (%) measurement mode with 512 total number of scans at 8 cm−1 resolution. Signal processing was performed using the Happ–Genzel apodization function.
Dynamic light scattering (DLS)
The hydrodynamic size (determined by volume%) was obtained and zeta-potential measurements were performed on a Zetasizer Nano-ZS (Malvern) at room temperature using a solid-state He-Ne laser. The total number of scans was 11 and each scan lasted for 10 s. Hydroxylated GQDs were suspended in MilliQ H2O at 0.03 mg mL−1 final concentration. Analysis was performed using Zetasizer Software 7.02.
Thioflavin T (ThT) fluorescence kinetic assay
A ThT fluorescence kinetic assay was performed to monitor Aβ fibrillization. Strong ThT fluorescence was recorded at 482 nm once the dye was bound to the surface grooves of amyloid fibrils. Aβ-m was freeze dried after a 3 h-treatment by hexafluoro-2-propanol. Then Aβ-m was dissolved in 10 μL of 0.1% NH4OH and Milli-Q water was then added to obtain a stock of 100 μM Aβ-m. A 100 μL phosphate-buffered saline (PBS) solution containing 20 μM of Aβ-m and 40 μM ThT, in the presence or absence of GQDs was prepared in a black 96 well plate. The plate was incubated at 37 °C and the ThT fluorescence intensity was recorded at Ex 440 nm/Em 485 nm over a time course of 50 h.
Transmission electron microscopy (TEM)
The amyloid aggregation of Aβ in the presence or absence of GQDs was imaged by TEM after 72 h incubation. A 10 μL drop of each sample was placed onto a glow-discharged, formvar/carbon-coated copper grids (400 mesh, ProSciTech). After 1 min blotting, the grids were negatively stained for 30 s with 5 μL of uranyl acetate (1%) and excess stain was dried on Whatman filter paper. The grids were inserted into specimen holders and imaged on an FEI Tecnai F20 microscope equipped with an energy dispersive spectroscopy detector at 200 kV.
Membrane fluidity measurements
Lipophilic 6-dodecanoyl-2-dimethylaminonaphthalene (laurdan) dye was used to monitor the membrane lipid order. Once partitioned into cell membranes, laurdan emitted fluorescence at 450 nm when the cell membranes were in the gel/liquid ordered phase and the fluorescence was redshifted to 500 nm in the liquid disordered phase under an excitation of 405 nm. The generalized polarization (GP) values between the ordered and disordered shifts indicated the membrane fluidity.
1.4 × 105 SH-SY5Y cells in 250 μL of complete DMEM/F12 media were seeded onto an 8-well chamber slide and cultured overnight. Prior to imaging, laurdan dye was added to each well to a final concentration of 50 μM and allowed to equilibrate with the cells for 1 h. Cells were observed and imaged under a Leica SP8 inverted confocal fluorescence microscope. Live fluorescence imaging at 37 °C and 5% CO2 was necessary to maintain cell vitality over the testing period. Laurdan was excited with a 405 nm laser line and its emission was read at 430–470 nm or 480–550 nm. Aβ species with or without GQDs were added to the respective wells and images were acquired after 3 h incubation. The dye solution without cells was imaged for calibration. The acquisition of GP images was derived using ImageJ software.49,50 Then the GP values of cell membranes were calculated according to equation
, where I represents the pixel intensity of cell membrane from ordered (430–470 nm) or disordered (480–530 nm) channels. GP shifts were derived by subtracting the GP distribution peak maximum of each sample with 3 h of incubation from the GP values derived from images taken at the beginning of the experiment (0 h).
Reactive oxygen species (ROS) generation
ROS detection was performed using an OxiSelect™ intracellular ROS detection kit. Human neuroblastoma (SH-SY5Y) cells were cultured in complete DMEM/F12 media with 10% fetal bovine serum (FBS). ∼50
000 cells per well were seeded into a Costar black/clear bottom 96-well plate and were cultured overnight. After 30 min incubation with 20 μg mL−1 H2DCFDA, SH-SY5Y cells were treated with Aβ-m in the presence and absence of GQDs for 3 h. The fluorescence intensity of fluorescent DCF was measured on a microplate reader CLARIOstar at Ex 488 nm and Em 535 nm. H2O2 (200 μM) was used as the positive control.
In vitro cytotoxicity
Cell mortality was detected by labelling the dead SH-SY5Y cells with propidium iodide (PI, 1 μM). SH-SY5Y cells were incubated with PI for 30 min prior to the treatment of 20 μM Aβ-o. The percentage of cell death (PI-positive cells) was acquired and quantified every hour for 24 h using an Operetta CLS high-content analyzer (PerkinElmer) at 37 °C and 5% CO2. H2O2 200 μM was used as the positive control.
Detection of Aβ-o distribution
Immunofluorescence was performed to reveal the in vitro distribution of Aβ-o. ∼1.2 × 105 SH-SY5Y cells/well were grown on an 8-well chamber slide (μ-Slide, Ibidi) overnight. SH-SY5Y cells were incubated with the samples of Aβ-o with or without GQDs for 3 h. After being gently washed with PBS, the cells were fixed by 4% paraformaldehyde for 15 min. A11 antibody (1
:
400, 70 μL) was then incubated with the cells at 4 °C overnight. After removing excess primary antibody and rinsing thoroughly with PBS, donkey anti-rabbit Alex 594 secondary antibody (1
:
500, 70 μL) was incubated with the cells at room temperature for 2 h. Then the cells were washed with PBS and further stained with Hoechst 33342 (1
:
1000) for 5 min. The chamber slide was transferred to a Leica SP8 inverted confocal fluorescence microscope, and the cells were observed and imaged using a 63×/1.40 numerical aperture oil immersion objective.
Statistical analysis
Data were presented as mean (n = 3) ± SD. One-way ANOVA with Tukey's multiple comparison tests was used for statistical analysis using GraphPad Prism 8 software. *represents P < 0.05, which was considered to be statistically significant.
Molecular dynamics simulations
Interactions of Aβ-m, Aβ-o and Aβ-f with GQDs were simulated by all-atom discrete molecular dynamics (DMD), a rapid and predictive molecular dynamics algorithm. A comprehensive description of the atomistic DMD algorithm can be found in previous publications.51–53 Briefly, the continuous interaction potentials in classic molecular dynamics were replaced by optimized discrete stepwise functions in DMD. By solving the ballistic equations of motion for only those particles participating in a collision, instead of solving Newton's equations of motion for every particle in the system by classic molecular dynamics, DMD can achieve a rapid computational speed and enhanced sampling efficiency. Thus, DMD simulations have been utilized by our group and others to study protein folding, amyloid aggregation, and interactions of proteins/peptides with nanoparticles.50,54–56 The DMD program is available via Molecules in Action, LLC (http://www.moleculesinaction.com/).
Similar to classical molecular dynamics, the interatomic interactions in our all-atom DMD simulations with implicit solvent included both bonded interactions (i.e., covalent bonds, bond angles, and dihedrals) and non-bonded interactions (i.e., van der Waals, solvation, hydrogen bond, and electrostatic terms). The interatomic interactions were adapted from the Medusa force field.57,58 The force field parameters for van der Waals, covalent bonds, bond angles and dihedrals were taken from the CHARMM force field.59 Solvation energy was implicitly calculated by the effective energy function proposed by Lazaridis and Karplus,60 whereas the distance- and angle-dependent hydrogen bond formation was explicitly modelled by a reaction-like algorithm.61 The screened electrostatic interactions between charged atoms were computed by the Debye–Hückel approximation with the Debye length assigned ∼10 Å to mimic a water dielectric constant of 80 and a physiological monovalent electrolyte concentration of 100 mM.
The initial structural coordinates of Aβ-m and Aβ-f were obtained from the protein databank (PDB code: 1Z0Q and 5OQV, respectively). A GQD was constructed using Avogadro with its surface randomly decorated by oxygen-containing functional groups (cf.Fig. 5A).62 The obtained GQD structure was of 3 nm in diameter and consisted of 349 total atoms, including 27 hydroxyls, 10 epoxides and 5 carboxyls. A cubic box with the periodic boundary condition was used and the dimension of the box was set to maintain the concentration of Aβ peptides to be the same as that of a single peptide in a cubic box with the dimension of 7.5 nm. Counter ions (Na+) were added accordingly to maintain the molecular systems at neutral charge conditions. The initial structure of GQD was relaxed with a 100 ns simulation. To ensure sufficient conformational sampling, 30 independent simulations starting from different initial configurations and velocities were performed for each system with the temperature fixed at 300 K using the Anderson's thermostat.63 Each independent simulation with Aβ-m and Aβ-f lasted for 500 ns and those with Aβ-o ran for 800 ns due to complex conformational changes.
Structural properties were analyzed after the simulations reached steady states. The dictionary of secondary structure of the protein (DSSP) program was adopted to calculate the secondary structure of the peptide.64 Residue-wise contact frequency maps and GQD-Aβ binding frequencies were calculated by defining residue–residue and residue–GQD contacts if the minimal distance between heavy atoms was less than 0.55 nm. The two-dimensional potential of mean force (PMF) was computed using the probability distribution function, i.e., −kBT
ln
P(Rg,Nβ-residues), where kB is the Boltzmann constant, kB is the simulation temperature of 300 K, and ln
P(Rg,Nβ-residues) the probability of a conformation having a given value of radius of gyration Rg and a total number of β-sheet residues of Nβ-residues.
3. Results and discussion
Inhibition of Aβ fibrillization by graphene quantum dots
The size of the hydroxylated GQDs was initially characterized via TEM imaging, showing a sub-5 nm size distribution (Fig. 1C). DLS measurements (Fig. S1c–e, ESI†) indicated a uniformly distributed particle size of 11.4 ± 3.5 nm with a polydispersity index (PDI) of 0.366, as well as a slightly negative surface charge of −7.8 ± 5.5 mV. The functional groups of GQDs were further determined with FTIR (Fig. S1a and b, ESI†). Specifically, a broad band between 3550 and 3000 cm−1 can be attributed to the stretching vibration of O–H, while a sharp double band in the 1124–1050 cm−1 region can be attributed to the stretching vibration of C–O indicating the presence of primary/secondary alcohol groups on the GQD surface. Moreover, vibrational bands owing to bending or stretching vibrations of C
C and C–H in Fig. S1a and b (ESI†) further confirmed the presence of alkene or alkane groups in the GQD core. The effects of GQDs on the kinetics and morphologies of Aβ aggregation, were first examined by a ThT fluorescence kinetic assay and TEM. This is essential for assessing the potency of GQDs for membrane fluidity recovery against Aβ. Typical sigmoidal curves, that are characteristic of amyloid protein aggregation and indicative of peptide conversions from Aβ-m to Aβ-o and Aβ-f, were observed by the changes in ThT fluorescence intensity with time (Fig. 1A). The fluorescence intensity reached a plateau in 20 h with the Aβ concentration of 20 μM, while TEM imaging revealed well-defined rigid mature fibril formation (Fig. 1C). The ThT fluorescence in the saturation phase registered marked reductions with increased GQD concentrations (Fig. 1A and B). Although the ThT fluorescence intensity increased with GQDs of 0.5 μg mL−1, compared to 20 μM Aβ alone, TEM images revealed the occurrence of amorphous aggregates only (Fig. 1C). At GQD concentration of 10 μg mL−1, the ThT fluorescence intensity at saturation was less than half of that for Aβ alone and the formation of fibrils was completely impeded (Fig. 1A–C).
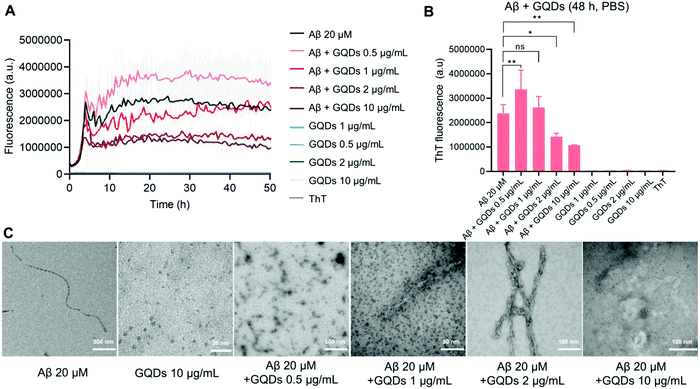 |
| Fig. 1 Inhibitory effects of graphene quantum dots on Aβ aggregation. (A) ThT fluorescence kinetic assay of Aβ42 (20 μM) aggregation in the presence and absence of GQDs with concentrations of 0.5, 1, 2 and 10 μg mL−1. (B) ThT fluorescence intensity of Aβ42 aggregation after 48 h of incubation with or without GQDs. The data points shown are the mean values (n = 3) ± SD. *, ** represent P < 0.01 and P < 0.001 between the compared groups, respectively. ns denotes no significant difference. (C) TEM imaging of Aβ42 aggregations in the presence and absence of GQDs at 72 h incubation timepoint. Aβ42 concentration: 20 μM. | |
Disruption of membrane fluidity by Aβ and its rescue by graphene quantum dots
We next evaluated the changes to membrane fluidity induced by Aβ and GQDs in vitro. The order of lipids in cell membrane, an indicator of membrane fluidity, was monitored by laurdan, a lipophilic dye capable of partitioning into cell membrane. Here, membrane fluidity was characterized by GP values, where a negative GP shift indicated a decreased membrane lipid order and a positive value reflecting an increased membrane order. Detailed descriptions of membrane fluidity measurement can be found in Methods and in previous publications.49,50 Specifically, the GP shifts (represented by “d”) for SH-SY5Y cells indicated that Aβ-m (+0.0293), Aβ-f (−0.0079), and GQDs (2 μg mL−1, −0.0702; 10 μg mL−1, −0.0836; 50 μg mL−1, −0.1508) by themselves only induced neglectable shifts in membrane fluidity (Fig. 2 and Fig. S2 in ESI†). In contrast, Aβ-o caused a large positive GP shift (+0.1793), indicating its effective role in increasing membrane lipid order which may explain the disruption in the membrane fluidity by this most toxic Aβ aggregate species (Fig. 2A). The more ordered membrane structure can be attributed to increased lipid head groups per unit area as well as perforation in neuronal cell membranes induced by Aβ-o. In comparison, the positive shift of membrane order was diminished (+0.1053) in the presence of GQDs at a low concentration of 2 μg mL−1. The membrane order was almost fully restored to that of control cells with GQDs of 10 μg mL−1 (−0.0411), where the recovery effect reached saturation (−0.0374) with GQDs of 50 μg mL−1. Taken together, we found that the disruption of the membrane induced by Aβ-o was effectively rescued by GQDs, and the molecular mechanisms of GQDs interacting with the Aβ species were further examined by DMD simulations (presented in a later section).
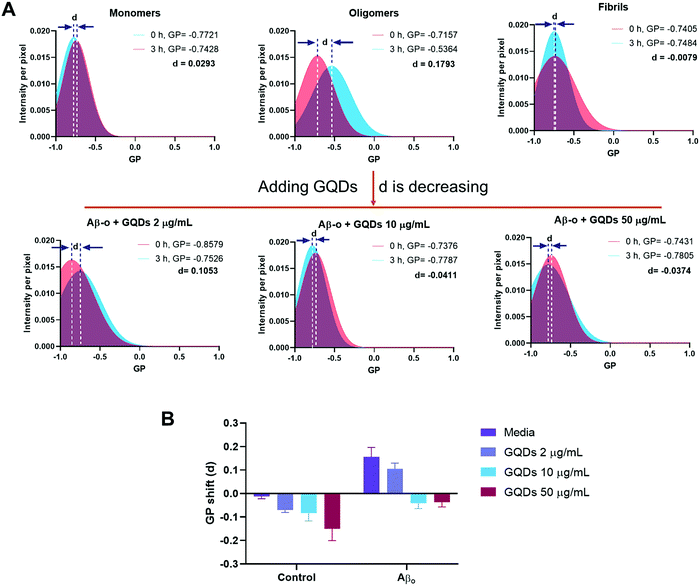 |
| Fig. 2 Effects of Aβ-m, Aβ-o, and Aβ-f on SH-SY5Y cell membrane fluidity in the presence and absence of GQDs. (A) GP shifts (represented by letter d) were recorded after a 3 h-treatment by Aβ-m, Aβ-o and Aβ-f (20 μM) of Aβ42. Compared with Aβ-m and Aβ-f, Aβ-o caused a large positive GP shift. GQDs prevented perturbation to membrane fluidity induced by Aβ-o and restored the GP values to that for control cells, especially at 10 and 50 μg mL−1. (B) Summaries of the GP values of panel A and Fig. S2 (ESI†). | |
Association of Aβ with the cell membrane is essential for the downstream biological and toxicological effects of the amyloidogenic peptide. Therefore, we focused on the cellular distribution of Aβ-o and changes induced by GQDs since Aβ-o induced the most significant shift in membrane fluidity. Anti-oligomer (A11) antibody was used to specifically recognize Aβ-o, but not Aβ-m or Aβ-f in vitro. Distribution of Aβ-o on SH-SY5Y cells was measured by confocal fluorescence imaging over 3 h of incubation (Fig. 3 & Fig. S3–S5, ESI†). As indicated by the red spots in the merged images, Aβ-o themselves were prone to association with cell membranes in 3 h of treatment (Fig. 3 and Fig. S4, ESI†). In the presence of GQDs, however, the intensity of Aβ-o around cell membranes declined markedly, and the cytoplasm was gradually lighted up by the complexes of Aβ-o and GQDs over time (Fig. 3 and Fig. S3, ESI†). Thus, the association of Aβ-o with the cell membrane was disrupted by GQDs, restoring membrane integrity perturbed by the peptide.
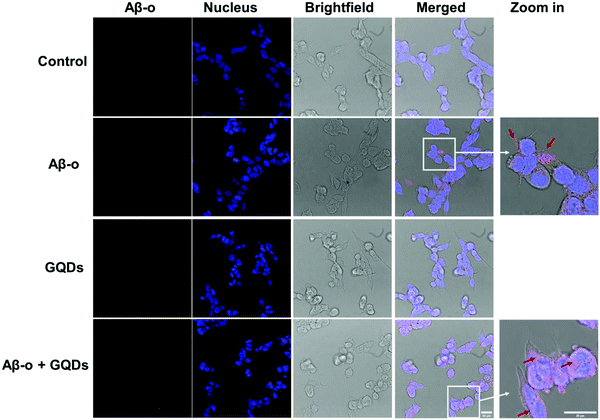 |
| Fig. 3 Aβ42 oligomers distribution on SH-SY5Y cells in the presence and absence of GQDs. Confocal images of Aβ-o (concentration: 20 μM) distribution after 3 h of treatment, including Aβ-o (red), nucleus (blue), bright-field (gray) and merged images for the 3 channels. Aβ-o were labeled by A11 antibody in vitro. The red arrows in zoomed-in images indicate the positions of Aβ-o. GQDs: 50 μg mL−1. Scale bars: 20 μm. | |
The obstruction of the membrane axis of Aβ by GQDs was further verified by assays on actin organization, ROS generation and cell viability. Immunofluorescence imaging of actin filaments (Em 488 nm) after a 3 h treatment showed that Aβ-o promoted the expression of actin filaments, whereas the actin filament intensities reduced with the increased concentration of GQDs (Fig. 4A and B). Aβ-m, Aβ-f and GQDs, as expected, elicited no toxicity in neurocytes (Fig. 4C and D). Although there was no significant amount of ROS generated by 3 h Aβ-o exposure, Aβ-o was the most potent species for inducing cell death after 20 h incubation. In comparison, cell mortality caused by Aβ-o (around 30% cell death after 20 h incubation) was significantly alleviated by the presence of GQDs (to less than 5%, with 10 μg mL−1 GQDs) (Fig. 4E). Together, GQDs displayed a potency for the mitigation of Aβ toxicity by effectively alleviating actin reorganization and suppressing cell death.
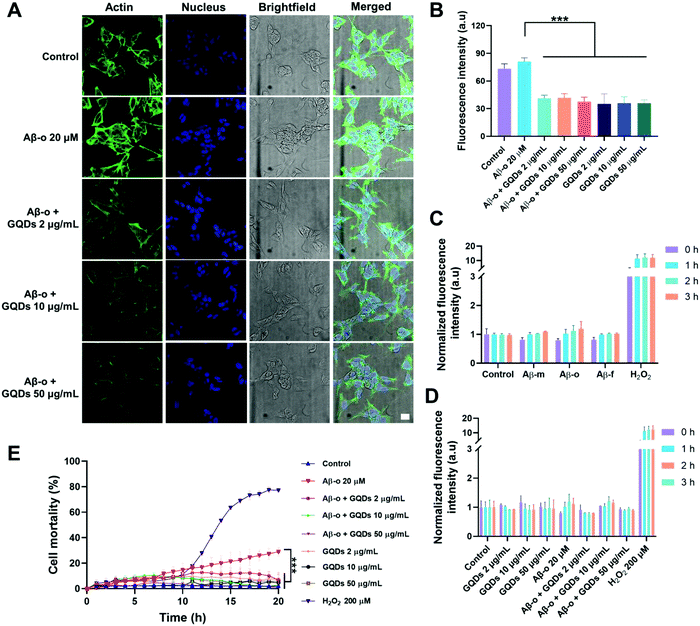 |
| Fig. 4 Actin organization, ROS generation and cell mortality of SH-SY5Y cells exposed to Aβ42 oligomers (Aβ-o, 20 μM) in the presence and absence of GQDs. (A) Immunofluorescence imaging of actin filaments after a 3 h treatment of Aβ-o (20 μM) and GQDs (2 μg mL−1, 10 μg mL−1 and 50 μg mL−1). Actin filaments were stained by phalloidin-iFluor 488 (green). (B) Calculation of the fluorescence intensity of actin filaments according to panel A using ImageJ software. Data points are depicted as mean values (n = 3) ± SD, via ne-way ANOVA, ***P < 0.0001 compared with Aβ-o. (C and D) ROS production of SH-SY5Y cells were identified by H2DCFDA staining after 3 h treatment with (C) Aβ-m, Aβ-o and Aβ-f and (D) Aβ-o with the combination of GQDs. H2O2 (200 μM) was used as positive control. (E) Cell mortality after 20 h treatment with Aβ-o and GQDs. Data are shown as mean values (n = 3) ± SD, via two-way ANOVA analyzed at 20 h, *** P < 0.0001 compared with Aβ-o. | |
Dynamics of Aβ-GQD interaction by discrete molecular dynamics simulations
To gain a molecular insight into the inhibition mechanism of GQDs on Aβ aggregation, we conducted all-atom DMD simulations. Various Aβ species, including Aβ-m, preformed Aβ-o and Aβ-f, were considered to reveal the interaction modes in accordance with the in vitro experiments. For Aβ-m, the peptide initially placed close to the GQD became adsorbed onto the nanoparticle surface in 200 ns (Fig. 5B). As illustrated by the distance between each Aβ residue and the GQD (Fig. 5B) and the corresponding snapshots of a typical simulation trajectory (Fig. 5D), the N-terminus of the peptide initiated the adsorption process with the helical structure between residues 11–22 remained intact (Fig. 5C and D). Subsequently, the C-terminus was brought into contact with the opposite surface of the GQD in 20 ns, after which the helical structure underwent unfolding gradually. The helix unfolding of Aβ residues 16–22 took a much longer time (about 200 ns), supporting its role as the rate-limiting step for Aβ conformational changes. After the system reached a steady state, the propensity of each Aβ residue to participate in a β-sheet structure was less than 0.1, significantly less than the control (Fig. 5E and Fig. S6, ESI†). For Aβ-m alone, the peptide was rich in unstructured coils and the average β-sheet structure content was about 28%, which was consistent with the value of 24% shown by circular dichroism spectroscopy.65 The amyloidogenic region of residues 16–22 (Aβ16-22), which played important roles in Aβ aggregation and fibril elongation,66 possessed a high propensity to form a helical structure, consistent with the NMR results.67 In the presence of the GQD, however, the ordered helical and β-sheet structures of Aβ-m were rendered into random coils which consisted of more than 80% in content (Fig. 5F). The robust effect of the GQD on reducing the ordered protein structure can be attributed to the strong binding affinity of the GQD for Aβ-m, with the binding frequency of each Aβ-m residue being around 0.8 or higher (Fig. 5G). The strong binding originated from the amphiphilic characters of the GQD and the Aβ peptide, which enabled the coexistence of multiple interactions, including aromatic stacking, electrostatic attraction between the positively charged residues of Aβ-m and the carboxylic acids of the GQD, hydrophobic interaction between the hydrophobic residues of the peptide and non-oxidated regions of the GQD, as well as hydrogen bonding between the polar residues of Aβ-m and the epoxide, carbonyl and hydroxyl groups of the GQD.
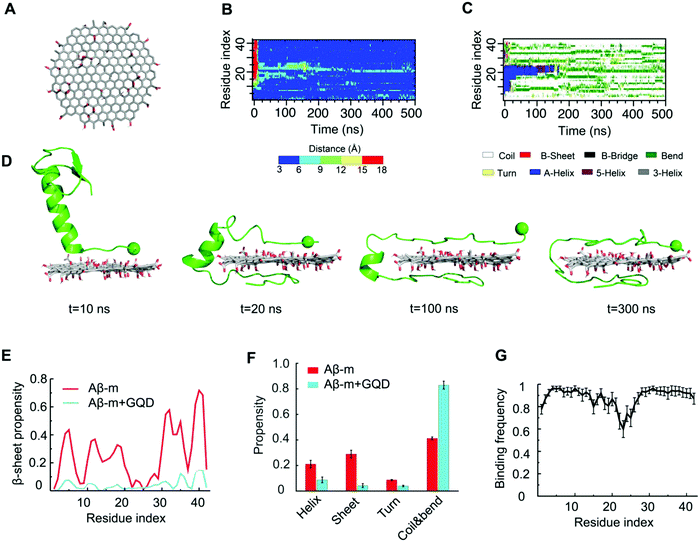 |
| Fig. 5 Interactions between Aβ-m and a graphene quantum dot. (A) Structure of the GQD used in the simulations. Time evolution of (B) distance between each Aβ residue and the GQD and (C) secondary structure of Aβ-m peptides from a typical DMD simulation trajectory. (D) Snapshots of the interaction process shown in (B and C). The GQD was represented by sticks and Aβ-m was shown as cartoon with the N-terminus indicated by a sphere. (E) β-Sheet propensity of each Aβ-m residue in the absence and presence of the GQD. (F) Secondary structure contents of Aβ-m with and without the GQD. (G) Binding frequency of each Aβ-m residue with the GQD. | |
To investigate the interactions between Aβ-o and GQDs, the dynamics and structural changes of Aβ tetramers in the absence and presence of a GQD were simulated. Stable Aβ tetramers were formed via the self-assembly of four Aβ-m peptides for 400 ns, after which a GQD was placed near the tetramer with the atomic distance larger than 1.5 nm (Fig. 6A). Coaggregation of 4 Aβ monomers randomly mixed with the GQD was also simulated to probe the effect of GQDs on the Aβ conformation. Upon the addition of the GQD, the β-sheet content of the preformed Aβ tetramer gradually decreased and reached the same level as that with the coaggregation system, both of which were noticeably lower than the control (Fig. 6B), consistent with the experimental results (Fig. 1). The averaged secondary structure propensities after the simulation reached steady states indicated that ordered β-sheet structure was rendered into coils and bends in the presence of the GQD, whereas the reduction of helical structure was subtle (Fig. 6C). To reveal the inhibition mechanism, the secondary structure of each Aβ residue together with the residue-wise intra- and inter-peptide contact frequency maps were calculated (Fig. 6D-F and Fig. S7, ESI†). The reduction of β-sheet propensity was most significant in residues 1–22 (Fig. 6D), covering the amyloidogenic core region Aβ16–22, due to the higher binding affinity of the GQD for the N-terminus than the C-terminus of Aβ-o illustrated by the probability distribution of the distance between each Aβ-o residue and the GQD surface (Fig. S8, ESI†). Besides, both the diagonal and anti-parallel regions of the inter-peptide contact map were effectively reduced, especially in the core region Aβ16–22. Thus, the GQD disrupted the Aβ-o by strongly binding with the N-termini of Aβ peptides and shifting the contact preference of Aβ peptides to the GQD instead of other peptides, thereby resulting in a reduction of inter-peptide contacts. This high Aβ-GQD binding affinity was expected to diminish the peptide–peptide and Aβ–membrane interactions, thus breaking down the membrane-axis of Aβ.
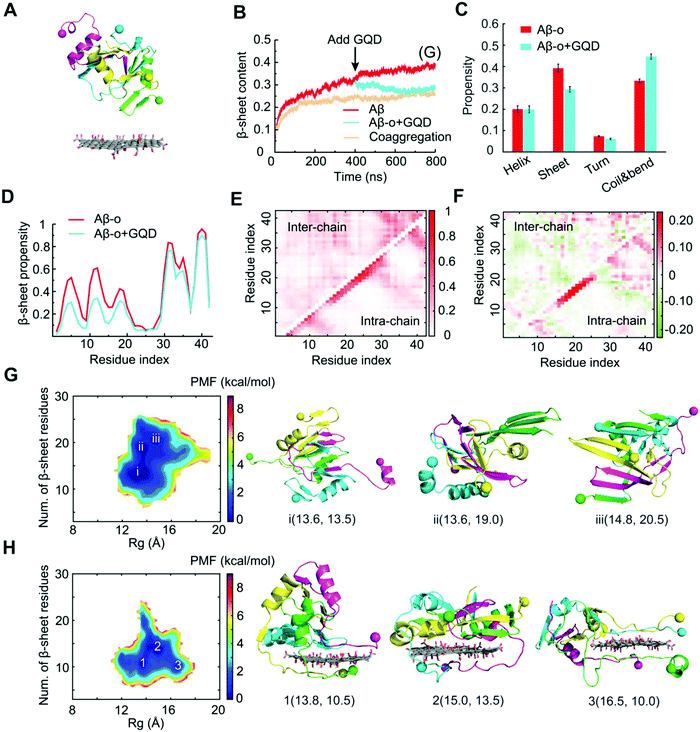 |
| Fig. 6 Interactions between Aβ-o with a graphene quantum dot. (A) Initial structure of the simulation systems. A preformed Aβ tetramer was placed near the GQD. (B) Time evolution of the β-sheet content. Aβ-o: a preformed Aβ tetramer. Coaggregation: four Aβ-m with the GQD. (C) Secondary structure propensities of Aβ after the simulations reached the steady state. (D) β-Sheet propensity of each Aβ residue in the absence and presence of the GQD. (E) Intra- and inter-peptide contact frequency maps for Aβ peptides. (F) Changes of the contact frequency maps in the presence of GQD compared with the control. (G and H) Two-dimensional potential of mean force (PMF) with respect to the number of β-sheet residues and radius of gyration for Aβ-o (G) and Aβ-o + GQD (H). The basins of the PMFs were labeled with typical snapshots presented on the right. The GQD was represented by sticks and Aβ-o in cartoon with the N-termini indicated by spheres. | |
To probe the conformational properties of Aβ-o in the absence and presence of the GQD, two-dimensional PMF (the effective free-energy landscape) as a function of the total number of β-sheet residues and Rg of Aβ-o was computed (Fig. 6G and H). In the absence of the GQD, the PMF of Aβ-o featured three basins with the number of β-sheet residues ranging between 13 and 21, corresponding to the helical-rich, β-sheet-containing and β-sheet-rich structures. In contrast, the number of β-sheet residues was suppressed to only 10.5-13.5 for the basins in the presence of the GQD. Moreover, the Rg value of the basins increased from 13.6–14.8 Å to 13.8–16.5 Å by the interaction of Aβ-o with the GQD, indicating that the Aβ aggregates became more extended in the presence of the GQD. Taken together, the DMD simulations revealed that strong interactions between the amphiphilic GQD and Aβ-o effectively converted the ordered structures of preformed Aβ-o into unstructured coils and extended the Aβ peptide conformations.
To understand the interaction between Aβ-f and GQDs, we further simulated the binding of a preformed Aβ-f with GQDs (Fig. 7). Two GQDs were placed near the Aβ-f (comprising 9 Aβ peptides) to maintain the same Aβ/GQD concentration ratio as in the Aβ-o simulations. The movement of Aβ-f was constrained to reduce the computational cost for such a large molecular system while reflecting the rigidity of mature fibrils. The conformations of the Aβ-f-GQD complexes showed that the GQDs could bind both the ends and sidewalls of the fibrils (Fig. 7A), indicating that the elongation and secondary nucleation phases could be obstructed by the GQDs. The binding frequency of each Aβ residue demonstrated that the GQDs had high binding affinities for the first 20 residues of the peptides on both the ends and sidewalls (Fig. 7B and C). The lower binding frequency of the GQDs with the C-termini of sidewalls compared to those on the ends of Aβ-f was due to the concave shape formed by the C-terminal regions. Therefore, the direct interaction between Aβ-f and cell membrane could also be attenuated by the fibrillar coating of GQDs. In addition, since the strong binding of Aβ with GQDs was equally contributed by a wide range of residues (Fig. 5G) and the oligomers and fibrils formed by different Aβ isomers shared similar structural characteristics,68,69 we expect GQDs can bind different Aβ isomers to disrupt their membrane-axes.
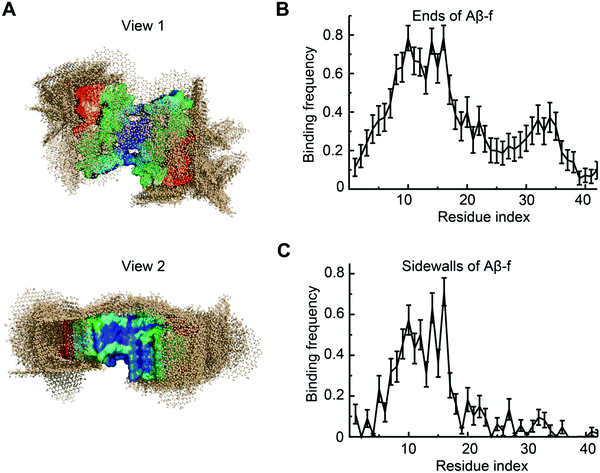 |
| Fig. 7 Interactions between Aβ-f and GQDs. (A) Overlaying of final snapshots from 30 independent simulations, where a preformed Aβ-f comprising 9 peptides was illustrated by its surfaces with molecular surface colored according to each residue's binding probability with two GQDs from low (blue) to high (red). GQD atoms were displayed in wheat spheres. (B, C) Binding frequencies of each Aβ residue at (B) ends and (C) sidewalls of Aβ-f with the GQDs. | |
4. Conclusions
Recent development of nanoparticle inhibitors against amyloid aggregation has led to a new frontier in nanomedicine,70 fueled by the advantageous physicochemical properties of nanomaterials and the need for novel and more effective therapeutic agents for neurological disorders and metabolic diseases. Many of these research efforts have benefited from our existing knowledge on the structure and aggregation kinetics of amyloidogenic proteins; yet only a few have connected the dots between the amyloid proteins, their membrane axis, and their nanoparticle inhibitors. Accordingly, in the present study we examined membrane fluidity perturbed by the three major forms of Aβ, and demonstrated the mitigation potential of GQDs in reverting SH-SY5Y cells to their native fluidic state, especially in the presence of the most invasive and toxic Aβ-o. We detailed the molecular mechanisms of the three peptide species interacting with GQDs using DMD simulations. While Aβ-m bound to the GQDs via strong electrostatic and hydrophobic interactions with H-bonding, the nanostructures reshaped the three basins of the PMF of Aβ-o to discourage the β-sheet propensity of the peptide residues. Furthermore, GQDs displayed a tendency to adhere to the sides and ends of an Aβ-f, thereby hindering their elongation. The affinities of GQDs for all three representative forms of Aβ, as revealed by the computer simulations and our in vitro experiments, ensured a strong association of the peptide with the nanostructure, overcoming intermolecular peptide–peptide interactions as well as the membrane axis of Aβ. Altogether, this study implicated GQDs as a potential AD nanomedicine from the new perspective of their capacity for recovering membrane fluidity and potentially restoring the homeostasis of neuronal cells.
Author contributions
P. C. K. and F. D. conceived the project. H. T., Y. L. and P. C. K. wrote the manuscript. Y. L. performed membrane fluidity and actin reorganization confocal fluorescence imaging, ROS assay and viability assay. A. K. and N. A. performed TEM, ThT and confocal imaging analysis. H. T., Y. S. and F. D. performed DMD computer simulations and analysis. All authors agreed on the presentation of the manuscript.
Conflicts of interest
The authors declare no conflict of interest.
Acknowledgements
This work was supported by NSF CBET-1553945 (Ding), NIH R35GM119691 (Ding), CanNano 2021GNPT02 (Ke) and NSFC 82104087 (Li).
References
- J. M. Long and D. M. Holtzman, Cell, 2019, 179, 312–339 CrossRef CAS PubMed.
- J. Hardy and G. Higgins, Science, 1992, 256, 184–185 CrossRef CAS PubMed.
- M. I. Ivanova, Y. Lin, Y.-H. Lee, J. Zheng and A. Ramamoorthy, Biophys. Chem., 2021, 269, 106507 CrossRef CAS.
- S. Sharma, P. Modi, G. Sharma and S. Deep, Biophys. Chem., 2021, 278, 106665 CrossRef CAS PubMed.
- C. E. G. Leyns, J. D. Ulrich, M. B. Finn, F. R. Stewart, L. J. Koscal, J. Remolina Serrano, G. O. Robinson, E. Anderson, M. Colonna and D. M. Holtzman, Proc. Natl. Acad. Sci. U. S. A., 2017, 114, 11524–11529 CrossRef CAS PubMed.
- A. de Calignon, M. Polydoro, M. Suárez-Calvet, C. William, D. H. Adamowicz, K. J. Kopeikina, R. Pitstick, N. Sahara, K. H. Ashe, G. A. Carlson, T. L. Spires-Jones and B. T. Hyman, Neuron, 2012, 73, 685–697 CrossRef CAS PubMed.
- S. M. Butterfield and H. A. Lashuel, Angew. Chem., Int. Ed., 2010, 49, 5628–5654 CrossRef CAS PubMed.
- G. P. Eckert, W. G. Wood and W. E. Muller, Curr. Protein Pept. Sci., 2010, 11, 319–325 CrossRef CAS.
- E. E. Ambroggio, D. H. Kim, F. Separovic, C. J. Barrow, K. J. Barnham, L. A. Bagatolli and G. D. Fidelio, Biophys. J., 2005, 88, 2706–2713 CrossRef CAS PubMed.
- P. C. Ke, R. Zhou, L. C. Serpell, R. Riek, T. P. J. Knowles, H. A. Lashuel, E. Gazit, I. W. Hamley, T. P. Davis, M. Fändrich, D. E. Otzen, M. R. Chapman, C. M. Dobson, D. S. Eisenberg and R. Mezzenga, Chem. Soc. Rev., 2020, 49, 5473–5509 RSC.
- P. C. Ke, M.-A. Sani, F. Ding, A. Kakinen, I. Javed, F. Separovic, T. P. Davis and R. Mezzenga, Chem. Soc. Rev., 2017, 46, 6492–6531 RSC.
- K. J. Korshavn, C. Satriano, Y. Lin, R. Zhang, M. Dulchavsky, A. Bhunia, M. I. Ivanova, Y.-H. Lee, C. La Rosa, M. H. Lim and A. Ramamoorthy, J. Biol. Chem., 2017, 292, 4638–4650 CrossRef CAS PubMed.
- M. F. Sciacca, F. Lolicato, C. Tempra, F. Scollo, B. R. Sahoo, M. D. Watson, S. García-Viñuales, D. Milardi, A. Raudino, J. C. Lee, A. Ramamoorthy and C. La Rosa, ACS Chem. Neurosci., 2020, 11, 4336–4350 CrossRef CAS.
- S. T. Ferreira and W. L. Klein, Neurobiol. Learn. Mem., 2011, 96, 529–543 CrossRef CAS PubMed.
- P. H. Nguyen, A. Ramamoorthy, B. R. Sahoo, J. Zheng, P. Faller, J. E. Straub, L. Dominguez, J.-E. Shea, N. V. Dokholyan, A. De Simone, B. Ma, R. Nussinov, S. Najafi, S. T. Ngo, A. Loquet, M. Chiricotto, P. Ganguly, J. McCarty, M. S. Li, C. Hall, Y. Wang, Y. Miller, S. Melchionna, B. Habenstein, S. Timr, J. Chen, B. Hnath, B. Strodel, R. Kayed, S. Lesné, G. Wei, F. Sterpone, A. J. Doig and P. Derreumaux, Chem. Rev., 2021, 121, 2545–2647 CrossRef CAS PubMed.
- T. L. Williams and L. C. Serpell, FEBS J., 2011, 278, 3905–3917 CrossRef CAS PubMed.
- D. C. Bode, M. Freeley, J. Nield, M. Palma and J. H. Viles, J. Biol. Chem., 2019, 294, 7566–7572 CrossRef CAS PubMed.
- M. Serra-Batiste, M. Ninot-Pedrosa, M. Bayoumi, M. Gairí, G. Maglia and N. Carulla, Proc. Natl. Acad. Sci. U. S. A., 2016, 113, 10866–10871 CrossRef CAS PubMed.
- N. Andrikopoulos, Y. Li, L. Cecchetto, A. Nandakumar, T. Da Ros, T. P. Davis, K. Velonia and P. C. Ke, Nanoscale, 2020, 12, 14422–14440 RSC.
- P. T. Lansbury and H. A. Lashuel, Nature, 2006, 443, 774–779 CrossRef CAS PubMed.
- J. McLaurin, R. Cecal, M. E. Kierstead, X. Tian, A. L. Phinney, M. Manea, J. E. French, M. H. L. Lambermon, A. A. Darabie, M. E. Brown, C. Janus, M. A. Chishti, P. Horne, D. Westaway, P. E. Fraser, H. T. J. Mount, M. Przybylski and P. St George-Hyslop, Nat. Med., 2002, 8, 1263–1269 CrossRef CAS PubMed.
- J. Bieschke, M. Herbst, T. Wiglenda, R. P. Friedrich, A. Boeddrich, F. Schiele, D. Kleckers, J. M. Lopez del Amo, B. A. Grüning, Q. Wang, M. R. Schmidt, R. Lurz, R. Anwyl, S. Schnoegl, M. Fändrich, R. F. Frank, B. Reif, S. Günther, D. M. Walsh and E. E. Wanker, Nat. Chem. Biol., 2012, 8, 93–101 CrossRef CAS.
- C. Cabaleiro-Lago, F. Quinlan-Pluck, I. Lynch, S. Lindman, A. M. Minogue, E. Thulin, D. M. Walsh, K. A. Dawson and S. Linse, J. Am. Chem. Soc., 2008, 130, 15437–15443 CrossRef CAS PubMed.
- E. N. Gurzov, B. Wang, E. H. Pilkington, P. Chen, A. Kakinen, W. J. Stanley, S. A. Litwak, E. G. Hanssen, T. P. Davis, F. Ding and P. C. Ke, Small, 2016, 12, 1615–1626 CrossRef CAS PubMed.
- N. Gao, H. Sun, K. Dong, J. Ren, T. Duan, C. Xu and X. Qu, Nat. Commun., 2014, 5, 3422 CrossRef PubMed.
- Q. Luo, Y.-X. Lin, P.-P. Yang, Y. Wang, G.-B. Qi, Z.-Y. Qiao, B.-N. Li, K. Zhang, J.-P. Zhang, L. Wang and H. Wang, Nat. Commun., 2018, 9, 1802 CrossRef PubMed.
- Y. Zhao, J. Cai, Z. Liu, Y. Li, C. Zheng, Y. Zheng, Q. Chen, H. Chen, F. Ma, Y. An, L. Xiao, C. Jiang, L. Shi, C. Kang and Y. Liu, Nano Lett., 2019, 19, 674–683 CrossRef CAS PubMed.
- Q. Chen, Y. Du, K. Zhang, Z. Liang, J. Li, H. Yu, R. Ren, J. Feng, Z. Jin, F. Li, J. Sun, M. Zhou, Q. He, X. Sun, H. Zhang, M. Tian and D. Ling, ACS Nano, 2018, 12, 1321–1338 CrossRef CAS PubMed.
- I. Javed, G. Peng, Y. Xing, T. Yu, M. Zhao, A. Kakinen, A. Faridi, C. L. Parish, F. Ding, T. P. Davis, P. C. Ke and S. Lin, Nat. Commun., 2019, 10, 3780 CrossRef.
- M. Mahmoudi, O. Akhavan, M. Ghavami, F. Rezaee and S. M. A. Ghiasi, Nanoscale, 2012, 4, 7322 RSC.
- A. Gladytz, B. Abel and H. J. Risselada, Angew. Chem., Int. Ed., 2016, 55, 11242–11246 CrossRef CAS PubMed.
- I. Javed, T. Yu, G. Peng, A. Sánchez-Ferrer, A. Faridi, A. Kakinen, M. Zhao, R. Mezzenga, T. P. Davis, S. Lin and P. C. Ke, Nano Lett., 2018, 18, 5797–5804 CrossRef CAS PubMed.
- J. R. Brender, S. Salamekh and A. Ramamoorthy, Acc. Chem. Res., 2012, 45, 454–462 CrossRef CAS PubMed.
- S. A. Kotler, P. Walsh, J. R. Brender and A. Ramamoorthy, Chem. Soc. Rev., 2014, 43, 6692–6700 RSC.
- M. Li, X. Yang, J. Ren, K. Qu and X. Qu, Adv. Mater., 2012, 24, 1722–1728 CrossRef CAS PubMed.
- M. Li, S. E. Howson, K. Dong, N. Gao, J. Ren, P. Scott and X. Qu, J. Am. Chem. Soc., 2014, 136, 11655–11663 CrossRef CAS PubMed.
- Z. Du, M. Li, J. Ren and X. Qu, Acc. Chem. Res., 2021, 54, 2172–2184 CrossRef CAS PubMed.
- K. Wang, L. Wang, L. Chen, C. Peng, B. Luo, J. Mo and W. Chen, Drug Delivery, 2021, 28, 580–593 CrossRef CAS PubMed.
- M. S. Said, G. R. Navale, A. Yadav, N. Khonde, S. S. Shinde and A. Jha, Biophys. Chem., 2020, 267, 106480 CrossRef CAS PubMed.
- Y. Li, H. Tang, N. Andrikopoulos, I. Javed, L. Cecchetto, A. Nandakumar, A. Kakinen, T. P. Davis, F. Ding and P. C. Ke, Adv. NanoBiomed. Res., 2021, 1, 2000040 CrossRef PubMed.
- S. Hong, B. L. Ostaszewski, T. Yang, T. T. O’Malley, M. Jin, K. Yanagisawa, S. Li, T. Bartels and D. J. Selkoe, Neuron, 2014, 82, 308–319 CrossRef CAS PubMed.
- M. F. M. Sciacca, S. A. Kotler, J. R. Brender, J. Chen, D. Lee and A. Ramamoorthy, Biophys. J., 2012, 103, 702–710 CrossRef CAS PubMed.
- M. Ko, T. Hattori, M. Abdullah, J.-S. Gong, T. Yamane and M. Michikawa, Brain Res., 2016, 1642, 376–383 CrossRef CAS.
- J. Habchi, S. Chia, C. Galvagnion, T.
C. T. Michaels, M. M. J. Bellaiche, F. S. Ruggeri, M. Sanguanini, I. Idini, J. R. Kumita, E. Sparr, S. Linse, C. M. Dobson, T. P. J. Knowles and M. Vendruscolo, Nat. Chem., 2018, 10, 673–683 CrossRef CAS PubMed.
- D. Kim, J. M. Yoo, H. Hwang, J. Lee, S. H. Lee, S. P. Yun, M. J. Park, M. Lee, S. Choi, S. H. Kwon, S. Lee, S.-H. Kwon, S. Kim, Y. J. Park, M. Kinoshita, Y.-H. Lee, S. Shin, S. R. Paik, S. J. Lee, S. Lee, B. H. Hong and H. S. Ko, Nat. Nanotechnol., 2018, 13, 812–818 CrossRef CAS PubMed.
- M. Wang, Y. Sun, X. Cao, G. Peng, I. Javed, A. Kakinen, T. P. Davis, S. Lin, J. Liu, F. Ding and P. C. Ke, Nanoscale, 2018, 10, 19995–20006 RSC.
- Y. Liu, L.-P. Xu, Q. Wang, B. Yang and X. Zhang, ACS Chem. Neurosci., 2018, 9, 817–823 CrossRef CAS PubMed.
- A. Faridi, W. Yang, H. G. Kelly, C. Wang, P. Faridi, A. W. Purcell, T. P. Davis, P. Chen, S. J. Kent and P. C. Ke, Biomacromolecules, 2019, 20, 4208–4217 CrossRef CAS PubMed.
- E. H. Pilkington, E. N. Gurzov, A. Kakinen, S. A. Litwak, W. J. Stanley, T. P. Davis and P. C. Ke, Sci. Rep., 2016, 6, 21274 CrossRef CAS PubMed.
- Y. Li, H. Tang, H. Zhu, A. Kakinen, D. Wang, N. Andrikopoulos, Y. Sun, A. Nandakumar, E. Kwak, T. P. Davis, D. T. Leong, F. Ding and P. C. Ke, ACS Appl. Mater. Interfaces, 2021, 13, 29936–29948 CrossRef CAS PubMed.
- E. A. Proctor, F. Ding and N. V. Dokholyan, Wiley Interdiscip. Rev.: Comput. Mol. Sci., 2011, 1, 80–92 CAS.
- F. Ding, D. Tsao, H. Nie and N. V. Dokholyan, Structure, 2008, 16, 1010–1018 CrossRef CAS PubMed.
-
F. Ding and N. V. Dokholyan, in Computational Modeling of Biological Systems, ed. N. V. Dokholyan, Springer US, Boston, MA, 2012, pp. 55–73 Search PubMed.
- N. I. Brodie, K. I. Popov, E. V. Petrotchenko, N. V. Dokholyan and C. H. Borchers, Sci. Adv., 2017, 3, e1700479 CrossRef PubMed.
- S. J. Bunce, Y. Wang, K. L. Stewart, A. E. Ashcroft, S. E. Radford, C. K. Hall and A. J. Wilson, Sci. Adv., 2019, 5, eaav8216 CrossRef.
- Y. Sun, A. Kakinen, X. Wan, N. Moriarty, C. P. J. Hunt, Y. Li, N. Andrikopoulos, A. Nandakumar, T. P. Davis, C. L. Parish, Y. Song, P. C. Ke and F. Ding, Nano Today, 2021, 38, 101125 CrossRef CAS PubMed.
- F. Ding and N. V. Dokholyan, PLoS Comput. Biol., 2006, 2, e85 CrossRef.
- S. Yin, L. Biedermannova, J. Vondrasek and N. V. Dokholyan, J. Chem. Inf. Model., 2008, 48, 1656–1662 CrossRef CAS PubMed.
- B. R. Brooks, R. E. Bruccoleri, B. D. Olafson, D. J. States, S. Swaminathan and M. Karplus, J. Comput. Chem., 1983, 4, 187–217 CrossRef CAS.
- T. Lazaridis, Curr. Opin. Struct. Biol., 2000, 10, 139–145 CrossRef CAS.
- F. Ding, J. M. Borreguero, S. V. Buldyrey, H. E. Stanley and N. V. Dokholyan, Proteins: Struct., Funct., Genet., 2003, 53, 220–228 CrossRef CAS.
- M. D. Hanwell, D. E. Curtis, D. C. Lonie, T. Vandermeersch, E. Zurek and G. R. Hutchison, J. Cheminf., 2012, 4, 17 CAS.
- H. C. Andersen, J. Chem. Phys., 1980, 72, 2384–2393 CrossRef CAS.
- W. Kabsch and C. Sander, Biopolymers, 1983, 22, 2577–2637 CrossRef CAS PubMed.
- K. Ono, M. M. Condron and D. B. Teplow, Proc. Natl. Acad. Sci. U. S. A., 2009, 106, 14745–14750 CrossRef CAS PubMed.
- J. R. Brender, A. Ghosh, S. A. Kotler, J. Krishnamoorthy, S. Bera, V. Morris, T. B. Sil, K. Garai, B. Reif, A. Bhunia and A. Ramamoorthy, Chem. Commun., 2019, 55, 4483–4486 RSC.
- S. Vivekanandan, J. R. Brender, S. Y. Lee and A. Ramamoorthy, Biochem. Biophys. Res. Commun., 2011, 411, 312–316 CrossRef CAS.
- S. L. Bernstein, N. F. Dupuis, N. D. Lazo, T. Wyttenbach, M. M. Condron, G. Bitan, D. B. Teplow, J.-E. Shea, B. T. Ruotolo, C. V. Robinson and M. T. Bowers, Nat. Chem., 2009, 1, 326–331 CrossRef CAS.
- M. Schmidt, C. Sachse, W. Richter, C. Xu, M. Fandrich and N. Grigorieff, Proc. Natl. Acad. Sci. U. S. A., 2009, 106, 19813–19818 CrossRef CAS.
- P. C. Ke, E. H. Pilkington, Y. Sun, I. Javed, A. Kakinen, G. Peng, F. Ding and T. P. Davis, Adv. Mater., 2020, 32, 1901690 CrossRef CAS.
Footnote |
† Electronic supplementary information (ESI) available: Characterization of GQDs (Fig. S1), effect of GQDs on the fluidity of SH-SY5Y cells (Fig. S2), distribution of Aβ-o in the presence of GQDs (Fig. S3), distribution of Aβ-o (Fig. S4) and GQDs (Fig. S5) on SH-SY5Y cells, secondary structure propensities of each Aβ-m (Fig. S6) and Aβ-o (Fig. S7) residue in the absence and presence of a GQD, and distance probability distribution of each Aβ-o residue relative to the GQDs (Fig. S8). See DOI: 10.1039/d1cp04246g |
|
This journal is © the Owner Societies 2022 |