DOI:
10.1039/D1CB00190F
(Review Article)
RSC Chem. Biol., 2022,
3, 201-219
Molecular probes for cellular imaging of post-translational proteoforms
Received
29th September 2021
, Accepted 4th January 2022
First published on 4th January 2022
Abstract
Specific post-translational modification (PTM) states of a protein affect its property and function; understanding their dynamics in cells would provide deep insight into diverse signaling pathways and biological processes. However, it is not trivial to visualize post-translational modifications in a protein- and site-specific manner, especially in a living-cell context. Herein, we review recent advances in the development of molecular imaging tools to detect diverse classes of post-translational proteoforms in individual cells, and their applications in studying precise roles of PTMs in regulating the function of cellular proteins.
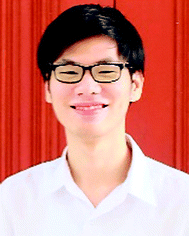
Surased Suraritdechachai
| Surased Suraritdechachai received his BSc (2017) and MSc (2019) in chemistry from Chulalongkorn University, Thailand. In 2020, he joined Vidyasirimedhi Institute of Science and Technology (VISTEC), Thailand, as a research assistant in Dr Chayasith Uttamapinant's team. At VISTEC, his research focuses on the development of molecular and chemical biology tools to study spatiotemporal control of protein expression. |
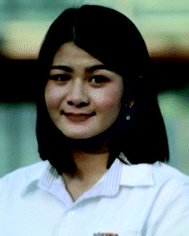
Benya Lakkanasirorat
| Benya Lakkanasirorat earned her BSc in biology (2018) from Chulalongkorn University, Thailand, and is currently a doctoral student under the supervision of Dr Chayasith Uttamapinant at VISTEC. Her projects in the Uttamapinant lab revolve around enzyme directed evolution for protein and proteoform detection using surface display technologies. |
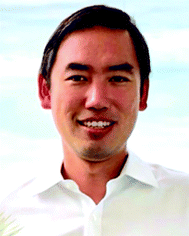
Chayasith Uttamapinant
| Dr Chayasith Uttamapinant is a chemical biologist with broad interests in biomolecular detection. He trained with Prof. Alice Ting at Massachusetts Institute of Technology, United States, and Prof. Jason Chin at Medical Research Council, Laboratory of Molecular Biology, United Kingdom, before joining VISTEC, Thailand, in 2018 as a lecturer. His lab is developing new tools to characterize locally synthesized proteins and proteoforms as well as open-source molecular diagnostic tools. |
1. Introduction
Linking a precise protein form to its property and function in the crowded cellular context remains an outstanding challenge in biological research. While proteins can change conformations or form transient contacts with cellular macromolecules without change to their chemical compositions, they often need to be post-translationally modified to elicit function. Such post-translational modifications (PTMs) create chemically or compositionally diverse forms of a single protein—or proteoforms1—and are intricately controlled in space and time within the cell, diversifying the forms and functions of proteins in different contexts. In cell biological research, PTMs and proteoforms are often studied for their roles in controlling complex signaling and regulatory networks. Hundreds of PTM types are now known (and catalogued in databases like Unimod2) and proteins can contain multiple PTMs, creating a staggering number of heterogenous proteoforms that are only theoretically limited by protein copy numbers within the cell.3
Proteoforms can be discovered systems-wide via top-down proteomic technologies,4 in which intact proteoforms are analyzed in whole without digestion to peptides. Due to inherently insensitive measurements of intact protein masses and low abundance of many proteoforms in cells, systems-level detection of proteoforms may need enrichment strategies5,6 for specifically modified proteomes (e.g. for phosphoproteomes7 and for proteolytic proteomes8,9). More popular bottom-up proteomic approaches with digested peptides can also be used for proteoform detection upon coupling to appropriate peptide assignment algorithms such as correlation-based functional proteoform assessment.10 Subcellular information of proteoforms is often not retained in mass spectrometry-based proteomic studies unless the investigators employ cellular fractionation11 or proximity labeling strategies12 to selectively enrich proteomes from a given location within the cell. Collectively, these technologies enable global and subcellular profiling of bona fide proteoforms for further functional characterizations.
Technologies to synthesize proteoforms have enabled their functional characterizations in vitro and recently, in cells. As many post-translational modifications are catalyzed by enzymes, enzyme-mediated approaches to site-specifically install PTMs are commonly used. However, this requires prior knowledge and means of production of enzymes responsible for a particular PTM. Several PTM enzymes, including many kinases and glycosyltransferases, also modify multiple sites on the same protein, creating a heterogenous mix of proteoforms and rendering characterizations of individual proteoforms difficult. To access homogenous proteoforms, protein semisynthesis13 or genetic code expansion can be used. In particular, genetic code expansion can incorporate PTM-modified amino acids (phosphorylated,14–16 methylated,17,18 acetylated,19 ubiquitinated,20,21etc.) to any user-defined site on a given protein, allowing greater flexibility in studying site-specific functions of PTMs than protein semisynthesis. Recently, protein-specific PTM installations mediated by enzymes (e.g. for glycoform synthesis22), by genetic code expansion,23 by bifunctional chimera molecules,24–26 and by precise electrophile and oxidant delivery27,28 have all been accomplished in living mammalian cells, allowing roles of protein- or site-specific PTMs in regulating protein function and signaling to be established.
While proteoform synthesis methods are immensely useful, they artificially introduce PTMs onto proteins and cannot be used to study native (and often reversible) formation and regulation of proteoforms. Cell-based imaging methods to probe the formation and dynamics of post-translational proteoforms are therefore needed and would provide insight unavailable to ex cellulo systems and biochemical characterizations. For example, DNA damage triggers complex interactions of PTMs (including phosphorylation, acetylation, methylation, ubiquitination, and SUMOylation) on histones and other chromatin-associated proteins, resulting in the proteins’ degradation and trafficking to and from the DNA damage sites in the cell.29 Obtaining the spatial and temporal information of proteoforms is crucial to understanding their cellular properties (e.g. stability, translocation, interaction, catalytic activity), and ultimately, how they function in coordinating DNA damage responses.
PTM dynamics can be tracked in real time via monitoring the subcellular activity of post-translational modifying enzymes (kinases/phosphatases, methyltransferases, and glycosyltransferases) using a suite of Förster resonance energy transfer (FRET)-based biosensors containing surrogate substrates for the enzymes; efforts to create this class of technologies to probe diverse biological systems have been reviewed.30 However, complementary molecular tools which enable direct visualization of PTM dynamics on desired protein targets are not as well-developed. While proteins can be easily genetically tagged for visualization, most PTMs are non-genetically encoded chemical modifiers which cannot be genetically tagged. Visualizing PTM placements on specific proteins therefore often require hybrid (bio)chemical-genetic and other innovative approaches to create the desired labeling specificity.
In this review, we discuss the available molecular tools for cellular imaging of post-translational proteoforms. We classify post-translational proteoform labeling methods based on different chemical nature of the modifications: addition of small chemical groups to protein side chains; protein–protein linkage creation; and protein cleavage (Fig. 1). Methods to visualize proteins modified with chemical groups are the most diverse; we further categorize them into technologies which provide site-specific information vs. those which indicate PTMs on proteins based on proximity-induced signals. As many chemical modifications on proteins are far from simple—glycosylation and ubiquitination in particular are highly complex in their structure and composition—we examine available technologies to partly address this complexity. In addition, we reviewed limited tools available for the detection of proteoforms containing non-enzymatic PTMs. Since all current probes for proteoform detection have significant—and different—limitations, we address these limitations and suggest a guideline for experimental validations of the probes when applied to proteins/systems of interest. Lastly, we discuss platforms for high-throughput and multiplexed protein imaging, which can be readily applied to imaging of cellular proteoforms, once suitable molecular probes for proteoform detection are in place.
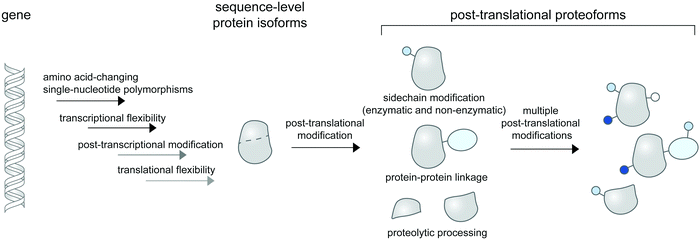 |
| Fig. 1 Post-translational proteoforms. Multiple sources of proteoforms exist: a gene can have single-nucleotide polymorphisms (SNPs) in its coding region; it can give rise to multiple RNA isoforms through splicing; and each mRNA form may produce multiple protein sequences through non-canonical translation initiation and termination. Thereafter, each protein sequence can be post-translationally modified—through addition of diverse chemical groups to its amino acid side chains (top), linkage to other proteins (middle), or proteolytic cleavage (bottom)—to generate post-translational proteoforms. Multiple modifications of a base protein sequence create a complex landscape of post-translational proteoforms in cells. | |
2. Molecular tools to detect proteoforms involving addition of functional groups
2.1 Site- and PTM-specific antibodies and binders
Specific antibodies for defined PTM states of a given protein can be developed (Fig. 2a),31,32 most commonly through animal immunization with a synthetic peptide segment from the protein target modified with the desired site-specific PTM. Beyond immunization, directed evolution techniques that select for binders with high affinity can be used to create antibodies and binders with desired specificity. If succeeded, such reagents provide the most direct route to the detection of post-translational proteoforms in cells. A large number of site-specific antibodies for phosphorylation, methylation, and acetylation are now commercially available. Alternative proteoform-specific binders with smaller size than traditional antibodies have also been developed (Fig. 2b). For instance, the Kimura group developed FabLEM (Fab-based live endogenous modification labeling) to visualize histone modification.33,34 Compared to monoclonal immunoglobulin G (IgG, ∼150 kDa), Fab fragments are smaller (∼50 kDa), diffuse faster and possess capability to pass through the nuclear membranes while retaining the recognition specificity. The FabLEM method is applicable to detection of several histone modifications34–36 including methylation, acetylation, and phosphorylation. It has also been used to monitor phosphorylation of RNA polymerase II (RNAP2) under different polymerase activity states in cultured cells.37,38 Histone and RNAP2 proteoforms could be monitored simultaneously: after activation of the glucocorticoid receptor transcription factor, a decrease in histone acetylation was observed followed by an increase in RNAP2 phosphorylation levels, indicating progression to transcription elongation. In conjunction with MS2-based nascent mRNA tagging, the Fab fragment could be used to monitor specific phosphorylation states of RNAP2 as it transcribes a single gene.38
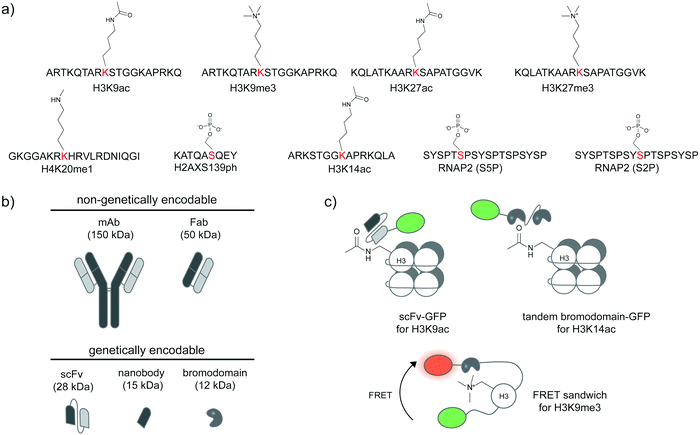 |
| Fig. 2 Detection of post-translational proteoforms by site-specific antibodies and binding proteins. (a) Examples of post-translational epitopes detectable by proteoform-specific antibodies and binders. Anti-H3K9ac from ref. 34; anti-H3K9me3 from ref. 34; anti-H3K27ac from ref. 34; anti-H3K27me3 from ref. 34; anti-H4K20me1 from ref. 32; anti-H2AXS139ph from ref. 39; anti-H3K14ac from ref. 40; anti-RNAP2 (S5P); anti-RNAP2 (S2P) from ref. 37. (b) Comparison of size and genetic targeting properties of antibody- and affinity-based reagents. (c) Genetically encoded detection of proteoforms by single-chain variable fragments (scFv), bromodomains, and FRET sandwiches. Proteoform-targeting moieties such as a scFv targeting H3K9ac41 and a bromodomain targeting H3K14ac40 are fused to reporters, such as green fluorescent protein (GFP). FRET sandwiches contain a fluorescent protein FRET pair and a PTM reader domain.42 | |
As direct loading of Fab fragments cannot provide sustained amount of Fab after rounds of cell division, thereby limiting long-term monitoring of proteoform states,33 the Kimura group further developed a genetically encodable fusion of a single-chain variable fragment (scFv, 28 kDa) and green fluorescent protein (GFP) (Fig. 2b), called modification-specific intracellular antibody or mintbody. In the first report, a mintbody for H3K9 acetylation allowed monitoring of increased acetylation levels in response to a histone deacetylase inhibitor (Fig. 2c).41 For in vivo applications, the H3K9 acetylation mintbody could monitor changes in acetylation levels during embryogenesis of transgenic Drosophila and zebrafish embryo,41 and also during Xenopus tail regeneration.43 Mintbody for H4K20me1 detection has also been developed to specifically monitor histone monomethylation in several organisms including Schizosaccharomyces pombe yeast, mammalian cell lines, and Caenorhabditis elegans.44,45
Single variable heavy-chain domain of antibodies (VHH), or nanobodies, are even smaller in size (15 kDa) than Fab or scFv, but elicit highly specific antigen binding compared to conventional antibodies. Derived from monomeric immunoglobulins found in camel and shark species,46 nanobodies can be expressed recombinantly in cells and act as intracellular antibodies similarly to scFv (Fig. 2b), but are more monomeric and stable inside cells than scFv.47 Various types of nanobody libraries can be prepared, and coupled to diverse selection methods—in particular to surface display technologies—to discover nanobodies with desired specificity.48,49 Nanobodies have been developed to detect specific proteoforms.50–52 A recent example is a phage display-derived nanobody specific for human H2AX histone phosphorylated at position Ser139, or γ-H2AX.39 A bivalent variant of this nanobody can be expressed recombinantly as a fluorescent protein fusion in a human lung carcinoma H1299 cell line, and used to visualize the formation of γ-H2AX foci in the cell nucleus, in response to genotoxic drug treatment and the resulting DNA replication stress.
Beyond antibody-based detection, several protein domains that bind histone PTMs with sequence specificity have been identified. For instance, a bromodomain can bind specifically to specific acetylated lysines on histones in live cells.53 A GFP-fused bromodomain can be used to visualize endogenous histone acetylation (Fig. 2c).40 The fluorescence signal from the bromodomain-based sensor colocalized with the H3K14ac antibody and increased in response to histone deacetylase inhibition. The sensor for histone H3K9me3 based on a chromodomain was also reported.54 These sensors could be coupled to a DNA-binding zinc finger domain to monitor locus-specific H3K9me3 modification,55 or to other histone reader domains to simultaneously report multiple histone modifications,56,57 or with a methyl binding domain to simultaneously visualize 5-methylcytosine.57
Further strategies to visualize histone proteoforms involve creation of FRET-based sandwich sensors through strategic fusion of the PTM recognition domain and the histone protein in between a fluorescent protein FRET pair (Fig. 2c). When the fused histone protein has high PTM levels, the histone reader domain folds to bind the modified histone, resulting in a change in FRET efficiency.42,58–61 This approach is applicable to live-cell monitoring of histone modifications including methylation, acetylation, and phosphorylation. A significant caveat is regulation of PTMs on histones embedded in these complex FRET sandwich sensors may differ from that of PTMs on endogenous histones.40
Beyond histone proteoforms, antibody-based reagents can be used to detect phosphorylated tau protein. Highly specific scFv for tau phosphorylated at Thr231 was evolved using yeast surface display, and has enabled phosphotau labeling in fixed cells and human brain tissues.62
2.2 Limitations of site-specific antibodies and binders
The development of antibody-based reagents is not trivial, can be costly and time-consuming, and is generally applicable to only a subset of PTMs with small, well-defined, homogeneous chemical structures (e.g. a phosphate group, a methyl group, or an acetyl group) which could act as a composite epitope along with the protein sequences surrounding the PTM for antibody recognition. Many small PTMs—for example the multiple states of non-enzymatic cysteine oxidation—cannot be easily recapitulated on synthetic peptides, making epitope creation for antibody selection difficult. As there is a limit to the epitope size recognizable by antibody-based reagents (typically 4–12 amino acids for protein sequences/patches63), developing site-specific antibody-based reagents for large PTM structures is even more strenuous; to date we have only seen few examples of site-specific antibodies for lipidated proteins (for palmitoylated PSD-9564) and none for glycosylated proteins.
Proteoform-specific antibodies and Fab fragments can be used for fixed-cell immunofluorescence but are seldom applicable to routine live-cell imaging due to their large size and cell impermeability. Such live-cell imaging studies to visualize post-translationally modified histones and transcription factors34,36,37,65 necessitate the use of invasive antibody delivery approaches such as microinjection, bead loading or electroporation.
The varying quality of commercial antibody-based reagents or their in-house development necessitate rigorous validation that the reagents can detect proteoforms with high specificity. While clear co-localization of signals from the immunostained proteoform with the signal from its parent protein is a minimal specificity threshold, we advocate for further validations using orthogonal approaches such as: genetic knockdowns of the target protein or the PTM-generating enzyme; mutagenesis of the PTM site on the protein; or chemical treatments known to modulate PTM levels on proteins of interest. For example, trichostatin A, a known histone deacetylase inhibitor, could be used to globally raise histone acetylation levels. Moreover, while intracellular expression of scFv, nanobodies, and protein domains has clear utility in monitoring specific proteoforms in live cells, one needs to finely tune the expression level of the reporter to reduce background from its off-target fraction.40
2.3 Proximity-mediated detection of proteoforms
In most cases, an antibody or binding protein for site-specific post-translational proteoforms is not available. The more generalizable approach to detect post-translational proteoforms thus is to use dual labeling: one for the protein target, and the other for the desired PTM. The proximity of the two signals is then used as an indicator of a PTM on the specific protein. For proximity-based detection of proteoforms, researchers must make three levels of choices: a protein labeling strategy; a PTM labeling strategy; and a mechanism to indicate the PTM is proximal (intramolecular) to the target protein. Here, we discuss the characteristics of the two most common proximity-induced strategies—Förster resonance energy transfer (FRET) and proximity ligation assay (PLA) in inferring PTMs on proteins, and how the choice of proximity-induced strategies may affect PTM labeling strategies. We do not discuss protein labeling in detail but provide examples of diverse strategies throughout the section; particularly, different strategies to detect proteins of interest in combination with metabolic labeling are summarized in Fig. 3.
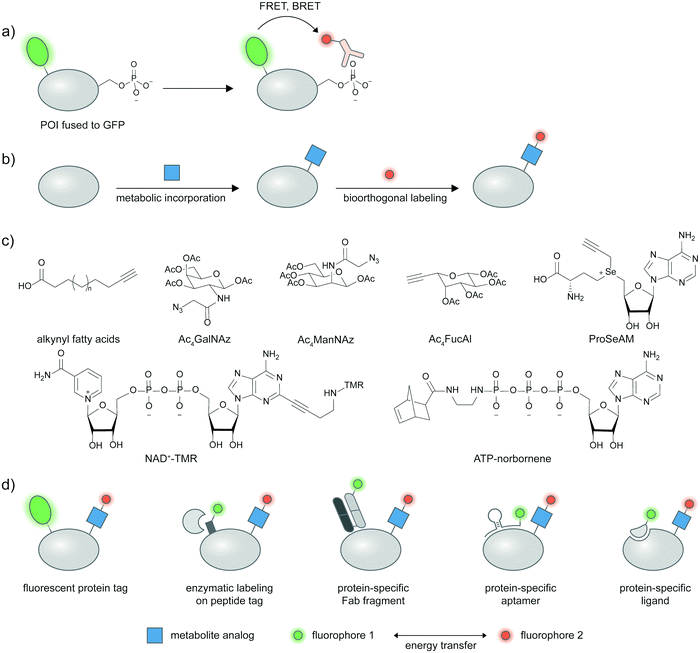 |
| Fig. 3 FRET based-proteoform detection via PTM-specific antibodies and metabolic labeling. (a) FRET-based detection of proteoforms via PTM-specific antibodies and a protein marker. Pan Antibodies for PTMs such as phosphoserine and phosphotyrosine are available, and can be coupled to a protein marker such as GFP and protein-specific antibodies for proteoform detection via proximity-induced Förster or bioluminescence resonance energy transfer (FRET or BRET). (b) General scheme for metabolic labeling on proteins. A clickable PTM substrate analog (blue square) is metabolically incorporated into cellular proteins via endogenous metabolic pathways. A click handle on PTM-bearing proteins is then chemically derivatized with a probe of interest (red circle) via bioorthogonal chemistry. (c) Examples of metabolic probes used for proteoform detection. Ac4GalNAz: tetraacetylated N-azidoacetyl galactosamine. Ac4ManNAz: tetraacetylated N-azidoacetyl mannosamine. Ac4FucAI: tetraacetylated 6-alkynyl fucose. ProSeAM: propargylic Se-adenosyl-L-selenomethionine. NAD+–TMR: nicotinamide adenine dinucleotide–tetramethylrhodamine conjugate. (d) Different strategies for specific protein tagging can be coupled to metabolic labeling to produce proteoform detection: fluorescent protein tagging; enzyme-mediated labeling of peptide tags; protein-specific Fab fragments; protein-specific aptamers; or protein-specific ligands. | |
2.4 FRET-based proteoform detection
FRET and PLA are most commonly employed to generate proximity-induced signals for proteoform detection. In FRET, the dipole-mediated resonance energy transfer between a donor and an acceptor fluorophore has strong distance dependence (r−6), enabling the efficiency of transfer to indicate the distance between—in our case—the label on the protein and the label on the PTM. The average diameter of proteins is ∼5 nm,66 acting as the upper limit of the PTM-protein label distance. Most FRET pairs have Förster radii (R0) of 2–10 nm, with a few specialized FRET pairs with even smaller R0 (down to 0.6–1 nm,67,68 though these have not been used in cell-based FRET assays). With suitable FRET pairs and protein/PTM labeling strategies that do not add much “bulk”, FRET should provide intra-protein resolution needed to infer the presence of a proteoform (i.e. the PTM and the protein are intramolecular). To complement energy transfer measurements, fluorescence correlation microscopy (FCS) can be used to assess correlated movement of the protein and PTM signals in live cells and provide further evidence of PTM–protein intramolecularity.
2.5 FRET-based proteoform detection with PTM-specific antibody-based reagents
In lieu of a site-specific antibody, researchers can use an antibody broadly specific to a desired post-translation modification in combination with a protein labeling strategy, and assess the extent of energy transfer between the protein and PTM labels as a proxy for proteoform presence. Pan-specific antibodies for phosphorylated residues (Ser, Thr, Tyr), different methylated lysine states, and acetylated lysine are widely commercially available. A pan-phosphohistidine antibody was reported.69 Antibodies for a range of carbohydrate epitopes are available, albeit with lower affinities than traditional antibodies against proteins/peptides.70 Antibodies against hydrophobic modifications like lipid PTMs are notoriously difficult to generate, though a pan-palmitoylation antibody was reported.71
Under such a FRET-based readout (Fig. 3a), the proximity-induced FRET signal of a labeled antibody and GFP-fused proteins has allowed visualization of phosphorylation in a protein-specific manner.72–74 In the seminal paper by the Bastiaens group, a GFP-fused epithelial growth factor receptor (EGFR) was recombinantly expressed in MCF7 cells and a cy3-labeled Fab fragment specific for phosphotyrosine was microinjected into the cells.72,73 FRET occurred when EGFR was phosphorylated in response to an externally supplied EGF ligand and measured via the fluorescence lifetime imaging technique (FLIM)-FRET.
2.6 FRET-based proteoform detection with metabolic labeling
In metabolic labeling, synthetic PTM substrate analogues are modified onto proteins by endogenous metabolic pathways. A biorthogonal functional handle on the metabolite analogues—such as an alkyne or an azide—can then undergo click chemistry derivatization with a fluorophore conjugate to provide detectable signal (Fig. 3b). Various PTMs can be labeled metabolically including lipidation, acetylation, and glycosylation.75,76 Combining the signal from metabolic labeling with a protein identification strategy allows imaging of these PTMs in a protein-specific manner. Structures of metabolic probes from studies highlighted below are shown in Fig. 3c, and different strategies to detect proteins of interest are summarized in Fig. 3d.
A fluorescent protein fusion tag can readily be coupled to metabolic labeling (Fig. 3d), and the proximity of the metabolic tag and the protein can be read through FRET signal. For example, a glucose transporter GLUT4–EGFP fusion was metabolically labeled with Ac4ManNAz and showed a trans-membrane FRET signal after the Ac4ManNAz moiety was derivatized with a rhodamine–alkyne.77 This enabled live-cell monitoring of accumulation of sialylated GLUT4 after insulin introduction, and internalization of sialylated GLUT4 after insulin removal. Glycosylation of cytosolic proteins such as tau, OGT, and Akt1 could be visualized in a similar manner using FLIM-FRET.78,79 Investigating methylation on GFP-fused proteins of interest is also possible through the use of a propargyl Se-adenosyl methionine (ProSeAM) analogue (Fig. 3c) and subsequent cy3–azide conjugation.80 SAM-based labeling has been applied to study the subcellular localization of Foxo1 which is affected by its methylation state, via FLIM-FRET. Poly(ADP-ribos)ylation of ARTD protein was also reported using EGFP fusion and a fluorophore conjugated NAD+ analog (Fig. 3c).81
To avoid interference to protein function from the large GFP tag, a strategy that uses a smaller tag such as LplA acceptor peptide (LAP) and enzymatic labeling has been developed (Fig. 3d).82,83 The LAP tag is recognized by lipoic acid ligase (LplA) mutants capable of covalently linking diverse small-molecule probes onto the tag. Using dual labeling with a sugar analogue Ac4ManNAl and an azide-containing LplA substrate, and subsequent bioorthogonal conjugation of a FRET donor and acceptor pair, sialylation of integrin αXβ2, EGFR, and growth factor-beta receptor type I (TβRI) could be specifically imaged in live cells. The authors highlighted the advantage of the smaller LAP tag in placing the fluorophore closer to the labeled glycan moiety in comparison to an EGFP fusion, resulting in higher FRET efficiency.
Live cell-compatible endogenous protein labeling techniques can also be used in combination with metabolic labeling to investigate proteoforms (Fig. 3d). An external Fab fragment conjugated to a fluorophore was used to visualize cell–surface integrin αVβ3. Metabolic labelling with Ac4ManNAz and subsequent derivatization with a cyclooctyne probe allows specific imaging of the sialylated protein via FRET.84 Exploiting native protein–protein interactions, labeled interleukin protein was used to tag a FRET donor to a surface protein interleukin 36 receptor while a FRET acceptor was installed via metabolic labeling with Ac4ManNAz (Fig. 3d).85
Through Systematic Evolution of Ligands by Exponential Enrichment (SELEX), nucleic acid aptamers can be evolved to bind a protein target with high affinity and specificity (Fig. 3d).86,87 Protein tyrosine kinase 7 (PTK7), a cell–surface protein overexpressed in subtypes of leukemia, could be recognized by an aptamer hybridized with a cy3-labeled oligonucleotide. The sialylation was probed by Ac4ManNAz and click derivatization with cy5–alkyne,88 enabling the resulting cy3–cy5 FRET signal to indicate sialylated PTK7. The FRET signal between cy3 and cy5 could be further enhanced through metal enhanced fluorescence, using a silver nanoparticle-functionalized aptamer.89
Expansion of metabolic probes for more types of PTMs would further enrich proteoform studies. In a recent example, a cell-permeable ATP analog bearing a norbornene group at the gamma-position was reported as a novel metabolic labeling tool for phosphorylation (Fig. 3c).90 The transfer of the phospho-norbornene group by purified or cellular kinases (the latter demonstrated via cell lysates) was confirmed in vitro on a peptide substrate, and in cellulo on murine double minute protein (MDM)–EGFP fusion. The norbornene appendage can then be chemically derivatized with tetrazine–probe conjugates e.g. tetrazine–cy3. The FRET signal between EGFP and cy3 informs phosphorylation extent on MDM and can be used to assess effects of phosphatase inhibitors on MDM phosphorylation.
2.7 Limitations of metabolic labeling
The major caveat with metabolic probes, especially ones with significantly altered or bulky motifs, is their potential interference with the native function of the PTM, and of the protein being modified; functional assays are therefore needed to validate the use of these analogs. For instance, some fluorescently labeled ATP analogs are known to have reduced activity with ATP-processing enzymes.91 The ProSeAM SAM analog was carefully characterized and found to be accepted by only a subset of lysine methyltransferases, despite its small propargyl modification.92–94 Beyond effects on PTM transfer processes, functional effects on the receiving end—the proteoform—must be carefully characterized. Continual developments to create minimally perturbative tools to label PTMs (for instance, the development of isosteric fluorinated cofactors taggable by the fluorine-thiol displacement reaction95) are crucial to the characterization of native proteoform function.
Metabolic probes can perturb the physiological relevance of intended studies through disruption of metabolic balance and gross toxicity to cells;96 researchers should keep in mind to assess cellular toxicity or gross effects of the metabolic probe and titrate its dose accordingly. Some metabolite analogs such as ProSeAM and the NAD+ analog are not membrane-permeable and must be loaded invasively via liposomes or electroporation.80,81 Efforts to engineer biosynthetic pathways to create cell-impermeant metabolite analogs intracellularly from cell-permeant precursors are promising,97,98 although these have not yet been applied to proteoform detection.
The orthogonality of certain metabolite analogs with respect to endogenous enzymatic processing has enabled cell-selective PTM and proteoform tagging, through cell-selective expression of engineered PTM transferase enzymes which can utilize these analogs. This bump-and-hole strategy to create orthogonal enzyme–metabolite analog pairs has been applied to methyltransferase,92,99 acetyltransferase,100 and GalNAc transferase enzymes.101
2.8 PLA-based proteoform detection
A standard proximity ligation assay (PLA) labels two targets using a pair of antibodies labeled with oligonucleotides (Fig. 4a).102,103 In proximity, the two tailored oligonucleotides can hybridize with a DNA template and ligated by T4 DNA ligase to form a circular DNA. This circular DNA acts as a template for rolling-circle amplification (RCA) to form a massively extended DNA product, which can be sensitively visualized by hybridization with a fluorophore-labeled oligonucleotide. PLA is versatile and has been widely used to visualize protein–protein interactions103,104 and post-translational proteoforms, the latter through the use of one antibody targeting the protein and the other targeting the PTM.105 Beyond antibody-mediated labeling, PLA can be coupled with other protein and PTM labeling strategies, provided that these strategies enable linkage of the PLA oligonucleotides to moieties of interest.
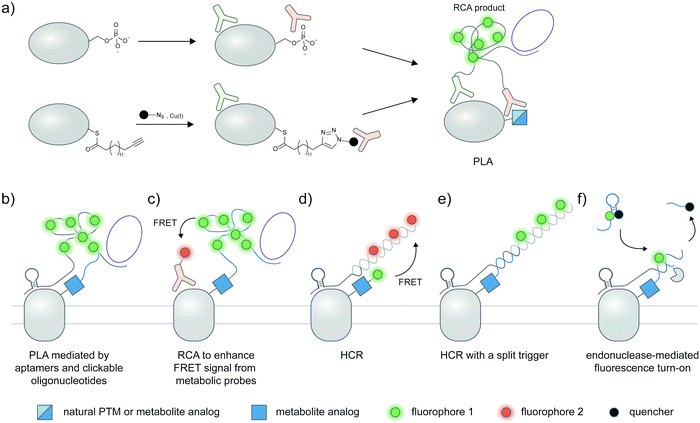 |
| Fig. 4 Strategies to amplify signal with nucleic acid probes for proteoform detection. (a) Proximity ligation assay (PLA) typically uses two oligonucleotide-labeled antibodies—one for the desired protein, and the other for the desired PTM—for proteoform detection. Connector oligos are used to join the oligonucleotide probes in proximity, the complex of which is then covalently linked by a DNA ligase to generate a circular DNA template for rolling circle amplification (RCA). The long single-stranded RCA product is then hybridized with fluorophore-labeled oligonucleotides. PTM antibodies for PLA can be pan-PTM antibodies (top path). Antibody recruitment to the PTM site can also be mediated by metabolic labeling and click derivatization (bottom path). (b) Protein-specific aptamers are also used to attach PLA oligonucleotides, and coupled with clickable oligonucleotides installed via metabolic labeling. (c) RCA can be used to specifically amplify the signal on the PTM marker, allowing more sensitive detection of the proteoform upon coupling to FRET.106 (d) Hybridization chain reaction (HCR) initiates assembly of labeled oligonucleotides via a trigger sequence at the aptamer-binding site on a specific protein. Such an extended assembly serves as an efficient FRET acceptor to a FRET donor installed via metabolic labeling.107 (e) HCR with a split trigger.108 Here, the trigger to initiate hybridization is split: one half is placed on the protein label (via an aptamer); the other half is placed on the PTM label (via metabolic then click labeling). Proximity-dependent reconstitution of trigger halves initiates hybridization. (f) A filter beacon architecture based on hybridization of oligonucleotides directed to a specific protein (via aptamers) and a PTM (via metabolic then click labeling).109 The hybridized sequence serves as a recognition site for a nicking endonuclease, resulting in cleavage and release of a fluorescence quencher and generation of fluorescence signal. | |
2.9 PLA-based proteoform detection with PTM-specific antibody-based reagents
PLA has been used to assess the phosphorylation extent of several proteins including ERK2 and SHC in K562 cells via a combination of the pan-phosphotyrosine antibody and several antibodies for target proteins (Fig. 4a, top).110 In another work, PLA with pan-phosphoserine antibody was used to study the regulation of phosphorylation of an endothelial transcription factor EGR by angiopoietin I activation of the PI3K/Akt pathway.111 In addition to cell lines, PLA can be performed in tissue sections to image endogenous phosphorylated proteins.112,113 Beyond phosphorylation, PLA has been used to monitor other protein-specific PTMs such as methylation of glucocorticoid receptor114 and acetylation of a transcription factor Foxp3.115
PLA can be further coupled to imaging flow cytometry, in a method called proximity ligation imaging cytometry (PLIC),116 to simultaneously sort cell populations while providing subcellular localization information of specific proteoforms. In this work, PLIC was used to assess levels of acetylation of Aire protein in mouse medullary thymic epithelial cells (mTECs), which are low-abundant cell populations in thymus tissue, using anti-Aire and anti-acetylated lysine antibodies.
2.10 PLA-based proteoform detection with metabolic labeling
Hannousch et al. reported a method to image palmitoylation of Wnt protein in mouse fibroblast L cells.117 Cells expressing Wnt protein were treated with 15-hexadecynoic acid (Fig. 3c), a clickable palmitoic acid analogue, then labeled with either biotin or Oregon Green to be recognized by an antibody. A palmitoylated Wnt signal was generated by proximity ligation assay from antibody targeting the tag and the protein (Fig. 4a, bottom), allowing the researchers to visualize the palmitoylated protein as it is trafficked through the secretory pathway. Later, the method was applied to study palmitoylation of sonic hedgehog, tubulin, and H-Ras in mammalian cells.118 PLA is compatible with tagging of various sugar analogues and has enabled detection of EGFR sialylation, EGFR fucosylation, and GalNAcylation on MUC1, a membrane protein known to be highly glycosylated.119
2.11 Limitations of PLA for proteoform detection
The reported spatial resolution of PLA is ∼40 nm, based on the approximate dimensions of two primary antibodies and the bridging oligonucleotide probes104 (we have not seen experimental measurements of spatial resolution provided by PLA). Such spatial resolution is simply not sufficient for intramolecularity between the PTM and the protein to be inferred. In most PLA setups for proteoform detection, the resolution is exacerbated through the use of two-tiered (primary and secondary) antibodies, degrading the spatial resolution of signal generation by further ∼30 nm. Rigorous validations are therefore needed when PLA is used for proteoform detection. Variations of PLA and other nucleic acid-based techniques with potentially improved spatial resolution are discussed in the next section.
Due to the exponential nature of nucleic acid amplification, PLA signals can suffer from saturation effects and are generally only semi-quantitative.120 Researchers should exercise caution when making quantitative interpretation (e.g. changes in PTM levels of proteins upon cell stimulation) using PLA signals. The punctate appearance of PLA signals—from individual massive RCA amplicons—reflects that only a fraction of proteoform molecules of interest is labeled; this is due to the stochastic nature of the enzymatic DNA amplification process.120 PLA is also limited to fixed cells due to the use of antibodies, PLA reaction conditions, and hybridization-based labeling conditions.
2.12 Variations of PLA for proteoform detection
Instead of bulky antibodies, oligonucleotides for PLA can be directed to protein targets via DNA aptamers, or to PTM targets via clickable metabolic probes. Since aptamers (∼2 nm)121 are smaller than antibodies, such combined use of smaller tags obviates the ∼30–60 nm uncertainty added by antibodies and may permit visualization of intramolecular PTM/protein relationships. In an example from the Xie group, PD-L1 is labeled with a protein-specific aptamer, metabolically labeled with Ac4ManNAz, and click-derivatized with an oligonucleotide probe (Fig. 4b).122 Proximity of the aptamer and the probe allowed ligation of additional oligonucleotides to generate circular DNA for subsequent RCA amplification. With this strategy, the researchers were able to distinguish MDA-MB-231 cells positive for glycosylated PD-L1 from PD-L1-negative BT-474 cells via confocal microscopy and FACS.
FRET can be used in conjunction with RCA to take advantage of the strengths of both proximity-induced techniques: FRET provides intra-protein resolution of proteoform imaging, while RCA allows multiple labeling of FRET donors/acceptors so detection sensitivity is enhanced. Here, the oligonucleotide installed on the PTM-containing protein via a metabolic probe was designed to hybridize with a DNA padlock probe; a ligation reaction then generated a circular DNA template for subsequent RCA and hybridization of donor fluorophore probes (Fig. 4c). Employing a protein-specific antibody conjugated to a FRET acceptor fluorophore, this approach was used to image GalNAcylation of endogenous MUC1 in MCF-7 cells and HA-tagged GPC3 in HEK293T;106,123 in both cases the FRET signal was increased upon performing RCA.
2.13 Proteoform detection with hybridization chain reaction and endonuclease-mediated filter beacon
Recently, a hybridization chain reaction (HCR) was used to probe protein-specific glycosylation on the cell surface. This technique is an enzyme-free signal amplification based on hybridization of two hairpin nucleotides that is partly complementary to each other (Fig. 4d).124,125 The trigger DNA hybridizes with one hairpin DNA, exposing an overhang that is complementary to another hairpin DNA. A series of hybridization events leads to the amplification of the fluorescent signal provided that one DNA hairpin is labeled with a fluorophore.
HCR was recently demonstrated by the Wang group as a method to detect sialylated PTK7. An aptamer sgc8 with a trigger sequence was designed to target PTK7. The protein can undergo metabolic labeling with Ac4ManNAz to install a FRET donor, followed by HCR with a hairpin DNA FRET acceptor, allowing the FRET signal from sialylated PTK7 to be detected.107 The same group later developed an assay to visualize sialylated PTK7 using a split trigger with one half on the aptamer probe and the other half on the clickable DNA (Fig. 4e).108 The hybridization of the hairpin for HCR occurs when the split triggers are in proximity. This approach helps reduce non-specific amplification and bypass the need for FRET, which generally provides low signal and dynamic range. The trigger sequence could also be protected within a hairpin structure, which can be unmasked upon hybridization with an oligonucleotide installed by metabolic labeling to allow HCR.126 This approach was used for fluorescence detection of sialylated proteins in cell lines, zebrafish larvae,126 and in a tumor model in BALB/c mice, the latter through coupling with photoacoustic imaging.127
In another DNA-based approach, the Ding group reported an elaborately designed clickable molecular beacon probe which could be installed via Ac4ManNAz metabolic labeling to visualize sialylation on MUC1 (Fig. 4f). Here the glycoform was detected via a MUC1-specific aptamer, which could hybridize with a Ac4ManNAz-directed clickable oligonucleotide. Hybridization generated a recognition site for a nicking endonuclease, resulting in cleavage and release of a fluorescence quencher appended to the clickable oligonucleotide.109 In another work, an aptamer-assisted labeling was demonstrated using an aptamer hybridized with a fluorophore-labeled clickable oligonucleotide.128 Such assisted labeling of a mesenchymal–epidermal transition factor (MET) metabolically labeled with Ac4ManNAz showed higher fluorescent signal compared to unassisted labeling.
3. Detection of proteins modified with ubiquitin and ubiquitin-like proteins
Covalent modifications of cellular proteins with a small, 8 kD ubiquitin (Ub) protein trigger proteasome-mediated degradation as well as other proteasome-independent cellular processes. Beyond ubiquitin, proteins can be covalently linked to other ubiquitin-like proteins such as Small Ubiquitin-like Modifier (SUMO) and Neural precursor cell Expressed, Developmentally Down-regulated 8 (NEDD8).129 Strategies to visualize Ub/Ub-like modifications for cellular imaging have been reviewed.130 In general, FRET,131,132 BRET133 or PLA-based proximal detection134–136 based on dual labeling of the Ub/Ub-like proteins and their protein substrates—via GFP tagging or immunofluorescence for both the protein target and the Ub/Ub-like modifier—can be accomplished.135,136
Another common strategy to visualize Ub/Ub-like modifications in living cells is to use bimolecular fluorescence complementation (BiFC) via split protein tagging. Split fluorescent proteins are most commonly used for cellular imaging applications, though other split protein systems137 can also be used for luminescence-based imaging or other modes of detection. Ubiquitin-mediated fluorescence complementation was reported in 2004.138 In the study, a transcription factor Jun was fused to the C-terminal fragment of yellow fluorescent protein and the complementary fragment was fused to ubiquitin. The reconstituted fluorescent signal indicated that ubiquitinated Jun was exported out of the nucleus and targeted to the lysosomes. Ubiquitination of transcription factor FOXO139 as well as SUMOylation of Jun and ATF6 in living cells were visualized by the same approach.140 A library of proteins can be screened for its SUMOylation extent by a similar split-protein approach (one fragment of the split fluorescent protein on the protein library; the other fragment on SUMO). SUMOylated proteins can be detected in a high-throughput manner by FACS, and their subcellular localization assessed by microscopy.141 While BiFC reporters are generally irreversible,142 reversible split protein reporters143,144 were recently developed and are better suited to monitor dynamic PTMs.
Proteins can be polyubiquitinated, and such polyubiquitination can occur on different attachment sites (e.g. Lys29, Lys48, Lys63). The complexity is further increased with PTMs on Ub, or incorporation of other Ub-like proteins to form a hybrid chain.145,146 These diverse structures of polyUb/Ub-like proteins play different roles within the cell ranging from signaling to degradation.147 Expression of Ub fused to fluorescent proteins has been used to visualize several forms of polyubiquitination based on proximity of two ubiquitins.148–151 This approach is straightforward but requires ectopic ubiquitin expression. Other approaches using antibodies and protein binding domains that bind a specific linkage form of polyubiquitination have been reported.130,145 Affimers targeting the Lys6 and Lys33 chains have been developed and their utilities demonstrated in western blot, imaging, and pull-down assays.152 A synthetic Fab fragment for the Lys29 chain was evolved from phage display, and used to enrich Lys29 chain-modified proteins for mass spectrometry-based proteomics studies.153 Imaging with fluorescently labeled Fab highlighted the concentration of K29 polyubiquitin signal around the midbody at telophase during the HeLa cell division. These specialized PTM detection tools could be combined with a protein identification signal to visualize protein-specific Ub/Ub-like modifications.
Beyond proteoform localization, a three-pronged detection approach to visualize a proteoform participating in protein–protein interactions was developed. The assay coupled FRET with BiFC to enable detection of protein ternary complexes,154 and was used to image interactions of a SUMOylated transcription factor BMAL1 with CREB-binding protein; this interaction resulted in the activation of the CLOCK-BMAL1 circadian clock.155
4. Detection of proteolytic proteoforms
Proteolytic processing can generate smaller proteoforms with distinct properties and functions from their pro-proteins. The resulting proteolytic proteoforms can act as hormones (insulin, adrenocorticotropic hormones) and signaling molecules (neuropeptides); some are associated with disease pathology (amyloid β peptides). Akin to the monitoring of protein–protein conjugation (e.g. ubiquitination), proteolytic proteoforms can be monitored via dual/multiplexed labeling of protein parts and subsequent proximity-based or simpler multicolored imaging readouts. The challenge lies in judicious epitope selection (for antibody-based detection), or placement of the recognition tag (for recombinant tag-based detection) to allow specific detection while minimizing interference with the complex, often sequential proteolytic processes.
To monitor proteolysis of specific precursor proteins, a pair of fluorophores could be attached to the target precursor protein (Fig. 5a). For example, a proteolytic sensor of neuregulin 1 (NRG1; a membrane protein that releases its ectodomain as an intercellular signal) was developed by fusing the protein with mCherry and EGFP at the extracellular N-terminal and the intracellular C-terminal respectively.156 The NRG1 cleavage could be monitored in cell culture and in neurons of zebrafish embryo. The imaging and fluorescence ratio illustrated that NRG1 shedding occurred in the axon more than in the neuron cell body. Ectodomain shedding of TGFα can be monitored in a similar manner.157 Similarly, processing and secretion of insulin in live Min6β cells could be visualized by fusion of GFP and mCherry to the A-peptide and C-peptide of proinsulin. Changes in the GFP-to-mCherry fluorescence intensity ratio indicated release of mature insulin, allowing effects of stimulation and drugs on insulin secretion to be measured.158 The approach is also applicable to the study of processing of amyloid precursor protein (APP) fused with two fluorescent proteins at its two terminis.159–161 After β-secretase-mediated cleavage, differential subcellular sorting of the N- and C-terminal APP fragments could be tracked within the cell.159,160
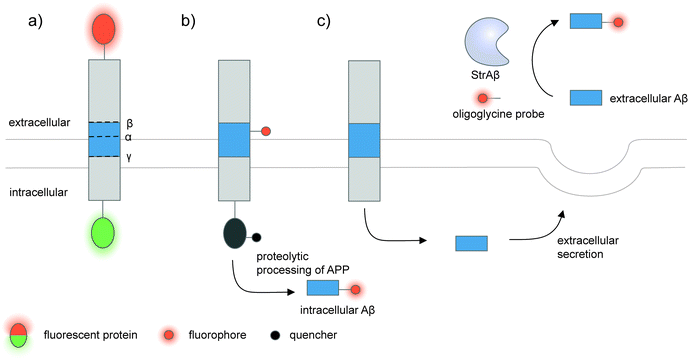 |
| Fig. 5 Strategies to detect proteolytic proteoforms derived from amyloid precursor protein (APP). Key proteolytic cleavage sites on transmembrane APP by α-, β-, and γ-secretases are illustrated. (a) APP can be dually labeled with two fluorescent proteins (red and green ovals), and its N- and C-terminal fragments upon cleavage separately tracked.159,160 (b) Genetic code expansion-mediated labeling of intracellular amyloid-β peptides.162 The amyloid-β-containing segment within APP is site-specifically labeled with an organic fluorophore (red circle) via genetic code expansion. The fluorescence signal from this label is suppressed prior to proteolysis by an intramolecular quencher (dark grey circle) appended to APP via enzyme-mediated labeling. Proteolysis along the endocytic pathway generates amyloid-β peptides and separates the fluorophore-labeled amyloid-β from the quencher-labeled precursor, resulting in enhanced fluorescence. (c) Sortase-mediated labeling of extracellular amyloid-β peptides.163 An engineered sortase specific for amyloid-β, called StrAβ, catalyzes transpeptidation to link an oligoglycine-fluorophore conjugate to the C-terminus of endogenous amyloid-β. | |
Many proteolytic proteoforms are small peptides, which are impossible to tag with large fluorescent proteins164 nor specifically detected with antibodies. To enable detection of such proteolytic peptides in cells, minimally invasive labeling strategies such as genetic code expansion can be used. Genetic code expansion employs an orthogonal aminoacyl–tRNA synthetase and tRNA pair to incorporate an unnatural amino acid typically at an amber stop codon introduced to the gene of interest.165 Diverse functional groups could be installed and further derivatized with fluorophores and tags using bioorthogonal chemistry. To distinguish the labeling signal of the processed peptide from that of the pro-protein, two fluorophore labels—one embedded in the processed peptide segment via genetic code expansion, and the other on the pro-protein—are required.166 Alternatively, a molecular beacon labeling strategy to visualize processed peptides has been developed (Fig. 5b).162 Here, a fluorophore was embedded in the peptide-containing segment of the pro-protein via genetic code expansion, while a fluorescence quencher was attached to another site within the pro-protein. Fluorescence was suppressed via distance-dependent FRET-based quenching while the pro-protein was intact, but elicited when the pro-protein was proteolyzed and the processed peptide liberated. Such molecular beacon labeling was used to label ∼40-amino-acid amyloid β peptides (Aβ, labeled with cy5 via genetic code expansion) as they are processed from APP (labeled with QSY21 quencher via HaloTag) along the endocytic pathway of live cells.
While genetic code expansion allows detection of proteolytic peptides, the method requires exogenous expression of engineered protein translation machineries (particularly an orthogonal aminoacyl–tRNA synthetase/tRNA pair) and is difficult to extend to in vivo and clinical work. Aβ plaques are pathological hallmarks of Alzheimer's disease, and the majority of Aβ processed from APP is secreted and aggregated in the extracellular fluids of the brain.167,168 To enable the detection of endogenous Aβ found in clinical fluids, the Liu group used yeast display-based directed evolution to change the substrate specificity of Staphylococcus aureus sortase A, such that the evolved enzyme, called StrAβ, now recognizes an LMVGG sequence at the termini of endogenous Aβ as a transpeptidation motif (Fig. 5c).163 Diverse probes (biotin, fluorophores) linked to a triglycine can then be conjugated to Aβ via StrAβ. StrAβ was successfully used to modify Aβ in human clinical cerebrospinal fluid (CSF) samples, allowing not only their direct detection, but further characterizations of its aggregation kinetics and a potential way to mark Aβ for targeted degradation.
5. Detecting non-enzymatic proteoforms
The preceding sections discuss the detection of proteoforms originated from enzymatic post-translational modifications; these are traditionally viewed as main regulators and diversifiers of protein function. On the other hand, the importance of non-enzymatic PTMs beyond markers of cellular stress has only begun to emerge (recent reviews here169–171). Non-enzymatic PTMs are generated from reactions between amino acid sidechains with suitable nucleophilicity or redox activity, and reactive (often oxidative or electrophilic) metabolites. Several non-enzymatic PTMs are now known to be reversible170 through changes in oxidative states of the cellular microenvironment or even through enzymes, providing relevance of this class of PTMs in protein regulation. Due to their recently recognized importance, there are much fewer cellular imaging tools for non-enzymatic proteoforms; the current technological gap provides opportunities for chemical biologists to develop new detection tools for non-enzymatic proteoforms, via design of clickable reagents or exploitation of unique reactivities of these PTMs.
Oxidation and nitrosylation of multiple amino acid sidechains—particularly cysteine—are examples of non-enzymatic PTMs resulted from cellular oxidants such as reactive oxygen species (ROS) or reactive nitrogen species (RNS). In some cases, these oxidative PTMs are known to dynamically regulate protein function.172 While imaging of almost all protein-specific oxidative PTMs is currently not possible, certain oxidative PTMs can be chemically derivatized to provide a detection handle on specific proteins (Fig. 6). To detect protein-specific sulfenyl cysteine residues, cells can be treated post-fixation with dimedone, followed by immunostaining with an anti-dimedonylated cysteine antibody. A protein-specific antibody could then be used to perform PLA, and has enabled the study of the spatiotemporal regulation of oxidized SH2 protein.173 The higher oxidation state of cysteine—sulfinylation—can also be probed using electrophilic diazenes to form stable sulfonamide adducts.174 The utility of electrophilic diazene probes could likely be extended to specific Cys-sulfinylated proteoform imaging.
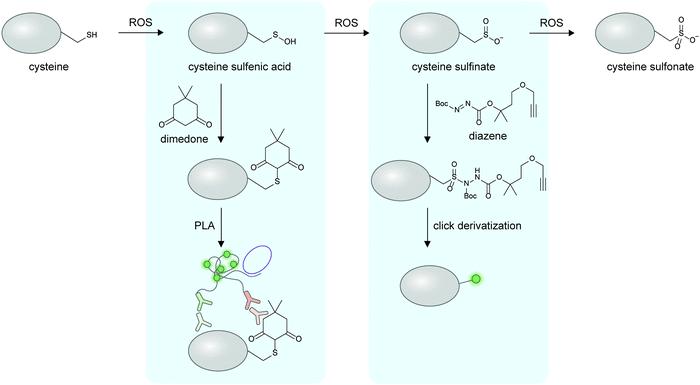 |
| Fig. 6 Detection of non-enzymatic oxidative proteoforms. The thiol group of cysteine can be oxidized sequentially to sulfenic acid, sulfinate, and sulfonate. Sulfenylated cysteines can be chemically derivatized with dimedone, the product of which can be detected with an antibody. Sulfinylated cysteines can be derivatized with electrophilic diazene compounds to install bioorthogonal functional groups and/or fluorophores. ROS, reactive oxygen species. | |
6. Monitoring proteoform turnover in cells
Proteoform labeling with genetically encoded and/or metabolic probes is generally compatible with real-time, dynamic imaging, though careful validations are needed to ensure the labels minimally interfere with the PTM installation/removal processes. FRET-based histone modification sensors are likely the most developed for real-time dynamic measurements. For example, a FRET-based reporter based on full-length H3 and a chromodomain HP1 could monitor the dynamics of H3K9me3 during the division of HeLa cells, and can be coupled to H3S10 phosphorylation monitoring via a second FRET sensor which detects phosphorylation on a small H3 peptide.61 In another work, dynamics of monomethylation of endogenous H3K20 during the cell cycle was tracked in real time by a specific mintbody.44 For time-coursed, non-real-time measurements, pulse metabolic labeling can be employed to look at proteoform turnover, as demonstrated with lipidated proteomes175 and specific lipidated proteins.176
7. Multiplexed detection of complex proteoforms
Cellular proteoforms are often complex and can contain multiple PTMs;177 detecting these complex species remains an outstanding challenge. The use of two or more antibody-based reporters with different fluorophores have been employed for multiplexed detection of PTMs within single cells.36,37,61 Multiplexed live cell monitoring of proteoforms has also been reported using EGFP- and SNAP-tagged mintbodies.178 Multiplexed PLA could be coupled to protein- and PTM-specific antibodies to simultaneously visualize protein-specific PTMs and protein–protein interactions (Fig. 7a).179 Aptamer-assisted labeling can be used in combination with metabolic labeling to probe multiple proteins bearing the same PTM; heterodimerization of HER2–EGFR glycoforms, metabolically labeled with Ac4ManNAz, could be visualized using two aptamer–probe conjugates (Fig. 7b).128
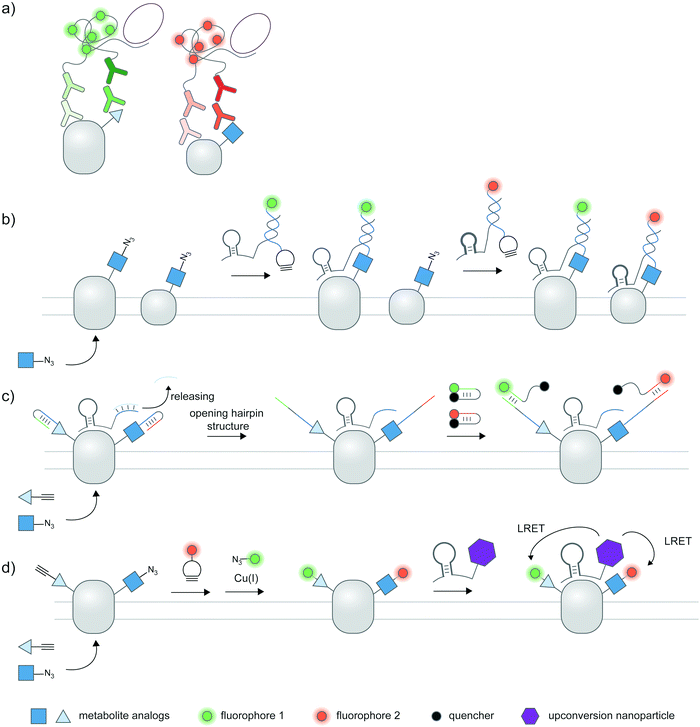 |
| Fig. 7 Multiplexed detection of proteoforms. (a) Multiplexed PLA as a basic strategy to detect multiple proteins or PTMs. (b) Detecting multiple specific proteins bearing the same PTM via multiple aptamers and metabolic labeling.128 (c) A hierarchical coding (HieCo)180 strategy to detect two PTMs on a specific protein. After metabolic labeling with two clickable sugar analogs, cascade & proximity-dependent hybridization events trigger binding of two molecular beacons in a protein- and sugar-specific manner, allowing simultaneous detection of two glycoforms. (d) Multiplexed energy transfer with an upconversion nanoparticle to detect two PTMs on a specific protein.181 The nanoparticle targeted to a specific protein serves as a sole LRET donor for two acceptor dyes, installed onto two sugar analogs via metabolic labeling. | |
Furthermore, several innovative strategies have been employed to tackle the challenge of multiplexed detection. To visualize glycoforms containing multiple types of monosaccharides at different compositions, Ac4ManNAz and Ac4FucAl probes were metabolically incorporated into the protein, followed by conjugation of two oligonucleotide probes, binding of an aptamer specific to MUC1 protein, and masking of the conjugated probes (Fig. 7c).180 Addition of unmasking oligonucleotides and molecular beacon probes allowed multiplexed detection of two sugars on the protein; different glycosylation patterns in different cell types can be monitored.
In another work, an upconversion nanoparticle was conjugated to an MUC1-specific aptamer.181 The nanoparticle provides two emission bands which allow luminescence resonance energy transfer (LRET) with two acceptor dyes—installed to two sugar types via metabolic labeling—upon a single near-infrared excitation (Fig. 7d). This system was successfully used for duplex imaging and quantification of fucosylated and sialylated MUC1, of which complex changes in glycosylation patterns can be tracked. The upconversion nanoparticle-conjugated aptamer was also used to identify phosphorylation and ubiquitination states of human epidermal growth factor receptor 2 (HER2).182 Here, ubiquitination was monitored through a cy5-labeled antibody for ubiquitin, while a small-molecule probe containing a Zn(II)–cyclen moiety and cy3 detected phosphorylation on proteins.
The chemical binders based on binuclear zinc complexes for diphosphorylated Tau protein have also been reported with low affinity toward monophosphorylated or non-phosphorylated Tau.183 These reporters were used to visualize hyperphosphorylated Tau in hippocampal sections from Alzheimer's disease patients183 and in primary neuron culture from mouse.184
8. Potential platforms for high-throughput detection of proteoforms
The ability to image proteoforms with highly complex modification states beyond two PTMs, or simultaneous imaging of multiple proteoforms, is difficult with current technologies. Tagging of genetically or metabolically incorporated probes often requires the use of mutually orthogonal bio-orthogonal chemistries,185 of which there are limited availabilities. While multiplexed amplification-based assays such as PLA are amenable to detecting up to 24 analytes,186 the extension of such assays to multicolor imaging in cells is limited by the color barrier of conventional fluorescence microscopy setup, which allows up to five spectrally separated fluorophores to be imaged at once. Modern microscopes with spectral unmixing and compensation capabilities,187 and ratiometric recoding of combinatorial fluorescent signals188 can overcome the color barrier, but are inevitably associated with higher errors from imperfect measurements. Instead of simultaneous imaging, iterations of immunostaining and signal removal can be performed with rounds of antibody stripping/elution and restaining189 (Fig. 8a), chemically cleavable fluorescent antibodies190 (Fig. 8b), and DNA-barcoded antibodies191 (Fig. 8c). Under optimized conditions such an iterative protocol (Fig. 8d) can be used to detect up to 40 protein targets, as demonstrated with iterative indirect immunofluorescence imaging (4i).189
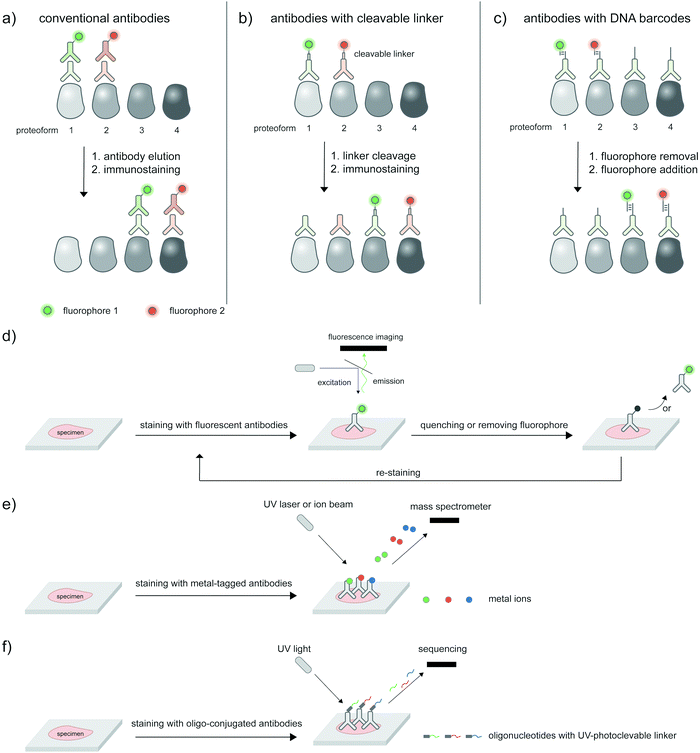 |
| Fig. 8 Potential technologies for high-throughput proteoform detection. These are multiplexed antibody-based protein profiling technologies currently used in spatial proteomic research. Iterative rounds of antibody staining can be accomplished via: (a) antibody stripping under optimized conditions, then restaining; (b) antibodies with cleavable fluorophores; and (c) DNA-barcoded antibodies as used in CODEX. (d) Iterative immunostaining and removal of labels/antibodies can be coupled to fluorescence microscopy to interrogate multiple proteoforms. (e) In lieu of fluorescence, mass cytometry-based techniques such as CyTOF can map released mass tags (rare-earth-metal isotopes) with high subcellular precision and multiplexity. (f) Digital spatial profiling sequences and quantifies indexed oligonucleotides photocleaved from a subcellular region of interest. In (e) and (f), antibodies are used to direct mass tags or indexed barcodes to desired biomolecules within the cell. | |
Beyond direct imaging of fluorescently labeled proteins and PTMs, increasingly high-throughput platforms to interrogate spatial proteomics may be extended to multiplexed, high-throughput detection of proteoforms, provided that suitable proteoform labeling tools are in place. Such platforms include primer extension and imaging-based CODEX,191 mass cytometry-based CyTOF192,193 (Fig. 8e) and barcode sequencing-based Digital Spatial Profiling194 (Fig. 8f); Hickey et al.195 provides a state-of-the-art review on these multiplexed protein profiling technologies. Antibody-based reagents for the detection of proteins, PTM types, or site-specific PTMs are naturally applicable to these platforms, but proximity-based measurements/assays needed to establish the PTM/protein intramolecularity are currently not applicable. The challenge to create new proteoform labeling tools which provide compatible readouts with these high-throughput technologies represents exciting opportunities for chemical biology tool developers.
9. Conclusion and outlook
The chemical diversity and heterogeneity of cellular proteoforms give rise to their complex functions, but pose a grand challenge to their specific detection in the cellular context. Advances in protein and antibody engineering, protein and metabolic labeling, nucleic acid-based detection, and other innovative strategies have created molecular probes capable of highlighting spatial localization and temporal dynamics of these proteoforms. Despite their immense utility, these probes are to be used with caution and rigorous validations since they are limited in one characteristic or another, as outlined throughout the review. The field would benefit from continual refinement of probes targeting frequent, well-studied post-translational modification types, as well as creation of new tools for even more diverse classes of post-translational modifications and proteoforms. Linking molecular probes to instrumental platforms with spatially resolved, highly sensitive and multiplexed detection capability could enable broad dissection of functional roles of the most predominant proteoforms of cellular proteomes in the near future.
Conflicts of interest
The authors declare no competing interests.
Acknowledgements
This work was supported by a start-up grant from VISTEC, Wellcome Trust (217249/Z/19/Z), Swiss National Science Foundation (IZSTZ0_193915), and Thailand Science Research and Innovation (Global Partnership Program from Program Management Unit-B), all to C. U. S. S. and B. L. were supported by research assistant and student funds from VISTEC respectively.
References
- L. M. Smith, N. L. Kelleher, M. Linial, D. Goodlett, P. Langridge-Smith, Y. Ah Goo, G. Safford, L. Bonilla, G. Kruppa, R. Zubarev, J. Rontree, J. Chamot-Rooke, J. Garavelli, A. Heck, J. Loo, D. Penque, M. Hornshaw, C. Hendrickson, L. Pasa-Tolic, C. Borchers, D. Chan, N. Young, J. Agar, C. Masselon, M. Gross, F. McLafferty, Y. Tsybin, Y. Ge, I. Sanders, J. Langridge, J. Whitelegge and A. Marshall, Nat. Methods, 2013, 10, 186–187 CrossRef CAS PubMed
.
- D. M. Creasy and J. S. Cottrell, Proteomics, 2004, 4, 1534–1536 CrossRef CAS PubMed
.
- R. Aebersold, J. N. Agar, I. J. Amster, M. S. Baker, C. R. Bertozzi, E. S. Boja, C. E. Costello, B. F. Cravatt, C. Fenselau, B. A. Garcia, Y. Ge, J. Gunawardena, R. C. Hendrickson, P. J. Hergenrother, C. G. Huber, A. R. Ivanov, O. N. Jensen, M. C. Jewett, N. L. Kelleher, L. L. Kiessling, N. J. Krogan, M. R. Larsen, J. A. Loo, R. R. Ogorzalek Loo, E. Lundberg, M. J. MacCoss, P. Mallick, V. K. Mootha, M. Mrksich, T. W. Muir, S. M. Patrie, J. J. Pesavento, S. J. Pitteri, H. Rodriguez, A. Saghatelian, W. Sandoval, H. Schlüter, S. Sechi, S. A. Slavoff, L. M. Smith, M. P. Snyder, P. M. Thomas, M. Uhlén, J. E. Van Eyk, M. Vidal, D. R. Walt, F. M. White, E. R. Williams, T. Wohlschlager, V. H. Wysocki, N. A. Yates, N. L. Young and B. Zhang, Nat. Chem. Biol., 2018, 14, 206–214 CrossRef CAS PubMed
.
- T. K. Toby, L. Fornelli and N. L. Kelleher, Annu. Rev. Anal. Chem., 2016, 9, 499–519 CrossRef CAS PubMed
.
- Y. Zhao and O. N. Jensen, Proteomics, 2009, 9, 4632–4641 CrossRef CAS PubMed
.
- C. G. Parker and M. R. Pratt, Cell, 2020, 180, 605–632 CrossRef CAS PubMed
.
- A. Leitner, Methods Mol. Biol., 2016, 1355, 105–121 CrossRef CAS PubMed
.
- K. Gevaert, M. Goethals, L. Martens, J. Van Damme, A. Staes, G. R. Thomas and J. Vandekerckhove, Nat. Biotechnol., 2003, 21, 566–569 CrossRef CAS PubMed
.
- O. Kleifeld, A. Doucet, U. auf dem Keller, A. Prudova, O. Schilling, R. K. Kainthan, A. E. Starr, L. J. Foster, J. N. Kizhakkedathu and C. M. Overall, Nat. Biotechnol., 2010, 28, 281–288 CrossRef CAS PubMed
.
- I. Bludau, M. Frank, C. Dörig, Y. Cai, M. Heusel, G. Rosenberger, P. Picotti, B. C. Collins, H. Röst and R. Aebersold, Nat. Commun., 2021, 12, 3810 CrossRef CAS PubMed
.
- N. Krahmer, B. Najafi, F. Schueder, F. Quagliarini, M. Steger, S. Seitz, R. Kasper, F. Salinas, J. Cox, N. H. Uhlenhaut, T. C. Walther, R. Jungmann, A. Zeigerer, G. H. H. Borner and M. Mann, Dev. Cell, 2018, 47, 205–221.e207 CrossRef CAS PubMed
.
- Y. Liu, R. Zeng, R. Wang, Y. Weng, R. Wang, P. Zou and P. R. Chen, Proc. Natl. Acad. Sci. U. S. A., 2021, 118, e2025299118 CrossRef CAS PubMed
.
- M. Holt and T. Muir, Annu. Rev. Biochem., 2015, 84, 265–290 CrossRef CAS PubMed
.
- D. T. Rogerson, A. Sachdeva, K. Wang, T. Haq, A. Kazlauskaite, S. M. Hancock, N. Huguenin-Dezot, M. M. Muqit, A. M. Fry, R. Bayliss and J. W. Chin, Nat. Chem. Biol., 2015, 11, 496–503 CrossRef CAS PubMed
.
- M. S. Zhang, S. F. Brunner, N. Huguenin-Dezot, A. D. Liang, W. H. Schmied, D. T. Rogerson and J. W. Chin, Nat. Methods, 2017, 14, 729–736 CrossRef CAS PubMed
.
- C. Fan, K. Ip and D. Söll, FEBS Lett., 2016, 590, 3040–3047 CrossRef CAS PubMed
.
- D. P. Nguyen, M. M. Garcia Alai, P. B. Kapadnis, H. Neumann and J. W. Chin, J. Am. Chem. Soc., 2009, 131, 14194–14195 CrossRef CAS PubMed
.
- D. P. Nguyen, M. M. Garcia Alai, S. Virdee and J. W. Chin, Chem. Biol., 2010, 17, 1072–1076 CrossRef CAS PubMed
.
- H. Neumann, S. M. Hancock, R. Buning, A. Routh, L. Chapman, J. Somers, T. Owen-Hughes, J. van Noort, D. Rhodes and J. W. Chin, Mol. Cell, 2009, 36, 153–163 CrossRef CAS PubMed
.
- S. Virdee, P. B. Kapadnis, T. Elliott, K. Lang, J. Madrzak, D. P. Nguyen, L. Riechmann and J. W. Chin, J. Am. Chem. Soc., 2011, 133, 10708–10711 CrossRef CAS PubMed
.
- M. Fottner, A. D. Brunner, V. Bittl, D. Horn-Ghetko, A. Jussupow, V. R. I. Kaila, A. Bremm and K. Lang, Nat. Chem. Biol., 2019, 15, 276–284 CrossRef CAS PubMed
.
- D. H. Ramirez, C. Aonbangkhen, H.-Y. Wu, J. A. Naftaly, S. Tang, T. R. O’Meara and C. M. Woo, ACS Chem. Biol., 2020, 15, 1059–1066 CrossRef CAS PubMed
.
- V. Beránek, C. D. Reinkemeier, M. S. Zhang, A. D. Liang, G. Kym and J. W. Chin, Cell Chem. Biol., 2018, 25(1067-1074), e1065 Search PubMed
.
- P.-H. Chen, Z. Hu, E. An, I. Okeke, S. Zheng, X. Luo, A. Gong, S. Jaime-Figueroa and C. M. Crews, ACS Chem. Biol., 2021, 16, 2808–2815 CrossRef CAS PubMed
.
- S. U. Siriwardena, D. N. P. Munkanatta Godage, V. M. Shoba, S. Lai, M. Shi, P. Wu, S. K. Chaudhary, S. L. Schreiber and A. Choudhary, J. Am. Chem. Soc., 2020, 142, 14052–14057 CrossRef CAS PubMed
.
- W. W. Wang, L.-Y. Chen, J. M. Wozniak, A. M. Jadhav, H. Anderson, T. E. Malone and C. G. Parker, J. Am. Chem. Soc., 2021, 143, 16700–16708 CrossRef CAS PubMed
.
- X. Fang, Y. Fu, M. J. C. Long, J. A. Haegele, E. J. Ge, S. Parvez and Y. Aye, J. Am. Chem. Soc., 2013, 135, 14496–14499 CrossRef CAS PubMed
.
- H.-Y. Lin, J. A. Haegele, M. T. Disare, Q. Lin and Y. Aye, J. Am. Chem. Soc., 2015, 137, 6232–6244 CrossRef CAS PubMed
.
- N. P. Dantuma and H. van Attikum, EMBO J., 2016, 35, 6–23 CrossRef CAS PubMed
.
- F. Hertel and J. Zhang, Biopolymers, 2014, 101, 180–187 CrossRef CAS PubMed
.
- T. Hattori and S. Koide, Curr. Opin. Struct. Biol., 2018, 51, 141–148 CrossRef CAS PubMed
.
- Y. Hayashi-Takanaka, K. Maehara, A. Harada, T. Umehara, S. Yokoyama, C. Obuse, Y. Ohkawa, N. Nozaki and H. Kimura, Chromosome Res., 2015, 23, 753–766 CrossRef CAS PubMed
.
- H. Kimura, Y. Hayashi-Takanaka, T. J. Stasevich and Y. Sato, Histochem. Cell Biol., 2015, 144, 101–109 CrossRef CAS PubMed
.
- Y. Hayashi-Takanaka, K. Yamagata, T. Wakayama, T. J. Stasevich, T. Kainuma, T. Tsurimoto, M. Tachibana, Y. Shinkai, H. Kurumizaka, N. Nozaki and H. Kimura, Nucleic Acids Res., 2011, 39, 6475–6488 CrossRef CAS PubMed
.
- Y. Hayashi-Takanaka, K. Yamagata, N. Nozaki and H. Kimura, J. Cell Biol., 2009, 187, 781–790 CrossRef CAS PubMed
.
- Y. Sato, L. Hilbert, H. Oda, Y. Wan, J. M. Heddleston, T.-L. Chew, V. Zaburdaev, P. Keller, T. Lionnet, N. Vastenhouw and H. Kimura, Development, 2019, 146, dev179127 CrossRef CAS PubMed
.
- T. J. Stasevich, Y. Hayashi-Takanaka, Y. Sato, K. Maehara, Y. Ohkawa, K. Sakata-Sogawa, M. Tokunaga, T. Nagase, N. Nozaki, J. G. McNally and H. Kimura, Nature, 2014, 516, 272–275 CrossRef CAS PubMed
.
- L. S. Forero-Quintero, W. Raymond, T. Handa, M. N. Saxton, T. Morisaki, H. Kimura, E. Bertrand, B. Munsky and T. J. Stasevich, Nat. Commun., 2021, 12, 3158 CrossRef CAS PubMed
.
- E. Moeglin, D. Desplancq, A. Stoessel, C. Massute, J. Ranniger, A. G. McEwen, G. Zeder-Lutz, M. Oulad-Abdelghani, M. Chiper, P. Lafaye, B. Di Ventura, P. Didier, A. Poterszman and E. Weiss, Cancers, 2021, 13, 3317 CrossRef CAS PubMed
.
- O. F. Sanchez, A. Mendonca, A. D. Carneiro and C. Yuan, ACS Sens., 2017, 2, 426–435 CrossRef CAS PubMed
.
- Y. Sato, M. Mukai, J. Ueda, M. Muraki, T. J. Stasevich, N. Horikoshi, T. Kujirai, H. Kita, T. Kimura, S. Hira, Y. Okada, Y. Hayashi-Takanaka, C. Obuse, H. Kurumizaka, A. Kawahara, K. Yamagata, N. Nozaki and H. Kimura, Sci. Rep., 2013, 3, 2436 CrossRef PubMed
.
- T. Ito, T. Umehara, K. Sasaki, Y. Nakamura, N. Nishino, T. Terada, M. Shirouzu, B. Padmanabhan, S. Yokoyama, A. Ito and M. Yoshida, Chem. Biol., 2011, 18, 495–507 CrossRef CAS PubMed
.
- M. Suzuki, C. Takagi, S. Miura, Y. Sakane, M. Suzuki, T. Sakuma, N. Sakamoto, T. Endo, Y. Kamei, Y. Sato, H. Kimura, T. Yamamoto, N. Ueno and K.-i. T. Suzuki, Genes Cells, 2016, 21, 358–369 CrossRef CAS PubMed
.
- Y. Sato, T. Kujirai, R. Arai, H. Asakawa, C. Ohtsuki, N. Horikoshi, K. Yamagata, J. Ueda, T. Nagase, T. Haraguchi, Y. Hiraoka, A. Kimura, H. Kurumizaka and H. Kimura, J. Mol. Biol., 2016, 428, 3885–3902 CrossRef CAS PubMed
.
- Y. Shinkai, M. Kuramochi and M. Doi, G3: Genes, Genomes, Genet., 2018, 8, 2249–2255 CrossRef CAS PubMed
.
- C. Hemers-Casterman, T. Atarhouch, S. Muyldermans, G. Robinson, C. Hamers, E. B. Songa, N. Bendahman and R. Hamers, Nature, 1993, 363, 446–448 CrossRef PubMed
.
- P. Bannas, J. Hambach and F. Koch-Nolte, Front. Immunol., 2017, 8, 1603 CrossRef PubMed
.
- S. Muyldermans, FEBS J., 2021, 288, 2084–2102 CrossRef CAS PubMed
.
- J. Helma, M. C. Cardoso, S. Muyldermans and H. Leonhardt, J. Cell Biol., 2015, 209, 633–644 CrossRef CAS PubMed
.
- J. Woods, SLAS Discovery, 2019, 24, 703–713 CAS
.
- H. J. Wagner, S. Wehrle, E. Weiss, M. Cavallari and W. Weber, Int. J. Mol. Sci., 2018, 19, 3444 CrossRef PubMed
.
- M. Rajan, O. Mortusewicz, U. Rothbauer, F. D. Hastert, K. Schmidthals, A. Rapp, H. Leonhardt and M. C. Cardoso, FEBS Open Bio, 2015, 5, 779–788 CrossRef CAS PubMed
.
- T. Kanno, Y. Kanno, R. M. Siegel, M. K. Jang, M. J. Lenardo and K. Ozato, Mol. Cell, 2004, 13, 33–43 CrossRef CAS PubMed
.
- O. F. Sánchez, A. Mendonca, A. Min, J. Liu and C. Yuan, ACS Omega, 2019, 4, 13250–13259 CrossRef PubMed
.
- C. Lungu, S. Pinter, J. Broche, P. Rathert and A. Jeltsch, Nat. Commun., 2017, 8, 1–13 CrossRef CAS PubMed
.
- A. M. F. Delachat, N. Guidotti, A. L. Bachmann, A. C. A. Meireles-Filho, H. Pick, C. C. Lechner, C. Deluz, B. Deplancke, D. M. Suter and B. Fierz, Cell Chem. Biol., 2018, 25, 51–56.e56 CrossRef CAS PubMed
.
- A. Mendonca, O. F. Sánchez, J. Xie, A. Carneiro, L. Lin and C. Yuan, Biochim. Biophys. Acta, Gene Regul. Mech., 2021, 1864, 194725 CrossRef CAS PubMed
.
- C.-W. Lin, C. Y. Jao and A. Y. Ting, J. Am. Chem. Soc., 2004, 126, 5982–5983 CrossRef CAS PubMed
.
- K. Sasaki, T. Ito, N. Nishino, S. Khochbin and M. Yoshida, Proc. Natl. Acad. Sci. U. S. A., 2009, 106, 16257–16262 CrossRef CAS PubMed
.
- S. Nakaoka, K. Sasaki, A. Ito, Y. Nakao and M. Yoshida, ACS Chem. Biol., 2015, 11, 729–733 CrossRef PubMed
.
- Q. Peng, S. Lu, Y. Shi, Y. Pan, P. Limsakul, A. V. Chernov, J. Qiu, X. Chai, Y. Shi, P. Wang, Y. Ji, Y.-S. J. Li, A. Y. Strongin, V. V. Verkhusha, J. C. Izpisua Belmonte, B. Ren, Y. Wang, S. Chien and Y. Wang, Proc. Natl. Acad. Sci. U. S. A., 2018, 115, E11681 CrossRef CAS PubMed
.
- D. Li, L. Wang, B. F. Maziuk, X. Yao, B. Wolozin and Y. K. Cho, J. Biol. Chem., 2018, 293, 12081–12094 CrossRef CAS PubMed
.
- S. Buus, J. Rockberg, B. Forsström, P. Nilsson, M. Uhlen and C. Schafer-Nielsen, Mol. Cell. Proteomics, 2012, 11, 1790–1800 CrossRef PubMed
.
- Y. Fukata, A. Dimitrov, G. Boncompain, O. Vielemeyer, F. Perez and M. Fukata, J. Cell Biol., 2013, 202, 145–161 CrossRef CAS PubMed
.
- S. Conic, D. Desplancq, A. Ferrand, V. Fischer, V. Heyer, B. Reina San Martin, J. Pontabry, M. Oulad-Abdelghani, K. Babu N., G. D. Wright, N. Molina, E. Weiss and L. Tora, J. Cell Biol., 2018, 217, 1537–1552 CrossRef CAS PubMed
.
- D. B. Lukatsky and E. I. Shakhnovich, Phys. Rev. E: Stat., Nonlinear, Soft Matter Phys., 2008, 77, 020901 CrossRef CAS PubMed
.
- M. H. Jacob, R. N. Dsouza, I. Ghosh, A. Norouzy, T. Schwarzlose and W. M. Nau, J. Phys. Chem. B, 2013, 117, 185–198 CrossRef CAS PubMed
.
- H. Sahoo, D. Roccatano, M. Zacharias and W. M. Nau, J. Am. Chem. Soc., 2006, 128, 8118–8119 CrossRef CAS PubMed
.
- J. M. Kee, R. C. Oslund, D. H. Perlman and T. W. Muir, Nat. Chem. Biol., 2013, 9, 416–421 CrossRef CAS PubMed
.
- O. Haji-Ghassemi, R. J. Blackler, N. Martin Young and S. V. Evans, Glycobiology, 2015, 25, 920–952 CrossRef CAS PubMed
.
- C. Fang, X. Zhang, L. Zhang, X. Gao, P. Yang and H. Lu, J. Proteome Res., 2016, 15, 956–962 CrossRef CAS PubMed
.
- P. J. Verveer, F. S. Wouters, A. R. Reynolds and P. I. H. Bastiaens, Science, 2000, 290, 1567–1570 CrossRef CAS PubMed
.
- A. R. Reynolds, C. Tischer, P. J. Verveer, O. Rocks and P. I. H. Bastiaens, Nat. Cell Biol., 2003, 5, 447–453 CrossRef CAS PubMed
.
- C. I. Maeder, M. A. Hink, A. Kinkhabwala, R. Mayr, P. I. H. Bastiaens and M. Knop, Nat. Cell Biol., 2007, 9, 1319–1326 CrossRef CAS PubMed
.
- K. N. Chuh, A. R. Batt and M. R. Pratt, Cell Chem. Biol., 2016, 23, 86–107 CrossRef CAS PubMed
.
- K. N. Chuh and M. R. Pratt, Curr. Opin. Chem. Biol., 2015, 24, 27–37 CrossRef CAS PubMed
.
- Y. Haga, K. Ishii, K. Hibino, Y. Sako, Y. Ito, N. Taniguchi and T. Suzuki, Nat. Commun., 2012, 3, 907 CrossRef PubMed
.
- W. Lin, L. Gao and X. Chen, ChemBioChem, 2015, 16, 2571–2575 CrossRef CAS PubMed
.
- F. Doll, A. Buntz, A.-K. Späte, V. F. Schart, A. Timper, W. Schrimpf, C. R. Hauck, A. Zumbusch and V. Wittmann, Angew. Chem., Int. Ed., 2016, 55, 2262–2266 CrossRef CAS PubMed
.
- F. Doll, R. R. Steimbach and A. Zumbusch, ChemBioChem, 2019, 20, 1315–1325 CrossRef CAS PubMed
.
- A. Buntz, S. Wallrodt, E. Gwosch, M. Schmalz, S. Beneke, E. Ferrando-May, A. Marx and A. Zumbusch, Angew. Chem., Int. Ed., 2016, 55, 11256–11260 CrossRef CAS PubMed
.
- W. Lin, Y. Du, Y. Zhu and X. Chen, J. Am. Chem. Soc., 2014, 136, 679–687 CrossRef CAS PubMed
.
- C. Uttamapinant, K. A. White, H. Baruah, S. Thompson, M. Fernández-Suárez, S. Puthenveetil and A. Y. Ting, Proc. Natl. Acad. Sci. U. S. A., 2010, 107, 10914–10919 CrossRef CAS PubMed
.
- B. Belardi, A. de la Zerda, D. R. Spiciarich, S. L. Maund, D. M. Peehl and C. R. Bertozzi, Angew. Chem., Int. Ed., 2013, 52, 14045–14049 CrossRef CAS PubMed
.
- N. Li, W. Zhang, L. Lin, S. N. A. Shah, Y. Li and J.-M. Lin, Anal. Chem., 2019, 16, 55 Search PubMed
.
- L. Jun, Y. Mingxu, P. Ying, L. Huixia, Y. Mao and T. Weihong, Curr. Med. Chem., 2011, 18, 4117–4125 CrossRef PubMed
.
- Y. Zhang, B. S. Lai and M. Juhas, Molecules, 2019, 24, 941 CrossRef PubMed
.
- T. Zhao, T. Li and Y. Liu, Nanoscale, 2017, 9, 9841–9847 RSC
.
- Y. Jeong, Y.-M. Kook, K. Lee and W.-G. Koh, Biosens. Bioelectron., 2018, 111, 102–116 CrossRef CAS PubMed
.
- Y. Li, X. Huang and J. Ren, Analyst, 2021, 146, 4506–4514 RSC
.
- A. Bhat, S. Li, D. Hammler, M. J. Winterhalder, A. Marx and A. Zumbusch, Int. J. Mol. Sci., 2021, 22, 8616 CrossRef CAS PubMed
.
- K. Islam, W. Zheng, H. Yu, H. Deng and M. Luo, ACS Chem. Biol., 2011, 6, 679–684 CrossRef CAS PubMed
.
- R. Wang, G. Ibáñez, K. Islam, W. Zheng, G. Blum, C. Sengelaub and M. Luo, Mol. BioSyst., 2011, 7, 2970–2981 RSC
.
- Z. Han, C.-w. Chou, X. Yang, M. G. Bartlett and Y. G. Zheng, ACS Chem. Biol., 2017, 12, 1547–1555 CrossRef CAS PubMed
.
- Z. Lyu, Y. Zhao, Z. Y. Buuh, N. Gorman, A. R. Goldman, M. S. Islam, H.-Y. Tang and R. E. Wang, J. Am. Chem. Soc., 2021, 143, 1341–1347 CrossRef CAS PubMed
.
- S.-S. Han, H.-E. Shim, S.-J. Park, B.-C. Kim, D.-E. Lee, H.-M. Chung, S.-H. Moon and S.-W. Kang, Sci. Rep., 2018, 8, 13212 CrossRef PubMed
.
- N. P. Westcott, J. P. Fernandez, H. Molina and H. C. Hang, Nat. Chem. Biol., 2017, 13, 302–308 CrossRef CAS PubMed
.
- R. Wang, K. Islam, Y. Liu, W. Zheng, H. Tang, N. Lailler, G. Blum, H. Deng and M. Luo, J. Am. Chem. Soc., 2013, 135, 1048–1056 CrossRef CAS PubMed
.
- R. Wang, W. Zheng, H. Yu, H. Deng and M. Luo, J. Am. Chem. Soc., 2011, 133, 7648–7651 CrossRef CAS PubMed
.
- C. Yang, J. Mi, Y. Feng, L. Ngo, T. Gao, L. Yan and Y. G. Zheng, J. Am. Chem. Soc., 2013, 135, 7791–7794 CrossRef CAS PubMed
.
- J. Choi, L. J. S. Wagner, S. B. P. E. Timmermans, S. A. Malaker, B. Schumann, M. A. Gray, M. F. Debets, M. Takashima, J. Gehring and C. R. Bertozzi, J. Am. Chem. Soc., 2019, 141, 13442–13453 CrossRef CAS PubMed
.
- I. Weibrecht, K.-J. Leuchowius, C.-M. Clausson, T. Conze, M. Jarvius, W. M. Howell, M. Kamali-Moghaddam and O. Söderberg, Expert Rev. Proteomics, 2010, 7, 401–409 CrossRef CAS PubMed
.
- O. Söderberg, M. Gullberg, M. Jarvius, K. Ridderstråle, K.-J. Leuchowius, J. Jarvius, K. Wester, P. Hydbring, F. Bahram, L.-G. Larsson and U. Landegren, Nat. Methods, 2006, 3, 995–1000 CrossRef PubMed
.
- R. Sable, N. Jambunathan, S. Singh, S. Pallerla, K. G. Kousoulas and S. Jois, Biotechniques, 2018, 65, 149–157 CrossRef CAS PubMed
.
- W. Lin, L. Gao and X. Chen, Curr. Opin. Chem. Biol., 2015, 28, 156–163 CrossRef CAS PubMed
.
- X. Zhang, R. Li, Y. Chen, S. Zhang, W. Wang and F. Li, Chem. Sci., 2016, 7, 6182–6189 RSC
.
- B. Yuan, Y. Chen, Y. Sun, Q. Guo, J. Huang, J. Liu, X. Meng, X. Yang, X. Wen, Z. Li, L. Li and K. Wang, Anal. Chem., 2018, 90, 6131–6137 CrossRef CAS PubMed
.
- Z. Li, B. Yuan, X. Lin, X. Meng, X. Wen, Q. Guo, L. Li, H. Jiang and K. Wang, Talanta, 2020, 215, 120889 CrossRef CAS PubMed
.
- Y. Liu, L. Liu, S. Li, G. Wang, H. Ju and L. Ding, Anal. Chem., 2019, 91, 6027–6034 CrossRef CAS PubMed
.
- L. Elfineh, C. Classon, A. Asplund, U. Pettersson, M. Kamali-Moghaddam and S. B. Lind, BMC Cancer, 2014, 14, 1–8 CrossRef PubMed
.
- A. V. Shah, G. M. Birdsey, C. Peghaire, M. E. Pitulescu, N. P. Dufton, Y. Yang, I. Weinberg, L. Osuna Almagro, L. Payne, J. C. Mason, H. Gerhardt, R. H. Adams and A. M. Randi, Nat. Commun., 2017, 8, 16002 CrossRef CAS PubMed
.
- M. Jarvius, J. Paulsson, I. Weibrecht, K. J. Leuchowius, A. C. Anderson, C. Wählby, M. Gullberg, J. Botling, T. Sjöblom, B. Markova, A. Östman, U. Landegren and O. Söderberg, Mol. Cell. Proteomics, 2007, 6, 1500–1509 CrossRef CAS PubMed
.
- Y. Xie and B. A. Perrino, Anal. Biochem., 2019, 577, 1–13 CrossRef CAS PubMed
.
- C. Poulard, J. Jacquemetton, T. H. Pham and M. Le Romancer, Methods, 2020, 175, 66–71 CrossRef CAS PubMed
.
- J. Jiao, R. Han, W. W. Hancock and U. H. Beier, Methods Mol. Biol., 2017, 1510, 287–293 CrossRef CAS PubMed
.
- A. Avin, M. Levy, Z. Porat and J. Abramson, Nat. Commun., 2017, 8, 1524 CrossRef PubMed
.
- X. Gao and R. N. Hannoush, Nat. Chem. Biol., 2014, 10, 61–68 CrossRef CAS PubMed
.
- X. Gao and R. N. Hannoush, Nat. Protocols, 2014, 9, 2607–2623 CAS
.
- X. Li, X. Jiang, X. Xu, C. Zhu and W. Yi, Carbohydr. Res., 2017, 448, 148–154 CrossRef CAS PubMed
.
- M. M. Mocanu, T. Váradi, J. Szöllosi and P. Nagy, Proteomics, 2011, 11, 2063–2070 CrossRef CAS PubMed
.
- J. Zhou and J. Rossi, Nat. Rev. Drug Discovery, 2017, 16, 181–202 CrossRef CAS PubMed
.
- Y. Fu, H. Qian, X. Zhou, Y. Wu, L. Song, K. Chen, D. Bai, Y. Yang, J. Li and G. Xie, Anal. Bioanal. Chem., 2021, 413, 6929–6939 CrossRef CAS PubMed
.
- X. Yang, Y. Tang, X. Zhang, Y. Hu, Y. Y. Tang, L. Y. Hu, S. Li, Y. Xie and D. Zhu, Microchim. Acta, 2019, 186, 1–9 CrossRef PubMed
.
- S. Bi, S. Yue and S. Zhang, Chem. Soc. Rev., 2017, 46, 4281–4298 RSC
.
- R. M. Dirks and N. A. Pierce, Proc. Natl. Acad. Sci. U. S. A., 2004, 101, 15275 CrossRef CAS PubMed
.
- J. Li, S. Liu, L. Sun, W. Li, S.-Y. Zhang, S. Yang, J. Li and H.-H. Yang, J. Am. Chem. Soc., 2018, 140, 16589–16595 CrossRef CAS PubMed
.
- Z. Liu, Y. Liang, W. Cao, W. Gao and B. Tang, Anal. Chem., 2021, 93, 8922 Search PubMed
.
- W. Yang, H. Nan, Z. Xu, Z. Huang, S. Chen, J. Li, J. Li and H. Yang, Anal. Chem., 2021, 93, 12265–12272 CrossRef CAS PubMed
.
- L. Cappadocia and C. D. Lima, Chem. Rev., 2018, 118, 889–918 CrossRef CAS PubMed
.
- S. J. van Wijk, S. Fulda, I. Dikic and M. Heilemann, EMBO Rep., 2019, 20, e46520 CrossRef PubMed
.
- C. Batters, H. Zhu and J. E. Sale, PLoS One, 2010, 5, e9008 CrossRef PubMed
.
- S. Ganesan, S. M. Ameer-beg, T. T. C. Ng, B. Vojnovic and F. S. Wouters, Proc. Natl. Acad. Sci. U. S. A., 2006, 103, 4089–4094 CrossRef CAS PubMed
.
- K. M. Riching, S. Mahan, C. R. Corona, M. McDougall, J. D. Vasta, M. B. Robers, M. Urh and D. L. Daniels, ACS Chem. Biol., 2018, 13, 2758–2770 CrossRef CAS PubMed
.
- L. R. Garcia, T. Tenev, R. Newman, R. O. Haich, G. Liccardi, S. W. John, A. Annibaldi, L. Yu, M. Pardo, S. N. Young, C. Fitzgibbon, W. Fernando, N. Guppy, H. Kim, L.-Y. Liang, I. S. Lucet, A. Kueh, I. Roxanis, P. Gazinska, M. Sims, T. Smyth, G. Ward, J. Bertin, A. M. Beal, B. Geddes, J. S. Choudhary, J. M. Murphy, K. Aurelia Ball, J. W. Upton and P. Meier, Nat. Commun., 2021, 12, 3364 CrossRef CAS PubMed
.
- I. Matic, J. Schimmel, I. A. Hendriks, M. A. van Santen, F. van de Rijke, H. van Dam, F. Gnad, M. Mann and A. C. O. Vertegaal, Mol. Cell, 2010, 39, 641–652 CrossRef CAS PubMed
.
- D. Tempé, E. Vives, F. Brockly, H. Brooks, S. De Rossi, M. Piechaczyk and G. Bossis, Oncogene, 2014, 33, 921–927 CrossRef PubMed
.
- T. Azad, A. Tashakor and S. Hosseinkhani, Anal. Bioanal. Chem., 2014, 406, 5541–5560 CrossRef CAS PubMed
.
- D. Fang and T. K. Kerppola, Proc. Natl. Acad. Sci. U. S. A., 2004, 101, 14782–14787 CrossRef CAS PubMed
.
- A. van der Horst, A. M. M. de Vries-Smits, A. B. Brenkman, M. H. van Triest, N. van den Broek, F. Colland, M. M. Maurice and B. M. T. Burgering, Nat. Cell Biol., 2006, 8, 1064–1073 CrossRef CAS PubMed
.
- X. Hou, Z. Yang, K. Zhang, D. Fang and F. Sun, Biochem. Biophys. Res. Commun., 2017, 494, 446–451 CrossRef CAS PubMed
.
- M. Komiya, A. Ito, M. Endo, D. Hiruma, M. Hattori, H. Saitoh, M. Yoshida and T. Ozawa, Sci. Rep., 2017, 7, 1–13 CrossRef CAS PubMed
.
- Y. Kodama and C.-D. Hu, Biotechniques, 2012, 53, 285–298 CrossRef CAS PubMed
.
- T.-L. To, Q. Zhang and X. Shu, Protein Sci., 2016, 25, 748–753 CrossRef CAS PubMed
.
- A. G. Tebo and A. Gautier, Nat. Commun., 2019, 10, 2822 CrossRef PubMed
.
- D. A. Pérez Berrocal, K. F. Witting, H. Ovaa and M. P. C. Mulder, Front. Chem., 2020, 7, 931 CrossRef PubMed
.
- C. Li, T. G. Nelson, A. C. O. Vertegaal and P. Thibault, Nat. Rev. Methods Primers, 2021, 1, 53 CrossRef
.
- R. Yau and M. Rape, Nat. Cell Biol., 2016, 18, 579–586 CrossRef CAS PubMed
.
- Z. Chen, Y. Zhong, Y. Wang, S. Xu, Z. Liu, I. V. Baskakov, M. J. Monteiro, M. Karbowski, Y. Shen and S. Fang, PLoS One, 2013, 8, e73482 CrossRef CAS PubMed
.
- Sjoerd J. L. van Wijk, E. Fiskin, M. Putyrski, F. Pampaloni, J. Hou, P. Wild, T. Kensche, Hernan E. Grecco, P. Bastiaens and I. Dikic, Mol. Cell, 2012, 47, 797–809 CrossRef CAS PubMed
.
- M. J. Pinto, J. R. Pedro, R. O. Costa and R. D. Almeida, Front. Mol. Neurosci., 2016, 43 Search PubMed
.
- Y. Nibe, S. Oshima, M. Kobayashi, C. Maeyashiki, Y. Matsuzawa, K. Otsubo, H. Matsuda, E. Aonuma, Y. Nemoto, T. Nagaishi, R. Okamoto, K. Tsuchiya, T. Nakamura, S. Nakada and M. Watanabe, Autophagy, 2018, 14, 347–358 CrossRef CAS PubMed
.
- M. A. Michel, K. N. Swatek, M. K. Hospenthal and D. Komander, Mol. Cell, 2017, 68, 233–246.e235 CrossRef CAS PubMed
.
- Y. Yu, Q. Zheng, S. K. Erramilli, M. Pan, S. Park, Y. Xie, J. Li, J. Fei, A. A. Kossiakoff, L. Liu and M. Zhao, Nat. Chem. Biol., 2021, 17, 896–905 CrossRef CAS PubMed
.
- Y. J. Shyu, C. D. Suarez and C.-D. Hu, Nat. Protoc., 2008, 3, 1693–1702 CrossRef CAS PubMed
.
- Y. Lee, S. K. Chun and K. Kim, Biochim. Biophys. Acta, Mol. Cell Res., 2015, 1853, 2697–2708 CrossRef CAS PubMed
.
- A. Kamezaki, F. Sato, K. Aoki, K. Asakawa, K. Kawakami, F. Matsuzaki and A. Sehara-Fujisawa, Sci. Rep., 2016, 6, 28873 CrossRef CAS PubMed
.
- S. Domínguez-García, N. Geribaldi-Doldán, R. Gómez-Oliva, F. A. Ruiz, L. Carrascal, J. Bolívar, C. Verástegui, M. Garcia-Alloza, A. J. Macías-Sánchez, R. Hernández-Galán, P. Nunez-Abades and C. Castro, Cell Death Dis., 2020, 11, 262 CrossRef PubMed
.
- M. Schifferer, D. A. Yushchenko, F. Stein, A. Bolbat and C. Schultz, Cell Chem. Biol., 2017, 24, 525–531.e524 CrossRef CAS PubMed
.
- N. Parenti, A. Del Grosso, C. Antoni, M. Cecchini, R. Corradetti, F. S. Pavone and M. Calamai, PeerJ, 2017, 5, e3086 CrossRef PubMed
.
- C. Villegas, V. Muresan and Z. Ladescu Muresan, Hum. Mol. Genet., 2013, 23, 1631–1643 CrossRef PubMed
.
- C. E. DelBove, X.-z. Deng and Q. Zhang, ACS Chem. Neurosci., 2018, 9, 2225–2232 CrossRef CAS PubMed
.
- K. Sappakhaw, K. Jantarug, S. A. Slavoff, N. Israsena and C. Uttamapinant, Angew. Chem., Int. Ed., 2021, 60, 3934–3939 CrossRef CAS PubMed
.
- C. J. Podracky, C. An, A. DeSousa, B. M. Dorr, D. M. Walsh and D. R. Liu, Nat. Chem. Biol., 2021, 17, 317–325 CrossRef CAS PubMed
.
- R. Hussein, R. Hashem and L. Rashed, Front. Mol. Neurosci., 2015, 8, 40 Search PubMed
.
- K. Lang and J. W. Chin, Chem. Rev., 2014, 114, 4764–4806 CrossRef CAS PubMed
.
- L. S. van Husen, S. Schedin-Weiss, M. N. Trung, M. A. Kazmi, B. Winblad, T. P. Sakmar, S. J. Elsässer and L. O. Tjernberg, J. Alzheimer's Dis., 2019, 72, 537–548 Search PubMed
.
- Y. W. Zhang, R. Thompson, H. Zhang and H. Xu, Mol. Brain, 2011, 4, 3 CrossRef CAS PubMed
.
- P. Seubert, C. Vigo-Pelfrey, F. Esch, M. Lee, H. Dovey, D. Davis, S. Sinha, M. Schlossmacher, J. Whaley, C. Swindlehurst, R. McCormack, R. Wolfert, D. Selkoe, I. Lieberburg and D. Schenk, Nature, 1992, 359, 325–327 CrossRef CAS PubMed
.
- E. Q. Jennings, K. S. Fritz and J. J. Galligan, Mol. Aspects Med., 2021, 101053, DOI:10.1016/j.mam.2021.101053
.
- R. Harmel and D. Fiedler, Nat. Chem. Biol., 2018, 14, 244–252 CrossRef CAS PubMed
.
- Q. Zheng, I. Maksimovic, A. Upad and Y. David, Protein Cell, 2020, 11, 401–416 CrossRef CAS PubMed
.
- M. Lo Conte and K. S. Carroll, J. Biol. Chem., 2013, 288, 26480–26488 CrossRef CAS PubMed
.
- R. Tsutsumi, J. Harizanova, R. Stockert, K. Schröder, P. I. H. Bastiaens and B. G. Neel, Nat. Commun., 2017, 8, 1–14 CrossRef CAS PubMed
.
- S. Akter, L. Fu, Y. Jung, M. L. Conte, J. R. Lawson, W. T. Lowther, R. Sun, K. Liu, J. Yang and K. S. Carroll, Nat. Chem. Biol., 2018, 14, 995–1004 CrossRef CAS PubMed
.
- R. N. Hannoush and N. Arenas-Ramirez, ACS Chem. Biol., 2009, 4, 581–587 CrossRef CAS PubMed
.
- M. M. Zhang, L. K. Tsou, G. Charron, A. S. Raghavan and H. C. Hang, Proc. Natl. Acad. Sci. U. S. A., 2010, 107, 8627 CrossRef CAS PubMed
.
- V. Csizmok and J. D. Forman-Kay, Curr. Opin. Struct. Biol., 2018, 48, 58–67 CrossRef CAS PubMed
.
-
Y. Sato and H. Kimura, in Multiplexed Imaging: Methods and Protocols, ed. E. Zamir, Springer US, New York, NY, 2021, pp. 31–41 DOI:10.1007/978-1-0716-1593-5_3
.
- K.-J. Leuchowius, C.-M. Clausson, K. Grannas, Y. Erbilgin, J. Botling, A. Zieba, U. Landegren and O. Söderberg, Mol. Cell. Proteomics, 2013, 12, 1563–1571 CrossRef CAS PubMed
.
- S. Li, Y. Liu, L. Liu, Y. Feng, L. Ding and H. Ju, Angew. Chem., Int. Ed., 2018, 57, 12007–12011 CrossRef CAS PubMed
.
- N. Wu, L. Bao, L. Ding and H. Ju, Angew. Chem., Int. Ed., 2016, 55, 5220–5224 CrossRef CAS PubMed
.
- F. Ma, L. Zhang, B. J. C. Wong and J. Lei, Chem. Commun., 2018, 54, 3648–3651 RSC
.
- A. Ojida, T. Sakamoto, M.-a. Inoue, S.-h. Fujishima, G. Lippens and I. Hamachi, J. Am. Chem. Soc., 2009, 131, 6543–6548 CrossRef CAS PubMed
.
- L. Ge and Y. Tian, Anal. Chem., 2019, 91, 3294–3301 CrossRef CAS PubMed
.
- Y. Hu and J. M. Schomaker, ChemBioChem, 2021, 22, 3254–3262 CrossRef CAS PubMed
.
- M. Lundberg, S. B. Thorsen, E. Assarsson, A. Villablanca, B. Tran, N. Gee, M. Knowles, B. S. Nielsen, E. González Couto, R. Martin, O. Nilsson, C. Fermer, J. Schlingemann, I. J. Christensen, H. J. Nielsen, B. Ekström, C. Andersson, M. Gustafsson, N. Brunner, J. Stenvang and S. Fredriksson, Mol. Cell. Proteomics, 2011, 10, M110.004978 CrossRef PubMed
.
- T. Zimmermann, J. Rietdorf and R. Pepperkok, FEBS Lett., 2003, 546, 87–92 CrossRef CAS PubMed
.
- J. Livet, T. A. Weissman, H. Kang, R. W. Draft, J. Lu, R. A. Bennis, J. R. Sanes and J. W. Lichtman, Nature, 2007, 450, 56–62 CrossRef CAS PubMed
.
- G. Gut, M. D. Herrmann and L. Pelkmans, Science, 2018, 361, eaar7042 CrossRef PubMed
.
- M. Mondal, R. Liao, L. Xiao, T. Eno and J. Guo, Angew. Chem., Int. Ed., 2017, 56, 2636–2639 CrossRef CAS PubMed
.
- Y. Goltsev, N. Samusik, J. Kennedy-Darling, S. Bhate, M. Hale, G. Vazquez, S. Black and G. P. Nolan, Cell, 2018, 174, 968–981.e915 CrossRef CAS PubMed
.
- C. Giesen, H. A. Wang, D. Schapiro, N. Zivanovic, A. Jacobs, B. Hattendorf, P. J. Schüffler, D. Grolimund, J. M. Buhmann, S. Brandt, Z. Varga, P. J. Wild, D. Günther and B. Bodenmiller, Nat. Methods, 2014, 11, 417–422 CrossRef CAS PubMed
.
- M. Angelo, S. C. Bendall, R. Finck, M. B. Hale, C. Hitzman, A. D. Borowsky, R. M. Levenson, J. B. Lowe, S. D. Liu, S. Zhao, Y. Natkunam and G. P. Nolan, Nat. Med., 2014, 20, 436–442 CrossRef CAS PubMed
.
- C. R. Merritt, G. T. Ong, S. E. Church, K. Barker, P. Danaher, G. Geiss, M. Hoang, J. Jung, Y. Liang, J. McKay-Fleisch, K. Nguyen, Z. Norgaard, K. Sorg, I. Sprague, C. Warren, S. Warren, P. J. Webster, Z. Zhou, D. R. Zollinger, D. L. Dunaway, G. B. Mills and J. M. Beechem, Nat. Biotechnol., 2020, 38, 586–599 CrossRef CAS PubMed
.
- J. W. Hickey, E. K. Neumann, A. J. Radtke, J. M. Camarillo, R. T. Beuschel, A. Albanese, E. McDonough, J. Hatler, A. E. Wiblin, J. Fisher, J. Croteau, E. C. Small, A. Sood, R. M. Caprioli, R. M. Angelo, G. P. Nolan, K. Chung, S. M. Hewitt, R. N. Germain, J. M. Spraggins, E. Lundberg, M. P. Snyder, N. L. Kelleher and S. K. Saka, Nat. Methods, 2021 DOI:10.1038/s41592-021-01316-y
.
Footnote |
† These authors contributed equally to this work. |
|
This journal is © The Royal Society of Chemistry 2022 |
Click here to see how this site uses Cookies. View our privacy policy here.