DOI:
10.1039/D2AN01615J
(Paper)
Analyst, 2022,
147, 5283-5292
A colorimetric chemical tongue detects and distinguishes between multiple analytes†
Received
2nd October 2022
, Accepted 17th October 2022
First published on 17th October 2022
Abstract
The rate-limiting step for diagnostics development is the discovery and validation of biomarker analytes. We describe a new analyte-agnostic and label-free approach based on colorimetric reactions involving type I polymerization photoinitiators. We demonstrate that a chemically diverse array of hydrogels embedded with cleaved type I photoinitiators could act as microreactors, undergoing colorimetric reactions with bound analytes. The colorimetric signatures produced were visually distinctive and readable with a flatbed document scanner. Signatures of a broad range of sample types were accurately differentiated by unsupervised clustering without knowledge of any analytes bound to the array. The principles described have the potential to enable scalable and cost-effective analysis of complex samples.
Introduction
Although photoinitiators were originally designed to facilitate the curing of various polymeric materials, they can in theory also be repurposed as colorimetric sensors. For example, when the type I photoinitiator 2,2-dimethoxy-2-phenylacetophenone (DMPA) is exposed to UV light, it undergoes photocleavage, producing free radical fragments which either participate in polymerization reactions or cross-react among themselves to create a spectrum of recombinant species.1 At least some of these recombinant species have the potential to react with analytes to produce colorimetric products. For instance, it is well-known that unreacted photoinitiator molecules undergo slow cleavage reactions to create recombination products which promote the phenomenon of yellowing.2 This yellowing increases with longer exposure to daylight, suggesting that the rate of color development is enthalpically-driven. Color development, although aesthetically undesirable for many commercial plastics, is potentially useful for analyte sensing. Indeed, benzil and benzoin which are related to photocleavage products of Type I photoinitiators2,3 have been shown to be effective spray reagents for visualizing organic compounds on Thin Layer Chromatograms.4
These observations suggest that many photopolymerized materials in common everyday use could be repurposed as chemical sensors with minimal modification. Hydrogels are especially relevant because they have the ability to sequester and concentrate analytes from challenging sample types such as urine.5 Rather than sensing a specific analyte, each hydrogel would bind with a group of analytes compatible with its physicochemical properties. Subsequently, these bound analytes would react with unconsumed photoinitiator species and recombination products to produce color. This type of array, often described as a cross-reactive array6 or chemical-tongue,7,8 would aggregate a larger amount of information than arrays which sense specific analytes. In this work, we describe a simple and economical sample profiling method based on repurposing photopolymerization initiators to create colorimetric cross-reactive chemical arrays.
Results
Photoinitiators produce colorimetric signals in hydrogels
2,2-Dimethoxy-2-phenylacetophenone (DMPA) is one of the most commonly-used initiators for photopolymerization reactions. DMPA, when exposed to ultraviolet (UV) light, splits it into a benzoyl fragment and a ketal fragment, which can further dimerize or recombine to create multiple products1,9,10 (Fig. 1a). Two such products, benzil and benzoin, are known to have colorimetric properties when heated with analytes.4 GC-MS analysis of DMPA cleavage products after a short exposure to UV light showed that benzil and benzoin methyl ether were indeed present (Fig. 1b and ESI Fig. S1†). Further, DMPA when heated in solution produced yellow products but only if exposed to UV light before heating (Fig. 1c). When mixtures containing different ratios of regular DMPA and UV-exposed DMPA were heated, colorimetric products were produced in direct proportion to the relative percentage of UV-exposed DMPA (Fig. 1d). These results together demonstrate that photocleaved DMPA is necessary for colorimetric products to be formed upon heating.
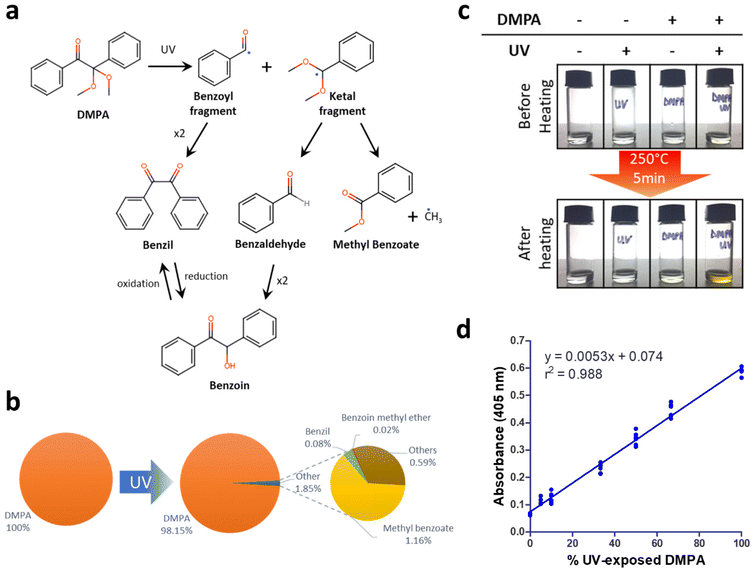 |
| Fig. 1 Pre-exposure of DMPA to UV light enables the generation of colorimetric products when heated. (a) Schematic of radicals formed after DMPA photocleavage as well as their recombinant products. (b) Summary of DMPA photocleavage products detected by GC-MS. (c) Vials of mineral oil with or without DMPA were either pre-exposed or not exposed to UV light for 1 hour before being heated at 250 °C for 5 minutes. Only the sample containing DMPA pre-exposed to UV produced colorimetric products. (d) Mixtures were created with various volumetric ratios of non-UV exposed DMPA and UV-exposed DMPA. Upon heating at 250 °C for 5 minutes, colorimetric products were produced in direct proportion to the relative percentage of UV-exposed DMPA in each mixture. Each data point comprised 6 replicates. | |
Polymers which encapsulate photocleaved DMPA can in theory be used to bind and visualize analytes without the need for labels. Analytes would bind differentially depending on the physical properties of the polymer, while the photocleaved DMPA would enable visualization upon heating. Creating an array of polymers with varying composition would then allow us to create a colorimetric signature, enabled by the variable binding of analytes across the different spots as well as their reaction with photocleaved DMPA.
To investigate this idea, prototype polymer arrays were created, featuring 14 distinct hydrogels (labeled A1 to A14) polymerized with DMPA on a microscope slide. Colorimetric signatures on these arrays were first profiled without analytes in relation to three variables (a) UV exposure time during polymerization, (b) polymeric DMPA concentration and (c) heating temperature. All arrays were imaged using a regular flatbed document scanner and spot intensities were analyzed using ImageJ (Fig. 2a).
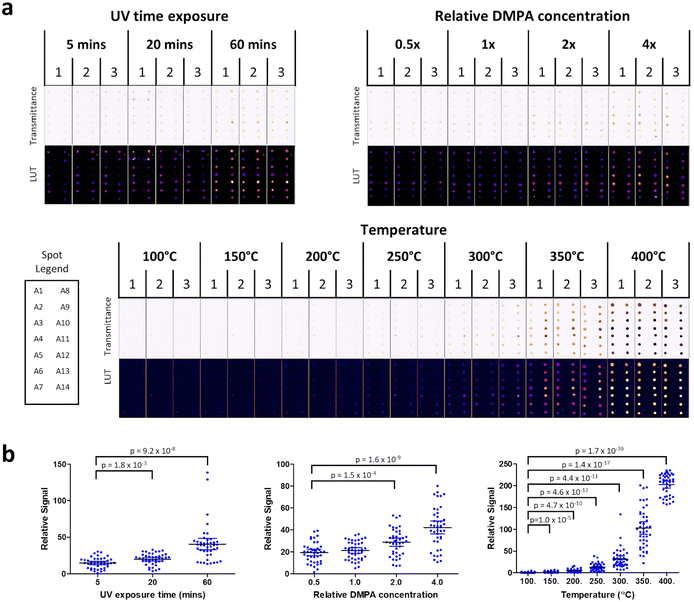 |
| Fig. 2 DMPA-cured hydrogels produce colorimetric signals which are dependent on UV exposure time, DMPA concentration and temperature. (a) An assortment of monomers A1 to A14 containing DMPA as a photoinitiator were photopolymerized on silanized microscope slides as shown in the spot legend. Slides in triplicate were studied in relation to the magnitude of colorimetric signals produced as three parameters were varied: UV exposure time (5, 20, 60 min), relative DMPA concentration (0.5×, 1×, 2×, 4×) and heating temperature (100 °C, 150 °C, 200 °C, 250 °C, 300 °C, 350 °C, 400 °C). Slide images acquired by a flatbed scanner in transmittance mode are shown, along with an inverted LUT (lookup table) version for visual clarity. (b) Scatter plots of the intensity values (relative signal) for each spot are displayed for all tested conditions. Means, 95% CI intervals and p-values are also reported. The data show that the colorimetric signal produced varies positively with increasing UV time, DMPA concentration and temperature. | |
First, UV exposure time was varied (5, 20 or 60 min) while keeping both DMPA concentration (1×) and heating temperature (250 °C) constant. It was observed that colorimetric signals increased with UV exposure. Further, a longer UV curing time was important to guarantee that the spots were fully cured. Hence, UV curing time for subsequent experiments was fixed at 60 min. Next relative DMPA concentration was varied (0.5×, 1×, 2×, 4×) while keeping heating temperature (250 °C) constant. It was observed that color intensity increased in proportion to DMPA concentration. Since colorimetric signals were visible at DMPA 2× and 4× in the absence of any analytes, we picked DMPA 1× concentration for all subsequent experiments because it was the concentration which produced a reasonably low background. Finally, heating temperature was varied (100 °C, 150 °C, 200 °C, 250 °C, 300 °C, 350 °C, 400 °C) while maintaining UV exposure and DMPA concentration at the values fixed from the previous two experiments. Heating temperature produced the largest effect on colorimetric signal, with a dramatic increase at 350 °C and 400 °C in the absence of analytes. To maintain a low background when analytes were absent, all subsequent experiments in this study used a heating temperature of 250 °C, DMPA concentration of 1× and UV curing time of 60 min.
Consistent with the earlier experiments with DMPA in solution, colorimetric signals in the DMPA cured hydrogels were observed within the hydrogel spots with color intensity increasing in proportion to UV exposure, DMPA concentration and temperature (Fig. 2b). Taken together, these data demonstrate that photocleavage products do play a pivotal role in forming colorimetric products. Alongside DMPA, we also tested two alternative photoinitiators: 1-hydroxycyclohexyl phenyl ketone (HCPK) and 2-hydroxy-2-methyl-1-phenyl- 1-propanone (HMPP). Similar results were observed for HCPK and HMPP, with both showing the same UV and concentration dependence in colorimetric signals as DMPA (ESI Fig. S2†). Further, HCPK and HMPP (tested alongside DMPA) also recapitulated the temperature dependence observed for DMPA (ESI Fig. S3†). Taken together, these data show that the observations with DMPA are generalizable to other similar photoinitiators.
Array signatures can distinguish between well-defined analytes
To test real-world analytes, we created an improved array (the ‘α-array’) by expanding the number of spots from 14 to 70 and by varying the constituents of the hydrogels to create diverse combinations of charge, polarity, hydrophobicity and size exclusion.
Such an array works on a very different principle, and would serve a different purpose, from other colorimetric agents used in ELISAs, such as 3,3′,5,5′-tetramethylbenzidine (TMB). Hence, the limitation of the array approach described here is that the limit of detection is high. To study the effective working analyte concentrations which produce colorimetric signals, both glucose and ascorbic acid were tested using the new 70-spot α-array at concentrations ranging from 0 to 55 mM (ESI Fig. S4†). It was observed for both analytes that visible colorimetric signatures were produced at 55 mM and 5.5 mM but not at concentrations below 5.5 mM. In short, the method described here would not be suitable for detecting low concentrations of analyte since its working concentration is within the millimolar range. On the other hand, this array would theoretically be well-positioned to create colorimetric profiles of complex mixtures and a spectrum of analyte classes, without the need for special adaptation.
To investigate the breadth of analytes able to be processed using this array, a panel of 39 samples spanning different analyte classes was assembled. The panel comprised small molecules, proteins, detergents, polymers, salts and complex mixtures. Six replicate arrays were incubated with each sample for 10 minutes, heated at 250 °C for 5 minutes and imaged on a flatbed scanner. These reaction conditions were chosen to minimize the background from reactions within photocleaved species, hence allowing the signal from reactions between photocleaved species and bound analytes to be visualized. Samples could be visually distinguished not just by spot intensity patterns but also by color (Fig. 3). For instance, some samples were blue-shifted while others were red-shifted. At a broad level, the blue shift was associated with analytes which had reducing properties, such as sodium hydroxide, sodium carbonate and cysteine. This observation is consistent with previous data showing that a mixture of benzoin and benzil in a reducing environment produces blue products.11 In contrast, the most red-shifted analytes (e.g. green tea, lysis buffer, milk, collagen) did not share a common theme. Although the exact mechanism is not yet accounted for, a red-shift may possibly be explained by benzil having been shown in previous studies to form red reaction products with many amino acids.4
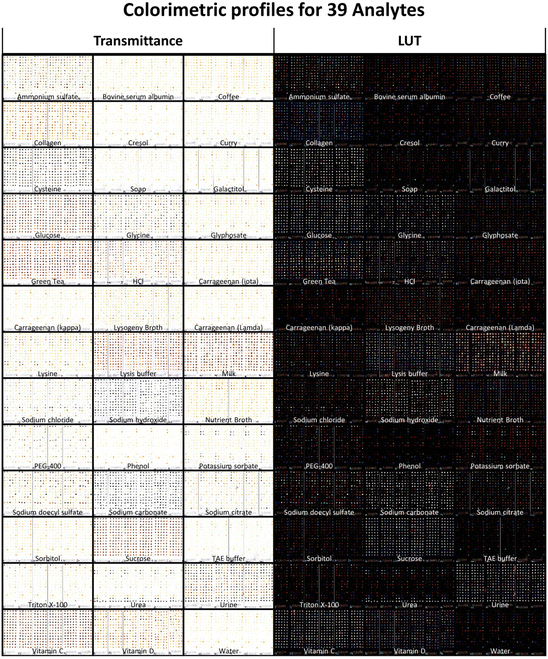 |
| Fig. 3 Visibly distinct colorimetric signatures are produced by various analytes. A total of 39 samples which includes small molecules, detergents, polymers, salts and complex mixtures were tested with 6 arrays by incubating at room temperature for 10 minutes, followed by heating at 250 °C for 5 minutes and imaging on a flatbed scanner. Each analyte exhibits a unique browning and colorimetric profile (transmittance and LUT respectively), hence demonstrating that the polymer array platform can not only detect but also differentiate between different types of samples. | |
Unsupervised hierarchical clustering was performed on all 234 (39 × 6) arrays. Clustering results were near-perfect with 97.8% (229/234) of arrays correctly clustered (Fig. 4). Of the 5 arrays which clustered incorrectly, 4 mis-clustered with other samples which shared similar chemical structures and properties: one glucose array was clustered with sucrose, one lambda-carrageenan array was clustered with kappa-carrageenan and two phenol arrays were clustered with cresol. The remaining mis-clustered array was lysine which was incorrectly clustered with sodium chloride, presumably due to both having fainter signatures. According to the hierarchical clustering dendrogram, urea is the analyte with the greatest distance from the other analytes. This is due to urea's very distinctive profile which features 7 spots at the bottom two rows and 4 spots to the top left exhibiting strong gray coloration, and the remaining spots being relatively low in color. Another relevant observation is that urea is a component of urine, and the urea array accordingly reflects the 7 + 4 gray spots of the urea profile as a subset of its own profile.
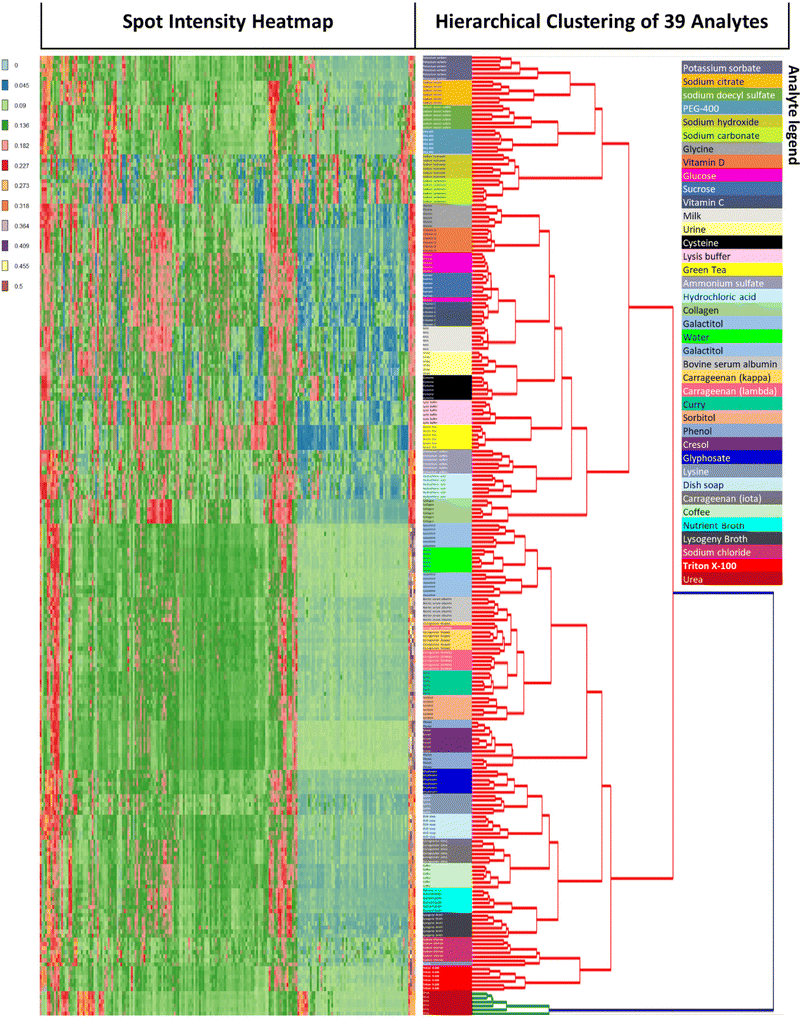 |
| Fig. 4 Colorimetric signatures co-cluster with analyte ID without supervision. Unsupervised hierarchical clustering was performed on 234 α-arrays covering 39 analytes and 6 replicates per analyte. Each analyte is represented in the legend in order of appearance in the dendrogram from top to bottom. Arrays and array spots were both clustered using the Euclidean distance measure. 229 out of 234 arrays (97.86%) were correctly clustered. The five misclassified arrays were 1× Glucose, 1× Carrageenan (lambda), 2× Phenol and 1× Lysine. | |
To our knowledge, this is the first demonstration of an array platform which is able to profile such a broad spectrum of sample types. Many of the samples tested were chemically similar and were yet able to be distinguished. For example, the lambda, kappa and iota forms of carrageenan were clustered separately with the exception of one array. Complex mixtures such as coffee, curry, green tea, LB media, milk and Nutrient Broth were distinguished without error. Also, all salts (potassium sorbate, sodium citrate, sodium carbonate, ammonium sulfate and sodium chloride) clustered perfectly. Given the accuracy of unsupervised clustering, it was not surprising that supervised linear discriminant analysis showed robust clustering of all 39 samples (ESI Fig. S5†).
To further study the data using a machine learning approach, one-shot learning using the k-nearest neighbors algorithm (KNN) was performed on the data set (Fig. 5). Very briefly, one array out of the 6 independent replicate arrays for each of the 39 analytes was randomly selected and used as a training dataset. Each analyte was hence represented by only one training example. The remaining 195 arrays not within the training dataset were then sequentially evaluated using KNN with k = 1 nearest neighbors. KNN classification performed well with an overall accuracy of 96.4% (188/195) and F1 score of 95.9% (Fig. 5 and ESI Fig. S6†). This accuracy was similar to the clustering results, but the spectrum of misclassifications was different from the clustering experiment. Misclassifications of lambda-carrageenan accounted for 4 of the 7 misclassifications. Notably, 3 lambda carrageenan arrays were misclassified as kappa carrageenan. The remaining misclassifications were single events: glucose as sucrose, glyphosate as iota-carrageenan, iota-carrageenan as coffee, and lambda carrageenan as curry. Differences in misclassification are to be expected since KNN will be sensitive to the initial randomly chosen training dataset. Despite having a single training instance per class, KNN classification still managed to achieve 96.4% accuracy, demonstrating that the results were robust.
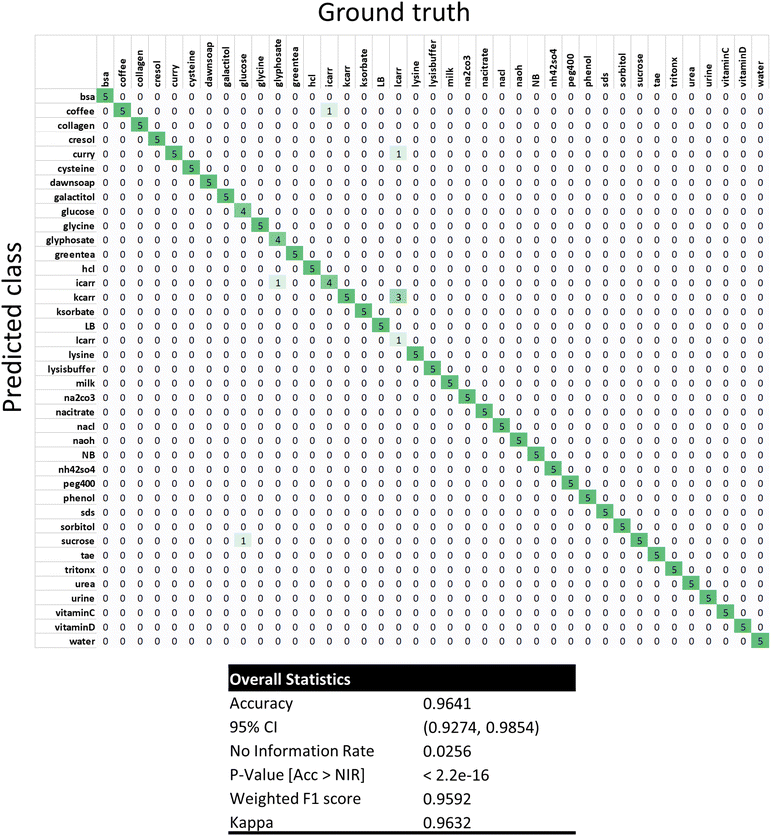 |
| Fig. 5 K-nearest neighbors (KNN) one-shot learning accuracy. One-shot learning using KNN with k = 1 nearest neighbors was performed. A confusion matrix is shown for 39 analytes each represented by 5 independent replicate arrays (total: 195 arrays). The training set for this classification consisted of an independent set of 39 arrays, with each array being the sole training example for one of the 39 analytes. Summary performance statistics are shown in the table below the confusion matrix. | |
Discussion
Hydrogel materials have long been used as polymeric sensors. In fact, hydrogel arrays have been developed before as analyte capture surfaces for analysis by mass spectrometry. The use of hydrogels as a chromatographic surface allows for the capture and immobilization of analytes in a hydrated environment with the hydrogel acting as a MALDI12 or SELDI13–17 target. As a specific example, Grus et al.14 demonstrated that a diversity of chromatographic surfaces (cation exchange, anion exchange, and reverse-phase) could be used with SELDI-TOF-MS to analyze patient tears and to differentiate between patients and dry eye. Hence, it has been known for a long time that hydrogels can play an analyte binding role. In this work, we show that hydrogels can also act as a reaction matrix to produce color as an alternative to using mass spectrometry as a readout for analyte binding. In essence, the very photoinitiators used to cure these hydrogels may also be used to create a visual signal.
Of interest to any chemist would be the mechanism by which colorimetric signals are produced on the array. Our current hypothesis is that colorimetric signals are produced by recombinant products created during UV-cleavage of DMPA, which comprise benzil and benzoin or derivatives thereof (Fig. 1). It is clear from a previous study that benzil or benzoin, when used as TLC spray reagents on the same analytes, are each independently able to produce a different spectrum of colorimetric signals.4 Since both benzil and benzoin are likely to be present in the array spots, the ratio of benzoin to benzil could hence be relevant to what colorimetric products are produced. Analytes could influence color production by skewing this ratio. For example, an analyte could selectively react with and deplete one but not the other. Alternatively, an analyte could promote the oxidation of benzoin to benzil, or conversely, the reduction of benzil to benzoin.18 Other plausibly relevant mechanisms exist. For example, the rearrangement of benzil into benzilic acid and related derivatives have been shown to produce colored products.19,20 Indeed, the colorimetric reaction potential of benzoin and benzil has been amply demonstrated in the literature.11,21 Since these colorimetric reactions are occurring within hydrogels, the reaction environment within these hydrogels (e.g. charge and size exclusion), as well as the type of analytes they bind, are likely to affect what colorimetric products are formed.
The pathways for how such reactions occur within hydrogels are part of ongoing research. The lack of full mechanistic knowledge should not however prevent us from exploring the practical potential of these colorimetric hydrogel arrays. The principle demonstrated in our study will certainly not replace mass spectrometry or ELISAs, since they are magnitudes more sensitive in their limit of detection relative to the hydrogel arrays. However, there are many use cases where analyte concentration is not limiting and where a cost-effective profiling tool based on colorimetric change might be more scalable and effective. These use cases would leverage on the advantages of the hydrogel array approach. One advantage of this approach is the ease of use. Contact with the sample and the application of heat are all that is required for colorimetric signal development. Another advantage is that this approach is analyte agnostic, hence avoiding the biomarker discovery bottleneck in diagnostic test development. The ability to interrogate the total analyte space and draw complex correlations is lost when focusing on a single or few biomarkers. A limitation of signature biomarkers is that the signatures by themselves do not contain knowledge of the analytes associated with a particular sample or disease class. However, if identification of the specific biomarkers is important, one can also use the described platform to identify specific biomarkers bound to the hydrogels which illuminate and rationalize the various signatures associated with a particular disease. Particularly in clinical assay development, the use of photoinitiators already present in hydrogels would remove the need for labels, hence avoiding the problems of label bias, the added effort of labeling reactions and background noise caused by non-specific labeling. Chemical signatures created this way could then be correlated with a desired classification output (e.g. analyte or disease classes) using machine learning techniques.
Methods
Silanization of glass slides
Borosilicate glass slides were initially washed 3 times with distilled water, followed by sonication in absolute ethanol for 5 minutes to remove surface contaminants. Slides were blow dried then completely dried in an oven at 120 °C for 5 minutes followed by coating with 3-methacryloxypropyltrimethoxylsilane through vapor deposition overnight at 93 °C and 580 mmHg. Thereafter, slides were dried in an oven at 120 °C for 1 hour.
Heating of UV-exposed DMPA in mineral oil
A DMPA solution was prepared by dissolving 30.6 mg of DMPA in 400 μl of DMSO and adding this solution to 3.6 ml of mineral oil in a 15 mL tube. The mixture was vortexed vigorously for a few seconds. 400 μl of the DMPA-mineral oil mixture was then added to each of two glass vials. One vial was exposed to UV light for 60 minutes (min) using a Biorad UV transilluminator (302 nm) and the other was used as a ‘non-UV exposed’ control. Additionally, controls without DMPA were also prepared by adding 400 μl of DMSO to 3.6 mL of mineral oil and vortexing vigorously for a few seconds. In a similar fashion, 400 μl of the DMSO-mineral oil mixture was then added to each of two glass vials. One vial was exposed to UV light for 60 min and the other was used as a non-UV control. All 4 samples were then heated on a hot plate at 250 °C for 5 min, and subsequently cooled to room temperature for 30 min. The related study on various ratios of Regular DMPA
:
UV-exposed DMPA (termed UV−
:
UV+) was carried out as follows. A DMPA solution was prepared by dissolving 1.5 g of DMPA in 6 ml of DMSO. Two glass vials of 700 μl DMPA were prepared and one was exposed to UV (365 nm) for 1 hour while the other was kept in the dark. Various UV−: UV+ ratios by volume were prepared in a final volume of 100 μl. To each preparation was added a reaction buffer of 100 μl DMSO + 3.8 ml Mineral Oil. For the DMPA negative control, 100 μl of DMSO was substituted for the DMPA solution. All 4 samples were heated on a hot plate at 250 °C for 5 min, and subsequently cooled to room temperature for 30 min. Spectrophotometry (Molecular Devices Emax Precision) was performed by reading the absorbance (405 nm) of each sample (read volume: 300 μl).
Mass spectrometry of DMPA exposed to UV
DMPA was dissolved in acetonitrile at 20 mg ml−1 and then either subjected to UV exposure, or no UV exposure as a negative control. The DMPA solutions were then transferred to new glass vials and analyzed by GCMS (Agilent). Column parameters were: Agilent® J&W™ DB-5 ms GC column, 30 m, 0.25 mm, 0.25um, 7 inch cage. Oven program was as follows: (1) 50 °C for 5 min; (2) 10 °C min−1 to 150 °C for 5 min; (3) 25 °C min−1 to 250 °C for 5 min. Solvent delay: 7.00 min. Low Mass: 50.0. High Mass: 500.0.
Photoinitiator (PI) solutions
PI solutions for DMPA and HCPK were prepared by combining 100 μl of 0.255 g ml−1 PI (in DMSO), 80 μl Glycerol, 400 μl distilled water and 3.42 ml DMSO. PI solution for HMPP was prepared by combining 30 μl of 1.04 g ml−1 PI (in DMSO), 80 μl Glycerol, 400 μl distilled water and 3.49 ml DMSO.
Single monomer arrays containing polymers A1 to A14
10 μl of DMPA PI solution was added to 10 μl of 14 monomer stock solutions labeled A1 to A14 (which were respectively acrylamide, 2-carboxyethyl acrylate, acrylic acid, 2-cyanoethyl acrylate, N-[tris(hydroxymethyl)methyl] acrylamide, hydroxypropyl acrylate isomers, 4-hydroxybutyl acrylate, N-hydroxyethyl acrylamide, N,N-dimethylacrylamide, N-isopropylacrylamide, N-(1,1-dimethyl-3-oxobutyl) acrylamide, 2-methacryloxyethyl phenyl urethane, 1-acryloyloxy-3-(methacryloyloxy) 2-propanol and ethylene glycol phenyl ether acrylate) and mixed well with a pipet. Arrays were created by spotting 1.5 μl of each solution onto a silanized glass slide and polymerized with a 302 nm UV transilluminator (Biorad 2000 UV transilluminator) for 60 min. UV light exposure catalyzes both the curing of the polymer and also the covalent attachment of the polymers onto the silanized glass surface.
DMPA, HCPK and HMPP temperature study using single monomer arrays
Single Monomer Arrays were made as described using PI solutions containing either DMPA, HCPK or HMPP. Three columns of polymers A1 to A14 were spotted, each photopolymerized with either DMPA, HCPK or HMPP. The arrays were then heated at varying temperatures on a heated plate at 100 °C, 150 °C, 200 °C, 250 °C, 300 °C, 350 °C or 400 °C for 5 min. The surface temperature of the heated plate was verified by an infrared thermometer during the heating process. The arrays were then digitally scanned for image analysis. All data points were generated in triplicate.
Digital scanning and data analysis of single monomer array data
Slides were scanned with a digital scanner (EPSON Perfection v500) at 1200 dpi and saved as JPEG images. The images were then converted to 8-bit grayscale and inverted. A central circular region of standardized area within each spot was selected using the area selection tool and analyzed by Image J software. Mean intensity values were measured for each spot. Additionally, background mean intensity values were also measured for each array. All spot mean intensity values were then background subtracted and then plotted against heating temperature for each photo-initiator species (DMPA, HCPK, HMPP) All data points were generated and analyzed in triplicate. Statistical analyses were performed using the Student's t-test to compare each data point against heating at 100 °C.
Hydrogel arrays
The α-arrays were created as described in the following patent application: Piloto, Obdulio, and Cheong, Ian Shen-Yi. 2016. Detectable arrays, systems for diagnosis, and methods of making and using the same. USPTO 20160274103:A1. US Patent, filed September 22, 2014, and issued September 22, 2016. Details of how these α-arrays were constructed are described in the ESI† Materials and methods.
Profiling of 39 analytes using α-arrays
A total of six α-arrays were incubated with each of 39 analytes for 10 min at room temperature. The analytes tested were as follows: Green Tea Extract, Lambda Carrageenan, Kappa Carrageenan, Iota Carrageenan, Coffee, Curry Powder all at 0.1% w/v; Sodium Chloride, Sodium Citrate, Sodium Carbonate, Ammonium Sulfate, Potassium Sorbate, HCl, NaOH, Urea, Sucrose, Glucose, Galactitol, Sorbitol, PEG400, SDS, Triton X-100, Glycine, Lysine, Cysteine, Collagen, Glyphosate, Bovine Serum Albumin, Phenol, m-Cresol, Vitamin C, Vitamin D, Dawn Ultra Soap all at 0.1% (w/v for solids, v/v for liquids); Whole milk (10% w/v); Human urine (40% v/v); TAE buffer, Lysis buffer from Applied Biosystems, Lysogeny Broth, Nutrient Broth all at 1X concentration; and Distilled water. After incubation, the arrays were removed and air dried at room temperature for 20 min and further dried on a heated plate at 100 °C for 20 min. Subsequently, the arrays were heated on a heated plate at 250 °C for 5 min to develop their colorimetric signal profiles. Arrays were scanned with a digital scanner (EPSON Perfection v500) at 1200 dpi and saved as RGB JPEG images. Each array spot was cropped using a fixed window size and then split into R, G and B channels for further analysis. The R, G and B intensities of each spot were obtained by summing the respective R, G and B pixel intensities for each spot window. A vector of 210 spot intensities (70 spots × 3 color channels) was obtained from each array data point. Each vector was ‘L1’ normalized. Unsupervised hierarchical clustering analysis was performed using the hierarchical_clustering.py software authored by Nathan Salomonis (J. David Gladstone Institutes, San Francisco California). ‘L2’ was used for both the row-wise and column-wise metrics. A heatmap was generated using the color gradient ‘gist_ncar’. To score the clustering results, misclassified arrays were first identified using the following two step process. Step 1: for each class of 6 arrays, identify the largest contiguous cluster. Step 2: any array which was not part of this largest contiguous cluster was considered misclassified. The clustering accuracy was thus calculated as (Total # of arrays − # of misclassified arrays)/Total # of arrays × 100%. For visualization, scanned array images were montaged using ImageJ. An ‘LUT’ (Look Up Table) version was also created by inverting the montage and applying the ‘brgbcmyw’ LUT in ImageJ. 2D and 3D Linear Discriminant Analyses were performed using the sklearn.lda.LDA module in the scikit-learn 0.16.1 library package. The general color profile of the scanned array images was obtained by using the Color Inspector 3D plugin in ImageJ to analyze the montage of 39 × 6 original array images.
One-shot learning using K-nearest neighbors (KNN)
The dataset generated from 39 analytes and 6 replicates were further analyzed by KNN. A vector of 210 spot intensities (70 spots × 3 color channels) was obtained from each array data point. Each vector was ‘L1’ normalized. KNN classification was performed using R (v 4.2.1). One array out of the 6 independent replicate arrays for each of the 39 analytes was randomly selected and used as a training dataset. The remaining 195 arrays not within the training dataset were sequentially evaluated using KNN with k = 1 nearest neighbors. The confusion matrix and all performance statistics were generated using the caret package.
Author contributions
Investigation (F. B. H. L., T. L., G. F., N. L., A. C., A. V., A. Nagesetti, A. Nelson, O. P., I. C.). Writing – original draft (F. B. H. L., T. C. C., K. S., O. P., I. C.). Writing – review & editing (F. B. H. L., T. C. C., O. P., I. C.). Validation (F. B. H. L., T. L., I. C.). Data curation (T. L., I. C.). Methodology (F. B. H. L., I. C.). Supervision (T. L., O. P.). Conceptualization (O. P., I. C.). Project administration (F. B. H. L.,). Formal analysis (I. C.). Funding acquisition (O. P., I. C.). Software (I. C.). Visualization (I. C.).
Data availability
All data is available in the main text or the ESI.†
Code availability
All experiments and implementation details are described in sufficient detail to support replication with non-proprietary libraries.
Conflicts of interest
F. B. H. L., T. L., O. P. and I. C. are co-inventors on patents regarding the published subject matter. F. B. H. L., T. L., A. Nagesetti, T. C. C., O. P. and I. C. hold stock and/or options in Entopsis. T. C. C. and K. S. are clinical advisers to Entopsis.
Acknowledgements
Research reported in this publication was supported by Entopsis Inc and a grant from the Thiel Foundation's Breakout Labs.
References
- H. Fischer, R. Baer, R. Hany, I. Verhoolen and M. Walbiner, J. Chem. Soc., Perkin Trans. 2, 1990, 2, 787–798 RSC.
- N. Arsu, R. S. Davidson and R. Holman, J. Photochem. Photobiol., A, 1995, 87, 169–175 CrossRef CAS.
- J. E. Baxter, R. S. Davidson, H. J. Hageman, G. T. M. Hakvoort and T. Overeem, Polymer, 1988, 29, 1575–1580 CrossRef CAS.
- E. O. Dare and A. S. Ajibola, Chromatographia, 2007, 66, 823–825 CrossRef CAS.
- T. A. Douglas, D. Tamburro, C. Fredolini, B. H. Espina, B. S. Lepene, L. Ilag, V. Espina, E. F. Petricoin 3rd, L. A. Liotta and A. Luchini, Biomaterials, 2011, 32, 1157–1166 CrossRef CAS PubMed.
- K. J. Albert, N. S. Lewis, C. L. Schauer, G. A. Sotzing, S. E. Stitzel, T. P. Vaid and D. R. Walt, Chem. Rev., 2000, 100, 2595–2626 CrossRef CAS PubMed.
- B. Rout, L. Motiei and D. Margulies, Synlett, 2014, 1050–1054 Search PubMed.
- R. Peri-Naor, Z. Pode, N. Lahav-Mankovski, A. Rabinkov, L. Motiei and D. Margulies, J. Am. Chem. Soc., 2020, 142, 15790–15798 CrossRef CAS PubMed.
- H. F. Gruber, Prog. Polym. Sci., 1992, 17, 953–1044 CrossRef CAS.
- M. R. Sandner and C. L. Osborn, Tetrahedron Lett., 1974, 15, 415–418 CrossRef.
- T. Abe and M. Iwaizumi, BCSJ, 1974, 47, 2593–2594 CrossRef CAS.
- T. W. Hutchens and T.-T. Yip, Rapid Commun. Mass Spectrom., 1993, 7, 576–580 CrossRef CAS.
- G. Reddy and E. A. Dalmasso, J. Biomed. Biotechnol., 2003, 2003, 237–241 CrossRef PubMed.
- F. H. Grus, V. N. Podust, K. Bruns, K. Lackner, S. Fu, E. A. Dalmasso, A. Wirthlin and N. Pfeiffer, Invest. Ophthalmol. Visual Sci., 2005, 46, 863–876 CrossRef PubMed.
- M. P. van Dieijen-Visser, J. A. P. Bons, D. de Boer and K. W. H. Wodzig, Ned. Tijdschr. Klin. Chem. Laboratoriumgeneeskd., 2007, 32, 88–93 Search PubMed.
- E. Fung, D. Diamond, A. H. Simonsesn and S. R. Weinberger, Methods Mol. Med., 2003, 86, 295–312 CAS.
- S. Vorderwülbecke, S. Cleverley, S. R. Weinberger and A. Wiesner, Nat. Methods, 2005, 2, 393–395 CrossRef.
- A. Lachman, J. Am. Chem. Soc., 1923, 45, 1529–1535 CrossRef CAS.
-
S. Selman, PhD thesis, University of Tennessee – Knoxville, 1959.
-
Comprehensive Organic Chemistry Experiments for the Laboratory Classroom, ed. C. A. M. A. Afonso, N. R. C. Candeias, S. D. Pereira, A. F. Trindade, J. A. S. Coelho, B. Tan and R. Franzén, Royal Society of Chemistry, 2016 Search PubMed.
- J. L. Ihrig and R. G. Caldwell, J. Am. Chem. Soc., 1956, 78, 2097–2101 CrossRef CAS.
|
This journal is © The Royal Society of Chemistry 2022 |