DOI:
10.1039/D1TA01299A
(Paper)
J. Mater. Chem. A, 2021,
9, 10466-10476
Combinatorial and machine learning approaches for the analysis of Cu2ZnGeSe4: influence of the off-stoichiometry on defect formation and solar cell performance†
Received
12th February 2021
, Accepted 7th April 2021
First published on 19th April 2021
Abstract
Solar cells based on quaternary kesterite compounds like Cu2ZnGeSe4 are complex systems where the variation of one parameter can result in changes in the whole system, and, as consequence, in the global performance of the devices. In this way, analyses that take into account this complexity are necessary in order to overcome the existing limitations of this promising Earth-abundant photovoltaic technology. This study presents a combinatorial approach for the analysis of Cu2ZnGeSe4 based solar cells. A compositional graded sample containing almost 200 solar cells with different [Zn]/[Ge] compositions is analyzed by means of X-ray fluorescence and Raman spectroscopy, and the results are correlated with the optoelectronic parameters of the different cells. The analysis results in a deep understanding of the stoichiometric limits and point defects formation in the Cu2ZnGeSe4 compound, and shows the influence of these parameters on the performance of the devices. Then, intertwined connections between the compositional, vibrational and optoelectronic properties of the cells are revealed using a complex analytical approach. This is further extended using a machine learning algorithm. The latter confirms the correlation between the properties of the Cu2ZnGeSe4 compound and the optoelectronic parameters, and also allows proposing a methodology for device performance prediction that is compatible with both research and industrial process monitoring environments. As such, this work not only provides valuable insights for understanding and further developing the Cu2ZnGeSe4 photovoltaic technology, but also gives a practical example of the potential of combinatorial analysis and machine learning for the study of complex systems in materials research.
Introduction
Cu2ZnSn(S,Se)4 (CZTSSe) based compounds, more widely known as kesterites, are considered as the natural earth-abundant and low toxicity successors of the more mature inorganic thin film photovoltaic (PV) technologies Cu(In,Ga)Se2 (CIGS) and CdTe which are based on scarce and/or toxic materials.1 Although a considerable amount of progress in the technological development and fundamental understanding of kesterites has been achieved in the last years, the record power conversion efficiency at laboratory scale has barely evolved since 2014 and is stagnated at around 13%.2 Sn is often regarded as the main culprit of this stagnation due, mainly, to the volatility of Sn(S,Se)x species3 that leads to morphological and compositional problems,4 and the instability of the Sn oxidation state that may lead to the formation of deep defects5,6 ultimately causing kesterite PV devices to exhibit a high Voc deficit. In this regard, the substitution of Sn by Ge is currently regarded as a promising strategy to improve the kesterite technology. Ge doping (CZTSSe:Ge) and alloying (CZTGSSe) has been demonstrated to enhance the performance of kesterite devices significantly by improving the Voc of the devices which is commonly attributed to the formation of liquid phases and better intermixing during high temperature synthesis, to improvements in carrier lifetime and to a reduction of band tailing.5,7–9 In addition, the partial substitution of Sn by Ge increases the bandgap of the kesterite semiconductor material enabling the creation of a graded bandgap through the development of in-depth compositional engineering strategies.10,11
On the other hand, the total substitution of Sn by Ge (CZGSSe) appears as an even more promising approach since, in addition to completely avoiding Sn-related issues, the wider bandgap of the material (∼1.5 eV for CZGSe and ∼2.2 eV for CZGS)12,13 opens the door to semi-transparent, tandem and photocatalytic water splitting applications. Significant advances have been made in the last years in pure Ge kesterite solar cells leading to CZGSe devices with efficiencies of up to 8.5%.14 Although promising, this value is very far from those of the best CZTSe devices. The highest efficiency levels reported for CZGSe are commonly achieved imitating the standard CZTSe and employing off-stoichiometry Cu-poor Zn-rich absorber compositions.14–17 However, this compositional ratio might not be the optimal one for CZGSe and might be one of the reasons holding back the development of this technology towards higher efficiencies as compared with the standard CZTSe. As such, fundamental studies that investigate the formation of CZGSe, secondary phases and point defects off-stoichiometry represent a very valuable asset for understanding this material and for paving the way to the development of strategies that may lead to a further development of the technology. In this context, Gunder et al. carried out a detailed investigation of defect formation in off-stoichiometric CZGSe powder.18 However, mainly Zn-rich and Cu-rich powder samples were synthesized, with few Cu-poor samples.
Finally, solar cells based on quaternary kesterite compounds and multilayer stacks like CZGSe are complex systems where the variation of one parameter can result in changes in the whole system, and, as consequence, in the global performance of the devices. In this way, analyses that take into account this complexity are necessary in order to overcome the existing limitations of this promising Earth-abundant photovoltaic technology.
In this work, we present a systematic study of a combinatorial CZGSe sample comprised by almost 200 individual solar cells with different [Zn]/[Ge] compositions in the Cu-poor regime. Structural, compositional and optoelectronic characterization are applied in a combinatorial way. Firstly, a complex analytical approach allows defining the off-stoichiometric limits of formation of the CZGSe kesterite phase and the optimum compositional range to obtain the highest efficiencies (up to 6.3%) in terms of the [Zn]/[Ge] ratio. This includes the study of solar cell performance dependence on the concentration of point defects. Secondly, we demonstrate the potential of applying machine learning (ML) for the analysis of the combinatorial sample. The ML methodology proves to enable effective prediction of cell efficiency based only on Raman spectra and compositional data, while the resulting discriminants show a linear correlation with point defect concentration and device efficiency pointing at a strong fundamental interconnection between point defects, Raman spectra, composition and cell performance. This works serves both as a fundamental study that provides valuable results for the development of the CZGSe technology and as a powerful example of how combinatorial analysis and machine learning can be used to unravel the critical parameters that govern the performance of complex optoelectronic devices.
Experimental details and methods
Sample preparation
A CZGSe combinatorial sample was prepared through the selenization of a compositionally graded Cu/Zn/Ge metallic stack precursor deposited by DC magnetron sputtering (Alliance AC450) on a 5 × 5 cm2 soda-lime glass/Mo substrate. In order to generate a compositional gradient, the Cu and Zn precursor layers were homogeneously deposited over the substrate while the Ge layer was deposited without substrate rotation generating a thickness gradient and, in turn, a [Zn]/[Ge] compositional gradient. A 3-zone tubular furnace (Hobersal) was employed to synthesize the CZGSe absorber. The 3 zones were kept at the same temperature during the whole process to ensure spatial homogeneity throughout the entire length of the furnace. Samples were placed inside a graphite box (69 cm3) together with crucibles containing 100 mg of Se (Alfa-Aesar powder, 200 mesh, 99.999%) and 5 mg of GeSe2 (American Elements, power, 99.999%) to perform a 2-step reactive thermal annealing in a Se + Ge atmosphere. It consisted in a first stage in which the furnace was kept at 330 °C and 1.5 mbar Ar pressure for 30 minutes and a second step at 480 °C and 1 bar Ar pressure for 15 minutes. The heating rate was set to 20 °C min−1 in both steps. The samples were let to cool down naturally.
The as-synthesized absorber was submitted to a chemical etching in diluted KCN (2% w/v, room temperature, 2 min). Immediately after, a CdS layer was deposited by chemical bath deposition (the process is detailed in ref. 19). The solar cell structure was then completed with i-ZnO (50 nm) and ITO (200 nm, 60 Ω sq−1 sheet resistance) layers deposited by DC-magnetron sputtering (Alliance Concept CT100). The sample was then scribed into 196 individual 3 × 3 mm2 solar cells (see Fig. S1†) using a manual microdiamond scriber (MR200 OEG). Neither anti-reflective coating nor metallic grids were used in the devices presented in this work.
Characterization techniques
The elemental composition of the different cells of the combinatorial sample was determined by X-ray fluorescence (XRF) using a Fischerscope XDV system with a 1 mm spot diameter, a 50 kV acceleration voltage, a Ni10 filter and a 45 s acquisition time. Raman analysis with blue (442 nm) and green (532 nm) excitation wavelengths were performed on the bare absorber, while measurements with NIR (785 nm) were performed in complete devices using Horiba Jobin Yvon FHR640 and iHR320 monochromators coupled with CCD detectors. The first monochromator is optimized for the UV and visible spectral ranges and was used with 442 nm (He–Cd gas laser) and 532 nm (solid state laser) excitation wavelengths. The second monochromator is optimized for the NIR range and was used with a 785 nm (solid state laser) excitation wavelength. The power density of the lasers was kept below 150 W cm−2 and the spot size was ∼70 μm. The measurements were performed in a backscattering configuration through a specific probe designed at IREC.
The J–V characteristics of the devices were obtained under simulated AM1.5 illumination (1000 W m−2 intensity at room temperature) using a pre-calibrated Class AAA solar simulator (Abet Technologies Sun 3000).
Machine learning methodology
A machine learning (ML) driven methodology based on a linear discriminant analysis (LDA) algorithm was employed to deepen into the complex dependence of solar cell optoelectronic parameters and composition on the different parameters found in the analysis of the Raman spectra. LDA is a dimension-reduction algorithm, capable of reducing high-dimensionality problems into a bi-dimensional one, discerning and employing the most relevant dimensions of the dataset. In order to test and implement the machine learning based LDA algorithm, the Python programing environment20 with the Scikit-Learn library21 was used. All the Raman spectra measured under different excitation conditions for each cell were used as input features (588 spectra, in total), and the data were randomly divided in 70% for training and 30% for testing. The algorithm was trained for 3 different classification targets, namely [Zn]/[Ge] ratio, Voc and efficiency. For each trained algorithm, the data was divided in 4 classification groups of approximately an equal amount of data. The amount of experimental data employed for the analysis (196 cells) is far from being considered “big data” and the results presented below are susceptible to further improvement for a more precise classification through the use of a higher number of training inputs. Nevertheless, this approach illustrates the applicability and potential of this methodology for material analysis by spectroscopic techniques.
Results and discussions
Off-stoichiometry limits and secondary phases
The chemical composition of every individual solar cell of the combinatorial samples was obtained by XRF. The mappings of the cationic ratios are presented in the Fig. S1† and, in Fig. 1, the obtained values are combined with the different off-stoichiometry kesterite types (see ref. 22 and 23 for more details about the off-stoichiometry lines of kesterite type compounds). It can be observed that the compositions of the different cells of the combinatorial samples cross the A- (almost perpendicularly), J- and L-type lines. On the one hand, the [Zn]/[Ge] ratio covers a wide range from almost 0.7 to 1.4, allowing to explore not only the typical Zn-rich compositions commonly used for kesterite type compounds, but also the Zn-poor region. This allows investigating the origin of the positive effect of Zn-rich compositions in kesterite PV devices. On the other hand, the [Cu]/([Zn]+[Ge]) ratio was maintained well below 1, ensuring the Cu-poor condition for all cells. It is worth mentioning that the non-stoichiometric compositions were quite different from previous studies of the same compound,18 where mainly Zn-rich and Cu-rich powder samples were synthesized and the few Cu-poor samples contained secondary phases.
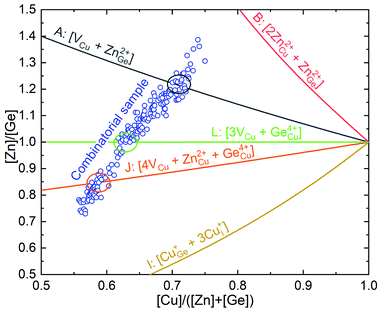 |
| Fig. 1 Cationic ratios of the CZGSe combinatorial sample (blue open circles) represented together with off-stoichiometric types of kesterite compounds (solid lines).22,23 | |
Raman spectroscopy was used as the main tool to analyse phase formation in the CZGSe absorber layer with varying [Zn]/[Ge] ratio. A multiwavelength analysis allowed to detect possible secondary phases and variations in the main kesterite phase (see Fig. 2). The blue excitation wavelength is well-known to be highly sensitive for detecting the ZnSe secondary phase.22,24 As shown in the figure, this secondary phase was found in cells with Zn-rich composition (see the spectrum of the [Zn]/[Ge] = 1.25 cell in Fig. 2, left). What is more, the cells on which a strong ZnSe peak was detected, also presented a shift to lower wavenumbers and an increase of the full width at half maximum (FWHM) of the main and the second most intense peaks of the kesterite phase under different excitation wavelengths not sensitive to ZnSe (see the spectrum of a cell with [Zn]/[Ge] = 1.25 under green excitation wavelength in Fig. 2, middle). This, according to the previous interpretations of the kesterite type compounds, can be related with an increased Cu/Zn disorder25 or with a phonon confinement effect due to a low grain size in the absorber.26 Since all the cells of the combinatorial sample were processed at the exact same temperature, the appearance of strong variations in the Cu/Zn disordering is unlikely (e.g. see ref. 27 and 28). In addition, it is hard to envision how the formation of the ZnSe phase could influence Cu/Zn disordering in the CZGSe compound. On the other hand, even taking into account the optimal temperature treatment for the formation of good crystalline quality CZGSe phase,16,29 the presence of ZnSe grains can greatly influence the formation and size of the kesterite grains,30 leading to a worsening of its crystalline quality and grain size, and causing the appearance of phonon confinement in agreement to the observed red shift and broadening of the kesterite Raman peaks.
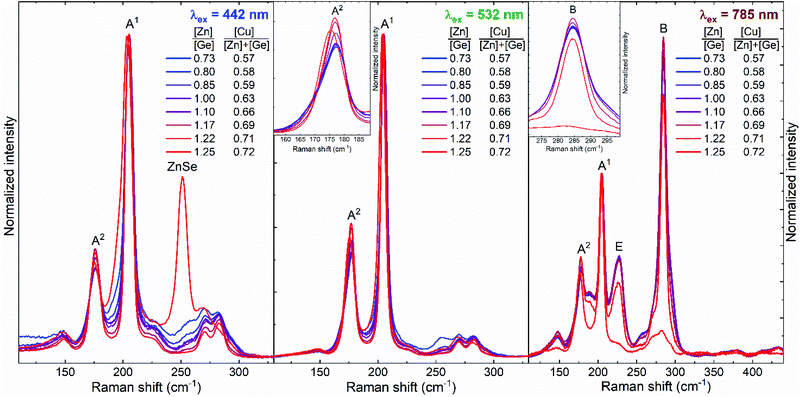 |
| Fig. 2 Examples of Raman scattering spectra measured in cells with different compositions under different excitation wavelength. | |
In addition to the ZnSe phase, the blue excitation wavelength is also sensitive to the GeSe2 phase. This is a 2D compound that has a direct band gap of ∼2.7 eV (close to the energy of the blue laser line −2.8 eV) and has its main Raman peak at around 210 cm−1.31 The latter is strongly overlapped with the main peak of CZGSe (A1 symmetry peak at 205 cm−1), which compromises the detection of this secondary phase. However, a strong resonance with the blue excitation wavelength should result in the appearance of, at least, a shoulder at the high wavenumber side of the main kesterite peak. However, the spectra in Fig. 2 (left) show no evidence of the presence of GeSe2, even for the lowest [Zn]/[Ge] ratios (highest Ge-content). Another Ge-based binary compound is GeSe. The orthorhombic crystalline polymorph of this binary compound has a direct band gap of about 1.53 eV and an intense A1 symmetry mode at 188 cm−1.32 This band gap value is very close to the resonant condition of this secondary phase under a 785 nm excitation wavelength. Nevertheless, no clear Raman peak of GeSe phase can be observed in the spectra acquired (Fig. 2, right). On the other hand, although the properties of the amorphous GexSe1−x phase strongly depend on the x value, an intense Raman band close to 200 cm−1 and assigned to the stretching mode of the GeSe4/2 corner-sharing tetrahedra can be defined as representative for most of the compositional polymorphs of these amorphous phases.33 Moreover, a GeSe9 liquid phase, recently found as one of the intermediate phases during the formation of CZGSe,16 might remain at the surface of the absorber layer. In the spectra measured under the green excitation wavelength, a slight broadening of the main peak of CZGSe with the decreasing [Zn]/[Ge] ratio can be observed. However, it cannot be unequivocally ascribed to the presence of amorphous GexSe1−x or liquid GeSe9 secondary phases since it could also be explained by intrinsic changes in the kesterite phase, that will be further discussed. Finally, elemental Ge and Ge-containing ternary phases (like Cu2GeSe3 or Cu2GeSe4) can be formed in very Zn-poor conditions. Although a good sensitivity of Raman spectroscopy to these phases is expected, their narrow band gap (below 1 eV, see ref. 34–37 for band gap and fingerprint Raman spectra) does not allow working in resonant conditions making the detection of small amounts of elemental Ge and Ge-containing ternary phases very challenging. In this way, it can be concluded that no Ge-related secondary phases are forming even for very Zn-poor compositions, or that the amount of these secondary phases is negligibly small, as no strong/sharp changes of the spectra of the cells of the CZGSe combinatorial sample with different compositions can be observed (in the [Zn]/[Ge] = 0.7–1.2 range). This differentiates the pure Ge-based from the pure Sn-based kesterites, where the formation of Sn-containing secondary phases has been observed even at Zn-rich compositions.38,39 Finally, it should be noted that no Cu-containing binary secondary phases are expected due to Cu-poor composition of all the cells of the combinatorial sample (see Fig. 1).
According to the phase formation analysis performed by means of Raman spectroscopy presented above, only a ZnSe secondary phase was clearly detected in the combinatorial sample under Zn-rich compositions. ZnSe becomes the dominant phase for [Zn]/[Ge] > 1.2 in some of the cells. This squeezes the upper limit in which the pure CZGSe kesterite phase can be formed to [Zn]/[Ge] ratios close to 1.2. On the contrary, the lower limit for the formation of the pure CZGSe kesterite phase can be considered close to 0.7, comparable with CZTSSe compounds.22,38 However, the presence of small amount of Ge-based secondary phases is hard to exclude from the data presented. Nevertheless, taking into account previous studies18,22 and the results presented here, it can be concluded that replacing of Sn with Ge does not lead to a significant shortening of the off-stoichiometry range for the formation of the CZGSe kesterite type structure. Likewise, the stoichiometry flexibility of CZGSe compounds might be one of the culprits for the existing limitations of the PV devices in the same way as for other kesterite-based compounds.4
Point defect formation
As mentioned above (see Fig. 1), the chemical composition of most of the cells is in the range from J-to A-type kesterite off-stoichiometric lines. According to this, the main point defect expected in the combinatorial sample are copper vacancies (VCu) and zinc or germanium in copper position (ZnCu and GeCu).22,38,40 In order to define the influence of these point defects on the Raman spectra, specific cells with chemical compositions close to the three off-stoichiometric lines crossed by the combinatorial sample (A-, J- and L-type lines) were analysed. Measurements under different excitation wavelengths resulted in the detection of the characteristic features that differentiate the Raman spectra of the different off-stoichiometry types of the CZGSe kesterite compound (see Fig. 3). Here, the spectra of the cells around the J- and L-type lines present a great similarity with just very subtle changes in the intensity of the band at 176 cm−1 and E/B symmetry peaks in the high wavenumber range (220–300 cm−1), which are mainly observed under 785 nm excitation (Fig. 3, right). In contrast, strong differences are observed for the spectra of the cells close to the A-type line with a decrease of the relative intensity and width of the peaks, except for the band at 176 cm−1. In previous works, the change in the intensity of the second most intense Raman band in the CZTSe compound was correlated, mainly, with a change of the concentration of VCu point defects, and was shown to have a crucial impact on the properties of the CZTSe absorber and on device performance.41–43 Taking this into account, it can be inferred that in the combinatorial sample analysed in this work, there is a higher concentration of VCu for the cells close to the J- and L-type lines, and it is reduced for the cells around the A-type line. This is in line with the observations made above, where an increase of the intensity of the Raman band at 176 cm−1 is observed with the increasing [Zn]/[Ge] ratio (see Fig. 2). However, the concomitant increase of the [Cu]/([Zn] + [Ge]) ratio (see Fig. 1), even if much smaller than the increase of the [Zn]/[Ge] ratio, is also expected to have a critical influence on the concentration of VCu.
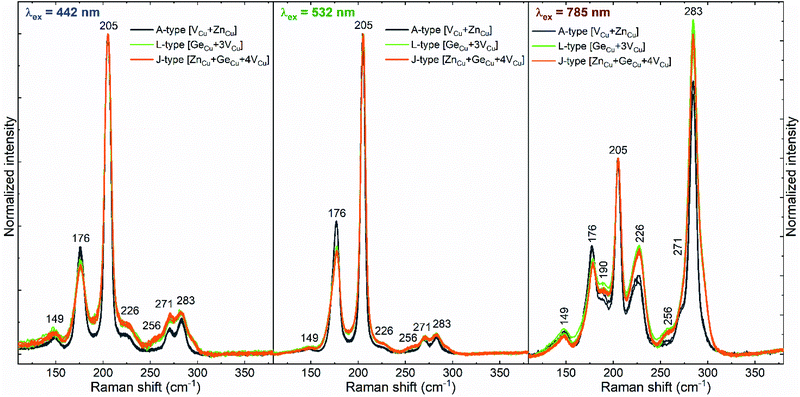 |
| Fig. 3 Examples of Raman spectra in the vicinity of different off-stoichiometric type lines measured under different excitation wavelength. | |
Taking a look at Fig. 3, a great similarity between the spectra corresponding to cells around the J- and L-type lines can be seen regardless the presence of the ZnCu point defect in some cells and its absence in others. This allows concluding that the ZnCu defect has a low influence on the Raman scattering spectra of the CZGSe compound. On the other hand, the GeCu substitutional defect presents a more significant influence on the Raman spectra, but mainly in the high wavenumber range (220–300 cm−1), where the relative intensity of the peaks increases with the higher Ge content (or lower [Zn]/[Ge] ratio). Nevertheless, it is hard to strictly distinguish the influence of the two substitutional point defects on the intensity of the peaks at the high frequency range and both of them will be considered in the analysis of the influence of the point defects on device performance presented in the next section.
Finally, it should be borne in mind that, for the analysis carried out above, only cells without ZnSe were used since the presence of this phase results in significant changes in the spectra measured under any excitation condition (e.g. see Fig. S2,† where spectra of the cells with similar compositions close to A-type off-stoichiometric line, but with and without ZnSe phase are presented). This indicates that Raman spectroscopy is a critical investigation tool to control the quality and phase purity of the kesterite type compounds.22
Influence of point defects on device performance
This section studies the influence of the point defects detected by Raman spectroscopy on solar cell performance. First, the analysis is focused on the dependence of the optoelectronic properties of the devices on the relative integrated intensity of the band at 176 cm−1 (calculated as A176/(A176 + A205) with A176 calculated in the 168–183 cm−1 range and A205 calculated in the 198–211 cm−1 range from the spectra measured under 532 nm excitation wavelength), which is inversely related to VCu concentration as previously reported.41,43Fig. 4a, shows a clear dependence of the efficiency of the solar cells with the concentration of VCu, with the highest efficiency achieved for a certain optimum concentration range of this defect, which corresponds to a relative integrated intensity of the Raman band at 176 cm−1 lying in the 0.325–0.350 range. Outside this optimum range, a deficit (higher band intensity) or excess (lower band intensity) of VCu defect concentration is expected, both having a negative influence on device performance. As such, three different regions (deficit, excess and optimum VCu) can be distinguished. A more detailed analysis of the evolution of the optoelectronic properties with defect concentration (Fig. 4 b–d) reveals that the main driving force behind the evolution of solar cell efficiency are the changes in the fill factor (FF) and short circuit current density (Jsc). Both parameters exhibit a similar tendency with defect concentration. Copper vacancies are a well-known beneficial point defect in kesterite and chalcopyrite based solar cells, which leads to the formation of a shallow acceptor level and has a strong influence on the electrical conductivity of the absorber layer.44,45 In this way, a deficit of this beneficial defect leads to a decrease of the charge carrier concentration. However, an excess results in the formation of a high amount of scattering centres which significantly decreases the mobility of the charge carriers. Both effects, have a direct influence on the electrical conductivity of the absorber layer and, in turn, on the FF and Jsc of the final devices as observed in Fig. 4. On the other hand, the open circuit voltage (Voc) shows only a slight dependence on VCu concentration, with only a sharp increase in the optimum defect range. These results differ from a previously published analysis of CZTSe samples, where the Voc was found to depend on the change of the relative intensity of the second most intense kesterite band (I2 around 170 cm−1).42 However, it should be noted that only cells around the A-type off-stoichiometric line were selected in the mentioned reference, which strongly reduces the number of possible parameters that can influence solar cell performance, at least from the point of view of point defects.
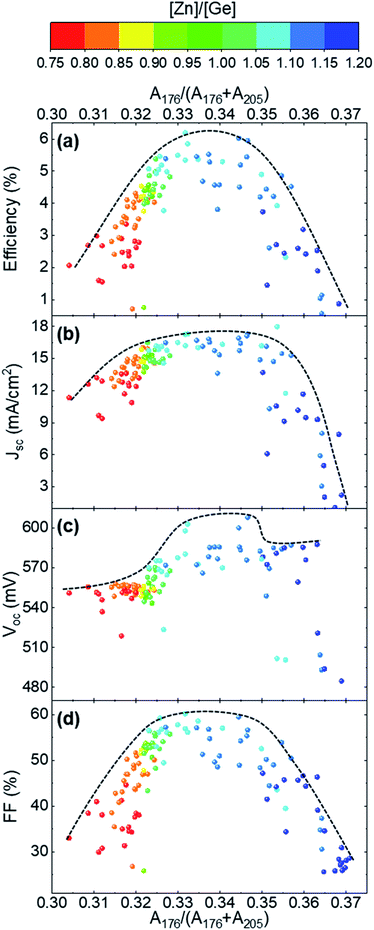 |
| Fig. 4 Dependence of optoelectronic properties (efficiency (a), short circuit current (b), open circuit voltage (c), fill factor (d)) of the cells from the relative integrated intensity of the Raman band at 176 cm−1. The colour scale corresponds to the [Zn]/[Ge] ratio. | |
Then, on a second stage, the influence of ZnCu and GeCu substitutional point defects on the optoelectronic properties of the devices was analysed. According to previous results, an increase of the relative intensity of the peaks in the high wavenumber range correlates with a higher concentration of substitutional defects.41–43Fig. 5a shows a parabolic dependence of solar cell efficiency with the variation of the relative integrated intensity of the Raman peaks in the range 235–300 cm−1. As in the case of VCu (see Fig. 4a), this shape is mainly governed by the changes in FF and Jsc (Fig. S3†) although in a less pronounced manner. This can be related to a slightly lower influence of the substitutional defects on these optoelectronic parameters. On the other hand, the analysis of the dependence of the Voc on the relative integrated intensity of the peaks in the 235–300 cm−1 range (Fig. 5b) shows a clearer influence of the substitutional defects on this parameter. Similarly to the analysis above, three regions can be distinguished: (1) low amount of defects (A235–300 < 0.190); (2) optimum amount of defects (0.190 < A235-300 < 0.215); (3) high amount of defect (A235-300 > 0.215). A relatively constant Voc value can be observed in region 1, followed by a sharp decrease in the second one, and a gentle decrease in region 3. Taking into account the [Zn]/[Ge] ratio, it can be seen that region 3 corresponds to Zn-poor conditions, for which GeCu substitutional defects are expected to prevail over the ZnCu defects. The former defect can form a deep donor defect (based on first principle calculations of the familiar Sn-containing kesterite compounds46) that increases the amount of non-radiative recombination, which finally decreases the Voc.47,48
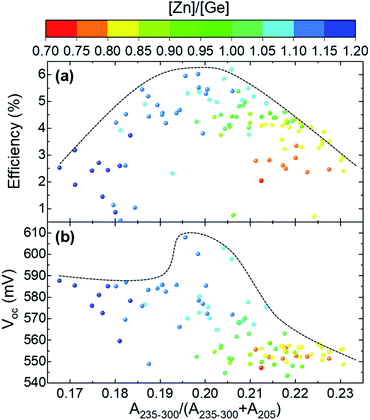 |
| Fig. 5 Dependence of (a) efficiency and (b) open circuit voltage of the cells on the relative integrated intensity of the Raman peaks in the range 235–300 cm−1. The colour scale corresponds to the [Zn]/[Ge] ratio. | |
A further analysis of the Raman spectra measured under different excitation wavelengths allows to establish additional failure mechanisms that lead to the decrease of the performance of the solar cells outside the optimum compositional range. A deep analysis of the spectra measured under blue and NIR excitations reveals that the lower efficiency in these ranges can be explained by two factors: the appearance of the ZnSe secondary phase and the change of the band gap of the absorber. The former effect can be seen in Fig. 6a where the relative intensity of the ZnSe peak is presented. The figure shows a clear decrease of solar cell performance with the increasing content of the ZnSe phase, proving the strong detrimental effect of this secondary phase. On the other hand, the band gap of CZGSe (around 1.5 eV) exhibits a resonant behaviour under NIR excitation conditions that leads to an increase of the intensity of the LO components of the E and B symmetry modes in kesterite type compounds.22,49,50 In the present study, this is observed by a strong enhancement of B symmetry peak at 283 cm−1 (see Fig. 2 and 3). However, the latter is not similar in all the cells, with some of them showing a rather low intensity of this peak (Fig. 6b). This can be related to the distancing of the CZGSe band gap from the excitation laser line. Previously, several works mentioned the effect of defects in the cations sublattice on the band gap of kesterite materials, but mainly the disorder of the Cu/Zn cations was discussed,51–53 while changes in the concentration of point defects was just briefly tackled.54,55 As mentioned above, in the combinatorial sample analysed in this work, it is not expected to have a significant difference in Cu/Zn disorder, while a clear change in the concentration of VCu, ZnCu and GeCu is observed. This implies that the concentration of point defects can lead to significant enough changes in the band gap of the CZGSe material that result in the observed decrease of the resonant effect in the Raman spectra.
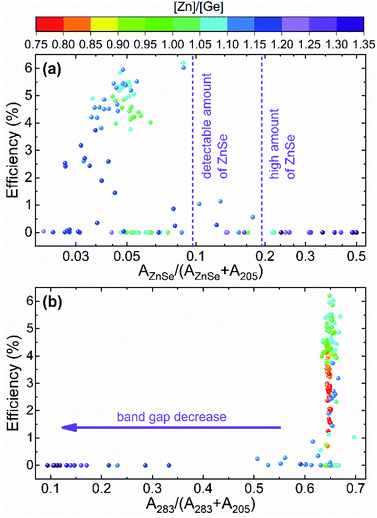 |
| Fig. 6 Dependence of solar cell efficiency with (a) relative integrated intensity of the ZnSe peak (spectra measured under 442 nm excitation), (b) relative integrated intensity of the peak at 283 cm−1 (spectra measured under 785 nm excitation). The colour scale corresponds to the relative integrated intensity of the band at 176 cm−1 calculated from the spectra measured under 532 nm excitation. | |
Finally, the complex analysis presented above reveals that the optimum compositional cationic range that allows achieving high efficiency devices is [Zn]/[Ge] = 1.05–1.15. This is in agreement with previously published values for high efficiency solar cells based on CZGSe.16,17,29 Furthermore, the efficiency of the devices seems to be less dependent on the [Cu]/([Zn] + [Sn]) ratio, as long as the Cu-poor condition is respected (e.g. similar efficiencies were obtained for [Cu]/([Zn] + [Sn]) = 0.67 in ref. 29 and 0.78 in ref. 17). Deviations from this optimum cationic ratio range towards stoichiometric and Zn-poor compositions result in an increase of the amount of both VCu and substitutional defects. This can be assumed to be the reason for the slight increase of the FWHM of the Raman peaks in the spectra measured in the cells with [Zn]/[Ge] ≤ 1.0, as discussed above (see Section 3.1 and Fig. 2). On the contrary, an increase of the Zn concentration in the system with [Zn]/[Ge] > 1.15, results in the decrease of both VCu and substitutional defects, in bandgap narrowing, and in an increase of the probability of the detrimental ZnSe secondary phase being formed. Note that the ZnSe phase was found to form also in cells within the optimum cationic range strongly influencing device performance and, as such, the formation of this phase should be controlled during the production process.
Machine learning approach for device performance analysis
The results presented in the previous section clearly indicate that there is a complex dependence between solar cell performance and the different parameters obtained from Raman spectroscopy, such as VCu/ZnCu/GeCu defect concentration or the presence of secondary phases. In this regard, the application of machine learning to the analysis of the Raman spectroscopic data can not only significantly reduce the analysis time, but also yield methodologies for solar cell efficiency prediction. For the present study, a LDA dimension-reduction algorithm was applied. This type of algorithm is widely used for spectral data analysis in different methods and fields of application.56–61 This is because of the high dimensionality nature of the spectroscopic data analysed in this work and the ability of LDA to reduce these dimensions to just a few while preserving most of the information, which allows for feature extraction rather than feature selection. Using the Raman spectra of each cell obtained with different wavelengths as input, the LDA algorithm was used to classify the different cells of the combinatorial sample according to the following classification targets: efficiency, Voc and [Zn/Ge] ratio. The 2-dimensional outputs for each classification targets are presented in Fig. 7 as a function of two discriminants (D1 and D2, with labels a, b, c for the three mentioned targets, respectively), along with the classification groups and training/test scores. As already mentioned above, the amount of data employed for the analysis (196 cells) is far from being considered “big data” and, as such, this methodology and the results presented below should be taken as a first approach and are susceptible to further improvement for a more precise classification through the use of a higher number of training inputs. In the case of the efficiency and Voc targets, a defined data classification clustering is observed with comparable scores. In Fig. 7a, it can be observed that low efficiency (<3.4%) cells are relatively well classified and separated from the groups with medium (3.4–4.4%) and high (>4.4%) efficiency. On the contrary, for the Voc target, while the cells with the highest voltages are well differentiated, the rest of groups show high overlapping (Fig. 7b). However, despite the relatively low classification score, it is worth noticing that there is no clear overfitting occurring by comparing the individual and overall scores of the algorithm, indicating that a larger data set could greatly improve the classification.
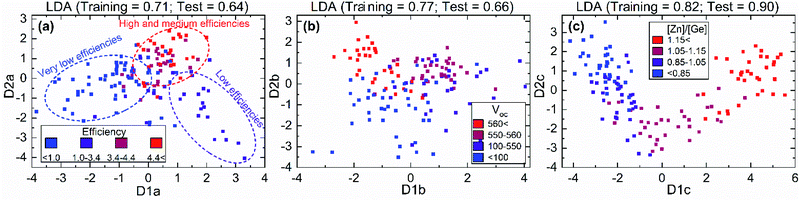 |
| Fig. 7 Results of the machine learning analysis of the Raman spectroscopic data for different classification targets: (a) efficiency, (b) Voc and (c) [Zn]/[Ge] ratio. The training and test scores obtained for each run are presented on the top of the panels. | |
In the case of the [Zn]/[Ge] ratio target shown in Fig. 7c, the classification resulted in a clear sequential correlation between the discriminants, from lower to higher ratios. The first 2 groups, cells with 0–0.85 and 0.85–1.05 [Zn]/[Ge] ratios, show significant overlapping leading to misclassifications in both training and test data which is reflected in the LDA algorithm scores. On the other hand, a good clustering is shown for the 1.05 < [Zn]/[Ge] < 1.15 and [Zn]/[Ge] > 1.15 groups. Even though the discriminants in LDA algorithms are of unclear nature and do not necessarily follow an underlying physical concept (at least in a straightforward way), the resulting curve in Fig. 7c shows great resemblance with that obtained from the analytical analysis of the influence of point defects on device performance (Fig. 4a). Bearing this in mind, the relative integrated intensity of the Raman peak at 176 cm−1 and efficiency of the solar cells in the combinatorial sample were plotted against the classification discriminants D1c and D2c (Fig. 8). The latter were mainly selected as they show the clearest differentiation between the different classification groups, and, thus, were expected to have a more pronounced dependence from the physical parameters of the solar cell devices. Despite the fact that none of the analytical parameters (relative integrated intensity of the Raman peak and solar cell efficiency) was directly used for the LDA algorithm, a pronounced linear-like correlation between parameters and the LDA discriminants can be observed. Moreover, a closer look to the obtained correlation, allows to define that the points that deviate from the correlation forming a cloud in Fig. 8a (highlighted with a dotted oval) correlate with zero efficiency solar cells. In this way, the behaviour of these cells is probably not related to the concentration of VCu defects, but to other critical parameters of the devices (i.e. presence of ZnSe in absorber, or bad absorber/buffer interface, etc.). Similarly, in case of the correlation of cell efficiency with the D2c parameter (Fig. 8b), the points that lie far from the proposed dashed line probably present some additional issues, not directly related to the absorber layer itself that is strictly analysed in the present study. These correlations, however, firstly, allow to get a glimpse of the possible physical meaning behind the D1c and D2c discriminants, and, secondly, prove that there exists a strong correlation of the VCu defects with the Raman spectra [Zn]/[Ge] ratio and solar cell efficiency. In this way, the variations of the VCu parameter are directly reflected on the other parameters. These multi-variable correlations are intrinsic to the CZGSe material itself and of importance to derive its properties. Moreover, this finding makes possible to predict solar cell efficiency using only Raman spectra and compositional data. This has an enormous potential both for research and industrial process monitoring applications. In addition, the results presented here illustrate a powerful example of why combinatorial analysis should be established as a standard procedure for the study of complex systems such as thin film solar cells based on chalcogenide compounds in order deepen into the critical parameters that govern their efficiency.
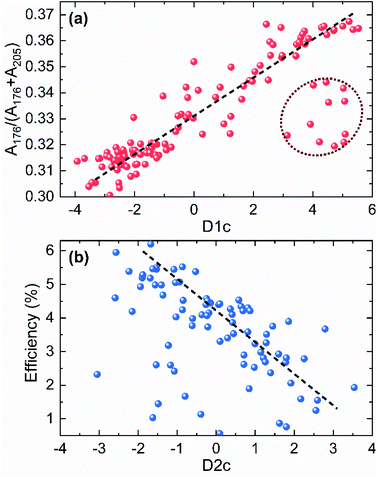 |
| Fig. 8 (a) Relative integrated intensity of the Raman peak at 176 cm−1 plotted against discriminant D1c, and (b) efficiency of the solar cells plotted against discriminant D2c of the [Zn]/[Ge] ratio LDA classification target. The explanation of the dotted oval line can be found in the main text. | |
Conclusions
This work has presented a complex analysis of the formation of secondary phases and point defects under off-stoichiometry conditions, as well as of their effect on PV performance, in a combinatorial CZGSe sample comprised by almost 200 individual solar cells covering a wide range of [Zn]/[Ge] compositions in the Cu-poor regime. Firstly, an analytical approach based on Raman spectroscopy and XRF has allowed defining the off-stoichiometric limits of formation of the CZGSe kesterite phase: 0.7 < [Zn]/[Ge] < 1.2. It has been observed that, close to the top limit, the probability of forming ZnSe increases and, above it, it may become the dominant phase, while the formation of other secondary phases is almost negligible in the whole range studied. As for defect formation, the footprint of VCu and substitutional ZnCu and GeCu defects on the vibrational properties of the CZGSe material has been analysed. Strong VCu-induced variations in the Raman spectra have been found, especially close to the J- and L-type off-stoichiometry lines, whereas a softer effect (mainly at high wavenumbers) has been observed for the substitutional defects. Finally, the complex analytical correlation of compositional, spectroscopic and optoelectronic data for each of the 200 solar cells, has allowed revealing that VCu controls the Jsc and FF of the devices while the substitutional defects have their main influence on the Voc leading to an optimum cationic compositional range of [Zn]/[Ge] = 1.05–1.15 for achieving the high efficiency (∼6%) solar cell devices. This has been further explored through the application of an LDA machine learning algorithm. Using just Raman spectra as input, the algorithm employed has been shown to be able to classify the different cells in terms of composition and optoelectronic parameters. These results confirm the deep intrinsic intertwining of the VCu defect concentration with the Raman spectra, [Zn]/[Ge] ratio and solar cell efficiency, and represent a powerful solar cell performance prediction methodology both for research and industrial process monitoring environments. As such, this work not only provides valuable insight for understanding and further developing the CZGSe photovoltaic technology and but also gives a practical example of the potential of combinatorial analysis and machine learning for the study of complex systems in materials research.
Authors contributions
Enric Grau-Luque: formal analysis, software, visualization, writing – original draft; Ikram Anefnaf: formal analysis, resources, writing – review & editing; Nada Benhaddou: formal analysis, resources; Robert Fonoll-Rubio: investigation; Ignacio Becerril-Romero: validation, writing – review & editing; Safae Aazou: funding acquisition, project administration, writing – review & editing; Edgardo Saucedo: conceptualization, funding acquisition, project administration; Zouheir Sekkat: funding acquisition, supervision; Alejandro Perez-Rodriguez: funding acquisition, supervision; Victor Izquierdo-Roca: conceptualization, methodology, validation, writing – review & editing; Maxim Guc: data curation, formal analysis, investigation, methodology, validation, visualization, writing – original draft.
Conflicts of interest
There are no conflicts to declare.
Acknowledgements
This work has received funding from the European Union's Horizon 2020 research and innovation programme under grant agreements no 777968 (INFINITE-CELL project) and 952982 (Custom-Art project), and was partially supported by the Spanish Ministry of Science, Innovation and Universities under the WINCOST (ENE2016-80788-C5-1-R) project. Authors from IREC belong to the SEMS (Solar Energy Materials and Systems) Consolidated Research Group of the “Generalitat de Catalunya” (ref. 2017 SGR 862) and are grateful to European Regional Development Funds (ERDF, FEDER Programa Competitivitat de Catalunya 2007–2013). MG acknowledges the financial support from Spanish Ministry of Science, Innovation and Universities within the Juan de la Cierva fellowship (IJC2018-038199-I).
References
- C. Candelise, M. Winskel and R. Gross, Prog. Photovoltaics Res. Appl., 2012, 20, 816–831 CrossRef.
- W. Wang, M. T. Winkler, O. Gunawan, T. Gokmen, T. K. Todorov, Y. Zhu and D. B. Mitzi, Adv. Energy Mater., 2014, 4, 1301465 CrossRef.
- J. J. Scragg, T. Ericson, T. Kubart, M. Edoff and C. Platzer-Björkman, Chem. Mater., 2011, 23, 4625–4633 CrossRef CAS.
- R. Fonoll-Rubio, J. Andrade-Arvizu, J. Blanco-Portals, I. Becerril-Romero, M. Guc, E. Saucedo, F. Peiró, L. Calvo-Barrio, M. Ritzer, C. S. Schnohr, M. Placidi, S. Estradé, V. Izquierdo-Roca and A. Pérez-Rodríguez, Energy Environ. Sci., 2021, 14, 507–523 RSC.
- C. J. Hages, S. Levcenco, C. K. Miskin, J. H. Alsmeier, D. Abou-Ras, R. G. Wilks, M. Bär, T. Unold and R. Agrawal, Prog. Photovoltaics Res. Appl., 2015, 23, 376–384 CrossRef CAS.
- S. Giraldo, M. Neuschitzer, T. Thersleff, S. López-Marino, Y. Sánchez, H. Xie, M. Colina, M. Placidi, P. Pistor, V. Izquierdo-Roca, K. Leifer, A. Pérez-Rodríguez and E. Saucedo, Adv. Energy Mater., 2015, 5, 1501070 CrossRef.
- S. Giraldo, E. Saucedo, M. Neuschitzer, F. Oliva, M. Placidi, X. Alcobé, V. Izquierdo-Roca, S. Kim, H. Tampo, H. Shibata, A. Pérez-Rodríguez and P. Pistor, Energy Environ. Sci., 2018, 11, 582–593 RSC.
- S. Kim, K. M. Kim, H. Tampo, H. Shibata, K. Matsubara and S. Niki, Sol. Energy Mater. Sol. Cells, 2016, 144, 488–492 CrossRef CAS.
- A. D. Collord and H. W. Hillhouse, Chem. Mater., 2016, 28, 2067–2073 CrossRef CAS.
- J. Andrade-Arvizu, V. Izquierdo-Roca, I. Becerril-Romero, P. Vidal-Fuentes, R. Fonoll-Rubio, Y. Sánchez, M. Placidi, L. Calvo-Barrio, O. Vigil-Galán and E. Saucedo, ACS Appl. Mater. Interfaces, 2019, 11, 32945–32956 CrossRef CAS PubMed.
- J. Márquez, H. Stange, C. J. Hages, N. Schaefer, S. Levcenko, S. Giraldo, E. Saucedo, K. Schwarzburg, D. Abou-Ras, A. Redinger, M. Klaus, C. Genzel, T. Unold and R. Mainz, Chem. Mater., 2017, 29, 9399–9406 CrossRef.
- S. Levcenko, R. Caballero, L. Dermenji, E. V. Telesh, I. A. Victorov, J. M. Merino, E. Arushanov, M. Leon and I. V. Bodnar, Opt. Mater., 2015, 40, 76–80 CrossRef CAS.
- M. León, S. Levcenko, R. Serna, G. Gurieva, A. Nateprov, J. M. Merino, E. J. Friedrich, U. Fillat, S. Schorr and E. Arushanov, J. Appl. Phys., 2010, 108, 093502 CrossRef.
- L. Choubrac, M. Bär, X. Kozina, R. Félix, R. G. Wilks, G. Brammertz, S. Levcenko, L. Arzel, N. Barreau, S. Harel, M. Meuris and B. Vermang, ACS Appl. Energy Mater., 2020, 3, 5830–5839 CrossRef CAS.
- L. Choubrac, G. Brammertz, N. Barreau, L. Arzel, S. Harel, M. Meuris and B. Vermang, Phys. Status Solidi, 2018, 215, 1800043 CrossRef.
- N. Benhaddou, S. Aazou, R. Fonoll-Rubio, Y. Sánchez, S. Giraldo, M. Guc, L. Calvo-Barrio, V. Izquierdo-Roca, M. Abd-Lefdil, Z. Sekkat and E. Saucedo, J. Mater. Chem. C, 2020, 8, 4003–4011 RSC.
- S. Sahayaraj, G. Brammertz, B. Vermang, T. Schnabel, E. Ahlswede, Z. Huang, S. Ranjbar, M. Meuris, J. Vleugels and J. Poortmans, Sol. Energy Mater. Sol. Cells, 2017, 171, 136–141 CrossRef CAS.
- R. Gunder, J. A. Márquez-Prieto, G. Gurieva, T. Unold and S. Schorr, CrystEngComm, 2018, 20, 1491–1498 RSC.
- M. Neuschitzer, Y. Sanchez, S. López-Marino, H. Xie, A. Fairbrother, M. Placidi, S. Haass, V. Izquierdo-Roca, A. Perez-Rodriguez and E. Saucedo, Prog. Photovoltaics Res. Appl., 2015, 23, 1660–1667 CrossRef CAS.
-
G. Van Rossum and F. L. Drake, Python 3 Reference Manual, CreateSpace, Scotts Valley, CA, 2009 Search PubMed.
- G. Varoquaux, L. Buitinck, G. Louppe, O. Grisel, F. Pedregosa and A. Mueller, GetMobile Mob. Comput. Commun., 2015, 19, 29–33 CrossRef.
- S. Schorr, G. Gurieva, M. Guc, M. Dimitrievska, A. Pérez-Rodríguez, V. Izquierdo-Roca, C. S. Schnohr, J. Kim, W. Jo and J. M. Merino, J. Phys. Energy, 2020, 2, 012002 CrossRef CAS.
- A. Lafond, L. Choubrac, C. Guillot-Deudon, P. Deniard and S. Jobic, Z. Anorg. Allg. Chem., 2012, 638, 2571–2577 CrossRef CAS.
- M. Dimitrievska, H. Xie, A. J. Jackson, X. Fontané, M. Espíndola-Rodríguez, E. Saucedo, A. Pérez-Rodríguez, A. Walsh and V. Izquierdo-Roca, Phys. Chem. Chem. Phys., 2016, 18, 7632–7640 RSC.
- M. Y. Valakh, O. F. Kolomys, S. S. Ponomaryov, V. O. Yukhymchuk, I. S. Babichuk, V. Izquierdo-Roca, E. Saucedo, A. Perez-Rodriguez, J. R. Morante, S. Schorr and I. V. Bodnar, Phys. Status Solidi RRL, 2013, 7, 258–261 CrossRef CAS.
- M. Dimitrievska, A. Fairbrother, A. Pérez-Rodríguez, E. Saucedo and V. Izquierdo-Roca, Acta Mater., 2014, 70, 272–280 CrossRef CAS.
- A. Ritscher, M. Hoelzel and M. Lerch, J. Solid State Chem., 2016, 238, 68–73 CrossRef CAS.
- D. M. Többens, G. Gurieva, S. Levcenko, T. Unold and S. Schorr, Phys. Status Solidi, 2016, 253, 1890–1897 CrossRef.
- N. Benhaddou, S. Aazou, Y. Sánchez, J. Andrade-Arvizu, I. Becerril-Romero, M. Guc, S. Giraldo, V. Izquierdo-Roca, E. Saucedo and Z. Sekkat, Sol. Energy Mater. Sol. Cells, 2020, 216, 110701 CrossRef CAS.
- A. Fairbrother, X. Fontané, V. Izquierdo-Roca, M. Placidi, D. Sylla, M. Espindola-Rodriguez, S. López-Mariño, F. A. Pulgarín, O. Vigil-Galán, A. Pérez-Rodríguez and E. Saucedo, Prog. Photovoltaics Res. Appl., 2014, 22, 479–487 CrossRef CAS.
- Y. Yang, S. C. Liu, W. Yang, Z. Li, Y. Wang, X. Wang, S. Zhang, Y. Zhang, M. Long, G. Zhang, D. J. Xue, J. S. Hu and L. J. Wan, J. Am. Chem. Soc., 2018, 140, 4150–4156 CrossRef CAS PubMed.
- H. R. Chandrasekhar and U. Zwick, Solid State Commun., 1976, 18, 1509–1513 CrossRef CAS.
- E. Sleeckx, L. Tichý, P. Nagels and R. Callaerts, J. Non. Cryst. Solids, 1996, 198–200, 723–727 CrossRef CAS.
- M. Fujii, S. Hayashi and K. Yamamoto, Jpn. J. Appl. Phys., 1991, 30, 687–694 CrossRef CAS.
- B. K. Sarkar, A. S. Verma and P. S. Deviprasad, Phys. B, 2011, 406, 2847–2850 CrossRef CAS.
- G. Marcano, C. Rincón, G. Marín, G. E. Delgado, A. J. Mora, J. L. Herrera-Pérez, J. G. Mendoza-Alvarez and P. Rodríguez, Solid State Commun., 2008, 146, 65–68 CrossRef CAS.
- S. G. Choi, A. L. Donohue, G. Marcano, C. Rincón, L. M. Gedvilas, J. Li and G. E. Delgado, J. Appl. Phys., 2013, 114, 033531 CrossRef.
- L. E. Valle Rios, K. Neldner, G. Gurieva and S. Schorr, J. Alloys Compd., 2016, 657, 408–413 CrossRef CAS.
- I. Becerril-Romero, L. Acebo, F. Oliva, V. Izquierdo-Roca, S. López-Marino, M. Espíndola-Rodríguez, M. Neuschitzer, Y. Sánchez, M. Placidi, A. Pérez-Rodríguez, E. Saucedo and P. Pistor, Prog. Photovoltaics Res. Appl., 2018, 26, 55–68 CrossRef CAS.
- G. Gurieva, L. E. Valle Rios, A. Franz, P. Whitfield and S. Schorr, J. Appl. Phys., 2018, 123, 161519 CrossRef.
- M. Dimitrievska, A. Fairbrother, E. Saucedo, A. Pérez-Rodríguez and V. Izquierdo-Roca, Appl. Phys. Lett., 2015, 106, 073903 CrossRef.
- M. Dimitrievska, A. Fairbrother, E. Saucedo, A. Pérez-Rodríguez and V. Izquierdo-Roca, Sol. Energy Mater. Sol. Cells, 2016, 149, 304–309 CrossRef CAS.
- M. Dimitrievska, F. Oliva, M. Guc, S. Giraldo, E. Saucedo, A. Pérez-Rodríguez and V. Izquierdo-Roca, J. Mater. Chem. A, 2019, 7, 13293–13304 RSC.
-
I. Repins, N. Vora, C. Beall, S. H. Wei, F. Yan, M. Romero, G. Teeter, H. Du, B. To, M. Young and R. Noufi, in Materials Research Society Symposium Proceedings, Cambridge University Press, 2012, vol. 1324, pp. 97–108 Search PubMed.
- M. Grossberg, J. Krustok, C. J. Hages, D. M. Bishop, O. Gunawan, R. Scheer, S. M. Lyam, H. Hempel, S. Levcenco and T. Unold, J. Phys. Energy, 2019, 1, 044002 CrossRef CAS.
- S. Chen, A. Walsh, X.-G. Gong and S.-H. Wei, Adv. Mater., 2013, 25, 1522–1539 CrossRef CAS PubMed.
- M. M. Islam, M. A. Halim, T. Sakurai, N. Sakai, T. Kato, H. Sugimoto, H. Tampo, H. Shibata, S. Niki and K. Akimoto, Appl. Phys. Lett., 2015, 106, 243905 CrossRef.
- S. Levcenko, J. Just, A. Redinger, G. Larramona, S. Bourdais, G. Dennler, A. Jacob and T. Unold, Phys. Rev. Appl., 2016, 5, 024004 CrossRef.
- M. Guc, S. Levcenko, I. V. Bodnar, V. Izquierdo-Roca, X. Fontane, L. V. Volkova, E. Arushanov and A. Pérez-Rodríguez, Sci. Rep., 2016, 6, 19414 CrossRef CAS PubMed.
- M. Guc, A. P. Litvinchuk, S. Levcenko, M. Y. Valakh, I. V. Bodnar, V. M. Dzhagan, V. Izquierdo-Roca, E. Arushanov and A. Pérez-Rodríguez, RSC Adv., 2016, 6, 13278–13285 RSC.
- G. Rey, A. Redinger, J. Sendler, T. P. Weiss, M. Thevenin, M. Guennou, B. El Adib and S. Siebentritt, Appl. Phys. Lett., 2014, 105, 112106 CrossRef.
- J. J. S. Scragg, J. K. Larsen, M. Kumar, C. Persson, J. Sendler, S. Siebentritt and C. Platzer Björkman, Phys. Status Solidi, 2016, 253, 247–254 CrossRef CAS.
- M. Valentini, C. Malerba, F. Menchini, D. Tedeschi, A. Polimeni, M. Capizzi and A. Mittiga, Appl. Phys. Lett., 2016, 108, 211909 CrossRef.
- D. P. Halliday, R. Claridge, M. C. J. Goodman, B. G. Mendis, K. Durose and J. D. Major, J. Appl. Phys., 2013, 113, 223503 CrossRef.
- T. Gershon, B. Shin, T. Gokmen, S. Lu, N. Bojarczuk and S. Guha, Appl. Phys. Lett., 2013, 103, 193903 CrossRef.
- N. M. Ralbovsky and I. K. Lednev, Spectrochim. Acta, Part A, 2019, 219, 463–487 CrossRef CAS PubMed.
- R. Chauhan, R. Kumar, V. Kumar, K. Sharma and V. Sharma, Forensic Sci. Int., 2021, 319, 110655 CrossRef CAS PubMed.
- Z. Guleken, B. Ünübol, R. Bilici, D. Sarıbal, S. Toraman, O. Gündüz and S. Erdem Kuruca, J. Pharm. Biomed. Anal., 2020, 190, 113553 CrossRef CAS PubMed.
- R. Chophi, S. Sharma and R. Singh, Forensic Chem., 2020, 17, 100209 CrossRef.
- T. Visnevschi-Necrasov, J. C. M. Barreira, S. C. Cunha, G. Pereira, E. Nunes and M. B. P. P. Oliveira, Food Res. Int., 2015, 76, 51–57 CrossRef CAS.
- Y. Wang, J. Zhu and X. Chen, Optik, 2020, 224, 165446 CrossRef CAS.
Footnote |
† Electronic supplementary information (ESI) available. See DOI: 10.1039/d1ta01299a |
|
This journal is © The Royal Society of Chemistry 2021 |
Click here to see how this site uses Cookies. View our privacy policy here.