DOI:
10.1039/D1SC04271H
(Edge Article)
Chem. Sci., 2021,
12, 14484-14493
Elucidating the structure-dependent selectivity of CuZn towards methane and ethanol in CO2 electroreduction using tailored Cu/ZnO precatalysts†
Received
4th August 2021
, Accepted 6th October 2021
First published on 8th October 2021
Abstract
Understanding the catalyst compositional and structural features that control selectivity is of uttermost importance to target desired products in chemical reactions. In this joint experimental–computational work, we leverage tailored Cu/ZnO precatalysts as a material platform to identify the intrinsic features of methane-producing and ethanol-producing CuZn catalysts in the electrochemical CO2 reduction reaction (CO2RR). Specifically, we find that Cu@ZnO nanocrystals, where a central Cu domain is decorated with ZnO domains, and ZnO@Cu nanocrystals, where a central ZnO domain is decorated with Cu domains, evolve into Cu@CuZn core@shell catalysts that are selective for methane (∼52%) and ethanol (∼39%), respectively. Operando X-ray absorption spectroscopy and various microscopy methods evidence that a higher degree of surface alloying along with a higher concentration of metallic Zn improve the ethanol selectivity. Density functional theory explains that the combination of electronic and tandem effects accounts for such selectivity. These findings mark a step ahead towards understanding structure–property relationships in bimetallic catalysts for the CO2RR and their rational tuning to increase selectivity towards target products, especially alcohols.
Introduction
The electrochemical CO2 reduction reaction (CO2RR) has the potential to mitigate rising CO2 levels while storing renewable energy in chemical bonds.1 Among the different products, alcohols are highly desirable as they possess high energy density and ease of storage.1 However, the progress toward obtaining them with high selectivity remains limited compared to that made in the production of gaseous hydrocarbon products.2
Copper is the only single metal capable of producing products beyond CO, including alcohols.1,2 While this catalyst holds record efficiencies towards methane and ethylene, its lower selectivity toward ethanol is attributed to the higher energy barrier of ethanol formation with respect to ethylene, whose pathway shares common intermediates with the former.1,2
Tandem schemes and modification of the electronic properties of copper achieved by coupling it with a second element are promising strategies to improve its selectivity towards alcohols, particularly ethanol.2–10 Most of the studies so far have focused on the composition dependence of the catalytic behaviour.2–10 However, the sensitivity of the catalytic performance to the atomic distribution within bimetallic catalysts remains still underexplored.2–10
Among other systems, CuZn stands out because of two reasons. On one hand, this catalyst consists of two Earth abundant metals, which makes it particularly appealing. On the other hand, the conclusions of previous studies on its catalytic behaviour are controversial.9–15 While high selectivity for ethanol was found in certain cases, preferential methane production was also reported, as well as selectivity towards CO.9–15 Composition certainly plays an important role in directing selectivity, with a higher Zn content generally favouring CO;13,16 yet other factors must come into play to justify the observed selectivity patterns towards methane or ethanol in similar compositional ranges.9–15 For example, CuZn nanostructured catalysts were shown to facilitate the formation of ethanol at a Zn content between 10% and 30%.9–12 Instead, CuZn nanoparticles with a similar Zn content were reported to favour selectivity toward methane.15 Unfortunately, direct comparisons between these two systems and, similarly, in other examples, are impeded by the striking structural differences and size regime of the catalysts, one being nanostructured thin films with agglomerated particles of sizes 100 nm to 1 μm, and the other consisting of 5 nm nanoparticles. Nevertheless, understanding the catalyst features which are optimal to direct the reaction pathway towards target products is of uttermost importance to move towards rational catalyst design, which is particularly urgent in the CO2RR.
In this work, we employ tailored Cu/ZnO precatalysts to link the composition and structure of CuZn catalysts to the promotion of ethanol versus methane. State-of-the-art material synthesis is coupled with operando X-ray absorption spectroscopy, various microscopy methods and theoretical calculations to find that the metal distribution plays a crucial role, together with the composition, in directing the selectivity of CuZn catalysts.
Results and discussion
Synthesis and characterization of the Cu/ZnO precatalysts
Cu/ZnO nanocrystals (NCs) were employed as precatalysts. They were synthesized via a previously developed seed-mediated approach to obtain tunable spatial configuration of Cu and ZnO domains within a single nano-object.25,26 Two different sizes of ZnO NCs were used as seeds, namely 13 nm and 60 nm (Fig. S1†). The Cu
:
Zn atomic ratios were varied by adjusting the concentration of seeds and the amount of Cu precursor (Fig. S2†).
Fig. 1 displays the electron microscopy characterization of two representative Cu/ZnO NCs obtained with the smaller (Fig. 1a and b) and with the bigger (Fig. 1c and d) ZnO seeds, both with a Zn atomic content equal to 54%. The former are composed of a central 25 nm Cu domain decorated by ZnO domains, similar to previous results.25 In the latter, each 60 nm ZnO seed is decorated by 25 nm Cu NCs. We will refer to these two types of Cu/ZnO NCs as Cu@ZnO and ZnO@Cu, respectively. The impact of the seed size on the final configuration of the Cu/ZnO NCs can be tentatively explained with strain accommodation arguments related to the high lattice mismatch between Cu and ZnO (Fig. S3†). Based on these arguments, we speculate that a higher misfit strain energy accumulates at the interfaces between the two domains in the ZnO@Cu NCs compared to the Cu@ZnO NCs. Additional characterization, including X-ray photoelectron spectroscopy (XPS) and X-ray diffraction (XRD), was performed to confirm the composition of the NCs and to rule out the possibility of a CuZn alloy in the as-synthesized NCs (Fig. S4†).
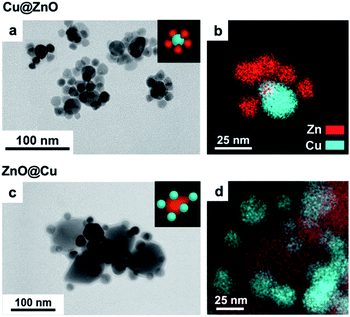 |
| Fig. 1 (a and c) Representative low-resolution TEM and (b and d) STEM-EDXS elemental maps of the Cu@ZnO and ZnO@Cu precatalysts, synthesized by using ZnO NCs with a diameter of 13 nm and 60 nm as seeds, respectively. | |
Characterization of the activated catalysts
In agreement with previous studies, we found that ZnO reduces to metallic Zn under CO2RR conditions (Fig. S5†) and, concomitantly, the catalytic performance changes over time.10,15 In order to decouple, or at least to minimize, the drastic effects of the structural changes on the catalytic behaviour, we opted for the introduction of a catalyst activation step, which involves the application of a cathodic potential of −1.1 V vs. RHE to the Cu/ZnO precatalysts. This potential was chosen to be close to the range in which the CO2RR normally occurs for Cu-based nano catalysts.6,26,27
The activated catalysts were characterized by scanning transmission electron microscopy-energy dispersive X-ray spectroscopy (STEM-EDXS), X-ray photoelectron spectroscopy (XPS) and operando X-ray absorption near edge structure (XANES) measurements (Fig. 2). Fig. 2a and b report the STEM-EDXS elemental maps of the activated Cu@ZnO and ZnO@Cu NCs. Both systems evolve towards a core@shell structure, where a central Cu domain is surrounded by a few nanometer-thick Zn shell. The size of the Cu core remains the same as the pre-catalysts, which is around 25 nm, and regulates the size of the activated catalysts. The Zn shell appears slightly more uniform in the case of ZnO@Cu NCs (Fig. 2b). The XPS data were consistent with an increase of the Zn
:
Cu ratio on the surface of the activated catalysts, which was more evident for ZnO@Cu, in line with the higher uniformity of the shell (Fig. S6 and Table S1†). Some variation in the elemental distribution is noticeable in STEM-EDX; however no pure Cu or Zn particles were detected on the electrodes.
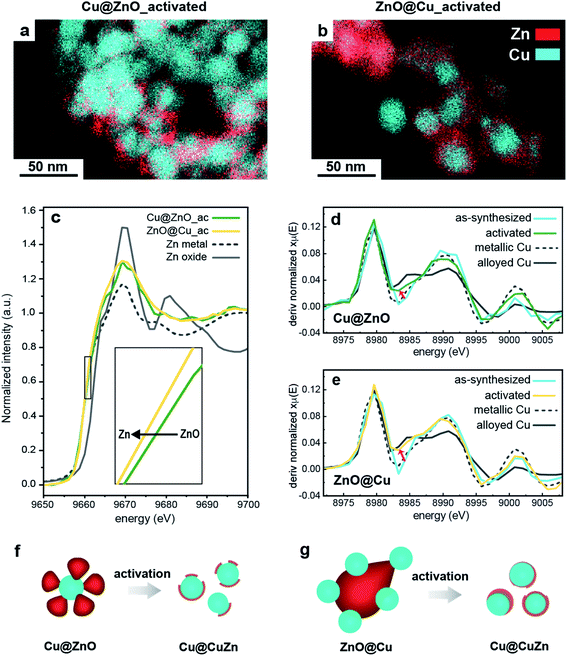 |
| Fig. 2 (a and b) EDXS elemental maps of Cu@ZnO and ZnO@Cu after activation at −1.1 VRHE for 1 hour. (c) Zn K-edge XANES spectra of ZnO@Cu and Cu@ZnO NCs after activation together with the spectra of reference Zn and ZnO particles. (d and e) First-order derivative of the Cu K-edge XANES spectra of the as-synthesized and activated samples. (f and g) Schematic of the morphological evolution of both NCs during activation. | |
While it provides unique insight into the morphological and compositional evolution, electron microscopy is a local technique. Information on the composition at the ensemble level, such as that provided by XANES measurements, is needed for the interpretation of the catalytic properties. Fig. 2c compares the Zn K-edge of the XANES spectra for Cu@ZnO and ZnO@Cu before and after activation. The data for the as-synthesized samples evidence that Zn is initially fully oxidized while Cu is mostly in its metallic form. Upon activation, the ZnO component evolves towards its reduced form for both precatalysts (Fig. 2c). Furthermore, their final spectra suggest the presence of cationic Zn in the activated Cu@ZnO but not in the activated ZnO@Cu NCs. No strikingly evident changes were observed for the Cu K-edge spectra upon activation.
In order to investigate the possibility of alloying between Cu and Zn, the first-derivative XANES spectra of the samples before and after activation were plotted along with Cu and Zn reference materials in metallic, alloyed and oxide forms (Fig. 2d, e and S7†). The data of the Cu K-edge in both activated catalysts are consistent with the partial alloying of Cu with Zn (Fig. 2d and e). This is particularly evident in ZnO@Cu (Fig. 2e), where the spectrum of the as-synthesized sample is similar to that of metallic Cu (note the spectra around 8982–8985 eV), while the final spectrum after activation clearly overlaps with that of the alloyed CuZn reference (the trend of changes is shown by a black arrow). As for the Zn K-edge, the changes in the line profiles of all the samples are consistent with metallic Zn and some degree of alloyed Zn (Fig. S7†).
Finally, elemental analysis indicated that a small fraction of Zn leaches into the electrolyte for both precatalysts, which suggests that some dissolution/reprecipitation processes might occur during the activation. The extent of Zn leaching during activation is similar in the two NCs. Indeed, the pre-catalysts with initial 54% Zn enter the course of the CO2RR with a Zn atomic percentage of ∼48%.
To summarize, we found that the activation of Cu@ZnO and ZnO@Cu yields Cu@CuZn core@shell structures (Fig. 2f and g). The Cu@ZnO-derived catalysts possess a less uniform shell and some residual cationic Zn species. The ZnO@Cu-derived catalysts exhibit a more uniform shell and the Zn domain is in a completely metallic state. The more drastic reconstruction of the ZnO@Cu NCs, which undergo a complete inversion of the core and the shell, can be speculatively attributed to their highly strained interfaces. Interestingly, a physical mixture of Cu and ZnO NCs does not evolve into the same type of structure (Fig. S6, S8 and Table S1†). The finding that the interface between Cu and ZnO in the precatalysts plays an important role in the reconstruction is certainly interesting and deserves further investigation. However, dedicated studies, which combine identical location or in situ microscopy studies with sensitive analytical techniques, are needed to further understand this phenomenon, which has indeed been reported for other bimetallic systems.27,28
CO2RR measurements
The next step was to study the catalytic performance of the activated Cu@ZnO and ZnO@Cu toward the CO2RR (Fig. 3, S9–S11†). Samples with a Zn atomic percentage equal to 54% were selected for further analysis as an initial electrocatalytic screening of the ZnO@Cu pre-catalysts indicated their higher selectivity towards ethanol (Fig. S10†). While a composition-dependent behavior was observed for the pre-catalysts with the same configuration, we found more intriguing the selectivity switch from ethanol to methane observed for activated ZnO@Cu and Cu@ZnO with the same composition. As discussed above, this observation is indeed highly relevant in the current state-of-the-art of CuZn systems.9–15Fig. 3 shows this difference in selectivity, which becomes particularly evident at potentials more negative than −1.1 VRHE. The activated ZnO@Cu reaches a maximum faradaic efficiency (FE) for ethanol at −1.3 V vs. RHE, corresponding to 39%. Instead, the activated Cu@ZnO reaches a maximum FE for methane of 52% at −1.4 vs. RHE. This high methane selectivity was not observed in any of the ZnO@Cu samples with different Zn concentrations (Fig. S10†), which highlights the importance of the precatalyst configuration rather than simply altering the Cu/Zn ratio. Another interesting observation is that the FE for CO is always higher for the activated ZnO@Cu than for the activated Cu@ZnO across the entire potential range. It is also noteworthy that the total current density (Jtotal = JCO2RR+HER) stays very similar for the two catalysts, along with a very similar electrochemically active surface area (ECSA, Fig. S9†).
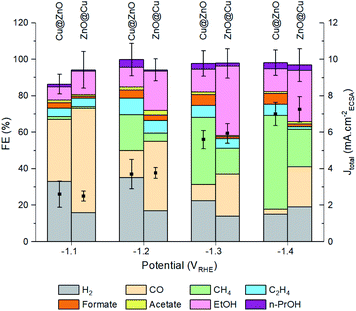 |
| Fig. 3 FEs for different products and CO2RR partial current densities at variable potentials for ZnO@Cu and Cu@ZnO, pre-activated at −1.1 VRHE (20 μg drop-cast on 1 cm2 glassy carbon). The error bars for total FE and Jtotal correspond to the standard deviation from three independent measurements. | |
To highlight the differences between the catalysts, Fig. 4 reports the FEEtOH/FECH4 ratio and the partial current densities of EtOH, CH4 and CO (JEtOH, JCH4 and JCO). The FEEtOH/FECH4 ratio is higher on the activated ZnO@Cu at all of the studied potentials (Fig. 4a). The higher JEtOH of the activated ZnO@Cu and the higher JCH4 of the activated Cu@ZnO across the entire potential range confirm the higher intrinsic activity of the former toward the formation of EtOH and that of the latter toward the production of CH4 (Fig. 4b and c). While JEtOH plateaus at higher overpotentials (Fig. 4b), JCH4 increases going toward more negative potentials (Fig. 4c). The observation that more cathodic potentials favour C1 pathways over C–C coupling is in agreement with the literature and explains the decrease of the FEEtOH/FECH4 ratio for both catalysts as the potential becomes more negative.29–31
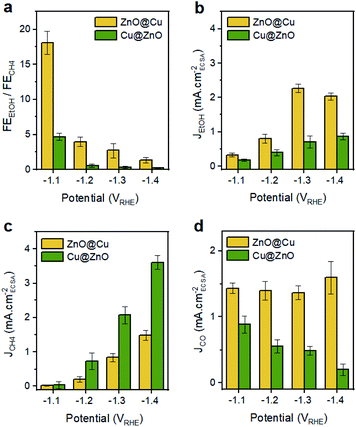 |
| Fig. 4 (a) FEEtOH/FECH4 and (b–d) ECSA-normalized partial current densities for EtOH, CH4 and CO over activated ZnO@Cu and Cu@ZnO NCs. | |
It is interesting to note that the trend of JCO as a function of potential is different for the two catalysts (Fig. 4d). The activity for CO production (indicated by JCO) remains pretty much constant for the activated ZnO@Cu, and instead it decreases as the potential becomes more negative for the activated Cu@ZnO. Regarding the latter, it is expected that a higher portion of surface-bound CO species is likely to be reduced completely to methane rather than undergoing desorption or acting as a C1 intermediate for C–C coupling.31,32 The contrasting potential-independent activity for CO production (indicated by JCO) in activated ZnO@Cu suggests that the ratio of active sites for supplying CO species in this sample is higher than that in Cu@ZnO. If this was not the case, we should have observed a drastic decrease in JCO, since a huge fraction of surface-bound CO has to be consumed as an intermediate for CH4 and EtOH production (see Fig. 4b and c). Nevertheless, the amount of residual CO that is desorbed in this sample remains unchanged.
It is worth noting that neither CH4 nor EtOH is produced in significant amounts from activated ZnO, Cu and Cu–ZnO physical mixtures, which confirms once again the importance of the precatalyst configuration in this study (Fig. S11†). As for the stability, both activated ZnO@Cu and Cu@ZnO samples retain their FEs toward the production of EtOH and CH4 for at least 10 hours (Fig. 5). This finding is opposite to other studies where the significant restructuring during the CO2RR leads to performance changes.15 As such, it highlights the importance of separating the structural changes of the catalysts during activation in order to achieve stable catalytic behavior.
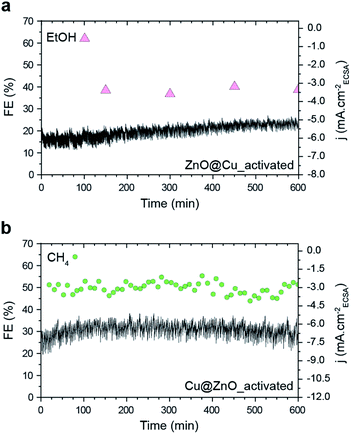 |
| Fig. 5 Ethanol and methane FE and Jtotal of (a) activated ZnO@Cu at −1.3 VRHE and (b) activated Cu@ZnO at −1.4 VRHE. These potentials were selected as they result in the maximum FE for ethanol and methane, respectively. | |
Characterization of the CuZn catalysts during and after the CO2RR
While the stability data do show the successful implementation of the activation step in achieving unchanged catalyst behaviour during operation, the different selectivities of the two catalysts still deserved further attention considering that they enter the course of the CO2RR with no substantial structural or compositional difference. To obtain near-atomic level information, the samples were characterized by high-resolution TEM (HRTEM) and STEM-EDXS after the CO2RR (Fig. 6). Operando XANES and extended X-ray absorption fine structure (EXAFS) measurements were also conducted on both catalysts (Fig. S12 and S13†). We selected −1.3 VRHE for the detailed characterization as the difference in selectivity between the two catalysts was maximized at this potential.
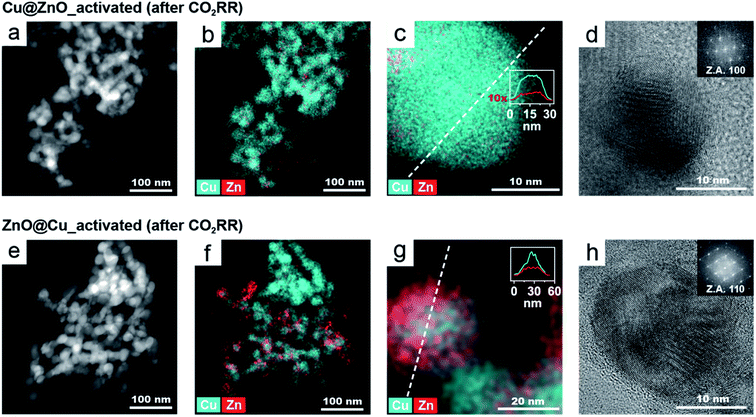 |
| Fig. 6 HAADF-STEM image (a and e), EDX elemental maps (b, c, f, and g) and HRTEM images (d and h) of activated Cu@ZnO (a–d) and activated ZnO@Cu NCs (e–h), after the CO2RR at −1.3 VRHE for 2.5 hours. Insets in the high-resolution EDX maps show an intensity-weighted line profile along the dashed line. Insets in the HRTEM images show the corresponding FFTs used to assign the crystal structures (Z.A = zone axis). | |
Fig. 6 shows the microscopy characterization of the catalysts post-CO2RR. The low magnification STEM-EDXS maps of both activated NCs after the CO2RR (Fig. 6a and e) evidence that their size and morphology remain stable during operation. However, the corresponding EDXS maps (Fig. 6b and f) show a lower amount of Zn in Cu@ZnO (5% Zn) compared to ZnO@Cu (19%) after the CO2RR. These values were also confirmed by complementary inductively coupled plasma-optical emission spectrometry (ICP-OES) analysis on the catalysts post electrolysis.
The EDXS maps at higher magnification and the line profiles (Fig. 6c and g) shows that the Zn and Cu signals are colocalized in each NC. The distinct plateau in the middle of the Zn profile indicates that Zn is mostly present close to the catalyst surface and that the relative Zn amount decreases towards the centre of the particle.
The lattice spacing in the HRTEM images (Fig. 6d and h) corresponds to metallic Cu with a fcc crystal structure. No crystalline domains corresponding to Zn or ZnO were identified in any HRTEM images (nor in their corresponding FFT images). Overall, the electron microscopy characterization indicates that for both catalysts post-CO2RR, the Zn atoms are dispersed at the surface. However, activated ZnO@Cu retains an almost 4-times higher concentration of Zn.
As for operando XAS, it should be noted that this technique provides information on the average NC structure. While during activation a very substantial reconstruction occurs, the changes during the CO2RR are limited to the surface; therefore only subtle differences were observed (Fig. S12 and S13†). Nevertheless, the data were consistent with the presence of CuZn alloys in the shell of the activated catalysts with the degree of alloyed Cu in the ZnO@Cu-derived catalyst being qualitatively higher compared to that of the Cu@ZnO-derived catalyst.
Discussion of the general mechanism
To rationalize the interplay between the Zn content and location within NCs and the resulting catalyst selectivity, we performed density functional theory (DFT) simulations. A (111) surface slab, which is the lowest surface energy under vacuum, was chosen as a model of the Cu spherical domains.33 We then studied the Zn impurity effect on the activity of Cu both as a function of its concentration and of its location. For the latter, we considered both the surface and subsurface as a preference of the Zn atoms to segregate and islanding in either location was not found (Fig. S14†).34
First, we found that the presence of one or more Zn atoms modifies the electronic structure of the system with respect to the case of a pure Cu surface (Fig. S15–S19†). Bader charge analysis35,36 shows a mean charge transfer of 0.15 e− from each Zn atom in the system. On average, the d-band center for Cu adsorption sites37,38 is downshifted with respect to the bare Cu surface by 0.4, 0.35, and 0.26 eV in the presence of one, two, or three Zn atoms. The same parameter evaluated at Zn adsorption sites is slightly upshifted.
We then moved to evaluate the impact of Zn on the energetics of the CO2 reduction pathway. As COH was a crucial intermediate towards CH4, we chose the formation energy of COH from adsorbed CO, ΔECOH, as the activity descriptor and utilized the computational hydrogen electrode model to evaluate the energetics of the elementary proton transfer step (see also the ESI†).39,40 We considered only Cu sites as the catalytically active ones because Cu is the only metal known to form CO2RR products beyond CO.41,42 As illustrated in Fig. 7a and S15–S16,† the ΔECOH value decreases as soon as one Zn atom is introduced into the copper slab and it changes to a lesser extent as the number of Zn atoms increases or their location (i.e. surface or subsurface) changes.
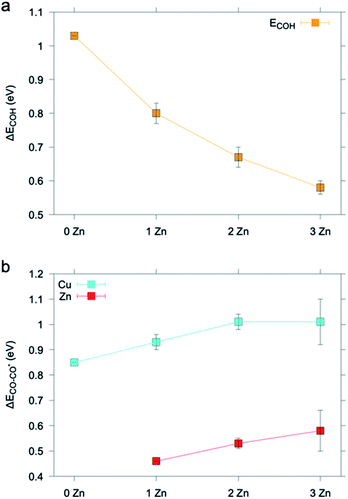 |
| Fig. 7 (a) ΔECOH and (b) ΔECO–CO* calculated on a pure Cu (111) slab embedding up to 3 Zn atoms. Results are averaged over data found for different non-equivalent adsorption sites (Fig. S18–S22†). The ΔECOH value found for the Cu (111) slab matches that previously reported in the literature.40 The ΔECO–CO* value found for the Cu (111) slab is in good agreement with that previously reported in the literature for CO adsorption and is an overestimate with respect to the experimental value.43 | |
To rationalize the higher CO production of the activated ZnO@Cu NCs, we considered the change of CO desorption energy, ΔECO–CO*,43 at the Cu and Zn sites as Zn atoms are added into Cu. Fig. 7b and S17–S19† show that ΔECO–CO* increases on both the Cu site and the Zn site as one to a few Zn atoms are added into the system. In agreement with d-band theory,37,38 the Zn adsorption site d-band upshift corresponds to a stronger metal-adsorbate interaction. Vice versa, the trends found for the case of Cu represent an exception to predictions drawn from d-band theory.44 This behavior has been already previously reported in other theoretical studies on the adsorption properties of Cu sites in CuZn systems.45 We note that future and dedicated studies may be conducted to clarify the origin of this exception.
The increase in the strength with which CO is adsorbed on the Cu sites is beneficial to the selective reduction of CO2 to C2+ products.31 On the other hand, the absolute value of ΔECO–CO* is lower for the Zn site, consistent with Zn being a CO-producing metal.41,46 This finding implies that the higher the Zn concentration is, the more the CO evolution is favored, which is also related to the increased possibility that Zn atoms cluster on the surface. Having learned this, we speculate that the increase in EtOH production for the activated ZnO@Cu NCs is further connected to tandem effects, which is consistent with previous observations.10,43 Notice that oxophilicity, i.e. the ability of secondary components to increase the formation of oxygenates, has also been proposed, and actually, the binding energy of O atoms to Zn is larger than that of Cu alone.47 Our computational results are in line with the experimental observations demonstrating higher alcohol formation for the samples containing more Zn on the surface of ZnO@Cu. In addition, it demonstrates how robust structure–activity relationships require the exquisite characterization of the fine details of the structure and how composition only analyses are a too simplified proxy for the structure.
Conclusions
In summary, this work has introduced tailored Cu/ZnO NCs as precatalysts for the CO2RR to investigate the compositional and structural sensitivities of the CO2RR in bimetallic Cu–Zn catalysts. We found that Cu@ZnO and ZnO@Cu NCs evolve towards alloyed CuZn in a core@shell configuration, with a Cu-rich core and a Zn-rich shell. The ZnO@Cu-derived and Cu@ZnO-derived catalysts showed selectivity for ethanol and methane, respectively. The main difference between the two stems from the higher degree of surface alloying and higher concentration of metallic Zn in the EtOH selective catalyst.
DFT simulations show that diluted amounts of Zn impurities affect the electronic structure of the catalyst and promote CH4 selectivity, while a higher Zn content increases the local CO concentration and enables a tandem conversion mechanism which determines the selectivity shift from CH4 to EtOH.
This conclusion helps to reconciliate previous results in the literature. In particular, a lower degree of alloying promotes the formation of CH4 is consistent with the results reported by Jeon et al., who investigated Cu–Zn nano catalysts in a similar size regime and composition.15 The higher degree of surface alloying is in line with another study, where CuZn bimetallic catalysts obtained by in situ electrochemical reduction of CuO and ZnO promoted the selectivity towards EtOH, in which case a tandem catalysis mechanism was proposed.10 In addition, the present results indicate that composition is not a single descriptor for activity and that the local structure is crucial to performance. Indeed, we provide evidence that materials with identical compositions evolve into different catalysts even under strong catalytic equilibration conditions.
Towards the future, synergies between tandem and electronic effects are to be sought after to rationally tune bimetallic catalyst selectivity towards desired products, such as alcohols. In this direction, studies on Cu@ZnO core@shell synthesized via colloidal ALD are envisioned to achieved atomic control and will help to investigate further this topic.48
Experimental section
Chemicals
Copper(I) acetate (or Cu(OAc), 97%), trioctylphosphine ({CH3(CH2)7}3P, or TOP, 90%), oleic acid (C17H33CO2H or OLAC, 90%), oleylamine (C17H33NH2 or OLAM, 70%), and 1-octadecene (C18H36 or ODE, 90%) were all purchased from Sigma Aldrich and used as received.
Synthesis of Cu/ZnO precatalysts
30 mL of an ODE solution containing pre-synthesized ZnO NCs (0.5 mM) in a 100 mL three-necked flask were degassed under dynamic vacuum for 20 min at 130 °C, after which they were heated under a N2 flow to 300 °C for 5 minutes. ZnO NCs of 13 nm or 60 nm were used as nucleation seeds for the copper (Fig. S1†). The copper precursor solution was prepared by mixing 0.1 mmol of Cu(OAc), 0.396 mmol of TOP, 0.02 mmol of OLAM and 0.02 mmol of OLAC in degassed ODE (1.4 mL). To obtain a Zn atomic percentage of 54%, 450 μL of this solution were then added dropwise to the flask containing the seeds at a rate of 0.18 mL min−1 using a syringe pump, during which the colour of the reaction mixture slowly changed from colourless to brown. At the end of the injection, after around 150 s, the reaction was stopped, and the mixture was allowed to cool down to room temperature by removing the heating mantle. The NCs were extracted and purified by repeated washing/centrifugation cycles inside a N2 glovebox to avoid Cu oxidation. 30 mL each of anhydrous EtOH and toluene were added to the reaction mixture at room temperature and the mixture was divided into four centrifugation vials; the particles were collected by centrifugation at 5000 rpm for 15 min. The precipitate was washed twice with EtOH to remove unreacted precursor and surfactants. Finally, the NCs were re-dispersed in hexane or toluene for storage.
Characterization
Electron microscopy.
Samples were drop-cast on a transmission electron microscopy (TEM) grid (Ted Pella, Inc.) prior to imaging. Bright field TEM images were taken with a FEI Tecnai Spirit at 120 keV. Scanning transmission electron microscopy and high angle annular dark field imaging (STEM-HAADF) as well as energy dispersive X-ray spectroscopy (EDXS) were performed on a FEI Tecnai Osiris transmission electron microscope in scanning mode at an accelerating voltage of 200 kV. This microscope is equipped with a high brightness X-FEG gun, silicon drift Super-X EDXS detectors and a Bruker Esprit acquisition software. Aberration-corrected high-resolution TEM (HRTEM) and STEM-HAADF data were acquired using a Thermo Fisher Scientific Titan Themis operated at 300 kV with dual aberration correctors.
X-ray photoelectron spectroscopy (XPS).
XPS was carried out using an Axis Supra (Kratos Analytical) instrument. Analysis was carried out using a monochromatic Al Kα X-ray source operating at 25 W with a beam size of 100 μm. The pass energy was set to 20 eV with a step size of 0.1 eV. Samples were measured on clean Si wafers, electrically insulated from the sample holder and charges were compensated. The binding energy of the spectra was calibrated by setting the C–C bond of the C 1s peak at 284.8 eV.
Inductively coupled plasma-optical emission spectrometry (ICP-OES).
ICP-OES was performed on an Agilent 5100 set-up to determine the elemental concentrations. Metal ions were digested after the evaporation of the hexane/toluene solvent (100 μL of the stock solution was used per analysis). 285 μL of HNO3 (70%) was added to the nanocrystals (NCs) and left overnight to ensure complete digestion of the samples. Following this step, 9.715 mL of Milli-Q water was added to the solution to reach the 2% acid content needed for the analysis. 5 standard solutions of each element were prepared to obtain the calibration curve that was used to determine the concentrations of the digested solutions.
X-ray diffraction (XRD).
XRD was carried out on a Bruker D8 Advance diffractometer with a Cu Kα source equipped with a Lynxeye one-dimensional detector. The diffractometer was operated at 40 kV and 40 mA with a Cu Kα source with a wavelength of λ = 1.54 Å.
Thermogravimetric analysis (TGA).
In order to determine the loading of metal oxide NC seeds, 60 μL of the NC stock solution was deposited in an aluminium pan, and the solvent was evaporated. The pan was then introduced into a TGA Q500 with an air flow rate of 15 mL min−1. The sample was heated to 600 °C with a heating ramp of 10 °C min−1. On the basis of the final weight, the concentrations of metal oxide solutions were calculated.
Electrocatalytic measurements
Electrochemical CO2RR.
The electrocatalytic testing was performed in a custom-made electrochemical flow cell. Glassy carbon plate electrodes (Type 2, Alfa Aesar) with a surface area of 2.5 cm × 2.5 cm were used as substrates. They were polished using Milli-Q water slurries of 1 μm diamond (Bioanalytical Systems, Inc.) and 50 nm γ-alumina (Bioanalytical Systems, Inc.) on polishing pads. The plates were rinsed and sonicated in Milli-Q water for 10 min and blown dry with nitrogen. The NC samples were drop-cast on the substrate from toluene suspensions, giving a mass loading of 15 μg. Electrochemical measurements were carried out using a Biologic SP-300 potentiostat. Platinum foil was employed as the counter electrode, and an Ag/AgCl reference electrode (leak free series from Innovative Instruments, Inc.) was used. Voltages were converted to the reversible hydrogen electrode (RHE) scale. Ambient-pressure CO2 electrolysis was performed in a custom-made gastight electrochemical cell made of poly(methyl methacrylate) and fitted with Buna-N O-rings. The configuration of the electrochemical cell was such that the working electrode sat parallel with respect to the counter electrode to ensure a uniform potential distribution across the surface. The geometric surface area of both electrodes was 1 cm2. Each of the compartments in this cell was filled with the electrolyte (CO2 saturated 0.1 M KHCO3 solution, 2.1 mL in each half). A Selemion AMV anion-exchange membrane was used to separate the anodic and cathodic compartments. The electrolyte solution was prepared by sparging a 0.05 M K2CO3 solution with CO2 for 1 h. Before CO2 electrolysis, the electrolyte in the cell was sparged with CO2 for at least 15 min. During electrolysis, CO2 was constantly bubbled through the electrolyte at a flow rate of 5 sccm to prevent the depletion of CO2 in the electrolyte and to enable continuous analysis of gaseous products using a gas chromatograph. The flow rate of CO2 was controlled with a mass flow-controller (Bronkhorst), and the gas was first humidified with water by passing it through a bubbler to minimize the evaporation of the electrolyte. The calibration of the gas chromatograph was done using calibration gas standards from Carbogen. CO, CH4, C2H4 and C2H6 were all calibrated from calibration mixtures at five different concentrations: 50, 100, 250, 500, and 1000 ppm. H2 was likewise calibrated using 500, 1000, 5000, and 10
000 ppm of H2 balanced in nitrogen. After passing through the cell, CO2 was flowed directly into the gas-sampling loop of the GC for online gaseous product analysis, which was carried out every 10 min. The chronoamperometry experiments were performed for a total of 2.5 hours. The liquid products were collected from the electrolyte after electrolysis and analysed by high-performance liquid chromatography (HPLC) on an UltiMate 3000 instrument from Thermo Scientific.
Operando X-ray absorption spectroscopy (XAS)
Operando.
XAS experiments were performed at the Swiss-Norwegian beamlines BM31 at the European Synchrotron Radiation Facility (ESRF) in France. The catalyst solution was drop-casted on a thin (2.5 × 2.5 × 0.5 mm3) glassy carbon (GC) support and a Kapton window allowed the X-rays to pass through. For standards and other ex situ measurements (typically in the pellet form and with the catalyst diluted in a light matrix such as boron nitride or cellulose to obtain an appropriate thickness, typically of 2–3 absorption lengths), we used a traditional system based on ionization chambers for transmission detection.
The measurements were carried out in fluorescence mode at an incident angle of approximately 45 degrees. A Si(111) double crystal monochromator (DCM) was used to condition the beam from the corresponding bending magnet. Fluorescence X-ray absorption near edge structure (XANES) spectra were acquired using a Vortex® one-element silicon drift detector (SDD) with XIA-Mercury digital electronics with a time-resolution of 1 min per spectrum at both Cu and Zn K-edges (i.e. 2 min per Cu/Zn cycle). Data extraction, normalization, and averaging were performed using the beamline dedicated software for QEXAFS data analysis, PrestoPronto.17
The Demeter package (Athena and Artemis)18 was employed for the EXAFS fitting of the catalyst. Finally, a multi-use beamline fixation support permits the cell alignment with respect to the incoming beam via an XYZ high-precision translation stage.
Multivariate curve resolution (MCR) analysis.
We have made use of multivariate curve resolution using an alternating least-square mathematical algorithm (MCR-ALS) to analyse the large number of XANES spectra generated during the operando CO2RR measurements. MCR-ALS is a data-reduction tool that can extract (even in a blind manner) kinetically pure component spectra and the corresponding concentration profiles out of an unresolved mixture.19–21 The MCR-ALS model relies on the D = CST + E mathematical equation, where D: initial matrix composed of all collected spectra, C: extracted concentration profiles, S: extracted spectra profiles (T stands for the transposed matrix), and E: error matrix containing the residuals and close to the experimental error. The MCR-ALS graphical user interface (GUI) for MATLAB used in this paper (freeware available at http://www.mcrals.info/) was applied on the Cu and Zn K-edge time-resolved datasets by employing positive constraints for both spectra and concentration profiles, and closure constraints for the concentration profiles (i.e. constant concentration of the absorbing atoms throughout the experiment).
Density functional theory calculations
Density Functional Theory (DFT) calculations were carried out with CP2K software.22 A DZVP-MOLOPT23 basis set was used in the calculations, with core electrons being described with dual-space Goedecker–Teter–Hutter24 pseudopotentials. The plane waves and relative cut-off were set to 500 Ry and 50 Ry respectively. For adsorption energy calculations, the (111) Cu facet was chosen, in agreement with the observed orientation found in XRD. A (4 × 4) slab with 4 layers was considered. Replicas along the axis normal to the (111) slabs are separated by 22 Å. Surface dipole corrections were applied along the plane normal to the surface. The two bottom layers of the surface were kept fixed during structural relaxations. The latter were deemed converged when all the atomic forces were found to be at least less than 0.009 H per Bohr (∼0.45 meV A−1). The DFT calculations of the energy of the system were instead converged when a change less than 10−6 H was registered between two successive self-consistent electronic density calculation loops.
Data availability
The most relevant inputs and outputs of the DFT calculations are available on the Materials Cloud, DOI: 10.24435/materialscloud:3r-gn.
Author contributions
S. B. V. and R. B. conceived and designed the experiments. S. B. V. carried out the chemical synthesis and the electrochemical experiments and analysed the results. S. B. V. and D. S. performed the XAS experiments. D. S. analyzed the XAS results. S. B. V. and J. V. performed the electron microscopy characterization. K. R. and N. L. developed the methodology and performed the DFT calculations. J. R. P. carried out and analyzed the XPS measurements. Y. T. G. contributed to the HPLC measurements. S. B. V. and R. B. assembled the figures and co-wrote the manuscript. All authors discussed the results and commented on the manuscript.
Conflicts of interest
There are no conflicts to declare.
Acknowledgements
This work was financially supported by the European Research Council (ERC) under the European Union's Horizon 2020 research and innovation program (grant agreement no. 715634-HYCAT). JRP and KR acknowledge the H2020 Marie Curie Individual Fellowship grants SURFCAT and NANOCO2RE with agreement numbers 837378 and 890414, respectively. D. S. was supported by the Sandoz Foundation. The theoretical effort was supported by EPFL computational facilities and the Swiss National Supercomputer Center (CSCS), project “sm54”. We thank Dr F. Dattila for useful discussions regarding the technical aspects of the simulations. This publication was created as part of NCCR Catalysis, a National Centre of Competence in Research funded by the Swiss National Science Foundation.
Notes and references
- S. Nitopi, E. Bertheussen, S. B. Scott, X. Liu, A. K. Engstfeld, S. Horch, B. Seger, I. E. L. Stephens, K. Chan, C. Hahn, J. K. Nørskov, T. F. Jaramillo and I. Chorkendorff, Chem. Rev., 2019, 119, 7610–7672 Search PubMed.
- D. Karapinar, C. E. Creissen, J. G. Rivera De La Cruz, M. W. Schreiber and M. Fontecave, ACS Energy Lett., 2021, 6, 694–706 Search PubMed.
- F. Li, Y. C. Li, Z. Wang, J. Li, D. H. Nam, Y. Lum, M. Luo, X. Wang, A. Ozden, S. F. Hung, B. Chen, Y. Wang, J. Wicks, Y. Xu, Y. Li, C. M. Gabardo, C. T. Dinh, Y. Wang, T. T. Zhuang, D. Sinton and E. H. Sargent, Nat. Catal., 2020, 3, 75–82 Search PubMed.
- C. G. Morales-Guio, E. R. Cave, S. A. Nitopi, J. T. Feaster, L. Wang, K. P. Kuhl, A. Jackson, N. C. Johnson, D. N. Abram, T. Hatsukade, C. Hahn and T. F. Jaramillo, Nat. Catal., 2018, 1, 764–771 Search PubMed.
- L. R. L. Ting, O. Piqué, S. Y. Lim, M. Tanhaei, F. Calle-Vallejo and B. S. Yeo, ACS Catal., 2020, 10, 4059–4069 Search PubMed.
- P. Iyengar, M. J. Kolb, J. R. Pankhurst, F. Calle-Vallejo and R. Buonsanti, ACS Catal., 2021, 11, 4456–4463 Search PubMed.
- S. Lee, G. Park and J. Lee, ACS Catal., 2017, 7, 8594–8604 Search PubMed.
- Y. C. Li, Z. Wang, T. Yuan, D. H. Nam, M. Luo, J. Wicks, B. Chen, J. Li, F. Li, F. P. G. De Arquer, Y. Wang, C. T. Dinh, O. Voznyy, D. Sinton and E. H. Sargent, J. Am. Chem. Soc., 2019, 141, 8584–8591 Search PubMed.
- D. Ren, B. S. H. Ang and B. S. Yeo, ACS Catal., 2016, 6, 8239–8247 Search PubMed.
- D. Ren, J. Gao, L. Pan, Z. Wang, J. Luo, S. M. Zakeeruddin, A. Hagfeldt and M. Grätzel, Angew. Chemie Int. Ed., 2019, 58, 15036–15040 Search PubMed.
- Z. Li, R. M. Yadav, L. Sun, T. Zhang, J. Zhang, P. M. Ajayan and J. Wu, Appl. Catal., A, 2020, 606, 117829 Search PubMed.
- A. H. M. da Silva, S. J. Raaijaman, C. S. Santana, J. M. Assaf, J. F. Gomes and M. T. M. Koper, J. Electroanal. Chem., 2020, 880, 114750 Search PubMed.
- H. Hu, Y. Tang, Q. Hu, P. Wan, L. Dai and X. J. Yang, Appl. Surf. Sci., 2018, 445, 281–286 Search PubMed.
- G. Keerthiga and R. Chetty, J. Electrochem. Soc., 2017, 164, H164–H169 Search PubMed.
- H. S. Jeon, J. Timosnenko, F. Scholten, I. Sinev, A. Herzog, F. T. Haase and B. R. Cuenya, J. Am. Chem. Soc., 2020, 141, 19879–19887 Search PubMed.
- L. Wang, H. Peng, S. Lamaison, Z. Qi, D. M. Koshy, M. B. Stevens, D. Wakerley, J. A. Zamora Zeledón, L. A. King, L. Zhou, Y. Lai, M. Fontecave, J. Gregoire, F. Abild-Pedersen, T. F. Jaramillo and C. Hahn, Chem. Catal., 2021, 1, 663–680 Search PubMed.
-
S. J. A. Figueroa and C. Prestipino, in Journal of Physics: Conference Series, Institute of Physics Publishing, 2016, vol. 712, 1–4, p. 012012 Search PubMed.
- B. Ravel and M. Newville,
J. Synchrotron Radiat.
, 2005, 12, 537–541 Search PubMed.
- C. Ruckebusch and L. Blanchet, Anal. Chim. Acta, 2013, 765, 28–36 Search PubMed.
- A. De Juan, J. Jaumot and R. Tauler, Anal. Methods, 2014, 6, 4964–4976 Search PubMed.
- J. Jaumot, R. Gargallo, A. De Juan and R. Tauler, Chemom. Intell. Lab. Syst., 2005, 76, 101–110 Search PubMed.
- J. Hutter, M. Iannuzzi, F. Schiffmann and J. Vandevondele, Wiley Interdiscip. Rev.: Comput. Mol. Sci., 2014, 4, 15–25 Search PubMed.
- J. VandeVondele and J. Hutter, J. Chem. Phys., 2007, 127, 114105 Search PubMed.
- M. Krack, Theor. Chem. Acc., 2005, 114, 145–152 Search PubMed.
- S. B. Varandili, J. Huang, E. Oveisi, G. L. De Gregorio, M. Mensi, M. Strach, J. Vavra, C. Gadiyar, A. Bhowmik and R. Buonsanti, ACS Catal., 2019, 9, 5035–5046 Search PubMed.
- S. B. Varandili, D. Stoian, J. Vavra, J. Pankhurst and R. Buonsanti, Chem. Sci., 2020, 11, 13094–13101 Search PubMed.
- M. Gatalo, P. Jovanovič, U. Petek, M. Šala, V. S. Šelih, F. Ruiz-Zepeda, M. Bele, N. Hodnik and M. Gaberšček, ACS Appl. Energy Mater., 2019, 2, 3131–3141 Search PubMed.
- M. Gatalo, P. Jovanovič, F. Ruiz-Zepeda, A. Pavlišič, A. Robba, M. Bele, G. Dražić, M. Gaberšček and N. Hodnik, J. Electrochem. Sci. Eng., 2018, 8, 87–100 Search PubMed.
- T. K. Todorova, M. W. Schreiber and M. Fontecave, ACS Catal., 2020, 10, 1754–1768 Search PubMed.
- I. Ledezma-Yanez, E. P. Gallent, M. T. M. Koper and F. Calle-Vallejo, Catal. Today, 2016, 262, 90–94 Search PubMed.
- K. P. Kuhl, E. R. Cave, D. N. Abram and T. F. Jaramillo, Energy Environ. Sci., 2012, 5, 7050 Search PubMed.
- L. Ou and J. Chen, Phys. Chem. Chem. Phys., 2019, 22, 62–73 Search PubMed.
- R. Tran, Z. Xu, B. Radhakrishnan, D. Winston, W. Sun, K. A. Persson and S. P. Ong, Sci. Data, 2016, 3, 1–13 Search PubMed.
- N. López and C. Vargas-Fuentes, Chem. Commun., 2012, 48, 1379–1391 Search PubMed.
- G. Henkelman, A. Arnaldsson and H. Jónsson, Comput. Mater. Sci., 2006, 36, 354–360 Search PubMed.
- R. F. W. Bader, Chem. Rev., 2002, 91, 893–928 Search PubMed.
- B. Hammer and J. K. Norskov, Nature, 1995, 376, 238–240 Search PubMed.
- A. Vojvodic, J. K. Nørskov and F. Abild-Pedersen, Top. Catal., 2014, 57, 25–32 Search PubMed.
- J. K. Nørskov, J. Rossmeisl, A. Logadottir, L. Lindqvist, J. R. Kitchin, T. Bligaard and H. Jónsson, J. Phys. Chem. B, 2004, 108, 17886–17892 Search PubMed.
- Z. Wang, Q. Yuan, J. Shan, Z. Jiang, P. Xu, Y. Hu, J. Zhou, L. Wu, Z. Niu, J. Sun, T. Cheng and W. A. Goddard, J. Phys. Chem. Lett., 2020, 11, 7261–7266 Search PubMed.
- X. Liu, J. Xiao, H. Peng, X. Hong, K. Chan and J. K. Nørskov, Nat. Commun., 2017, 8, 1–7 Search PubMed.
- A. Bagger, W. Ju, A. S. Varela, P. Strasser and J. Rossmeisl, ChemPhysChem, 2017, 18, 3266–3273 Search PubMed.
- Y. Huang, Y. Chen, T. Cheng, L. W. Wang and W. A. Goddard, ACS Energy Lett., 2018, 3, 2983–2988 Search PubMed.
- H. Xin and S. Linic, J. Chem. Phys., 2010, 132(1–4), 221101 Search PubMed.
- X. Fan, Q. L. Tang, X. Zhang, T. T. Zhang, Q. Wang, X. X. Duan, M. L. Zhang and M. Y. Yao, Appl. Surf. Sci., 2019, 469, 841–853 Search PubMed.
-
Y. Hori, in Modern Aspects of Electrochemistry, Springer, New York, 2008, pp. 89–189 Search PubMed.
- L. R. L. Ting, R. García-Muelas, A. J. Martín, F. L. P. Veenstra, S. T.-J. Chen, Y. Peng, E. Y. X. Per, S. Pablo-García, N. López, J. Pérez-Ramírez and B. S. Yeo, Angew. Chem., 2020, 132, 21258–21265 Search PubMed.
- A. Loiudice, M. Strach, S. Saris, D. Chernyshov and R. Buonsanti, J. Am. Chem. Soc., 2019, 141, 8254–8263 Search PubMed.
Footnote |
† Electronic supplementary information (ESI) available. See DOI: 10.1039/d1sc04271h |
|
This journal is © The Royal Society of Chemistry 2021 |
Click here to see how this site uses Cookies. View our privacy policy here.