DOI:
10.1039/D1SC04249A
(Edge Article)
Chem. Sci., 2021,
12, 15353-15361
SiRNA-templated 3D framework nucleic acids for chemotactic recognition, and programmable and visualized precise delivery for synergistic cancer therapy†
Received
3rd August 2021
, Accepted 4th November 2021
First published on 5th November 2021
Abstract
Developments in framework nucleic acids (FNAs) are limited by complicated synthesis, by-product interference, and low framework utilization. Herein, simple core–shell spherical 3D FNAs (ST-SFNAs) preparation is presented based on siRNA-templated linear polymerization followed by hybridization chain reaction branched polymerization. Without by-products, all components exhibited their special functions to obtain high space utilization of ST-SFNAs. ST-SFNAs were covered by catalase and folic acid-functionalized liposome membranes. The catalase endowed ST-SFNAs with chemotactic activities in the H2O2 reaction catalyzed by catalase. Furthermore, combined with functionalized folic acids' targeting folate receptors, the synergistic chemotactic recognition of cancer cells was obtained. This dramatically promoted targeted cellular uptakes compared with traditional active or passive targeting pathways. Subsequently, the cascaded-logical programmable release of drugs was precisely controlled by targeting glutathione and ATP (via S–S bond and ATP aptamer on the inner g-DNA cover). This was visualized by “turn on” fluorescent signals generated by special hybridization of released hairpin DNAs with survivin mRNA biomarkers. Simultaneously, biocompatible synergistic therapy was achieved by simultaneously releasing doxorubicin and siRNA. With its high utilization for synergistic chemotactic recognition, programmable and visualized delivery, as well as synergistic therapy, an efficient platform for maximizing the therapeutic efficacy has been developed. This would initiate further FNA-based material development for a variety of biological applications.
Introduction
Nucleic acids (e.g., DNA and RNA) have recently inspired tremendous efforts for constructing sophisticated nanostructures by folding or assembling specifically designed nucleic acids.1–6 Framework nucleic acids (FNAs) were constructed by organizing molecules in a plug-and-play manner with nanoscale addressability. They are highly attractive as delivery carriers of drugs for precise diagnosis and treatment in vivo.7–17 Compared with DNA origami techniques, DNA hydrogels can obtain more orderly and intensive frameworks for efficient applications.18–21 However, the development of FNAs still remains challenging because of the following problems: (1) to enhance the precise target-delivery efficiency, the structural integrity and stability must strictly be guaranteed, which greatly burdens both design and synthesis. (2) The loading efficiency is still limited by components with little contribution to subsequent bio-applications. These components lead to low FNA utilization and could thus be regarded as “useless components”. (3) The present synthesis of DNA hydrogels is still hindered by the requirement of metal templates22–25 or the by-products generated by side-polymerization.26–30 Therefore, simple preparation of FNAs, with orderly and stable frameworks but fewer by-products or useless components, is the current bottleneck of further FNA development.
Furthermore, accurate activation of FNA nanocarriers for target delivery as well as controllable and precise cargo release have become key issues that must be addressed to enable further developments. For these purposes, the step-by-step modifications of tumor-targeting ligands on nanomaterials are widely used for the following photochemical caging.31,32 However, these accumulation and release processes still suffer from low targeting activity, passive and disjointed dynamics, phototoxicity, and low tissue penetration. These obstacles strongly obstruct efficient and biocompatible in vivo applications. Recently, chemotactic movements,33,34 cellular computation,35 programming drug delivery,22,36,37 and stimuli-triggered/controlled release38–42 have heralded the modern era of advanced bio-applications. Therefore, endowing FNAs with advanced functions, including logic-controlled recognition, precise delivery, and synergistic treatments, is strongly worthy of studying.
Herein, to strongly increase the utilization of FNAs, spherical core–shell 3D FNAs were simply prepared based on the hybridization chain reaction (HCR) using siRNA (a gene silencing reagent) as the template. The prepared FNAs were termed siRNA-templated spherical FNAs (ST-SFNAs). Without the requirement of metals or other polymers as needed by traditional methods, this siRNA-templated cross-linking by DNA monomers makes full use of all components. Thus, without by-products or “useless components” for construction, the ST-SFNAs possess a high space utilization of 3D spherical frameworks for loadings. The frameworks were further modified with synergistic targeting elements, logical stimulators, and loading with synergistic drugs for efficient biological applications. Verified by both in vitro and in vivo tests, chemotactic recognition, cascaded-logical controlled, precise, and visualized drug delivery, and synergistic cancer therapy have been achieved. The present work developed an extreme platform of 3D FNAs for biological applications via a simple and smart design.
Results and discussion
Design of siRNA-templated 3D ST-FNAs
As illustrated in Scheme 1A, ST-SFNAs featured a multifunctional core–shell nanostructure, where the cores of ST-FNAs are covered by noncationic liposome membranes. Innovatively, without using metal templates, ST-SFNA cores were prepared with drugs of single-stranded dangling siRNA as templates. Using siRNA as an initiator, the subsequent hybridization chain reactions (HCRs) were initiated for core construction. For HCR polymerization, four hairpin DNA monomers were designed with single-stranded toeholds as HCR components (the sequences of which are shown in Table S1, ESI†). As designed, H1 and H3 possessed the abilities to hybridize with the target of survivin mRNA. Therefore, with siRNAs as templates, and H1 and H3 as HCR components, the cores were endowed with extremely high utilization toward in vivo applications.
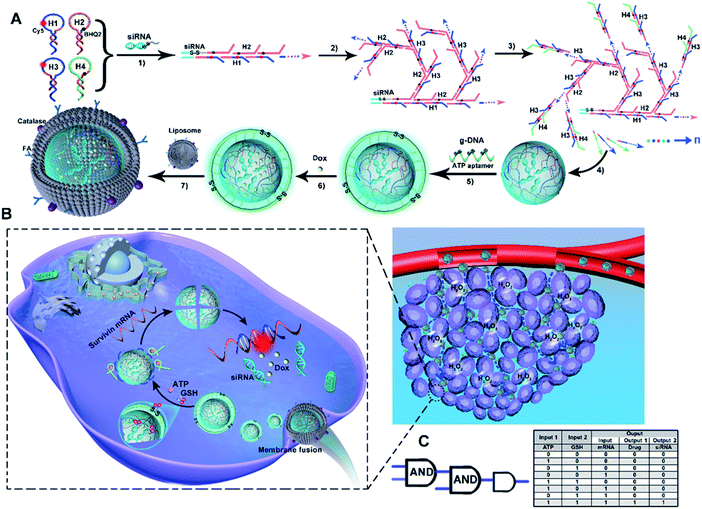 |
| Scheme 1 Design of siRNA-templated 3D spherical FNAs (ST-SFNAs). (A) Illustration of synthesis. (B) Illustration of target-triggered, programmable, and visualized delivery of siRNA and Dox. (C) The truth table of the core logic circuit. | |
In detail, the template of siRNAs triggered the linear polymerization of H1 and H2 (Scheme 1A-1), followed by branched chain polymerization with the addition of H3 and H4 (Scheme 1A-2 and 3). Here, H1 and H3 were labelled with Cy5. H2 and H4 were labelled with the corresponding quenchers of BHQ2, which could turn off Cy5 signals via the HCR. Then, the cores of ST-SFNAs were generated under polymerization recycling (Scheme 1A-4). Thereafter, the HCR was terminated by adding g-DNAs to break the polymerization of DNA branches, which yielded a controllable size of ST-SFNA cores. Simultaneously, g-DNAs were added as a cover for ST-SFNA cores (Scheme 1A-5). Furthermore, disulfide linkages (S–S) and ATP aptamers in g-DNAs also acted as cascaded logical gates for the dual-recognition of GSH and ATP. Subsequently, another small molecular drug doxorubicin (Dox) was loaded into the cores of ST-SFNAs by simple incubation (Scheme 1A-6). Therefore, the g-DNAs can not only terminate the HCR to obtain the final size of ST-SFNA cores, but also generate programmed responses for glutathione (GSH) and ATP. This is an ideal logic-based biological computing system for activating controllable and specific targeting. Finally, to ensure smooth intracellular delivery, ST-FNA cores were covered with noncationic liposome membranes to avoid RNase degradation. Moreover, these liposome membranes were modified with catalase and folic acid (FA) to exhibit chemotactic penetration and synergistic targeting. This process depended on responses to the excess H2O2 in tumor microenvironments and the overexpression of folate receptors (FRs) on the surface of cancer cells (Scheme 1A-7). Therefore, ST-SFNAs were prepared with high utilization of each component and were identified as extreme motors for subsequent biological applications.
Scheme 1B illustrates the cascaded-logical controlled and precise drug delivery. Covered by catalase and folic acid-functionalized liposome membranes, ST-SFNAs accumulated and entered cancer cells via chemotactic movement and synergistic targeting. This process was achieved under the chemotactic power of the H2O2 reaction catalyzed by catalase and targeting of FA to FR on the surface of tumor cells (see the inset of Scheme 1B). This dramatically promoted cellular uptakes compared with traditional active or passive targeting pathways.43–45 Simultaneously, liposome membranes were fused46 to expose ST-SFNA cores with g-DNA covers. Subsequently, by virtue of responses to GSH, ATP, or survivin mRNA, external and internal cascaded-logic gates (with a user-programmed Boolean logic-based algorithm) were constructed for programmable and precise releases (Scheme 1C). In detail, with the overexpression of GSH and ATP in cancer cells, the g-DNA cover was cleaved. This synergetic cleaving was obtained based on the reduction of S–S by GSH and the interaction between ATP and its aptamer on the cover. Then, the small GSH molecule further permeated into internal ST-SFNA cores to break framework structures via S–S reduction. Therefore, the external logic gate was opened by input responses to GSH and ATP, which simultaneously released H1 and H3 to hybridize with the targets of survivin mRNA. This hybridization led to the collapse of frameworks, which emitted Cy5 fluorescent (FL) signals because of the enlarged distance between Cy5 and BHQ2. This process can be visualized by “turn on” FL signals. Herein, FL responses acted as both output signals of the external logical gate and input signals of the internal logical gate. This user-programmed algorithm based on Boolean logic finally resulted in the precise release of drugs (i.e., Dox and siRNA). Therefore, with synergistic targeting and chemotaxis, a cascade-logic controlled ST-SFNA system was constructed for programmable, visualized, and precise delivery of drugs into cancer cells.
Morphology characterization of ST-SFNAs
To confirm the successful preparation of ST-SFNAs, morphology characterization was employed. Demonstrated by transmission electron microscopy (TEM), ST-SFNA cores were about 67 nm (Fig. 1A), and the final ST-SFNAs were about 109 nm after liposome coverage (Fig. 1B). This was slightly larger than the pure liposome sphere of 102 nm (Fig. 1C) because of the presence of ST-SFNAs in cores. However, all sizes were slightly smaller than those obtained by dynamic light scattering (DLS) (Fig. 1D), because of the hydration of ST-SFNAs under aqueous conditions. In fact, the size of ST-SFNAs can be conveniently controlled by varying the reaction time of the branched chain polymerization (Fig. S1, ESI†), which can be flexibly designed for subsequent in vivo applications. Considering that the size is important for their fates in the bloodstream, about 109 nm liposome-covered ST-SFNAs were adopted for the subsequent experiments. Moreover, the zeta potential measurement also confirmed the successful coverage of ST-SFNA cores by liposomes. As shown in Fig. 1E, the zeta potential of cores (black line) increased when they were covered by the liposomes (blue line), which is because of the highest zeta potentials of liposomes (red line).
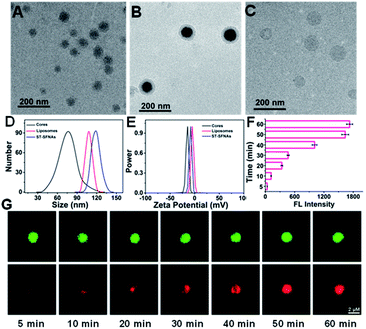 |
| Fig. 1 Morphology characterization of ST-SFNAs. TEM images of ST-SFNA cores (A), present ST-SFNAs (B) and pure liposome spheres (C). (D) DLS data. (E) Zeta potentials of three particles. Rhodamine signals (F) and images (G) of ST-SFNA cores after incubation with rhodamine for different times. This was used to verify the diffusion ability of small molecules in cores. ST-SYBR Green I was selected as the co-localization regent. | |
Furthermore, the diffusion ability was assessed by incubating ST-SFNA cores with rhodamine molecules (with red FL signals) for different times. As demonstrated in Fig. 1F, increased FL signals were obtained by prolonging the incubation time. Co-localized by SYBR Green I (green signals), the red signal of rhodamine in Fig. 1G demonstrated good diffusion within about 50 min. This could be due to the good framework structures of cores for the diffusion of small molecules. This is crucial for the activation of the first logical gate, which relies on the diffusion of GSH into ST-SFNA cores.
Feasibility of cascaded-logical controlled precise drug delivery
Agarose gel electrophoresis was employed for evaluating the formation of ST-SFNA cores and the feasibility for cascaded-logical controlled precise drug delivery. Fig. 2A-i shows the image of different components involved in the synthesis. As demonstrated, the primary products of siRNA-triggered linear polymerization of H1 and H2 (lane 6) moved slower than raw components (lanes 2–4). However, the primary product moved faster than ST-SFNA cores (after branched chain polymerization with H3 and H4) (lane 7). The slowest migration of final g-DNA covered ST-SFNA cores (lane 8) confirmed successful HCR-based synthesis and g-DNA coverage. In addition, the cascaded-logic controlled collapse of frameworks by hybridization with survivin mRNA was further explored by electrophoresis with different stimuli added. As shown in Fig. 2A-ii and S2,† significant bands at low molecular weights were only observed in the presence of GSH, ATP, and survivin (survivin DNA, a DNA analogue of survivin mRNA). This was due to the synergetic cleavage of g-DNAs by GSH and ATP, which subsequently initiated target hybridization combined with framework collapse. This cascaded-logical collapse was further quantified by the turn on FL signals of Cy5 (Fig. 2B) generated by extending the distance of Cy5 from BHQ2.
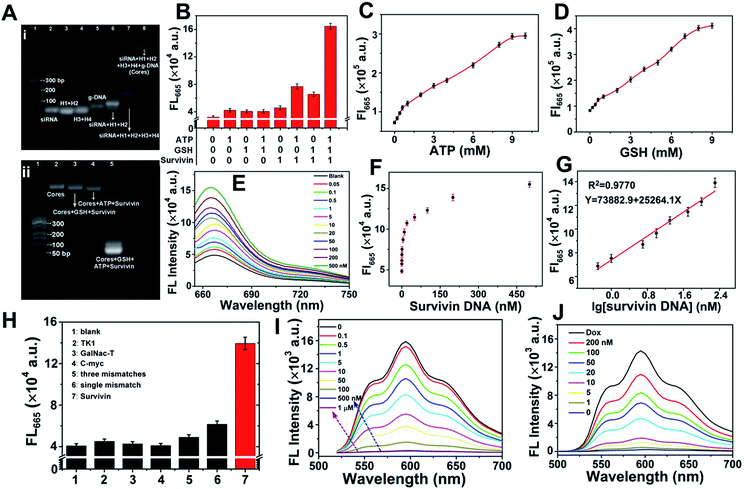 |
| Fig. 2 Evaluations of cascaded-logical controlled drug delivery. (A) Agarose gel electrophoresis images: (i) ST-SFNA core construction. Lanes 1–8: DNA marker, siRNA, H1 + H2, H3+H4, g-DNA, siRNA + H1+H2, ST-FNA cores, and g-DNA covered ST-SFNA cores. (ii) Cascaded-logical responses of ST-SFNA cores. Lane (1): DNA marker; (2): ST-SFNA cores; (3): ST-SFNA cores with added GSH and survivin; (4): ST-SFNA cores with added ATP and survivin; (5): ST-SFNA cores with added GSH, ATP, and survivin. (B) FL responses under all input conditions. (C) FL responses to different concentrations of ATP. GSH: 5 mM; survivin: 200 nM. (D) FL responses to different concentrations of GSH. ATP: 5 mM; survivin: 200 nM. (E) Fluorescence spectra at different concentrations of survivin. (F) FL responses versus survivin concentration. (G) Linear relationship of (F) (0.5 to 200 nM, R2 = 0.9770). (H) Selectivity. (I) FL spectra of Dox (2 μM) loaded into different concentrations of ST-SFNAs in PBS (pH = 7.4). (J) FL spectra of Dox-loaded ST-SFNAs (2 μM) during the release of Dox with different concentrations of survivin. ATP and GSH: 1 mM. | |
In addition, based on Cy5 signals, effective first logical-responses to ATP and GSH from 1 to 10 mM were obtained (Fig. 2C and D). They are feasible for cellular logical applications.30,35,47 The FL signals of Cy5 at 665 nm increased with increasing survivin concentration (Fig. 2E). This signal acted as both the output signal of the first logical response and the input signal of the second logical simulation. The curves of FL intensities versus survivin concentration are shown in Fig. 2F. This resulted in a wide dynamic range from 0.5 to 200 nM (Fig. 2G) at a limit of detection of 0.04 nM (S/N = 3). Furthermore, the significantly higher FL response to survivin compared with other mRNA or mismatched ones (Fig. 2H) demonstrated good selectivity of the developed method. In fact, based on these selective responses to survivin, the loading of Dox into ST-SFNAs (Fig. 2I) as well as the release of Dox from ST-SFNAs (Fig. 2J) can also be conveniently visualized and monitored.
Exploration of the chemotactic behavior of ST-SFNAs
To evaluate the chemotactic behavior of ST-SFNAs by virtue of surface modification with catalase, the movements in response to H2O2 were characterized. As shown in Fig. S4A–C,† H2O2 absorption was negatively correlated with catalase concentration. This was due to the faster consumption of H2O2 at a higher concentration of catalase,48 confirming the concentration-dependent catalytic behavior of ST-SFNAs. Moreover, the responses can also be directly visualized via the generation of bubbles (Fig. S4D†).
Subsequently, the propulsion behavior was assessed and visualized by confocal laser scanning microscopy (CLSM). The trajectories were analyzed with the Chemotaxis and Migration Tool and ImageJ Software. According to the tracking trajectories of ST-SFNAs under different concentrations of H2O2 (Video S1–4†), time-lapse images were displayed (Fig. 3A). As demonstrated, in the presence of the “fuel” H2O2, the “motors” of ST-SFNAs caused directional propulsion instead of random Brownian motion. Additionally, the directionalities of ST-SFNAs also exhibited active propulsive movement at higher H2O2 concentrations (Fig. 3C–E). Brownian motion only occurred in the absence of H2O2 (Fig. 3B). Furthermore, the mean square displacement (MSD) was plotted versus time based on chemotactic movement (Fig. 3F). As demonstrated, typical Brownian motion was obtained with a linear change without H2O2. The average speeds of ST-SFNAs at 3, 6, and 10 mM were calculated to be 13.2, 16.1, and 22.6 μm s−1, respectively (Fig. 3G). The data also matched the positive correlation between chemotactic movement and H2O2 concentrations. In fact, the fluidic distribution of catalase on liposome membranes49 would also synergically promote chemotactic movement. Therefore, the effective chemotactic movement of ST-SFNAs was confirmed, which greatly contributes to the chemotactic penetration and synergistic targeting of cancer cells.
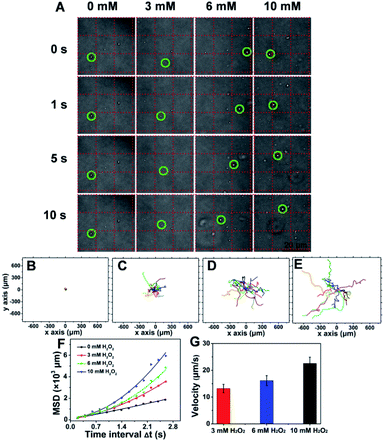 |
| Fig. 3 Chemotactic movement in response to different concentrations of H2O2. (A) Bright field images of ST-SFNAs in the presence of different H2O2 amounts at different times. At different H2O2 concentrations, ST-SFNAs appeared at different positions with different directed propulsive motions. (B–E) Directionality of ST-SFNAs in response to 0, 3, 6, or 10 mM H2O2. (F) MSD of ST-SFNAs and Brownian motions of 15 particles. (G) Velocity of ST-SFNAs. | |
Intracellular exploration of cascaded-logical precise drug delivery and treatments
To evaluate cascaded-logical precise drug delivery and treatment by the present ST-SFNAs, intracellular examinations were employed using HeLa cells as models. Firstly, remarkedly higher cellular uptake of ST-SFNAs was observed compared with those without liposome membrane covers (Fig. S5†). Then, the penetration capability of ST-SFNAs into the deep regions of tumor tissues was explored with 3D HeLa-cell-based multicellular spheroids (MCSs) as an in vitro tumor model.50,51 Two FL signals including those of Cy5 from ST-SFNAs (red) and loaded Dox (yellow) were recorded for these evaluations. Fig. 4A exhibits the CLSM Z-stacking scanning for examining the penetration depth. The worst penetration was obtained without the liposome membrane (cores, Fig. 4A). Without the cover of the liposome membrane, FL signals were mostly located at the periphery of MCSs when the scanning depth exceeded 70 μm. The best penetration depth of 130 μm was observed with both catalase and FA modified on the liposome membrane (ST-SFNAs-(Cat + FA), Fig. 4A). Moreover, this depth was superior to that of single modified ST-SFNAs with catalase (ST-SFNAs–Cat) or FA (ST-SFNAs–FA). Therefore, with liposome membrane covers modified with catalase and FA, effective synergistic targeting delivery into cancer cells or tumor tissues has been confirmed.
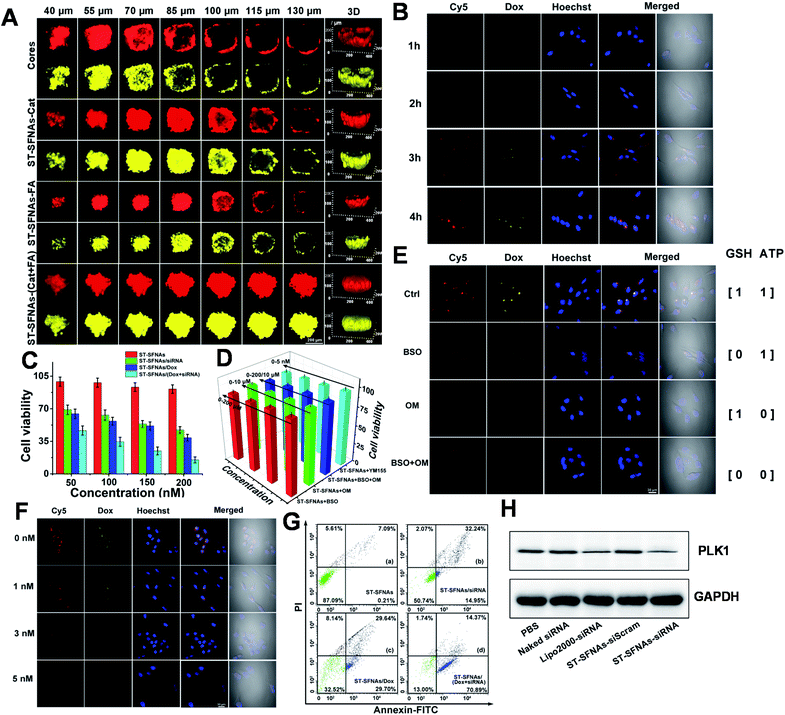 |
| Fig. 4 Intracellular analysis of cascaded-logic circuits for precise drug delivery. (A) CLSM Z-stack scanning of HeLa tumor spheroids after 4 h of treatment with no-lipid covered ST-SFNAs (cores), single modified ST-SFNAs with catalase (ST-SFNAs–Cat), or FA (ST-SFNAs–FA), and present ST-SFNAs with both catalase and FA modified (ST-SFNAs-(Cat + FA)). 3D model of ST-SFNAs showing the corresponding positions of CLSM Z-stacking scanning in the tumor. Red channel: Cy5 from ST-SFNAs; yellow channel: loaded Dox. (B) CLSM images of HeLa cells after incubation with ST-SFNAs at 37 °C for 1–4 h. (C) Viabilities of HeLa cells treated with ST-SFANs without loaded drug, only loaded with siRNA, only loaded with Dox, and loaded with both drugs. (D) Cytotoxicity for cells treated with an exogenous inhibitor and ST-SFANs. BSO: inhibitor of GSH; OM: inhibitor of ATP, YM155: inhibition of ATP. (E) CLSM images of HeLa cells under different first-logical gate conditions (ATP and GSH). Inhibitors: 10 μM OM and 100 μM BSO. ST-SFNAs: 200 nM, 37 °C, and 4 h. (F) CLSM images under different second-logical conditions (survivin mRNA). HeLa cells treated with different concentrations of YM155. ST-SFNAs: 200 nM. (G) Flow cytometry analysis of HeLa cells treated with ST-SFNAs. (a) Without loaded drug, (b) loaded with siRNA, (c) loaded with Dox, and (d) loaded with both drugs. (H) Western blot analysis of PLK1 expressions in HeLa cells treated with different siRNA (anti-PLK1) formulations. ST-SFNAs were modified with both catalase and FA unless otherwise stated. | |
Subsequently, ST-SFNAs were incubated with HeLa cells for cell imaging, which resulted in satisfactory internalization after 4 h (Fig. 4B). In addition, a much lower cell viability was obtained after the treatment with ST-SFNAs loaded with siRNA and Dox (Fig. 4C). This confirmed the killing of HeLa cells via cascaded-logical controlled drug release. Moreover, to examine the treatment selectivity, low levels of GSH, ATP, and survivin mRNA were adjusted using exogenous modulators of L-buthionine sulfoximine (BSO),52,53 oligomycin (OM),54,55 and YM155,56,57 respectively. As illustrated in MTT results (Fig. 4D), under low expressions of these three factors, ST-SFNAs exhibited no obvious influence on cell viability. The failure of cell killing with low expression of logical stimulators (GSH, ATP, and survivin mRNA) also confirmed the cascaded-logical controlled drug delivery. Moreover, the different groups of HeLa cells, with different levels of stimulator expressions on ATP, GSH, and survivin mRNA, were also imaged. As a result, only significant signals of Cy5 (representing survivin mRNA) and Dox were obtained with both ATP an GSH present (output 1) (Fig. 4E). In addition, different concentrations of survivin mRNA in cells can also be imaged. This was proved by the exhibition of decreased FL signals with increasing survivin mRNA inhibitor (YM155) (Fig. 4F). Therefore, cascaded-logical delivery can be achieved and imaged, thus visualizing the controllable and programmable release of drugs for cancer treatments.
Furthermore, the treatment effect of gene silencing by the present ST-SFNAs was examined in a HeLa-GFP cell line, constitutively expressed with Enhanced Green Fluorescent Protein (GFP). As demonstrated by CLSM imaging and flow cytometry, the fluorescent GFP signal decreased after treatment with ST-SFNAs (Fig. S6†), which confirmed the gene silencing effect. Furthermore, the in vitro antitumor performance of these therapeutic ST-SFNAs was extensively evaluated using flow cytometry. As shown in Fig. 4G, a high total apoptotic ratio of about 85.26% was obtained for HeLa cells with both siRNA and Dox loading. In addition, as an oncogenic target for siRNA therapy, polo-like kinase 1 (PLK1) was selected as an effective biomarker for evaluating gene silencing. PLK1 is a key regulator of mitotic progression in mammalian cells and is often overexpressed in cancer cells.58,59 By analysing the total protein extractions from HeLa cells by western blotting, the lowest expression of PLK1 was obtained after the treatment with ST-SFNAs. These results are comparable to traditional Lipo 2000-loading (Fig. 4H). Therefore, the best treatment effect was obtained by loading both siRNA and Dox into ST-SFNAs, which further confirmed the synergistic enhancement of the apoptosis-inducing capability of ST-SFNAs.
In vivo application of ST-SFNAs
Finally, therapeutic evaluation of ST-SFNAs in HeLa xenograft-tumor-bearing mice was carried out via intravenous injection. Firstly, synergistic targeting and chemotactic penetration of ST-SFNAs were evaluated, which were driven by both catalase and FA modified on the surface of ST-SFNAs. Different nanoparticles, including bare ST-SFNAs without any modification as well as ST-SFNAs modified with catalase, FA, and both catalase and FA, were prepared. As shown in Fig. 5A, far clearer signals of tumor accumulation were obtained by the present ST-SFNAs-(Cat–FA) compared with other groups after 6 h of intravenous injections. This result also matches images of different tissues from different mice (Fig. 5B and S7†). Quantitative analyses of tissue signals within a certain area were further employed by using ROI tools. As shown in Fig. 5C, the highest values in tumors were obtained by ST-SFNAs-(Cat–FA), which also confirmed their superior accumulation in tumors. Therefore, surface dual-functionalization ensured the best accumulation at tumor sites, under synergistic targeting in response to H2O2 and FA receptor overexpression on the surface of cancer cells.
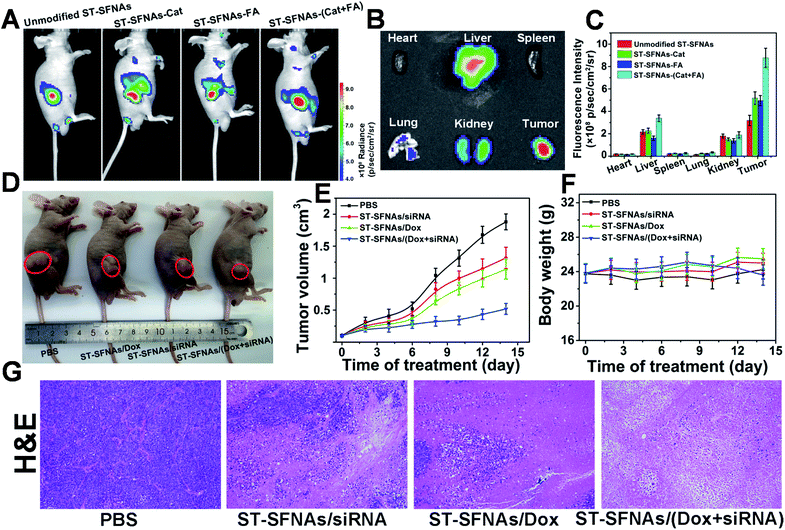 |
| Fig. 5
In vivo explorations on tumor targeting and antitumor activity. (A) Fluorescence image of tumor-bearing mice after the injection of the bare core of ST-SFNAs, catalase, FA, and ST-SFNAs modified with both catalase and FA. (B) Fluorescence images of different tissues. (C) Mean FL signals of tumor and normal tissues. (D) Representative images of mouse xenograft tumor mice treated with PBS, ST-SNAs loaded with siRNA, Dox, and both drugs for 14 days. Injection volume: 200 μL of nanoparticles including 4 mg kg−1 Dox and/or 1 mg kg−1 siRNA. (E) Growth curves of HeLa tumor xenografts in mice after treatments with (1) PBS (control), (2) ST-SFNAs loaded with siRNA, (3) Dox, and (4) Dox and siRNA. (F) Histochemical analyses (H&E) of HeLa tumor tissue after different treatments for 14 days. (G) Body-weight curves of HeLa tumor-bearing mice after different treatments. | |
Subsequently, the in vivo synergistic antitumor effect from ST-SFNA-loaded Dox and siRNA was evaluated in HeLa xenograft tumor-bearing mice. After tumors reached 100–120 mm3, nude mice were randomly divided into four groups, and were treated with PBS, siRNA-loaded, Dox-loaded, and ST-SFNAs loaded with both drugs. After 14 days, the smallest tumor size was obtained in mice treated with Dox and siRNA loaded ST-SFNAs (Fig. 5D). Fig. 5E shows the monitoring of tumor sizes over these 14 days. The good synergistic therapeutic effect was also demonstrated by histopathological examination (H&E) of tumor tissues from different groups (Fig. 5G). Notably, no significant changes in the weights of mice were recorded during the treatments of all groups (Fig. 5F). This demonstrated good biocompatibility of the present ST-SFNAs. In addition, the H&E images of major organs from different groups demonstrated little influence on the tissues of the heart, liver, spleen, lung, and kidney (Fig. S8†). Therefore, with their good biocompatibility, the present ST-SFNAs can precisely deliver drugs for synergistic treatments in vivo.
Conclusions
In summary, templated with siRNA and using hairpin DNA monomers as the main components, spherical core–shell 3D ST-SFNAs were prepared based on linear polymerization followed by branched polymerization of the HCR. Without by-products or useless components, stable 3D spherical frameworks with high spatial utilization were obtained for efficient loading and intracellular delivery. In addition, a cascaded-logical controlled and precise release system was constructed via coverage with g-DNA (with a S–S bond and ATP aptamer). Simultaneously, “turn on” FL signals were generated via hybridization of a special sequence of released hairpin DNAs with survivin mRNA. This made the whole release process visualizable. Furthermore, synergistic chemotactic recognition, accumulation, and membrane fusion into cancer cells were achieved by the outmost catalase and FA-modified liposome membranes. Therefore, with this sophisticated design, the two drugs of Dox and siRNA were efficiently loaded and precisely delivered for synergistic therapy with good biocompatibility. This has generated an efficient FNA-based platform to maximize therapeutic efficacy by comprehensive improvement of loading capacity, chemotactic recognition, cascaded-logical controlled precise release, and synergistic therapy. Inspired by the design and application of this platform, engineering of other biomaterials could also be expanded for wider biological application.
Ethical statement
All animal experimental protocols were reviewed and approved by the Animal Care and Use Committee of Institute of Beijing Normal University and complied with all relevant ethical regulations.
Author contributions
The manuscript was written through contributions of all authors. All authors have given approval to the final version of the manuscript.
Conflicts of interest
There are no conflicts to declare.
Acknowledgements
N. Na, J. Li, Y. Zhang, and J. Sun gratefully acknowledge the financial support provided by the National Natural Science Foundation of China (NNSFC, 21874012) and the National Key Research and Development Program of China (No. 2019YFC1805600). J. Ouyang is grateful for the financial support provided by NNSFC (21974010).
Notes and references
- N. C. Seeman, Annu. Rev. Biochem., 2010, 79, 65–87 CrossRef CAS PubMed.
- J. I. Cutler, E. Auyeung and C. A. Mirkin, J. Am. Chem. Soc., 2012, 134, 1376–1391 CrossRef CAS PubMed.
- D. Shu, Y. Shu, F. Haque, S. Abdelmawla and P. Guo, Nat. Nanotechnol., 2011, 6, 658–667 CrossRef CAS PubMed.
- D. Luo, Adv. Drug Delivery Rev., 2010, 62, 591 CrossRef CAS PubMed.
- G. Zhu, R. Hu, Z. Zhao, Z. Chen, X. Zhang and W. Tan, J. Am. Chem. Soc., 2013, 135, 16438–16445 CrossRef CAS PubMed.
- S. M. Douglas, H. Dietz, T. Liedl, B. Högberg, F. Graf and W. M. Shih, Nature, 2009, 459, 414–418 CrossRef CAS PubMed.
- M. R. Jones, N. C. Seeman and C. A. Mirkin, Science, 2015, 347, 1260901 CrossRef PubMed.
- F. Praetorius and H. Dietz, Science, 2017, 355, eaam5488 CrossRef PubMed.
- K. F. Wagenbauer, C. Sigl and H. Dietz, Nature, 2017, 552, 78–83 CrossRef CAS PubMed.
- Y. Yang, J. Wang, H. Shigematsu, W. Xu, W. M. Shih, J. E. Rothman and C. Lin, Nat. Chem., 2016, 8, 476–483 CrossRef CAS PubMed.
- P. C. Nickels, B. Wünsch, P. Holzmeister, W. Bae, L. M. Kneer, D. Grohmann, P. Tinnefeld and T. Liedl, Science, 2016, 354, 305 CrossRef CAS PubMed.
- Z. Zhang, Y. Yang, F. Pincet, M. C. Llaguno and C. Lin, Nat. Chem., 2017, 9, 653–659 CrossRef CAS PubMed.
- J. Song, Z. Li, P. Wang, T. Meyer, C. Mao and Y. Ke, Science, 2017, 357, eaan3377 CrossRef PubMed.
- F. Zhang, S. Jiang, S. Wu, Y. Li, C. Mao, Y. Liu and H. Yan, Nat. Nanotechnol., 2015, 10, 779–784 CrossRef CAS PubMed.
- S. Liu, G. Chistol, C. L. Hetherington, S. Tafoya, K. Aathavan, J. Schnitzbauer, S. Grimes, P. J. Jardine and C. Bustamante, Cell, 2014, 157, 702–713 CrossRef CAS PubMed.
- G. Kong, M. Xiong, L. Liu, L. Hu, H.-M. Meng, G. Ke, X.-B. Zhang and W. Tan, Chem. Soc. Rev., 2021, 50, 1846–1873 RSC.
- Z. Qing, J. Hu, J. Xu, Z. Zou, Y. Lei, T. Qing and R. Yang, Chem. Sci., 2020, 11, 1985–1990 RSC.
- P. Song, D. Ye, X. Zuo, J. Li, J. Wang, H. Liu, M. T. Hwang, J. Chao, S. Su, L. Wang, J. Shi, L. Wang, W. Huang, R. Lal and C. Fan, Nano Lett., 2017, 17, 5193–5198 CrossRef CAS PubMed.
- S. H. Um, J. B. Lee, N. Park, S. Y. Kwon, C. C. Umbach and D. Luo, Nat. Mater., 2006, 5, 797–801 CrossRef CAS PubMed.
- J. Song, M. Lee, T. Kim, J. Na, Y. Jung, G. Y. Jung, S. Kim and N. Park, Nat. Commun., 2018, 9, 4331 CrossRef PubMed.
- N. Park, S. H. Um, H. Funabashi, J. Xu and D. Luo, Nat. Mater., 2009, 8, 432–437 CrossRef CAS PubMed.
- M. Xiao, W. Lai, F. Wang, L. Li, C. Fan and H. Pei, J. Am. Chem. Soc., 2019, 141, 20354–20364 CrossRef CAS PubMed.
- B. Liu, J. Sun, J. Zhu, B. Li, C. Ma, X. Gu, K. Liu, H. Zhang, F. Wang, J. Su and Y. Yang, Adv. Mater., 2020, 32, 2004460 CrossRef CAS PubMed.
- J. B. Lee, Y. H. Roh, S. H. Um, H. Funabashi, W. Cheng, J. J. Cha, P. Kiatwuthinon, D. A. Muller and D. Luo, Nat. Nanotechnol., 2009, 4, 430–436 CrossRef CAS PubMed.
- J. Tang, C. Yao, Z. Gu, S. Jung, D. Luo and D. Yang, Angew. Chem., Int. Ed., 2020, 59, 2490–2495 CrossRef CAS PubMed.
- R. K. Pathak and S. Dhar, J. Am. Chem. Soc., 2015, 137, 8324–8327 CrossRef CAS PubMed.
- L. Zhang, S. R. Jean, S. Ahmed, P. M. Aldridge, X. Li, F. Fan, E. H. Sargent and S. O. Kelley, Nat. Commun., 2017, 8, 381 CrossRef PubMed.
- X. Gao, S. Li, F. Ding, X. Liu, Y. Wu, J. Li, J. Feng, X. Zhu and C. Zhang, Adv. Mater., 2021, 33, 2006116 CrossRef CAS PubMed.
- F. Li, W. Yu, J. Zhang, Y. Dong, X. Ding, X. Ruan, Z. Gu and D. Yang, Nat. Commun., 2021, 12, 1138 CrossRef CAS PubMed.
- J. Li, C. Zheng, S. Cansiz, C. Wu, J. Xu, C. Cui, Y. Liu, W. Hou, Y. Wang, L. Zhang, I. t. Teng, H.-H. Yang and W. Tan, J. Am. Chem. Soc., 2015, 137, 1412–1415 CrossRef CAS PubMed.
- H. Chu, J. Zhao, Y. Mi, Y. Zhao and L. Li, Angew. Chem., Int. Ed., 2019, 58, 14877–14881 CrossRef CAS PubMed.
- J. Zhao, H. Chu, Y. Zhao, Y. Lu and L. Li, J. Am. Chem. Soc., 2019, 141, 7056–7062 CrossRef CAS PubMed.
- A. Somasundar, S. Ghosh, F. Mohajerani, L. N. Massenburg, T. Yang, P. S. Cremer, D. Velegol and A. Sen, Nat. Nanotechnol., 2019, 14, 1129–1134 CrossRef CAS PubMed.
- B. J. Toebes, F. Cao and D. A. Wilson, Nat. Commun., 2019, 10, 5308 CrossRef PubMed.
- P. Zhang, D. Gao, K. An, Q. Shen, C. Wang, Y. Zhang, X. Pan, X. Chen, Y. Lyv, C. Cui, T. Liang, X. Duan, J. Liu, T. Yang, X. Hu, J.-J. Zhu, F. Xu and W. Tan, Nat. Chem., 2020, 12, 381–390 CrossRef CAS PubMed.
- A. Schudel, A. P. Chapman, M.-K. Yau, C. J. Higginson, D. M. Francis, M. P. Manspeaker, A. R. C. Avecilla, N. A. Rohner, M. G. Finn and S. N. Thomas, Nat. Nanotechnol., 2020, 15, 491–499 CrossRef CAS PubMed.
- C. Wiraja, Y. Zhu, D. C. S. Lio, D. C. Yeo, M. Xie, W. Fang, Q. Li, M. Zheng, M. Van Steensel, L. Wang, C. Fan and C. Xu, Nat. Commun., 2019, 10, 1147 CrossRef PubMed.
- J. Han, Y. Cui, Z. Gu and D. Yang, Biomaterials, 2021, 273, 120846 CrossRef CAS PubMed.
- A. Fischer, S. Lilienthal, M. Vázquez-González, M. Fadeev, Y. S. Sohn, R. Nechushtai and I. Willner, J. Am. Chem. Soc., 2020, 142, 4223–4234 CrossRef CAS PubMed.
- Y. Zhang, Y. Zhang, G. Song, Y. He, X. Zhang, Y. Liu and H. Ju, Angew. Chem., Int. Ed., 2019, 58, 18207–18211 CrossRef CAS PubMed.
- S. Wang, G. Yu, W. Yang, Z. Wang, O. Jacobson, R. Tian, H. Deng, L. Lin and X. Chen, Adv. Sci., 2021, 8, 2002927 CrossRef CAS PubMed.
- J. Zhang, M. He, C. Nie, M. He, Q. Pan, C. Liu, Y. Hu, T. Chen and X. Chu, Chem. Sci., 2020, 11, 7092–7101 RSC.
- X. Gong, R. Li, J. Wang, J. Wei, K. Ma, X. Liu and F. Wang, Angew. Chem., Int. Ed., 2020, 59, 21648–21655 CrossRef CAS PubMed.
- J. Feng, W. Yu, Z. Xu and F. Wang, Chem. Sci., 2020, 11, 1649–1656 RSC.
- J. Liu, X. Lu, T. Wu, X. Wu, L. Han and B. Ding, Angew. Chem., Int. Ed., 2021, 60, 1853–1860 CrossRef CAS PubMed.
- P. Guo, J. Yang, J. Huang, D. T. Auguste and M. A. Moses, Proc. Natl. Acad. Sci. U. S. A., 2019, 116, 18295 CrossRef CAS PubMed.
- R. Mo, T. Jiang and Z. Gu, Angew. Chem., Int. Ed., 2014, 53, 5815–5820 CrossRef CAS PubMed.
- S. Sengupta, K. K. Dey, H. S. Muddana, T. Tabouillot, M. E. Ibele, P. J. Butler and A. Sen, J. Am. Chem. Soc., 2013, 135, 1406–1414 CrossRef CAS PubMed.
- M. M. Baksh, M. Jaros and J. T. Groves, Nature, 2004, 427, 139–141 CrossRef CAS PubMed.
- Y. Wang, S. Luo, Y. Wu, P. Tang, J. Liu, Z. Liu, S. Shen, H. Ren and D. Wu, ACS Nano, 2020, 14, 17046–17062 CrossRef CAS PubMed.
- H.-J. Li, J.-Z. Du, X.-J. Du, C.-F. Xu, C.-Y. Sun, H.-X. Wang, Z.-T. Cao, X.-Z. Yang, Y.-H. Zhu, S. Nie and J. Wang, Proc. Natl. Acad. Sci. U. S. A., 2016, 113, 4164 CrossRef CAS PubMed.
- D. Trachootham, J. Alexandre and P. Huang, Nat. Rev. Drug Discovery, 2009, 8, 579–591 CrossRef CAS PubMed.
- Z. Dong, L. Feng, Y. Chao, Y. Hao, M. Chen, F. Gong, X. Han, R. Zhang, L. Cheng and Z. Liu, Nano Lett., 2019, 19, 805–815 CrossRef CAS PubMed.
- T. K. Hodges, Nature, 1966, 209, 425–426 CrossRef CAS.
- J. Symersky, D. Osowski, D. E. Walters and D. M. Mueller, Proc. Natl. Acad. Sci. U. S. A., 2012, 109, 13961 CrossRef CAS PubMed.
- K. Yamanaka, T. Nakahara, T. Yamauchi, A. Kita, M. Takeuchi, F. Kiyonaga, N. Kaneko and M. Sasamata, Clin. Cancer Res., 2011, 17, 5423 CrossRef CAS PubMed.
- Y. Voges, M. Michaelis, F. Rothweiler, T. Schaller, C. Schneider, K. Politt, M. Mernberger, A. Nist, T. Stiewe, M. N. Wass, F. Rödel and J. Cinatl, Cell Death Dis., 2016, 7, e2410 CrossRef CAS PubMed.
- K. S. Lee, T. R. Burke, J.-E. Park, J. K. Bang and E. Lee, Trends Pharmacol. Sci., 2015, 36, 858–877 CrossRef CAS PubMed.
- G. de Cárcer, S. V. Venkateswaran, L. Salgueiro, A. El Bakkali, K. Somogyi, K. Rowald, P. Montañés, M. Sanclemente, B. Escobar, A. de Martino, N. McGranahan, M. Malumbres and R. Sotillo, Nat. Commun., 2018, 9, 3012 CrossRef PubMed.
Footnote |
† Electronic supplementary information (ESI) available. See DOI: 10.1039/d1sc04249a |
|
This journal is © The Royal Society of Chemistry 2021 |
Click here to see how this site uses Cookies. View our privacy policy here.