DOI:
10.1039/D1SC04146K
(Edge Article)
Chem. Sci., 2021,
12, 14301-14308
Learning the structure–activity relationship (SAR) of the Wittig reaction from genetically-encoded substrates†
Received
28th July 2021
, Accepted 8th October 2021
First published on 21st October 2021
Abstract
The Wittig reaction can be used for late stage functionalization of proteins and peptides to ligate glycans, pharmacophores, and many other functionalities. In this manuscript, we modified 160
000 N-terminal glyoxaldehyde peptides displayed on phage with the Wittig reaction by using a biotin labeled ylide under conditions that functionalize only 1% of the library population. Deep-sequencing of the biotinylated and input populations estimated the rate of conversion for each sequence. This “deep conversion” (DC) from deep sequencing correlates with rate constants measured by HPLC. Peptide sequences with fast and slow reactivity highlighted the critical role of primary backbone amides (N–H) in accelerating the rate of the aqueous Wittig reaction. Experimental measurement of reaction rates and density functional theory (DFT) computation of the transition state geometries corroborated this relationship. We also collected deep-sequencing data to build structure–activity relationship (SAR) models that can predict the DC value of the Wittig reaction. By using these data, we trained two classifier models based on gradient boosted trees. These classifiers achieved area under the ROC (receiver operating characteristic) curve (ROC AUC) of 81.2 ± 0.4 and 73.7 ± 0.8 (90–92% accuracy) in determining whether a sequence belonged to the top 5% or the bottom 5% in terms of its reactivity. This model can suggest new peptides never observed experimentally with ‘HIGH’ or ‘LOW’ reactivity. Experimental measurement of reaction rates for 11 new sequences corroborated the predictions for 8 of them. We anticipate that phage-displayed peptides and related mRNA or DNA-displayed substrates can be employed in a similar fashion to study the substrate scope and mechanisms of many other chemical reactions.
Introduction
Profiling multiple substrates under the same reaction conditions is a cornerstone of mechanistic organic chemistry. Optimization of chemical reactions, discovery of catalytic systems, and mechanistic studies are based on measurements of reaction rates of multiple substrates and conditions, and all these situations need a more efficient way to select the right substrates from a large number of compounds. The data collected in such structure–activity relationship (SAR) studies serve as an essential input for developing mechanistic hypotheses and decision making in the discovery of new reactions, however, measurement of the rates of a plurality of substrates under different reaction conditions one-by-one is time consuming.1 Quantitative analyses starting from the pioneering work of Hammett and co-workers on linear free energy relationships (LFER)2 to modern approaches that employ multiple linear regression (MLR)3 and other machine learning (ML) methods4 permit converting observations from SAR studies to quantitative models that relate reactivity to observable physical properties such as pKa or theoretically calculated parameters such as HOMO/LUMO energies. These models, combined with “qualitative chemical intuition,” allow prediction of optimal conditions and substrates for a particular reaction and provide critical insight into reaction mechanisms. The most valuable input for these models consists of both “positive” and “negative” data (i.e., fast and slow reactions). The same requirements exist in other machine learning fields: sets with positive and negative observations serve as the most effective input for the training of models.5–7 Methods that allow collection of a large unbiased set of reactivity data facilitate building models that minimize the bias that could originate from human decision making such as conscious selection of substrates with anticipated reactivity.
The high-throughput screening (HTS) technique was developed in the early 1990s for the discovery of pharmaceutically valuable molecules.8,9 It was later repurposed for high-throughput acquisition of SAR information. Such HTS approaches have facilitated not only optimization of chemical reactions10 but also discovery of catalytic systems,11–14 and unexpected chemical transformations.9 Screening of a mixture of multiple substrates in one solution15 expands the traditional one-well-one-reaction format.9,16 Multisubstrate screening17,18 can evaluate yields and enantiomeric excesses for multiple substrates converted by a catalyst,19 and determine reaction kinetics and corresponding rate constants of a large number of reactions in a few experiments (340 measurements in 17 experiments),20 and it can be used to discover new transformations. Traditional considerations in multisubstrate approaches are throughput and non-linearity. The complexity of the mixture should be amenable to separation by liquid or gas chromatography (LC or GC) and the concentration of individual substrates should be sufficiently high to permit the analysis by mass-spectrometry (MS). However, if substrates are present at high concentrations, nonlinear effects21 may originate in such mixtures due to the cross-reactivity between multiple substrates and products. Genetically-encoded (GE) peptide libraries9,16 and DNA-encoded small molecule libraries (DEL)22–26 associate the DNA message with each substrate, and this message can be amplified from a single copy number. This amplification makes it possible to perform screening at very low concentrations of substrates. In term low concentration minimizes undesired cross-substrate reactions (Fig. S2†) while conveniently increasing the number of substrates that can be interrogated to 106–1012 scale. In this manuscript, we perform multisubstrate screening using >105 genetically-encoded substrates present in one solution at 10 attomolar/substrate concentration and employ deep-sequencing to monitor the reactions. Such a concentration of substrates minimizes the interaction between the substrates and can be used to collect valuable information to understand the SAR of the Wittig reaction.
Phage display and DEL technology have been successfully applied to discover new reactions.22–24,26–29 A traditional application of DEL or GE-peptide libraries in reaction discovery aims to enrich a rare subset of substrates that exhibit a faster reactivity than the average population. Such an approach can be broadly characterized as a “gain of function” screen. Knowledge emanating from the screenings focused on “gain” in chemical reactivity is insufficient for comprehensive structure–activity models. The problem can be easily resolved if the genetically-encoded screening is modified to identify both “gain” and “loss” of function in chemical reactivity. Here we demonstrate modification of GE-libraries under kinetically-controlled conditions that convert ∼1% of the library and deep-sequencing of the modified population identified both the fast and slow-reacting substrates. We implement such a GE-screening to identify the peptides that increase and decrease the rate of the Wittig reaction in water and then train the dataset use machine learning to predict peptide reactivity.
The Wittig reaction is a versatile and biocompatible carbon–carbon bond forming process; the product of the reaction can serve as a versatile electrophile or dienophile building block, or as a warhead for reversible covalent trapping of biological nucleophiles. The Wittig reaction has been employed to synthesize DNA-encoded libraries and modify phage displayed libraries of aldehyde-peptides.30,31 The substrates for the Wittig reaction—libraries of peptide aldehydes have been generated by NaIO4 oxidation of readily available phage-displayed libraries with N-terminal Ser.32–34 Aldehydes can also be introduced by unnatural amino acid mutagenesis35 or by using formyl glycine generating enzymes.36 The mechanism of the Wittig reaction in water remains a topic of extensive research.37–39 It proceeds through a formal cycloaddition with an early oxaphosphetane (OPA) transition state (TS). The stereo-electronic properties of the aldehydes influence the geometry and energy around the OPA manifold and change the rate and stereo chemical outcome of the Wittig reaction. Peptide aldehyde substrates contain a rich repertoire of functional groups that could potentially stabilize or destabilize the OPA TS. The effect of hydroxyl groups with pKa of 7–15, ammonium ions with pKa 7–12, carboxylates, aromatic rings with different “π-basicity” and different H-bond donors and acceptors on the stability of OPA in water is not obvious. The GE-screen described in this report profiled the effect of a diverse combination of these groups in 105 peptide-aldehydes displayed on phage. The predictions built on these observations illustrated the unique role of primary amides in stabilizing the transition state via short-range non-covalent interactions.
Results and discussion
Wittig reaction in the linear phage library
Multisubstrate profiling of the Wittig reaction repurposed classical tagging strategies employed for physical separation of the reacted subset of library members from the remaining unreacted population.32,40 In particular, successful Wittig reaction between phage-displayed peptide-aldehydes and biotin-tagged ylides introduced biotin into a product. Tagged products can be separated from the unreacted population due to its affinity to streptavidin and analyzed by deep-sequencing. We employed periodate oxidation32 of linear SXXXX libraries displayed on phage41 to produce libraries of aldehyde substrates denoted as CHO–XXXX (Fig. 1a, X denotes any of the 20 amino acids). The resulting library of (20)4 = 160
000 substrates can be characterized completely by Illumina sequencing (Fig. 1).41 Change in the library composition that leads to either enrichment or depletion of substrates in these libraries can be, therefore, reliably quantified.
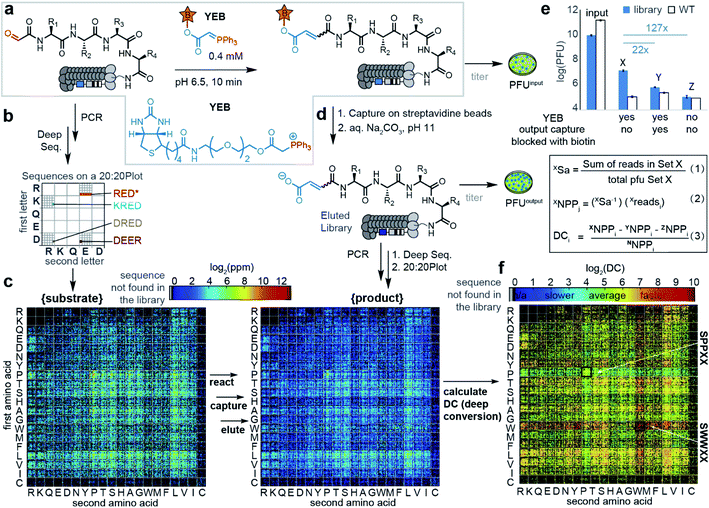 |
| Fig. 1 (a) Wittig reaction on phage libraries. PFU is a plaque-forming unit. (b) Reading of a 20 × 20 plot.41 (c) 20 × 20 plots displaying library-wide DC values of peptide substrates and the Wittig products. Black pixels in the 20 × 20 plot correspond to sequences not observed during sequencing. (d) After reacting for 10 min, the biotinylated Wittig products were captured by streptavidin beads and subjected to sequencing. (e) Pull-down titering data showing higher capture of phage expressing peptides (blue bars) vs. wild type phage (white bars) and higher capture in the 1% biotinylated set (X) than in controls (Y and Z). To calculate the deep conversion of a particular peptide “i” (DCi), we calculate the number of phage particles (NPPi) that display peptides using deep sequencing number of reads (readsi) observed in the deep sequencing and sampling factor (Sa) that relates reads in deep sequencing (readsi) with NPPi. The detailed description of eqn (1)–(3) is provided in Scheme S1.† (f) 20 × 20 plots for SX4 selections. Plots with amino acids at other positions can be found in Fig. S3.† | |
Based on previously reported average rates of the reaction on phage (k = 0.2 M−1 s−1),31 a 10 minute reaction time and 0.4 mM ylide ester biotin (YEB) should convert ∼5% of the population to a biotinylated product (Fig. S2†). Kinetic modeling of the distribution of the products in the multisubstrate reaction predicts that interrupting the reaction at 5% conversion of the aldehyde population and counting the copy number of the biotinylated products make it possible to identify substrates with rates that range from 0.002 M−1 s−1 to 20 M−1 s−1 (Fig. S1d†). Therefore, interruption of the reaction at 1–5% conversion should make it possible to identify substrates that react 100 times faster or 100 times slower than the average substrate. Adding these pre-determined concentrations of YEB to 109 phage virions and quenching with acidic buffer at the specified time intervals, ensured reproducible biotinylation of ∼1% of the population of phage clones.
The pull-down process selects not only the “specific” biotinylated population but also the peptide sequences that bind non-specifically to the components in the system such as protein streptavidin agarose beads. A set of two controls assessed the magnitude of such non-specific binding to be 1–5% of the specific biotin-streptavidin interaction. Recovery of biotinylated peptides by streptavidin blocked biotin-binding sites (set Y, Fig. 1e) and recovery of the unreacted aldehyde-peptide population by streptavidin (set Z, Fig. 1e) was decreased by factors of 22 and 120 when compared to the recovery of the “specific” population (set X, Fig. 1e). We collected these “specific” and control populations in 3–5 independent experiments, and subjected them to PCR and Illumina sequencing. The combined data were used to identify the normalized copy number of the peptides that were biotinylated during the Wittig reaction (Fig. 1d and S2,† all the sequencing data are available in the ESI also as http://VT_unfiltered_Feb.txt).
Fig. 1e and Scheme S1† summarize the calculations of the absolute number of biotinylated particles from sequencing data accounting for factors like the sequencing depth and amount of phage particles in each specific experiment. “Ni” (copy number of the peptide in the naïve library) was critical to account for sequences that were present in a high copy number in naïve libraries but did not react fast in the Wittig reaction. Applying normalization and sampling correction (Sa) to peptide sequences, values denoted as “Deep Conversion” (DC) for over 50
000 peptide aldehyde sequences were generated (all the DC values are available in http://MLinput.txt). The DC values are conceptually related to reactivities of sequences: the higher the DC value, the more reactive is the peptide. We found that the most straightforward approach for observing the relation between the sequence and conversion was by using a library-wide visualization tool referred to as the “20 × 20 plot” used in our previous studies (Fig. 1f and S3†).41 To illustrate the importance of normalization in calculation of DC values, we noted that biotin-tagged SPPXX sequences were observed in high copy numbers in captured population and they can be mistakenly interpreted as “most reactive” substrates (Fig. 1c). On the other hand, these sequences were also present in high copy numbers in the naïve library (Fig. 1c) and the introduction of normalization predicted that SPPXX sequences in fact have the lowest DC values in the Wittig reaction (Fig. 1f).
To confirm that the observations predicted by high and low deep conversion (DC) indeed correlated with experimentally determined reactivities of peptide aldehydes, we selected a series of peptides predicted to be “fast”, “medium” and “slow”, synthesized them and validated the reaction rate (Fig. S4–S6†). The results showed an agreement between the experimentally determined conversion measured by HPLC and DC (Fig. 2) in the range of two orders of magnitude for both values. In particular, we reported previously that the average rate for the library of peptide aldehydes displayed on phage was 0.23 ± 0.09 M−1 s−1.31 This rate constant was similar to the rate constant for “average” synthetic peptide sequences that did not contain Trp or Pro residues in the first two positions (Fig. 2a). In contrast, synthetic aldehydes HCO–PPLA and HCO–PPPL exhibited rates of 0.017 ± 0.005 and 0.014 ± 0.03 M−1 s−1 respectively. These sequences were up to 13–16-fold slower than that of the average population. This observation was in line with the observed decrease in DC for SPPXX sequences highlighted by using the 20 × 20 plot (Fig. 1f). To test the effect of proline in a specific position, we systematically evaluated the reactivity of HCO–PPPA, HCO–PPAA, HCO–PAAA, HCO–APAA and HCO–AAAP sequences (Fig. 2a and S9,† all the peptide traces can be found in Fig. S31–S50†). Replacing either the 1st or 2nd amino acid with proline leads only to a modest 2–3 fold decrease in the rate whereas simultaneous replacement of the 1st and 2nd amino acids with prolines resulted in a 10-fold decrease. Subsequent introduction of proline in the 3rd or 4th position had little additional effect on the rate of the Wittig reaction. In analysis of HCO–PXXX sequences, we observed a known side reaction that consumed HCO–PXXX aldehydes via proline-assisted 6-exo-trig attack of an amide nitrogen on the aldehyde (Fig. S10†).42 In HPLC assay, the rate of the Wittig reaction for HCO–PXXX was measured to be 0.12–0.17 M−1 s−1 and the rates of cyclization were in a similar range. Combination of both processes as can be seen in the kinetic equation in Fig. 2b,contributed to an apparent decrease in DC. We also examined the factors that led to an increase in DC and focused on peptides with tryptophan in the first and second positions. The introduction of only one tryptophan in peptides HCO–QWLH, HCO–WIVR, HCO–HWFP, HCO–LWYR and HCO–WLPR (Fig. 2a) had no statistically significant increase from average reactivity, whereas sequences HCO–WWPQ and HCO–WWGL with two tryptophan residues in both positions, exhibited significant increases from the average rate and more than 50-fold increase from the lowest rate in the population. These results suggested that the first and second N-terminal amino acids play a critical synergistic role in the rate of the Wittig reaction, possibly by stabilizing or destabilizing the OPA TS. These observations also confirmed that DC values serve as a good predictive surrogate for fast and slow reactions.
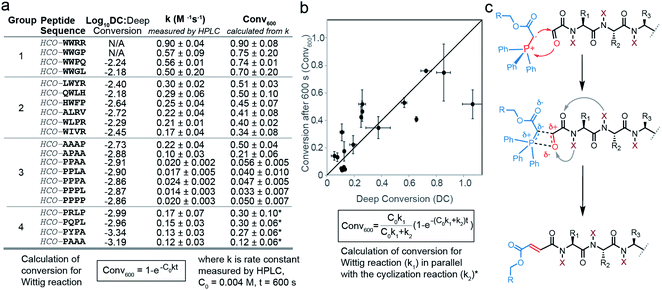 |
| Fig. 2 (a) Deep conversion (DC), rate constants measured by HPLC and conversion at 600 seconds (Conv600). Sequences marked as “N/A” in the Log10 DC column were not detected in the naïve library but were predicted by bioinformatics analysis of the sequencing data, and all other sequences came from the selection. The group and order are based on families of peptide sequences. Group 1 contains fast reacting sequences that begin with WW, group 2 contains average reacting sequences ordered by the decreasing reaction rate, group 3 contains slow reacting sequences which are sorted by increased prevalence of Pro (P) (from one to two, three and four Pro residues) and group 4 contains sequences that exhibit 6-exo-trig side reactions in addition to the Wittig reaction. (b) Plot of DC vs. Conv600. Calculations of the conversion from experimentally measured rate constants. (c) Reaction mechanism of the Wittig reaction in peptides. | |
Investigation of the transition states of the Wittig reaction
Proline, unlike all other proteogenic amino acids forms no intramolecular hydrogen bonds between the amide hydrogen and carbonyl groups nearby (Fig. 2c and S9†). Another piece of evidence for the role of backbone N–H emanated from measurement of the rates of the Wittig reaction with di-alanine and di-Sarcosine oxaloyl aldehydes (Fig. 3c). An HCO–Sarcosine–Sarcosine aldehyde devoid of backbone N–H exhibited a 5-fold decrease in reactivity when compared to isomeric HCO–Ala–Ala aldehyde. To test further whether backbone primary amide contributes to stabilization of the transition state, we performed DFT calculations of the transition state geometry using the Gaussian 09 software43 and B3LYP 6-31G(d) basis set. Due to the conformational flexibility and complexity of oxyclic tetrapeptides and without access to a putative starting structure for the TS, the TS could not be located for the full tetrapeptide. On the other hand, the TS geometries of simpler model dipeptides such as HCO–Ala–Ala and HCO–Sarcosine–Sarcosine and a simple ylide CH3OCO(CH2)–P+Ph3 were successfully optimized using DFT; further details on the computational methods are provided in the ESI.† Similar to the previous calculations of the TS of the Wittig reaction for stabilized ylides, we observed two transition states and TS1 exhibits a higher energy barrier than TS2 (Fig. S11†).37,38 We focused on the geometry and relative energies of TS1 to understand the preferences in the rate limiting step of these reactions. In the optimized geometry of TS1 of HCO–Ala–Ala, we observed two intramolecular hydrogen bonds between the backbone N–H and two carbonyls of the oxaloyl (Fig. 3a). The analysis shown in Fig. 3 is focused on the E-isomer of TS1 because both DFT calculations and experiments favor formation of the E Wittig product (Fig. S11†). In TS1 of HCO–Sarcosine–Sarcosine, N-methylated backbone amides were unable to form such interactions and showed 10 kcal mol−1 higher than the HCO–Ala–Ala TS1 (Fig. 3b). As these two TS1 have the same atomic composition, the difference in energy interactions can be neglected. Thus, the energy barrier of HCO–Ala–Ala TS1 can be attributed to the stabilization of HCO–Ala–Ala TS1 by two hydrogen bonds. Experimental measurements of the rate constants of the reaction between HCO–Ala–Ala, HCO–Sarcosine–Sarcosine and ylide CH3OCOCH2PPH3, corroborated the results from computations (Fig. 3c). We were also able to obtain TS1 and TS2 for HCO–Pro–Pro and HCO–Trp–Trp substrates and observed a similar role of backbone amides in TS1 (Fig. S12 and S13†).
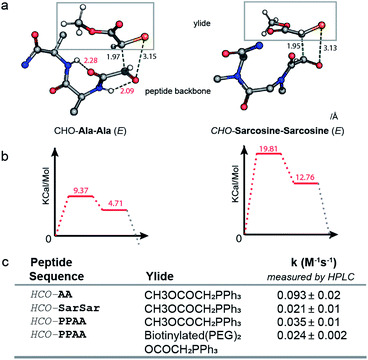 |
| Fig. 3 (a) Geometries of E Ala–Ala and Sarcosine–Sarcosine rate determined transition states (TS1) in gas (B3LYP 6-31G(d)). First and second amides formed intramolecular hydrogen bonds and distances are labelled in red. Distances in OPA are labelled in black. (b) Energy gap of E Ala–Ala and Sarcosine–Sarcosine transition states, and TS1 is selected as the rate-limiting step. (c) Model peptide rate constants measured by HPLC. | |
To determine whether the change in the rate of the Wittig reaction was due to the influence of amino acid on the OPA TSs or the inherent reactivity of substrates towards any nucleophilic attack, we measured the rate of the reaction of HCO–PPAA and HCO–WWRR sequences with 2,4-dinitrophenylhydrazine. To our surprise, the reactivity for hydrazine ligation was reversed (Fig. S14 and S15†) and HCO–PPAA reacted at least 2 times faster than HCO–WWRR both at pH 0 and pH 5. These results (Fig. S16 and S17†) demonstrate that the increase in reactivity is not due to the inherent increase of the electrophilicity of the substrate but due to reaction-specific effects such as the geometry and the relative energy of the TS.
DFT calculations showed lower energies of TS1-E barriers when compared to the TS1-Z configuration for all calculated substrates (Fig. S11†). HPLC and NMR analysis confirmed that the E product was favoured over Z for HCO–Ala–Ala (E/Z 4.5
:
1) and HCO–Sarcosine–Sarcosine (E/Z 3.6
:
1) (Fig. S21 and S22†). We note that the selection process was not designed to select sequences that react stereoselectively. Still, we noticed interesting changes in E/Z selectivity in the Wittig reaction of “fast” (CHO–WWRR 1
:
1 E/Z), “medium” (CHO–HWFP 1
:
9 E/Z) and “slow” (CHO–PPAA 4.5
:
1 E/Z ) reacting sequences (Fig. S18–S20†). These results provide additional evidence that the side chains of the amino acids and backbone amides might exert different influences on the E-OPA vs. Z-OPA transition states.
Application to the cyclic library
Applying the conditions (10 min. reaction, 0.4 mM [YEB]) that convert 1% of the population to another library SXCX3C, we found that the effect of amino-acid residues on conversion was significantly attenuated when compared to the SX4 library (Fig. S23 and S24,† all the DC values are available in http://SXCXXXC.csv). These results were not surprising because the previous observations suggested that synergistic contributions from the amino acids at both positions 1 and 2 are critical to change the reactivity. However, in the SXCX3C library the synergy between position 1 and 2 is not possible because this library contains a constant cysteine at the second position. As in SX4, the presence of proline in the first, but not the third position led to a decrease of DC due to proline-assisted 6-exo-trig attack of amide nitrogen on aldehyde to afford intramolecular cyclization.42 In the SXCX3C library, tryptophan and tyrosine in the first (but not the third) position lead to a modest increase in conversion. The synthesis and testing of five sequences confirmed that the placement of Tyr in the first position led to a detectable increase in the rate (Fig. S23f†) whereas peptides with Tyr in the third position exhibited average rates similar to the average rate of peptides from the SX4 library. Modification of the SXCX3C library was not useful for identification of new sequences with low or high reactivity; still, a uniform reactivity of such a library is an advantage. Such a library can be considered as an example of library in which majority of the members exhibit the same reactivity towards one chemical modification.
Machine learning using DC values to predict non-observed sequences
Analysis by deep-sequencing made it possible to observe 60
432 of the 160
000 possible tetramer amino acid sequences. Measurement of the rates of the remaining 99
568 sequences was not possible for multiple reasons, such as low or unreliable count of these peptide sequences in the naïve population. We sought to extrapolate the reactivity of the “missing” 99
568 peptides using a machine learning model trained using the data from the “observed” 60
432 sequences. As a proof-of-principle, we trained a classification model. The experimentally observed data were split into ‘HIGH’ or ‘LOW’ deep conversion subgroups where HIGH and LOW are defined by the highest and lowest 5% of the DC values obtained by experimental observation (Fig. S26†). As sequences with single Pro in the first position exhibit a side-reaction, these sequences were not used for training. Using XGBoost44 (gradient boosted decision trees) (Fig. S27†), we trained two binary classifiers: one to identify sequences belonging to the HIGH subgroup and the other to identify sequences belonging to the LOW subgroup (Fig. S28,† all the prediction probabilities are available in http://Predictions-160-Probabilities.csv). The input features included the quantitative chemical properties of each of the amino acids in the tetramer sequences, namely z-scale45 descriptors (3 × 4 AA position = 12 features), VHSE46 (vectors of hydrophobic, steric, and electronic properties) descriptors (8 × 4 AA position = 32 features), and sequence patterns based on permutations of 20 amino acids among 4 positions within the tetramer sequences (2396 features) to yield a total of 2440 features (more details about features can be found in the ESI† section titled “Feature Engineering”). Evaluation of respective classifiers showed the area under the receiver operating characteristic curve (ROC AUC) scores of 81.2 ± 0.4 and 73.7 ± 0.8 for HIGH and LOW, using 5-fold stratified cross-validation. It also showed F1-scores of 33.7 ± 0.9 and 19.0 ± 0.9 for the respective classifiers (Table S4†). We deployed the learned models into a publicly available web app (http://44.226.164.95/, Fig. S29†) which allows users to compute the probability of sequences belonging to both the HIGH and LOW class. Using this model, we predicted the class labels of 99
568 amino acid sequences not observed in sequencing. Fig. 4 compares two 20 × 20 plots, one shows the experimentally measured labels for 60
432 sequences (Fig. 4a) and the other shows predicted labels for peptides never observed in deep sequencing (Fig. 4b). The learned models (Fig. 4b) suggest definitive patterns of reactivity that were not clearly observed in the scarce experimental data (Fig. 4a). 8 peptides predicted from machine learning (5 predicted fast and 3 predicted slow) were synthesized and the experimental reaction rates were tested (Table S5†). From three predicted slow peptides (sequences do not initiate with PP), all three showed slower experimental reaction rates than 6 predicted fast peptides, two were faster than the average, two exhibited average reactivity, and interestingly one exhibited a complete class switch, and the rate of the Wittig reaction of CHO–RYIP was the slowest of all tested sequences (0.003 M−1 s−1). It is possible that the model cannot reliably predict the reactivity of motifs with N-terminal Arg because they are censored from naïve libraries.41
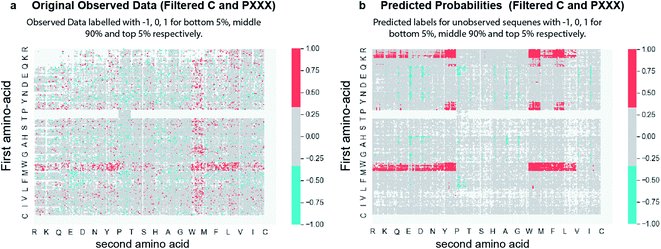 |
| Fig. 4 (a) 20 × 20 plots showing original observed data with class labels for HIGH (top 5%), MEDIUM (middle 90%) and LOW (bottom 5%) as 1, 0, and −1 respectively. Sequences not observed experimentally are shown in white. (b) 20 × 20 plot showing the predicted labels of 99 568 non-observed sequences with 1, 0, and −1 corresponding to HIGH, MEDIUM, and LOW, respectively (Fig. S30†). 60 432 sequences from original observed data were omitted from this plot (showed as white pixels). Red points denote peptides with high probability of the ‘fast’ label, while blue denotes peptides with high probability of the ‘slow’ label. Data for PXXX sequences but not PPXX were excluded from training and predictions on purpose due to side reactions in these sequences. | |
Conclusions
In conclusion, a genetically encoded library of >105 phage-displayed peptide aldehydes provided a rich dataset guiding SAR studies for the Wittig reaction in water with a stabilized ylide (YEB). The study highlighted the cooperative effect of the first and second N-terminal amino acids on the rate of the reaction. Low reactivity of the PP motif highlights the role of backbone amides in stabilization of the TS of the Wittig reaction. DFT computations corroborated the experimental observations and suggested intramolecular hydrogen bonds with backbone amides as an important stabilization factor for the transition state of the Wittig reaction. The 50-fold dynamic range of the reaction rate suggested a 3 kcal mol−1 contribution to the Wittig TS from the peptide and a large fraction of this contribution emanates from the backbone amides.
Coupling of the deep sequencing methodology to investigation of chemical reactivity and SAR studies with only minor changes can be applied to investigate other M13 virion compatible reactions already known to be compatible with the M13 virion (thiol SN2,47–50 thiol SNAr,51 oxime ligation,32,52 copper catalyzed azide alkyne cycloaddition,53 copper-free azide alkyne cycloaddition,54 and Diels Alder cycloaddition31). These investigations, in principle would follow the same experimental design: (i) couple the reactive group to biotin; (ii) terminate the reaction at 1–5% conversion and (iii) sequence the reactive (biotinylated) population. This approach can also illustrate the SAR of other reactions that have potential to be applied on phage such as multicomponent reactions compatible with aqueous conditions55 or chemistry that has already been explored on proteins, unprotected peptides and other display platforms34,40,56–58 As chemical modification of DNA and RNA-displayed libraries is also possible, the SAR of reactions that modify these libraries could be investigated in a similar fashion. In principle, peptide libraries combined with calibrated mass spectrometry analysis,59 could permit analogous SAR analysis, however, the dynamic range of the mass-spectrometry instrument should be sufficient to quantify peptides both enriched and depleted in the reaction.
The SAR data sets produced by deep-sequencing are sufficiently large for training of machine learning models. As a proof-of-principle example, we trained a classification model and attained a respectable accuracy as determined by cross-validation in a held-out dataset. Most importantly, testing of ML models has to extend beyond simple evaluation of accuracy in a cross-validation. Experimental data showed that ML predictions are more reliable on slow reacting peptides. We envision several important next steps in the application of ML approaches to such datasets: (i) replacing peptide-centric descriptors with all-atomic molecular descriptors will make it possible to extrapolate the reactivity of the non-peptide structure from a peptide dataset; (ii) training of quantitative regression models in place of classification will make it possible to predict the structure with higher or lower reactivity than any of the experimentally observed structures.
Data availability
The datasets supporting this article have been uploaded as part of the ESI.† All the MATLAB scripts are available at SupplementaryData.rar. The deep sequencing data with DNA reads, raw counts can be found in VT_unfiltered_Feb.txt, and were uploaded to http://48hd.cloud/ server with an unique alphanumeric name (e.g., 20170829-09WIooPA-VT) and an unique static URL can be found in the ESI Section 3.2.† All the Gaussian output files are available in SupplementaryData.rar/DFT calculation. Machine learning algorithm info is available at https://github.com/derdalab/GESAR.
Author contributions
V. T. performed synthesis of chemical and biochemical reagents, modification, selection, and analysis of the phage display libraries. K. Y. performed synthesis, and modification of peptides. S. M. and S.·V. K. designed and implemented the machine learning algorithm to produce the machine learned model. S. V. K. developed the prediction web app. K. Y. and K. A.-D., performed the DFT computation. R. D., V. T., K. Y. and S. M. wrote the manuscript, edited the final manuscript and contributed intellectual and strategic input. All authors approved the final manuscript.
Conflicts of interest
There are no conflicts to declare.
Acknowledgements
The authors acknowledge funding from NSERC (RGPIN-2016-402511 to R. D.), Amii (Alberta Machine Intelligence Institute (to R. G. and S. V. K.) and NSERC Accelerator Supplement (to R. D.). Infrastructure support was provided by CFI New Leader Opportunity (to R. D.). We thank Dr Ryan T. McKay at the University of Alberta NMR spectrometry facility, and Dr Randy Whittal and Béla Reiz at the University of Alberta mass spectrometry facility.
Notes and references
- M. T. Reetz, Angew. Chem., Int. Ed., 2002, 41, 1335–1338 CrossRef CAS PubMed.
- L. P. Hammett, Chem. Rev., 1935, 17, 125–136 CrossRef CAS.
- T. Wang, M.-B. Wu, J.-P. Lin and L.-R. Yang, Expert Opin. Drug Discovery, 2015, 10, 1283–1300 CrossRef CAS PubMed.
- C. B. Santiago, J.-Y. Guo and M. S. Sigman, Chem. Sci., 2018, 9, 2398–2412 RSC.
- P. S. Kutchukian, J. F. Dropinski, K. D. Dykstra, B. Li, D. A. DiRocco, E. C. Streckfuss, L.-C. Campeau, T. Cernak, P. Vachal, I. W. Davies, S. W. Krska and S. D. Dreher, Chem. Sci., 2016, 7, 2604–2613 RSC.
- A. R. Katritzky, V. S. Lobanov and M. Karelson, Chem. Soc. Rev., 1995, 24, 279–287 RSC.
- K. C. Harper, E. N. Bess and M. S. Sigman, Nat. Chem., 2012, 4, 366–374 CrossRef CAS PubMed.
- B. A. Bunin, M. J. Plunkett and J. A. Ellman, Proc. Natl. Acad. Sci. U. S. A., 1994, 91, 4708–4712 CrossRef CAS PubMed.
- K. D. Collins, T. Gensch and F. Glorius, Nat. Chem., 2014, 6, 859–871 CrossRef CAS PubMed.
- J. A. Selekman, J. Qiu, K. Tran, J. Stevens, V. Rosso, E. Simmons, Y. Xiao and J. Janey, Annu. Rev. Chem. Biomol. Eng., 2017, 8, 525–547 CrossRef PubMed.
- D. D. Devore and R. M. Jenkins, Comments Inorg. Chem., 2014, 34, 17–41 CrossRef CAS.
- G. Gasparini, M. Dal Molin and L. J. Prins, Eur. J. Org. Chem., 2010, 2010, 2429–2440 CrossRef.
- M. T. Reetz, Angew. Chem., Int. Ed., 2001, 40, 284–310 CrossRef CAS PubMed.
- M. T. Reetz, Angew. Chem., Int. Ed., 2008, 47, 2556–2588 CrossRef CAS PubMed.
- D. W. Robbins and J. F. Hartwig, Science, 2011, 333, 1423–1427 CrossRef CAS PubMed.
- Y. Maeda, O. V. Makhlynets, H. Matsui and I. V. Korendovych, Annu. Rev. Biomed. Eng., 2016, 18, 311–328 CrossRef CAS PubMed.
- X. Gao and H. B. Kagan, Chirality, 1998, 10, 120–124 CrossRef CAS.
- C. Gennari, S. Ceccarelli, U. Piarulli, C. A. G. N. Montalbetti and R. F. W. Jackson, J. Org. Chem., 1998, 63, 5312–5313 CrossRef CAS.
- H. Kim, G. Gerosa, J. Aronow, P. Kasaplar, J. Ouyang, J. B. Lingnau, P. Guerry, C. Farès and B. List, Nat. Commun., 2019, 10, 770 CrossRef PubMed.
- M. R. anderHeiden, H. Plenio, S. Immel, E. Burello, G. Rothenberg and H. C. J. Hoefsloot, Chem.–Eur. J., 2008, 14, 2857–2866 CrossRef CAS PubMed.
- T. Satyanarayana, S. Abraham and H. B. Kagan, Angew. Chem., Int. Ed., 2009, 48, 456–494 CrossRef CAS PubMed.
- M. W. Kanan, M. M. Rozenman, K. Sakurai, T. M. Snyder and D. R. Liu, Nature, 2004, 431, 545–549 CrossRef CAS PubMed.
- M. M. Rozenman, M. W. Kanan and D. R. Liu, J. Am. Chem. Soc., 2007, 129, 14933–14938 CrossRef CAS PubMed.
- Y. Chen, A. S. Kamlet, J. B. Steinman and D. R. Liu, Nat. Chem., 2011, 3, 146–153 CrossRef CAS PubMed.
- K. D. Hook, J. T. Chambers and R. Hili, Chem. Sci., 2017, 8, 7072–7076 RSC.
- A. I. Chan, L. M. McGregor and D. R. Liu, Curr. Opin. Chem. Biol., 2015, 26, 55–61 CrossRef CAS PubMed.
- F. Tanaka, R. Fuller, L. Asawapornmongkol, A. Warsinke and S. Gobuty, Bioconjugate Chem., 2007, 18, 1318–1324 CrossRef CAS PubMed.
- G. M. Eldridge and G. A. Weiss, Bioconjugate Chem., 2011, 22, 2143–2153 CrossRef CAS PubMed.
- R. K. V. Lim, N. Li, C. P. Ramil and Q. Lin, ACS Chem. Biol., 2014, 9, 2139–2148 CrossRef CAS PubMed.
- B. N. Tse, T. M. Snyder, Y. Shen and D. R. Liu, J. Am. Chem. Soc., 2008, 130, 15611–15626 CrossRef CAS PubMed.
- V. Triana and R. Derda, Org. Biomol. Chem., 2017, 15, 7869–7877 RSC.
- S. Ng, M. R. Jafari, W. L. Matochko and R. Derda, ACS Chem. Biol., 2012, 7, 1482–1487 CrossRef CAS PubMed.
-
S. Ng, K. F. Tjhung, B. M. Paschal, C. J. Noren and R. Derda, in Peptide Libraries: Methods and Protocols, ed. R. Derda, Springer New York, New York, NY, 2015, pp. 155–172 Search PubMed.
- S. Ng, M. R. Jafari and R. Derda, ACS Chem. Biol., 2012, 7, 123–138 CrossRef CAS PubMed.
- L. Wang, A. Brock, B. Herberich and P. G. Schultz, Science, 2001, 292, 498–500 CrossRef CAS PubMed.
- I. S. Carrico, B. L. Carlson and C. R. Bertozzi, Nat. Chem. Biol., 2007, 3, 321–322 CrossRef CAS PubMed.
- R. Robiette, J. Richardson, V. K. Aggarwal and J. N. Harvey, J. Am. Chem. Soc., 2005, 127, 13468–13469 CrossRef CAS PubMed.
- R. Robiette, J. Richardson, V. K. Aggarwal and J. N. Harvey, J. Am. Chem. Soc., 2006, 128, 2394–2409 CrossRef CAS PubMed.
- P. A. Byrne and D. G. Gilheany, Chem. Soc. Rev., 2013, 42, 6670–6696 RSC.
- C. P. Ramil and Q. Lin, Chem. Commun., 2013, 49, 11007–11022 RSC.
- B. He, K. F. Tjhung, N. J. Bennett, Y. Chou, A. Rau, J. Huang and R. Derda, Sci. Rep., 2018, 8, 1214 CrossRef PubMed.
- K. Rose, J. Chen, M. Dragovic, W. Zeng, D. Jeannerat, P. Kamalaprija and U. Burger, Bioconjugate Chem., 1999, 10, 1038–1043 CrossRef CAS PubMed.
-
M. J. Frisch, G. W. Trucks, H. B. Schlegel, G. E. Scuseria, M. A. Robb, J. R. Cheeseman, G. Scalmani, V. Barone, B. Mennucci, G. A. Petersson, H. Nakatsuji, M. Caricato, X. Li, H. P. Hratchian, A. F. Izmaylov, J. Bloino, G. Zheng, J. L. Sonnenberg, M. Hada, M. Ehara, K. Toyota, R. Fukuda, J. Hasegawa, M. Ishida, T. Nakajima, Y. Honda, O. Kitao, H. Nakai, T. Vreven, A. Montgomery Jr, J. E. Peralta, F. Ogliaro, M. Bearpark, J. J. Heyd, E. Brothers, K. N. Kudin, V. N. Staroverov, R. Kobayashi, J. Normand, K. Raghavachari, A. Rendell, J. C. Burant, S. S. Iyengar, J. Tomasi, M. Cossi, N. Rega, J. M. Millam, M. Klene, J. E. Knox, J. B. Cross, V. Bakken, C. Adamo, J. Jaramillo, R. Gomperts, R. E. Stratmann, O. Yazyev, A. J. Austin, R. Cammi, C. Pomelli, J. W. Ochterski, R. L. Martin, K. Morokuma, V. G. Zakrzewski, G. A. Voth, P. Salvador, J. J. Dannenberg, S. Dapprich, A. D. Daniels, Ö. Farkas, J. B. Foresman, J. V. Ortiz, J. Cioslowski and D. J. Fox, Gaussian 09 (Revision A.02), Gaussian, Inc., Wallingford CT, 2016 Search PubMed.
-
T. Chen and C. Guestrin, Proceedings of the 22nd acm sigkdd international conference on knowledge discovery and data mining, 2016, pp. 785–794 Search PubMed.
- C. Ge, E. Spånning, E. Glaser and Å. Wieslander, Mol. Plant, 2014, 7, 121–136 CrossRef CAS PubMed.
- J. Xie, Z. Xu, S. Zhou, X. Pan, S. Cai, L. Yang and H. Mei, PLoS One, 2013, 8, e74506 CrossRef CAS PubMed.
- S. Li and R. W. Roberts, Chem. Biol., 2003, 10, 233–239 CrossRef CAS PubMed.
- M. R. Jafari, L. Deng, P. I. Kitov, S. Ng, W. L. Matochko, K. F. Tjhung, A. Zeberoff, A. Elias, J. S. Klassen and R. Derda, ACS Chem. Biol., 2014, 9, 443–450 CrossRef CAS PubMed.
- S. Chen, J. Morales-Sanfrutos, A. Angelini, B. Cutting and C. Heinis, ChemBioChem, 2012, 13, 1032–1038 CrossRef CAS PubMed.
- S. Ng and R. Derda, Org. Biomol. Chem., 2016, 14, 5539–5545 RSC.
- S. Kalhor-Monfared, M. R. Jafari, J. T. Patterson, P. I. Kitov, J. J. Dwyer, J. M. Nuss and R. Derda, Chem. Sci., 2016, 7, 3785–3790 RSC.
- Z. M. Carrico, M. E. Farkas, Y. Zhou, S. C. Hsiao, J. D. Marks, H. Chokhawala, D. S. Clark and M. B. Francis, ACS Nano, 2012, 6, 6675–6680 CrossRef CAS PubMed.
- F. Tian, M.-L. Tsao and P. G. Schultz, J. Am. Chem. Soc., 2004, 126, 15962–15963 CrossRef CAS PubMed.
- T. Urquhart, E. Daub and J. F. Honek, Bioconjugate Chem., 2016, 27, 2276–2280 CrossRef CAS PubMed.
- M. C. Pirrung and K. D. Sarma, J. Am. Chem. Soc., 2004, 126, 444–445 CrossRef CAS PubMed.
- R. J. Spears and M. A. Fascione, Org. Biomol. Chem., 2016, 14, 7622–7638 RSC.
- R. A. Goodnow, C. E. Dumelin and A. D. Keefe, Nat. Rev. Drug Discovery, 2017, 16, 131–147 CrossRef CAS PubMed.
- J. R. Frost, J. M. Smith and R. Fasan, Curr. Opin. Struct. Biol., 2013, 23, 571–580 CrossRef CAS PubMed.
- C. Zhang, M. Welborn, T. Zhu, N. J. Yang, M. S. Santos, T. Van Voorhis and B. L. Pentelute, Nat. Chem., 2016, 8, 120–128 CrossRef CAS PubMed.
Footnote |
† Electronic supplementary information (ESI) available. See DOI: 10.1039/d1sc04146k |
|
This journal is © The Royal Society of Chemistry 2021 |
Click here to see how this site uses Cookies. View our privacy policy here.