DOI:
10.1039/D1SC03898B
(Edge Article)
Chem. Sci., 2021,
12, 13940-13948
Computational maturation of a single-domain antibody against Aβ42 aggregation†
Received
28th July 2021
, Accepted 23rd August 2021
First published on 7th October 2021
Abstract
The expansion of structural databases and the increase in computing power are enabling approaches for antibody discovery based on computational design. It has already been shown that it is possible to use this approach to generate antibodies for specific epitopes on challenging targets. Here we describe an application of this procedure for antibody maturation through the computational design of mutational variants of increased potency. We illustrate this procedure in the case of a single-domain antibody targeting an epitope in the N-terminal region of Aβ42, a peptide whose aggregation is closely associated with Alzheimer's disease. We show that this approach enables the generation of an antibody variant with over 200-fold increased potency against the primary nucleation process in Aβ42 aggregation. Our results thus demonstrate that potentiated antibody variants can be obtained by computational maturation.
Introduction
Antibodies are increasingly common diagnostic and therapeutic agents for a wide range of diseases.1–3 Powerful methods have been developed for antibody discovery, including immunization and display methods.4,5 These methods, however, still present some challenges.6,7 One area that has proven difficult for therapeutic antibodies is the maturation of biological activity while preserving other important properties such as epitope selectivity, conformational stability, and solubility.8–10 The high-throughput nature of display methods (up to 1010 variants in the case of phage display) enables the exploration of the chemical space accessible at the various positions of the antibody–target interface.11 The availability from these approaches of panels of antibody variants with different potency offers the opportunity to carry out structure–activity relationship (SAR) studies, which allow the accurate assessment of the modifications of biological effects brought about by small physicochemical changes in the starting structure.12,13 Given the intrinsic randomness in the generation of mutational variants through combinatorial studies, however, it may be difficult to follow specific strategies in SAR-guided antibody maturation processes.
Here, to address this issue we report the use of a rational computational design of antibody variants, in order to open the way to systematic SAR investigations. In this proof-of-concept study, we use a single-domain antibody,14,15 called DesAb–Aβ3–9,16 carrying an in silico designed peptide (HETLTLR) grafted into the third complementarity-determining region (CDR3) targeting the N-terminus region (residues 3 to 9) of Aβ42.16–19 As the aggregation of Aβ42 into amyloid fibrils is one of the fundamental molecular processes underlying Alzheimer's disease (AD), the inhibition of Aβ42 aggregation has emerged as a major therapeutic strategy for AD.20 However, since the aggregation of Aβ42 is a complex process consisting of tightly coupled microscopic mechanisms, the perturbation of this process in a non-controlled manner can potentially exacerbate the toxicity associated with amyloid formation.21 To address this problem, we employ fluorescence-based Aβ42 aggregation assays coupled with a chemical kinetics framework to elucidate the inhibitory mechanism of the different single-domain antibody variants. We thus performed a round of site-directed mutagenesis by employing rationally designed CDR3 sequences to gain a 240-fold improvement in inhibiting the primary nucleation rate of Aβ42 aggregation, without compromising the stability of the parent single-domain antibody.
Results
Computational design of a panel of antibody variants
We have previously introduced the cascade method18 of designing antibody complementarity determining regions (CDRs) to target linear epitopes of interest within intrinsically disordered proteins or protein regions.16–18 This modular, combinatorial design strategy has proven to be rather successful, as all designed antibodies (DesAbs) that we tested experimentally so far, targeting proteins associated with misfolding diseases, were stable and active.16–19 This method is described in detail in ref. 18. Briefly, complementary peptides to be grafted into CDRs are designed with a combinatorial strategy based on the analysis of the interactions between amino acid sequences from the protein data bank (PDB). The cascade procedure starts by collecting from the PDB all fragments facing in a β-strand any subsequence of the target epitope of at least three residues. Then, starting from the longest of these fragments, referred to as initializer, complementary peptides are grown to the length of the target epitope by joining some of the other fragments following three rules: (i) fragments generating the same complementary peptide must come from b-strands of the same type (i.e., parallel or antiparallel), (ii) they must partly overlap with their neighboring fragments, and (iii) the overlapping regions must be identical in terms of both sequence and hydrogen-bonding pattern.18,19
The computational design strategy employed in this work relies on the fact that for most target epitopes the cascade procedure can generate a number of candidate CDRs. In particular, it is often the case that for a given initializer fragment, multiple candidate CDRs can be constructed by extending the initializer through different choices of fragments of shorter length, all identified as interacting with the chosen epitope. We thus exploited this fact to design a panel of antibody variants predicted to bind to the same target epitope and differing by only one or few amino acids, so to enable a structure–activity relationship (SAR) study (Fig. 1).
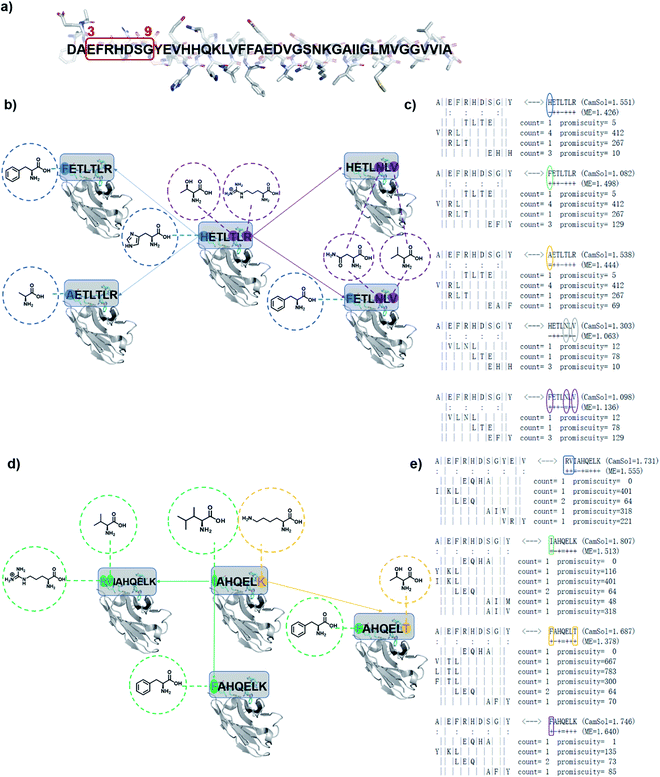 |
| Fig. 1 Schematic representation of designed antibodies (DesAbs) against Aβ42 aggregation. (a) Target epitope within the Aβ42 peptide sequence (residues 3–9). (b) A first group of DesAbs with different CDR3 regions is based on the -ETL-L- motif and, a second group (d) on the -AHQEL- motif. These two groups differ also in the predicted backbone–backbone hydrogen bonding pattern they form with the target epitope (colon symbols in (c) and (e)). In the group based on the -ETL-L- motif, we mutated systematically three positions, two at the N-terminus (blue) and one at the C-terminus (purple) to obtain the five DesAbs shown in panel (b), which include the parent DesAb-HETLTLR.16 (c and d) In the group based and on the -AHQEL- motif, we mutated two positions, one at the N-terminus (green) and one at the C-terminus (yellow) to obtain the four DesAbs shown in panel (d). (c and e) Fragment-composition of the designed peptides grafted in the CDR3 of the DesAbs, as resulting from the cascade procedure, together with their count and promiscuity scores (for details see ref. 18). The sequence of the target epitope (residues 3–9 of Aβ42) is shown to the left; colon marks denote residues predicted to be involved in hydrogen-bonds with the designed peptide, whose sequence is shown to the right, while the fragments composing it are below the target epitope; as the binding is antiparallel, these fragments are represented in the C- to N-terminus direction.18 The selected mutations are circled, and result from the choice of different fragments in the cascade procedure. Complementary peptide candidates with low predicted solubility (CamSol score) were not considered. | |
Specifically, we generated two groups of designed complementary peptides (paratopes) with different hydrogen bonding patterns to target the same epitope consisting in the N-terminal residues 3–9 of Aβ42 (Fig. 1). The first group is based on the -ETL-L- motif from the parent DesAb-HETLTLR (WT),16 and the second group is based on the -AHQEL- motif (Fig. 1). We grafted these selected paratopes onto the CDR3 loop of a human single-domain antibody scaffold, which has been shown to be stable and tolerant to the replacement of the CDR3.22,23
Characterisation of the DesAbs
All DesAbs were purified to high purity (>95%, Methods and Fig. S1†). Circular dichroism (CD) spectroscopy revealed that all DesAbs have a similar secondary structure content, fully compatible with that of a well folded single-domain antibody (Fig. 2a).17 All DesAbs are highly stable, exhibiting a thermal stability in accordance with previous reports (melting temperature, Tm ≈ 73 °C)16 (Fig. 2b).
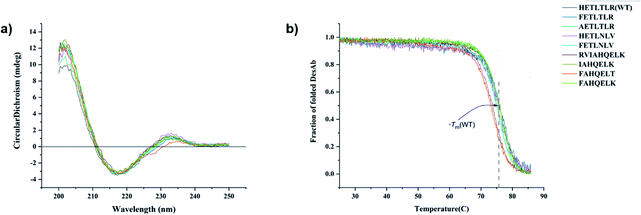 |
| Fig. 2 Biophysical characterization of the 9 DesAbs studied in this work. (a and b) Circular dichroism (CD) spectra (a) and CD thermal denaturation (b). All the DesAb samples were measured at the concentration of 10 μM. | |
All the DesAbs delay Aβ42 aggregation
Next, in order to compare and quantify the activity of these DesAbs to prevent the formation of Aβ42 aggregates, we measured their effects by applying in vitro kinetic aggregation assays using thioflavin T (ThT). ThT is an amyloid-specific fluorescent probe that can specifically interact with the stacked β-sheet structure of the amyloid fibrils.24,25 We monitored the process of aggregation of Aβ42 at 1 μM concentration in the presence of increasing concentrations of the DesAbs (DesAb
:
Aβ42 ratios 0
:
1, 1
:
8, 1
:
4 and 1
:
2) in triplicate at 37 °C under quiescent conditions, using a highly reproducible protocol previously described16,26–30 (Fig. 3). Our results indicate that DesAb-FETLTLR is particularly effective in delaying Aβ42 aggregation, with a potentiated activity compared with that of the parent DesAb (DesAb-HETLTLR). In a previous study16 DesAb-HETLTLR was found to be one of the most potent DesAbs in inhibiting both primary and secondary nucleation of Aβ42, and consequently decreasing the formation of oligomers.
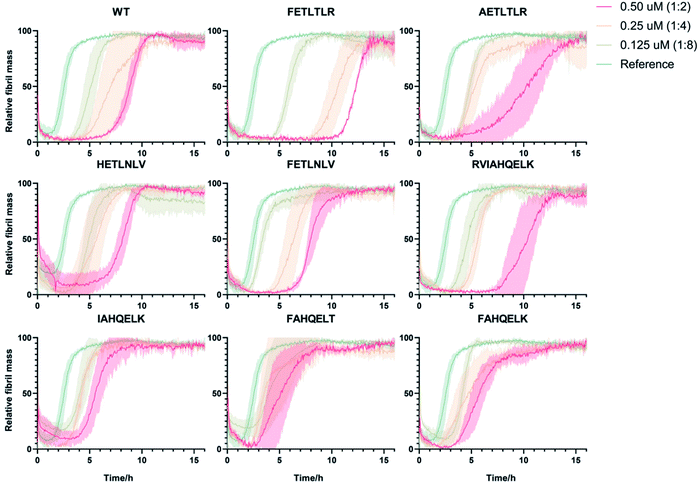 |
| Fig. 3 Unseeded kinetic aggregation assays of the 9 DesAbs studied in this work. We monitored the aggregation process of Aβ42 at 1 μM concentration in the presence of increasing concentrations of the DesAbs in triplicate at 37 °C under quiescent conditions. We tested the DesAb : Aβ42 ratios 0 : 1 (as reference, green), 1 : 8 (light green), 1 : 4 (orange) and 1 : 2 (red) finding in all cases a dose-depended delay of the aggregation process. | |
Effects of the DesAbs on the microscopic rate constants
To accurately identify the mechanisms of inhibition of aggregation of the DesAbs, we determined the changes in the global kinetic parameters k+kn and k+k2 that are affected by the antibodies (k+, kn, and k2 are the elongation, primary, and secondary rate constants, respectively) by fitting the aggregation curves (Fig. S2†) with the integrated rate laws as described in Methods. To decouple the effects of each DesAb on the combined rate constants k+kn and k+k2, we measured the changes in k+ from seeded experiments, which probe selectively the elongation of preformed Aβ42 fibrils. For this purpose, we carried out an additional set of experiments where the aggregation of monomeric Aβ42 in the absence and presence of each DesAb was monitored following the addition of 40% of preformed Aβ42 fibril seeds (Fig. 4). From these seeded experiments, we found that none of the DesAbs significantly affected the rate of elongation of Aβ42 fibrils, in agreement with previous reports for the parent DesAb-HETLTLR16 and with the fact that the target epitope is not part of the amyloid core. Fitting the aggregation curves (Fig. S3†) enabled us to determine the decreases in the single microscopic rate constant for each DesAb.
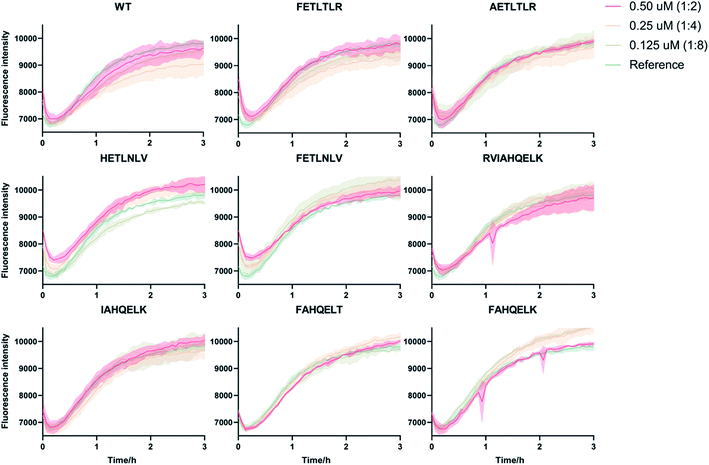 |
| Fig. 4 Seeded kinetic aggregation assays of the 9 DesAbs studied in this work. We monitored the aggregation process of Aβ42 at 1 μM concentration in the presence of increasing concentrations of the DesAbs in triplicate at 37 °C under quiescent conditions. 40% seeds were added before starting the aggregation reaction to monitor specifically the effects of the antibody variants on Aβ42 elongation. We tested the DesAb : Aβ42 ratios 0 : 1 (as reference, green), 1 : 8 (light green), 1 : 4 (orange) and 1 : 2 (red) finding in all cases minimal effect on fibril elongation. | |
From these individual microscopic rate constants (Fig. 5), we found that the DesAbs tended to show weak relative effects on secondary nucleation, while some of them showed strong inhibition of primary nucleation. In particular, DesAb-FETLTLR and DesAb-RVIAHQELK were more effective in inhibiting primary nucleation of Aβ42 and had a further potentiated activity compared with DesAb-HETLTLR.
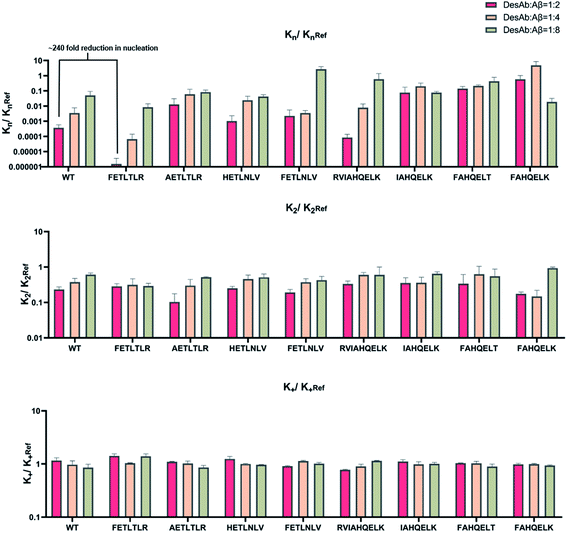 |
| Fig. 5 Relative changes in the microscopic rate constants of Aβ42 aggregation in the presence of the 9 DesAbs studied in this work. In the three columns, we report the relative changes kn/knRef (left), k2/k2Ref (middle), and k+/k+Ref (right) for the rate constants of primary nucleation (kn), secondary nucleation (k2) and elongation (k+). In the three rows, we report different DesAb : Aβ42 ratios, 1 : 2 (top), 1 : 4 (middle) and 1 : 8 (bottom). | |
Importantly, we found that only one amino acid difference between DesAb-FETLTLR and DesAb-HETLTLR resulted in around 240-fold improvement in the inhibition of primary nucleation (Fig. 5). We also noted that DesAb-AETLTLR, which has also only one amino acid difference to these two potent DesAbs, lost some of the WT activity on the primary nucleation, but gained a little on the secondary nucleation.
We also measured the effects of the DesAbs on the overall oligomer production during the aggregation reaction (the oligomer flux, Fig. 6). We found that DesAb-AETLTLR exhibited the strongest inhibition of oligomer production (over 75%) when used at relatively high concentrations (DesAb
:
Aβ 1
:
2), although it ranked more poorly at lower DesAb concentrations. Compared with the inhibition of primary nucleation, the secondary nucleation inhibition of all DesAb variants was weaker in a relative sense (Fig. 5). However, the aggregation of Aβ42 is dominated by secondary nucleation processes, which are orders of magnitude faster than the primary ones. Therefore, a weaker relative inhibition of secondary processes can lead to a stronger absolute inhibition, and thus a better inhibition of oligomer formation. Although DesAb-AETLTLR is less potent on primary nucleation than the WT, it showed the strongest secondary nucleation inhibition at the stoichiometry DesAb
:
Aβ 1
:
2, which in turn makes it the strongest inhibitor of oligomer production at this concentration. We also found that DesAb-FETLTLR maintained its potency even at low DesAb concentrations.
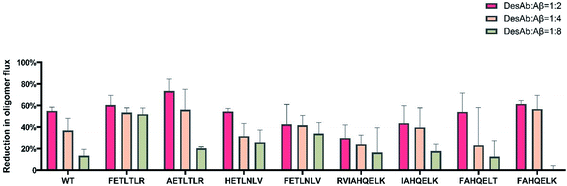 |
| Fig. 6 Inhibition of oligomer formation by the DesAbs. We present the percent reduction for the 9 DesAbs used in this work at decreasing stoichiometries, DesAb : Aβ42 1 : 2 (red), 1 : 4 (orange) and 1 : 8 (green). | |
The DesAbs delay Aβ42 aggregation in the presence of brain homogenates
To further investigate the potential of our DesAbs in a more physiological context, we measured the activity of the DesAbs by applying in vitro kinetic aggregation assays in the presence of 1% w/v homogenate of a human brain sample with cerebrovascular disease (Fig. 7). Both primary nucleation processes and seeded aggregation have been shown to be affected by the presence of complex biological mixtures such as those arising from human plasma, brain homogenate, and cerebrospinal fluid.31 The assays we performed in 1% w/v brain homogenate demonstrated that, even within a complex mixture such as human brain homogenate, both of our DesAb-HETLTLR(WT) and DesAb-FETLTLR retained their inhibitory activity, and the potency ranking was conserved. This result further highlights the specificity of the DesAbs as they were still able to bind to their target and exert their effects on kinetic sub-processes in the Aβ42 aggregation cascade in a complex cell lysate reaction matrix.
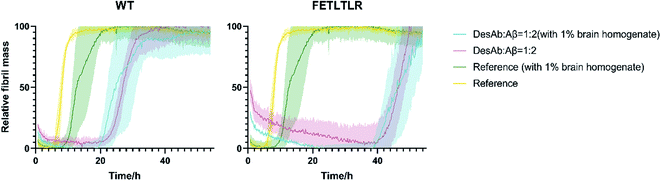 |
| Fig. 7 Comparison of unseeded kinetic aggregation assays of DesAb-HETLTLR(WT) and DesAb-FETLTLR in the presence of human brain homogenates. We monitored the aggregation process of Aβ42 at 1 μM concentration in the presence of the DesAbs in quadruplicate at 37 °C with and without 1% human Alzheimer's brain tissue homogenates under quiescent conditions. We tested the following conditions: 1 μM Aβ42 in buffer (as reference, yellow), 1 μM Aβ42 in buffer with 1% brain homogenates (green), 0.5 μM DesAb and 1 μM Aβ42 in buffer (1 : 2 ratio, magenta) and 0.5 μM DesAb and 1 μM Aβ42 in buffer with 1% brain homogenates (blue). | |
Conclusions
We have reported a proof of principle of the possibility of performing a systematic activity maturation of antibodies using computational design (Fig. 8). Starting from a potent single-domain antibody targeting the N-terminal region of Aβ42, we have shown that the investigation of different residue choices still predicted to bind the target epitope, as well as of different hydrogen-bonding patterns, enable the selection of an antibody mutant with over two orders of magnitude increase in potency in inhibiting primary nucleation. We anticipate that, upon further developments, the computational maturation approach that we presented will be of general applicability for increasing the affinity in protein–protein interactions.
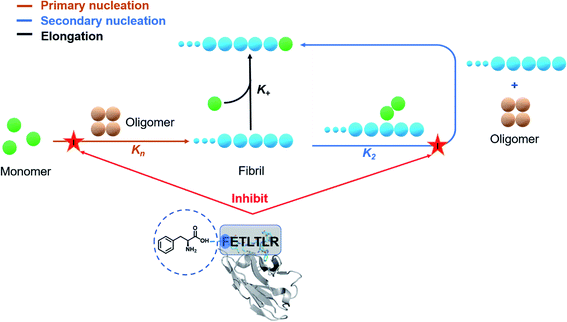 |
| Fig. 8 Effects of the DesAb-FETLTLR on the network kinetics. The largest effect of the DesAb-FETLTLR described in this work is on primary nucleation and secondary nucleation, while elongation is less affected. The inhibition of the nucleation processes corresponds to a reduction in the production of Aβ42 oligomers. | |
Materials and methods
Computational design
The computational design was carried out using the cascade method.18,19 In brief, the complementary peptides are built through a fragment-based procedure. The first step is the identification of short peptide fragments that interact in a β-strand conformation with short peptide fragments of the target sequence in at least one of the protein structures in the PDB database. The short peptide fragments are then linked together to form a complementary peptide (paratope) for the given epitope.
We first generated potential candidates with different hydrogen binding patterns to target the N-terminus of Aβ42 (residues 3–9, which covers the epitope of aducanumab (residues 3–7),32 the therapeutic antibody from Biogen Inc. that was recently approved for the treatment of AD), and then selected those with high complementarity score C and CamSol score. Our parent antibody (DesAb-HETLTLR, Fig. S4†) was previously shown to be able to inhibit both primary and secondary nucleation of Aβ42 and decrease the formation of oligomers.16
Circular dichroism
Far-ultraviolet (UV) circular dichroism (CD) spectroscopy was used to analyze the secondary structure of the DesAbs in solution, as recorded by a Jasco J-810 spectropolarimeter equipped with an Applied Photophysics Chirascan system and a Quantum TC125 temperature control unit, using a 0.1 cm pathlength cuvette. Samples contained 10 μM protein in 20 mM sodium phosphate (NaP) and 100 mM sodium chloride buffer at pH 8. The far-UV CD spectra of all DesAbs were recorded from 200 to 250 nm at 25 °C and averaged over three scans, and the spectrum of the buffer was subtracted from the averaged data. All spectra were measured as θ (in mdeg).
Thermal stability assay
The thermal stability of the DesAbs was analyzed by monitoring the CD signal at 207 nm from 25 to 90 °C at a rate of 0.5 °C min−1. Data points were acquired every 0.1 °C with a bandwidth of 1 nm. Analysis of the thermal unfolding curves was performed, assuming a two-state unfolding model.
Aβ42 expression and purification
Aβ42 peptides were expressed in E. coli BL21 (DE3) pLysS cells (Agilent Technologies) and extracted, as described previously.25 Before running kinetics experiments, the purified lyophilized Aβ42 peptide was dissolved in 6 M GuHCl (pH 8) and incubated for at least 45 min on ice. This solution was then subjected to gel filtration using a Superdex 75 10/300 GL column on an Äkta Pure system (GE Healthcare), and the peak corresponding to the monomeric Aβ42 peptide was collected in low-binding test tubes (Corning) on ice. The column was equilibrated with 20 mM NaP buffer supplemented with 200 μM EDTA at pH 8.
DesAbs expression and purification
Genes encoding the two groups of DesAbs were generated through site directed mutagenesis from the gene of the WT DesAb-HETLTLR (Fig. S4†) in a pet28 plasmid. The different antibodies were expressed in E. coli SHuffle T7 LysY (NEB) competent cells (Table S1†). Cells were plated and starter cultures inoculated from single colonies were grown overnight for 15 hours at 37 °C at 200 rpm in LB medium supplemented with kanamycin (50 μg mL−1). Glycerol stocks were made by taking 700 μL of the starter cells supplemented with 700 μL glycerol, and stored at −80 °C.
Growth was started in 1 L LB medium supplemented with kanamycin (50 μg mL−1) at 26 °C for about 8 hours (to OD600 = 0.6–0.8), the temperature was then lowered to 20 °C and IPTG was added to a final concentration of 0.2 mM. After overnight expression at 20 °C, cells were harvested by centrifugation at 7500 rpm (JA-8.1 rotor, Beckmann Coulter) and resuspended in 45 mL 20 mM sodium phosphate (pH 8.0) buffer supplemented with 10 mM imidazole and one tablet of Roche Complete EDTA-free protease inhibitor cocktail. Cells were then lysed using sonication for 5 minutes at 40% amplitude in 15 s on and 45 s off cycles. The supernatant containing the protein was separated from cell debris using centrifugation at 18
000 rpm (JA-20 rotor, Beckmann Coulter). The cleared lysate was loaded onto a Ni2+-NTA Superflow column (Qiagen), equilibrated with 20 mM sodium phosphate buffer (pH 8.0) containing 10 mM imidazole. Non-specifically bound proteins were then washed away with 100 mL of 20 mM NaP buffer containing 60 mM imidazole, and the his-tagged DesAb was eluted with 20 mM NaP buffer containing 200 mM imidazole. The purity of the collected fractions was assessed by SDS-PAGE, and only the purest fractions were collected and dialysed in 20 mM NaP 100 mM NaCl buffer for 20 hours, and finally concentrated to a concentration between 10 μM and 30 μM via an Amicon Ultra – 15/10k M.w. cut-off filter (Millipore), flash frozen and stored in the −80 °C.
Aggregation assay
Solutions were prepared containing monomeric 1 μM Aβ42 peptide in the presence of increasing amounts of each DesAb (90% v/v of 1 μM Aβ42 peptide and 10% v/v of DesAbs, final buffer would be 20 mM NaP 10 mM NaCl 180 μM EDTA, supplemented with 20 μM thioflavin T (ThT)). Seeded experiments were performed in the presence of 40% preformed fibrils that were prepared freshly the same day27 with increasing antibody-to-Aβ42 monomer ratio (0
:
1, 1
:
8, 1
:
4 and 1
:
2). Unseeded kinetic aggregation assays of the DesAb-HETLTLR(WT) and DesAb-FETLTLR were also performed with and without the presence of 1% w/v human cerebrovascular disease brain homogenate prepared as previously described.31 Each sample was then pipetted into multiple wells of a low-binding 96-well half-area plate of black polystyrene with a clear bottom and polyethylene glycol coating (Corning 3881) (80 μl per well). Plates were sealed to prevent evaporation. Aggregation assays were performed at 37 °C under quiescent conditions using a CLARIO or OMEGA star plate reader (BMG Labtech). The ThT fluorescence was measured through the bottom of the plate every minute with an excitation filter of 440 nm and an emission filter of 480 nm.
Kinetic analysis
The kinetic aggregation of amyloid proteins is typically described as a nucleation–elongation mechanism. Fluorescent dyes, such as ThT, whose fluorescence increase upon binding to the characteristic β-sheet structure within amyloids, are used to monitor fibril formation. Total fibril mass concentration is then plotted as a function of time and results in a sigmoidal shaped function, in which the rate limiting step is the formation of nuclei. The time evolution of the total fibril mass concentration, M(t), in the absence of pre-aggregated seeds is described by the following integrated rate law:
where the kinetic parameters B±, C±, k, k∞ and
∞ have been previously described in detail,33,34 and are functions of the two combinations of the microscopic rate constants k+kn and k+k2, where kn, k+, and k2 are the rate constants of primary nucleation, elongation, and secondary nucleation rate constants, respectively.
The decrease of k+ in the presence of DesAbs can be directly calculated from the seeded experiments (Fig. 5).16 We thus evaluated the rate of the formation of fibrillar aggregates using by estimating the slope r of a linear fit of the seeded data (Fig. S2†), and then using r = 2k+P0m, where P0 is the number of seeds introduced in the system and m is the initial monomer concentration. All the fitting process was performed using Python. The k+ values derived from the high-seeded aggregation profiles were used for to calculate kn and k2 from the fitting of the unseeded data, which yields k+kn and k+k2; only data points from 0.15 to 0.72 relative fibril mass were used for this fit. Finally, the k+, kn and k2 values obtained from the previous fitting process were used to check the fitting quality by fitting the data points of unseeded experiments from 0.05 to 0.95 relative fibril mass (Fig. S3†). The relative decrease in the apparent rate constant k+, kn and k2 was evaluated by dividing the rate in the presence of DesAbs with the value obtained in their absence (Fig. 5).
The perturbation of the different microscopic reaction rate constants (kn, k2 and k+) also results in dramatically different effects on the generation of low molecular weight oligomeric species of Aβ42. To illustrate this behaviour, we calculated the time evolution of the rate of generation of new on-pathway oligomers from monomers (Fig. S5–S7†). Using the rate constants kn, k2 and k+, in the presence or absence of DesAbs, we can estimate the reactive flux toward oligomers rn(t) as
rn(t) = knm(t)nc + k2M(t)m(t)n2 |
Since at the end of the reaction only a negligible number of oligomers is detectable in the system (everything has converted to fibrils), the total number of fibrils present at the end of the process is indicative of the total number of on-pathway oligomers generated throughout the reaction. This value was calculated by integrating the nucleation rate rn(t) over the reaction (Fig. 6).
Data availability
All study data are included in the article and/or ESI.†
Author contributions
P. S. and M. V. designed research; J. L., C. F., M. A. M. and P. S. performed research; J. L., P. S. and M. V. analyzed data; and J. L., M. A. M., P. S. and M. V. wrote the paper.
Conflicts of interest
There are no conflicts to declare.
Acknowledgements
P. S. is supported by a Royal Society University Research Fellowship (URF\R1\201461).
References
- P. J. Carter and G. A. Lazar, Next generation antibody drugs: pursuit of the ‘high-hanging fruit’, Nat. Rev. Drug Discovery, 2018, 17(3), 197 CrossRef CAS PubMed.
- A. L. Grilo and A. Mantalaris, The increasingly human and profitable monoclonal antibody market, Trends Biotechnol., 2019, 37(1), 9–16 CrossRef CAS PubMed.
- H. Kaplon, M. Muralidharan, Z. Schneider and J. M. Reichert, Antibodies to watch in 2020, mAbs, 2020, 1703531 CrossRef PubMed.
- E. T. Boder, K. S. Midelfort and K. D. Wittrup, Directed evolution of antibody fragments with monovalent femtomolar antigen-binding affinity, Proc. Natl. Acad. Sci. U. S. A., 2000, 97(20), 10701–10705 CrossRef CAS PubMed.
- A. R. Bradbury, S. Sidhu, S. Dübel and J. McCafferty, Beyond natural antibodies: the power of in vitro display technologies, Nat. Biotechnol., 2011, 29(3), 245–254 CrossRef CAS PubMed.
- A. Bradbury and A. Plückthun, Reproducibility: Standardize antibodies used in research, Nature, 2015, 518(7537), 27–29 CrossRef CAS PubMed.
- A. Gray, A. R. Bradbury, A. Knappik, A. Plückthun, C. A. Borrebaeck and S. Dübel, Animal-free alternatives and the antibody iceberg, Nat. Biotechnol., 2020, 38(11), 1234–1239 CrossRef CAS PubMed.
- R. A. Lerner, Combinatorial antibody libraries: new advances, new immunological insights, Nat. Rev. Immunol., 2016, 16(8), 498 CrossRef CAS PubMed.
- J. K. Liu, The history of monoclonal antibody development–progress, remaining challenges and future innovations, Ann. Med. Surg., 2014, 3(4), 113–116 CrossRef PubMed.
- A.-M. Wolf Pérez, P. Sormanni, J. S. Andersen, L. I. Sakhnini, I. Rodriguez-Leon, J. R. Bjelke, A. J. Gajhede, L. De Maria, D. E. Otzen and M. Vendruscolo,
In vitro and in silico assessment of the developability of a designed monoclonal antibody library, mAbs, 2019, 11(2), 388–400 CrossRef PubMed.
- S. S. Sidhu, Phage display in pharmaceutical biotechnology, Curr. Opin. Biotechnol., 2000, 11(6), 610–616 CrossRef CAS PubMed.
- A. Cherkasov, E. N. Muratov, D. Fourches, A. Varnek, I. I. Baskin, M. Cronin, J. Dearden, P. Gramatica, Y. C. Martin and R. Todeschini, QSAR modeling: where have you been? Where are you going to?, J. Med. Chem., 2014, 57(12), 4977–5010 CrossRef CAS PubMed.
-
R. Guha, On exploring structure–activity relationships, in In silico models for drug discovery, Springer, 2013, pp. 81–94 Search PubMed.
- I. Jovčevska and S. Muyldermans, The therapeutic potential of nanobodies, BioDrugs, 2020, 34(1), 11–26 CrossRef PubMed.
- S. Muyldermans, A guide to: generation and design of nanobodies, FEBS J., 2020, 288(7), 2084–2102 CrossRef PubMed.
- F. A. Aprile, P. Sormanni, M. Perni, P. Arosio, S. Linse, T. P. Knowles, C. M. Dobson and M. Vendruscolo, Selective targeting of primary and secondary nucleation pathways in Aβ42 aggregation using a rational antibody scanning method, Sci. Adv., 2017, 3(6), e1700488 CrossRef PubMed.
- F. A. Aprile, P. Sormanni, M. Podpolny, S. Chhangur, L.-M. Needham, F. S. Ruggeri, M. Perni, R. Limbocker, G. T. Heller and T. Sneideris, Rational design of a conformation-specific antibody for the quantification of Aβ oligomers, Proc. Natl. Acad. Sci. U. S. A., 2020, 117(24), 13509–13518 CrossRef CAS PubMed.
- P. Sormanni, F. A. Aprile and M. Vendruscolo, Rational design of antibodies targeting specific epitopes within intrinsically disordered proteins, Proc. Natl. Acad. Sci. U. S. A., 2015, 112(32), 9902–9907 CrossRef CAS PubMed.
- P. Sormanni, F. A. Aprile and M. Vendruscolo, Third generation antibody discovery methods: in silico rational design, Chem. Soc. Rev., 2018, 47(24), 9137–9157 RSC.
- T. P. Knowles, M. Vendruscolo and C. M. Dobson, The amyloid state and its association with protein misfolding diseases, Nat. Rev. Mol. Cell Biol., 2014, 15(6), 384–396 CrossRef CAS PubMed.
- P. Arosio, M. Vendruscolo, C. M. Dobson and T. P. Knowles, Chemical kinetics for drug discovery to combat protein aggregation diseases, Trends Pharmacol. Sci., 2014, 35(3), 127–135 CrossRef CAS PubMed.
- P. A. Barthelemy, H. Raab, B. A. Appleton, C. J. Bond, P. Wu, C. Wiesmann and S. S. Sidhu, Comprehensive analysis of the factors contributing to the stability and solubility of autonomous human VH domains, J. Biol. Chem., 2008, 283(6), 3639–3654 CrossRef CAS PubMed.
- A. R. A. Ladiwala, M. Bhattacharya, J. M. Perchiacca, P. Cao, D. P. Raleigh, A. Abedini, A. M. Schmidt, J. Varkey, R. Langen and P. M. Tessier, Rational design of potent domain antibody inhibitors of amyloid fibril assembly, Proc. Natl. Acad. Sci. U. S. A., 2012, 109(49), 19965–19970 CrossRef CAS PubMed.
- M. Biancalana and S. Koide, Molecular mechanism of Thioflavin-T binding to amyloid fibrils, Biochim. Biophys. Acta, Proteins Proteomics, 2010, 1804(7), 1405–1412 CrossRef CAS PubMed.
- S. I. Cohen, S. Linse, L. M. Luheshi, E. Hellstrand, D. A. White, L. Rajah, D. E. Otzen, M. Vendruscolo, C. M. Dobson and T. P. Knowles, Proliferation of amyloid-β42 aggregates occurs through a secondary nucleation mechanism, Proc. Natl. Acad. Sci. U. S. A., 2013, 110(24), 9758–9763 CrossRef CAS PubMed.
- E. Hellstrand, B. Boland, D. M. Walsh and S. Linse, Amyloid β-protein aggregation produces highly reproducible kinetic data and occurs by a two-phase process, ACS Chem. Neurosci., 2010, 1(1), 13–18 CrossRef CAS PubMed.
- J. Habchi, S. Chia, R. Limbocker, B. Mannini, M. Ahn, M. Perni, O. Hansson, P. Arosio, J. R. Kumita and P. K. Challa, Systematic development of small molecules to inhibit specific microscopic steps of Aβ42 aggregation in Alzheimer's disease, Proc. Natl. Acad. Sci. U. S. A., 2017, 114(2), E200–E208 CrossRef CAS PubMed.
- S. Chia, J. Habchi, T. C. Michaels, S. I. Cohen, S. Linse, C. M. Dobson, T. P. Knowles and M. Vendruscolo, SAR by kinetics for drug discovery in protein misfolding diseases, Proc. Natl. Acad. Sci. U. S. A., 2018, 115(41), 10245–10250 CrossRef CAS PubMed.
- P. R. Lindstedt, F. A. Aprile, P. Sormanni, R. Rakoto, C. M. Dobson, G. J. Bernardes and M. Vendruscolo, Systematic Activity Maturation of a Single-Domain Antibody with Non-canonical Amino Acids through Chemical Mutagenesis, Cell Chem. Biol., 2020, 28(1), 70–77 CrossRef PubMed.
- S. Linse, T. Scheidt, K. Bernfur, M. Vendruscolo, C. M. Dobson, S. I. Cohen, E. Sileikis, M. Lundqvist, F. Qian and T. O'Malley, Kinetic fingerprints differentiate the mechanisms of action of anti-Aβ antibodies, Nat. Struct. Mol. Biol., 2020, 1–9 Search PubMed.
- M. A. Metrick II, N. do Carmo Ferreira, E. Saijo, A. G. Hughson, A. Kraus, C. Orru, M. W. Miller, G. Zanusso, B. Ghetti, M. Vendruscolo, B. Caughey, T. Bussière and S. Hamann, Million-fold sensitivity enhancement in proteopathic seed amplification assays for biospecimens by Hofmeister ion comparisons, Proc. Natl. Acad. Sci. U. S. A., 2018, 116(46), 23029–23039 CrossRef PubMed.
- J. W. Arndt, F. Qian, B. A. Smith, C. Quan, K. P. Kilambi, M. W. Bush, T. Walz, R. B. Pepinsky, T. Bussière and S. Hamann, Structural and kinetic basis for the selectivity of aducanumab for aggregated forms of amyloid-β, Sci. Rep., 2018, 8(1), 1–16 CAS.
- S. I. Cohen, P. Arosio, J. Presto, F. R. Kurudenkandy, H. Biverstål, L. Dolfe, C. Dunning, X. Yang, B. Frohm and M. Vendruscolo, A molecular chaperone breaks the catalytic cycle that generates toxic Aβ oligomers, Nat. Struct. Mol. Biol., 2015, 22(3), 207 CrossRef CAS PubMed.
- G. Meisl, J. B. Kirkegaard, P. Arosio, T. C. Michaels, M. Vendruscolo, C. M. Dobson, S. Linse and T. P. Knowles, Molecular mechanisms of protein aggregation from global fitting of kinetic models, Nat. Protoc., 2016, 11(2), 252–272 CrossRef CAS PubMed.
Footnotes |
† Electronic supplementary information (ESI) available. See DOI: 10.1039/d1sc03898b |
‡ Current address: Department of Structural Biology and Chemistry, Institut Pasteur, 25-28 Rue du Dr Roux, 75015 Paris. |
|
This journal is © The Royal Society of Chemistry 2021 |
Click here to see how this site uses Cookies. View our privacy policy here.