Making sense of sensemaking: using the sensemaking epistemic game to investigate student discourse during a collaborative gas law activity
Received
22nd September 2020
, Accepted 5th December 2020
First published on 8th December 2020
Abstract
Beyond students’ ability to manipulate variables and solve problems, chemistry instructors are also interested in students developing a deeper conceptual understanding of chemistry, that is, engaging in the process of sensemaking. The concept of sensemaking transcends problem-solving and focuses on students recognizing a gap in knowledge and working to construct an explanation that resolves this gap, leading them to “make sense” of a concept. Here, we focus on adapting and applying sensemaking as a framework to analyze three groups of students working through a collaborative gas law activity. The activity was designed around the learning cycle to aid students in constructing the ideal gas law using an interactive simulation. For this analysis, we characterized student discourse using the structural components of the sensemaking epistemic game using a deductive coding scheme. Next, we further analyzed students’ epistemic form by assessing features of the activity and student discourse related to sensemaking: whether the question was framed in a real-world context, the extent of student engagement in robust explanation building, and analysis of written scientific explanations. Our work provides further insight regarding the application and use of the sensemaking framework for analyzing students’ problem solving by providing a framework for inferring the depth with which students engage in the process of sensemaking.
Introduction
Historically, the field of chemistry education research has been interested in investigating students’ problem-solving skills and classifying common misconceptions held by students (Yuriev et al., 2017; Cooper and Stowe, 2018). Arguably more important than problem-solving and algorithmic processing, educators are also interested in supporting students in developing deeper understanding of the problems presented and the scientific concepts underlying them—that is, student engagement in sensemaking. Looking at the wider educational research literature, there has been a growing body of work surrounding the ways in which students engage in the process of sensemaking along with an attempt to develop a clear definition for this elusive construct. This work has generally involved three different bodies of literature: sensemaking as a cognitive process, sensemaking as an argumentative discourse practice, and sensemaking as a generalized approach towards science learning (Odden and Russ, 2019a). Synthesizing the fragmented literature on sensemaking, Odden and Russ (2019a) proposed a definition of the process to provide theoretical consistency for the field, defining sensemaking as “a dynamic process of building or revising an explanation in order to ‘figure something out’—to ascertain the mechanism underlying a phenomenon in order to resolve a gap or inconsistency in one's understanding,” (pp. 191–192). This definition of sensemaking stands in contrast to the process of answer-making, in which students’ goal is to provide an answer to complete the given task (Chen et al., 2013; Odden and Russ, 2018). Using this more concrete definition for sensemaking, researchers can better identify when students are engaging in sensemaking and instructors can work to foster and support students’ engagement in the process of sensemaking. Because the work by Odden and Russ (2019a) is relatively recent, it has not yet been applied to contexts such as chemistry. The chemistry community can benefit from the sensemaking framework discussed and applied in work by Odden and Russ (2018, 2019a, 2019b) because it serves as a new approach to characterize student learning in a chemistry context.
Since the process of sensemaking involves an iterative building of ideas, a collaborative learning environment serves as a well-suited context for investigating students’ sensemaking. Active learning pedagogies that utilize a collaborative learning environment have been widely incorporated into undergraduate chemistry courses due to their immense benefit to student learning (Freeman et al., 2014; Theobald et al., 2020). One common active learning pedagogy applied in the chemistry community is Process Oriented Guided Inquiry Learning (POGIL). Recently, our research group synthesized the published research surrounding POGIL, noting the overwhelming use of pre/post assessments to measure the effects of POGIL but a limited amount of research into the specific features of POGIL activities that help facilitate the observed positive learning gains (Rodriguez et al., 2020b). Our review showcases the need for qualitative work that can elucidate the specific features of active learning pedagogies that promote student learning. More than recognizing that these interventions improve student learning, further research is needed to address the ways in which active learning approaches influence student learning and support students in doing more than simply providing an answer.
Although the existing literature on student engagement in active learning pedagogies has provided a wealth of insight for instructors, analyzing student discourse using sensemaking will augment the existing literature by providing a new lens to uncover the role of collaborative activities in promoting student learning. We are interested in the extent to which collaborative learning promotes student movement beyond answer-making and towards the process of sensemaking. Therefore, we are interested in addressing the following research question: In what ways do students engage in the process of sensemaking during a collaborative gas law activity?
Review of relevant literature
Process oriented guided inquiry learning
A large body of literature indicates active learning approaches such as POGIL improve student content knowledge, promote scientific discourse, and increase motivation (Freeman et al., 2014; Walker and Warfa, 2017; Rodriguez et al., 2020b; Theobald et al., 2020). POGIL is a pedagogical approach founded in constructivist principles that aims to replace lecture by having students collaboratively work in small groups to build new knowledge (Moog and Farrell, 2017). This collaborative approach was developed in the chemistry community, but has been applied in a wide range of disciplines, including aviation (Vacek, 2011), pre-service education (Irwanto et al., 2018), and finance and marketing (Hale and Mullen, 2009; Maurer, 2014). This approach, as outlined by the POGIL project, is defined by the following key features: students working in small groups of 3–4 with assigned student roles; use of an approved POGIL activity; and a facilitator who guides students as they work through the activity (as opposed to lecturing) (Hanson, 2011; The POGIL Project, 2020b). These POGIL activities are structured around the principles of the learning cycle (Karplus and Thier, 1968; Lawson, 1988), where students develop their own understanding in a guided progression by first analyzing trends in data (exploration), using the data to develop a definition for a concept (concept invention), and finally applying the knowledge they constructed (application). The collaborative aspect of POGIL is driven by small groups in which students have assigned roles in order to distribute the workload of the group. Additionally, a main tenet of the POGIL approach is developing students’ process skills—the processes that occur as students construct knowledge; for example, process skills include problem solving, information processing, teamwork, oral and written communication, and management (Moog et al., 2008; POGIL Project, 2020).
Our recent literature review analyzed the extant research surrounding POGIL, pointing to the predominant use of pre/post assessments to gauge the effect of POGIL interventions, which indicates improvement in student learning across different dimensions (Rodriguez et al., 2020b). Aside from the pre/post assessments, there has been less research focused on the features of a POGIL classroom such as student roles, process skills, and the role of the facilitator in promoting student learning. Outside research using pre/post assessments, the existing qualitative studies have commonly analyzed student discourse using Toulmin's Argumentation Pattern (TAP) model to make inferences about learning from students’ argumentation (Daubenmire and Bunce, 2008; Becker et al., 2013, 2015; Kulatunga and Lewis, 2013; Kulatunga et al., 2014; Daubenmire et al., 2015; Moon et al., 2016, 2017b, 2017a; Stanford et al., 2016, 2018). With regards to the structure of POGIL activities, there have been a few studies that analyzed the ways in which the learning cycle influenced arguments generated by students (Kulatunga et al., 2014), promoted use of causal reasoning (Moon et al., 2016, 2017a), and the ways question language guided coordination of students’ responses across the chemistry triplet (Stanford et al., 2018). The work by Kulatunga et al. (2014) showed that the learning cycle scaffolds students in constructing arguments especially for the application phase, and that argumentation was limited during the exploration phase, due to the large use of directed questions. Other research has shown that the students are limited in the causal reasoning they employ and that there is a clear link between the question prompt and depth of explanations provided by students (Moon et al., 2016, 2017a; Stanford et al., 2018).
The ideal gas law
The data presented in this article was collected while students worked through an activity designed for a general chemistry sequence at the university level. This activity was developed as part of a larger project in our research group that focused on the creation and validation of Process Oriented Guided Inquiry Learning (POGIL) activities that emphasized student reasoning surrounding the nature and purpose of models (Rodriguez et al., 2020c).
In the existing literature related to gas laws, research has commonly focused on students’ conceptual understanding of specific gas law problems and general problem solving. For instance, Nurrenbern and Pickering's (1987) foundational work showed that general chemistry students do not perform as well when problems gas law problems are framed conceptually rather than algorithmically, with follow-up studies by other researchers making supporting these claims (Pickering, 1990; Sawrey, 1990; Nakhleh, 1993; Nakhleh and Mitchell, 1993; Zoller et al., 1995; Nakhleh et al., 1996; Stamovlasis et al., 2005; Cracolice et al., 2008; Sanger et al., 2013). In a similar vein, Madden et al. (2011) noted that students will also solve problems algebraically without displaying underlying conceptual understanding. Additionally, Matijaevi et al. (2016) pointed to students’ difficulty in translating gas law equations to pictorial representations which further highlights their limited conceptual understanding of the laws. Other research focused on students’ conceptions of gas laws has uncovered a general lack of deep conceptual reasoning behind the behavior of gases, more specifically with regards to the particulate nature of gases and how to relate the behavior of gases to physical situations (De Berg, 1995; Lin et al., 2000; Kautz et al., 2005a, 2005b; Robertson and Shaffer, 2013, 2016). Moreover, Schuttlefield et al. (2012), detailed the specific features of problems that led students to incorrect answers, finding that number format (i.e., scientific, general, decimal) and unit conversions (for variables like volume and temperature) were particularly challenging for students. In an effort to understand student's problem-solving approaches when working with gas laws, Tang and Pienta (2012) characterized the process of students’ problem solving into three stages: problem reading, problem planning, and calculation. They found that unsuccessful students spent larger amounts of time in the planning phase, while both successful and unsuccessful students spent similar amounts of time in the reading phase; suggesting students need scaffolding to move them past the planning stage and into the calculation phase (Tang and Pienta, 2012). Chen et al. (2019) also analyzed students problem-solving through the lens of systems thinking, showing that students struggle to incorporate higher-order systems thinking ability and fail to retrieve essential conceptual material related to gas law problems.
In summary, the research into students’ reasoning with ideal gas law problems suggests students need more scaffolded tasks that engage them in deeper conceptual understanding. A common method used in chemistry contexts that reinforces conceptual knowledge is the use of dynamic, particulate-level simulations that students can use to actively investigate molecular interactions. Studies have shown that dynamic, particle-level representations improve students’ conceptual knowledge (Williamson and Abraham, 1995; Sanger et al., 2000; Tasker and Dalton, 2006; Akaygun and Jones, 2013a, 2013b). Given this research, our activity was designed to focus on a conceptual understanding by having students use a dynamic simulation to construct the ideal gas law and investigate limitations of the model.
Theoretical perspectives
Sensemaking
In the research literature, work related to sensemaking has been addressed from three main perspectives: as a cognitive process, a discursive practice, and a discursive frame. From a cognitive perspective, the research on sensemaking followed two main theories of learning: knowledge integration, and knowledge-in-pieces (KiP). The knowledge integration framework proposes that students have existing mental models and the sensemaking process occurs when students assimilate and compare a new model to their existing mental models (Chi et al., 1994; Shen and Linn, 2011). In the knowledge-in-pieces perspective, knowledge is built out of a network of many fine-grained cognitive units or resources, and students engage in problem solving through the activation of clusters of resources within their network (diSessa, 1993; Clark, 2006). This perspective posits that students thinking about a concept is more than a unitary, stable cognitive unit, but rather comprised of many fine-grained cognitive resources that are activated within specific contexts (diSessa, 1993; Mestre, 2005). As a discursive practice, sensemaking has been conceptualized as a collaborative process involving construction and critique. Importantly, within this, is the role of argumentation, that is, the building of arguments with data and using reasoning to substantiate claims. Ford (2012) proposed that this process is reliant upon construction (connection of knowledge elements) and critique (establishing coherence of connections) which can occur in an individual's mind or as a social process involving argumentation among a group. Finally, sensemaking has also been viewed as a general approach toward science learning also known as a frame—the expectations or goals people have for a specific activity. Frames have also been defined in the way students conceptualize a task or how they would answer the question “What's going on here?” (Tannen, 1993). Importantly, frames are dynamic and students will move among frames, especially within a group where individual's frames may interfere with one another (Tannen, 1993). Simple frames such as memorizing, list-making, and reproducing scientific information have been described in the literature; the focus of this paper is the sensemaking frame, which has the goal of building an explanation for a concept that is not yet fully understood (Odden and Russ, 2019b). Moreover, according to Odden and Russ (2019b), sensemaking is a process mediated through the reoccurrence of student-generated “vexing questions” that move students forward as they attempt to resolve the question posed.
In this study we adopt the definition Odden and Russ (2019a) proposed for sensemaking, “a dynamic process of building or revising an explanation in order to ‘figure something out’—to ascertain the mechanism underlying a phenomenon in order to resolve a gap or inconsistency in one's understanding.” Their definition combined the three literature strands discussed in this section by acknowledging that sensemaking entails integration of new knowledge into old (cognitive) and the dynamic process of building an explanation (discursive) with the ultimate goal of “figuring something out” (framing). This definition also aligns with a further development in defining the sensemaking process where Odden and Russ (2018) framed sensemaking as an epistemic game. Epistemic games were initially proposed by Collins and Ferguson (1993) as a way of describing the ways in which inquiry unfolds (i.e., the generation of new knowledge), where game is a reference to the coherent set of “rules” an individual utilizes in a particular context and epistemic is a reference to the type of ideas and assumptions that influence and mediate the construction of knowledge. According to Tuminaro and Redish (2007), epistemic games can be identified and distinguished from one another based on the presence of ontological and structural components. The ontological components involve (1) a knowledge base, which reflects the cognitive resources relevant for the game (e.g., mathematical reasoning, physics or chemistry principles, etc.) and (2) an epistemic form, which can be described as the target structure used to guide inquiry, that is, the desired product or outcome that motivates continued engagement in the epistemic game. The structural components of an epistemic game involve the specific conditions that begin and end an epistemic game (entry condition and ending condition) and the actions taken within a particular game (moves).
The epistemic games framework has previously been applied to chemistry (Sevian and Couture, 2018; Rodriguez et al., 2020a) and physics (Tuminaro and Redish, 2007; Chen et al., 2013) contexts. Types of epistemic games proposed by Tuminaro and Redish (2007) in a physics problem solving context include: mapping meaning to mathematics, recursive plug and chug, and the physical mechanism game. For example, a game named “pictorial analysis” has a target epistemic form that involves a knowledge base rooted in physics principles and an epistemic form centered on the generation of a representation that identifies spatial relationships within a problem statement such as a free-body diagram, circuit diagram, or schematic of a physical situation. Specific moves that occur in this game involve identifying a target concept, choosing an external representation, telling a conceptual story based on relations among objects, and filling the gaps of the representation (Tuminaro and Redish, 2007). Relevant to this work, another epistemic game that has been described is the “answer-making” game, in which the moves of the epistemic game involve students either (1) trying to remember the answer to a problem and subsequently justify this answer using mathematics and conceptual reasoning or (2) using mathematics and conceptual reasoning to reach an answer (Chen et al., 2013). This epistemic game is in contrast to the “sensemaking epistemic game”, with both games provided in Fig. 1 (Chen et al., 2013; Odden and Russ, 2018). For clarity, in Fig. 1 we have depicted a simplified version of the answer-making game—illustrating the scenario where students do not remember the answer—in order to highlight similarities and differences with the sensemaking epistemic game. The sensemaking epistemic game begins by students (0) gathering initial knowledge before (1) noticing a gap in understanding followed by (2) iteratively building an explanation that ultimately results in (3) resolving the gap in understanding. Importantly, the answer-making epistemic game is different from the sensemaking epistemic in terms of the framing of the task—for the answer-making epistemic game, rather than focusing on understanding the problem, there is an attempt to remember something they already know (i.e., brainstorming frame) and to provide a sufficient solution to complete the task (i.e., oral examination frame) (Russ et al., 2012; Odden and Russ, 2019b). Using the language of epistemic games, one of the key differences between sensemaking and answer-making is the target epistemic form, in which the goal of sensemaking is understanding a problem and the goal of answer-making is completing a problem. In the next section we detail how this body of literature played a role in adapting the sensemaking framework as an analytical lens.
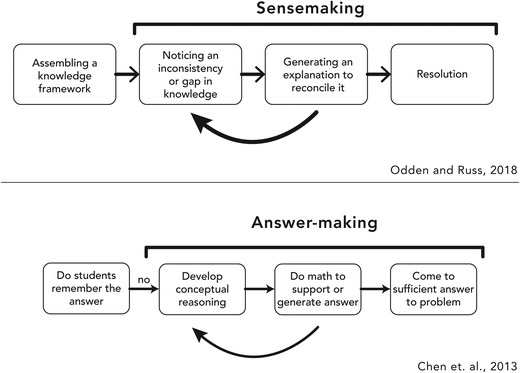 |
| Fig. 1 Sensemaking epistemic game and answer-making epistemic game adapted from Odden and Russ (2018); Chen et al. (2013), respectively. The answer-making game has been simplified and only depicts the scenario in which the reasoning leads to answer (instead of the answer leading to justification), which most closely matches the structure of the sensemaking epistemic game. | |
Methods
Research setting
Participants in the study were enrolled in a lecture-based first-semester general chemistry course at a large Midwestern University. The course was comprised of science and engineering majors, and each week students attended three 50 minute lectures, one 50 minute discussion session, and a laboratory session. Students were informed that participation had no impact on their course grade and that they could decline participation at any point throughout the study. All research activities were completed in accordance with our university's Institutional Review Board.
Activity development
The activity used for this study was part of a larger research project that focused on engaging students in developing and using models. We developed a total of six activities based on the writing and implementation guidelines outlined by the POGIL Project (The POGIL Project, 2020c). Our group's activities were developed using multiple iterations of data collection and revision. This process involved piloting within our research group, interviews with chemistry faculty familiar with POGIL for content validity, individual and group student interviews for response validity (Standards For Educational And Psychological Testing, 2012), and final implementation in discussion sections. Revisions were made to the activities after each stage of the development process. More detailed information about the validation process of the ideal gas law activity and the other activities developed can be found in our previous publication (Rodriguez et al., 2020c). For this article, we have included the complete ideal gas law activity titled “Gas Laws: Using a Dynamic Computer Simulation to Construct the Ideal Gas Law” in the Appendix.
Previously published gas law POGIL activities involved presenting students with the ideal gas law and prompting them to investigate the different relationships among variables in the expression (e.g., P, V, n, T) (Hanson, 2011; Moog and Farrell, 2017). Instead, for this study, our activity relied on students using an interactive simulation from the Concord Consortium that allowed them to investigate the relationship between variables included in the ideal gas law (e.g., temperature and pressure) as well as those included in the van der Waals equation (e.g., particle size and particle attraction) (The Concord Consortium, 2020). A reproduction of this simulation is provided below in Fig. 2. The students used this simulation to gather data to draw inferences about the influence of various variables (i.e., temperature, pressure, number of particles, particle size, particle attraction, and particle mass) on the volume of a gas within each of the pistons.
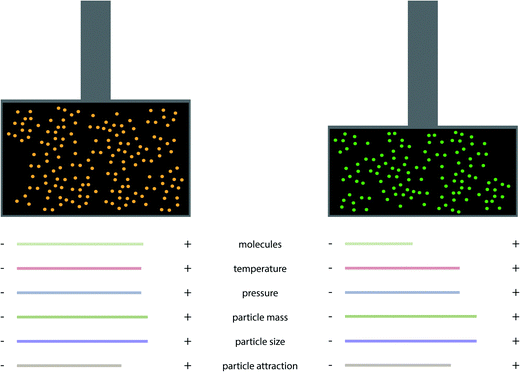 |
| Fig. 2 Reproduction of the simulation developed by the Concord Consortium and used by students in the gas law activity. Students had the ability to modify the six variables above (−, decrease variable; +, increase variable) and examine in real time. | |
The overarching question guiding students in the first learning cycle is a scenario based on the National Football League's 2014 American Football Conference (AFC) Championship Game scandal known commonly as “Deflate-gate.” This game was widely covered on the news because of reports that a team had purposefully deflated game footballs, while others argued that due to the extremely cold temperatures the footballs deflated without external influence. In the trial that followed, data about pressure and temperature were provided, making this scenario an attractive topic for an application question investigating a real-world scenario. In the exploration phase of the activity, students use the simulation to explore the effect of the number of particles, temperature, and pressure on the volume of an ideal gas. In the concept invention phase, students construct the ideal gas law and determine the value of the gas constant R. Finally, in the application phase students use the newly constructed equation to draw conclusions regarding whether the data support the idea that the New England Patriots cheated by intentionally underinflating footballs.
The second learning cycle of the activity focuses on the idea that models may be changed or different models may be necessary by having students examine the assumptions of the ideal gas law. In the exploration phase, students consider the effect of variables not included in the ideal gas law: particle mass, particle size, and particle attraction. Then, during the concept invention phase, the students predict the effect of these variables under certain conditions and complete a table that emphasizes the influence the variables may have on the ideal gas law equation under different conditions. In the final application phase, students are prompted to reason about the relationship between a real and an ideal gas.
Data collection
The interview data used for this study was collected during student group interviews conducted as part of the development process for the activity (Rodriguez et al., 2020c). There were three group interviews with a total of n = 11 students (two groups of four students, one group of three students). Each of these group interviews was conducted in about 50 minutes to mirror the time frame of a typical discussion session. Interviews were facilitated by a researcher who followed the POGIL guidelines: students working together in groups, assigned student roles (Manager, Presenter, Recorder, Reflector), and minimal intervention from a facilitator (e.g., students asked a question, students reported their answers, etc.) (The POGIL Project, 2020a). Before beginning the activity, students were presented with descriptive role cards that indicated general responsibilities for each assigned role, and in the case of the three-membered group, the Reflector and Recorder roles were combined. During the interview, we collected audio and video recordings of the student groups as well as any written work from the activity worksheets. Audio data was transcribed verbatim for analysis.
Data analysis
To help with data presentation and build a narrative of the interview data, pseudonyms were assigned for the students in each group based on their assigned role (see Table 1) (e.g., Manager names begin with M: Michael, Macy, and Melissa). Given that Group 3 only contained three students, Cassie served the role of both recorder and reflector. Analysis of the data reported here involved two steps informed by epistemic games: (1) coding using the structural components of the sensemaking epistemic game and (2) analytical memoing that focused on the ontological components of the sensemaking epistemic game, specifically the target epistemic form. For the purposes of this work, we focus less on the knowledge base, which is assumed to be chemistry ideas in this context.
Table 1 Pseudonyms used for the students in each group
|
Group 1 |
Group 2 |
Group 3 |
Manager |
Michael |
Macy |
Melissa |
Presenter |
Paula |
Penny |
Prudence |
Recorder |
Chris |
Chloe |
Cassie |
Reflector |
Faith |
Florence |
Developing a sensemaking coding scheme: identifying the structural components of the sensemaking game
Given that Odden and Russ (2018) developed the sensemaking epistemic game inductively, the first challenge in analyzing our data was finding a way to deductively use the sensemaking epistemic game description to identify cases of sensemaking. This required us to first develop an operationalizable coding scheme for the sensemaking game that we could apply to our full dataset in order to identify the instances in which students were engaging in the structural pieces of sensemaking. Therefore, we built on the work by Odden and Russ (2018) by developing more explicit code definitions that would allow us to deductively identify the structural components of the sensemaking game: building a knowledge framework, entry condition, explanation building, and resolution.
As part of this process, we developed code definitions related to determining what constituted as an entry condition in the context of our activity. Odden and Russ (2018) described the entry condition as a point in the dialogue where a student notices an inconsistency between old and new knowledge, which transitions the students into a sensemaking game with the ultimate goal being to resolve the inconsistency. For our study context, we initially made the assumption that the questions of the activity serve as the gap in understanding students were trying to resolve; therefore, students vocalizing these questions served as a sufficient entry condition. Moreover, because we considered restating the question to serve as the entry condition, we did not require building knowledge framework to be a necessary precursor to sensemaking and this element was not included in our analysis. This was done because we found that students did not gather initial knowledge before beginning a question, but rather the question served as the entry condition, initiating the sensemaking process. The extent to which these assumptions are valid were subsequently addressed as part of the second layer of analysis, discussed in the next section.
The final code definitions are presented in Table 2 and once the definitions were refined; they were used as a deductive coding scheme by the primary researcher to identify potential instances of sensemaking across each group. This analysis was done on a question-by-question basis, with each question serving as the unit of analysis (Campbell et al., 2013). For the purposes of this study, we considered a potential episode of sensemaking to be when students engaged in the following steps (in order): entry condition, explanation building, and resolution.
Table 2 Final code definitions developed for the identification of sensemaking. These codes were adapted from the sensemaking epistemic game description provided by Odden and Russ (2018) with the exception of building a knowledge framework, which we did not include in our coding scheme
Sensemaking codes |
Code |
Definition |
Entry condition |
Initial voicing of a gap in understanding or the question itself as a complete sentence which initiates a period of explanation building. Entry condition often shifts pace of dialogue and focuses the group's discussion. Not a question related to the purpose of a problem or questions that serve to sustain explanation building. Only one entry condition can be coded for each question. |
Explanation building |
Students work together to gather data in order to build an explanation specifically intended to resolve the gap in understanding. Iteratively critiquing, questioning, and building off of each other as they construct a coherent explanation. Interruptions by the facilitator are not counted in explanation building. |
Resolution |
Explicit voicing of a final conclusion to the entry condition the group constructed on their own which is not followed by more critiquing/construction by others. Confident response not posed as a question or with hedging language (I feel like, maybe, probably etc.) |
Analytic memoing: identifying the epistemic form of the sensemaking game
The coding process discussed above provided a way to identify the specific moves associated with the sensemaking epistemic game and served as potential instances of students’ sensemaking. However, we wanted further evidence that students were engaging in sensemaking, which is important in order to justify the assumption that providing a question in the activity is sufficient as an entry condition for the process of sensemaking. Thus, after determining potential episodes of sensemaking based on the structural components of the epistemic game, we focused on providing evidence for students’ epistemic form, that is, considering the nature of the claims we can make regarding students’ goal for the task—to provide an answer or to make sense of the problem-solving scenario provided. To this end, we found it productive to move beyond binary classifications, instead focusing on the extent to which we can provide evidence for sensemaking. This approach was informed, in part, by Russ et al.'s (2008) approach toward analyzing students’ mechanistic reasoning, which focused on enumerating characteristic features of mechanistic reasoning (e.g., describing the target phenomena, identifying setup conditions, identifying entities, etc.), with a greater number of features providing a stronger case that students are engaging in mechanistic reasoning. One of the affordances of this approach is the acknowledgement of the productive features of students’ discourse and the identification of areas where students may need more support. Based on previous literature, we developed a list of distinguishing features related to sensemaking, which we used to provide evidence for the sensemaking epistemic form: (1) real-world phenomena, a feature of the question context that allows students to draw connections to their experiences; (2) robust explanation building involving collaborative construction (student discussions that build on each other's ideas), critique (in which students evaluate, assess, or question the plausibility of a peer's claim), and vexing questions (the repeated emergence of a question that guides and sustains explanation building); and (3) quality of explanation, in this context, evaluated based on completeness of students’ written work in terms of claim, evidence and reasoning.
In order to characterize the quality of students’ explanations (3), we used the framework of scientific explanations developed and outlined in McNeill et al. (2006). This aligned well with the sensemaking epistemic game which is focused on students’ understanding phenomena because, as outlined by McNeill et al. (2006), scientific explanations deal with students working to explain how or why a phenomena occurs. Within this model, an explanation contains: a claim (i.e., conclusion), which is then supported by evidence to support the claim, and finally reasoning to connect the claim to the evidence. Within our dataset we required that in order to code a passage for reasoning the response must have already been coded with claim and evidence. The written responses analyzed were those collected specifically from the students who served as the Recorder, whose role was to document student group responses as they worked through the activity.
As discussed by Birks et al. (2008), engaging in the process of memoing is a useful analytic strategy for extracting meaning from qualitative data. We found the memoing process to be more productive and generative (as opposed to coding) because of the small sample size and the idiosyncratic nature of group discussions. Moreover, the level of detail provided by memoing in contrast to coding, afforded rich descriptions of students’ nuanced discussions. For our dataset, two researchers first used a line-by-line analysis for a subset of questions, involving discussing, annotating, and recording memos related to students’ discourse. In particular, we focused on the specific features related to sensemaking such as real-world context, robust explanation building, and written explanations as discussed above. Following this, the primary researcher expanded this analysis to encompass more questions from the dataset in order to provide examples and contrasting cases for each feature. To reiterate, the more of these features that are exhibited in students’ discussions, the stronger the claims we can make that students are engaging in sensemaking, and subsequently, the more convincing the claims made that the activity questions are sufficient entry conditions to initiate student engagement in sensemaking.
Interrater reliability and agreement
To establish reliable code definitions, we used an iterative process to develop and apply codes related to sensemaking and scientific explanations. For the sensemaking and scientific explanation codes, the first author coded the complete dataset and a second independent researcher coded 15% of the dataset. Kappa values were calculated using Nvivo software, with an initial value of 0.81 for the sensemaking codes and 0.61 for scientific explanations which refer to “strong” and “moderate” agreement respectively (McHugh, 2012). Next, to further refine code definitions, the two researchers reconciled any differences in coding for both scientific explanations and sensemaking codes and reached a final consensus on code definitions. A second round of coding was done with an additional 10% of the dataset for the updated scientific explanations codes, which resulted in a Kappa value of 0.93.
Findings
Our reported findings begin with an overview of the general trends and results elucidated from our analysis related to the structural components of the sensemaking game. Following this, we provide evidence to support claims made regarding student engagement in the sensemaking game by targeting features that allude to the ontological components of the game, specifically aiming to draw inferences about students’ epistemic form.
Overview of student engagement in the structural components of sensemaking
To begin addressing our research question, we first identified potential cases of students engaging in sensemaking by applying our codes for the structural components of the sensemaking game to each group's discourse as they completed the activity. Fig. 3 summarizes the presence of sensemaking codes for each question (1–16) with complete instances of sensemaking highlighted using an orange box (for reference, see the Appendix for a complete list of activity questions). The groupings in Fig. 3 indicate the application of codes to specific problems based on students’ discussion (entry condition, explanation building, resolution), and if a question has all three codes assigned, this could suggest student engagement in sensemaking. Of note, the codes depicted in Fig. 3 do not represent time on task or the time spent within a specific code but serve to show the collective occurrence of codes. Furthermore, if students moved on from a question but later revisited the question, we took this into account, which is reflected in codes indicated in Fig. 3. For example, if students only participated in explanation building, moved on to another question, but later circled back and voiced a resolution, this instance would be coded as explanation building and resolution. Alternatively, if they revisited a question to continue explanation building, this would remain coded as explanation building. Notably in Fig. 3, Group 3 did not complete questions 13–16 due to time constraints of the interview. Lastly, before discussing the observed trends, to better illustrate the application of sensemaking codes, in Fig. 4 we have provided an example of student discourse that meets the criteria for application of the codes related to the structural components of sensemaking and therefore serves as a candidate for potential engagement in sensemaking. Taken from Group 1 completing Question 8, the group first voiced the question (entry condition), then worked together to resolve the question (explanation building), and lastly stated the group's final answer to the question (resolution). Building on this initial summary of data, in the sections that follow we will discuss general trends in the data related to the structural components of sensemaking, followed by specific analyses of features used to infer students’ epistemic form on questions where the structural components of the sensemaking game were observed.
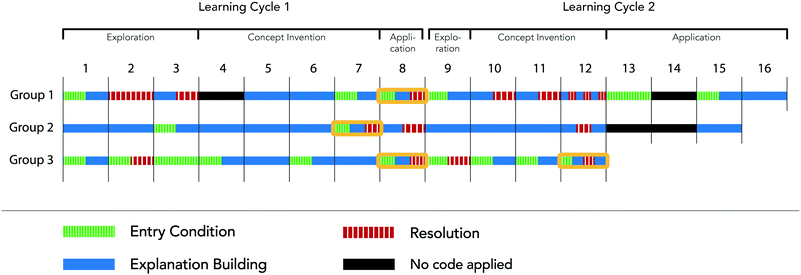 |
| Fig. 3 Diagram providing an overview of student engagement in the structural components of sensemaking for each question (1–16) in the gas law activity. Note that this is identified based on characterization of students’ discourse as involving (1) entry condition, (2) explanation building, and (3) resolution. Questions containing all three codes, suggesting potential for engagement in sensemaking, are highlighted using an orange box. | |
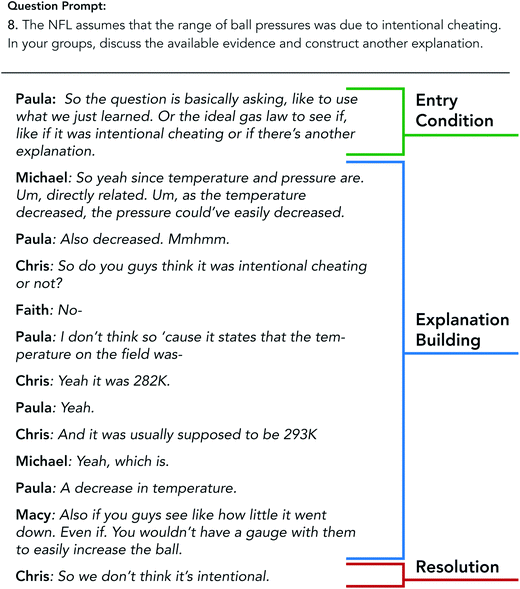 |
| Fig. 4 Group 1 discourse while working on Question 8 of the activity representing the structural components of sensemaking: entry condition, explanation building, and resolution. | |
Trends among group engagement in the structural components of the sensemaking process
The summary provided in Fig. 3 allowed us to notice general trends related to each group's progress through the activity. Overall, Group 3 had the highest number of entry conditions among the groups, which commonly, involved the Manager (who is responsible for guiding the group's work) restating the question before the group began building their explanation. Similarly, Group 1 had the highest number of resolutions, which were often led by the Recorder (who is responsible for documenting all the answers discussed by the group and recording the final conclusions on the provided worksheet). Examples of these entry conditions and resolutions are provided in Table 3, demonstrating that these instances were often voiced by a single student, but also could involve a combination of dialogue from multiple students. Lastly, the presentation of data in Fig. 3 indicates that Group 2 spent the majority of the activity in the explanation building phase, without having an explicit reference to the goal of the problem (entry condition) or a final statement about the group's general consensus before advancing to the next question (resolution).
Table 3 Examples of entry conditions generally voiced by the Manager (Melissa and Michael) and resolutions generally voiced by the Recorder (Chris and Cassie)
Entry condition
|
Melissa:
Use the simulation to explore the relationship between volume and temperature. How does the volume change when you increase the temperature?
|
Melissa:
Using your observations from 1 through 3 to answer the questions below. Is volume proportional or inversely proportional to the number of molecules.
|
Michael:
So it says, um, the relationship between the volume and the number of molecules.
|
Paula:
Mmhmm. When you increase them or when you decrease them.
|
Resolution
|
Chris:
High pressure made volume decreases, which creates the molecule attraction more.
|
Chris:
So we don’t think it's intentional.
|
Prudence:
Okay, yeah I got the 82
|
Cassie:
point 0-8-2. Okay.
|
It is also important to discuss the structure of a POGIL activity—which was used in the design of the activity—and how this potentially influenced the data seen in Fig. 3. As mentioned previously, the learning cycle within a POGIL activity is divided into three phases: exploration, concept invention, and application, which are labled in Fig. 3. These phases have different goals: recognizing trends in data during exploration, developing a definition during concept invention, and applying what they have learned during application. First, we see that students working through the exploration phases did not involve all the structural components of the sensemaking game and there was generally a lack of explanation building for the exploration phases of the learning cycles, which often involved students gathering data using the interactive simulation. Once students had become familiar with the simulation, they were able to target their data collection and simply note their observations as answers to the question without engaging in a collaborative period of explanation building. Contrastingly, we see that Questions 7 and 12 in the concept invention phase and Question 8 of the application phase involved the structural components of sensemaking, which is fitting because this is where students construct and apply new concepts. This trend of limited engagement in sensemaking for the exploration phase and more potential for engagement during the concept invention and application phases, supports evidence in previous work that involved analyzing the effect of question prompt on the depth of students’ generated arguments. Kulatunga et al. (2014) noted that the directed questions in the exploration phase did not elicit strong argumentation building by students, but rather served the role of supporting students in scaffolding arguments in subsequent questions. Similar to Kulatunga et al. (2014), we assert that although the exploration phase tended to not engage students in sensemaking, these initial questions provide students the necessary information needed to engage in explanation building in later questions, thus, supporting future sensemaking.
In addition, in Fig. 3 it is notable that Questions 13 and 14 did not have any codes applied. For context, the question preceding this, Question 12, prompted students to predict the effect of variables not included in the ideal gas law (particle attraction, particle size). In Questions 13 and 14, students were then asked to make similar claims as they did in Question 12. Due to the similar material, students often quickly voiced an answer that was agreed upon by the entire group without a period of explanation building.
Building a case for engagement in sensemaking: evidence for students’ epistemic form
As discussed above, our coding scheme specifically addressed the structural components of the sensemaking game in order to identify potential participation in sensemaking. However, this does not provide sufficient evidence for students’ sensemaking in the way that it does not address the overall target epistemic form that guided their inquiry. Below we discuss features from extant literature to further support our argument that students engaged in the process of sensemaking while completing the activity.
The question connected to a real-world phenomenon.
The first piece we use to infer students’ epistemic form is based on the definition of sensemaking proposed by Odden and Russ (2019a) and a distinction made be Chen et al. (2013) with regards to the difference between answer-making and sensemaking. Odden and Russ (2019a) argued that sensemaking involves discussing the underlying mechanism of a phenomenon. This can be supplemented with a Chen et al. (2013) notion that sensemaking with Chen et al. (2013) notion sensemaking is distinct from answer making because students are working to make sense of the world when they are engaging in sensemaking. That said, our first piece of analysis for identifying students’ epistemic form is based on whether or not the context of the question is connected to a real-world phenomenon, which allows students to draw connections to their experiences and prior knowledge. Consider the student dialogue provided in Fig. 5 for Question 7 and Question 8 taken from the explanation building of Groups 2 and 3. Although this dialogue is taken from the explanation building phase of their discourse, both groups completed the structural components of the sensemaking game for the respective questions (see Fig. 3 for overview of structural components code application). Interestingly, for both of these questions, group dialogue involved the structural components of sensemaking; however, the question prompts differ in terms of the context. Although Question 7 involves real-world data for variables like pressure and temperature, the connection is not made explicit, as is the case for Question 8, which involves considering the pressure and temperature of footballs indoors and outdoors. Based on the definition of sensemaking by Odden and Russ (2019a), Question 8 provides a greater opportunity for students to use scientific principles to explain a real-world context. Further evidence of this is seen in terms of what students are expected to do for each question and the resulting student discourse. In this example, Group 2 simply had to plug values into an equation to find the gas constant, without drawing any connection to the data or working to reason about the value. This highlights an example of students engaging in the structural components of sensemaking but lacks connection to a real-world phenomenon. This is different, however, in the dialogue presented in Fig. 5 from Group 3 as they participated in explanation building while completing Question 8. In addition to the context of the question relating to a real-world phenomenon, with data rooted in that context, the students connected their own experiences to the problem (“… just guessing off of what I know from Massachusetts and being in the middle of winter”), which helped them make sense of the data presented. By framing the question around a real-world context, students are more likely to engage in the process of sensemaking (Question 8). In contrast, questions framed like Question 7 tend to limit students to answer-making because they are unable to connect the problem to a tangible phenomenon or individual experiences, and thus, have no need to make deeper connections.
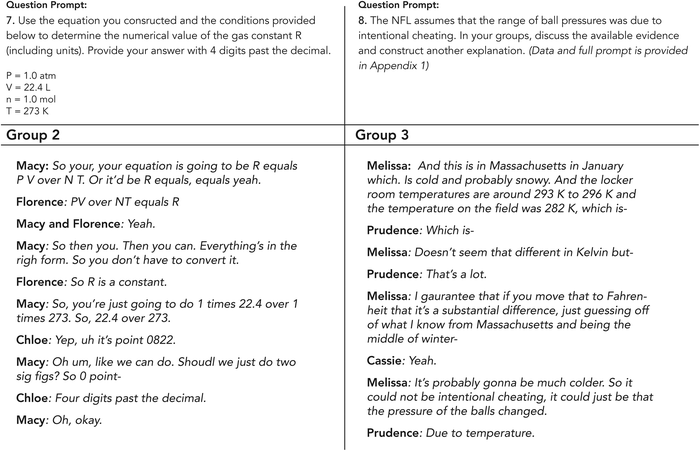 |
| Fig. 5 Dialogue of Group 2 as they completed Question 7 and Group 3 as they completed Question 8. Both of the groups engaged in the structural components of sensemaking for their respective questions. The real-world connection of Question 8 allowed the students to make deeper connections with the data and help them engage in the process of sensemaking. Both episodes are taken from the explanation building phase for the respective groups, for a question in which they completed the structural components of the sensemaking game. | |
Students engaged in robust explanation building.
Next, we will discuss students’ engagement in what we call robust explanation building, which further suggests that the groups engaged in the process of sensemaking. We again drew on the existing literature by looking for instances in which students were constructing and critiquing as well as looking for what Odden and Russ (2019b) call vexing questions. Construction and critique were noted by Odden and Russ (2018) as discourse and argumentation markers of the sensemaking epistemic game and have been discussed by others as important features of students’ sensemaking (Ford, 2012). Building off of this work, we specifically looked for the ways in which students collaboratively constructed and critiqued each other's explanations as part of the explanation building process. Additionally, we looked for the presence of vexing questions, or questions that students felt compelled to answer, and thus sustained the sensemaking process (Odden and Russ, 2019b). Moreover, we observed instances in which the activity question functioned as a vexing question, further supporting our assumption that the activity questions can serve as a sufficient entry condition into sensemaking.
For analysis of robust explanation building, consider the dialogue presented below in Fig. 6. This contains pieces from the explanation building of Group 3 completing Question 12 (far left), Group 1 completing Question 8 (middle), and Group 2 completing Question 8 (far right). For these, all groups engaged in the structural components of the sensemaking game for their respective questions. Beginning on the far left with Group 3, the group engaged in robust explanation building that leveraged each group member's ideas as they constructed an explanation for the question. This is exhibited in the collaborative nature of their dialogue, with each group member voicing their thoughts and building off of each other's contributions. Additionally, we see the use of critique from the group such as Cassie saying “I’m thinking more mass for volume” which led the group to their ultimate resolution in the final line. Similarly, the dialogue of Group 1 in the middle of Fig. 6 highlights productive construction of an argument. Michael began with an explanation that connects many of the final elements of their explanation, but the entire group did not fully contribute to the explanation building until Chris voiced the question “So do you guys think it was intentional or not?” This question acted much like a vexing question that connected back to the question prompt and also served as an implicit critique to Michael, acknowledging that the group had not fully answered the question. Chris’ question also shifted the group into a period of construction, where they collaborated to gather correct data to construct an explanation to the question. These two examples showcase instances of groups engaging in productive discourse that included constructing and critiquing in order to reach a consensus (resolution). As a disclaimer, with the example of Group 3 completing Question 12, we would like to acknowledge that this dialogue reflects non-normative scientific reasoning. Although working to construct an explanation for the question, the group ultimately reached an incorrect conclusion. This represents a limitation of the sensemaking framework in that it focuses more on the process of explanation building leading to a resolution, rather than emphasizing the ultimate product of sensemaking.
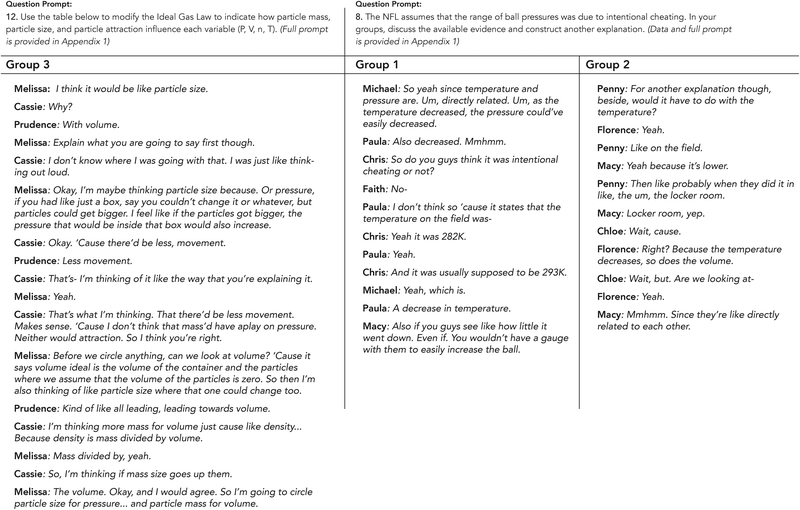 |
| Fig. 6 Dialogue of Group 3 as they completed Question 12 and Groups 1 and 2 as they completed Question 8. All of the groups engaged in the structural components of sensemaking for their respective questions. Groups 3 and 1 engage in productive, robust explanation building that includes elements construction and critique as well as evidence of the activity question being a vexing question for the students. | |
For contrast, while the first two episodes of dialogue in Fig. 6 represent productive explanation building, the final piece of dialogue from Group 2 highlights an unproductive instance of explanation building where the group is not engaged in construction and critique. The group began by attending to useful data related to the temperature, but the group did not allow for potential critiques, such as comments from Chloe (“wait, cause” and “wait, but are we looking at-”), limiting the overall depth of their explanation building. Additionally, unlike the first example from Group 3, the dialogue of Group 2 included short snippets of dialogue, with minimal reasoning behind their contributions. Whereas Melissa of Group 3 used a concrete hypothetical example of molecules in a box getting larger which would cause an increase of pressure, the dialogue of members in Group 2 was much shorter chunks of dialogue and pertained more to specific pieces of data that was not further justified with reasoning.
Lastly, in line with the importance of thorough explanation building as part of the sensemaking process, our data show that students were influenced by the language of the question prompt throughout their explanation building. Expanding on this, we analyzed all questions that explicitly asked students to justify answers with an explanation (8, 10, 11, 15) and searched for instances in which wording from the question prompt were present in their explanation building. For example, “explain your reasoning” for Questions 10, 11, and 15 and “discuss the available evidence and construct another explanation” for Question 8. Table 4 summarizes these instances for each. The most striking observation from this analysis was the ways the question prompt was exhibited in student dialogue for Question 8 that was not seen to the same extent for the other questions. Students typically referenced specific wording from Question 8 such as evidence and explanation to support their construction of an explanation. Importantly, although instructors often include “explain your reasoning” at the end of a question to prompt student's explanations, these data suggest that scaffolding the question prompt with more explicit language that indicates how students should accomplish this (e.g., “discuss the available evidence and construct another explanation”) grounds the discussion and helps promote more robust explanation building for the group.
Table 4 Instances of student dialogue throughout data set that mirrored language within the question prompt
|
Question 8: in your groups, discuss the available evidence and construct another explanation. |
Questions 10, 11, and 15: explain your reasoning. |
* Indicates we did not observe instances of students’ dialogue involving the language from the question prompt as they completed the question. |
Group 1 |
* |
* |
Group 2 |
Penny: So basically our evidence could be that the range as which is accepted in the official rules |
Florence: We gotta reason yeah. Have to do the reasoning why does it do that (Question 10) |
Chloe: For another explanation though |
Group 3 |
Melissa: But 1 know that this is the explanation it wants us to give because all of the background evidence |
* |
Prudence: So the evidence would be |
Students constructed complete scientific explanations.
For the final piece of evidence to suggest students were engaging in the sensemaking game, we analyzed students’ written responses using the scientific explanations framework. Building on the work of McNeill et al. (2006) that posits scientific explanations serve to explain how or why a phenomenon occurs, we looked for the presence of complete scientific explanations as a justification for inferring students’ epistemic form. Of the instances in which students engaged in the structural components of sensemaking (Questions 7, 8 and 12), Question 8 was the only question that required a written explanation by the students. Below in Fig. 7 are the final written explanations with codes for scientific explanations alongside the group's structural components of the sensemaking game. In Fig. 7 we see that Groups 1 and 3 provided complete scientific explanations whereas Group 2 only provided a claim with evidence. This finding fits well with our analysis of group's dialogue for Question 8 where Groups 1 and 3 engaged in more robust explanation building compared to Group 2. In the process of robust explanation building, Groups 1 and 3 attended to more specific data like temperature and collaborated to develop deeper reasoning behind their explanations which was ultimately reflected in their written responses. We argue that questions requiring written explanations help solidify student reasoning, requiring them to explicitly communicate their final answer in a succinct way.
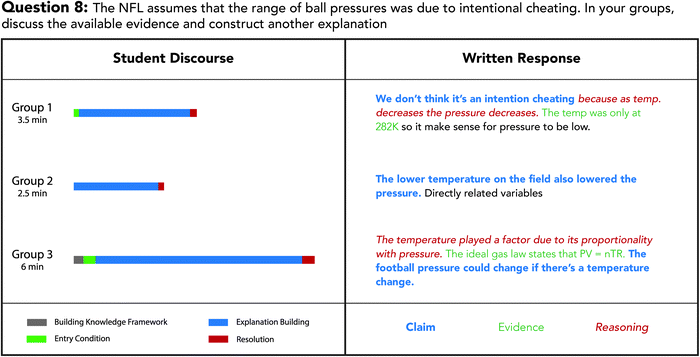 |
| Fig. 7 Sensemaking map with length representing time spent in a specific phase and written response for Question 8 analyzed using the scientific explanations protocol outlined in the Methods. | |
Summary: student engagement in sensemaking.
Supplementing the presence of the structural components of the sensemaking game with evidence for students’ epistemic form helps build a case for student engagement in the process of sensemaking. Although it is challenging to draw inferences about students’ expectations and framing of the task, triangulation of different types of evidence is a productive approach. Based on our analysis, in addition to meeting the criteria for the structural components of sensemaking, we posit that the more we observe the hallmarks of sensemaking as students work through a given task (real-world context, robust engagement in explanation building, and construction of complete scientific explanations), the more we can substantiate claims that students are participating in sensemaking instead of answer-making.
For the questions in which students participated in the structural components of sensemaking, we summarized the analysis in Fig. 8, with the representation indicating whether we observed the indicated features of sensemaking (a green checkmark) or not (a red X). Overall, our analysis provides evidence for the extent to which students participated in the process of sensemaking, showing that there is more evidence to make the claim that Group 1 and Group 3 successfully engaged in sensemaking while completing Question 8. Although to a lesser extent, Group 3 also engaged in sensemaking for Question 12, and as explained above, the group came to a scientifically non-normative resolution, highlighting a limitation of the sensemaking framework. Finally, Group 2 completing Question 7 highlights an instance of a group participating in the structural components of the game with little evidence for the sensemaking epistemic form. This suggests the group's discourse may have been more aligned with the epistemic form of the answer-making game, that is, working to simply provide the correct answer (Chen et al., 2013).
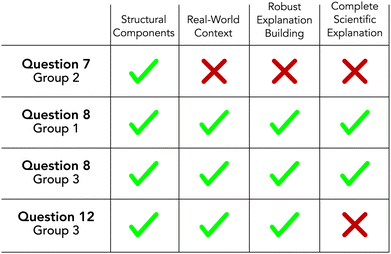 |
| Fig. 8 Summary of the present components for inferring epistemic form (real-world context, robust explanation building, and complete scientific explanations) for questions in which groups completed the structural components of the sensemaking epistemic game. This suggests that Groups 1 and 3 engaged in sensemaking for Question 8 and to a lesser extent Group 3 engaged in sensemaking in Question 12. | |
Limitations
This work reflects our analysis of student engagement in the process of sensemaking, focusing on the interactions that occurred as three groups of students worked through a collaborative learning activity. As a limitation of this work, it should be noted that the data was collected for a small sample in the context of clinical interviews as opposed to a classroom environment. Thus, claims regarding generalizability of results are limited. Nevertheless, the data collected herein was part of a larger project that also involved implementation of activities in a discussion section with similar student engagement noted. The decision to emphasize the data collected in a clinical context was based on the flexibility afforded for follow-up questions and time-on-task that would not be feasible in a classroom, providing the space to collect data that would help address our research question.
With regards to the gas law activity used for data collection, although the activity was designed using the principles of a POGIL activity, the activity has not been officially endorsed by the POGIL Project. Furthermore, with regards to the fidelity of implementation, the facilitator was a graduate student that had not been through formal training as a POGIL facilitator. Nevertheless, our implementation highlights the broad utility of these activities, given that universities generally rely on graduate teaching assistants to lead group activities like the gas law activity used for this study.
As another limitation, we found the sensemaking framework difficult to apply in practice, which involved making modifications and changes in order to develop a context-specific definition of sensemaking. Although our description of how to apply sensemaking was operationalized for our dataset, we posit that our analysis can be applied to other contexts with minor changes (e.g., what counts as an “entry condition” might change based on the data analyzed) and we found the framing related to finding evidence to build a case for sensemaking to be a productive direction for future work. Furthermore, we argue that sensemaking as a construct and what can be categorized as sensemaking depends on the context, requiring researchers to be explicit with respect to their methodological and analytical decisions.
Conclusions and implications
Sensemaking as a method of analyzing student problem solving
We used the sensemaking epistemic game described by Odden and Russ (2018) as a lens for characterizing general chemistry students discourse as they worked collaboratively to solve problems. A goal of our work was to augment the extant literature surrounding sensemaking by providing an approach for assessing the extent to which students engaged in the process of sensemaking. This analysis included analyzing for the structural components of the sensemaking game followed by a layer of analysis targeted at inferring a group's epistemic form (in order to distinguish it from the answer-making epistemic game). In terms of providing evidence regarding students’ epistemic from we looked for three factors, including: a question's connection to a real-world context, students participating in robust explanation building (construction, critique, vexing questions), and finally students constructing complete scientific explanations. We argue that this process helped to further unpack the process of sensemaking and provides a framework for future researchers to assess students’ engagement in sensemaking. Our work outlines an approach researchers and instructors can use to assess the process of sensemaking and distinguish this process from answer-making, which has proven to be an elusive task (Chen et al., 2013).
Within our analysis, we made an assumption that an activity question can successfully serve as an entry condition into the sensemaking process. Given our in-depth analysis of the data indicating that students engaged in the markers of sensemaking discussed in the literature, this suggests that restating a question can serve as the entry condition for an episode of sensemaking. This differs from the proposed definition of an entry condition where students explicitly recognize a some gap in knowledge, resulting in the unfolding of the subsequent moves of the sensemaking game (Odden and Russ, 2018). Based on our analysis, we argue that future work involving the sensemaking framework can include restating a question as a satisfactory entry condition. This serves as a lower threshold for observing engagement in sensemaking that is relevant for commonly used pedagogical approaches such as POGIL. Furthermore, there was evidence that students treated the activity questions similarly to the idea of vexing questions proposed by Odden and Russ (2019b) where students reiterated the question, resulting in further explanation building.
Next, we would like to address a question posed by Odden and Russ (2019b) regarding whether sensemaking can occur for students learning new material. Given the design of the activity was rooted in the learning cycle, which is intended to be a first introduction to content, this exploratory work suggests students can engage in the process of sensemaking while learning new material, with the qualifier that the questions need to be scaffolded intentionally to support this process. Our analysis provided evidence that Groups 1 and 3 participated in the process of sensemaking while completing Question 8; nevertheless, claims regarding sensemaking related to new material are limited since we would need more data regarding whether or not this was in fact students’ first encounter with the material (i.e., data regarding prior coursework would be necessary). More work can be done in the context of POGIL and other contexts to further investigate this aspect and uncover students’ sensemaking of new material.
Lastly, in terms of future work concerning the sensemaking framework, we encourage researchers to use the framework to investigate student's reasoning across other concepts in chemistry and across science. As we highlighted, POGIL activities and collaborative learning that is rooted in the learning cycle present a useful environment to investigate students’ sensemaking related to the development of new concepts, which the community can build on with our study. Additionally, although outside the scope of this manuscript, we noted a pattern among the facilitator's discourse and its effect on how students engaged in sensemaking, with the facilitator discourse involving two general categories of interjections: (1) discourse that was able to quickly guide student reasoning to facilitate further explanation building and (2) discourse that diverged away from student-led discussion, resulting in a discussion dominated by the instructor. This emergent theme compliments the existing literature that previously noted the importance of the facilitator in the classroom (Daubenmire and Bunce, 2008; Kulatunga and Lewis, 2013; Warfa et al., 2014; Becker et al., 2015; Daubenmire et al., 2015; Moon et al., 2016; Stanford et al., 2016, 2018) and we suggest future work is needed that specifically focuses on leveraging the sensemaking epistemic game as a means of analyzing the role of the facilitator in supporting student learning.
Supporting student engagement in the process of sensemaking
For researchers, we argue that the analysis covered in this work serves as a useful gauge for the assessment of student engagement in sensemaking, and for practitioners, this framework provides insight regarding how to construct activities and questions that engage students in sensemaking. The first of these features concerns students’ engagement in the structural components of sensemaking (entry condition, explanation building, and resolution). In general, we found that students most often participated in explanation building without an explicit voicing of an entry condition or resolution. Our data further suggest the importance of roles, specifically the manager (guides the group's work) and recorder (records the group's consensus to a problem) who influence the presence of entry conditions and resolutions made by a group. Therefore, a suggestion to help promote sensemaking in the classroom would be for facilitators to further encourage the use of assigned roles, specifically reinforcing that the manager should read the question aloud and the recorder should verbally summarize the group's final response prior to recording the answer on paper. While a seemingly simple suggestion, this is an important aspect of group work because explicitly voicing an entry condition serves as a way to direct the group as they build an explanation and explicitly stating a resolution ensures that all members of a group agree on the ultimate explanation they built.
With regards to the non-structural pieces that we used as a way to assess students’ epistemic form, real-world context and construction of scientific explanations specifically deal with the structure of a task or question. Therefore, another connection to practice for those wanting to engage students in sensemaking would be to relate questions to real-world examples, as was done in Question 8 of our activity. As seen in our data, this provided the potential for students to connect the presented data with their personal experiences. Furthermore, by requiring a written explanation, the group is asked to formally outline their argument which can also further engage students in the process of sensemaking. We therefore suggest instructors use questions that ask students to provide written explanations as part of the question. This is of particular importance given constructing explanations and engaging in argument from evidence are highlighted as critical science practices under the Next Generation Science Standards (National Research Council, 2012).
Finally, in addition to asking students to provide their reasoning for constructed response questions, explicit scaffolding is necessary to engage students in robust explanation building. While the other aspects above reflect a binary characterization of a question (i.e., the question is connected to real-world context or is not), depth of engagement in explanation building exists more as a spectrum for potential student participation. We noted evidence that students often used language from specific question prompts such as in Question 8, “discuss the available evidence and construct another explanation”. In contrast, prompts using the generic “explain your reasoning” tended to not provide a strong scaffold for students to construct explanations. We argue that by using more scaffolded question prompts similar to Question 8—prompting that explicitly communicates how we want to students to explain their reasoning—can serve to better support students’ robust explanation building and promote engagement in sensemaking. Using this work, we can construct questions that prompt students to go beyond the simple task of providing an answer and engage them in the process of sensemaking while they develop a response.
Conflicts of interest
There are no conflicts to declare.
Appendix
Gas laws: using a dynamic computer simulation to construct the ideal gas law
Model: dynamic computer simulation.
In the first part of this activity, your team will use a computer simulation of molecular-level gas behavior to explore the relationships between volume, temperature, pressure, and number of molecules. For this activity, you will only need one computer. To get started, have your group open the following link in a browser window:
http://mw2.concord.org/tmp.jnlp?address=http://mw.concord.org/public/student/gaslaws/gaslab.cml
Exploration.
1. Use the simulation to explore the relationship between volume and number of molecules. How does the volume change when you increase the number of molecules? How does the volume change when you decrease the number of molecules?
2. Use the simulation to explore the relationship between volume and temperature. How does the volume change when you increase the temperature? How does the volume change when you decrease the temperature?
3. Use the simulation to explore the relationship between volume and pressure. How does the volume change when you increase the pressure? How does the volume change when you decrease the pressure?
4. In the next section you will be prompted to consider proportional and inversely proportional relationships. To practice making these connections, indicate if the relationship provided in the boxes below between the variables volume (V) and a variable A is proportional (i.e., V ∝ A) or inversely proportional (i.e., V ∝ 1/A).
|
Presenter: Report answers for Questions 1–3 to instructor before moving onto the next questions. |
Concept invention.
5. Use your observations from Questions 1–3 to answer the questions below.
a. Is volume proportional or inversely proportional to the number of molecules? (Circle one)
b. Is volume proportional or inversely proportional to temperature? (Circle one)
c. Is volume proportional or inversely proportional to pressure? (Circle one)
d. Fill in the expression below to indicate how volume is related to each variable. Is the relationship proportional (e.g., the variable P belongs in the numerator box, P) or inversely proportional (e.g., the variable P belongs in the denominator box, 1/P)? Use V for volume, n for number of molecules, T for temperature, and P for pressure.
6. The expression above indicates volume is proportional (α) to the variables listed, not equal (=). If the right side of the expression is multiplied by the constant R, then the equation becomes the Ideal Gas Law, which indicates the relationship between variables used to describe a sample of gas. Using this information and the equation written above, write the Ideal Gas Law (in terms of volume) in the box below.
|
Presenter: Report answer for Question 6 to instructor before moving onto the next questions. |
7. Use the equation you constructed and the conditions provided below to determine the numerical value of the gas constant R (including units). Provide your answer with 4 digits past the decimal.
P = 1.0 atm
V = 22.4 L
n = 1.0 mol
T = 273 K
Application
Tom Brady and the New England Patriots won the 2014 American Football Conference Championship (AFC) amid a scandal that has become known as “Deflate-gate.” Brady and the Patriots were accused of under-inflating footballs during the AFC Championship game, which would make the balls easier to grip, throw and catch, especially in cold or rainy conditions. The following information was included in the NFL's investigation into the event:
• The official rules of the NFL require footballs to be inflated to a gauge pressure between 0.85 to 0.92 atm when measured by the referees; the rules do not specify the temperature at which measurement is to be made, but referees typically do this in the locker room (293–296 K).
• The AFC championship conference took place in Foxborough, MA on Jan 18, 2015. The temperature on the field was 282 K.
• The pressure of the footballs was measured on the field at halftime; the following measurements were recorded. All measurements are in atm.
1.82 |
1.76 |
1.78 |
1.75 |
1.78 |
1.81 |
1.84 |
1.79 |
1.77 |
1.74 |
1.77 |
|
Use the evidence above and your understanding of the Ideal Gas Law to answer the following questions.
8. The NFL assumed that the range of ball pressures was due to intentional cheating. In your groups, discuss the available evidence and construct another explanation.
Exploration.
9. Three variables included in the simulation do not appear in the Ideal Gas Law equation: particle mass, particle size, and particle attraction.
a. Use the simulation to explore the relationship between volume and particle mass. What changes, if any, do you observe in volume as particle mass increases?
b. Use the simulation to explore the relationship between volume and particle size. What changes, if any, do you observe in volume as particle size increases?
c. Use the simulation to explore the relationship between volume and particle attraction. What changes, if any, do you observe in volume as particle attraction increases?
Concept invention.
10. Under what conditions for temperature will these new variables (e.g., particle attraction) be have more of an effect? Explain your reasoning.
High Temperature or Low Temperature (Circle one)
11. Under what conditions for pressure will these new variables (e.g., particle attraction) have more of an effect? Explain your reasoning.
High Pressure or Low Pressure (Circle one)
|
Presenter: Report answers for Questions 10 and 11 to instructor before moving onto the next questions. |
12. Use the table below to modify the Ideal Gas Law to indicate how particle mass, particle size, and particle attraction influence each variable (P, V, n, T). To illustrate, the final row, temperature (T), has been completed for you.
e. Column 1 shows the ideal gas law which has been solved for the variable of interest for each row. You do not need to do anything in Column 1.
f. For Column 2, circle the variable that will influence P, V, n, or T under non-ideal conditions. For the completed row (T), none of the variables influence temperature (option iv).
g. For Column 3, circle the operator (+, −) to indicate how you think the equation will change in “real” (non-ideal) conditions given the variable selected in Column 2. In the completed row (T), since none of the variables influence temperature, Treal = Tideal, so the variable d was crossed out and replaced with a zero.
Application
13. The actual measured pressure (Preal) is larger or smaller (circle one) than expected (Pideal).
14. The actual measured volume (Vreal) is larger or smaller (circle one) than expected (Videal).
15. Would you expect helium (atomic radius = 28 pm) or xenon (atomic radius = 140 pm) to behave more like an “ideal gas”? Explain your reasoning.
|
Column 1 |
Column 2 (Select One) |
Column 3 (Circle + or − to indicate whether the variable is greater or smaller under real conditions compared to ideal conditions) |
P
|
|
i. Particle mass |
P
real = Pideal ± a |
a = your selection in Column 2 |
ii. Particle size |
iii. Particle attraction |
iv. None of these variables should be used to modify pressure |
V
|
|
i. Particle mass |
V
real = Videal ± b |
* Videal is the volume of the container and the particles, where we assume that the volume of the particles is zero. |
ii. Particle size |
b = your selection in Column 2 |
iii. Particle attraction |
iv. None of these variables should be used to modify volume |
n
|
|
i. Particle mass |
n
real = nideal ± c |
ii. Particle size |
c = your selection in Column 2 |
iii. Particle attraction |
iv. None of these variables should be used to modify number of molecules |
T
|
|
i. Particle mass |
|
ii. Particle size |
d = your selection in Column 2 |
iii. Particle attraction |
* Since none of the variables should be used to modify T, Treal= Tideal |
iv. None of these variables should be used to modify temperature |
16. Consider a Superbowl that will take place in Minnesota, where the all-time low temperature is −60 °F (222 K). A weather model predicts that the temperature may be near this all-time low on the game date. What recommendations do you have for the referees regarding the pressure of the footballs to be used in the game? Sketch a particulate-level representation to describe the gas molecules inside the football (1) in the locker room and (2) outside. Assume the volume is held constant.
Acknowledgements
This work is funded by NSF Grant DUE-1611622. Special thanks to the Becker research group for their helpful feedback on this project and specifically Leah B. Scharlott with her immense help developing the coding scheme. Also, to Toby Thecat for his unwavering support throughout the lifetime of this paper.
References
- Akaygun S. and Jones L. L., (2013a), Dynamic visualizations: tools for understanding the particulate nature of matter, in Concepts of Matter in Science Education, Tsaparlis G. and Sevian H., (ed.), Innovations in Science Education and TEchnology, pp. 281–300.
- Akaygun S. and Jones L. L., (2013b), Research-based design and development of a simulation of liquid–vapor equilibrium, Chem. Educ. Res. Pract., 14(3), 324–344.
- Becker N., Rasmussen C., Sweeney G., Wawro M., Towns M. and Cole R., (2013), Reasoning using particulate nature of matter: an example of a sociochemical norm in a university-level physical chemistry class, Chem. Educ. Res. Pract., 14(1), 81–94.
- Becker N., Stanford C., Towns M. and Cole R., (2015), Translating across macroscopic, submicroscopic, and symbolic levels: the role of instructor facilitation in an inquiry-oriented physical chemistry class, Chem. Educ. Res. Pract., 16(4), 769–785.
- Birks M., Chapman Y. and Francis K., (2008), Memoing in qualitative research: Probing data and processes, Journal of Research in Nursing, 13(1), 68–75.
- Campbell J. L., Quincy C., Osserman J. and Pedersen O. K., (2013), Coding in-depth semistructured interviews: problems of unitization and intercoder reliability and agreement, Soci. Meth. Res., 42(3), 294–320.
- Chen Y., Irving P. W. and Sayre E. C., (2013), Epistemic game for answer making in learning about hydrostatics. Physical review special topics, Phys. Educ. Res., 9(1), 010108.
- Chen Y.-C., Wilson K. and Lin H.-S., (2019), Identifying the challenging characteristics of systems thinking encountered by undergraduate students in chemistry problem-solving of gas laws, Chem. Educ. Res. Pract., 20(3), 594–605.
- Chi M. T. H., De Leeuw N., Chiu M.-H. and Lavancher C., (1994), Eliciting self-explanations improves understanding, Cogn. Sci., 18(3), 439–477.
- Clark D. B., (2006), Longitudinal conceptual change in students’ understanding of thermal equilibrium: an examination of the process of conceptual restructuring, Cogn. Instr., 24(4), 467–563.
- Collins A. and Ferguson W., (1993), Epistemic forms and epistemic games: structures and strategies to guide inquiry, Educ. Psychol., 28(1), 25–42.
- Cooper M. M. and Stowe R. L., (2018), Chemistry education research—from personal empiricism to evidence, theory, and informed practice, Chem. Rev., 118(12), 6053–6087.
- Cracolice M. S., Deming J. C. and Ehlert B., (2008), Concept learning versus problem solving: a cognitive difference, J. Chem. Educ., 85(6), 873–878.
- Daubenmire P. L. and Bunce D. M., (2008), What do students experience during POGIL instruction? in Process Oriented Guided Inquiry Learning (POGIL), Moog R. S. and Spencer J. N., (ed.), ACS Symposium Series, pp. 87–99.
- Daubenmire P. L., Bunce D. M., Draus C., Frazier M., Gessell A. and van Opstal M. T., (2015), During POGIL Implementation the Professor Still Makes a Difference, J. Coll. Sci. Teach., 44(5), 72–81.
- De Berg K. C., (1995), Student understanding of the volume, mass, and pressure of air within a sealed syringe in different states of compression, J. Res. Sci. Teach., 32(8), 871–884.
- diSessa A. A., (1993), Toward an Epistemology of Physics, Cogn. Instr., 10(2–3), 105–225.
- Ford M., (2012), A Dialogic Account of SenseMaking in Scientific Argumentation and Reasoning, Cogn. Instr., 30, 207–245.
- Freeman S., Eddy S. L., McDonough M., Smith M. K., Okoroafor N., Jordt H. and Wenderoth M. P., (2014), Active learning increases student performance in science, engineering, and mathematics, Proc. Natl. Acad. Sci. U. S. A., 111(23), 8410–8415.
- Hale D. and Mullen L. G., (2009), Designing Process-Oriented Guided-Inquiry Activities: A New Innovationl for Marketing Classes, Mark. Educ. Rev., 19(1), 73–80.
- Hanson D. M., (2011), Foundations of chemistry: applying POGIL principles, Pacific Crest.
- Lin H.-s., Cheng H.-j. and Lawrenz F., (2000), The assessment of students and teachers’ understanding of gas laws. (Statistical Data Included), J. Chem. Educ., 77(2), 235.
- Irwanto I., Saputro A. D., Rohaeti E. and Prodjosantoso A. K., (2018), Promoting Critical Thinking and Problem Solving Skills of Preservice Elementary Teachers through Process-Oriented Guided-Inquiry Learning (POGIL), Int. J. Instr., 11(4), 777–794.
- Karplus R. and Thier H. D., (1968), A new look at elementary school science, Sci. Educ., 52(1), 91–91.
- Kautz C. H., Heron P. R. L., Loverude M. E. and McDermott L. C., (2005a), Student understanding of the ideal gas law, Part I: A macroscopic perspective, Am. J. Phys., 73(11), 1055–1063.
- Kautz C. H., Heron P. R. L., Shaffer P. S. and Mcdermott L. C., (2005b), Student understanding of the ideal gas law, Part II: A microscopic perspective, Am. J. Phys., 73(11), 1064–1071.
- Kulatunga U. and Lewis J. E., (2013), Exploration of peer leader verbal behaviors as they intervene with small groups in college general chemistry, Chem. Educ. Res. Pract., 14(4), 576–588.
- Kulatunga U., Moog R. S. and Lewis J. E., (2014), Use of Toulmin's Argumentation Scheme for Student Discourse to Gain Insight About Guided Inquiry Activities in College Chemistry, J. Col. Sci. Teach., 43(5), 78–86.
- Lawson A. E., (1988), A Better Way to Teach Biology, Am. Biol. Teach., 50(5), 266–278.
- Madden S. P., Jones L. L. and Rahm J., (2011), The role of multiple representations in the understanding of ideal gas problems, Chem. Educ. Res. Pract., 12(3), 283–293.
- Matijaevi I., Korolija J. N. and Mandi L. M., (2016), Translation of P = kT into a pictorial external representation by high school seniors, Chem. Educ. Res. Pract., 17(4), 656–674.
- Maurer T. W., (2014), Teaching Financial Literacy with Process-Oriented Guided-Inquiry Learning (POGIL), J. Finan. Educ., 40(3/4), 140–163.
- McHugh M. L., (2012), Interrater reliability: the kappa statistic, Biochem. Med., 22(3), 276.
- McNeill K. L., Lizotte D. J., Krajcik J. and Marx R. W., (2006), Supporting Students’ Construction of Scientific Explanations by Fading Scaffolds in Instructional Materials, J. Learn. Sci., 15(2), 153–191.
- Mestre J. P., (2005), Transfer of learning from a modern multidisciplinary perspective, IAP.
- Moog R. S. and Farrell J. J., (2017), Chemistry: a guided inquiry.
- Moog R. S., Spencer J. N. and American Chemical Society, (ed.), (2008), Process oriented guided inquiry learning (POGIL), American Chemical Society; Distributed by Oxford University Press.
- Moon A., Stanford C., Cole R. and Towns M., (2017a), Analysis of inquiry materials to explain complexity of chemical reasoning in physical chemistry students’ argumentation, J. Res. Sci. Teach., 54(10), 1322–1346.
- Moon A., Stanford C., Cole R. and Towns M., (2017b), Decentering: A Characteristic of Effective
Student–Student Discourse in Inquiry-Oriented Physical Chemistry Classrooms, J. Chem. Educ., 94(7), 829–836.
- Moon A., Stanford C., Cole R. and Towns M. H., (2016), The nature of students’ chemical reasoning employed in scientific argumentation in physical chemistry, Chem. Educ. Res. Pract., 17(2), 353–364.
- Nakhleh M. B., (1993), Are our students conceptual thinkers or algorithmic problem solvers? Identifying conceptual students in general chemistry, J. Chem. Educ., 70(1), 52–55.
- Nakhleh M. B., Lowrey K. A. and Mitchell R. C., (1996), Narrowing the gap between concepts and algorithms in freshman chemistry, J. Chem. Educ., 73(8), 759–762.
- Nakhleh M. B. and Mitchell R. C., (1993), Concept learning versus problem solving: There is a difference, J. Chem. Educ., 70(3), 190–192.
- National Research Council, (2012), A framework for K-12 science education: Practices, crosscutting concepts, and core ideas, National Academies Press.
- Nurrenbern S. C. and Pickering M., (1987), Concept learning versus problem solving: Is there a difference? J. Chem. Educ., 64(6), 508.
- Odden T. O. B. and Russ R. S., (2019a), Defining sensemaking: Bringing clarity to a fragmented theoretical construct, Sci. Educ., 103(1), 187–205.
- Odden T. O. B. and Russ R. S., (2019b), Vexing questions that sustain sensemaking, Int. J. Sci. Educ., 41(8), 1052–1070.
- Odden T. O. B. and Russ R. S., (2018), Sensemaking epistemic game: A model of student sensemaking processes in introductory physics, Phys. Rev. Phys. Educ. Res., 14(2), 020122.
- Pickering M., (1990), Further studies on concept learning versus problem solving, J. Chem. Educ., 67(3), 254–255.
- POGIL Project, (2020), Additional resources, POGIL.
- Robertson A. D. and Shaffer P. S., (2016), University student reasoning about the basic tenets of kinetic-molecular theory, part II: pressure of an ideal gas. (PHYSICS EDUCATION RESEARCH SECTION)(Author abstract). Am. J. Phys., 84(10), 795.
- Robertson A. and Shaffer P., (2013), University student and K-12 teacher reasoning about the basic tenets of kinetic-molecular theory, Part I: Volume of an ideal gas, Am. J. Phys., 81(4), 303–312.
- Rodriguez J.-M. G., Bain K. and Towns M. H., (2020a), The role of epistemology and epistemic games in mediating the use of mathematics in chemistry: Implications for mathematics instruction and research on undergraduate mathematics education, Int. J. Res. Undergrad. Math. Ed., 6, 279–301.
- Rodriguez J.-M. G., Hunter K. H., Scharlott L. J. and Becker N. M., (2020b), A review of research on process oriented guided inquiry learning: Implications for research and practice, J. Chem. Educ., 97(10), 3506–3520.
- Rodriguez J.-M. G., Lazenby K., Scharlott L. J., Hunter K. H. and Becker N. M., (2020c), Supporting engagement in metamodeling ideas in general chemistry: Development and validation of activities designed using process oriented guided inquiry learning criteria, J. Chem. Educ., 97(12), 4276–4286.
- Russ R. S., Lee V. R. and Sherin B. L., (2012), Framing in cognitive clinical interviews about intuitive science knowledge: Dynamic student understandings of the discourse interaction, Sci. Educ., 96(4), 573–599.
- Russ R. S., Scherr R. E., Hammer D. and Mikeska J., (2008), Recognizing mechanistic reasoning in student scientific inquiry: A framework for discourse analysis developed from philosophy of science, Sci. Educ., 92(3), 499–525.
- Sanger M. J., Phelps A. J. and Fienhold J., (2000), Using a computer animation to improve students’ conceptual understanding of a can-crushing demonstration. (Statistical Data Included), J. Chem. Educ., 77(11), 1517.
- Sanger M. J., Vaughn C. K. and Binkley D. A., (2013), Concept learning versus problem solving: Evaluating a threat to the validity of a particulate gas law question, J. Chem. Educ., 90(6), 700–709.
- Sawrey B. A., (1990), Concept learning versus problem solving: Revisited, J. Chem. Educ., 67(3), 253–254.
- Schuttlefield J. D., Kirk J., Pienta N. J. and Tang H., (2012), Investigating the Effect of Complexity Factors in Gas Law Problems, J. Chem. Educ., 89(5), 586–591.
- Sevian H. and Couture S., (2018), Epistemic games in substance characterization, Chem. Educ. Res. Pract., 19(4), 1029–1054.
- Shen J. and Linn M., (2011), A Technology-Enhanced Unit of Modeling Static Electricity: Integrating scientific explanations and everyday observations, Int. J. Sci. Educ., 33, 1597–1623.
- Stamovlasis D., Tsaparlis G., Kamilatos C., Papaoikonomou D. and Zarotiadou E., (2005), Conceptual understanding versus algorithmic problem solving: Further evidence from a national chemistry examination, Chem. Educ. Res. Pract., 6(2), 104–118.
- Standards For Educational And Psychological Testing, (2012).
- Stanford C., Moon A., Towns M. and Cole R., (2016), Analysis of Instructor Facilitation Strategies and Their Influences on Student Argumentation: A Case Study of a Process Oriented Guided Inquiry Learning Physical Chemistry Classroom, J. Chem. Educ., 93(9), 1501–1513.
- Stanford C., Moon A., Towns M. and Cole R., (2018), The Impact of Guided Inquiry Materials on Student Representational Level Understanding of Thermodynamics, in Engaging Students in Physical Chemistry, Teague C. M. and Gardner D. E., (ed.), ACS Symposium Series, pp. 141–168.
- Tang H. and Pienta N., (2012), Eye-Tracking Study of Complexity in Gas Law Problems, J. Chem. Educ., 89(8), 988–994.
- Tannen D., (1993), in Framing in discourse, Tannen D., (ed.), New York: Oxford University Press.
- Tasker R. and Dalton R., (2006), Research into practice: visualisation of the molecular world using animations, Chem. Educ. Res. Pract., 7(2), 141–159.
- The Concord Consortium, (2020), Visual, Interactive Simulations for Teaching & Learning Science, Molecular Workbench.
- The POGIL Project, (2020a), Implementing POGIL, The POGIL Project.
- The POGIL Project, (2020b), What is POGIL? The POGIL Project.
- The POGIL Project, (2020c), Writing Guidelines, The POGIL Project.
- Theobald E. J., Hill M. J., Tran E., Agrawal S., Arroyo E. N. and Behling S., et al., (2020), Active learning narrows achievement gaps for underrepresented students in undergraduate science, technology, engineering, and math, Proc. Natl. Acad. Sci. U. S. A., 117(12), 6476.
- Tuminaro J. and Redish E. F., (2007), Elements of a cognitive model of physics problem solving: Epistemic games, Phys. Rev. ST Phys. Educ. Res., 3(2), 020101.
- Vacek J., (2011), Process Oriented Guided Inquiry Learning (POGIL), A Teaching Method From Physical Sciences, Promotes Deep Student Learning In Aviation, Coll. Aviation Rev. Int., 29, 78–88.
- Walker L. and Warfa A.-R. M., (2017), Process oriented guided inquiry learning (POGIL®) marginally effects student achievement measures but substantially increases
the odds of passing a course, PLoS One, 12(10), e0186203.
- Warfa A.-R. M., Roehrig G. H., Schneider J. L. and Nyachwaya J., (2014), Role of Teacher-Initiated Discourses in Students’ Development of Representational Fluency in Chemistry: A Case Study, J. Chem. Educ., 91(6), 784–792.
- Williamson V. M. and Abraham M. R., (1995), The effects of computer animation on the particulate mental models of college chemistry students, J. Res. Sci. Teach., 32(5), 521–534.
- Yuriev E., Naidu S., Schembri L. S. and Short J. L., (2017), Scaffolding the development of problem-solving skills in chemistry: guiding novice students out of dead ends and false starts, Chem. Educ. Res. Pract., 18(3), 486–504.
- Zoller U., Lubezky A., Nakhleh M. B., Tessier B. and Dori Y. J., (1995), Success on algorithmic and LOCS vs. conceptual chemistry exam questions, J. Chem. Educ., 72(11), 987–989.
|
This journal is © The Royal Society of Chemistry 2021 |