DOI:
10.1039/D1RA07845C
(Paper)
RSC Adv., 2021,
11, 38616-38631
In silico analysis of SARS-CoV-2 papain-like protease potential inhibitors†
Received
23rd October 2021
, Accepted 23rd November 2021
First published on 1st December 2021
Abstract
The emergent outbreak caused by severe acute respiratory syndrome coronavirus 2 continues spreading and causing huge social and economic disruption. Papain-like protease (PLpro) has a crucial role in the cleavage of viral polyproteins, and disruption of host responses. PLpro is considered an important goal for the development of SARS-CoV-2 inhibitors. ZINC101291108 (lead 1) and ZINC16449029 (lead 2) were identified as potent SARS-CoV-2 PLpro inhibitors with IC50 values of 0.085 μM and 0.063 μM, respectively. Molecular dynamics simulations (MD) were carried out for lead 1, 2 and several reported SARS-CoV-2 inhibitors. Analysis results of the simulations confirmed the stability of both compounds and showed that they adopted two confirmations along the simulation period. The per-residue decomposition results revealed that the key residues involved in inhibitor binding were E167, P247, P248, Y264, Y268 and Q269. H-bond analyses showed H-bonds with G266 and N267 and salt bridges with G209 and Y273, which are essential for strengthening the substrate-binding pocket. Both inhibitors showed hydrophobic interactions with the S4 site and BL2 loop residues. The RMSD of the BL2 loop with the two inhibitors was investigated, and the results showed that the Y268 and Q269 BL2 loop residues moved outward to accommodate the large size of lead 2. The van der Waals interaction was the main energy contribution that stabilized lead 2, while van der Waals and electrostatic interactions were the main energy contributions stabilizing lead 1. Rational design strategies were suggested to replace the 2-(2-hydroxybenzylidene) hydrazine moiety with naphthalene or nitrobenzene at the P4 position of lead 2 and introduce polar substituents as aniline and benzoate groups at position P1 to enhance hydrophobic interactions and H-bonds, respectively.
1 Introduction
In December of 2019, a global health crisis emerged that was caused by a novel form of a coronavirus (COVID-19).1 The first human infected with severe acute respiratory syndrome coronavirus 2 (SARS-CoV-2) was identified in Wuhan, China,2 and the virus spread rapidly worldwide, causing a significant mortality rate and substantial economic and social disruption.3 Related coronavirus infectious diseases, SARS-CoV4,5 and Middle East respiratory syndrome (MERS-CoV),6,7 occurred at pandemic levels in 2002 and 2012, respectively. Coronaviruses belong to clade B of the genus Betacoronavirus8 and have an envelope single-stranded (+) sense RNA genome (ssRNA).9 The ssRNA is used to produce 15 nonstructural proteins from two large polypeptides: PP1a and PP1ab.10
Two cysteine protease enzymes encoded by SARS-CoV-2 play a significant role in virus proliferation.11 First protease is the main protease Mpro is also known as chymotrypsin-like protease 3CLpro12 and the second one is Papain-Like Protease PLpro. SARS-CoV-2 Mpro compromises of 306 amino acids and consists of three domains: N-terminal domain I, N-terminal domain II, and C-terminal domains III.13 The Mpro cuts the polyproteins PP1a and PP1ab to give several NSPs, involving the three subunits14 Nsp7, Nsp8 and Nsp8 that compromise the viral RNA polymerase complex.15 Besides, the integral membrane proteins Nsp4 and Nsp6.16 SARS-CoV-2 PLpro cuts the two polyproteins and generates Nsp1, Nsp2 and Nsp3 subunits. The Nsp3–Nsp4 and Nsp6 are the essential constituent of the organelles replication which are needed for the viral polymerase function in infected cells.17
On the other hand, the tetrapeptide LXGG motif found between Nsp1/Nsp2, Nsp2/Nsp3 and Nsp3/Nsp4 viral proteins18 corresponds to the P4–P1 substrate of cysteine proteases.19 PLpro recognizes the LXGG motif, and hydrolysis of the peptide bond on the carboxyl side of glycine at P1 results in the release of viral proteins Nsp1, Nsp2 and Nsp3, which are important for the replication.20
Ubiquitin and ISG15 proteins have an LXGG motif at their C-terminus, and PLpro shows deubiquitinating and deISG15ylating activities21 through recognition and cleavage of the LXGG motif in both proteins.22 Due to these activities of PLpro, the enzyme is involved in preventing chemokines and cytokines that activate the host innate immune response against SARS-CoV-2.23,24 In addition to proteolytic activity of PLpro to the viral proteins PP1a and PP1b, it plays an essential role in the inhibition of the innate immune response of the host.25 Therefore, the two proteases play a significate role in the viral lifecycle through transcription and replication26 which render both proteases an excellent targets for developing SARS-CoV-2 inhibitors. The drug design research to develop SARS-CoV-2 Mpro inhibitors are huge.27 However, the volume of the research to develop SARS-CoV PLpro is very few.
For that, preventing SARS-CoV-2 PLpro activity is considered a significant target in the design and discovery of anti-SARS-CoV-2 drugs. SARS-CoV-2 PLpro inhibitors not only inhibit viral replication but also inhibit the dysregulation of signalling cascades in the innate immune system.28 SARS-CoV-2 PLpro enzyme has two distinct domains: the “thumb–palm–fingers” catalytic domain and the small N-terminal ubiquitin-like (Ubl) domain (Fig. 1).29 The Ubl domain consists of residues 1–60, and its specific function is not well understood.28 The finger subdomain contains a Zn ion binding site—Zn is essential for structural integrity and protease activity—and Zn ions coordinate with four cysteine residues (C189, 192, 224 and 226).30 The palm subdomain includes the catalytic residues C111, H272 and D286, the thiolate form of C111 acting as a nucleophilic residue and D286 promoting deprotonation of H272, which acts as a base.31 The mobile loop (G266–G271) is adjacent to the active site that closes upon substrate or inhibitor binding (Fig. S1†).28 There is a pocket near the catalytic triad that accommodates ligands and possibly guides the substrate towards the catalytic triad for cleavage.32 Recent research focused on the good draggability of the substrate binding pocket indicates it as important binding site for designing and discovering SARS-CoV-2 PLpro inhibitors.32 Covalently bound peptide ligands VIR250 and VIR251,33 the small molecules GRL0617 (ref. 34) and rac5c35 have been found to bind to this pocket and inhibit PLpro protein activity. The research on SARS-CoV-2 PLpro has been studied by the scientific community, this studies include insight into PLpro structure,28 function33 and utilization the similarity between SARS-CoV PLpro and SAR-CoV-2 PLpro to develop novel inhibitors.27 Several researches focus on repurposed other protease inhibitors for example remdesivir which is RNA polymerase inhibitor.36 Also, HCV protease inhibitors were found to inhibit proteolytic activity of SARS-CoV-2 PLpro.16 In addition, broad computational approaches were employed37 as novel and fast techniques for discovering promising and nontoxic SARS-CoV-2 PLpro inhibitors.36,38 For instance M. U. Mirza et al. used the structure-based virtual screening to identify novel Human ubiquitin carboxyl-terminal hydrolase-2 (USP2) as SARS-CoV-2 inhibitors.39
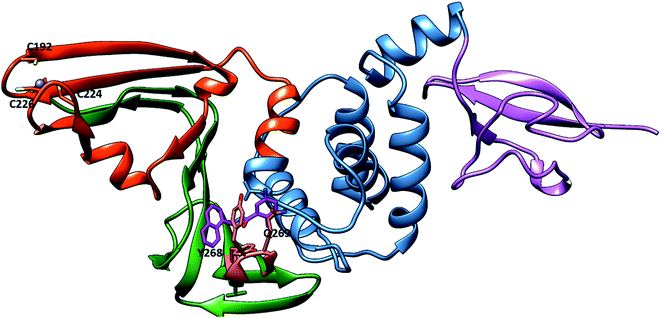 |
| Fig. 1 Representation of SARS-CoV-2 PLpro in the cartoon representation (PDB: 7JRN). The protein contains the ubiquitin-like (UBL) (purple), thumb (blue), palm (orange red), and finger (green) domains. In the putative site, BL2 loop (Salmon) with key residues Y268 and Q269. The GRL0617 (sick, violet). The bound Zn2+ ion in the finger domain is shown as a blue sphere coordinated with C192, C224 and C226. | |
In our previous study using structure-based virtual screening we discovered that compounds ZINC101291108 (lead 1); 4-[2-(2-{[(6-hydroxy-2,4-dioxo-1,2,3,4-tetrahydropyrimidin-5-yl)(4-hydroxy-3-methoxyphenyl)methyl]amino}-4-oxo-4,5-dihydro-1,3-thiazol-5-yl)acetamido]benzoic acid and ZINC16449029 (lead 2); 1,2,5-oxadiazole-3-carboximide acid, 4,4′-(methylenediimino)bis,bis[[(2-hydroxyphenyl)methylene]hydrazide (Table 1) showed an excellent activity against SARS-CoV-2 Mpro and potent activity against SARS-CoV-2 PLpro.40 these compounds showed the most promising activity against PLpro, with IC50 values of 0.085 μM and 0.063 μM, respectively. In order to rational the promising results of lead 1 and lead 2, the molecular dynamics simulations of both compounds complexed with SARS-CoV-2 PLpro were studied.
Table 1 Chemical structures of promising compounds and GRL0617 and their binding energies by BUDE
Compounds |
Structure |
BUDE binding |
Ki (nm) |
Energy kJ mol−1 |
GRL0617 |
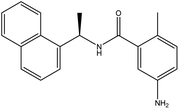 |
−59.98 |
24.8 |
Lead 1 |
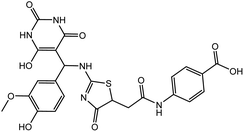 |
−82.96 |
115.85 |
Lead 2 |
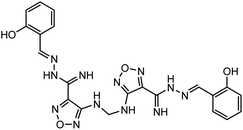 |
−79.58 |
216.8 |
To understand the binding mode of lead 1 and lead 2 and the key residues involved in the interactions, comprehensive computational approaches including molecular docking for both compounds with the Bristol University Docking Engine (BUDE)41,42 and molecular dynamic (MD) simulations were performed for both compounds and the reported SARS-CoV-2 PLpro inhibitors; GRL0617, compounds 2, 3, 6,28 Vaniprevir (VAN) and Simeprevir (SIM).16 The conformational stability of the docked complexes was analysed along the MD simulation using various parameters, such as root mean square deviation (RMSD), root mean square fluctuation (RMSF), radius of gyration (Rg), hydrogen bond monitoring, solvent accessible surface area (SASA), free energy calculation and per-residue decomposition.
2 Computational methods
2.1. Docking procedure
In this study, the cocrystal structures of SARS-CoV-2 PLpro with a GRL0617 inhibitor (PDB: 7JRN)43 and free liganded protein (PDB: 6WZU)28 were downloaded from the Research Collaboratory for Structural Bioinformatics (RCSB) Protein Data Bank (https://www.rcsb.org/). The protonation state of all amino acid residues was assigned at pH 7.4 using the PDB2PQR server,44 H atoms were added, and water molecules were removed by MOE software.45 Missing residues were added by the MODELLER programme46 through a Chimera graphical interface.47 Lead 1, lead 2 and reported SARS-CoV inhibitors B004, B005, B009, B010 and B013 (ref. 27) and SARS CoV-2 inhibitors Vaniprevir (VAN), Simeprevir (SIM),16 VIR250, VIR251,33 Rac5C, compounds 2, 3 and 6.28 were draw with ChemDraw Ultra 12 and energies were minimized with MOE. Bristol University Docking Engine program (BUDE)41 was used for docking and screening the compounds against SARS-CoV-2 PLpro. The docking site was located at X = 10.87, Y = 11.32, and Z = 31.59, which is the centre of the GRL0617 ligand. This binding pocket is considered a putative pocket. To validate the docking protocol, GRL0617 and reported inhibitors were redocked with BUDE in the SARS-CoV-2 PLpro crystal structures.
2.2. MD simulations
MD simulations were performed for the docked pose of lead 1, lead 2, GRL0617 and reported SARS-CoV-2 PLpro inhibitors; compounds 2, 3, 6, Vaniprevir (VAN), and Simeprevir (SIM) using GROMACS-2019.2.48 The topologies of ligands were created using Acpype.49 The Amber99SB-ildn50,50b force field was used for all simulations under periodic boundary conditions. The systems were solvated in a cubic box with a minimum margin of 1 nm with a water model (spc216)51 and filled with TIP3P water.52 Then, 0.15 M counter ions (Na+ and Cl−) were added to neutralize the system, and energy minimization was carried out for all the neutralized systems using the steepest descent and conjugate gradients (50
000 steps) followed by conjugate gradients. The NVT ensemble at a constant temperature of 300 K was employed for 100 ps and then equilibrated with NPT conditions for 100 ps. The particle mesh Ewald (PME) method was used to calculate the long-range electrostatic forces53 and van der Waals (rvdw) interactions. The cut-offs for van der Waals and coulombic interactions were set at 1.2 nm.54 The bonds and angles were constrained with the LINC algorithm. Molecular dynamics simulations (100 ns) were executed for the complexes. All post MD analyses RMSD, RMSF, SASA and Rg were performed using Gromacs tools and plotted via the Xmgrace55 program. Trajectory visualizations were performed using PyMOL,56 and VMD-1.9.1 (ref. 57) images were created with Chimera software.47
2.3. MM-PBSA binding free energy calculation
The binding free energies for the hits and reported inhibitors were calculated using the MM/PBSA58 method using MMPBSA.py.59 The calculation was carried out on the 500 snapshots extracted from the last 50 ns. The binding energy was calculated by.
ΔGbind = Gcomplex − (Gprotein + Gligand) |
ΔGbind is the free energy of the protein–ligand binding.
The MM/PBSA per-residue decomposition was calculated for lead1 and lead 2 complexes to determine the contribution of each residue to the binding energy using MmPbSaDecomp.py.
3 Results and discussion
3.1. Characteristics of the substrate-binding site in PLpro
The PLpro catalytic domain consists of 3 subdomains. The first is the “thumb-fingers-palm,” with the catalytic residues (C111, H272 and D286) located at the interface between the thumb and palm, forming the active site. The second is a groove-like pocket directly adjacent to the PLpro active site; previous studies focused on this putative binding site due its draggability.60 The BL2 loop flexibility results in the opening and closing conformation, indicating the ability of this binding site to accommodate ligands with different small or large scaffolds. BL2 residues Y268 and Q269 have different conformations in free-ligand proteins and liganded proteins, and these residues are arranged to accommodate the ligands61 (Fig. S1†). G266, another BL2 loop residue, provided binding specificity to the inhibitors through the formation of H-bonds.61 The S4 hydrophobic site formed from P247, P248, Y272 and T301 recognizes the LXGG peptide and accommodates the leucine side chain in the hydrophobic pocket. These hydrophobic residues are conserved in SARS-CoV and SAR-CoV-2, which suggests that inhibitors that bind to the S4 hydrophobic site may be promising PLpro inhibitors.28
3.2. Molecular docking
To validate the docking protocol, the reported SARS-CoV inhibitors (B004, B005, B009, B010 and B013) (Fig. 2) and SARS-CoV-2 inhibitors (Vaniprevir (VAN), Simeprevir (SIM), VIR250, VIR251, Rac5C, compound 2, compound 3 and compound 6) (Fig. 3) were docked with BUDE and the docking results showed an excellent binding score. In addition, the binding efficacy of all reported compounds (Table S1†) were very small values which indicates the greater binding of the ligands to the target proteins. These results of excellent binding score and small values of binding scores are in correlation with the biological results of the reported compounds, which indicates the reliability of the docking procedures. In addition, the RMSD values of the reported compounds were <1 Å which confirm the validity of the docking protocol. Lead 1, lead 2 and the reference compound GRL0617 were docked with BUDE, and illustrated promising binding energy and inhibition constant (Table 1). The inhibition constant was in consistent with the IC50, as lead 2 the most promising inhibitors showed the best inhibition constant.37
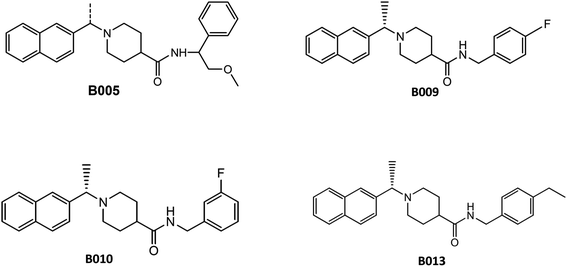 |
| Fig. 2 Structure of SARS-CoV PLpro inhibitors. | |
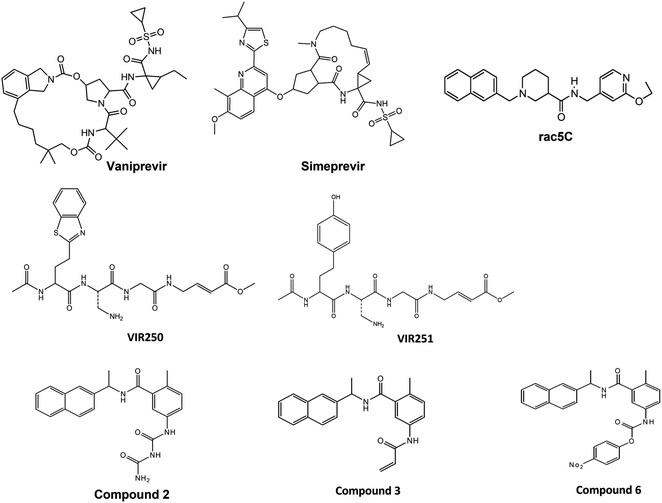 |
| Fig. 3 Structure of SARS-CoV_2 PLpro inhibitors. | |
3.3. Molecular dynamics simulations
To understand the complex stability and interaction profile of the active inhibitor inside the putative pocket of SARS-CoV-2 PLpro, MD simulations of PLpro-native ligand (GRL0617) and PLpro-ligands complexes were performed for a period of 100 ns. Furthermore, molecular dynamic simulations were performed for the reported potential SARS-CoV-2 inhibitors (compound 2, 3, 6, vaniprevir (VAN), simeprevir (SIM)). Structural parameters, including RMSD, RMSF, Rg, SASA, H-bonds, per-residue, and binding energy calculation were evaluated as a function of time.
3.3.1. RMS-deviation and RMS-Fluctuation. The stability of docked complexes lead 1, lead 2, GRL0617, compounds 2, 3, 6, SIM and VAN complexed with SARS-CoV-2 PLpro were subjected to Cα RMSD analyses (Fig. 4A and B) to assess the flexibility of PLpro residues. Compounds GRL0617 and lead 1 reached the equilibrium at 20 ns while lead 2 at 30 ns. GRL0617 and lead 1 illustrated an average RMSD values of 0.20 nm while average RMSD of lead 2 was 0.27 nm. The ligand-free protein showed an average RMSD of 0.24 nm (Fig. 4A, Table S2†). Compound 2, 3 and 6 illustrated an average RMSD value of 0.21 nm, SIM and vaniprevir (VAN) showed RMSD values 0.31 nm and 0.26 nm respectively (Fig. 4B, Table S2†). Overall, lead 1 revealed the lowest RMSD value among the all inhibitors and lower than RMSD of ligand-free protein. These results indicated that lead 1 stabilize SARS-CoV-2 PLpro protein. On the other hand, the RMSD value of lead 2 was slightly higher than the RMSD of the most inhibitors and the free-ligand protein, but it was stable along the simulations, indicating a strong binding of lead 2 within the PLpro binding site.
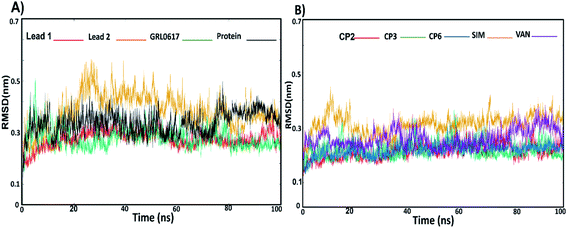 |
| Fig. 4 The RMSD values; (A) lead1-protein complex (red), lead 2–protein complex (yellow), GRL0617–protein complex (green) protein (black). (B) RMSD values of compound 2-protein complex (red), compound 3–protein complex (green), compound 6–protein complex (blue), simeprevir (SIM)–protein complex (yellow), vaniprevir (VAN)–protein-complex (violet). | |
Furthermore, to identify the flexible and rigid regions of the complexes, RMSF analyses were performed to measure the average residue flexibility of the PLpro-docked complexes. Nearly all inhibitors-complexes illustrated similar average RMSF values of ∼0.13 nm (Table S2†). It could be observed that the UBL domain residues (1–60) showed a high flexibility amplitude for the all systems and mainly for free-ligand protein (Fig. 5A and B); this flexibility was due to it not possessing any ligands, so the UBL domain retained its freedom of movement. This observation is consistent with the previously published findings showing that the highly flexible PLpro domains are finger domains, especially the zinc-binding region, UBL domain and BL2 loop.34 All systems showed a decrease in the flexibility of the catalytic residues C111, H272 and D286 and S4 site residues P247, P278, Y264 and Y273. The greatest residue fluctuation observed with the PLpro-lead 2 system was in the residue range N267-Q269, which are a part of the BL2 loop. The Y268 residue showed the highest RMSF (5 Å), considering that Y268 played an important role in opening and closing the BL2 loop, which suggests that the BL2 loop adopted a specific conformation to accommodate lead 2. At the same time, a decrease in the movement amplitude of the residue range (267–271) BL2 loop in the presence of lead 1 and GRL0617 molecules indicated that both inhibitors stabilized the BL2 loop and that the BL2 loop adopted the closed conformation. The second highest fluctuation region in all systems was in the finger subdomain region, particularly the Zn binding site (C189, 192, 224, 226), which agrees with previous data.62 Compounds 2, 3, 6, SIM and VAN showed similar RMSF pattern to lead 1 and 2 (Fig. 5C, Table S2†), the highest fluctuation in the BL2 loop while lowest fluctuation was in residue range (267–271). It could be concluded that lead1 and lead 2 stabilized SARS-CoV-2 PLpro in a similar manner to the reported inhibitors.
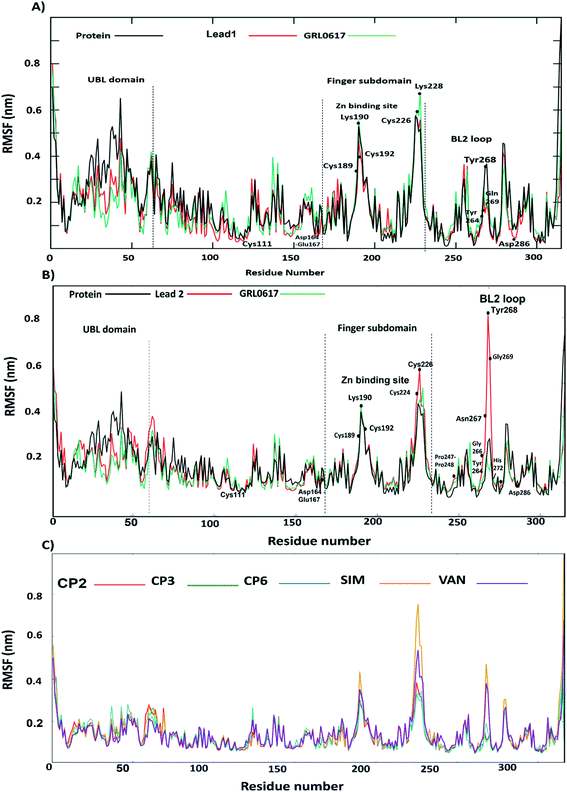 |
| Fig. 5 The RMSF values; (A) lead 1–protein complex (red), GRL0617–protein complex (green), protein (black). (B) lead 2–protein complex (red), GRL0617–protein complex (green) protein (black), (C) RMSF value; compound 2–protein complex (red), compound 3–protein complex (green), compound 6–protein complex (blue), simeprevir (SIM)–protein complex (yellow), vaniprevir (VAN)–protein-complex. | |
3.3.2. RMSD of BL2 loop. The BL2 loop showed a significant flexibility in the free-ligand protein, particularly residues N267 and Q269, and most importantly, residue Y268 (Fig. S2A, B and Table S2†). It is believed that the BL2 loop plays an important role in opening and closing the putative pocket to accommodate small/large inhibitors. The high amplitude of Y268 fluctuation with lead 2 encouraged us to investigate BL2 movements by measuring the RMSD of the BL2 loop complexed with the inhibitors. The average RMSD of the BL2 loop of the ligand-free protein, GRL0617 and lead 1 was 0.11 nm, while that of lead 2, SIM and VAN were 0.13. Compounds 2, 3 and 6 showed BL2 RMSD around 0.12 nm. Fig. S2† showed that the RMSD graph of BL2 loop lead 1, lead 2 and GRL0617 complexes were smooth, except lead 2-complex illustrated an interruption from 50–60 ns (Fig. S2A†). Interactions of lead 2 with the BL2 residues showed that N267, Y268 and Q269 displaced to give a space to accommodate lead 2 (Fig. S2B†). These results suggested that the movement of BL2 gave an area to accommodate the large inhibitors as lead 2, SIM and VAN.
3.3.3. Rg and SASA. The radius of gyration (Rg) was used to evaluate the compactness of the PLpro receptor-docked complexes. The results showed that the Rg values of GRL0617 and lead 1, lead 2, compounds 2, 3, 6, SIM and VAN complexes range between 2.3 and 2.4 nm and the complexes remained stable along the MD simulation (Fig. 6A, B and Table S2†). The Rg results revealed that the stable folding behaviour of PLpro after binding with lead 1 and lead 2 complexes. This suggested that both compounds bind strongly to the putative PLpro site, in similar manner to the reported compounds.
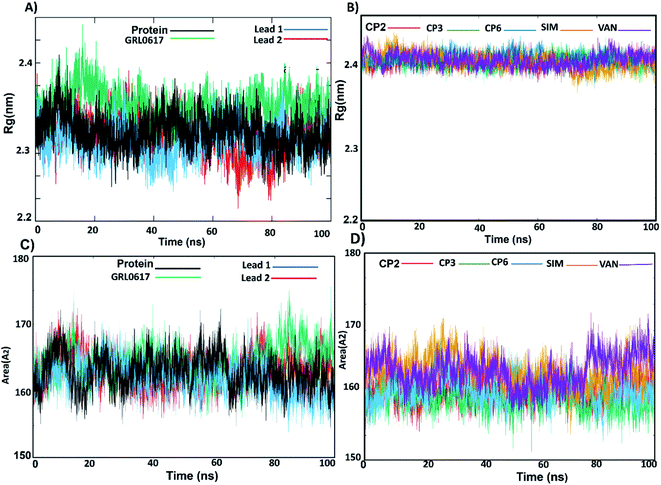 |
| Fig. 6 (A) Radius of gyration (Rg) of lead 1 (blue), lead 2 (red), GRL0617 (green) complexed with the protein and the nonligand protein (black). (B) Rg of CP2 (red), CP3 (green), CP6 (blue), simeprevir (SIM) (yellow) and vaniprevir (VAN) (violet) complexed with the protein. (C) SASA of lead 1 (blue), lead 2 (red), GRL0617 (green) complexed with the protein and the nonligand protein (black) (D) SASA of CP2, CP3, CP6, simeprevir (SIM) and vaniprevir (VAN) complexed with the protein. | |
Solvent-accessible surface area (SASA) calculations were used to estimate the water accessibility at the putative site of SARS-CoV-2 PLpro with and without inhibitor binding (Fig. 6C, D and Table S2†). The obtained results showed that the average SASA values for unliganded protein, GRL0617, lead 1 and lead 2, compounds 2, 3, 6, SIM and VAN were 163.0, 161.9, 163.2 and 164.4, 163.4, 161.5, 163.7, 167.2 and 167.3 Å2, respectively. GRL0617 reduced water accessibility to the binding pocket compared with the free ligand protein. Lead 1 showed SASA values like the free-protein while the SASA value of lead 2 was slightly higher than that of the free-liganded protein by a small value. Sim and VAN showed the highest SASA values. This suggested that further structural modification of lead 2 should be made by shrinking the size of the molecule group at the P4 site to better occupy the hydrophobic S4 site.
3.3.4. H-Bonds and distance monitoring. To identify the contribution of hot-spot residues to the PLpro protein-ligand interaction, H-bond analyses of complexes were estimated along a 100 ns simulation. From the MD of GRL0617, it was shown that it formed 3 continuous H-bonds with occupancy 99.8% (Fig. 7A); Q269 and Y264 (BL2 loop residues) formed H-bond with GRL0617 in occupancy (99%, 41%), respectively. Residues D164, N267 and Y268 formed H-bonds with the native ligand with approximately 20% occupancy. Residues D164, G266, N267 and Y273 established salt bridge interactions of 64%, 80%, 22%, and 98%, respectively. The hydrophobic contribution by residue Pro248 was observed with an occupancy of 100% (Fig. 7B). Compounds lead 1 and lead 2 formed H-bonds with occupancy (91.8% and 75.9%) (Table S2†). Lead 1 formed three H-bonds, and only two H-bonds were continuous (Fig. 8A). Lead 2 showed four H-bonds, two H-bonds were continuous along the simulation time, while the third H-bond persisted until 80 ns (Fig. 8B). In MD simulation of lead 1, Q269 was highly involved in H-bond stability for more than 90%, and the salt bridge contribution was observed by residues Q209, Y268 and Y273 with occupancy (90%, 70% and 40%). A246, P247 and P248 contributed by hydrophobic interactions with occupancy (70%, 90%, 90%) (Fig. 8C). Lead 2 was stable within the PLpro binding pocket through H-bonds with G266, N267 and P248 (32%, 15% and 35%) and salt bridges (more than 30%), while D164 and E167 contributed 15%. P247 and P248 (S4 site residues) showed hydrophobic contributions of 65% and 40%, respectively (Fig. 8D). Compounds 2, 3 and 6 formed two H-bonds while SIM and VAN formed one H-bond (Fig. S3†).
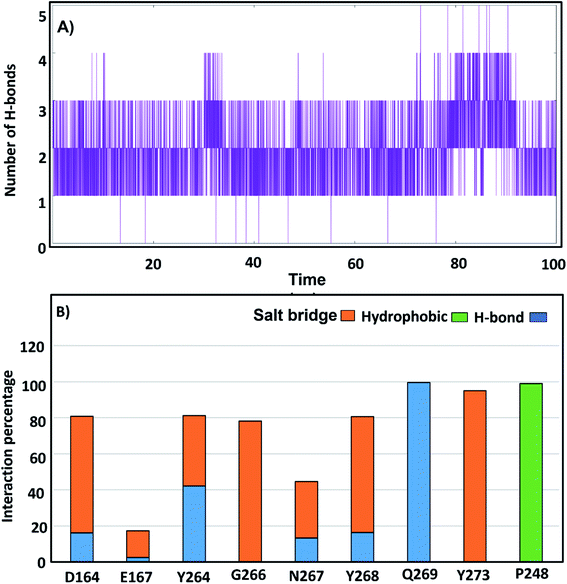 |
| Fig. 7 (A) number of H-bonds of GRL0617 with SARS-CoV-2 PLpro putative pocket. (B) Percentage of H-bonds, salt bridge and hydrophobic occupations of the SARS-CoV-2 PLpro residues contributed to GRL0617. | |
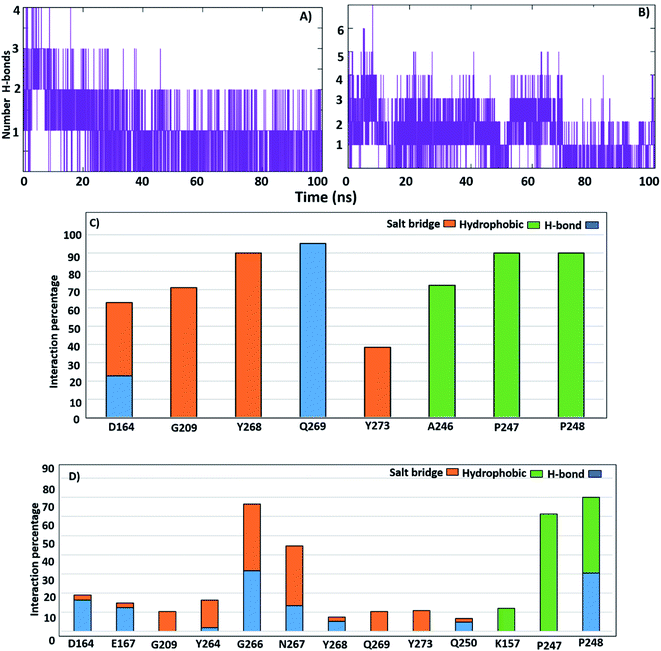 |
| Fig. 8 Number of H-bonds with SARS-CoV-2 PLpro putative pocket; (A) lead 1. (B) Lead 2. Percentage of H-bonds, salt bridge and hydrophobic occupations of the SARS-CoV-2 PLpro residues; (C) lead1. (D) Lead 2. | |
To study the H-bonds and salt bridge deeply, the distance between the two inhibitors and key residues was investigated. The N2 atom of lead 1 formed an H-bond at distance 2 Å distance in the first 20 ns of the simulation then continued the remaining of the MD simulation at distance 0.4 nm while O3 formed H-bond at distance 0.6 nm in first 20 ns then continued the remaining of the dynamic at 0.3 nm (Fig. S4A and B†). This indicated that compound lead 1 adopted two conformations during the simulation and that the H-bond with Q269 was stable and continuous during the simulation, which helped stabilize the compound (Fig. S4A, B†). The distance between N3 and O3 of lead 1 and Y268 fluctuated between 0.3 nm and 0.7 nm and then continued at approximately 0.3 nm, while the distance between lead 1 and Q209 in the first 20 ns was approximately 1.2 nm and then continued the simulation at 0.2 nm. These results supported the salt-bridge interactions between lead 1 and Y268 and Q209 (Fig. S4C and D†). The distance between lead 1 and P247 and P248 was approximately 0.25 in all the simulations, which may explain the hydrophobic interaction with hydrophobic S4 site residues. However, the distance between lead 1 and S245 and A246 was more than 0.6 nm during the first 23 ns and then continued at 0.25 nm (Fig. S4E†).
Lead 2 showed H-bonds with P248 and G266, and the distance between lead 2 and P248 was approximately 7–11 Å in the first 23 ns and then became approximately 0.2 nm. From 70 ns, the distance increased again to approximately 0.7 nm (Fig. S5A†). Conversely, from 23 ns until the end of the simulation, the distance between lead 2 and G266 was stable at 0.2 nm (Fig. S5C and D†). The distance between lead 2 and N267 fluctuated at approximately 1 nm during the first 18 ns and then continued from 18–60 ns at 0.2 nm. Followed by a sharp increase in the distance and fluctuation from 60 ns until the end of the simulation (Fig. S5E†).
3.3.5. MD simulation trajectories analysis. During the MD simulation of lead 1-PLpro complex, we observed that at the start of the dynamics, the first confirmation 2-(3-hydroxy-4-methoxyphenylamino) thiazol-4(5H)-one was stable inside hydrophobic site S4, 5-a5-amino-2-methoxyphenol formed hydrophobic interactions with P248 and T301, and thiazole showed π–π stacking with Y264 and Y273. The 5-(aminomethyl)-6-hydroxypyrimidine-2,4(1H,3H)-dione moiety and 4-acetamidobenzoic acid oriented up and interacted with Y268 and Q269 (BL2 loop) (Fig. 9A and B). From 30 ns to the end of the simulation, the 5-amino-2-methoxyphenol moved up to face A246 and P247 and formed an H-bond with G209, while the 5-(aminomethyl)-6-hydroxypyrimidine-2,4(1H,3H)-dione moiety, 4-acetamidobenzoic acid and thiazole moieties remained in their position and continued to interact with Y268 and Q269 (Fig. 9C–F).
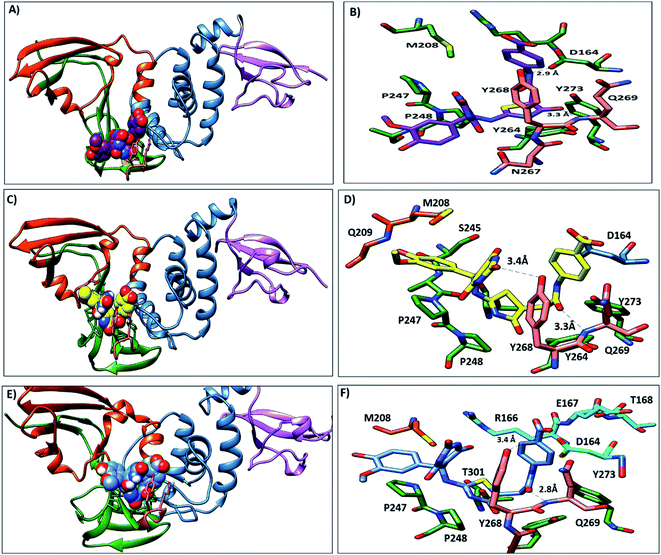 |
| Fig. 9 Representative structures of lead1 within PLpro putative pocket. (A) Structure of lead 1 (spheres, carbon atoms violet) at the beginning of MD simulation. (B) Interaction of lead 1 with the key residues. (C) Lead 1 after 30 ns (sphere, carbon atoms yellow), (D) the interaction of lead 1 at 30 ns and it is observed that 5-amino-2-methoxyphenol moved upward and showed hydrophobic interactions with P247 and A246 and 6-hydroxy-pyrmidine-dione formed H-bond with Y268. (E) Lead 1 at the end of the simulation (sphere, carbon atoms blue), (F) the interaction of lead 1 at the end of the MD. | |
Lead 2 structure consisted of two moieties of N-(2-hydroxy benzylidene-amino)-1,2,5-oxadiazole-3-carboxamidine. At the beginning of lead 2-PLpro simulation, N-(2-hydroxy benzylidene amino)-1,2,5-oxadiazole-3-carboxamidine moiety accommodated in the S4 site formed hydrophobic interactions with P248, P247, Y264 and Y273 (Fig. 10A and B). On the other hand, the second moiety N-(2-hydroxy benzylidene-amino)-1,2,5-oxadiazole-3-carboxamidine was located between the BL2 loop residues Y268 and Q269 and the back residues R166 and E167. Then, N-(2-hydroxy benzylideneamino)-1,2,5-oxadiazole-3-carboxamidine moved up and formed hydrophobic interactions with P247 and P248. Y268 and Q269 moved outward, which facilitated movement of the second N-(2-hydroxy benzylideneamino)-1,2,5-oxadiazole-3-carboxamidine moiety, accommodated the front pocket and interacted with the BL2 loop (Fig. 10C–F).
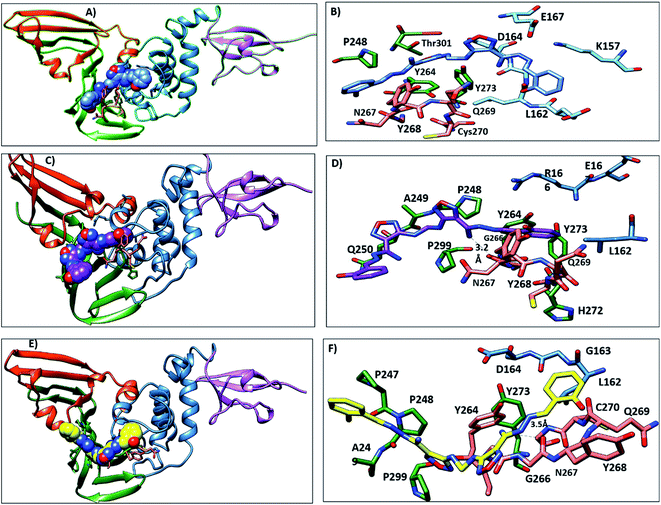 |
| Fig. 10 Representative structures of lead 2 within PLpro putative pocket. (A) Structure of lead 2 (spheres, carbon atoms blue) at starting of MD simulation. (B) interaction of lead 2 with the key residues (C) lead 2 after 30 ns (sphere, carbon atoms violet), (D) the interaction of lead 2 at 30 ns and it is observed that N-(2-hydroxy benzylidene-amino)- oxadiazole showed hydrophobic interactions with A249 and P299 and NH moiety formed H-bond with G266. (E) Lead 2 at the end of the simulation (sphere, carbon atoms yellow), (F) the interaction of lead 2 at the end of the MD. | |
3.4. Free energy binding for complexes
The binding free energies of lead 1, lead 2 and all reference compounds were calculated using the MM-PBSA method on 500 snapshots extracted from the last 50 ns of simulations. Lead 1, 2 and references complexes showed negative binding free energies (Table 2), indicating that all the complexes were stable. Compound 6 demonstrated the highest free binding energy (−115.4 ± 0.5 kJ mol−1), followed by compound 2 and GRL0617 (−92.3 ± 0.6 and −90.4 ± 0.6 kJ mol−1) respectively. Lead 1 and VAN showed the lowest free binding energies (−27.2 ± 0.6 and −26.1 ± 0.8 kJ mol−1) respectively. Lead 2 showed a moderate free binding energy (−55.6 ± 0.1 kJ mol−1). For all inhibitor-complexes, the contributions of van der Waals (ΔEvdw), electrostatic (ΔEele) and non-polar solvation (ΔGnp) were attractive for protein–ligands interactions. The (ΔGnp) contributed to the free binding energy with low values, the polar free solvation energy (ΔGpol) was positive for all the systems, indicating that the unfavourable bindings of inhibitors with the enzyme. Furthermore, van der Waals interaction represented the main interactions for all inhibitors, Despite of the promising inhibition results of lead 1 and 2 they demonstrated weak to moderate free binding energy compared to the refence compounds.
Table 2 Energy contribution of the promising hits and reported inhibitors to the total free energy
Inhibitors |
ΔEvdwa |
ΔEelea |
ΔGpola |
ΔGnpa |
ΔGbinda |
ΔEvdW, contributions by van der Waals interactions; ΔEele, electrostatic energy; ΔGpol, polar solvation energy; ΔGnp, nonpolar solvation energy, ΔGbind, binding affinity. a (kJ mol−1). |
Simeprevir (SIM) |
−110.6 ± 1.3 |
−12.4 ± 1.6 |
84.2 ± 3.2 |
−11.9 ± 0.1 |
−50.8 ± 1.2 |
Vaniprevir (VAN) |
−113.1 ± 0.7 |
31.8 ± 0.8 |
67.2 ± 0.7 |
−13.1 ± 0.1 |
−27.2 ± 0.6 |
GRL0617 |
−128.5 ± 0.8 |
−43.0 ± 0.3 |
94.7 ± 0.7 |
−13.6 ± 0.1 |
−90.4 ± 0.6 |
Lead 1 |
−136.2 ± 0.6 |
−139.6 ± 1.1 |
264.1 ± 1.2 |
−14.4 ± 0.1 |
−26.1 ± 0.8 |
Compound 2 |
−168.4 ± 0.4 |
−61.6 ± 1.2 |
155.1 ± 1.4 |
−17.35 ± 0.1 |
−92.3 ± 0.6 |
Compound 3 |
−171.4 ± 0.4 |
−61.06 ± 0.6 |
162.0 ± 0.8 |
−17.3 ± 0.1 |
−87.8 ± 0.5 |
Compound 6 |
−171.3 ± 0.6 |
−53.8 ± 0.4 |
125.9 ± 0.7 |
−16.2 ± 0.1 |
−115.4 ± 0.5 |
Lead 2 |
−174.8 ± 0.7 |
−62.6 ± 0.7 |
199.7 ± 0.9 |
−17.7 ± 0.1 |
−55.6 ± 0.1 |
3.5. Protein-inhibitor binding affinity
The key residues involved in the binding of ligands to the SARS-CoV-2 PLpro were investigated using ΔGbind residue calculation based on the MM/PBSA method. The obtained results are shown in Fig. 12. Y264, Y268 and Q269, the highest hotspot residues, contributed to both inhibitors lead 1 and lead 2 with ΔGbind of residues (−7.80, −12.58 and −4.67 kJ mol−1) and (−10.11, −5.86, −0.75 kJ mol−1), respectively. Y264, Y268 and Q269 are part of the BL2 loop, which is important for closing and opening the putative pocket (Fig. 12); these findings indicated good binding of the inhibitors within the pocket, although the Q269 contribution was small for lead 2. The hydrophobic residues P247 and P248 contributed to the binding energy of lead 1 at −5.79 kJ mol−1 and −5.62 kJ mol−1 and for lead 2 at −4.79 kJ mol−1 and −6.31 kJ mol−1, respectively. D164 showed a strong contribution (−9.5 kJ mol−1) to the binding energy of lead 1, which agrees with the H-bond analyses (Fig. 11). These results suggested that the key residues contributing to the binding energy for both lead 1 and lead 2 are P247, P248, Y264, Y268 and Q269.
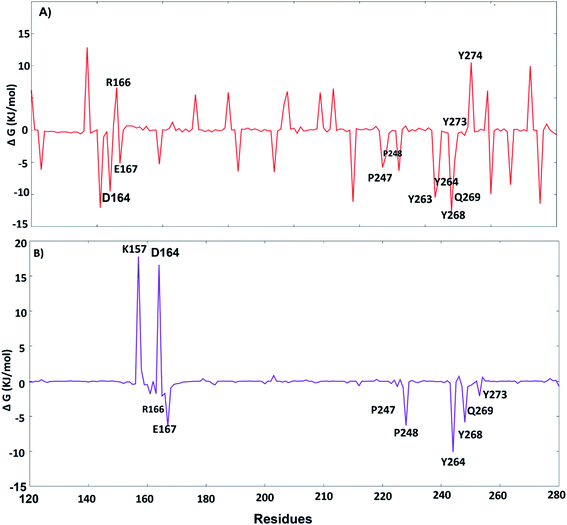 |
| Fig. 11 Per-residue free energy decomposition: (A) lead 1, (B) lead 2. | |
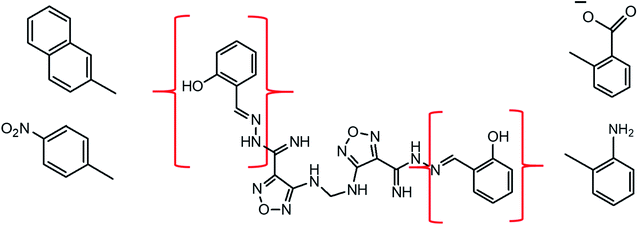 |
| Fig. 12 Rational drug design of the SARS-CoV-2 PLpro inhibitors.2D structure of lead 2 with possible modified fragments. | |
3.6. Rational drug design
According to the results, the lead 2 inhibitor showed a promising IC50 as a PLpro inhibitor, moderate free binding energy, and good H-bond interactions with BL2 loops. However, its free binding energy was higher than GRL06177, compounds 2, 3 and 6. To improve the binding of lead 2 and hence its IC50, structural modification of P4 and P1 sites is required, our suggestions are as follows: (i) replacement of 2-(2-hydroxy benzylidene) hydrazine with a naphthalene moiety, addition of a nitrobenzene moiety to facilitate hydrophobic interaction with P248, Y264 and T301 and maintain the binding with the S4 site; (ii) replacement of 2-(iminomethyl)phenol with aniline or benzoate to reduce the size of the molecule and enhance H-bonds with Y268 and Q269, which are important in the stabilization of the BL2 loop (Fig. 12). Docking studies were performed for predicted compounds (Table 3), all predicted derivatives showed binding score better than lead 1 and lead 2 expect derivative 3 with amino group. These results revealed that the predicted structures are likely promising SARS-CoV-2 PLpro inhibitors.
Table 3 Chemical structures of predicted compounds and their binding energies by BUDE
Compounds |
Structure |
BUDE binding |
Energy kJ mol−1 |
Derivative 1 |
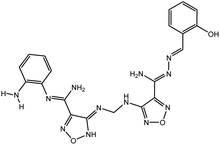 |
−77.5 |
Derivative 2 |
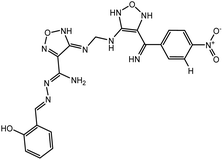 |
−80.7 |
Derivative 3 |
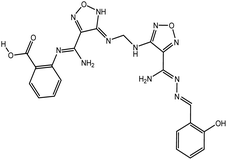 |
−83.1 |
Derivative 4 |
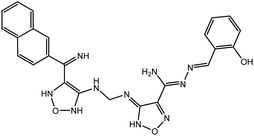 |
−91.7 |
4 Conclusion
Lead 1 and lead 2 illustrated promising IC50 values (0.085 μM and 0.063 μM, respectively), against SARS-CoV-2 PLpro which encouraged us to study the molecular dynamics of the two compounds within SARS-CoV-2 PLpro binding pocket, and compare the results with the reference compounds GRL0617, compounds 2, 3, 6, SIM and VAN using post MD analyses, binding free energy and per residue free energy decomposition tools. Both inhibitors lead 1 and 2 were stable within the binding pocket along the simulation. H-bonds and ligand interactions showed strong H-bonds for both inhibitors lead 1 and lead 2 with percentages of 91.8% and 75.9%, respectively. The distances between lead 1 and key residues suggested that the compounds adopted one conformation in the first 23 ns and changed to a second conformation in the rest of the dynamics. Also, lead 2 adopted two confirmations during the simulation and stabilized through interactions with P248, G266 and N267.
Analysis of the MD simulation trajectory of lead 1 complex showed that the compound was stable in the binding pocket along the simulation, while MD of lead 2 illustrated that the BL2 loop moved outward and adopted a second conformation to accommodate the inhibitor. The ΔGbind residue decomposition for both inhibitors revealed that residues E167, P247, P248, Y264, Y268 and Q269 are important for the binding of lead 1 and lead 2. D164 is important for binding of lead 1.
The rational design of lead 2 suggested that replacement of the 2-(2-hydroxybenzylidene) hydrazine moiety with naphthalene or nitrobenzene at the P4 position of lead 2 reduced the large size of the molecule and consequently improved the hydrophobic interactions with P248, Y264 and Y273 (S4 hydrophobic residue). However, replacement of the 2-(iminomethyl) phenol in P1 with a polar substituent as an aniline and benzoate group enhanced interactions with BL2 residues Y268 and Q269. These potential results can be helpful for the development of derivatives of lead 1 and lead 2 structures with more potent SARS-CoV-2 PLpro inhibition abilities.
Abbreviations
PLpro | Pain like protease |
BUDE | Bristol University Docking Engine |
Coronavirus | (CoV) |
MM-PBSA | Molecular mechanics Poisson–Boltzmann surface area |
RMSD | Root Mean Square Deviation |
RMSF | Root Mean Square Fluctuation |
Funding
No funding for this study.
Conflicts of interest
The author declares no competing financial interest.
Acknowledgements
The authors are grateful to Dr Richard Session at the molecular modelling laboratory, University of Bristol, for providing computing resources and access to the BUDE program.
References
- X. Li, W. Wang, X. Zhao, J. Zai, Q. Zhao, Y. Li and A. Chaillon, Transmission dynamics and evolutionary history of 2019-nCoV, J. Med. Virol., 2020, 92(5), 501–511 CrossRef CAS PubMed.
- X. Qian, R. Ren, Y. Wang, Y. Guo, J. Fang, Z. Wu, M. o. S. Committee and Society of Global Health, Chinese Preventive Medicine Association. Fighting against the common enemy of COVID-19: a practice of building a community with a shared future for mankind, Infect. Dis. Poverty, 2020, 9(1), 34 CrossRef PubMed.
- F. A. Rabi, M. S. Al Zoubi, G. A. Kasasbeh, D. M. Salameh and A. D. Al-Nasser, SARS-CoV-2 and coronavirus disease 2019: what we know so far, Pathogens, 2020, 9(3), 231 CrossRef CAS PubMed.
- C. Drosten, S. Günther, W. Preiser, S. Van Der Werf, H.-R. Brodt, S. Becker, H. Rabenau, M. Panning, L. Kolesnikova and R. A. Fouchier, Identification of a novel coronavirus in patients with severe acute respiratory syndrome, N. Engl. J. Med., 2003, 348(20), 1967–1976 CrossRef CAS PubMed.
- R.-H. Xu, J.-F. He, M. R. Evans, G.-W. Peng, H. E. Field, D.-W. Yu, C.-K. Lee, H.-M. Luo, W.-S. Lin and P. Lin, Epidemiologic clues to SARS origin in China, Emerging Infect. Dis., 2004, 10(6), 1030 CrossRef PubMed.
- R. J. De Groot, S. C. Baker, R. S. Baric, C. S. Brown, C. Drosten, L. Enjuanes, R. A. Fouchier, M. Galiano, A. E. Gorbalenya and Z. A. Memish, Commentary: Middle east respiratory syndrome coronavirus (mers-cov): announcement of the coronavirus study group, J. Virol., 2013, 87(14), 7790–7792 CrossRef CAS PubMed.
- A. M. Zaki, S. Van Boheemen, T. M. Bestebroer, A. D. Osterhaus and R. A. Fouchier, Isolation of a novel coronavirus from a man with pneumonia in Saudi Arabia, N. Engl. J. Med., 2012, 367(19), 1814–1820 CrossRef CAS PubMed.
- E. De Wit, A. L. Rasmussen, D. Falzarano, T. Bushmaker, F. Feldmann, D. L. Brining, E. R. Fischer, C. Martellaro, A. Okumura and J. Chang, Middle East respiratory syndrome coronavirus (MERS-CoV) causes transient lower respiratory tract infection in rhesus macaques, Proc. Natl. Acad. Sci., 2013, 110(41), 16598–16603 CrossRef CAS PubMed.
- D. Kim, J.-Y. Lee, J.-S. Yang, J. W. Kim, V. N. Kim and H. Chang, The architecture of SARS-CoV-2 transcriptome, Cell, 2020, 181(4), 914–921 CrossRef CAS PubMed.
- R. Giri, T. Bhardwaj, M. Shegane, B. R. Gehi, P. Kumar, K. Gadhave, C. J. Oldfield and V. N. Uversky, Understanding COVID-19 via comparative analysis of dark proteomes of SARS-CoV-2, human SARS and bat SARS-like coronaviruses, Cell. Mol. Life Sci., 2021, 78(4), 1655–1688 CrossRef CAS PubMed.
- E. Snijder, E. Decroly and J. Ziebuhr, The nonstructural proteins directing coronavirus RNA synthesis and processing, Adv. Virus Res., 2016, 96, 59–126 CAS.
- D. A. Milenković, D. S. Dimić, E. H. Avdović and Z. S. Marković, Several coumarin derivatives and their Pd (II) complexes as potential inhibitors of the main protease of SARS-CoV-2, an in silico approach, RSC Adv., 2020, 10(58), 35099–35108 RSC.
- S. A. Amin, S. Banerjee, K. Ghosh, S. Gayen and T. Jha, Protease targeted COVID-19 drug discovery and its challenges: Insight into viral main protease (Mpro) and papain-like protease (PLpro) inhibitors, Bioorg. Med. Chem., 2020, 115860 Search PubMed.
- J. Lei, Y. Kusov and R. Hilgenfeld, Nsp3 of coronaviruses: structures and functions
of a large multi-domain protein, Antiviral Res., 2018, 149, 58–74 CrossRef CAS PubMed.
- W.-j. Guan, Z.-y. Ni, Y. Hu, W.-h. Liang, C.-q. Ou, J.-x. He, L. Liu, H. Shan, C.-l. Lei and D. S. Hui, Clinical characteristics of coronavirus disease 2019 in China, N. Engl. J. Med., 2020, 382(18), 1708–1720 CrossRef CAS PubMed.
- K. Bafna, K. White, B. Harish, R. Rosales, T. A. Ramelot, T. B. Acton, E. Moreno, T. Kehrer, L. Miorin and C. A. Royer, Hepatitis C virus drugs that inhibit SARS-CoV-2 papain-like protease synergize with remdesivir to suppress viral replication in cell culture, Cell Rep., 2021, 35(7), 109133 CrossRef CAS PubMed.
- F. Wu, S. Zhao, B. Yu, Y.-M. Chen, W. Wang, Z.-G. Song, Y. Hu, Z.-W. Tao, J.-H. Tian and Y.-Y. Pei, A new coronavirus associated with human respiratory disease in China, Nature, 2020, 579(7798), 265–269 CrossRef CAS PubMed.
- A. M. Mielech, Y. Chen, A. D. Mesecar and S. C. Baker, Nidovirus papain-like proteases: multifunctional enzymes with protease, deubiquitinating and deISGylating activities, Virus Res., 2014, 194, 184–190 CrossRef CAS PubMed.
- B. H. Harcourt, D. Jukneliene, A. Kanjanahaluethai, J. Bechill, K. M. Severson, C. M. Smith, P. A. Rota and S. C. Baker, Identification of severe acute respiratory syndrome coronavirus replicase products and characterization of papain-like protease activity, J. Virol., 2004, 78(24), 13600–13612 CrossRef CAS PubMed.
- Y.-S. Han, G.-G. Chang, C.-G. Juo, H.-J. Lee, S.-H. Yeh, J. T.-A. Hsu and X. Chen, Papain-like protease 2 (PLP2) from severe acute respiratory syndrome coronavirus (SARS-CoV): expression, purification, characterization, and inhibition, Biochemistry, 2005, 44(30), 10349–10359 CrossRef CAS PubMed.
- S. Li, C. Wang, Y. Jou, S. Huang, L. Hsiao, L. Wan, Y. Lin, S. Kung, C. Lin and S. C. P.-L. P. Inhibits, the TLR7 Signaling Pathway through Removing Lys63-Linked Polyubiquitination of TRAF3 and TRAF6, Int. J. Mol. Sci., 2016, 17, 678 CrossRef PubMed.
- K. Ratia, A. Kilianski, Y. M. Baez-Santos, S. C. Baker and A. Mesecar, Structural basis for the ubiquitin-linkage specificity and deISGylating activity of SARS-CoV papain-like protease, PLoS Pathog., 2014, 10(5), e1004113 CrossRef PubMed.
- S. G. Devaraj, N. Wang, Z. Chen, Z. Chen, M. Tseng, N. Barretto, R. Lin, C. J. Peters, C.-T. K. Tseng and S. C. Baker, Regulation of IRF-3-dependent innate immunity by the papain-like protease domain of the severe acute respiratory syndrome coronavirus, J. Biol. Chem., 2007, 282(44), 32208–32221 CrossRef CAS PubMed.
- M. A. Clementz, Z. Chen, B. S. Banach, Y. Wang, L. Sun, K. Ratia, Y. M. Baez-Santos, J. Wang, J. Takayama and A. K. Ghosh, Deubiquitinating and interferon antagonism activities of coronavirus papain-like proteases, J. Virol., 2010, 84(9), 4619–4629 CrossRef CAS PubMed.
- D. Shin, R. Mukherjee, D. Grewe, D. Bojkova, K. Baek, A. Bhattacharya, L. Schulz, M. Widera, A. R. Mehdipour and G. Tascher, Papain-like protease regulates SARS-CoV-2 viral spread and innate immunity, Nature, 2020, 587(7835), 657–662 CrossRef CAS PubMed.
- M. Macchiagodena, M. Pagliai and P. Procacci, Identification of potential binders of the main protease 3CLpro of the COVID-19 via structure-based ligand design and molecular modeling, Chem. Phys. Lett., 2020, 750, 137489 CrossRef CAS PubMed.
- S. A. Amin, S. Banerjee, S. Gayen and T. Jha, Protease targeted COVID-19 drug discovery: What we have learned from the past SARS-CoV inhibitors?, Eur. J. Med. Chem., 2021, 113294 CrossRef CAS PubMed.
- J. Osipiuk, S.-A. Azizi, S. Dvorkin, M. Endres, R. Jedrzejczak, K. A. Jones, S. Kang, R. S. Kathayat, Y. Kim and V. G. Lisnyak, Structure of papain-like protease from SARS-CoV-2 and its complexes with non-covalent inhibitors, Nat. Commun., 2021, 12(1), 1–9 CrossRef PubMed.
- R. Hilgenfeld, From SARS to MERS: crystallographic studies on coronaviral proteases enable antiviral drug design, FEBS J., 2014, 281(18), 4085–4096 CrossRef CAS PubMed.
- N. Barretto, D. Jukneliene, K. Ratia, Z. Chen, A. D. Mesecar and S. C. Baker, The papain-like protease of severe acute respiratory syndrome coronavirus has deubiquitinating activity, J. Virol., 2005, 79(24), 15189–15198 CrossRef CAS PubMed.
- C.-Y. Chou, H.-Y. Lai, H.-Y. Chen, S.-C. Cheng, K.-W. Cheng and Y.-W. Chou, Structural basis for catalysis and ubiquitin recognition by the severe acute respiratory syndrome coronavirus papain-like protease, Acta Crystallogr., Sect. D: Biol. Crystallogr., 2014, 70(2), 572–581 CrossRef CAS PubMed.
- T. Huynh, W. Cornell and B. Luan, In silico Exploration of Inhibitors for SARS-CoV-2’s Papain-Like Protease, Front. Chem., 2021, 8, 1–9 RSC.
- W. Rut, Z. Lv, M. Zmudzinski, S. Patchett, D. Nayak, S. J. Snipas, F. El Oualid, T. T. Huang, M. Bekes and M. Drag, Activity profiling and crystal structures of inhibitor-bound SARS-CoV-2 papain-like protease: A framework for anti–COVID-19 drug design, Sci. Adv., 2020, 6(42), eabd4596 CrossRef CAS PubMed.
- X. Gao, B. Qin, P. Chen, K. Zhu, P. Hou, J. A. Wojdyla, M. Wang and S. Cui, Crystal structure of SARS-CoV-2 papain-like protease, Acta Pharm. Sin. B, 2021, 11(1), 237–245 CrossRef CAS PubMed.
- T. Klemm, G. Ebert, D. J. Calleja, C. C. Allison, L. W. Richardson, J. P. Bernardini, B. G. Lu, N. W. Kuchel, C. Grohmann and Y. Shibata, Mechanism and inhibition of the papain-like protease, PLpro, of SARS-CoV-2, EMBO J., 2020, 39(18), e106275 CrossRef CAS PubMed.
- M. A. Alamri, M. T. ul Qamar, M. U. Mirza, S. M. Alqahtani, M. Froeyen and L.-L. Chen, Discovery of human coronaviruses pan-papain-like protease inhibitors using computational approaches, J. Pharm. Anal., 2020, 10(6), 546–559 CrossRef PubMed.
- Ž. B. Milanović, M. R. Antonijević, A. D. Amić, E. H. Avdović, D. S. Dimić, D. A. Milenković and Z. S. Marković, Inhibitory activity of quercetin, its metabolite, and standard antiviral drugs towards enzymes essential for SARS-CoV-2: the role of acid–base equilibria, RSC Adv., 2021, 11(5), 2838–2847 RSC.
- A. Stasiulewicz, A. W. Maksymiuk, M. L. Nguyen, B. Bełza and J. I. Sulkowska, SARS-CoV-2 Papain-Like Protease Potential Inhibitors—In Silico Quantitative Assessment, Int. J. Mol. Sci., 2021, 22(8), 3957 CrossRef CAS PubMed.
- M. U. Mirza, S. Ahmad, I. Abdullah and M. Froeyen, Identification of novel human USP2 inhibitor and its putative role in treatment of COVID-19 by inhibiting SARS-CoV-2 papain-like (PLpro) protease, Comput. Biol. Chem., 2020, 89, 107376 CrossRef CAS PubMed.
- S. A. Elseginy, B. Fayed, R. Hamdy, N. Mahrous, A. Mostafa, A. M. Almehdi and S. S. Soliman, Promising anti-SARS-CoV-2 drugs by effective dual targeting against the viral and host proteases, Bioorg. Med. Chem. Lett., 2021, 43, 128099 CrossRef CAS PubMed.
- M. Martineau, S. McIntosh-Smith and W. Gaudin, in Evaluating OpenMP 4.0's effectiveness as a heterogeneous parallel programming model, 2016 IEEE International Parallel and Distributed Processing Symposium Workshops (IPDPSW), IEEE, 2016, pp. 338–347 Search PubMed.
-
(a) S. McIntosh-Smith, J. Price, R. B. Sessions and A. A. Ibarra, High performance in silico virtual drug screening on many-core processors, International journal of high performance computing applications, 2015, 29(2), 119–134 CrossRef PubMed;
(b) S. A. Elseginy, Virtual screening and structure-based 3D pharmacophore approach to identify small-molecule inhibitors of SARS-CoV-2 Mpro, J. Biomol. Struct. Dyn., 2021, 1–17 CrossRef PubMed.
- C. Ma, M. D. Sacco, Z. Xia, G. Lambrinidis, J. A. Townsend, Y. Hu, X. Meng, T. Szeto, M. Ba and X. Zhang, Discovery of SARS-CoV-2 Papain-like Protease Inhibitors through a Combination of High-Throughput Screening and a FlipGFP-Based Reporter Assay, ACS Cent. Sci., 2021, 1245–1260 CrossRef CAS PubMed.
- T. J. Dolinsky, J. E. Nielsen, J. A. McCammon and N. A. Baker, PDB2PQR: an automated pipeline for the setup of Poisson–Boltzmann electrostatics calculations, Nucleic Acids Res., 2004, 32(2), W665–W667 CrossRef CAS PubMed.
- S. Vilar, G. Cozza and S. Moro, Medicinal chemistry and the molecular operating environment (MOE): application of QSAR and molecular docking to drug discovery, Curr. Top. Med. Chem., 2008, 8(18), 1555–1572 CrossRef CAS PubMed.
- U. Pieper, N. Eswar, F. P. Davis, H. Braberg, M. S. Madhusudhan, A. Rossi, M. Marti-Renom, R. Karchin, B. M. Webb and D. Eramian, MODBASE: a database of annotated comparative protein structure models and associated resources, Nucleic Acids Res., 2006, 34(1), D291–D295 CrossRef CAS PubMed.
- E. F. Pettersen, T. D. Goddard, C. C. Huang, G. S. Couch, D. M. Greenblatt, E. C. Meng and T. E. Ferrin, UCSF Chimera—a visualization system for exploratory research and analysis, J. Comput. Chem., 2004, 25(13), 1605–1612 CrossRef CAS PubMed.
- H. Berendsen, D. vanderSpoel and R. vanDrunen, Comput. Phys. Commun., 1995, 91, 43–56 CrossRef CAS.
- A. W. S. Da Silva and W. F. Vranken, ACPYPE-Antechamber python parser interface, BMC Res. Notes, 2012, 5(1), 1–8 CrossRef PubMed.
-
(a) J. Wang, R. M. Wolf, J. W. Caldwell, P. A. Kollman and D. A. Case, Development and testing of a general amber force field, J. Comput. Chem., 2004, 25(9), 1157–1174 CrossRef CAS PubMed;
(b) K. Lindorff-Larsen, S. Piana, K. Palmo, P. Maragakis, J. L. Klepeis, R. O. Dror and D. E. Shaw, Improved side-chain torsion potentials for the Amber ff99SB protein force field, Proteins: Struct., Funct., Bioinf., 2010, 78(8), 1950–1958 CrossRef CAS PubMed.
- H. J. Berendsen, J. P. Postma, W. F. van Gunsteren and J. Hermans, Interaction models for water in relation to protein hydration, in Intermolecular forces, Springer, 1981, pp. 331–342 Search PubMed.
- W. L. Jorgensen, J. Chandrasekhar, J. D. Madura, R. W. Impey and M. L. Klein, Comparison of simple potential functions for simulating liquid water, J. Chem. Phys., 1983, 79(2), 926–935 CrossRef CAS.
- M. J. Abraham, T. Murtola, R. Schulz, S. Páll, J. C. Smith, B. Hess and E. Lindahl, GROMACS: High performance molecular simulations through multi-level parallelism from laptops to supercomputers, SoftwareX, 2015, 1, 19–25 CrossRef.
- H. Wang, F. Dommert and C. Holm, Optimizing working parameters of the smooth particle mesh Ewald algorithm in terms of accuracy and efficiency, J. Chem. Phys., 2010, 133(3), 034117 CrossRef PubMed.
- P. Turner, XMGRACE, Version 5.1. 19, Center for Coastal and Land-Margin Research, Oregon Graduate Institute of Science and Technology, Beaverton, OR 2005 Search PubMed.
- W. L. DeLano, The PyMOL user's manual, DeLano Scientific, San Carlos, CA, 2002, p. 452 Search PubMed.
- W. Humphrey, A. Dalke and K. Schulten, VMD: visual molecular dynamics, J. Mol. Graphics, 1996, 14(1), 33–38 CrossRef CAS PubMed.
- R. Kumari, R. Kumar, O. S. D. D. Consortium and A. Lynn, g_mmpbsa A GROMACS tool for high-throughput MM-PBSA calculations, J. Chem. Inf. Model., 2014, 54(7), 1951–1962 CrossRef CAS PubMed.
- B. R. Miller III, T. D. McGee Jr, J. M. Swails, N. Homeyer, H. Gohlke and A. E. Roitberg, MMPBSA. py: an efficient program for end-state free energy calculations, J. Chem. Theory Comput., 2012, 8(9), 3314–3321 CrossRef PubMed.
- A. M. Mielech, A. Kilianski, Y. M. Baez-Santos, A. D. Mesecar and S. C. Baker, MERS-CoV papain-like protease has deISGylating and deubiquitinating activities, Virology, 2014, 450, 64–70 CrossRef PubMed.
- Y. K. Bosken, T. Cholko, Y.-C. Lou, K.-P. Wu and C.-e. A. Chang, Insights into dynamics of inhibitor and ubiquitin-like protein binding in SARS-CoV-2 papain-like protease, Front. Mol. Biosci., 2020, 7, 174 CrossRef CAS PubMed.
- S. A. Amin, K. Ghosh, S. Gayen and T. Jha, Chemical-informatics approach to COVID-19 drug discovery: Monte Carlo based QSAR, virtual screening and molecular docking study of some in-house molecules as papain-like protease (PLpro) inhibitors, J. Biomol. Struct. Dyn., 2020, 1–10 CrossRef PubMed.
Footnote |
† Electronic supplementary information (ESI) available. See DOI: 10.1039/d1ra07845c |
|
This journal is © The Royal Society of Chemistry 2021 |
Click here to see how this site uses Cookies. View our privacy policy here.