DOI:
10.1039/D1RA06321A
(Paper)
RSC Adv., 2021,
11, 35165-35173
User-friendly lab-on-paper optical sensor for the rapid detection of bacterial spoilage in packaged meat products
Received
20th August 2021
, Accepted 23rd October 2021
First published on 31st October 2021
Abstract
A lab-on-paper colorimetric sensor for detection and quantification of bacterial meat spoilage is reported. Bromocresol purple (BCP) and bromothymol blue (BTB) were used for the construction of the proposed sensor. An Android application allowing fast detection and accurate quantification of bacteria in the spoiled chicken meat samples was developed. The sensor was applied to the determination of spoilage in real chicken-meat samples, at chiller and room temperatures, and can be used for producing smart meat-packaging films. Linearity ranges were found to be 11.2 × 103 to 1.12 × 106 and 38.0 × 103 to 1.12 × 106 CFU g−1 for BTB and BCP, respectively. The calibration plots showed correlation coefficients (r) of 0.9998 (slope: 2.48 g CFU−1) and 0.9999 (slope: 1.95 g CFU−1) in case of bromothymol blue and bromocresol purple, respectively. The Android application uses standard images to plot a calibration curve for calculating the microbial count in the samples and relates it to the standard limits. Thereafter, the application shows a message with the product's freshness degree ranging from excellent to poor.
Introduction
Meat is among the vast category of food products which are considered as highly perishable foods. Meat products consist of complex biomolecules (e.g. proteins and fats) in addition to water, so they may be susceptible to rapid spoilage by lipid oxidation and/or microbial contamination. Rapid spoilage and loss of fresh meat quality usually cause health threats to consumers and economic losses to producers which should not be underestimated.1 Generally, food quality is detected during the food supply chain by relatively expensive equipment such as gas chromatography,2 electromagnetic interrogation,3 spectroscopy4 and chemical sensing with electronic noses.5 The chemical methods applied for meat quality tests are via inspection of triphenyltetrazolium chloride (TTC), total volatile basic nitrogen (TVBN) and pH measurements.6 Selective enzymes such as putrescine oxidase and monoamine oxidases were used for the determination of different spoilage indicator amines such as histamine and putrescine.7,8 However, these methods are appropriate only for laboratory-based quality control tests and/or research levels; because they are time consuming, expensive, and require tedious procedure and well-trained personnel. Therefore, these methods cannot be used by end users at the point of consumption in order to check food quality. Basically, consumers determine food freshness by sensory evaluation (i.e. checking the product's odour, colour and texture) or through checking the date of expiration; however, it is difficult to make a decision via meat smell or texture.9 Therefore, developing a simple low-cost sensor platform with high ability to indicate the degree of food freshness may be a good step in minimizing losses in the food supply chain.
Accordingly, researchers made numerous efforts in the literature to develop techniques for monitoring meat freshness. Among these techniques are electronic devices, biosensors and colorimetric sensors.8,10,11 Since the process of spoilage is strongly related to changes in odour, several successful attempts to employ electronic noses, based on semiconductor metal oxides or conducting polymers, for monitoring meat spoilage were developed.12–14 Recently, few colorimetric sensor applications were reported based on optical dyes.15–18 The most widely used dyes for colorimetric sensors are solvatochromic and redox dyes19 which can be utilized for monitoring organic volatile compounds,20 industrial toxic chemicals19 and environmental pollutants.21 In a previous study,22 researchers developed a smart indicator for the detection of fish spoilage by using bromocresol green whose colour changes from yellow to blue as the pH of the sample increases upon TVBN production. In order to make more accurate systems for monitoring food freshness, several approaches suggested the use of a mixture of two or more pH-indicator dyes.23,24 Yet, almost all of these approaches lack the advantage of accurate quantification of the microbial count using a smart phone-based Android application. In fact, they are able to just differentiate among spoiled and fresh meat samples without counting the microbes that cause the spoilage reactions. In addition, they resembled the classical acid–base titration technique and sometimes it could be difficult to determine the spoilage threshold onset, where it may be too late or too early; since it is associated with the rate of microbial growth.25
Smart packaging operates chemical sensors to monitor food safety and quality from producers to consumers. This technology led to different sensor designs such as freshness indicators, and leakage, carbon dioxide, oxygen and pH detectors.10 Moreover, volatile gases produced from food can be used for freshness monitoring; on this basis, many sensor fabrications with optical dyes (e.g. natural dyes, pH indicators and Lewis dyes) were used for measuring gas products evolving from spoiled meat.26 In this case, the smart packaging is based on colorimetric indicators able to change their colours due to a reaction with volatile compounds produced by microorganisms/enzymes found in meat. For instance, Lee et al. used alizarin red S (ARS), bromocresol green (BCG), bromophenol blue (BPB), bromothymol blue (BTB), m-cresol purple (mCP), cresol red (CR), curcumin (CU) and thymol blue (TB) to prepare a colorimetric array for freshness monitoring of chicken breast.27 Moreover, Taghizadeh-Behbahani and co-workers proposed a method for determining acid dissociation constants via using a microfluidic system.28 Other work reported by Magnaghi describes the use of pH-indicator-embedded cellulose sheets for meat spoilage monitoring.29 The use of smart freshness indicators on packaged meat products has become the most comfortable, practical, simple and fast method because it allows for spoilage detection with naked eyes.30 Several commercialized freshness indicators are available for packaging applications such as Fresh-Check, CheckPoint and On Vu™.31 However, these freshness indicators do not allow the quantification of the amount of bacteria that cause spoilage to the product. Accurate bacterial quantification can enable the consumer to rank the product's freshness according to governmental standards; and hence it shall be easy to make a decision whether the product is suitable for customer use. However, quantifying spoilage bacteria is usually a lab-based process which requires certain sophisticated instruments that cannot be used in field.
Recently, smartphones have become an integral part of our daily lives due to their worldwide spread.32 These small devices can be employed in analytical procedures for field analysis in a number of biological/chemical applications33 as well as medical diagnosis.34,35 In the present work, an on-package lab-on-paper (LOP) smart-phone-based dual colorimetric sensor for meat freshness detection was developed. In addition, an Android application was developed to allow for easy and fast microbial quantification/ranking by end users. The proposed dual sensor is based on BCP and BTB as chromophores incorporated into a filter paper sensor.
It has been shown that smart phone utilization for colorimetric recognition can give the same precision as benchtop spectrometers.36 Smart-phone applications could be used to read pH changes in saliva and sweat,37 changes in blood typing based paper sensors,38 and to detect specific nucleic acid sequences in liquid samples.39 Various models of RGB colour as HSL or CIE give colour information from smart phone images.
With the advantages of smart phone integration and optical dyes for freshness detection, we aim at presenting a disposable LOP dual colorimetric sensor for rapid and easy chicken-meat quality monitoring. The on-package sensor is fabricated using the pH-indicator dyes BCP and BTB and was glued onto the inner side of the packaging film before storage. A smart phone high-definition camera was used to capture sensor images that are in turn manipulated with a pre-installed Android application to read the colour changes and provide qualitative/quantitative information about meat freshness. A study somewhat close to the work described herein reported a solution-based model for the application of smartphones in colorimetric detection of analytes in complex ambient light conditions.40
The novelty of the current work can be summarized in (i) providing an Android application for giving a primary indication about the amount of bacteria in the meat sample, (ii) application of a wide pH range-responsive paper-based sensor for colorimetric detection of changes in the meat product pH to indicate spoilage, and (iii) omitting the need for polymeric membranes and/or nanofiber materials for the preparation of the LOP, and exchanging them with the cost-effective filter papers.
Experimental
Reagents and chemicals
Standard BTB (Sigma-Aldrich, Germany), BCP (Sigma-Aldrich, Germany) and Whatman filter paper no. 1 were used to prepare the dual LOP colorimetric sensor. Stock solutions of the indicator dyes were prepared by dissolving accurately weighed amounts of each in 95% ethanol to give a concentration of 1% (w/v). Peptone water (Oxoid, CM0009) was used for microbial analysis by the aerobic plate count (APC) method.
Dual sensor preparation
Circular filter-paper pieces with diameters of 15 and 35 mm were accurately cut and soaked into 20 mL of the indicator stock solutions overnight at ambient temperature. Thereafter, the obtained wet coloured pieces were dried for 20 minutes at 40 °C. Finally, the small sensors were glued over the large ones in a concentric manner in order to form the dual sensor. The simultaneous use of two dyes (dual sensor) provides the advantage of wider pH range (BCP: 5.2–6.8 and BTB: 6.0–7.6); so that minute changes in the status of the meat product can be detected.
Application to chicken-fillet samples
Fresh boneless chicken fillet samples of normal pH (<5.8) were obtained from local poultry meat markets (Dokki, Giza, Egypt). The chicken fillet was cut into six pieces (each weighs 250 ± 10 g) and were divided into 2 groups (3 samples each) and placed in polypropylene-trays films. To show the applicability of the dual sensor for spoilage monitoring during storage, the sensor was placed onto the inner side of the chicken fillet packages to become in direct contact with the package atmosphere and the samples were kept under two different conditions; the first group was kept at room temperature (25 ± 2 °C) and the second group was kept in chiller temperature (4 ± 2 °C) and examined periodically for microbiological population, pH and TVBN. The evolving gaseous products of bacterial spoilage can interact with the dye in the sensor in presence of humidity causing the desired colour change which can then be detected by the Android smartphone. The spoilage of these samples was monitored with the aid of the developed Android application. An Infinix Note 4 smart phone's camera was used to capture the images of the sensors periodically before manipulation with the as-developed Android application. Thereafter, the RGB values of the sensors were measured and used for absorbance calculations using a blank dual sensor paper according to the work reported elsewhere.32 All experiments were carried out in triplicates. It is worth to mention that the dual sensors were not in direct contact with the meat itself so as to avoid chemical contamination of the samples.
For data acquisition and processing, the images captured by the used smartphone were analysed by the developed software based on calculating the light absorption according to the formula: A = −log[In/Iblank], where A is the absorbance, In and Iblank are the R, G or B values of the sample and blank, respectively.32 The software works automatically without any intervention by the end user.
Microbiological analysis
Microbial growth in chicken meat samples was investigated according to ref. 25. A 10 g sample of meat was aseptically weighed and then added to 90 mL of sterile bacteriological peptone water. The mixture was homogenized in Seward Stomacher (Lab Blender 400, Seward Lab, London, UK) for 60 s at room temperature. By using 0.1% peptone water, a serial decimal solution was prepared and duplicate 1 mL samples at the appropriate dilution ratio were poured onto the agar plate and incubated at 37 °C for 48 h. Colony-forming units (CFUs) were counted and reported as log [CFU g−1].
Measurement of pH and TVBN
The pH of the meat samples was measured periodically using a pH meter. Ten grams of meat samples were thoroughly homogenized with 100 mL of distilled water and the homogenate was used for pH determination.41
The TVBN content was measured according to the method developed elsewhere in ref. 42. Briefly, 5 g of the sample were homogenized with 20 mL of distilled water and filtered with a Whatman filter paper no. 1. The TVBN content was then determined in the filtrate with the Conway micropipette diffusion method and expressed as mg TVBN per 100 g of the chicken meat (mg%).
Sensory evaluation
Sensory evaluation of the chicken meat samples was carried out for refrigerated as well as room temperature samples by a group of 9 panelists. Panelists were asked to evaluate texture, odour and colour of the investigated samples. The sensory testing was carried out under the same conditions (i.e. lighting and ventilation). A simple 3-point scoring system was adopted for freshness status of chicken meat, “fresh”, “medium” and “spoiled”. Each attribute was scored on a continuous 3 to 0 hedonic scale where 3 being the highest quality score (fresh), 2 given to the acceptable product, 1 is the limit of acceptance or rejection and 0 given to the unacceptable sample (spoiled).43
Results and discussion
Smart packaging has become a promising technology in food industry as it allows the consumer to determine food freshness level directly before purchase/consumption. Here, a LOP freshness indicator was integrated with a newly developed smart-phone Android application and applied to monitoring freshness changes in chicken meat during the course of storage. The LOP sensor, employing the pH-sensitive dyes BCP and BTB, can respond to the changes caused by microbial spoilage via showing gradual changes in colour easily detectable by naked eyes (Fig. 1a). Furthermore, the proposed smart-phone Android application is able to make accurate expectations of the amount of bacteria found in the meat samples. Principally, meat products need to be refrigerated in order to increase its shelf life, yet they spoil rapidly at room temperature. Therefore, the ability of the proposed dual sensor to monitor the spoilage process at different temperatures (chilling and room temperatures) was studied by dividing the samples into two groups; the first group was stored in the refrigerator at 4 °C while the second group was stored at room temperature. The chicken meat samples were checked during the storage time by capturing images of the dual sensors three times daily using an Infinix Note 4 smart phone. Moreover, the amount of bacteria/metabolites was assayed by the standard microbiological/chemical tests in order to be compared with the proposed sensor results. Furthermore, the pH of the test samples was continuously monitored throughout the experiments.
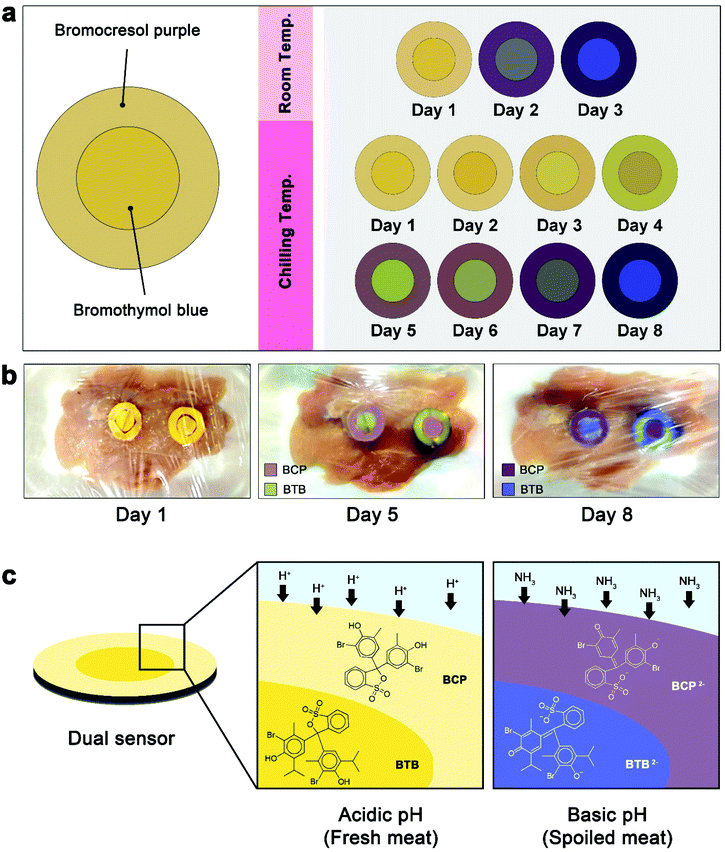 |
| Fig. 1 (a) The structure of the dual sensor platform and the obtained color changes at chiller and room temperatures. The numbers indicate storage periods in days. (b) Smart-phone images of one of the test chicken meat samples in days 1, 5 and 8 of storage at chiller temperature of 4 °C. The photos show the gradual change in dual sensor colors upon progression of bacterial spoilage. (c) Color reactions of BCP and BTB in fresh and spoiled meat samples. | |
The selection of BCP and BTB as indicator dyes relies on their abilities to demonstrate obvious and easily detectable colour changes according to the gradual increase in microbial count. These dyes are usually used as pH indicators in titrimetric analyses, where each indicator has a working pH range.44,45 BCP and BTB are yellow at acidic pH and change to purple and blue, respectively, at slightly basic media as a result of deprotonation (Fig. 1c).
Android application
Nowadays, Android is arguably the most popular platform for smart phone devices in the world. It brought the whole internet, especially Google, to all users of mobile devices such as smart phones, tablets, etc. Actually, this technology changed how we can communicate with one another. In addition, Android is supported with a built-in marketplace, “Google Play”, open for distributing new Android applications; thus facilitating the arrival of new applications to almost all end users.
In the present work, a novel Android application was developed for analysing colour changes in the samples and making freshness tests (Fig. 2). The application makes use of reference RGB colours (obtained from previously analysed standard samples) to construct a calibration graph from which it can accurately calculate the microbial counts in the test samples. The user just captures a photo for the dual LOP sensor present on the packaging film of the meat product and the Android application calculates the microbial count in it and makes a rapid freshness ranking based on the standard acceptable limits of the APC counts. At the end, a message appears on the smart phone screen where “Excellent” indicates 100% fresh meat, “Good” indicates APC counts directly below the limit and “Poor” indicates spoiled meat. The integration of the developed application made the freshness test easy and very fast; hence allowing the application of the proposed sensor at the point of purchase as well as in monitoring the freshness of stored meat products for a long time within few seconds. The detailed theoretical bases of data acquisition from the obtained smart phone images, absorbance calculations and construction of the calibration plots are given in the work reported by Abo Dena and Bayoumi.32 Briefly, black objects do absorb all colours of the electromagnetic spectrum and never reflect any while coloured ones absorb certain wavelengths only and appear coloured with the reflected portion of the spectrum. Intuitively, white-coloured objects do not absorb any wavelengths and reflect the R, G and B colours (i.e. they reflect the white colour coming out from the sun). The amount of the absorbed R, G or B colour can be calculated from eqn (1):
|
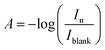 | (1) |
where
A is the theoretically calculated absorbance, and
In and
Iblank are the R, G or B values of the sample and blank, respectively.
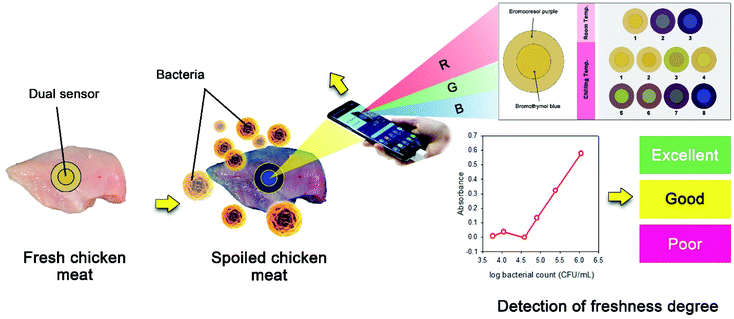 |
| Fig. 2 Schematic illustrating the process of checking the freshness of chicken meat with the proposed smart phone-based LOP dual sensor platform. After the analysis of the obtained RGB color, the Android application uses a calibration curve in order to provide a freshness grade for the test sample. | |
Microbial analysis
Commonly, there are some methods of microbial analysis for meat quality evaluation such as APC, Pseudomonas spp., lactic acid bacteria and Enterobacteriaceae counts. In addition, Escherichia coli can colonize in stored meat products resulting in progressive spoilage reactions. Microbial analysis of chicken meat samples was carried out with the APC method and the results are shown in Fig. 3(a and b). The acceptable limit of microorganisms for human consumption in fresh poultry is below 6–7 log CFU g−1.24 APC of the investigated chicken meat samples exceeded the limit (6 log CFU g−1) after 3 days of storage at room temperature and after 8 days of storage at chiller temperature. Responses of BCP and BTB colours were proportional to the observed changes in microbial populations. The samples analysed by APC method were used as standards for constructing calibration plots for the proposed sensors (Fig. 4).
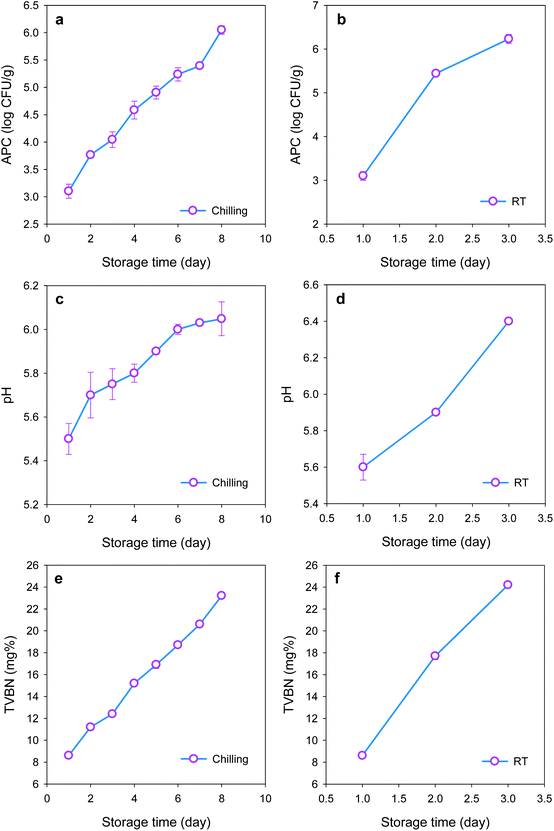 |
| Fig. 3 Changes in aerobic bacterial count (a and b), sample pH (c and d) and TVBN levels (e and f) of the investigated chicken fillet samples stored at chiller and room temperatures. RT: room temperature. | |
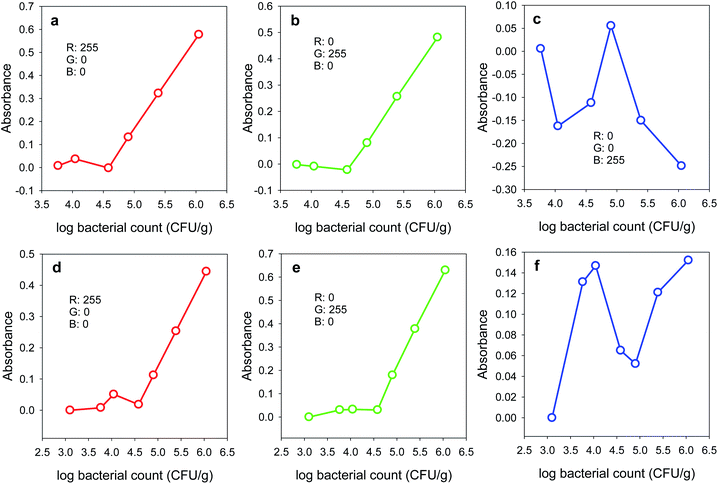 |
| Fig. 4 Calibration plots obtained using the calculated absorbance values acquisited from the LOP dual sensors images when applied to chicken meat samples using BTB (a–c) and BCP (d–f). The colors denote the R-, G-, and B-colors used to calculate the absorbance in the corresponding plot. | |
pH and total volatile basic nitrogen
The pH of chicken meat samples at chiller and room temperatures are given in Fig. 3(c and d) which shows that the pH value varied from 5.5 for fresh meat (day 1) to 6.4 for the spoilage threshold (day 3 at room temperature). On the other hand, the pH of chicken meat at chiller temperature varied from pH 5.5 in fresh stage (day 1) to 6.5 in spoilage stage (day 8). It was obvious that there are changes in the dual sensor colours in response to pH changes in the package environment. So, the colour change of both BCP and BTB is related to the pH of the chicken meat indicating that spoilage occurs more readily at pH > 6.0 than at the normal pH of fresh meat (<5.8).46 From the above results, we can conclude that spoilage reactions cause complete rotting of meat after 3 days at room temperature and after 8 days at chiller temperature. Moreover, the sensor showed accurate responses with the spoilage onset at both temperatures.
Generally speaking, spoilage of meat products results in the formation of ammonia (NH3) and other volatile amines which lead to the elevation of TVBN levels. Thus, TVBN, the by-products of protein breakdown by the action of microbial proteolytic enzymes, is an extremely important parameter in determining chicken-meat quality.47 In the presence of moisture, TVBN of spoiled chicken meat can react with the acidic form of the freshness indicator dye leading to a change in the indicator's colour.24 The level of TVBN in chicken breast at the spoilage stage is 20–25 mg%.47 Fig. 3(e and f) shows the results of measuring TVBN levels for the monitored chicken-meat samples where the TVBN levels increased with the increase of the storage period from 8.6 mg% in the fresh state to 24.2 mg% in the spoilage state after 3 days at room temperature and to 23.2 mg% in spoilage stage after 8 days of chilling conditions. It was shown that the change in sensor colour follows the TVBN values during the storage period.
Selection of RGB values and calibration plots construction
Based on the mechanism of colour absorption/reflection reported in ref. 32, the calculated absorbance values vary significantly with using each of the three RGB colours. Therefore, the colour which gives the highest sensitivity as well as the best linearity of the calibration plot should be investigated. Here, the standard plots obtained by plotting the calculated absorbance values (using the R-, G- or B-values) against the APC of the chicken fillet samples are introduced (Fig. 4). For both BCP and BTB, it is obvious that both the R- and G-values give acceptable linearity in the calibration plots. However, in case of BTB, the red colour gives higher absorbance values and in turn the sensitivity will be better. The obtained linearity ranges were found to be 11.2 × 103 to 1.12 × 106 and 38.0 × 103 to 1.12 × 106 CFU g−1 for BTB and BCP, respectively. The calibration plots showed correlation coefficients (r2) of 0.9998 (slope: 2.48 g CFU−1) and 0.9999 (slope: 1.95 g CFU−1) in case of BTB and BCP, respectively. In addition, both BTB and BCP demonstrated a quantification limit (LOQ) of 38.41 × 103 CFU g−1 and a detection limit (LOD) of 11.64 × 103 CFU g−1. However, calculating the absorbance values using the green colour provides the best sensitivity in case of BCP. Due to the very low values of absorbance calculated with the blue colour, the B-values were not selected for constructing the calibration plots as they resulted in non-linear relationships. Consequently, the Android application was designed to measure the R-values in case of BTB and the G-values in case of BCP, and then use these values to calculate the light absorbance and construct the calibration plots.
Sensory freshness evaluation
Sensory evaluation (especially odour and texture) of chicken meat freshness was carried out as described in the Experimental section. During storage, any off-flavour, colour variation and off-odour makes the meat product undesirable and forces the consumers to reject it. Sensory evaluation was recorded with the colour change in the as-prepared dual LOP sensor. Odour scores of chicken meat are given in Table 1 which shows a similar response to that of the dual LOP sensor. Upon gradual spoilage, the freshness of the chicken meat samples decreased obviously and changes in texture were observed by the panelists with concurrent gradual changes of the dual sensor colours from yellow to blue (BTB) and from yellow to purple (BCP). Each attribute was scored on a continuous 3 to 0 hedonic scale with 3 being the highest quality score (fresh), 2 given to the acceptable product, 1 is the limit of acceptance or rejection point and 0 for the unacceptable sample (completely spoiled).43
Table 1 Changes in sensory evaluation parameters of chicken meat samples and the response of dual LOP sensor colours at chiller and room temperatures. Standard deviations are added after the (±) signs
Storage time (day) |
Texture |
Odour |
Colour |
Sensor colour |
Chiller temperature |
0 |
3.0 ± 0.021 |
3.0 ± 0.230 |
3.0 ± 0.034 |
 |
1 |
3.0 ± 0.020 |
3.0 ± 0.230 |
3.0 ± 0.023 |
 |
2 |
2.8 ± 0.013 |
2.6 ± 0.130 |
2.8 ± 0.012 |
 |
3 |
2.7 ± 0.012 |
2.6 ± 0.043 |
2.6 ± 0.002 |
 |
4 |
2.2 ± 0.030 |
2.4 ± 0.023 |
2.0 ± 0.340 |
 |
5 |
1.9 ± 0.014 |
1.8 ± 0.420 |
1.9 ± 0.123 |
 |
6 |
1.4 ± 0.123 |
1.3 ± 0.230 |
1.3 ± 0.320 |
 |
7 |
0.7 ± 0.543 |
0.4 ± 0.230 |
0.5 ± 0.322 |
 |
8 |
0.0 ± 0.000 |
0.0 ± 0.000 |
0.0 ± 0.000 |
 |
![[thin space (1/6-em)]](https://www.rsc.org/images/entities/char_2009.gif) |
Room temperature |
0 |
3.0 ± 0.020 |
3.0 ± 0.230 |
3.0 ± 0.023 |
 |
1 |
2.0 ± 0.133 |
2.4 ± 0.032 |
2.3 ± 0.321 |
 |
2 |
1.0 ± 0.124 |
1.3 ± 0.032 |
0.9 ± 0.022 |
 |
3 |
0.0 ± 0.000 |
0.0 ± 0.000 |
0.0 ± 0.000 |
 |
Generally, colour changes of the freshness indicator can be recognized by the naked eye but human inspection is subjective and variable. This makes the final judgment regarding meat products freshness difficult and inaccurate; therefore, it is highly recommended to use colour-measuring instruments.48 Recently, smart phones became ubiquitous as colour-measuring devices both in purely didactic works and in real applications.49
The suggested LOP sensor is economic and sensitive with rapid response to small changes in the sample pH.
It can predict the freshness conditions of chicken meats and also track hourly/daily changes under different storing conditions. The sensor can be easily applied to other types of meat products and for estimating the shelf life of the test product even with naked eyes using a standard colour panel. However, the advantage of the proposed sensing method over those reported in the literature is the integration of the Android application which provides fast primary indication of APC in food samples.
It is worth to mention that the indicator sensor proposed herein does not perform a direct measurement of bacterial growth, otherwise it relates bacterial activity (detected by the sensor colour change) to it. Therefore, due to possible differences in sample preparation/processing and the type of bacteria and their activity, RGB values do not absolutely mean CFU. So that, the current dual sensor is mainly designed for simple use by the end users, not for accurate bacterial CFU analyses. In other words, the sensor can provide a primary indication about the amount of bacteria present in a certain meat sample (see Fig. 2).
Conclusions
The present work demonstrates the preparation of a LOP colorimetric sensor for progressive-spoilage monitoring in stored chicken meat products. An Android application was developed in order to help in detecting the degree of chicken-meat spoilage within few seconds. The developed dual sensor relies on the use of the indicator dyes, BTB and BCP, as they demonstrated gradual colour changes during the spoilage process at room and chiller temperatures. Other spoilage parameters such as the levels of TVBN as well as the sample pH were measured in order to assess the proposed sensor performance. In addition, sensory evaluation of the investigated samples was carried out by a group of 9 panelists. The as-prepared sensor was successfully applied to freshness evaluation of chicken meat products over a period of 8 days (for refrigerated samples) and 3 days (for room temperature samples). The developed Android application has the ability to plot a standard curve using the R- (for BTB) and G- (for BCP) values from which it can expect the degree of freshness based on microbial counts of the standard samples measured by the APC method. The proposed sensors have many advantages including the ease of use via the first-to-develop Android application, easy and economic preparation since it is prepared from simple filter papers and common pH indicator dyes, and the high contrast between the sensor colours in different conditions which allows easy detection by the human eyes. In addition, the proposed sensor is the first of its kind to be suitable for quantitative analysis of the spoilage-causing microbes, as all the previously reported sensors depend only on the qualitative detection of spoilage in meat samples without being able to quantify the amount of bacteria and relate it to the international standards.
Author contributions
Ahmed S. Abo Dena: conceptualization, methodology, investigation, data curation, visualization, writing – review and editing, resources. Shaimaa A. Khalid: methodology, investigation, writing – original draft. Ahmed F. Ghanem: preparation of the meat packaging polymeric films. Ahmed Ibrahim Shehata: software. Ibrahim M. El-Sherbiny: conceptualization, writing – review and editing, resources, supervision.
Conflicts of interest
There are no conflicts to declare.
Notes and references
- G. Sahoo, N. S. S. Mulla, Z. A. Ansari and C. Mohandass, Indian J. Pharm. Sci., 2012, 74, 348 CrossRef CAS PubMed.
- G. Monti, L. De Napoli, P. Mainolfi, R. Barone, M. Guida, G. Marino and A. Amoresano, Anal. Chem., 2005, 77, 2587–2594 CrossRef CAS PubMed.
- H. Tao, M. A. Brenckle, M. Yang, J. Zhang, M. Liu, S. M. Siebert, R. D. Averitt, M. S. Mannoor, M. C. McAlpine and J. A. Rogers, Adv. Mater., 2012, 24, 1067–1072 CrossRef CAS PubMed.
- R. Karoui, G. Downey and C. Blecker, Chem. Rev., 2010, 110, 6144–6168 CrossRef CAS PubMed.
- A. Loutfi, S. Coradeschi, G. K. Mani, P. Shankar and J. B. B. Rayappan, J. Food Eng., 2015, 144, 103–111 CrossRef CAS.
- Y. Zhang, Y. Mao, K. Li, P. Dong, R. Liang and X. Luo, Asian-Australas. J. Anim. Sci., 2011, 24, 713–722 CrossRef.
- W. Henao-Escobar, O. Domínguez-Renedo, M. A. Alonso-Lomillo and M. J. Arcos-Martínez, Talanta, 2013, 117, 405–411 CrossRef CAS PubMed.
- M. A. Alonso-Lomillo, O. Domínguez-Renedo, P. Matos and M. J. Arcos-Martínez, Anal. Chim. Acta, 2010, 665, 26–31 CrossRef CAS PubMed.
- H.-N. Chun, B. Kim and H.-S. Shin, Food Sci. Biotechnol., 2014, 23, 1719–1725 CrossRef CAS.
- A. S. Hernández-Cázares, M.-C. Aristoy and F. Toldrá, Food Chem., 2010, 123, 949–954 CrossRef.
- B. Bóka, N. Adányi, D. Virág, M. Sebela and A. Kiss, Electroanalysis, 2012, 24, 181–186 CrossRef.
- V. Y. Musatov, V. V. Sysoev, M. Sommer and I. Kiselev, Sens. Actuators, B, 2010, 144, 99–103 CrossRef CAS.
- X. Hong, J. Wang and Z. Hai, Sens. Actuators, B, 2012, 161, 381–389 CrossRef CAS.
- O. S. Papadopoulou, E. Z. Panagou, F. R. Mohareb and G.-J. E. Nychas, Food Res. Int., 2013, 50, 241–249 CrossRef.
- Y. Salinas, J. V. Ros-Lis, J.-L. Vivancos, R. Martínez-Máñez, D. M. Marcos, S. Aucejo, N. Herranz, I. Lorente and E. Garcia, Food Control, 2014, 35, 166–176 CrossRef.
- Y. Salinas, J. V. Ros-Lis, J.-L. Vivancos, R. Martínez-Máñez, S. Aucejo, N. Herranz, I. Lorente and E. Garcia, Sens. Actuators, B, 2014, 190, 326–333 CrossRef CAS.
- Q. Chen, Z. Hui, J. Zhao and Q. Ouyang, LWT--Food Sci. Technol., 2014, 57, 502–507 CrossRef CAS.
- S.-Y. Kim, J. Li, N.-R. Lim, B.-S. Kang and H.-J. Park, Food Chem., 2016, 211, 440–447 CrossRef CAS PubMed.
- S. H. Lim, J. W. Kemling, L. Feng and K. S. Suslick, Analyst, 2009, 134, 2453–2457 RSC.
- M. C. Janzen, J. B. Ponder, D. P. Bailey, C. K. Ingison and K. S. Suslick, Anal. Chem., 2006, 78, 3591–3600 CrossRef CAS PubMed.
- H. N. Kim, W. X. Ren, J. S. Kim and J. Yoon, Chem. Soc. Rev., 2012, 41, 3210–3244 RSC.
- A. Pacquit, J. Frisby, D. Diamond, K. T. Lau, A. Farrell, B. Quilty and D. Diamond, Food Chem., 2007, 102, 466–470 CrossRef CAS.
- A. Nopwinyuwong, S. Trevanich and P. Suppakul, Talanta, 2010, 81, 1126–1132 CrossRef CAS PubMed.
- C. Rukchon, A. Nopwinyuwong, S. Trevanich, T. Jinkarn and P. Suppakul, Talanta, 2014, 130, 547–554 CrossRef CAS PubMed.
- B. Kuswandi, F. Damayanti, J. Jayus, A. Abdullah and L. Y. Heng, J. Math. Fundam. Sci., 2015, 47, 236–251 CrossRef CAS.
- Z. Li and K. S. Suslick, ACS Sens., 2016, 1, 1330–1335 CrossRef CAS.
- K. Lee, H. Park, S. Baek, S. Han, D. Kim, S. Chung, J.-Y. Yoon and J. Seo, Food Packag. Shelf Life, 2019, 22, 100408 CrossRef.
- M. Taghizadeh-Behbahani, B. Hemmateenejad and M. Shamsipur, Chem. Pap., 2018, 72, 1239–1247 CrossRef CAS.
- L. R. Magnaghi, F. Capone, C. Zanoni, G. Alberti, P. Quadrelli and R. Biesuz, Foods, 2020, 9(5), 684 CrossRef CAS PubMed.
- P. Kaewklin, U. Siripatrawan, A. Suwanagul and Y. S. Lee, Int. J. Biol. Macromol., 2018, 112, 523–529 CrossRef CAS PubMed.
- Y. W. Park, S. M. Kim, J. Y. Lee and W. Jang, Mol. Cell. Toxicol., 2015, 11, 277–285 CrossRef CAS.
- A. S. Abo Dena and E. E. Bayoumi, J. Radioanal. Nucl. Chem., 2018, 318, 1439–1445 CrossRef CAS.
- A. Ozcan, Lab Chip, 2014, 14, 3187–3194 RSC.
- Y. Chen, G. Fu, Y. Zilberman, W. Ruan, S. K. Ameri, Y. S. Zhang, E. Miller and S. R. Sonkusale, Food Control, 2017, 82, 227–232 CrossRef CAS.
- H. Zhu, I. Sencan, J. Wong, S. Dimitrov, D. Tseng, K. Nagashima and A. Ozcan, Lab Chip, 2013, 13, 1282–1288 RSC.
- L. Shen, J. A. Hagen and I. Papautsky, Lab Chip, 2012, 12, 4240–4243 RSC.
- V. Oncescu, D. O'Dell and D. Erickson, Lab Chip, 2013, 13, 3232–3238 RSC.
- L. Guan, J. Tian, R. Cao, M. Li, Z. Cai and W. Shen, Anal. Chem., 2014, 86, 11362–11367 CrossRef CAS PubMed.
- H. Yu, Y. Tan and B. T. Cunningham, Anal. Chem., 2014, 86, 8805–8813 CrossRef CAS PubMed.
- X. Bao, S. Jiang, Y. Wang, M. Yu and J. Han, Analyst, 2018, 143, 1387–1395 RSC.
- R. G. Brannan, Meat Sci., 2009, 81, 589–595 CrossRef CAS PubMed.
- B. F. Cobb, III, I. Alaniz and C. A. Thompson, Jr, J. Food Sci., 1973, 38, 431–436 CrossRef.
- P. S. Taoukis, K. Koutsoumanis and G. J. E. Nychas, Int. J. Food Microbiol., 1999, 53, 21–31 CrossRef CAS PubMed.
- A. S. Abo Dena and W. M. I. Hassan, Spectrochim. Acta, Part A, 2016, 163, 108–114 CrossRef CAS PubMed.
- O. E. Sherif, Y. M. Issa and A. S. Abo Dena, Int. J. Res. Pharm. Chem., 2014, 4, 181–191 Search PubMed.
- R. H. Dainty, Int. J. Food Microbiol., 1996, 33, 19–33 CrossRef CAS PubMed.
- D. Kim, S. Lee, K. Lee, S. Baek and J. Seo, Food Sci. Biotechnol., 2017, 26, 37–42 CrossRef CAS PubMed.
- K. Leon, D. Mery, F. Pedreschi and J. Leon, Food Res. Int., 2006, 39, 1084–1091 CrossRef.
- G. Witjaksono, N. H. F. B. M. Hussin, A. A. S. Rabih and S. Alfa, IOP Conference Series: Materials Science and Engineering, IOP Publishing, 2017, vol. 237, p. 12024 Search PubMed.
|
This journal is © The Royal Society of Chemistry 2021 |