DOI:
10.1039/D1RA05630A
(Paper)
RSC Adv., 2021,
11, 34462-34478
Pharmacophore modeling, docking and the integrated use of a ligand- and structure-based virtual screening approach for novel DNA gyrase inhibitors: synthetic and biological evaluation studies†
Received
23rd July 2021
, Accepted 28th September 2021
First published on 25th October 2021
Abstract
Fluoroquinolones, a class of compound, act via inhibiting DNA gyrase and topoisomerase IV enzymes. This is an important class of drugs with high success rates for the treatment of tuberculosis and other bacterial infections. An indirect drug design approach was used to develop a meaningful pharmacophore model using the HypoGen module of Discovery Studio 2.0 on a set of 27 structurally diverse compounds with a wide range of biological activity (5 log units). The best hypothesis had three hydrogen bond acceptors (HBA) and one hydrophobic (Hy) moiety, showing r = 0.95, and it predicts the test set of 44 compounds well, with r2 = 0.823. The same features (acceptor and hydrophobic functionality) were validated at the binding site of the DNA gyrase active site using GOLD version 3.0.1 and Molegro Virtual Docker, which showed corresponding hydrogen bond interactions and also π–π stacking interactions that correlated well with the PIC50 values (r2 = 0.6142). The thoroughly validated model was used to screen an extensive database of 0.25 million compounds to identify potential leads. The validated model was implemented for the identification, design, synthesis, and biological evaluation of leads. Ten new chemical entities were synthesized based on our scaffold hopping techniques from the identified virtual screening and tested against the tuberculosis bacterium to obtain preliminary MIC values. The results showed that 3 out of 10 synthesized compounds exhibited good MICs, from 1.25 to 50 μM. This proves the robustness and applicability of the developed model, which is a promising tool for identifying new topoisomerase II inhibitors for the treatment of tuberculosis.
Introduction
Tuberculosis is one of the leading causes of mortality globally, killing at least two million people every year.1 Every third person gives a positive result for the purified protein derivative (PPD) test, indicating that one-third of the world population harbors the bacillus in its latent form.2,3 In recent years, co-infection with HIV due to immuno-suppression has worsened the situation,4 with the number of infected cases increasing to more than nine million and the death toll touching two million.5 The emergence of multi-drug-resistant (MDR) and extremely-drug-resistant (XDR) strains has proved to be highly fatal to the treatment process and has led to a dearth of drugs to combat these strains.6
Fluoroquinolones (FQ), synthetic derivatives of nalidixic acid, were developed in the 1980s to combat bacterial infection and have become an indispensable part of the current antitubercular treatment regimen.7,8 They are known to exhibit broad-spectrum activity against mycobacteria and can be used to treat drug-resistant strains. They primarily target DNA gyrase,9,10 which is the only enzyme that relieves the super-helical strain in DNA ahead of the replication fork.9 Topoisomerase IV, mainly a decatenating agent during replication, is a secondary target for all quinolones.11 DNA gyrase and topoisomerase IV consist of two subunits, namely gyrA and gyrB for the former and parC and pare for the latter. Fluoroquinolones bind to bacterial DNA via π–π stacking of the planar quinolone rings with the nucleic acid residues of the DNA.12 This ternary enzyme–DNA complex prevents DNA replication, leading to cell death. Fluoroquinolones strengthen the covalent interaction between the enzyme tyrosyl and the phosphate ester of DNA.
In the past few decades, drug discovery research has initiated the design of drugs with fewer side effects using direct and indirect drug design techniques. These techniques play an important role in discovering new chemical entities (NCEs) as potential leads. The receptor-based (direct) approach to CADD (computer-aided drug design) is used when a reliable model of the receptor (preferentially complexed with a ligand) is available from X-ray diffraction, NMR, or homology modeling.13,14 This approach involves binding and interactions between the ligand and the receptor in the biological system. It involves molecular docking, which is accomplished by using Molegro virtual docker, Autodock, GOLD, and Schrodinger.15 The indirect drug design approach is based on predictive pharmacophore models with different structural features necessary for receptor binding.16–18 This may be achieved by using software such as Catalyst (HypoGen and HipHop), SYBYL/comparative molecular field analysis (CoMFA),19 and comparative molecular similarity indices analysis (CoMSIA).20
Pharmacophore modeling utilizes a diverse set of compounds known to act by the same mechanism of action. The HypoGen module in Catalyst generates quantitative meaningful pharmacophore-based data from a structurally diverse set of compounds spanning over 3–4 orders of activity. On the other hand, in HipHop module, pharmacophore generation is based on a small set of known active compounds. In most cases, pharmacophore modeling provides basic features in terms of hydrogen bond donors (HBD), hydrogen bond acceptors (HBA), hydrophobic groups (HY), aromatic rings (AR), positively charged/ionizable groups (PG) and negatively charged/ionizable groups (NG). Additionally, shape and excluded volume functions also assist in the binding site organization. Pharmacophore-based validated models can show good percentage success rates in retrieving diverse leads from a large database.18,21–23
In recent years, several DNA gyrase inhibitors have been examined as inhibitors of Mycobacterium tuberculosis as well as other mycobacterial infections.24,25 FQs are active against Mycobacterium tuberculosis and were the first new antitubercular agents after rifampicin, and are recommended for rifampicin-resistant mycobacterial infection.26,27 Therefore the ofloxacin moxifloxacin was significantly studied and included as a first-line treatment and ciprofloxacin as a second-line treatment.28,29 WHO experts also include quinolones as part of a third-line treatment containing four drugs (an aminoglycoside, ethionamide, pyrazinamide, and ofloxacin) during the initial phase and two drugs (ethionamide and ofloxacin) during the continuation phase.30
Up to the present time, few fluoroquinolones were under optimization against M. tuberculosis.31 Some attempts had been made based on structure–activity relationships of quinolones at the C7 position as lipophilic substitution at this position was favored to increase the activity of these motifs.9,32 A QSAR approach was also applied by Klopman et al. to develop a predictive model for the characterization of anti-M. avium–M. intracellulare complex activity.33,34 Gozalbes et al. also reported the molecular topology-based prediction of activity and its applications in terms of virtual screening for Mycobacterium avium and M. intracellulare.35 Therefore, it appears interesting to develop a quantitative pharmacophore model for DNA gyrase inhibitors of Mycobacterium tuberculosis validated by a test set and an external dataset of clinically available compounds and binding mode studies. The pharmacophore model can quantitatively explain the requirements of important features for quinolones. The model can again be verified by using the binding interaction in terms of docking and finally can be used as a query for 3D database screening. The pharmacophore was developed by using 71 structurally diverse compounds that are assayed by a similar protocol. The selection criteria were based on diversity in the structures and activity profiles (3–4 orders). To the best of our knowledge, this is the first pharmacophore model for quinolones using quantitative means that has been validated by an external dataset and predicted well the docking and binding interactions at the active site of the receptor.
Crystal studies of the active site (PDB: 3ILW, 3FOF) co-crystallized with moxifloxacin (3FOF) and the N-terminal domain of DNA gyrase subunit (3ILW) have revealed a wealth of information regarding the binding of the quinolones to the active site of DNA gyrase and also as to their mechanism of resistance.36,37 A pharmacophore model was developed using novel reported quinolones with potent antitubercular activity against DNA gyrase using Discovery Studio version 2.0 and the three-dimensional structure–activity relationship was investigated. Their binding modes were studied by docking them into the active site and by studying their interactions.
Results and Discussion
Selection of the dataset
A diverse set of 332 compounds from the literature that would appropriately represent various substitutions on the quinolone nucleus were considered. Out of these, only 71 molecules were chosen for the model building because these compounds, even with the diverse structures, had a common assay method (BACTEC screening assay), thus removing the discrepancy between the biological activities arising from analysis using different assay methods.
Training set: selection and model building
A set of 27 structurally diverse quinolones, spanning 5 log activities, were chosen to form the training set. Their structures and biological activity38–41 are shown in Fig. 1. Their activities in MIC were converted from μg ml−1 to nM to normalize the dataset.
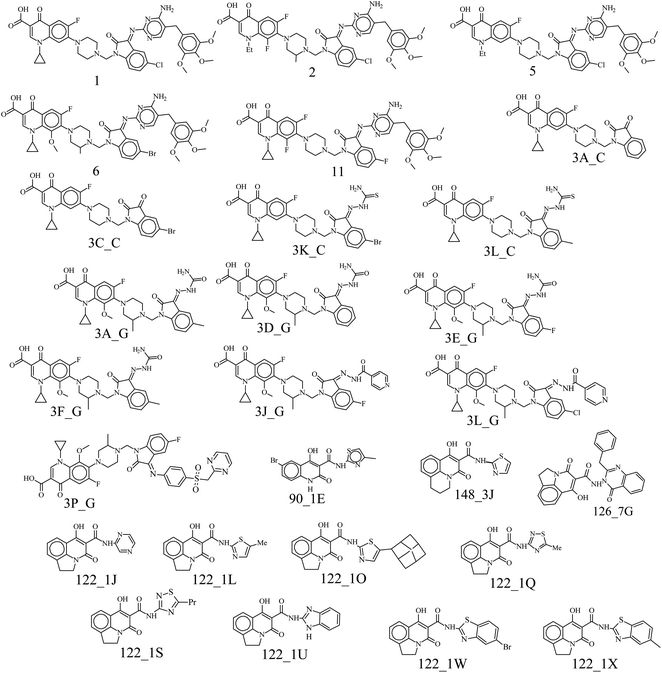 |
| Fig. 1 Training set compounds. | |
Cost analysis
A major assumption that catalyst uses in the generation of hypotheses is Occam's razor, which states that the simplest model is the best.24 The first among the ten hypotheses is the best hypothesis based on statistical parameters and correlations. The various cost values relating to the pharmacophore hypotheses are listed in Table 1. The difference between the null cost (170.98) and the total cost (121.54) was 49.44, indicative of a 75–90% probability of a true representation of the data. The larger the difference between the null and total costs, the greater the measure of the statistical soundness. These theoretical cost values are measured in units of bits. The null cost is the cost of generating a hypothesis when the error cost is high, the fixed cost is where the error is the least, and the total cost is the actual cost of the hypothesis. It was observed that as we go down the table, the difference between cost values falls from 49.44 to 35.38. As we go towards more minor differences between null and total costs, to values below 40, the probability of a hypothesis being true falls rapidly to less than 50% reliable. Comparing the rest of the cost parameters of the hypotheses: error cost (EC), correlation coefficient (r) between actual and estimated pMIC, root mean square deviation (RMSD) < 1, it is obvious that hypothesis 1 is the best amongst them. The configuration cost was 16.2 for all the hypotheses (less than 17), corresponding to 216.2 hypotheses. A value >17 indicates that correlation for any generated pharmacophore is most likely due to chance correlation since catalyst cannot consider more than 217 hypotheses in the optimization phase, and so the rest are left out. The configuration cost can be reduced by limiting the maximum and minimum features given as inputs for the generation of the hypotheses. It also has a low RMSD of 0.80, resulting in a lower value of the error cost (99.38).
No. |
TC |
NC − TC |
EC |
WC |
r |
RMSD |
Config. |
Ex. V. |
F |
No: hypothesis number in ascending order of total cost; TC: total cost; NC − TC: null cost − total cost; EC: error cost; WC: weight cost; r: correlation coefficient; RMSD: root mean square deviation; Config.: configuration cost; Ex. V: number of excluded volumes; H: hydrophobic feature; A: H-bond acceptor feature; D: H-bond donor feature; NI: negatively ionizable group; PI: positively ionizable group. |
1 |
121.54 |
49.44 |
99.38 |
5.96 |
0.95 |
0.80 |
16.2 |
3 |
3A, 1H |
2 |
129.45 |
41.53 |
109.77 |
3.48 |
0.88 |
1.18 |
16.2 |
2 |
2A, 1NI |
3 |
130.94 |
40.04 |
113.00 |
1.74 |
0.85 |
1.28 |
16.2 |
3 |
3A, 1H |
4 |
131.23 |
39.75 |
111.30 |
3.73 |
0.87 |
1.23 |
16.2 |
3 |
2A, 1NI |
5 |
132.52 |
38.46 |
114.76 |
1.55 |
0.84 |
1.33 |
16.2 |
2 |
1A, 1D, 2H, 1NI |
6 |
132.65 |
38.33 |
111.19 |
5.25 |
0.87 |
1.30 |
16.2 |
2 |
3A |
7 |
133.96 |
37.02 |
116.53 |
1.22 |
0.82 |
1.38 |
16.2 |
1 |
2A, 2H |
8 |
134.53 |
36.45 |
116.22 |
2.11 |
0.83 |
1.37 |
16.2 |
3 |
3A |
9 |
134.91 |
36.07 |
117.57 |
1.14 |
0.82 |
1.40 |
16.2 |
2 |
2A, 2H |
10 |
135.90 |
35.38 |
118.13 |
1.27 |
0.81 |
1.42 |
16.2 |
3 |
2A, 2H, 1NI |
Mapping of features onto the pharmacophore
All the models have at least one acceptor, one hydrophobic feature and one excluded volume. An examination of the mapping of the molecules onto the best pharmacophore 1 reveals several important features essential for activity. The two oxo groups at positions 1 and 3 of the quinolone nucleus are an important feature for activity, mapping two acceptor features in the pharmacophore. Compounds in the dataset were chosen to study the variation in substitution patterns on all positions of the quinolone nucleus. Ciprofloxacin derivatives18,19 explored the substitution effect on the I and VII positions, while the rest14–17 explored possibilities in the I, II, III, V, VI, VII positions. Compounds of the series 3X_Y (where X = A–Q), Y = C, G) have the characteristic piperidine group that acts as a linker and positions the isatinyl moiety to map onto the second acceptor feature shown in Fig. 2. All the other compounds in the dataset miss this feature, i.e., A_2. Hydrophobic feature (H_4) is another essential moiety for all the compounds (Fig. 2); the examination of the mapping of the training (Table 2) and test set (Table 3) compounds reveals that this (as indicated using the cyan sphere) is present in all molecules. The carboxylic acid moiety, which is prevalent in the 3X_Y series and in the Z series (Z = 1–12), may mislead us into thinking that it is essential for activity. Still, the essential feature required for activity is the 1,3-oxo group, which is present in all the compounds, in the III and IV positions in some (series 3X_Y), or in the II and III positions in some others. It is very clear that the hydrophobic moiety in the N-1 position is important for activity. Some compounds in the dataset are massive, with molecular weights greater than 800 amu. This pharmacophore may not correctly estimate these compounds due to their large size or may show false-positive results due to high log
P values. But they add to the diversity of the dataset and provide essential information, thus are included in the dataset. The three excluded volumes play an important part in predicting the activity of too big molecules, for example, the Z series. Besides, it is observed that the presence of a hydrogen bond acceptor group in the isatinyl group, when connected to the quinolone nucleus by a linker, is essential for activity. The model proposed by this pharmacophore imposes qualitative upper and lower limits on the size of the molecules. Too small a size will not be able to bind into the pocket effectively, while too huge molecules have steric hindrance, which reduces their activity.
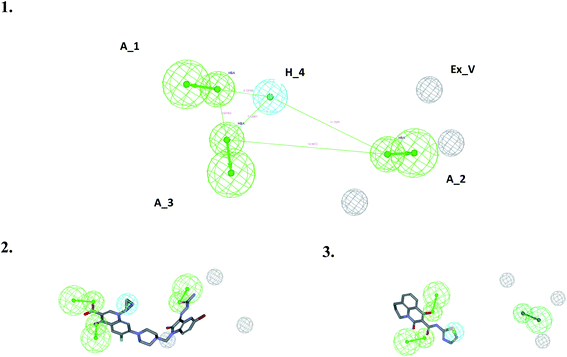 |
| Fig. 2 The best pharmacophore and the mapping of the most and least active compounds. (1) The best pharmacophore generated using HypoGen with three acceptors (green spheres) and one hydrophobic group (cyan spheres) (including three excluded volumes (grey spheres)). (2) Mapping of the most active compound 3K_C. (3) Mapping of the least active compound 148_3J. | |
Table 2 Actual and estimated activities of the training seta
Comp. |
Actual MIC |
Est. MIC |
Error factor |
Fit value |
A_1 |
A_2 |
A_3 |
H_4 |
Actual scale |
Est. scale |
Actual MIC: reported MIC (nM); Est. MIC: estimated MIC; error factor: Est. MIC/actual MIC or its inverse, whichever is higher; fit value: how well the features in the pharmacophore overlap the chemical features in the molecule; A_1, A_2, and A_3: acceptor features 1, 2, and 3; H_4: hydrophobic feature; actual scale and estimated scale: all compounds having an activity <10 nM were assigned ‘+++++’, those with activity between 10 and 100 nM were assigned ‘++++’, those with activity between 100 and 1000 nM were assigned ‘+++’, those with activity between 1000 and 10 000 nM were assigned ‘++’, and those >10 000 nM were assigned ‘+’. |
1 |
3926 |
2042.6 |
1.92 |
8.49 |
1 |
0 |
1 |
1 |
++ |
++ |
2 |
1908.82 |
2447.82 |
1.28 |
8.41 |
1 |
1 |
1 |
1 |
++ |
++ |
5 |
3632.31 |
1456.85 |
2.49 |
8.64 |
1 |
1 |
1 |
1 |
++ |
++ |
6 |
3522.73 |
1206.82 |
2.92 |
8.72 |
1 |
1 |
1 |
1 |
++ |
++ |
11 |
998.98 |
386.41 |
2.59 |
9.21 |
1 |
1 |
1 |
1 |
+++ |
+++ |
122_1J |
5319.15 |
5793.63 |
1.09 |
8.04 |
1 |
0 |
1 |
1 |
++ |
++ |
122_1L |
10020.81 |
3299.15 |
3.04 |
8.28 |
1 |
0 |
1 |
1 |
+ |
++ |
122_1O |
14449.2 |
4893.62 |
2.95 |
8.11 |
1 |
0 |
1 |
1 |
+ |
++ |
122_1Q |
4915.4 |
4575.62 |
1.07 |
8.14 |
1 |
0 |
1 |
1 |
++ |
++ |
122_1S |
2258.12 |
3618.62 |
1.6 |
8.24 |
1 |
0 |
1 |
1 |
++ |
++ |
122_1U |
1177.07 |
4694.02 |
3.99 |
8.13 |
1 |
0 |
1 |
1 |
++ |
++ |
122_1W |
7325.41 |
6244.26 |
1.17 |
8 |
1 |
0 |
1 |
1 |
++ |
++ |
122_1X |
17245.66 |
5339.09 |
3.23 |
8.07 |
1 |
0 |
1 |
1 |
+ |
++ |
126_7G |
2339.88 |
4272.61 |
1.83 |
8.17 |
1 |
0 |
1 |
1 |
++ |
++ |
148_3D |
2929.47 |
4464.89 |
1.52 |
8.15 |
1 |
0 |
1 |
1 |
++ |
++ |
148_3J |
22954.31 |
10995.1 |
2.09 |
7.76 |
1 |
0 |
1 |
1 |
+ |
+ |
3A_C |
1.59 |
5.72 |
3.6 |
11.04 |
1 |
1 |
1 |
1 |
+++++ |
+++++ |
3A_G |
328.09 |
207.97 |
1.58 |
9.48 |
1 |
1 |
1 |
1 |
+++ |
+++ |
3C_C |
2.74 |
8.13 |
2.97 |
10.89 |
1 |
1 |
1 |
1 |
+++++ |
+++++ |
3D_G |
21.13 |
13.24 |
1.6 |
10.68 |
1 |
1 |
1 |
1 |
++++ |
++++ |
3E_G |
159.83 |
148.91 |
1.07 |
9.63 |
1 |
1 |
1 |
1 |
+++ |
+++ |
3F_G |
1254.83 |
193.49 |
6.49 |
9.51 |
1 |
1 |
1 |
1 |
++ |
+++ |
3J_G |
1161.28 |
1373.24 |
1.18 |
8.66 |
1 |
1 |
1 |
1 |
++ |
++ |
3K_C |
1.21 |
6.21 |
5.13 |
11.01 |
1 |
1 |
1 |
1 |
+++++ |
+++++ |
3L_G |
290.65 |
746.28 |
2.57 |
8.93 |
1 |
1 |
1 |
1 |
+++ |
+++ |
3P_G |
130.41 |
219.4 |
1.68 |
9.46 |
1 |
1 |
1 |
1 |
+++ |
+++ |
90_1E |
1025.72 |
2847.61 |
2.78 |
8.35 |
1 |
0 |
1 |
1 |
++ |
++ |
Table 3 Actual and estimated activities of the test seta
Compd |
Actual MIC |
Est. MIC |
Error factor |
Fit value |
A_1 |
A_2 |
A_3 |
A_4 |
Actual scale |
Est. scale |
For details of the abbreviations, refer to Table 2. |
3 |
2096.83 |
3720.43 |
1.77 |
8.48 |
1 |
0 |
1 |
1 |
++ |
++ |
4 |
2099.31 |
3718.66 |
1.77 |
8.48 |
1 |
0 |
1 |
1 |
++ |
++ |
7 |
2068.72 |
4029.20 |
1.95 |
8.48 |
1 |
0 |
1 |
1 |
++ |
++ |
8 |
698.83 |
3928.02 |
5.62 |
8.96 |
1 |
1 |
1 |
1 |
++ |
+++ |
9 |
2143.90 |
3812.93 |
1.78 |
8.47 |
1 |
1 |
1 |
1 |
++ |
++ |
10 |
2076.79 |
4071.39 |
1.96 |
8.48 |
1 |
0 |
1 |
1 |
++ |
++ |
12 |
791.48 |
3938.00 |
4.98 |
8.9 |
1 |
1 |
1 |
1 |
++ |
+++ |
122_1A |
5868.65 |
10 491.39 |
1.79 |
8.03 |
1 |
0 |
1 |
1 |
+ |
++ |
122_1B |
2960.50 |
20 949.25 |
7.08 |
8.33 |
1 |
0 |
1 |
1 |
+ |
++ |
122_1M |
2702.05 |
4994.40 |
1.85 |
8.37 |
1 |
0 |
1 |
1 |
++ |
++ |
122_1N |
5740.03 |
16 258.68 |
2.83 |
8.04 |
1 |
0 |
1 |
1 |
+ |
++ |
122_1P |
5199.45 |
5142.74 |
1.01 |
8.08 |
1 |
0 |
1 |
1 |
++ |
++ |
122_1R |
1974.17 |
2353.65 |
1.19 |
8.5 |
1 |
0 |
1 |
1 |
++ |
++ |
122_1T |
2262.06 |
2258.12 |
1 |
8.45 |
1 |
0 |
1 |
1 |
++ |
++ |
122_1V |
9998.07 |
2238.93 |
1.57 |
7.8 |
1 |
0 |
1 |
1 |
++ |
++ |
126_6B |
3522.94 |
19 512.35 |
5.54 |
8.25 |
1 |
0 |
1 |
1 |
+ |
++ |
126_6C |
1852.36 |
19 512.35 |
10.53 |
8.53 |
1 |
0 |
1 |
1 |
+ |
++ |
126_7F |
2036.09 |
9167.86 |
4.5 |
8.49 |
1 |
0 |
1 |
1 |
++ |
++ |
148_3C |
4009.12 |
11 755.43 |
2.93 |
8.2 |
1 |
0 |
1 |
1 |
+ |
++ |
148_3E |
4112.10 |
5526.82 |
1.34 |
8.19 |
1 |
0 |
1 |
1 |
++ |
++ |
148_3F |
3464.69 |
11 167.01 |
3.22 |
8.26 |
1 |
0 |
1 |
1 |
+ |
++ |
3B_C |
7.28 |
2.97 |
2.45 |
10.94 |
1 |
1 |
1 |
1 |
+++++ |
+++++ |
3B_G |
10.78 |
643.96 |
59.73 |
10.77 |
1 |
1 |
1 |
1 |
+++ |
+++++ |
3C_G |
425.87 |
622.95 |
1.46 |
9.17 |
1 |
1 |
1 |
1 |
+++ |
+++ |
3D_C |
5.69 |
3.09 |
1.84 |
11.04 |
1 |
1 |
1 |
1 |
+++ |
+++ |
3E_C |
5.05 |
2.85 |
1.77 |
11.1 |
1 |
1 |
1 |
1 |
+++++ |
+++++ |
3F_C |
8.24 |
2.68 |
3.07 |
10.88 |
1 |
1 |
1 |
1 |
+++++ |
+++++ |
3G_C |
9.43 |
1.24 |
7.6 |
10.83 |
1 |
1 |
1 |
1 |
+++++ |
+++++ |
3G_G |
86.27 |
607.37 |
7.04 |
9.86 |
1 |
1 |
1 |
1 |
+++ |
++++ |
3H_C |
4.57 |
1.39 |
3.28 |
11.14 |
1 |
1 |
1 |
1 |
+++++ |
+++++ |
3H_G |
804.92 |
329.13 |
2.45 |
8.89 |
1 |
1 |
1 |
1 |
+++ |
+++ |
3I_C |
14.15 |
1.38 |
10.25 |
10.65 |
1 |
1 |
1 |
1 |
+++++ |
++++ |
3J_C |
12.58 |
2.61 |
4.82 |
10.7 |
1 |
1 |
1 |
1 |
+++++ |
++++ |
3K_G |
2335.66 |
584.09 |
4 |
8.43 |
1 |
0 |
1 |
1 |
+++ |
++ |
3L_C |
11.38 |
10.82 |
1.05 |
10.74 |
1 |
1 |
1 |
1 |
++++ |
++++ |
3M_G |
1555.34 |
152.98 |
10.17 |
8.93 |
1 |
1 |
1 |
1 |
+++ |
++ |
3N_G |
105.96 |
249.61 |
2.36 |
8.61 |
1 |
1 |
1 |
1 |
+++ |
+++ |
3O_G |
422.62 |
254.84 |
1.66 |
9.77 |
1 |
1 |
1 |
1 |
+++ |
+++ |
3Q_G |
283.2 |
256.13 |
1.11 |
9.17 |
1 |
1 |
1 |
1 |
+++ |
+++ |
90_1A |
2338.24 |
1221.35 |
1.91 |
9.35 |
1 |
1 |
1 |
1 |
++ |
++ |
90_1B |
2818.81 |
1156.21 |
2.44 |
8.43 |
1 |
0 |
1 |
1 |
++ |
++ |
90_1C |
2551.84 |
1102.44 |
2.31 |
8.35 |
1 |
0 |
1 |
1 |
++ |
++ |
90_1D |
2551.84 |
2323.02 |
1.1 |
8.39 |
1 |
0 |
1 |
1 |
++ |
++ |
90_1F |
2960.22 |
1825.76 |
1.62 |
8.39 |
1 |
0 |
1 |
1 |
++ |
++ |
Validation of the pharmacophore model
The predictive ability of a pharmacophore model can be only estimated on a definitive basis by using a test set.25 The best hypothesis, shown in Table 1, was validated using a test set of 44 compounds (Table 3). Fig. 3 shows the graph showing the correlation between actual and estimated pMIC values, with r2 = 0.823, showing that the model is highly predictive. The same model was validated against the external set of compounds, which are available commercially for the treatment of TB; the details can be found in ESI Fig. S1.† Validation of the pharmacophore model using structurally diverse and potent DNA gyrase inhibitors was also carried out for the external test set of compounds (ESI S1, Page ESI S3†).
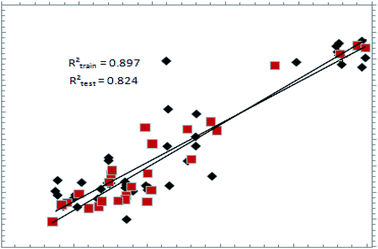 |
| Fig. 3 A graph of actual vs. estimated pMIC values for training (red squares) and test (black diamonds) sets. Rtrain2: regression coefficient for the training set; Rtest2: regression coefficient for the test set. | |
Docking results
Our aim in this endeavor was to study the different binding modes of the molecules under investigation and use this information to verify the best pharmacophore model. All 71 compounds were docked into the quinolone binding pocket of 3ILW and 3FOF and their results analyzed. The docking was carried out using two PDB structures containing the N-terminal domain of the DNA gyrase subunit. 3ILW was the crystal structure of the DNA gyrase reaction core of Mycobacterium tuberculosis while 3FOF contains moxifloxacin co-crystallized with the DNA quinolone cleavage complex (Fig. 4). The docking results are summarised in Table 4 along with the amino acid residues interacting in the active site (QBP) (Table 5).
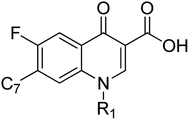 |
| Fig. 4 The core structure of the series used for pharmacophore development. | |
Table 4 Representative docking scores in terms of goldscore, rerank score, and binding affinity
Name |
Moldock score |
Rerank score |
GOLD score |
Gatifloxacin |
−80.1775 |
−66.2217 |
36.34 |
3k_C |
−151.21 |
−68.1317 |
54.69 |
Ciprofloxacin |
−84.6599 |
−68.7114 |
42.67 |
Moxifloxacin |
−90.0372 |
−71.7867 |
33.24 |
Prulifloxacin |
−123.372 |
−96.7243 |
52.63 |
148_3J |
−89.565 |
−43.4861 |
32.31 |
Table 5 Binding interactions of the compounds with important amino acids of PDB ID 3ILW and 3FOF
Comp. |
H-Bond |
Hydrophobic |
π–π stacking interactions with quinolone |
4-CO |
3-COOH |
F6 |
C7 |
N1 |
C7 |
3ILW |
3K_C |
|
Gly47, Asn172, Gly177 |
|
|
Arg98 |
Arg98, Gln277, Ser104, Trp103 |
His52 |
Cipro |
|
Gly47, Asn172, Gly177 |
3CO Lys49 |
|
Arg98 |
|
His52 |
Gati |
|
Asn-172, Asn-176 |
|
|
|
Lys49, Gly179 |
His52 |
Moxi |
|
Gly47, Asn172, Gly177 |
8-OCH3 Arg-98 |
Gly47 |
|
|
His52 |
![[thin space (1/6-em)]](https://www.rsc.org/images/entities/char_2009.gif) |
3FOF |
3K_C |
|
Ser79, Ser80, Asp-78 |
|
|
|
|
Ade1, Ade5, Thy15, Thy4 |
Cipro |
|
Ser79, Ser80 |
|
|
|
|
Ade1, Ade5, Thy15, Thy4 |
Gati |
|
Ser79, Ser80 |
|
|
|
|
Ade1, Ade5, Thy15, Thy4 |
Moxi |
|
Ser79, Ser80 |
|
|
|
|
Ade1, Ade5, Thy15, Thy4 |
The carboxylic acid group at the C3 position showed hydrogen bond interactions in all PDBs when docked with 3K_C (Fig. 5A), 148_3J (Fig. 5B), ciprofloxacin (Fig. 5C), moxifloxacin and gatifloxacin. In the case of 3ILW, interactions were observed with amino acid residues like Gly47, Asn172, and Gly177 (Fig. 5A, B and C, respectively). It was observed that the fluorine atom at the C6 position of the quinolone ring has essential hydrophobic interactions with the Lys49 residue. These were the most important interactions that decided the affinity of these molecules towards DNA gyrase. In addition, the interaction of the hydrophobes at C7 also plays an important role in the affinity of the molecules. The interactions and scores were analyzed by two software packages, MVD and GOLD, which allowed us to differentiate these non-bonded interactions as H-bonding, π–π stacking, and hydrophobic, as shown in Fig. 5. The lack of hydrophobicity at C6 and C7 caused the loss of hydrophobic interactions that were otherwise observed in 3K_C (Fig. 5A), resulting in the loss of activity of 148_3J (Fig. 5B), the least active molecule in the series.
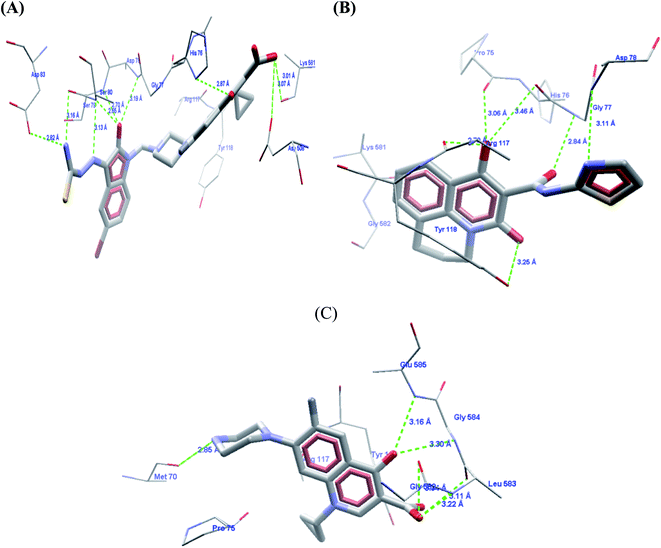 |
| Fig. 5 Comparative docking analysis of (A) 3K_C, (B) 148_3J, and (C) ciprofloxacin, which shows the same binding pattern at the C3 position along with the requirement for a hydrophobic function as per the pharmacophore model at the N1 position. The cyclopropyl ring in this arrangement of ciprofloxacin shows hydrogen bonding with Arg98. | |
The docking analysis in the case of 3FOF showed strong hydrogen bonding interactions with Ser79 and Ser80 residues. This was also observed for moxifloxacin, which was co-crystallized with the protein structure. van der Waals interactions, via π–π stacking with Ade1, Ade5, Thy4 and Thy15 nucleotide bases, hold it in position in the binding site. The cooperative binding model developed by Shen also exhibited the same interactions in which the quinolone molecule associates itself by π–π ring stacking and the tail-to-tail hydrophobic interactions between the N1 substituents.48 The cyclopropyl ring is an important pharmacophoric feature at the N1 substituent, playing a dual role of imparting hydrophobicity and keeping the quinolone nucleus in the unionized form in the cellular environment. The same hypothesis also supported that highly acidic substituents at C3 and a less acidic substituent at the C7 amino functionality will enhance the activity of quinolones.49 Table 4 shows the representative figures of docking in terms of goldscore, rerank score, and binding affinity and interactions with important amino acids are shown in Table 5. The docking analysis using GOLD and Molegro showed that the hydrophobic substituents on the quinolone moiety impart activity to these moieties due to π–π interactions. The interactions can be classified accordingly in both of these protein structures: (i) residues imparting hydrophobic interactions (Lys49, Gly179 and Gly177). (ii) Residues imparting H-bond interactions (Lys49, Asn172). (iii) π–π stacking interactions with the His52 residues, nucleotide bases like Ade1, Ade5, Thy15, and Thy4, and amino acids His52 and Phe64 (Fig. 6).
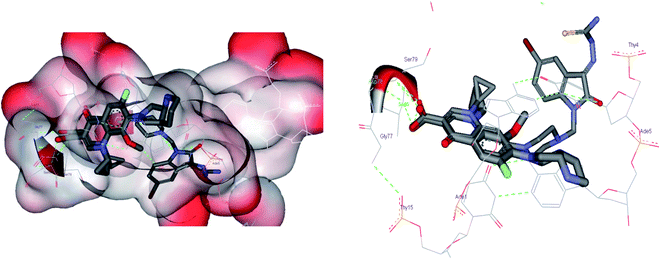 |
| Fig. 6 Docking poses using 3FOF of the most active compound 3K_C and moxifloxacin, showing the π–π stacking interactions of the quinolone nucleus with nucleotide bases such as Ade-1, Ade-5, Thy-5, and Thy-15 with hydrogen bonding interactions of the carboxylic acid part with Ser-79 and Ser-80. The van der Waals surface added diagram also shows that the molecule was bound by these forces. The hydrogen bond acceptor function in the pharmacophore model also supports the evidence produced by docking. The docking analysis was important in this case to study the important interactions in the quinolone binding pocket. The QBP was found to be hydrophobic and the quinolone moiety was found to be located in between the nucleotide moieties to show π–π interactions. The quinolone moiety was found to be blocked in between the base pairs by van der Waals forces. | |
Insight into the binding site
To correlate the pharmacophore-based model with the structure-based model, the Interaction Generation protocol from Discovery Studio 2.0 was used. It was observed that three features as described by the pharmacophore model as HBA (3) and HY (1) were correctly fulfilled as per the binding site requirements. The binding site interactions of 3K_C (Fig. 7A and B) show that the thiourea (S atom) substituent on the isatinyl moiety acts as an acceptor function. The cyclopropyl ring at the N1 position plays an important role as a hydrophobe along with an additional Hy example in the form of the C6 fluorine atom, which undergoes hydrophobic interactions with Lys49. The C3 carboxylic acid function and the 4-oxo group provide the two HBA functionalities. Additionally, it was observed that the presence of an acceptor functionality on the isatinyl substituent of the piperazinyl ring increased the activity by providing the third HBA on the pharmacophore. This was corroborated by the higher fit values observed in the case of prulifloxacin, which had acceptor moieties in the piperazinyl ring.
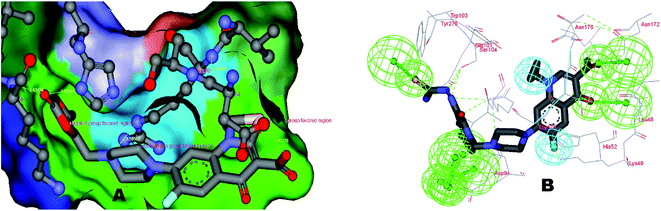 |
| Fig. 7 (A) Binding interactions of 3KC with topoisomerase I. (B) LUDI-based pharmacophore development for 3KC. | |
A study of van der Waals and solvent surfaces revealed that the hydrophobic features are optimum up to the piperazinyl moiety indicated by sky blue surface in Fig. 7A and B, with the van der Waals surface and solvent surface disclosing that the hydrophobic interactions are favored at the N piperazinyl moiety. Then it can be possible to substitute the piperazinyl moiety with polar compounds with acceptor functionalities. The pharmacophore mapping of prulifloxacin supports the same essentialities because prulifloxacin had the highest map values and complete mapping of the pharmacophore developed. The stearic bulk is favored, as indicated by the requirement of HBA in terms of the green surface at the terminal portion of the isatinyl moiety as well as for prulifloxacin. The red surface indicates the negative group-favored regions of the molecule.
Virtual screening
Virtual screening plays an important role in enriching the databases with active compounds and filtering the inactive or less active compounds before in vitro assays and actual experimentation. Virtual screening is capable of achieving good results when integrated with drug discovery processes. It can decrease the cost and time needed for the drug discovery process. In this challenge to explore the probable leads, we screened ∼1.9 million compounds based on the pharmacophore from the databases NCI (NIH 250
250), GSK (13
784), Maybridge (125
465), zinc 11 (∼300
000), liginfo database (1
192
232), Assinex, and the available database of inhouse compounds was used as a screening compound library. We used rigorous filters to avoid unwanted compounds, i.e. toxic compounds or nondrug-like leads. All ligands were firstly washed, i.e. ions and metals were removed, and the remaining structures were neutralized using the CHARMM force field from the prepare ligand protocol available in Discovery Studio 2.0. In this case, we applied a modified Lipinski's rule20 of five for the initial screening because the molecules in the dataset chosen to develop the pharmacophore model also contain leads with MW > 500 so one rule is softened to avoid the exclusion of the important molecules from screening due to higher molecular weight. After the redundancy check, a total of 146 compounds (Table 6) were retained with A
log
P of 2.07 and number of two hydrogen bond acceptors (2H) and one hydrophobe. In order to model the 3D space, 100 conformers were generated for each of the isomers using the diverse conformation generation protocol in Discovery Studio 2.0. A flowchart showing the virtual screening protocol is presented in Fig. 8.
Table 6 Leads identified from virtual screening experiments
S. no. |
Compound structure |
Fit value |
Docking (GOLD) |
Rerank score |
Binding affinity |
1 |
 |
8.806 |
43.75 |
−64.7715 |
−19.17 |
2 |
 |
8.796 |
49.26 |
−61.5138 |
−22.02 |
3 |
 |
8.78 |
52.24 |
−69.172 |
−24.70 |
4 |
 |
8.776 |
50.6 |
−55.6985 |
−21.90 |
5 |
 |
8.772 |
40.32 |
−59.1919 |
−19.89 |
6 |
 |
8.766 |
56.2 |
−66.0826 |
−21.06 |
7 |
 |
8.727 |
51.94 |
−77.9666 |
−24.56 |
8 |
 |
8.41 |
26.9 |
−58.3587 |
−16.71 |
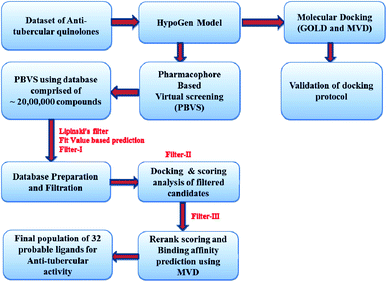 |
| Fig. 8 A flow chart of the virtual screening protocol. | |
The screening library protocol was used for screening the prepared library on the pharmacophore. The best fit method was applied for the screening library and a minimum of 300 hits were allowed to be retrieved from the library in each run. The differentiation criteria applied for the molecules retrieved from the screening were fit values and subsequent docking scores in the form of goldscore and rerank score from GOLD and Molegro software. The binding affinity was also calculated using the Molegro 4.0.1 module. The screened ligands were retrieved using fit value and the docking scores in the terms of GOLD score and Molegro rerank score with consideration of binding affinity predicted by the model. The initial screening retrieved 200 molecules as probable leads, which were further screened by the docking protocol to minimize the errors in the screening of false active compounds in virtual screening (Table 6). The docking model was developed using the quinolone binding pocket in M. tuberculosis using the two PDB codes of 3IFZ and 3FOF. The leads retrieved by the ligand- and structure-based screening are presented in Table 6. The leads were also filtered by the fit value function and three out of four pharmacophore features must map to fulfill the criteria. Table 6 shows all the compounds screened along with their fit values, respective Molegro and GOLD scores, and binding affinity predicted by MVD. A combinatorial library of ciprofloxacin derivatives was designed the state of the art techniques in drug design (ESI S2, Page ESI S4†). The binding affinity and activity were also correlated for the test set of compounds (ESI S3, Page ESI S5†).
Design of compounds for targeted activity
The rational protocol for the drug design and discovery resulted in the identification of cores that can be used in further synthesis and biological evaluation studies. The identified leads like ZINC00113092, ZINC00166174, ZINC00166179 and ZINC00166172 already explained the importance of the sulfonamide core for the biological activity. The idea is to mimic the benzene sulfonamide with methyl piperidine derivatives to obtain a good SAR and novel biological activity for the target.
The identified scaffolds resulted in the design of a library of compounds VM1–VM200 out of these top scoring leads. VM1–VM11 (Table 7) were prioritized for the synthesis. These leads mapped all the optimum features required for the targeted activity well.
Table 7 The predicted activities and scores from docking experiments
Code |
Predicted activity, nM |
Fit value |
Predicted activity, μM |
MolDock score |
Rerank score |
VM10 |
10 410 |
7.8 |
10.4098 |
−123.404 |
−94.6469 |
VM11 |
15 899.6 |
7.6 |
15.8996 |
−66.7065 |
−51.9028 |
VM8 |
21 695.9 |
7.5 |
21.6959 |
−118.902 |
−87.5353 |
VM3 |
46 578.4 |
7.1 |
46.5784 |
−93.2507 |
−72.4736 |
VM2 |
49 336.2 |
7.1 |
49.3362 |
−112.586 |
−89.1212 |
VM4 |
411 486.0 |
6.2 |
411.486 |
−94.9706 |
−75.1147 |
VM9 |
773 296.0 |
5.9 |
773.296 |
−118.432 |
−122.467 |
VM1 |
832 716.0 |
5.9 |
832.716 |
−77.7294 |
−66.0824 |
VM7 |
852 525.0 |
5.9 |
852.525 |
−127.869 |
−100.766 |
VM5 |
879 571.0 |
5.9 |
879.571 |
−108.244 |
−84.0281 |
VM6 |
953 993.0 |
5.8 |
953.993 |
−101.022 |
−74.0222 |
SAR for the identified leads
The identified leads for the synthesis were subjected to the ligand pharmacophore mapping using the previously reported protocols for virtual screening. These leads were prioritized based on their mapping and the predicted biological activities. The pharmacophore map clearly indicated the optimum requirement of the features for the antitubercular activity. Compound VM-2 ((3S,4R)-4-(4-fluorophenyl)-1-methylpiperidin-3-yl)methyl 4-methylbenzenesulfonate showed the mapping of the two tosyl groups from 4-methylbenzenesulfonate and one hydrophobic function supported by 4-fluorophenyl on the identified features from the pharmacophore model (Fig. 9A). Compound VM-7 (3S,4R)-4-(4-fluorophenyl)-1-methyl-3-((trityloxy)methyl)piperidine showed the same mapping pattern for the identified activity (Fig. 9B). The oxygen from the trityloxy group and the nitrogen from the piperidine group are responsible for the mapping of the two hydrogen bond acceptor functions while the benzene ring from the trityloxy functionality is responsible for the one hydrophobic functionality of the pharmacophore model. In conclusion, we can state that the two HBA and two HY functions were responsible for the targeted biological activity from this SAR study, which may be verified by synthesis and biological evaluation in subsequent experiments. The synthesis of the identified leads is presented in Fig. 10 and the data are also reported in following section.
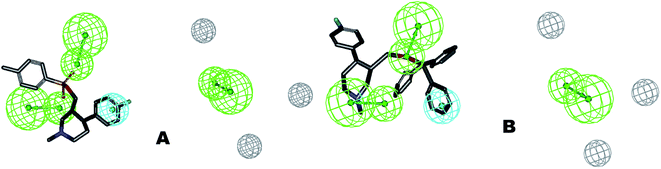 |
| Fig. 9 The ligand pharmacophore mapping of (A) VM-2 and (B) VM-7, the top scoring compounds with good mapping scores. | |
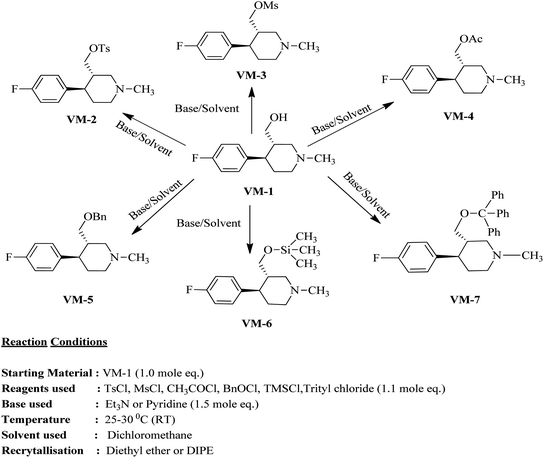 |
| Fig. 10 Synthesis of the designed leads. | |
Antimycobacterial screening
In this study, we performed phenotypic whole-cell screening using M. bovis BCG as a surrogate host and identified ((3S,4R)-4-(4-fluorophenyl)-1-methylpiperidin-3-yl)methyl 4-methylbenzenesulfonate to be a lead compound. The identified lead showed good in vitro activity against M. tuberculosis.
Strains, culture conditions, and chemicals. M. smegmatis, M. bovis BCG, and M. tuberculosis H37Rv were cultured in Middlebrook (MB) 7H9 broth supplemented with 10% albumin dextrose saline, 0.2% glycerol, and 0.05% Tween 80 or MB 7H11 agar supplemented with 10% oleic acid–albumin–dextrose saline as per standard protocols. E. coli culturing was performed in Luria–Bertani (LB) broth or agar at 37 °C and 200 rpm. A chemical library containing approximately 2300 compounds was obtained from the National Cancer Institute Developmental Therapeutic Program (NCI-DTP; https://dtp.cancer.gov/). The compounds in this library belong to either a diversity set, a mechanistic set, or a natural product set. All other chemicals used in this study, unless mentioned otherwise, were procured from Sigma-Aldrich (St. Louis, MO, USA). For MIC determination assays, compounds were prepared as 50 mM stock solutions in DMSO and evaluated for antimycobacterial activity at concentrations ranging from 50 to 0.05 M. For these assays, various strains were grown in MB 7H9 medium until the optical density at 600 nm (OD600) was 0.2, then diluted 1000 times and added to 96-well plates containing drugs. The MIC99 value was defined as the drug concentration at which no visible bacterial growth was observed. For MIC determination, 96-well plates were incubated for either 1 day in the case of E. coli, 2 days in the case of M. smegmatis, or 14 days in the case of M. bovis BCG and M. tuberculosis as per standard protocols.50,51The binding interactions of the most active ligand VM-2 were analyzed in the binding site of topoisomerase I. The important interactions of VM-2 with amino acid Tyr93 showed that the molecule has good probability for binding in the active site of the protein. The ligand also showed hydrophobic interactions with amino acids Gly88, Ala126, Met127 and Ala90. The other stearic and electrostatic interactions with amino acids Asp94, Met81 and Asp89 also contribute to the higher binding scores of the molecule as compared to the standard ligand. The binding scores of these molecules showed that these molecules are good hits for further development. Fig. 11 shows the interactions of these compounds with the target protein. Table 8 shows the MIC values of the molecules.
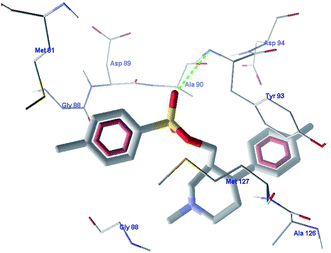 |
| Fig. 11 The binding interactions of VM-2 with the target protein. | |
Table 8 The MIC values of synthesized compounds
S. no. |
Compound code |
Chemical structure |
MIC |
1 |
VM-1 |
 |
>50 |
2 |
VM-2 |
 |
1.25–3.125 |
3 |
VM-3 |
 |
>50 |
4 |
VM-4 |
 |
>50 |
5 |
VM-5 |
 |
>50 |
6 |
VM-6 |
 |
>50 |
7 |
VM-7 |
 |
6.25–12.5 |
8 |
VM-8 |
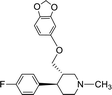 |
50 |
9 |
VM-9 |
 |
>50 |
10 |
VM-10 |
 |
>50 |
11 |
VM-11 |
 |
>50 |
Materials and methods
The pharmacophore was designed and optimized using the HypoGen module of the software Discovery Studio 2.0. Running on a Windows based PC.
Computational details
The two-dimensional structures of the molecules were constructed using MDL ISIS Draw 2.5 software and their activity (MIC) was converted to nM. They were then exported to Discovery Studio 2.0 software, CHARMM42 force field was applied, and the energy was minimized to the closest local minimum. Their conformations were generated using the BEST method of the diverse conformation module of Discovery Studio, which uses the Poling algorithm43 to generate a maximum of 255 conformers covering all possible energy subsets within a threshold of 20 kcal mol−1 about the global minimum structure. The HypoGen module of Discovery Studio software was used to generate a quantitative model to predict antitubercular activity from the given dataset. The molecules, on examination, were found to contain hydrogen bond donors and acceptors, hydrophobic groups and negatively ionizable groups. These parameters with a maximum of five and a minimum of zero were given as inputs to generate the pharmacophore. The minimum inter-feature distance was set to 3.5 Å, considering that some molecules are very large in size. The rest of the parameters were set to their default values. In addition, three excluded volumes were defined. These are regions within the hypothesis wherein the molecule will not be mapped. This was included because catalyst cannot differentiate between molecules that are inactive due to steric hindrance and those that are actually inactive. The ten highest-scoring unique hypotheses are then exported.23
Docking studies of quinolones
Hardware specifications. GOLD version 3.0.1 (ref. 29) and Molegro Virtual Docker (2010) 4.1.0 were executed on a Windows XP-based PC running on an Intel(R) Core™ 2 Quad CPU with 4.00 GB RAM.
Target protein preparation. The crystal structure of DNA gyrase co-crystallized with moxifloxacin from Mycobacterium tuberculosis was used for the docking analysis of these compounds. Crystal structures (PDB ID 3ILW and 3FOF) were downloaded from the protein data bank. Hydrogen atoms were added by using the CHARMM force field.20 The protein was subjected to minimization using the steepest descent (gradient < 0.1) and conjugate gradient algorithms (gradient < 0.01) using the CHARMM force field force field available in Discovery Studio 2.0.21
Docking simulations using Moldock. Moldock software version 4.1.0 combines a differential evolution algorithm with a cavity prediction algorithm. Previously, only differential evolution was applied to molecular docking, which, when combined with the cavity prediction algorithm results in fast and accurate binding modes.44 The scoring function in Moldock is based on Piecewise Linear Potential (PLP), which was introduced by Jones et al.45 and further extended by Yang and Chen in GEMDOCK.46 In this case, the hydrogen bond directionality was taken into account to improve the Moldock scoring functions. EPLP is the piecewise linear potential using two parameters, one for steric resemblance (van der Waals) between the atoms and the other one for hydrogen bond interactions. The docking accuracy was further increased by reranking the highest ranked poses. Ten docking runs was found to be optimal to achieve high docking accuracy.The protein was kept rigid during the docking process, therefore ligand properties were indicated in individuals. The cavity detection algorithm is independent of the orientation of the target molecule. The fitness scores in the docking were calculated using Escore given by: Escore = Einter + Eintra, where Einter is the ligand–protein interaction energy. The PDBs mentioned above were prepared using the docking algorithm by assigning the bond orders, if missing, charges and flexible torsions. In the Molegro docking wizard, the prepared protein was imported and potential binding sites were calculated using the cavity detection algorithm in the Moldock optimizer. The radius of the docking search sphere was set to 10 Å. The cavity detection algorithm (guided differential evolution) was used to focus the search during docking runs. The parameters were fixed for docking runs as follows: number of runs: 10, population size: 50, crossover rate: 0.9, scaling factor: 0.5, and maximum iterations: 2000. Pose clustering was applied to retain the best scoring pose and the best five top scoring poses were retained. The fitness scores calculated by the Moldock scoring function were then evaluated.
Docking simulations using GOLD. GOLD (genetic optimization of ligand docking)47 was used to dock all the molecules under study. For each docking run, 100
000 operations were performed on a population of five islands of 100 individuals. Goldscore, implemented in GOLD, was used to evaluate the results of the docking simulations. Early termination of the genetic optimization was assigned if any five conformations of the docked ligand were found to have an RMSD value ≥1.5 Å. The centre of the binding site cavity was set at the Ser80 residue in the case of 3FOF and at the Lys49 residue in the case of 3ILW with the radius of the sphere set to 10 Å. The GOLD fitness score was calculated from the contributions of hydrogen bonds in the protein ligand complex, ligand strain and van der Waals interactions between the protein and the ligand. The top three scoring conformations for each compound were selected for evaluation.
Synthesis of VM-2 ((3S,4R)-4-(4-fluorophenyl)-1-methylpiperidin-3-yl)methyl 4-methylbenzenesulfonate from VM-1. Alcohol (VM-1, 5.0 g, 1.0 mol eq.) and TEA (4.68 mL, 1.5 mol eq.) were added to CH2Cl2 (25 mL) at 0 °C. Then a solution of tosyl chloride (4.69 g, 1.1 mol eq.) in CH2Cl2 (5 mL) was added dropwise. The reaction mixture was stirred at 0 °C for 30 min then stirred at 25–30 °C for 3–4 h. TLC was used to check that the reaction was completed. Water (10 mL) was added into the reaction mass and then the organic phase was washed with a saturated solution of NaHCO3 (10 mL × 2), followed by brine solution. The organic layer was collected and dried over Na2SO4 and the solvent was evaporated under vacuum. The crude product was purified by column chromatography (silica gel; n-hexane/ethyl acetate = 3
:
1) to afford the desired compound (yield: 6.10 g). MS: 378.15 (M + H), IR (KBr pellets) ν cm−1: 3074, 3035, 2792, 1458, 1361, 1180, 1130, 1H NMR: δ 1.62–1.81 (2H, 1.72 (m, 10.2–10.3), 1.71 (dd, J = 13.6, 3.3, 2.9, 2.7 Hz)), 2.15–2.43 (8H, 2.38 (dd, J = 10.3, 8.5, 2.7 Hz), 2.24 (td, J = 10.2, 7.0, 2.6 Hz), 2.33 (s), 2.33 (s)), 2.45–2.60 (2H, 2.49 (dd, J = 8.5, 2.9, 2.6 Hz), 2.54 (dd, J = 15.0, 10.3 Hz)), 2.67 (1H, dd, J = 15.0, 2.6 Hz), 2.96 (1H, td, J = 10.2, 3.3 Hz), 4.61–4.66 (2H, 4.63 (d, J = 7.0 Hz), 4.63 (d, J = 7.0 Hz)), 7.04 (2H, dd, J = 8.5, 1.4, 0.5 Hz), 7.20 (2H, dd, J = 8.5, 1.1, 0.5 Hz), 7.34 (2H, dd, J = 7.9, 1.7, 0.4 Hz), 7.74 (2H, dd, J = 7.9, 1.5, 0.4 Hz). Spectroscopic data (IR, NMR and mass) for VM-2 are provided in ESI S8 and S9.† HPLC data for the same is provided in ESI S12 to S15.†
Synthesis of VM-3 ((3S,4R)-4-(4-fluorophenyl)-1-methylpiperidin-3-yl)methyl methanesulfonate from VM-1. Alcohol (VM-1, 5.0 g, 1.0 mol eq.) and TEA (4.68 mL, 1.5 mol eq.) were added to CH2Cl2 (25 mL) at 0 °C. Then a solution of mesyl chloride (2.82 g, 1.1 mol eq.) in CH2Cl2 (5 mL) was added dropwise. The reaction mixture was stirred at 0 °C for 30 min then stirred at 25–30 °C for 3–4 h. TLC was used to checked that the reaction was completed. Water (10 mL) was added into the reaction mass, and then the organic phase was washed with a saturated solution of NaHCO3 (10 mL × 2), followed by brine solution. The organic layer was collected and dried over Na2SO4 and the solvent was evaporated under vacuum. The crude product was purified by column chromatography (silica gel; n-hexane/ethyl acetate = 3
:
1) to afford the desired compound (yield: 5.2 g). MS: 302.12 (M + H), IR (KBr pellets) ν cm−1: 3043, 3035, 2927, 2781, 1458, 1168, 1053, 1H NMR: δ 1.62–1.81 (2H, 1.72 (dtd, J = 13.6, 10.2, 2.6 Hz), 1.71 (dd, J = 13.6, 3.3, 2.9, 2.7 Hz)), 2.14–2.43 (5H, 2.37 (dd, J = 10.3, 8.5, 2.7 Hz), 2.24 (td, J = 10.2, 6.9, 2.6 Hz), 2.33 (s)), 2.45–2.60 (2H, 2.49 (dd, J = 8.5, 2.9, 2.6 Hz), 2.54 (dd, J = 15.0, 10.3 Hz)), 2.67 (1H, dd, J = 15.0, 2.6 Hz), 2.88–3.01 (4H, 2.95 (td, J = 10.2, 3.3 Hz), 3.00 (s)), 4.64–4.68 (2H, 4.66 (d, J = 6.9 Hz), 4.66 (d, J = 6.9 Hz)), 7.04 (2H, dd, J = 8.5, 1.4, 0.5 Hz), 7.20 (2H, dd, J = 8.5, 1.1, 0.5 Hz).
Synthesis of VM-4 ((3S,4R)-4-(4-fluorophenyl)-1-methylpiperidin-3-yl)methyl acetate from VM-1. Alcohol (VM-1, 5.0 g, 1.0 mol eq.) and TEA (4.68 mL, 1.5 mol eq.) were added to CH2Cl2 (25 mL) at 0 °C. Then a solution of acetyl chloride (1.93 g, 1.1 mol eq.) in CH2Cl2 (5 mL) was added dropwise. The reaction mixture was stirred at 0 °C for 30 min then stirred at 25–30 °C for 3–4 h. TLC was used to check that the reaction was completed. Water (10 mL) was added into the reaction mass, and then the organic phase was washed with a saturated solution of NaHCO3 (10 mL × 2), followed by brine. The organic layer was collected and dried over Na2SO4 and the solvent was evaporated under vacuum. The crude product was purified by column chromatography (silica gel; n-hexane/ethyl acetate = 5
:
1) to afford the desired compound as a yellow coloured oil (yield: 3.60 g). MS: 266.15 (M + H), IR (neat) ν cm−1: 3066, 3039, 2939, 2785, 1226, 1458, 1161, 1064, 1H NMR: δ 1.61–1.80 (2H, 1.71 (dtd, J = 13.6, 10.2, 2.6 Hz), 1.71 (dd, J = 13.6, 3.3, 2.9, 2.7 Hz)), 2.05 (3H, s), 2.16–2.44 (5H, 2.38 (dd, J = 10.3, 8.4, 2.7 Hz), 2.26 (ttd, J = 10.2, 7.8, 2.6 Hz), 2.33 (s)), 2.45–2.67 (3H, 2.49 (dd, J = 8.4, 2.9, 2.6 Hz), 2.60 (dd, J = 14.6, 10.3 Hz), 2.62 (dd, J = 14.6, 2.6 Hz)), 2.85 (1H, td, J = 10.2, 3.3 Hz), 4.10–4.15 (2H, 4.12 (d, J = 7.8 Hz), 4.12 (d, J = 7.8 Hz)), 7.04 (2H, dd, J = 8.5, 1.4, 0.5 Hz), 7.20 (2H, dd, J = 8.5, 1.1, 0.5 Hz).
Synthesis of VM-5 (3S,4R)-3-((benzyloxy)methyl)-4-(4-fluorophenyl)-1-methylpiperidine from VM-1. Alcohol (VM-1, 5.0 g, 1.0 mol eq.) and TEA (4.68 mL, 1.5 mol eq.) were added to CH2Cl2 (25 mL) at 0 °C. Then a solution of benzoyl chloride (3.46 g, 1.1 mol eq.) in CH2Cl2 (5 mL) was added dropwise. The reaction mixture was stirred at 0 °C for 30 min then stirred at 25–30 °C for 3–4 h. TLC was used to check that the reaction was completed. Water (10 mL) was added into the reaction mass, and then the organic phase was washed with a saturated solution of NaHCO3 (10 mL × 2), followed by brine. The organic layer was collected and dried over Na2SO4 and the solvent was evaporated under vacuum. The crude product was purified by column chromatography (silica gel; n-hexane/ethyl acetate = 5
:
1) to afford the desired compound as a red coloured oil (yield: 5.40 g). MS: 328.17 (M + H), IR (neat) ν cm−1: 3062, 3039, 2939, 2785, 2742, 1458, 1273, 1222, 1111, 1068, 1H NMR: δ 1.61–1.81 (2H, 1.71 (dtd, J = 13.6, 10.2, 2.6 Hz), 1.71 (dd, J = 13.6, 3.3, 2.9, 2.7 Hz)), 2.16–2.43 (5H, 2.37 (ddd, J = 10.3, 8.4, 2.7 Hz), 2.26 (ttd, J = 10.2, 7.8, 2.6 Hz), 2.33 (s)), 2.45–2.59 (2H, 2.49 (dd, J = 8.4, 2.9, 2.6 Hz), 2.52 (dd, J = 14.7, 10.3 Hz)), 2.65 (1H, dd, J = 14.7, 2.6 Hz), 2.86 (1H, td, J = 10.2, 3.3 Hz), 4.09–4.14 (2H, 4.12 (d, J = 7.8 Hz), 4.12 (d, J = 7.8 Hz)), 7.04 (2H, dd, J = 8.5, 1.4, 0.5 Hz), 7.20 (2H, dd, J = 8.5, 1.1, 0.5 Hz), 7.46 (2H, dd, J = 8.5, 7.5, 1.3, 0.4 Hz), 7.59 (1H, tt, J = 7.5, 1.5 Hz), 8.01 (2H, dd, J = 8.5, 1.9, 1.5, 0.4 Hz).
Synthesis of VM-6 (3S,4R)-4-(4-fluorophenyl)-1-methyl-3-(trimethylsilyl)oxy)methyl) piperidine from VM-1. Alcohol (VM-1, 5.0 g, 1.0 mol eq.) and TEA (4.68 mL, 1.5 mol eq.) were added to CH2Cl2 (25 mL) at 0 °C. Then a solution of TMSCl (2.68 g, 1.1 mol eq.) in CH2Cl2 (5 mL) was added dropwise. The reaction mixture was stirred at 0 °C for 30 min then stirred at 25–30 °C for 3–4 h. TLC was used to check that the reaction was completed. Water (10 mL) was added into the reaction mass, and then the organic phase was washed with a saturated solution of NaHCO3 (10 mL × 2), followed by brine. The organic layer was collected and dried over Na2SO4 and the solvent evaporated under vacuum. The crude product was purified by column chromatography (silica gel; n-hexane/ethyl acetate = 3
:
1) to afford the desired compound (yield: 4.60 g). MS: 296.18 (M + H), IR (KBr pellets) ν cm−1: 3043, 2943, 2789, 1226, 1458, 1134, 1072, 1H NMR: δ −0.02 (9H, s), 1.64–1.86 (2H, 1.76 (dtd, J = 13.6, 10.2, 2.6 Hz), 1.71 (dd, J = 13.6, 3.3, 2.9, 2.7 Hz)), 2.14 (1H, ttd, J = 10.2, 7.1, 2.6 Hz), 2.32–2.61 (7H, 2.38 (dd, J = 10.3, 8.3, 2.7 Hz), 2.51 (dd, J = 14.7, 10.3 Hz), 2.56 (dd, J = 14.7, 2.6 Hz), 2.33 (s), 2.49 (dd, J = 8.3, 2.9, 2.6 Hz)), 2.92 (1H, td, J = 10.2, 3.3 Hz), 3.48–3.53 (2H, 3.51 (d, J = 7.1 Hz), 3.51 (d, J = 7.1 Hz)), 7.04 (2H, dd, J = 8.5, 1.4, 0.5 Hz), 7.20 (2H, dd, J = 8.5, 1.1, 0.5 Hz).
Synthesis of VM-7 (3S,4R)-4-(4-fluorophenyl)-1-methyl-3-((trityloxy)methyl)piperidine from VM-1. Alcohol (VM-1, 5.0 g, 1.0 mol eq.) and TEA (4.68 mL, 1.5 mol eq.) were added to CH2Cl2 (25 mL) at 0 °C. Then a solution of trityl chloride (6.86 g, 1.1 mol eq.) in CH2Cl2 (5 mL) was added dropwise. The reaction mixture was stirred at 0 °C for 30 min then stirred at 25–30 °C for 3–4 h. TLC was used to check that the reaction was completed. Water (10 mL) was added into the reaction mass, and then the organic phase was washed with a saturated solution of NaHCO3 (10 mL × 2), followed by brine. The organic layer was collected and dried over Na2SO4, and the solvent evaporated under vacuum. The crude product was purified by column chromatography (silica gel; n-hexane/ethyl acetate = 3
:
1) to afford the desired compound (yield: 8.20 g). MS: 466.24 (M + H), IR (KBr pellets) ν cm−1: 3035, 3032, 2924, 2785, 1222, 1458, 1076, 1064, 1H NMR: δ 1.62–1.82 (2H, 1.71 (dd, J = 13.6, 3.3, 2.9, 2.7 Hz), 1.72 (dtd, J = 13.6, 10.2, 2.6 Hz)), 2.15 (1H, ttd, J = 10.2, 7.5, 2.6 Hz), 2.32–2.44 (4H, 2.38 (dd, J = 10.3, 8.7, 2.7 Hz), 2.33 (s)), 2.44–2.60 (3H, 2.50 (dd, J = 8.7, 2.9, 2.6 Hz), 2.49 (dd, J = 15.4, 2.6 Hz), 2.53 (dd, J = 15.4, 10.3 Hz)), 2.97 (1H, td, J = 10.2, 3.3 Hz), 3.74–3.79 (2H, 3.77 (d, J = 7.5 Hz), 3.77 (d, J = 7.5 Hz)), 7.04 (2H, dd, J = 8.5, 1.4, 0.5 Hz), 7.20 (2H, dd, J = 8.5, 1.1, 0.5 Hz), 7.29–7.40 (9H, 7.35 (dd, J = 8.0, 7.4, 1.6, 0.6 Hz), 7.31 (tt, J = 7.4, 1.4 Hz)), 7.93 (6H, dd, J = 8.0, 1.4, 1.2, 0.6 Hz). IR, NMR, and mass spectroscopic data for VM-7 are provided on ESI Pages S10 and S11.† HPLC data for the same are included on ESI Pages S12 to S15.†
Synthesis of VM-8 (3S,4R)-3-((benzo[d][1,3]dioxol-5-yloxy)methyl)-4-(4-fluorophenyl)-1-methylpiperidine from VM-2. Starting material VM-2 (10.0 g, 1.0 mol eq.), benzo[d][1,3]dioxol-5-ol (4.4 g, 1.2 mol eq.), KOH (5.22 g, 3.0 mol eq.) and DMF (30 mL) were placed in a round bottom flask and stirred at 70–75 °C under nitrogen for 3 h. After completion of the reaction (monitored by TLC) the reaction mixture was cooled to 40–45 °C and stirred for about 1 h. Distilled water (120 mL) was added slowly dropwise into the reaction mass at 40–45 °C. Solid precipitated out during the addition of distilled water at 40–45 °C. The reaction mass was gradually cooled to 25–30 °C and stirred for 2 h at the same temperature. The crystals were collected by filtration, washed with distilled water (10 mL) and dried under vacuum at 60–65 °C for 12–14 h. Re-crystallization of the solid material was done in IPA to obtained VM-8 (yield: 5.10 g) as a light brown coloured solid (Fig. 12). MS: 344.30 (M + H), IR (KBr pellets) ν cm−1: 3074, 3024, 2943, 2924, 2785, 1184, 1083, 1037, 1H NMR (CDCl3, 400 mHz): 1.76–1.87 (m, 2H), 1.965–3.18 (m, 2H), 2.02–2.95 (m, 2H), 2.16–2.23 (m, 1H), 2.33 (s, 3H), 2.38–2.45 (m, 1H), 3.39–3.55 (m, dd, J = 2.8 Hz, J = 9.2 Hz, 2H), 5.82 (s, 2H), 6.09–6.11 (dd, J = 2.4 Hz, J = 8.4 Hz, 1H), 6.322–6.328 (d, J = 2.4 Hz, 1H), 6.57–6.659 (d, J = 8.4 Hz, 1H), 6.91–6.96 (m, 2H), 7.11–7.15 (m, 2H).
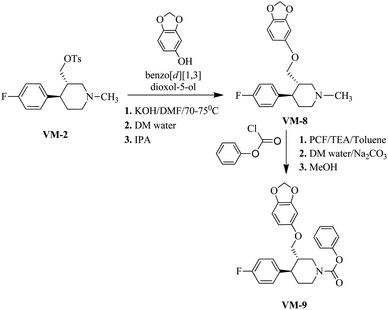 |
| Fig. 12 The synthesis of the designed leads. | |
Synthesis of VM-9 (3S,4R)-phenyl 3-((benzo[d][1,3]dioxol-5-yloxy)methyl)-4-(4-fluorophenyl)piperidine-1-carboxylate from VM-8. A solution of VM-8 (5.0 g, 1.0 mol eq.) and triethyl amine (3.04 mL, 1.5 mol eq.) in toluene (25 mL) was cooled to 0–5 °C and phenyl chloroformate (2.50 g, 1.1 mol eq.) was added dropwise. After warming to room temperature, the mixture was stirred for 18 h. For workup, the mixture was diluted with toluene (15 mL) and washed with saturated aqueous Na2CO3 solution (3 × 20 mL) followed by washing with saturated NaCl solution (20 mL). The organic phase was dried with anhydrous Na2SO4 and evaporated under vacuum, giving a crude product, which was recrystallized in methanol to obtain VM-9 (yield: 7.50 g) as a brown coloured solid. MS: 450.20 (M + H), IR (KBr pellets) ν cm−1: 3058, 3082, 2962, 2924, 2904, 2787, 1450, 1188, 1095, 1041, 1H NMR (CDCl3, 400 mHz): 1.74–1.85 (m, 2H), 1.98–3.16 (m, 2H), 2.02–2.92 (m, 2H), 2.18–2.24 (m, 1H), 2.38–2.45 (m, 1H), 3.39–3.55 (m, dd, J = 2.8 Hz, J = 9.2 Hz, 2H), 5.82 (s, 2H), 6.09–6.11 (dd, J = 2.4 Hz, J = 8.4 Hz, 1H), 6.320–6.326 (d, J = 2.4 Hz, 1H), 6.55–6.66 (d, J = 8.4 Hz, 1H), 6.90–6.98 (m, 2H), 7.12–7.16 (m, 2H).
Synthesis of VM-10 (3S,4R)-3-((benzo[d][1,3]dioxol-5-yloxy)methyl)-4-(4-fluorophenyl)-1-tosylpiperidine from (3S,4R)-3-((benzo[d][1,3]dioxol-5-yloxy)methyl)-4-(4-fluorophenyl) piperidine. To a solution of (3S,4R)-3-((benzo[d][1,3]dioxol-5-yloxy)methyl)-4-(4-fluorophenyl)piperidine (5.0 g, 1.0 mol eq.) in dichloromethane (25 mL), pyridine (1.80 g, 1.5 mol eq.) was added and the mixture was cooled to 0 °C. Then, tosyl chloride solution (3.18 g, 1.1 mol eq. in 10 mL of DCM) was added dropwise at 0 °C. The solution was stirred at 0 °C for 30 min and left at room temperature (25–30 °C) until completion of the reaction (determined by TLC). The DCM layer was washed with HCl (1 N) (10 mL × 2), water (10 mL × 2) and brine (10 mL × 2). The organic layer was dried over Na2SO4 and the solvent was removed under reduced pressure. The crude residue was purified by column chromatography (100–200 mesh on silica gel, eluent 10% EtOAc–hexane) to isolate VM-11 (yield: 4.6 g) as a white coloured solid (Fig. 13). MS: 484.15 (M + H), IR (KBr pellets) ν cm−1: 3047, 3039, 2920, 2877, 1456, 1604, 1496, 1342, 1165, 1095, 1H NMR (MeOD, 400 mHz): 1.96–2.12 (m, 2H), 2.42–2.54 (m, 1H), 2.95 (m, 3H), 2.94–2.99 (m, 1H), 3.15–3.60 (m, 2H), 3.14–3.70 (m, 2H), 3.50–3.72 (m, 2H), 5.86 (s, 2H), 6.12–6.16 (dd, J = 2.4 Hz, J = 8.4 Hz, 1H), 6.361–6.367 (d, J = 2.4 Hz, 1H), 6.590–6.611 (d, J = 8.4 Hz, 1H), 7.011–7.055 (m, 2H), 7.264–7.299 (m, 2H), 7.40–7.42 (m, 2H), 7.72–7.75 (m, 2H).
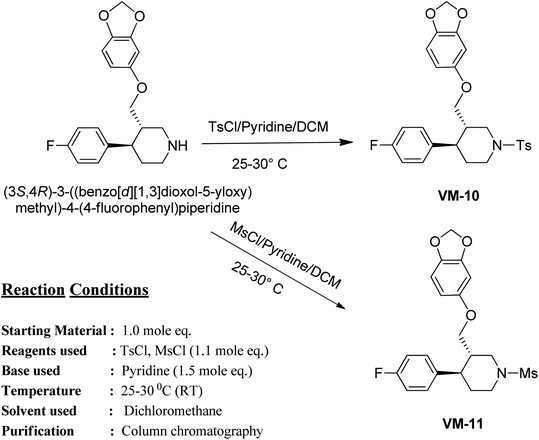 |
| Fig. 13 The synthesis of the designed leads. | |
Synthesis of VM-11 (3S,4R)-3-((benzo[d][1,3]dioxol-5-yloxy)methyl)-4-(4-fluorophenyl)-1-(methylsulfonyl)piperidine from (3S,4R)-3-((benzo[d][1,3]dioxol-5-yloxy) methyl)-4-(4-fluorophenyl)piperidine. To a solution of (3S,4R)-3-((benzo[d][1,3]dioxol-5-yloxy)methyl)-4-(4-fluorophenyl)piperidine (5.0 g, 1.0 mol eq.) in dichloromethane (25 mL), pyridine (1.80 g, 1.5 mol eq.) was added and the mixture was cooled to 0 °C. Then, mesyl chloride solution (1.91 g, 1.1 mol eq. in 10 mL of DCM) was added dropwise at 0 °C. The solution was stirred at 0 °C for 30 min and left at room temperature (25–30 °C) until completion of the reaction (determined by TLC). The DCM layer was washed with HCl (1 N) (10 mL × 2), water (10 mL × 2) and brine (10 mL × 2). The organic layer was dried over Na2SO4 and the solvent was removed under reduced pressure. The crude residue was purified by column chromatography (100–200 mesh on silica gel, eluent 10% EtOAc–hexane) to isolate VM-11 (yield: 4.3 g) as an off white coloured solid. MS: 430.10 (M + Na), IR (KBr pellets) ν cm−1: 3046, 3020, 2927, 2920, 1604, 1505, 1226, 1111, 1099, 1H NMR (MeOD, 400 mHz): 1.99–2.15 (m, 2H), 2.41–2.50 (m, 1H), 2.95 (m, 3H), 2.933–3.00 (m, 1H), 3.12–3.55 (m, 2H), 3.12–3.68 (m, 2H), 3.50–3.68 (m, 2H), 5.82 (s, 2H), 6.139–6.16 (dd, J = 2.4 Hz, J = 8.4 Hz, 1H), 6.361–6.367 (d, J = 2.4 Hz, 1H), 6.590–6.611 (d, J = 8.4 Hz, 1H), 7.011–7.055 (m, 2H), 7.264–7.299 (m, 2H).
Author contributions
Conceptualization: Deepti Mathpal, Mukesh Masand, Anisha Thomas, Vishal M. Balaramnavar; data curation: Mohd Saeed; formal analysis: Gaffar Sarwar Zaman; funding acquisition: NA; investigation: Mehnaz Kamal, Talha Jawaid, Pramod K. Sharma, Madan M. Gupta; methodology: Santosh Kumar, Swayam Prakash Srivastava, Vishal M. Balaramnavar; project administration: Vishal M. Balaramnavar; resources: Irfan Ahmad; software: Mohd Saeed; supervision: Santosh Kumar, Swayam Prakash Srivastava; validation: Vishal M. Balaramnavar; visualization: Mehnaz Kamal, Talha Jawaid; writing – original draft: Vishal M. Balaramnavar; writing – review & editing: Anisha Thomas; biological evaluation: authors to be added.
Conflicts of interest
There are no conflicts to declare.
Acknowledgements
The authors are thankful to the Deanship of Scientific Research, King Khalid University, Abha, Saudi Arabia, for financially supporting this work through a Small Research Group Project under grant number (R.G.P.1/48/42). Authors are also thankful to Thsti (Translation Health Science and Technology Institute) NCR Biotech Science Cluster, 3rd Milestone, Faridabad – Gurugram Expressway, PO box #04 for supporting the biological evaluation part of the manuscript.
References
- C. Dye, Lancet, 2006, 367, 938–940 CrossRef.
- P. Pahal and S. Sharma, in StatPearls, StatPearls Publishing, 2020 Search PubMed.
- D. Butler, Nature, 2000, 406, 670–672 CrossRef CAS PubMed.
- A. MacNeil, P. Glaziou, C. Sismanidis, S. Maloney and K. Floyd, Morb. Mortal. Wkly. Rep., 2019, 68, 263 CrossRef PubMed.
- D. Canetti, N. Riccardi, M. Martini, S. Villa, A. Di Biagio, L. Codecasa, A. Castagna, I. Barberis, V. Gazzaniga and G. Besozzi, Tuberculosis, 2020, 122, 101921 CrossRef PubMed.
- A. Singh, A. K. Gupta and S. Singh, in NanoBioMedicine, Springer, 2020, pp. 285–314 Search PubMed.
- S. Soldevila and F. Bosca, Spectrochim. Acta, Part A, 2020, 227, 117569 CrossRef CAS PubMed.
- M. V. de Almeida, M. F. Saraiva, M. V. de Souza, C. F. da Costa, F. R. Vicente and M. C. Lourenço, Bioorg. Med. Chem. Lett., 2007, 17, 5661–5664 CrossRef CAS PubMed.
- L. L. Shen, L. A. Mitscher, P. N. Sharma, T. O'donnell, D. W. Chu, C. S. Cooper, T. Rosen and A. G. Pernet, Biochemistry, 1989, 28, 3886–3894 CrossRef CAS PubMed.
- S. C. Kampranis and A. Maxwell, Proc. Natl. Acad. Sci., 1996, 93, 14416–14421 CrossRef CAS PubMed.
- D. C. Hooper and E. Rubinstein, Quinolone antimicrobial agents, ASM Press, Washington, DC, 2003 Search PubMed.
- M. Kumar, S. Dahiya, P. Sharma, S. Sharma, T. P. Singh, A. Kapil and P. Kaur, PLoS One, 2015, 10, e0126560 CrossRef PubMed.
- M. H. Baig, K. Ahmad, G. Rabbani, M. Danishuddin and I. Choi, Curr. Neuropharmacol., 2018, 16, 740–748 CrossRef CAS PubMed.
- X. Lin, X. Li and X. Lin, Molecules, 2020, 25(6), 1375 CrossRef CAS PubMed.
- L. G. Ferreira, R. N. Dos Santos, G. Oliva and A. D. Andricopulo, Molecules, 2015, 20, 13384–13421 CrossRef CAS PubMed.
- O. Dror, D. Schneidman-Duhovny, Y. Inbar, R. Nussinov and H. J. Wolfson, J. Chem. Inf. Model., 2009, 49, 2333–2343 CrossRef CAS PubMed.
- V. M. Balaramnavar, I. A. Khan, J. A. Siddiqui, M. P. Khan, B. Chakravarti, K. Sharan, G. Swarnkar, N. Rastogi, H. Siddiqui and D. P. Mishra, J. Med. Chem., 2012, 55, 8248–8259 CrossRef CAS PubMed.
- V. M. Balaramnavar, R. Srivastava, N. Rahuja, S. Gupta, A. K. Rawat, S. Varshney, H. Chandasana, Y. S. Chhonker, P. K. Doharey and S. Kumar, Eur. J. Med. Chem., 2014, 87, 578–594 CrossRef CAS PubMed.
- D. Maciejewska, T. Zolek and F. Herold, J. Mol. Graphics Modell., 2006, 25, 353–362 CrossRef CAS PubMed.
- G. Klebe, U. Abraham and T. Mietzner, J. Med. Chem., 1994, 37, 4130–4146 CrossRef CAS PubMed.
- K. Ahmad, V. M. Balaramnavar, M. H. Baig, A. K. Srivastava, S. Khan and M. A. Kamal, CNS Neurol. Disord.: Drug Targets, 2014, 13, 1346–1353 CrossRef CAS PubMed.
- S. Varshney, K. Shankar, M. Beg, V. M. Balaramnavar, S. K. Mishra, P. Jagdale, S. Srivastava, Y. S. Chhonker, V. Lakshmi and B. P. Chaudhari, J. Lipid Res., 2014, 55, 1019–1032 CrossRef CAS PubMed.
- A. Saxena, V. M. Balaramnavar, T. Hohlfeld and A. K. Saxena, Eur. J. Pharmacol., 2013, 721, 215–224 CrossRef CAS PubMed.
- J. M. Musser, Clin. Microbiol. Rev., 1995, 8, 496–514 CrossRef CAS PubMed.
- M. Barančoková, D. Kikelj and J. Ilaš, Future Med. Chem., 2018, 10, 1207–1227 CrossRef PubMed.
- P. E. Almeida Da Silva and J. C. Palomino, J. Antimicrob. Chemother., 2011, 66, 1417–1430 CrossRef CAS PubMed.
- A. Bahuguna and D. S. Rawat, Med. Res. Rev., 2020, 40, 263–292 CrossRef PubMed.
- A. Chopra and I. Ra, Understanding antibacterial action and resistance, Ellis Horwood Limited, London, United Kingdom, 1996 Search PubMed.
- A. Fillion, A. Aubry, F. Brossier, A. Chauffour, V. Jarlier and N. Veziris, Antimicrob. Agents Chemother., 2013, 57, 4496–4500 CrossRef CAS PubMed.
- N. Veziris, C. Truffot-Pernot, A. Aubry, V. Jarlier and N. Lounis, Antimicrob. Agents Chemother., 2003, 47, 3117–3122 CrossRef CAS PubMed.
- J. Sarathy, L. Blanc, N. Alvarez-Cabrera, P. O’Brien, I. Dias-Freedman, M. Mina, M. Zimmerman, F. Kaya, H. P. Ho Liang, B. Prideaux, J. Dietzold, P. Salgame, R. M. Savic, J. Linderman, D. Kirschner, E. Pienaar and V. Dartois, Antimicrob. Agents Chemother., 2019, 63, e00583-19 Search PubMed.
- T. D. M. Pham, Z. M. Ziora and M. A. T. Blaskovich, Medchemcomm, 2019, 10, 1719–1739 RSC.
- G. Klopman, D. Fercu, T. E. Renau and M. R. Jacobs, Antimicrob. Agents Chemother., 1996, 40, 2637–2643 CrossRef CAS PubMed.
- G. Klopman, J.-Y. Li, S. Wang, A. J. Pearson, K. Chang, M. R. Jacobs, S. Bajaksouzian and J. J. Ellner, Antimicrob. Agents Chemother., 1994, 38, 1794–1802 CrossRef CAS PubMed.
- R. Gozalbes, M. Brun-Pascaud, R. García-Domenech, J. Gálvez, P.-M. Girard, J.-P. Doucet and F. Derouin, Antimicrob. Agents Chemother., 2000, 44, 2764–2770 CrossRef CAS PubMed.
- E. M. Tretter, A. J. Schoeffler, S. R. Weisfield and J. M. Berger, Proteins, 2010, 78, 492–495 CrossRef CAS PubMed.
- I. Laponogov, M. K. Sohi, D. A. Veselkov, X.-S. Pan, R. Sawhney, A. W. Thompson, K. E. McAuley, L. M. Fisher and M. R. Sanderson, Nat. Struct. Mol. Biol., 2009, 16, 667–669 CrossRef CAS PubMed.
- I. Ukrainets, A. Tkach, E. Mospanova and E. Svechnikova, Chem. Heterocycl. Compd., 2007, 43, 1014–1019 CrossRef CAS.
- I. Ukrainets, A. Tkach and L. Grinevich, Chem. Heterocycl. Compd., 2008, 44, 956 CrossRef CAS.
- D. Sriram, P. Yogeeswari, J. S. Basha, D. R. Radha and V. Nagaraja, Bioorg. Med. Chem., 2005, 13, 5774–5778 CrossRef CAS PubMed.
- D. Sriram, T. R. Bal, P. Yogeeswari, D. R. Radha and V. Nagaraja, J. Gen. Appl. Microbiol., 2006, 52, 195–200 CrossRef CAS PubMed.
- B. R. Brooks, R. E. Bruccoleri, B. D. Olafson, D. J. States, S. A. Swaminathan and M. Karplus, J. Comput. Chem., 1983, 4, 187–217 CrossRef CAS.
- P. W. Sprague, Automated chemical hypothesis generation and database searching with Catalyst, in Perspectives in Drug Discovery and Design, ed. K. Muller, ESCOMB. V. Science Publishers , Leiden, The Netherlands, 1995, pp. 1–21 Search PubMed.
- R. Thomsen and M. H. Christensen, J. Med. Chem., 2006, 49, 3315–3321 CrossRef CAS PubMed.
- G. Jones, P. Willett, R. C. Glen, A. R. Leach and R. Taylor, J. Mol. Biol., 1997, 267, 727–748 CrossRef CAS PubMed.
- J. M. Yang and C. C. Chen, Proteins: Struct., Funct., Bioinf., 2004, 55, 288–304 CrossRef CAS PubMed.
- M. L. Verdonk, J. C. Cole, M. J. Hartshorn, C. W. Murray and R. D. Taylor, Proteins: Struct., Funct., Bioinf., 2003, 52, 609–623 CrossRef CAS PubMed.
- L. L. Shen, Adv. Pharmacol., 1994, 29, 285–304 Search PubMed.
- H. Nikaido and D. Thanassi, Antimicrob. Agents Chemother., 1993, 37, 1393 CrossRef CAS PubMed.
- S. Kidwai, C.-Y. Park, S. Mawatwal, P. Tiwari, M. G. Jung, T. P. Gosain, P. Kumar, D. Alland, S. Kumar, A. Bajaj, Y-K Hwang, C. S. Song, R. Dhiman, Ill Young Lee and R. Singh, Antimicrob. Agents Chemother., 2017, 61(11), e00969 CrossRef CAS PubMed.
- S. Kidwai, R. Bouzeyen, S. Chakraborti, N. Khare, S. Das, T. P. Gosain, A. Behura, L. Chhuttan Meena, R. Dhiman, M. Essafi, B. Avinash, D. K. Saini, N. Srinivasan and D. Mahajan, Ramandeep Singh Antimicrob. Agents Chemother., 2019, 63(9), e00996 CrossRef CAS PubMed.
Footnotes |
† Electronic supplementary information (ESI) available. See DOI: 10.1039/d1ra05630a |
‡ These two authors have equal contributions for the manuscript. |
|
This journal is © The Royal Society of Chemistry 2021 |
Click here to see how this site uses Cookies. View our privacy policy here.