DOI:
10.1039/D1RA04576H
(Paper)
RSC Adv., 2021,
11, 28876-28891
Lipid polymer hybrid nanocarriers as a combinatory platform for different anti-SARS-CoV-2 drugs supported by computational studies†
Received
14th June 2021
, Accepted 4th August 2021
First published on 27th August 2021
Abstract
The COVID-19 pandemic caused by SARS-CoV-2 has demonstrated the potential of emergent pathogens to severely damage public health and global economies. As a consequence of the pandemic, millions of people have been forced into self-isolation, which has negatively affected the global economy. More efforts are needed to find new innovative approaches that could fundamentally change our understanding and management of this disaster. Herein, lipid polymer hybrid nanoparticles (LPH NPs) were utilized as a platform for the delivery of azithromycin or niclosamide in combination with piroxicam. The obtained systems were successfully loaded with both azithromycin and piroxicam (LPHAzi–Pir) with entrapment efficiencies (EE%) of 74.23 ± 8.14% and 51.52 ± 5.45%, respectively, or niclosamide and piroxicam (LPHNic–Pir) with respective EE% of 85.14 ± 3.47% and 48.75 ± 4.77%. The prepared LPH NPs had a core–shell nanostructure with particle size ≈ 125 nm and zeta potential ≈ −16.5 irrespective of drug payload. A dose-dependent cellular uptake of both LPH NPs was observed in human lung fibroblast cells. An enhanced in vitro antiviral efficacy of both LPHAzi–Pir and LPHNic–Pir was obtained over the mixed solution of the drugs. The LPH NPs of azithromycin or niclosamide with piroxicam displyed a promising capability to hinder the replication of SARS-CoV-2, with IC50 of 3.16 and 1.86 μM, respectively. These results provide a rationale for further in vivo pharmacological as well as toxicological studies to evaluate the potential activity of these drugs to combat the COVID-19 outbreak, especially the concept of combination therapy. Additionally, the molecular docking of macrolide bioactive compounds against papain-like protease (PDB ID:6wuu) was achieved. A ligand-based study, especially rapid overlay chemical structure (ROCS), was also examined to identify the general pharmacophoric features of these compounds and their similarity to reported anti-SARS-CoV-2 drugs. Molecular dynamic simulation was also implemented.
1. Introduction
In March 2020, WHO declared COVID-19, which is caused by the severe acute respiratory syndrome-associated coronavirus 2 (SARS-CoV-2), a pandemic.1 Although vaccination is expected to reduce the spread of COVID-19, eruptions of this pandemic are expected to continue potentially over the next few years.2,3 Defining the SARS-CoV-2 virus genome sequence was a decisive tool concerning the identification of potential drug targets.4 During pandemic periods, it is improper to pharmaceutically develop new drug entities in a complicated process that could take up to 10 years.5 In this case, outlining new therapeutic benefits for previously approved therapeutic agents through drug repurposing could be a worthwhile strategy.6,7 Recently, different drugs have either been suggested for or subjected to repurposing to combat COVID-19.8 Macrolides are considered as broad spectrum antibiotics that resemble the first-line therapy for lower respiratory tract infections in adults.9 According to the US and European authorities, macrolides are the drug of choice either as a first-line treatment in cases of pneumonia, or as acceptable alternatives. In France, the recommendations include amoxicillin as a first choice or a macrolide, while in Germany, the first choice is penicillin and the alternative drug is a macrolide, and in Italy, the first choices are penicillin, amoxicillin, ampicillin plus sulbactam, and amoxicillin plus clavulanic acid, and the alternative is a macrolide as approved combinations.9 This prompted us to explore this class of antimicrobials, searching for a COVID-19 remedy. Among these agents, azithromycin is a macrolide antibiotic with broad activity against both Gram-positive and Gram-negative bacteria. In addition, azithromycin has antibacterial activity against both Haemophilus influenza and Streptococcus pneumonia, which are responsible for lower respiratory tract diseases.10 Previous studies have reported the in vitro antiviral activity of azithromycin against a wide variety of viruses like Zika, Ebola, rhinovirus, enterovirus, influenza, and recently SARS-CoV-2 virus.11 Niclosamide, a widely used anthelminthic, was repurposed in the treatment of many pulmonary diseases like asthma and cystic fibrosis, as well as COVID-19, due to its bronchodilation effect, inhibition of mucus production, and release of pro-inflammatory cytokines.12 In addition, niclosamide has a reported antibacterial activity which could be beneficial in combating pulmonary superinfections.13 Moreover, piroxicam, which is a potent nonsteroidal anti-inflammatory that could decrease the cytokine storm, had an in vitro antiviral activity against herpes simplex virus type 1.14
Nanotechnology is considered the most noticeable tool in the development of new drug carrier systems, providing versatile clinical applications and scale-up for industrial production.15 Polymeric nanoparticles (NPs) and lipid nanocarriers were reported to be the main investigated types of NPs that had been approved by the FDA for clinical applications.16 Both types of NPs provide good drug loading, biodegradability, biocompatibility, ease of modification by different targeting moieties, structural integrity, and stability characteristics.17,18 However, polymeric NPs suffer from poor loading of hydrophilic bioactive compounds and uncontrolled drug release.19 In addition, lipid nanocarriers are often associated with drug leakage during storage and rapid in vivo clearance from plasma.20 Hence, in the last decade, a delivery system possessing the advantages of both polymer- and lipid-based formulations, and referred to as lipid–polymer hybrid nanoparticles (LPH NPs), was proposed. They are composed of polymer cores and lipid shells.21 Therefore, LPH NPs with their inimitable structure could combine features of both polymeric and lipid NPs and circumvent their drawbacks.20 By virtue of their unique structure, LPH NPs have versatile competence to encapsulate different types of payloads, such as hydrophilic or hydrophobic drugs, or nucleic acids.22 The lipid–polymer combination would endow the LPH NPs with structural integrity, serum stability, sustained drug release (from the polymer core), and high biocompatibility (from the lipid shell).21
In our previous study for the repurposing of many chemotherapeutic drugs as anti-SARS-CoV-2 agents, azithromycin and niclosamide displayed strong anti-SARS-CoV-2 activity. Moreover, we found that piroxicam is preferable to other non-steroidal anti-inflammatory drugs in the management of COVID-19. In this regard, the strategy for this work, Scheme 1, is elaborated based on a drug combination of azithromycin or niclosamide with piroxicam.23 This study aims to scrutinize the ability of LPH NPs as a suitable platform for the co-delivery of these combinations as possible relevant approaches against COVID-19. To this end, we fabricated multi-payload LPH NPs by a single nanoprecipitation self-assembly technique. The developed platforms were characterized and their antiviral activity was assessed against SARS-CoV-2. Chemoinformatic studies and molecular dynamic simulation were also implemented to understand their binding interaction and to figure out the most relevant macrolide drugs for further studies to combat the COVID-19 outbreak. Moreover, MD simulation studies of the most promising drugs were performed to evaluate the dynamic stability of the complex, which revealed that the drug significantly stabilizes the Mopar and Spike protein of SARS-CoV-2.
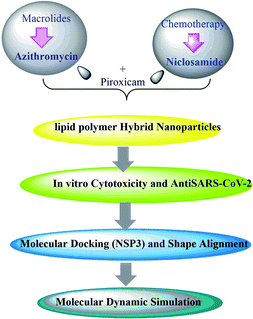 |
| Scheme 1 Design and working steps. | |
2. Materials and method
2.1. Materials
A co-polymer of DL-lactide/glycolide (50/50) conjugate and acid terminated with an inherent viscosity midpoint of 0.2 dL g−1 (PURASORB PLGA) was purchased from Corbion (Netherlands). Lecithin soybean (3-sn-phosphatidylcholine ≥99% (TLC) lyophilized powder), dialysis tubing (MWCO 12 kDa), dimethylformamide (DMF), acetonitrile (HPLC grade), methanol (HPLC), absolute ethanol, and Triton X-100 were supplied by Sigma-Aldrich, UK. DSPE-PEG 2000 was purchased from Avanti Polar Lipids (USA). Gibco RPMI-1640 media, fetal bovine serum (FBS), penicillin/streptomycin, GlutaMAX, trypsin/EDTA, phosphate-buffered saline (PBS) and 1,1′-dioctadecyl-3,3,3′,3′-tetramethylindocarbocyanine perchlorate (DiI) were obtained from ThermoFisher Scientific (UK). Potassium dihydrogen orthophosphate, sodium hydroxide, sodium chloride, potassium chloride, sodium dibasic hydrogen orthophosphate, hydrochloric acid, and Tween 80 (polysorbate 80) were obtained from Fluka Chemika-BioChemika, Switzerland. The tested drugs, azithromycin, niclosamide, and piroxicam, were kindly donated by the Egyptian International Pharmaceutical Industries “EIPICO”, the Holding Company for Pharmaceuticals, Chemicals, and Medical Appliances “HoldiPharma”, and the National Organization for Drug Control and Research in Egypt. All solvents used were of HPLC grade; methanol and acetonitrile were purchased from Sigma-Aldrich Chemical Co. (St Louis, MO, USA). The macrolide bioactive compounds are addressed in the Table S1 ESI file.†10,24–41
2.2. HPLC determination of azithromycin, piroxicam, and niclosamide
2.2.1. Standard solutions preparation. Azithromycin (10 mg) was dissolved in 10 mL of methanol under sonication for 15 minutes to prepare an azithromycin standard stock solution (1 mg mL−1). The solution was then filtered in a 10 mL volumetric flask before being made up to the final volume with methanol. Piroxicam and niclosamide standard stock solutions (1 mg mL−1 in methanol) were prepared separately in the same way as azithromycin.
2.2.2. Chromatographic conditions. The utilized HPLC system was a Dionex UltiMate 3000 HPLC (Thermo Scientific™, Dionex™, Sunnyvale, CA, USA). The instrument is composed of a WPS-3000TSL autosampler, an LPG-3400SD quaternary pump, a VWD-3000 variable wavelength detector, and a TCC-3000SD column thermostat. Data processing and acquisition were carried out with Chromeleon 7 software. The stationary phase was a Thermo Hypersil ODS C18 column (250 × 4.6 mm, 5 μm). The chromatographic conditions were run in an isocratic elution mode, using a mobile phase consisting of 10 mM phosphate buffer (pH = 3.5) and acetonitrile in a ratio of 80
:
20, v/v. The volume of injection was 10 μL, the flow rate was 1 mL min−1, the wavelengths of detection were 332, 360, and 210 nm for niclosamide, piroxicam, and azithromycin, respectively, and the column temperature was set at 40 °C. The mobile phase was filtered with a 0.45 μm filter before injection. Niclosamide, piroxicam, and azithromycin were eluted at 4.03, 2.95, and 3.56 minutes, respectively.
2.2.3. Construction of calibration curves. Different aliquots were transferred from azithromycin, niclosamide, and piroxicam stock solutions (1 mg mL−1) separately into a series of 10 mL volumetric flasks. The chromatograms for the prepared solutions were recorded by applying the previously described chromatographic conditions. The construction of azithromycin, niclosamide, and piroxicam calibration curves was performed by plotting the peak area of each solution against the equivalent concentration, from which the regression equations were computed. The calibration curve showed a linear relationship in the range of 80–240 μg mL−1 for azithromycin, 0.1–100 μg mL−1 for niclosamide, and 0.5–50 μg mL−1 for piroxicam.
2.2.4. Validation of the chromatographic method. The accuracy, and intraday and interday precision of the chromatographic method were evaluated through triplicate analysis of three niclosamide concentrations (1, 10, 50 μg mL−1), three piroxicam concentrations (1, 10, 20 μg mL−1), and three azithromycin concentrations (100, 140, 200 μg mL−1) on the same/different days followed by calculation of the % recovery, the standard deviation (SD) and the percentage relative standard deviation (% RSD).
2.3. Preparation of LPH NPs
Different LPH NPs formulations co-loaded with azithromycin and piroxicam (LPHAzi–Pir) or niclosamide and piroxicam (LPHNic–Pir) were prepared by a single-step nanoprecipitation self-assembly technique.42 Briefly, PLGA (5 mg mL−1), piroxicam (0.75 mg mL−1) and azithromycin (0.75 mg mL−1) or niclosamide (0.75 mg mL−1) were dissolved in DMF (1 mL) to form the organic phase. The aqueous phase was prepared by dissolving lecithin (0.5 mg mL−1), DSPE-PEG 2000 (0.5 mg mL−1) and Tween® 80 (10 mg mL−1) in 4% v/v hydroalcoholic solution (9 mL) at 70 °C for 15 min to ensure the complete desolvation of the lipid. Subsequently, the aqueous phase was titrated dropwise with the organic phase under magnetic stirring (500 rpm) for 2 h at room temperature. The ratio of organic to aqueous phase was kept at 1
:
9 v/v. The resultant LPH NPs whitish cloudy dispersions were centrifuged at 12
000 rpm for 45 min at 4 °C using Amicon® Ultrafilters (MWCO 10 K). The obtained LPH NPs formulations were re-dispersed in PBS (1 mL, pH 7.4) and stored at 4 °C for further analysis. For the sake of comparison, LPH NPs loaded with azithromycin, niclosamide or piroxicam were prepared and assigned as LPHAzi, LPHNic, and LPHPir respectively. For the cellular uptake study, DiI-labelled LPH was prepared by dispersing the DiI (1% w/w of the total lipid) in the alcoholic aqueous phase, and the LPH NPs were prepared as described above. To prove the superiority of LPH NPs over their PLGA NPs counterparts, different PLGA NPs loaded with azithromycin, with or without piroxicam, were prepared by the nanoprecipitation method (ESI data†).
2.4. In vitro characterization of the prepared LPH NPs
2.4.1. Particle size, size distribution, and zeta potential. The particle size, size distribution, stated as the polydispersity index (PDI), and zeta potential of the obtained LPH NPs formulations were measured by dynamic light scattering (DLS) using a Nanosizer ZS Series (Malvern Instruments, Southborough, MA). Briefly, different LPH NPs formulations as well as their PLGA NP counterparts were diluted with deionized water (1
:
10 v/v) and moved to disposable plain folded capillary zeta cells. All the measurements were the mean of 20 runs, and each run was conducted in triplicate at 25 °C.
2.4.2. Determination of entrapment efficiency % and loading efficiency %. Azithromycin, niclosamide, and piroxicam entrapment efficiency (EE%) values were quantified directly by determining the amount of each drug in the LPH NPs or PLGA NPs. Briefly, different LPH NPs pellets were dissolved in methanol (10 mL) using a bath sonicator (Clifton™ Heated Timed Ultrasonic Bath, Fisher Scientific, UK) for 2 h to ensure the dissolution of the LPH NPs pellets. The amount of azithromycin, niclosamide, and piroxicam was quantified using the developed validated HPLC described previously.The EE% was calculated using the following equation:
|
 | (1) |
The loading efficiency (LE%) of piroxicam and azithromycin or niclosamide was calculated using the following equation
|
 | (2) |
2.4.3. Transmission electron microscopy (TEM). One drop of LPHAzi–Pir and LPHNic–Pir was placed on a carbon-coated copper 300-mesh grid and allowed to dry for 10 min. Samples were stained with one drop of 1% phosphotungstic acid for 5 min before visualization using a transmission electron microscope (TEM, Jeol, JEM-1230, Japan).
2.4.4. In vitro serum stability assay. The in vitro serum stability of both LPHAzi–Pir and LPHNic–Pir was evaluated by measuring the change in particle size, PDI, and zeta potential as well as EE% of the payloads after incubation with 10% and 50% v/v fetal bovine serum (FBS) for 4, 24, and 48 h at 37 ± 0.5 °C.43
2.4.5. In vitro hemolytic assay. The haemo-compatibility of the obtained LPHAzi–Pir and LPHNic–Pir was assessed using fresh red blood cells (RBCs) from male albino rats. The study was performed according to ethical guidelines of the Faculty of Pharmacy, University of Sadat City for animal experiments. In brief, blood was collected from male albino rats (aged 2–3 months, 200 g ± 10%) through a tail vein in a heparinized tube (1 mL from each animal). RBCs were collected by centrifugation for 10 min at 4000 rpm at 4 °C. The obtained RBCs were incubated with different concentrations of LPHAzi–Pir or LPHNic–Pir for 2 h at 37 °C. Subsequently, the samples were centrifuged under the same conditions, and the absorbance of each supernatant was determined at 545 nm.44 The hemolysis % was calculated according to the following equation, where RBCs incubated with either Triton X-100 (0.5 w/v %) or PBS (pH 7.4) were considered the positive and negative controls, respectively. |
 | (3) |
2.4.6. Determination of cellular uptake by flow cytometry. The cellular internalization of LPHAzi–Pir and LPHNic–Pir was determined using flow cytometry (BD FACS Calibur™ flow cytometer, BD Biosciences). Human lung fibroblast (CCD-19Lu) cells were seeded in a 24-well plate at a density of 50 K cells per well for 24 h. Cells were incubated with equivalent concentrations of two different drugs (azithromycin or niclosamide), namely 25 and 50 nM, for 4 h. Subsequently, cells were washed three times with PBS, trypsinized, and centrifuged at 1750 rpm for 3 min at 4 °C. The collected cells were re-suspended into 200 μL of PBS and LPH NPs uptake was quantified by measuring the fluorescence using an FL2 detector.45 Data analysis was performed using Flowjo™ software (Treestar).
2.5. In vitro cytotoxicity and antiviral activity
2.5.1. SARS-CoV-2 virus titration by TCID50. Vero-E6 cells were seeded into 96-well tissue culture plates and incubated overnight until confluency under 5% CO2 conditions in a humidified 37 °C incubator. Serial dilutions of the virus were prepared, and the cell monolayers were then washed with 1× PBS and subjected to virus adsorption for 1 h at 37 °C. The virus was then discarded and 200 μL of DMEM (sublimated with 2% antibiotic antimycotic and 4% BSA) were added. After 48–72 h incubation, the virus titer was then calculated using the Reed and Munch equation.46
2.5.2. Determination of anti-SARS-CoV-2 activity. This study was accomplished according to,8 with minor modifications. The cell monolayers were seeded into 96-well tissue culture plates and incubated overnight. 50 μL of DMEM containing varying concentrations of the tested compounds were incubated with 50 μL of 100 TCID50 of the virus for 1 h at 37 °C, then overlaid with cell monolayer and incubated at 37 °C in a 5% CO2 incubator for 72 h. Parallel to that, treated cells with the same concentrations of the tested compounds but untreated with the virus were used to determine the cytotoxicity (CC50) of the tested preparations. Cells were fixed with 4% paraformaldehyde and stained with 0.1% crystal violet (CV). After dissolving the CV stain with methanol, the optical density of the color was measured at 570 nm. The IC50 of the compound is the amount required to reduce the virus-induced cytopathic effect (CPE) by 50%, relative to the virus control. Inhibitory concentration 50% (IC50) values were calculated using the nonlinear regression analysis of GraphPad Prism software (version 5.01) by plotting log inhibitor versus normalized response (variable slope).
2.6. Statistical analysis
Three replicates were done for each experiment and the recorded results were the mean ± SD. For comparing two variables a Student's t-test was applied. For comparing different parameters between groups one-way analysis of variance (ANOVA) was applied followed by the Tukey HSD test. All the analyses were performed using SPSS 18 (Chicago, USA) and differences were considered significant at a probability (p) value < 0.05.
2.7. Molecular modelling studies
2.7.1. Structure-based study (molecular docking). The molecular docking studies were operated using the OpenEye software [Fast Rigid Exhaustive Docking (FRED) Receptor, version 2.2.5; OpenEye Scientific Software, SantaFe, NM (USA); http://www.eyesopen.com], under academic license (The Laboratory of Yaseen A. M. Mohamed Elshaier, 2021). A virtual library of target compounds was used, and their energies were minimized using the MMFF94 force field, followed by the generation of multi-conformers using the OMEGA application. The library was compiled in one file by Omega. The target proteins were retrieved from PDB and the created receptor was operated by the OeDocking application. Multiple scoring functions were engaged to predict the energy profile of the ligand–receptor complex. The Vida application was used as a visualization method to represent the pose of the ligands and the potential binding interactions of the ligands to the receptor of interest.
2.7.2. Ligand-based study (shape similarity and ROCS analysis). ROCS application OpenEye scientific software was used. The query molecules were selected based on high similarity [https://www.eyesopen.com/]. A compound library was adopted as the database file. Both query and database files were energy minimized with Omega applications. A very fast personal PC using a vROCS interface was employed in the ROCS runs. The ROCS analysis contains two files, which must be in the 3D format: (a) examined macrolide compounds as a database file, (b) standard drugs as query file. The result was visualized with the Vida application. Compound conformers were scored based upon the Gaussian overlap to the query and the best scoring parameters are Tanimoto combo scores (shape + color), where the highest score is the best match with the query compound.
2.8. MD simulation
MD simulation studies of azithromycin in complex with viral papain-like cysteine protease (PLpro, NSP3) were performed using Gromacs 41. MD simulation studies of azithromycin in complex with viral papain-like cysteine protease (PLpro, NSP3) were performed on Schrodinger's Desmond module23. The Desmond System Builder tool was employed to generate the solvated water-soaked system, the TIP3P model. An orthorhombic simulation was a box that generated at least 10 Å from the outer surface of the protein with periodic boundary conditions. A reasonable amount of counterions were added to neutralize the system, which was obtained by adding 0.15 M NaCl into the simulation panel, so the isosmotic condition was conserved. MDS was achieved at an ambient pressure of 1.013 bar, and a temperature of 300 K, with 1000 frames saved to the trajectory, for a 50 ns period. Analysis of simulation was performed using a simulation interaction diagram, including the protein–ligand root mean square deviation (RMSD), protein root mean square fluctuations (RMSF), ligand RMSF, protein–ligand contacts, ligand–protein contacts, and ligand torsion profile.
The RMSD values produced were 1.33 Å and 1.29 Å. The RMSD values are less than the X-ray resolution of the corresponding protein which are 1.68 Å, and 1.5 Å, which indicates that the generated model has a good correlation with the experimental X-ray crystal structures.
3. Results and discussion
Generally, disease treatment strategies for the most infectious require the co-administration of more than one drug.40 The co-delivery of a pharmacologically active drug into single nanoparticles could maximize therapeutic outcomes.47 This could be attributed to the ability of different nanocarriers to improve drug solubility, prolong drug circulation time, and control drug release of the payloads in the same cell with possible targeting tuning the physicochemical properties of the nanoparticles.48 In this context, we designed an LPH NPs composed of a PLGA polymeric core and a mixture of lecithin and DSPE-PEG 2000 as a lipidic core able to co-load piroxicam with either azithromycin (LPHAzi–Pir) or niclosamide (LPHNic–Pir).
3.1. HPLC determination of azithromycin, piroxicam, and niclosamide
HPLC methods were developed and validated for azithromycin, piroxicam and niclosamide quantification and determination of entrapment efficiency % and loading efficiency %.
The optimization of the chromatographic conditions involved the use of different mobile phase compositions, flow rate, and detection wavelength until the optimum conditions were reached by detecting each drug at its λmax to attain maximum sensitivity. HPLC chromatograms of azithromycin, piroxicam, and niclosamide are shown in Fig. S1–S3.†
The chromatographic method was validated as per ICH guidelines.49 Regarding linearity and range, azithromycin exhibited a linear correlation between peak area and concentration in the concentration range 80–240 μg mL−1. As for piroxicam, the linearity range was 0.5–50 μg mL−1 and for niclosamide, the range was 0.1–100 μg mL−1. Correlation coefficients were 0.9999, 0.9996, and 0.9999 for azithromycin, piroxicam, and niclosamide; respectively. All regression parameters were computed as shown in Table 1.
Table 1 Quantitative analysis and regression parameters
Compound |
Slope |
Intercept |
R |
Range (μg mL−1) |
LOD (μg mL−1) |
LOQ (μg mL−1) |
Azithromycin |
1.134 |
0.256 |
0.9999 |
80–240 |
2.02 |
6.13 |
Piroxicam |
0.112 |
0.024 |
0.9996 |
0.5–50 |
0.029 |
0.09 |
Niclosamide |
2.341 |
1.377 |
0.9999 |
0.1–100 |
0.014 |
0.05 |
The accuracy of the method was ascertained as the mean recovery % ± SD of three concentrations for each drug; niclosamide concentrations were 1, 10, and 50 μg mL−1, piroxicam concentrations 1, 10, and 20 μg mL−1, and azithromycin concentrations were 100, 140, and 200 μg mL−1. The mean recovery % ± SD were 100.63 ± 0.821, 99.75 ± 0.543, and 100.85 ± 0.732 for niclosamide, piroxicam, and azithromycin; respectively. As for the precision of the HPLC method, it was assured by the % RSD for three concentrations for intraday and interday analysis.
% RSD for niclosamide were found to be 1.53 and 1.76 for intraday and interday analysis, respectively. % RSD values were 1.03 and 1.25 for piroxicam and 1.45 and 1.89 for azithromycin intraday and interday evaluation, respectively.
3.2. In vitro characterization of the prepared LPH NPs
Tables 2 and 3 reveal that the measured particle size of the prepared LPH NPs ranged from 110.25 ± 3.54 to 126.54 ± 8.45 nm with PDI < 0.3 indicating the preparation of monodispersed systems.50 The measured particle sizes of all the prepared PLGA NPs were less than 100 nm (Table S2†). By comparing the PLGA NP sizes with their LPH NPs counterparts, a significantly higher particle size could be observed in all LPH NPs formulations (P < 0.05). This could be attributed to the presence of a lipid coat on the polymeric core in the LPH NPs formulations. The presence of lipids increases the viscosity of the preparation solution, which would hinder the breakdown of the droplets into smaller particles, opposing the shear force impact of stirring that leads to the formation of particles of larger size. Similar results were reported by Baek and colleagues.
Table 2 In vitro characterization of the prepared azithromycin and/or piroxicam-loaded LPH NPs
Formulation |
Particle sizea,e (nm) |
PDIa,e |
Surface chargeb,e (mV) |
Azithromycinc,e EE% |
Piroxicamc,e EE% |
Azithromycind,e LE% |
Piroxicamd,e LE% |
Measured by dynamic light scattering. Surface charge measured by electrophoresis. Calculated as a percentage of initial drug added, determined by HPLC. Calculated as a percentage of entrapped drug weight to the LPH NPs weight. Expressed as mean ± SD (n = 3). LPH NPs composed of PLGA (5 mg mL−1), lecithin (0.5 mg mL−1), DSPE-PEG 2000 (0.5 mg mL−1), Tween® 80 (10 mg mL−1), with the assigned drugs (0.75 mg mL−1). |
LPHAzif |
110.25 ± 3.54 |
0.25 ± 0.02 |
−15.64 ± 2.51 |
83.26 ± 4.69 |
— |
7.59 ± 0.84 |
— |
LPHPirf |
118.59 ± 4.57 |
0.18 ± 0.01 |
−17.65 ± 1.87 |
— |
69.11 ± 6.57 |
— |
5.94 ± 0.53 |
LPHAzi–Pirf |
125.32 ± 7.51 |
0.17 ± 0.02 |
−16.54 ± 2.45 |
74.23 ± 8.14 |
51.52 ± 5.45 |
6.75 ± 0.58 |
4.41 ± 0.74 |
Table 3 In vitro characterization of the prepared niclosamide and/or piroxicam-loaded LPH NPs
Formulation |
Particle sizea,e (nm) |
PDIa,e |
Surface chargeb,e (mV) |
Niclosamidec,e EE% |
Piroxicamc,e EE% |
Niclosamided,e LE% |
Piroxicamd,e LE% |
Measured by dynamic light scattering. Surface charge measured by electrophoresis. Calculated as a percentage of initial drug added, determined by HPLC. Calculated as a percentage of entrapped drug weight to the LPH weight. Expressed as mean ± SD (n = 3). LPH NPs composed of PLGA (5 mg mL−1), lecithin (0.5 mg mL−1), DSPE-PEG 2000 (0.5 mg mL−1), Tween® 80 (10 mg mL−1), with the assigned drugs (0.75 mg mL−1). |
LPHNicf |
122.54 ± 6.41 |
0.22 ± 0.02 |
−18.78 ± 2.11 |
82.11 ± 4.91 |
— |
7.11 ± 0.46 |
— |
LPHPirf |
118.59 ± 4.57 |
0.18 ± 0.01 |
−17.65 ± 1.87 |
— |
69.11 ± 6.57 |
— |
5.94 ± 0.53 |
LPHNic–Pirf |
126.54 ± 8.45 |
0.24 ± 0.03 |
−16.554 ± 3.25 |
85.14 ± 3.47 |
48.75 ± 4.77 |
6.87 ± 0.34 |
4.11 ± 0.54 |
All the proposed LPH NPs exhibited a negative surface charge expressed as a zeta potential, due to the presence of lecithin as a lipid coat layer.51 In addition, all the prepared PLGA NPs showed a significantly higher zeta potential (as an absolute value) than LPH NPs formulations (P < 0.05) (Table S2†). This may be attributed to the ability of the lipid coat to shift the shear plane of the diffusion layer to a longer distance, thus decreasing the absolute value of the zeta potential.
Interestingly, EE% values of azithromycin or niclosamide were significantly higher than that of piroxicam (P < 0.05) (Tables 2 and 3). A concomitant significantly higher LE% for azithromycin and niclosamide than for piroxicam could also be noticed (P < 0.05). Moreover, the co-loading of either azithromycin or niclosamide with piroxicam significantly reduced the EE% of the latter (P < 0.05). This could be explained by the lower partition coefficient of piroxicam (1.8) compared to the more lipophilic azithromycin and niclosamide with respective partition coefficients of 3.98 and 4.49.52–54 The EE% values of azithromycin, niclosamide and piroxicam in PLGA NPs ranged from 31.59 ± 2.25% to 65.24 ± 4.25% (Table S3†). These results were in accordance with previous reports indicating the superiority of LPH NPs EE% over their counterpart PLGA NPs EE%. This could be attributed to the presence of a lipid coat on the polymeric core that could serve as a physical barrier that prevents drug leakage finally, the direct effect of particle size on entrapment efficiency % should not be overlooked. The higher particle size, attained with LPH NPs over polymeric NPs, procures a longer diffusion path for the drug, enhancing its encapsulation.
Table 4 Selectivity index (SI) for the tested formulated and native drug mixtures
Drug mixture |
Formula |
CC50 |
IC50 |
Selectivity index (CC50/IC50) |
Azithromycin/piroxicam |
Native (equal molar proportions) |
249.4 |
3.163 |
78.85 |
LPHAzi+Pir (piroxicam) |
23.73 |
1.798 |
13.2 |
LPHAzi+Pir (azithromycin) |
15.21 |
1.216 |
12.5 |
Niclosamide/piroxicam |
Native (equal molar proportions) |
43.1 |
1.861 |
23.2 |
LPHNic+Pir (piroxicam) |
28.38 |
0.82 |
34.6 |
LPHNic+Pir (niclosamide) |
62.84 |
1.49 |
42.2 |
3.3. Morphological characterization
In TEM micrographs, LPHAzi–Pir and LPHNic–Pir appeared as spherical non-aggregated nanostructures with a core–shell particle, where the PLGA core appeared as a white core surrounded by a gray lipid shell (Fig. 2A and B). Both LPHAzi–Pir and LPHNic–Pir had particle sizes of 120–130 nm, which is consistent with the range obtained by the dynamic light scattering technique.
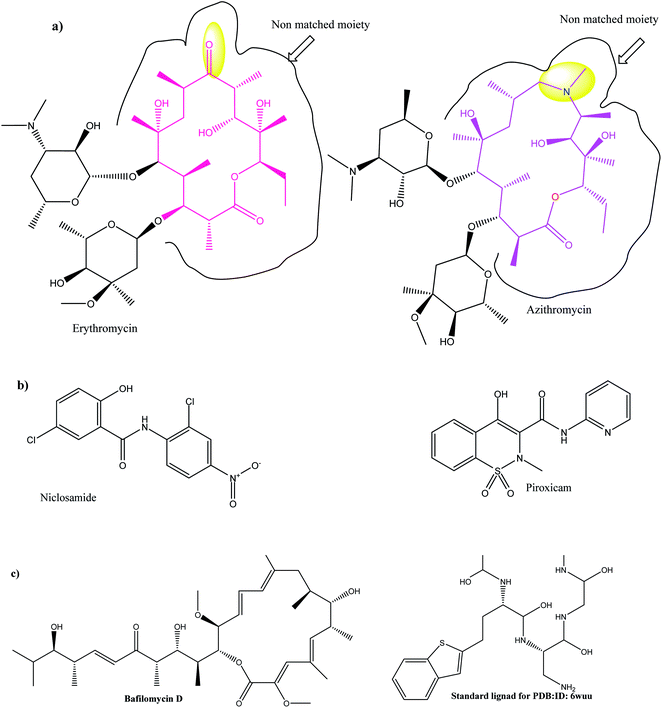 |
| Fig. 1 (a) 2D topology alignment of the macrolides azithromycin and erythromycin; (b) niclosamide and piroxicam as anti-SARS-CoV-2 drugs; (c) bafilomycin D (left) and co-crystalized ligand of PDB: ID:6wuu (right). | |
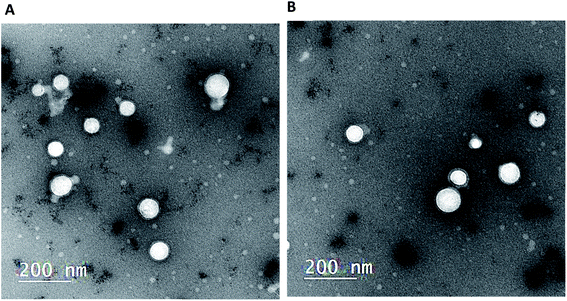 |
| Fig. 2 Transmission electron micrograph of the prepared LPH NPs. Both LPHAzi–Pir (A) and LPHNic–Pir (B) appeared as a core–shell structure with particle size 120–130 which agreed with the size obtained by dynamic light scattering. | |
3.4. In vitro serum stability of the proposed LPH NPs systems
The stability of both LPHAzi–Pir and LPHNic–Pir dispersions was assessed in the presence of FBS (10% and 50% v/v) at 37 °C for 4, 24, and 48 h. Fig. 3 and 4 show insignificant changes in particle size, PDI, zeta potential as well as, EE% for azithromycin, niclosamide and piroxicam after incubation with serum (P > 0.05). The negatively charged LPH NPs provides an electrostatic repulsion, with serum protein preventing any significant change in LPH NPs assigned properties.43,55
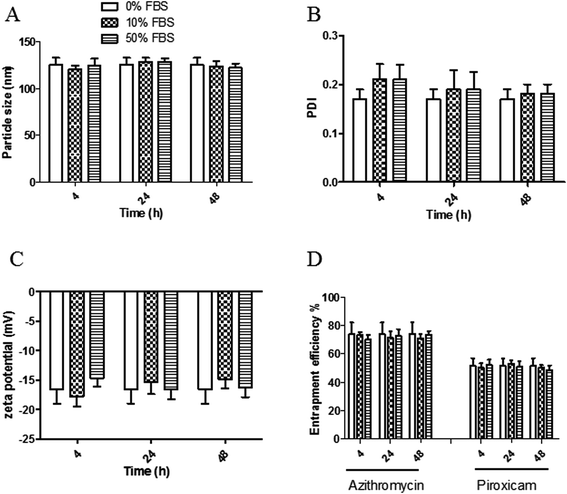 |
| Fig. 3 In vitro serum stability of the prepared LPHAzi–Pir. The obtained LPHAzi–Pir was incubated with 0%, 10%, and 50% FBS for 4, 24, and 48 h; then particle size (A), PDI (B), zeta potential (C) and entrapment efficiency % (D) were measured using DLS as described. Data points represent mean and SD (n = 3). Statistical analysis was carried out using one-way ANOVA followed by a Tukey HSD test and P < 0.05 was considered significant. Serum protein had a neglible effect on LPHAzi–Pir properties. | |
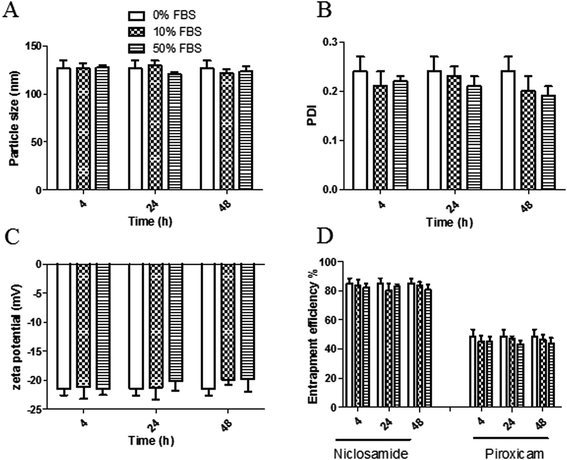 |
| Fig. 4 In vitro serum stability of the prepared LPHNic–Pir. The obtained LPHNic–Pir was incubated with 0%, 10%, and 50% FBS for 4, 24, and 48 h; then particle size (A), PDI (B), zeta potential (C) and entrapment efficiency % (EE%) were measured using DLS as described. Data points represent mean and SD (n = 3). Statistical analysis was carried out using one-way ANOVA followed by a Tukey HSD test and P < 0.05 was considered significant. Serum protein had an insignificant effect on LPHNic–Pir particle size, PDI, or zeta potential. | |
3.5. In vitro hemolytic assay
The hemolytic activity of the fabricated LPHAzi–Pir and LPHNic–Pir was studied as an indicator of the biocompatibility of the proposed systems.45 The calculated hemolysis in both formulations, illustrated in Fig. 5, was less than 5% at all tested concentrations, which is in accordance with the accepted limit of the nanoparticle hemolysis threshold.56 By virtue of their components PLGA and DSPE-PEG 2000 which are approved by FDA, as well as lecithin which is a natural lipid, the fabricated LPH NPs systems could be considered biocompatible delivery systems.57
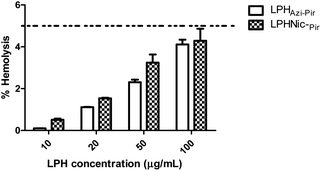 |
| Fig. 5 The in vitro hemolysis assay of the proposed LPH NPs systems. Rat RBCs were incubated with either LPHAzi–Pir or LPHNic–Pir at different FH concentrations (10–100 μg mL−1) for 2 h at 37 °C. Positive and negative controls were 0.5% w/v Triton X-100 and PBS (pH 7.4), respectively. Samples were centrifuged at 4000 rpm for 5 min at 4 °C and the absorbance of the released hemoglobin was determined at 545 nm. The data point represents the mean and SD (n = 3). The dotted line represents the acceptable hemolysis range. | |
3.6. In vitro cellular uptake
The cellular uptake of both DiI fluorescence-labeled LPHAzi–Pir and LPHNic–Pir both at azithromycin or niclosamide equivalent concentrations of 25 and 50 nM was quantified after 4 h of incubation into human lung fibroblast (CCD-19Lu) cells. Fig. 6A and B show representative histograms of LPHAzi–Pir and LPHNic–Pir cellular internalization. As depicted in Fig. 6C, a dose-dependent increase in cellular uptake of both LPHAzi–Pir and LPHNic–Pir with increasing concentration from 25 to 50 nM significantly increased the mean fluorescence intensity by 1.6–1.7 fold (P < 0.05). These results follow previous reports which mentioned the positive effect of concentration on cellular uptake.58,59
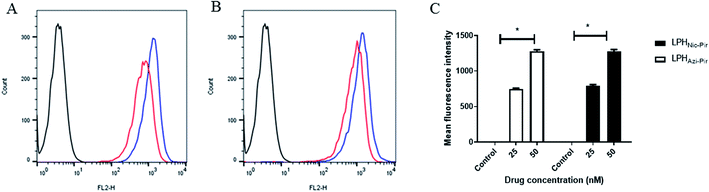 |
| Fig. 6 In vitro cellular uptake of the prepared LPH NPs systems in human lung fibroblast (CCD-19Lu) cells. Cells were incubated with either LPHAzi–Pir or LPHNic–Pir at 25 (red) or 50 nM (blue) for 4 h. Flow cytometry histogram for uptake of LPHAzi–Pir (A) or LPHNic–Pir (B). Cellular uptake was quantified by mean fluorescence intensity using flow cytometry and an FL-2 detector (C). LPH NPs uptake was dose dependent. Results are expressed as mean ± SD (n = 3). | |
3.7. In vitro cytotoxicity and antiviral activity
Previous studies showed the individual potency of azithromycin, niclosamide, and piroxicam against SARS-CoV-2 in vitro and in vivo.8 However, studies on the possible synergistic effect of combining these drugs have not yet been undertaken. To identify the suitable concentrations to define the antiviral activity of the tested drug combinations, a half-maximal cytotoxic concentration “CC50” was calculated for the drug combination in LPH NPs against the solution (Table 4 and Fig. 7). The respective calculated IC50 for azithromycin and piroxicam in LPH NPs were 1.216 and 1.798 μM compared to 3.163 μM for each drug solution. Moreover, the piroxicam LPHNic–Pir IC50 was 1.489 and 0.82 μM for niclosamide and piroxicam, respectively. In contrast, the niclosamide–piroxicam solution revealed a higher IC50 for both drugs (1.861 μM). Antiviral screening revealed that the LPH NPs for the tested azithromycin/piroxicam (Fig. 7A) and niclosamide/piroxicam (Fig. 7B) drug mixtures exhibit better in vitro activity against SARS-CoV-2 “NRC-03-nhCoV” compared with the corresponding drug solutions (Fig. 7).
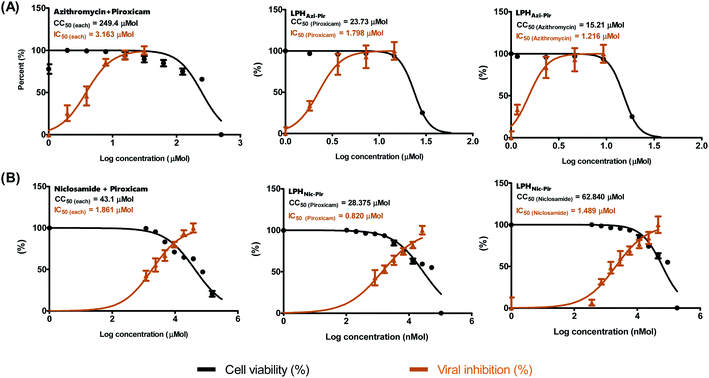 |
| Fig. 7 Anti-SARS-CoV-2 activities of tested drugs in LPH NPs. Half-maximal cytotoxic concentrations (CC50) on Vero E6 cells and half-maximal inhibitory concentrations (IC50) against NRC-03-nhCoV in Vero E6 were performed for (A) azithromycin and piroxicam combinations in LPH NPs and drug mixture solution, and (B) niclosamide and piroxicam combinations in LPH NPs and drug mixture solution. The CC50 and IC50 values were calculated using the nonlinear regression analysis of GraphPad Prism software (version 5.01) by plotting log inhibitor versus normalized response (variable slope). | |
3.8. Molecular modelling
3.8.1. Molecular docking study. This was operated using the OpenEye Scientific software60,61 (academic license 2021). The outputs ranked and sorted the compounds based on their consensus score values. The lower the consensus score value of a compound the stronger its binding to the receptor (Table S4†).Among the SARS-CoV-2 proteins, the papain-like protease (PLpro, nsp3 domain) shows increasing importance in SARS-CoV-2 drug ability.62
In the current work, viral papain-like cysteine protease (PLpro, NSP3) was selected as a target protein because it represents a promising target for the development of antiviral drugs (PDB: ID:6wuu63) against SARS-CoV-2. To validate our study, the docked co-crystallized ligand exhibited bonding interaction very similar to the reported co-crystallized complex.63 It formed two HBs with Gly:163A and Asp:164A through the terminal peptide moiety, while the remaining structure of this ligand occupied the receptor through hydrophobic–hydrophobic interaction (Fig. 8A). Azithromycin occupied the receptor with the formation of different binding interactions, especially HBs with Thr:301A, Arg:166A, and Glu:167:A (Fig. 8B). In an analysis of the quaternary structure of the receptor, Glu:176A was adopted very close to Gly:163A in the same cleft. In a comparison of azithromycin with erythromycin, erythromycin illustrated binding to the receptor with Asp:164A alone. It was prevented from interaction with the key amino acids Gly:163A-, Asp:164A-, and Arg:166-Glu:167A. Niclosamide displayed HB with Tyr:273A. The anilide moiety adopted strong interaction with the clefts containing the peptides Lu:162- and A-Arg:166A-Gly:163A and Met:208A-Gln:269A (Fig. 8C). Among the macrolide bioactive compounds, bafilomycin D showed the strongest binding score to the receptor (consensus score 9, Table S4†). It showed a high overlay on the standard ligand with hydrophobic–hydrophobic interaction (Fig. S3†).
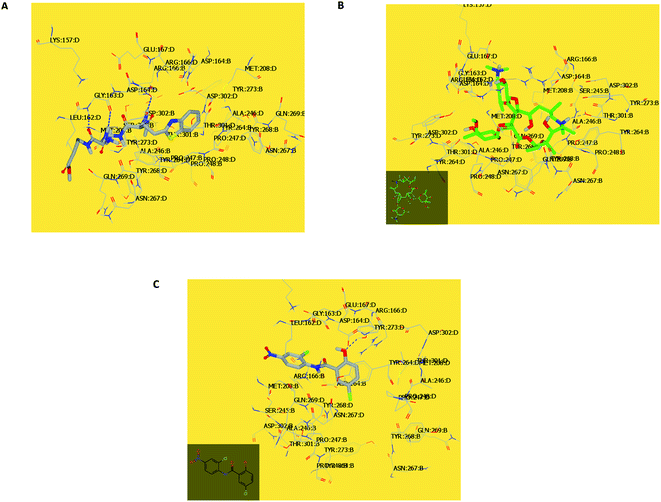 |
| Fig. 8 (A) Standard ligand co-crystallized with PEDB: ID:6wuu; (B) azithromycin docked with PDB: ID:6wuu; (C) niclosamide docked with PDB: ID:6wuu. | |
3.8.2. Ligand-based study (shape alignment and rapid overlay chemical structure (ROCS)). Rapid overlay chemical structure (ROCS) is a chemoinformatic technique directed at predicting the similarities between the three-dimensional structures of chemical compounds. High similarity in the shape of the compounds reproduces high similarity in their biology, whereas high similarity in biology is not mirrored in similarities in the structures.ROCS is implemented in different drug discovery and development applications, e.g., lead-hopping, molecular alignment, as well as structural predictions. This work aims to identify macrolide molecules (data set file) that are as extraordinary as azithromycin (the query file) as a reported drug prescribed in COVID-19 management.64 Upon the generated results, we can identify the best macrolides to be prioritized for further biological studies.
Herein, we focused on the output “Tanimoto scores” which include Tanimoto Combo (TC), Shape Tanimoto (ST), and Colour Tanimoto (CT). The TC score is the summation of both ST and CT. It had a value between 0 and 2 and was the score used for ranking the hit list (Table 5). According to the generated study, the macrolides erythromycin, saccharthriolide B, tedanolide C, and saccharthriolide showed the highest TC scores compared to azithromycin (Table 6). In this regard, the shape alignment of azithromycin and erythromycin using the vROCS tool was conducted. From Fig. 9A and 1a, we can see that the macrocyclic moieties in both drugs were not overlaid and matched while the sugar parts matched very well. It is very clear from a previous docking study that the amino functionality in this ring participated in an essential interaction with key amino acids.
Table 5 Tanimoto scores for macrolide compounds to azithromycin drug recommended in COVID-19 disease generated by vROCS application
Name |
Tanimoto combo |
Shape Tanimoto |
Color Tanimoto |
Azithromycin |
0, 2.0000 |
1.0000 |
1.0000 |
Erythromycin |
0.7470 |
0.4390 |
0.3080 |
Saccharthriolide B |
0.6150 |
0.4900 |
0.1260 |
Tedanolide C |
0.6040 |
0.4510 |
0.1530 |
Saccharthriolide |
0.6010 |
0.4760 |
0.1250 |
Bromophycolide |
0.5980 |
0.4790 |
0.1190 |
α-Ketoamide |
0.5810 |
0.4840 |
0.0970 |
Bromophycolide |
0.5720 |
0.4750 |
0.0970 |
Bafilomycin D |
0.5700 |
0.4460 |
0.1230 |
Bafilomycin |
0.5670 |
0.4510 |
0.1160 |
Umifenovir |
0.5600 |
0.4800 |
0.0800 |
JBIR |
0.5590 |
0.4390 |
0.1200 |
Erthronolide |
0.5560 |
0.4020 |
0.1530 |
Chalcomycin |
0.5520 |
0.4020 |
0.1500 |
Remdesivir |
0.5490 |
0.4100 |
0.1400 |
GW479439 |
0.5470 |
0.4060 |
0.1410 |
Bromophycolide |
0.5360 |
0.4110 |
0.1250 |
Supertoside A |
0.5320 |
0.4240 |
0.1080 |
Doxycycline |
0.5290 |
0.4180 |
0.1110 |
Chalcomycin A |
0.5270 |
0.3750 |
0.1520 |
Akaemycin |
0.5260 |
0.3790 |
0.1460 |
Bromophycolide |
0.5160 |
0.3800 |
0.1370 |
Standard ligand |
0.4880 |
0.0690 |
0.5640 |
N3 |
0.4080 |
0.4080 |
0.1070 |
Spike ligand |
0.5130 |
0.3990 |
0.1140 |
Saccharothriolide C |
0.5130 |
0.3830 |
0.1300 |
Macrophelide |
0.4920 |
0.3790 |
0.1130 |
PC786 |
0.4820 |
0.3860 |
0.0970 |
Seimatopolide_ |
0.4820 |
0.3460 |
0.1360 |
Aspergillide |
0.4730 |
0.3470 |
0.1260 |
Supertolide |
0.4710 |
0.4090 |
0.0620 |
Bafilomycin B1 |
0.4650 |
0.3400 |
0.1250 |
Nitazoxanide |
0.4550 |
0.3370 |
0.1180 |
Niclosamide |
0.4550 |
0.3310 |
0.1240 |
Halichondramide |
0.4450 |
0.3050 |
0.1400 |
Seimatopolide B |
0.4400 |
0.3520 |
0.0880 |
Modiolide D |
0.4090 |
0.3100 |
0.0990 |
Modiolide E |
0.4030 |
0.2780 |
0.1250 |
Balticolid |
0.3980 |
0.2850 |
0.1130 |
Modiolide |
0.3870 |
0.2090 |
0.1780 |
Table 6 Tanimoto scores for macrolides compounds to standard ligand generated by vROCS application
Name |
Tanimoto combo |
Shape Tanimoto |
Color Tanimoto |
Standard ligand (ID:6WUU) |
2 |
1.0000 |
1.0000 |
Umifenovir |
0.63 |
0.5140 |
0.1160 |
Nitazoxanide |
0.623 |
0.4630 |
0.1590 |
Sofosbuvir |
0.618 |
0.4940 |
0.1240 |
Chalcomycin A |
0.591 |
0.4820 |
0.1090 |
Bafilomycin D |
0.587 |
0.5070 |
0.0800 |
Seimatopolide |
0.584 |
0.4920 |
0.0920 |
N3_64 |
0.57 |
0.4120 |
0.1580 |
α-Ketoamide |
0.563 |
0.4300 |
0.1330 |
Supertolide |
0.558 |
0.4320 |
0.1260 |
Supertoside A |
0.554 |
0.4520 |
0.1010 |
Chalcomycin |
0.55 |
0.4530 |
0.0970 |
Remdesivir |
0.542 |
0.4740 |
0.0670 |
Seimatopolide B |
0.532 |
0.3860 |
0.1460 |
Bafilomycin |
0.525 |
0.4560 |
0.0690 |
Saccharothriolide C |
0.504 |
0.3790 |
0.1250 |
Saccharthriolide B |
0.503 |
0.4180 |
0.0850 |
Bromophyycolide |
0.502 |
0.3770 |
0.1250 |
Spike ligand |
0.496 |
0.4000 |
0.0960 |
JBIR |
0.484 |
0.4000 |
0.0840 |
Niclosamide |
0.475 |
0.3800 |
0.0950 |
Saccharthriolide |
0.474 |
0.3860 |
0.0880 |
PC786 |
0.473 |
0.3980 |
0.0750 |
GW479439x |
0.464 |
0.3170 |
0.1480 |
Modiolide D |
0.457 |
0.3320 |
0.1250 |
Modiolide E |
0.456 |
0.3300 |
0.1250 |
Balticolid |
0.445 |
0.2610 |
0.1840 |
Bafilomycin B1 |
0.442 |
0.3790 |
0.0630 |
Macrophelide |
0.439 |
0.3380 |
0.1010 |
Akaemycin |
0.439 |
0.3270 |
0.1110 |
Erthronolide B |
0.438 |
0.3170 |
0.1210 |
Halichondramide |
0.437 |
0.3090 |
0.1270 |
Bromophycolide |
0.436 |
0.3050 |
0.1310 |
Bromophycolide |
0.435 |
0.3430 |
0.0920 |
Aspergillide |
0.432 |
0.3270 |
0.3270 |
Doxycycline |
0.43 |
0.3600 |
0.0700 |
Tedanolide C |
0.412 |
0.3280 |
0.0850 |
Erythromycin |
0.391 |
0.2790 |
0.1120 |
Azithromycin |
0.384 |
0.2780 |
0.1070 |
Bromophycolide |
0.382 |
0.3420 |
0.0400 |
Modiolide |
0.371 |
0.2660 |
0.1050 |
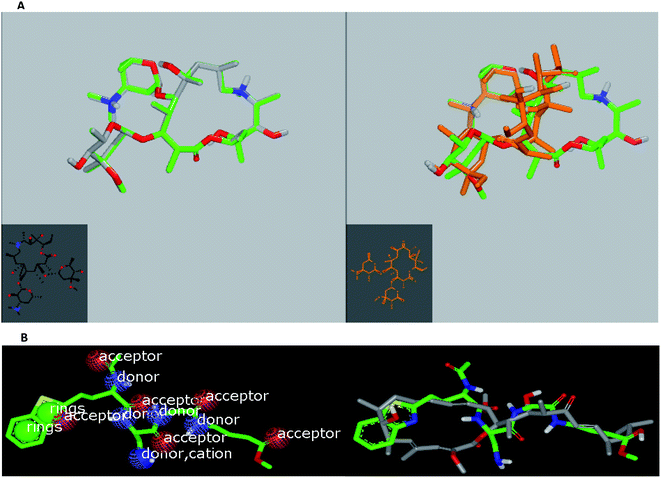 |
| Fig. 9 (A) Left: molecular topology of azithromycin; right: azithromycin (green) alignment with erythromycin (brown). (B) Left: shape and volume color of standard ligand and right: overlay of bafilomycin D with standard ligand. | |
ROCS analysis for these macrolides against the standard ligand showed that chalcomycin A, bafilomycin D, and seimatopolide showed the highest scores, which were more than those reported for some anti-SARS-CoV-2 drugs (Table 6).
With the results obtained from docking and ROCS studies, we can expect the macrolides bafilomycin D and bafilomycin to be subjected to further biological and semi-synthetic studies.
3.8.3. Molecular dynamic simulation (MDS). MDS studies were implemented to define the time-dependent behavior of the target protein upon administration of azithromycin. Root mean square deviation (RMSD), root mean square fluctuation (RMSF) and protein–ligand interactions were analyzed to estimate the stability of the simulated complexes.65,66
3.8.3.1. MDS of viral papain-like cysteine protease (PLpro, NSP3). In the RMSD plot of azithromycin in a complex with PLpro, NSP3 showed that the protein remains significantly stable upon binding with azithromycin throughout the entire simulation period (Fig. 10). The protein RMSD trajectory of viral papain-like cysteine protease (PLpro, NSP3) SARS-CoV-2 (PDB: ID:6wuu) shows stability from 0 to 35 ns, then tends to increase with gradual fluctuations (Fig. 10).
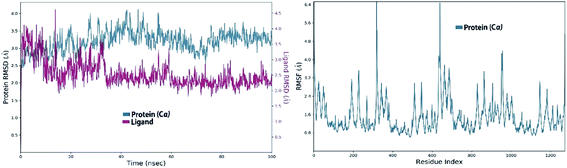 |
| Fig. 10 Left: RMSD of viral papain-like cysteine protease (PLpro, NSP3) in complex with azithromycin; and right: RMSF of viral papain-like cysteine protease (PLpro, NSP3). | |
An azithromycin property study consisting of ligand RMSD, the radius of gyration (rGyr), molecular surface area (MolSA), solvent accessible surface area (SASA), and polar surface area (PSA) is shown in Fig. 11. The RMSD of the ligand displayed no fluctuation during the ns simulation time (ns). Lastly, all the azithromycin properties illustrate equilibrium during most of the simulation time, which imitates the stability of azithromycin in the active site of the protein.
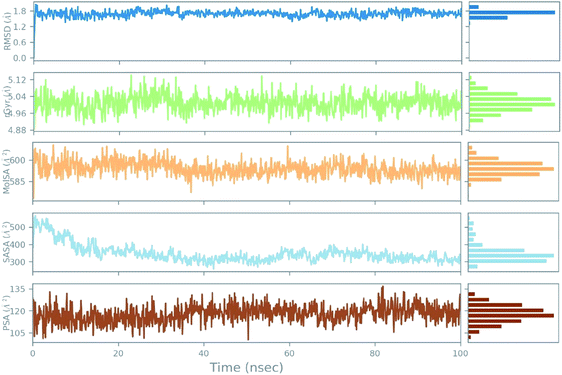 |
| Fig. 11 The ligand property trajectory of the azithromycin-6wuu complex during the 100 ns simulation. | |
The stable ligand–protein complex was examined using protein–ligand contact histograms which are categorized as HB, hydrophobic, ionic, or water bridges, as illustrated in Fig. 12.
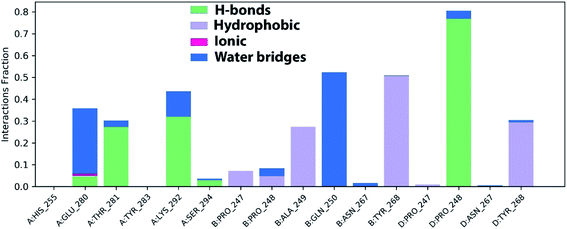 |
| Fig. 12 Histogram of PLpro, NSP3-azithromycin contact throughout the trajectory. | |
Then, the previous histogram was represented in the two timeline panels in Fig. 13. In the upper part of Fig. 13, the panel displays the total number of SARS-CoV-2 protein contacts with azithromycin in each trajectory frame. The number of contacts fluctuates from zero to nine over the trajectory course. The contribution of amino acids in each trajectory frame of 100 ns MD simulation is represented in the lower part of Fig. 13. This shows which amino acid residues interact with the ligand in each trajectory frame. Some residues undergo higher interaction (darker orange shading) than other amino acids.
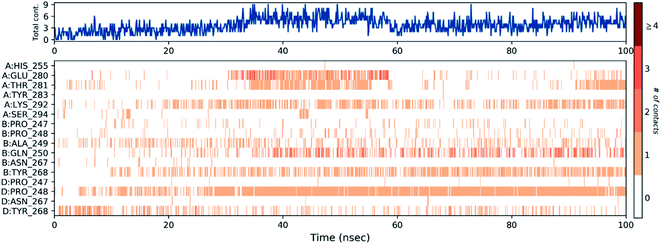 |
| Fig. 13 The total number of contacts/interactions in each trajectory frame of PLpro, NSP3-azithromycin; the interaction shown by the active site amino acids in each trajectory frame of the complex. | |
4. Conclusions
The development of new ways to fight COVID-19 with tuneable attributes, achieving an optimal safety profile, and avoiding unwanted adverse responses is highly warranted. Based on their great promise, LPH NPs were successfully fabricated as a platform for the combinatory delivery of azithromycin or niclosamide with piroxicam. The obtained nanosystems had a particle size of ≈125 nm with a relatively high EE% for the assigned drugs. Dose-dependent cellular internalization was depicted for both LPHAzi–Pir and LPHNic–Pir in CCD-19Lu human lung fibroblasts. The in vitro antiviral activity of both LPHAzi–Pir and LPHNic–Pir showed superior efficacy over individual drug solutions. The obtained results suggest the encouraging capability of LPH NPs as a delivery system for repurposed drugs for combatting the COVID-19 pandemic. Chemoinformatic and MDS analyses for targeted drugs were also addressed, which will open an avenue for further applications in this urgent pandemic. Our findings could be very promising for subjecting the aforementioned LPH NPs formulations to more in vitro and in vivo studies targeting COVID-19 patients.
Conflicts of interest
There are no conflicts to declare.
Acknowledgements
The paper was based on work supported by the University of Sadat City (USC) under grant 15/2020. Dr Yaseen A. M. M. Elshaier acknowledges OpenEye scientific software for providing the academic license. All animal procedures were performed in accordance with the Guidelines for Care and Use of Laboratory Animals of “National Research Centre (NRC)” and experiments were approved by the “Medical Research Ethics Committee (MREC)” of the NRC under permission code: 19-083.
References
- A. D. Brunaugh, et al., Development and evaluation of inhalable composite niclosamide-lysozyme particles: A broad-spectrum, patient-adaptable treatment for coronavirus infections and sequalae, PLoS One, 2021, 16(2), e0246803 CrossRef CAS PubMed.
- M. Patki, et al., Self-injectable extended release formulation of Remdesivir (SelfExRem): A potential formulation alternative for COVID-19 treatment, Int. J. Pharm., 2021, 597, 120329 CrossRef CAS PubMed.
- S. M. Kissler, et al., Projecting the transmission dynamics of SARS-CoV-2 through the postpandemic period, Science, 2020, 368(6493), 860–868 CrossRef CAS PubMed.
- J. S. Morse, et al., Learning from the Past: Possible Urgent Prevention and Treatment Options for Severe Acute Respiratory Infections Caused by 2019-nCoV, ChemBioChem, 2020, 21(5), 730–738 CrossRef CAS PubMed.
- S. Pushpakom, et al., Drug repurposing: progress, challenges and recommendations, Nat. Rev. Drug Discovery, 2019, 18(1), 41–58 CrossRef CAS PubMed.
- T. T. Ashburn and K. B. Thor, Drug repositioning: identifying and developing new uses for existing drugs, Nat. Rev. Drug Discovery, 2004, 3(8), 673–683 CrossRef CAS PubMed.
- D. B. Mahmoud, Z. Shitu and A. Mostafa, Drug repurposing of nitazoxanide: can it be an effective therapy for COVID-19?, J. Genet. Eng. Biotechnol., 2020, 18(1), 35 CrossRef PubMed.
- A. Mostafa, et al., FDA-Approved Drugs with Potent In Vitro Antiviral Activity against Severe Acute Respiratory Syndrome Coronavirus 2, Pharmaceuticals, 2020, 13(12), 443 CrossRef CAS.
- E. Rubinstein and I. Levy, Macrolides as first line therapy in adult lower respiratory tract infections: pros and cons, Clin. Microbiol. Infect., 1996, 1, 2S23–2S26 CrossRef CAS.
- M. Azhdarzadeh, et al., Anti-bacterial performance of azithromycin nanoparticles as colloidal drug delivery system against different gram-negative and gram-positive bacteria, Adv. Pharm. Bull., 2012, 2(1), 17–24 Search PubMed.
- D. Echeverría-Esnal, et al., Azithromycin in the treatment of COVID-19: a review, Expert Rev. Anti-Infect. Ther., 2021, 19(2), 147–163 CrossRef PubMed.
- K. Kunzelmann, Getting hands on a drug for Covid-19: Inhaled and Intranasal Niclosamide, The Lancet Regional Health - Europe, 2021, 4, 100094 CrossRef.
- R. Rajamuthiah, et al., Repurposing Salicylanilide Anthelmintic Drugs to Combat Drug Resistant Staphylococcus aureus, PLoS One, 2015, 10(4), e0124595 CrossRef PubMed.
- A. Astani, U. Albrecht and P. Schnitzler, Piroxicam inhibits herpes simplex virus type 1 infection in vitro, Pharmazie, 2015, 70(5), 331–336 CAS.
- M. J. Mitchell, et al., Engineering precision nanoparticles for drug delivery, Nat. Rev. Drug Discovery, 2021, 20(2), 101–124 CrossRef CAS PubMed.
- C. L. Ventola, Progress in Nanomedicine: Approved and Investigational Nanodrugs, P T, 2017, 42(12), 742–755 Search PubMed.
- N. Tahir, et al., Development and optimization of methotrexate-loaded lipid-polymer hybrid nanoparticles for controlled drug delivery applications, Int. J. Pharm., 2017, 533(1), 156–168 CrossRef CAS PubMed.
- K. Thanki, et al., Engineering of small interfering RNA-loaded lipidoid-poly(DL-lactic-co-glycolic acid) hybrid nanoparticles for highly efficient and safe gene silencing: A quality by design-based approach, Eur. J. Pharm. Biopharm., 2017, 120, 22–33 CrossRef CAS PubMed.
- D. Dehaini, et al., Ultra-small lipid–polymer hybrid nanoparticles for tumor-penetrating drug delivery, Nanoscale, 2016, 8(30), 14411–14419 RSC.
- K. Hadinoto, A. Sundaresan and W. S. Cheow, Lipid–polymer hybrid nanoparticles as a new generation therapeutic delivery platform: A review, Eur. J. Pharm. Biopharm., 2013, 85(3, part A), 427–443 CrossRef CAS PubMed.
- Z. Chaudhary, et al., Lipid polymer hybrid carrier systems for cancer targeting: a review, Int. J. Polym. Mater. Polym. Biomater., 2018, 67(2), 86–100 CrossRef CAS.
- A. Mukherjee, et al., Lipid-polymer hybrid nanoparticles as a next-generation drug delivery platform: state of the art, emerging technologies, and perspectives, Int. J. Nanomed., 2019, 14, 1937–1952 CrossRef CAS PubMed.
- A. Mostafa, et al., FDA-Approved Drugs with Potent In Vitro Antiviral Activity against Severe Acute Respiratory Syndrome Coronavirus 2, Pharmaceuticals, 2020, 13(12), 443 CrossRef CAS PubMed.
- K. Anjum, et al., Marine Sponges as a Drug Treasure, Biomol. Ther., 2016, 24(4), 347–362 CrossRef CAS.
- R. N. Asolkar, et al., Chalcomycin B, a new macrolide antibiotic from the marine isolate
Streptomyces sp. B7064, J. Antibiot., 2002, 55(10), 893–898 CrossRef CAS.
- J. Bao, et al., A New Macrolide from a Marine-derived Fungus Aspergillus sp, Nat. Prod. Commun., 2013, 8(8), 1934578X1300800825 CrossRef.
- B. Wilkinson, G. Foster, B. A. M. Rudd, N. L. Taylor, A. P. Blackaby, P. J. Sidebottom, D. J. Cooper, M. J. Dawson, A. D. Buss, S. Gaisser, I. U. Böhm, C. J. Rowe, J. Cortés, P. F. Leadlay and J. Staunton, Novel octaketide macrolides related to 6-deoxyerythronolide B provide evidence for iterative operation of the erythromycin polyketide synthase, Chem. Biol., 2000, 7(2), 111–117 CrossRef CAS.
- C. Chevallier, et al., Tedanolide C: A Potent New 18-Membered-Ring Cytotoxic Macrolide Isolated from the Papua New Guinea Marine Sponge Ircinia sp, J. Org. Chem., 2006, 71(6), 2510–2513 CrossRef CAS.
- Z. T. Dame and P. Ruanpanun, Production of macrolide antibiotics from a cytotoxic soil Streptomyces sp. strain ZDB, World J. Microbiol. Biotechnol., 2017, 33(7), 139 CrossRef PubMed.
- M. V. D'Auria, et al., A Novel Cytotoxic Macrolide, Superstolide B, Related to Superstolide A, from the New Caledonian Marine Sponge Neosiphonia superstes, J. Nat. Prod., 1994, 57(11), 1595–1597 CrossRef PubMed.
- N. T. Hiep, et al., Polyhydroxylated macrolides from Seimatosporium discosioides and their effects on the activation of peroxisome proliferator-activated receptor gamma, J. Nat. Prod., 2012, 75(4), 784–788 CrossRef PubMed.
- T. Hosoe, et al., A new antifungal macrolide, eushearilide, isolated from Eupenicillium shearii, J. Antibiot., 2006, 59(9), 597–600 CrossRef CAS.
- S. Kodani, et al., Isolation and structural determination of a new macrolide, makinolide, from the newly isolated Streptomyces sp. MK-30, J. Antibiot., 2012, 65(6), 331–334 CrossRef CAS PubMed.
- Y.-H. Liu, P. B. Shinde, J.-K. Hong, C.-O. Lee, K.-S. Im and J.-H. Jung, Trisoxazole Macrolide from a Marine Sponge Sarcotragus Species, Nat. Prod. Sci., 2005, 11(1), 50–53 CAS.
- S. Lu, et al., Saccharothriolides A–C, novel phenyl-substituted 10-membered macrolides isolated from a rare actinomycete Saccharothrix sp, Chem. Commun., 2015, 51(38), 8074–8077 RSC.
- S. E. Rossiter, M. H. Fletcher and W. M. Wuest, Natural Products as Platforms To Overcome Antibiotic Resistance, Chem. Rev., 2017, 117(19), 12415–12474 CrossRef CAS PubMed.
- M. A. M. Shushni, et al., Balticolid: a new 12-membered macrolide with antiviral activity from an ascomycetous fungus of marine origin, Mar. Drugs, 2011, 9(5), 844–851 CrossRef CAS PubMed.
- M. R. d. A. Weslei Bruno Botero, I. Z. Carlos, M. C. Polesib and L. C. dos Santos, Aromatic Polyketides and Macrolides from Microsphaeropsis arundinis, J. Braz. Chem. Soc., 2020, 31(2), 364–369 Search PubMed.
- N. T. M. P. M. R. A. H. J. M. Whipps, Antimicrobial activity of Coniothyrium minitans and its macrolide antibiotic macrosphelide A, J. Appl. Microbiol., 2009, 106, 2048–2056 CrossRef PubMed.
- J. Woodcock, J. P. Griffin and R. E. Behrman, Development of novel combination therapies, N. Engl. J. Med., 2011, 364(11), 985–987 CrossRef CAS.
- H. Zhang, et al., Marine-Derived Macrolides 1990–2020: An Overview of Chemical and Biological Diversity, Mar. Drugs, 2021, 19(4), 180 CrossRef CAS PubMed.
- H. M. Abdel-Bar, et al., Combinatory Delivery of Etoposide and siCD47 in a Lipid Polymer Hybrid Delays Lung Tumor Growth in an Experimental Melanoma Lung Metastatic Model, Adv. Healthcare Mater., 2021, 10(7), 2001853 CrossRef CAS PubMed.
- X. Zhao, et al., Co-delivery of HIF1α siRNA and gemcitabine via biocompatible lipid-polymer hybrid nanoparticles for effective treatment of pancreatic cancer, Biomaterials, 2015, 46, 13–25 CrossRef CAS PubMed.
- R. A. H. Ishak, N. M. Mostafa and A. O. Kamel, Stealth lipid polymer hybrid nanoparticles loaded with rutin for effective brain delivery – comparative study with the gold standard (Tween 80): optimization, characterization and biodistribution, Drug Delivery, 2017, 24(1), 1874–1890 CrossRef CAS.
- M. Hamdi, et al., An integrated vitamin E-coated polymer hybrid nanoplatform: A lucrative option for an enhanced in vitro macrophage retention for an anti-hepatitis B therapeutic prospect, PLoS One, 2020, 15(1), e0227231 CrossRef CAS PubMed.
- L. J. Reed and H. Muench, A simple method of estimating fifty per cent endpoints12, Am. J. Epidemiol., 1938, 27(3), 493–497 CrossRef.
- L. Ma, M. Kohli and A. Smith, Nanoparticles for combination drug therapy, ACS Nano, 2013, 7(11), 9518–9525 CrossRef CAS PubMed.
- A. Z. Wang, R. Langer and O. C. Farokhzad, Nanoparticle delivery of cancer drugs, Annu. Rev. Med., 2012, 63, 185–198 CrossRef CAS.
- P. Borman and D. Elder, Q2(R1) Validation of Analytical Procedures, in ICH Quality Guidelines, 2017, pp. 127–166 Search PubMed.
- M. Danaei, et al., Impact of Particle Size and Polydispersity Index on the Clinical Applications of Lipidic Nanocarrier Systems, Pharmaceutics, 2018, 10(2), 57 CrossRef.
- N. Tahir, et al., Lipid-polymer hybrid nanoparticles for controlled delivery of hydrophilic and lipophilic doxorubicin for breast cancer therapy, Int. J. Nanomed., 2019, 14, 4961–4974 CrossRef CAS.
- I. E. Shohin, et al., Biowaiver Monographs for Immediate Release Solid Oral Dosage Forms: Piroxicam, J. Pharm. Sci., 2014, 103(2), 367–377 CrossRef CAS PubMed.
- G. Piccaro, et al., Activity of lipophilic and hydrophilic drugs against dormant and replicating Mycobacterium tuberculosis, J. Antibiot., 2015, 68(11), 711–714 CrossRef CAS.
- Z. Mandic, Voltammetric study of the partitioning of macrolide antibiotics at the water/nitrobenzene interface. Relationship to the pharmacokinetic profiling of macrolides, ADMET & DMPK, 2014, 2(3), 168–178 Search PubMed.
- J. M. Chan, et al., PLGA–lecithin–PEG core–shell nanoparticles for controlled drug delivery, Biomaterials, 2009, 30(8), 1627–1634 CrossRef CAS PubMed.
- R. A. H. Ishak, N. M. Mostafa and A. O. Kamel, Stealth lipid polymer hybrid nanoparticles loaded with rutin for effective brain delivery - comparative study with the gold standard (Tween 80): optimization, characterization and biodistribution, Drug Delivery, 2017, 24(1), 1874–1890 CrossRef CAS.
- L. Zhang, et al., Self-Assembled Lipid−Polymer Hybrid Nanoparticles: A Robust Drug Delivery Platform, ACS Nano, 2008, 2(8), 1696–1702 CrossRef CAS PubMed.
- H. M. Abdel-Bar and R. A. el Basset Sanad, Endocytic pathways of optimized resveratrol cubosomes capturing into human hepatoma cells, Biomed. Pharmacother., 2017, 93, 561–569 CrossRef CAS.
- L. Jiang, et al., Cellular uptake mechanism and intracellular fate of hydrophobically modified pullulan nanoparticles, Int. J. Nanomed., 2013, 8, 1825–1834 Search PubMed.
- A. E. Allam, H. K. Assaf, H. A. Hassan, K. Shimizuc and Y. A. M. M. Elshaier, An in silico perception for newly isolated flavonoids from peach fruit as privileged avenue for a countermeasure outbreak of COVID-19, RSC Adv., 2020, 10, 29983 RSC.
- B. P. B. Kelley, S. P. Brown, G. L. Warren and S. W. Muchmore, POSIT: Flexible Shape-Guided Docking For Pose Prediction, J. Chem. Inf. Model., 2015, 55, 1771–1780 CrossRef CAS.
- C. N. Cavasottoa, S. M. Lamas and J. Magginice, Functional and druggability analysis of the SARS-CoV-2 proteome, Eur. J. Pharmacol., 2021, 890, 173705 CrossRef PubMed.
- W. Rut, et al., Activity profiling and crystal structures of inhibitor-bound SARS-CoV-2 papain-like protease: A framework for anti-COVID-19 drug design, Sci. Adv., 2020, 6(42), 1–12 Search PubMed.
- M. A. A. Fayed, et al., Structure- and Ligand-Based in silico Studies towards the Repurposing of Marine Bioactive Compounds to Target SARS-CoV-2, Arabian J. Chem., 2021, 14(4), 103092 CrossRef CAS.
- S. Beura and P. Chetti, In-silico strategies for probing chloroquine based inhibitors against SARS-CoV-2, J. Biomol. Struct. Dyn., 2020, 1–13 Search PubMed.
- K. M. S. Baby, Mehta CH Targeting SARS-CoV-2 RNA-dependent RNA polymerase: An in silico drug repurposing for COVID-19, F1000Research, 2020, 9, 1166 CAS.
Footnote |
† Electronic supplementary information (ESI) available. See DOI: 10.1039/d1ra04576h |
|
This journal is © The Royal Society of Chemistry 2021 |