DOI:
10.1039/D1RA02636D
(Review Article)
RSC Adv., 2021,
11, 20944-20960
Droplet flow cytometry for single-cell analysis
Received
4th April 2021
, Accepted 6th June 2021
First published on 14th June 2021
Abstract
The interrogation of single cells has revolutionised biology and medicine by providing crucial unparalleled insights into cell-to-cell heterogeneity. Flow cytometry (including fluorescence-activated cell sorting) is one of the most versatile and high-throughput approaches for single-cell analysis by detecting multiple fluorescence parameters of individual cells in aqueous suspension as they flow past through a focus of excitation lasers. However, this approach relies on the expression of cell surface and intracellular biomarkers, which inevitably lacks spatial and temporal phenotypes and activities of cells, such as secreted proteins, extracellular metabolite production, and proliferation. Droplet microfluidics has recently emerged as a powerful tool for the encapsulation and manipulation of thousands to millions of individual cells within pico-litre microdroplets. Integrating flow cytometry with microdroplet architectures surrounded by aqueous solutions (e.g., water-in-oil-in-water (W/O/W) double emulsion and hydrogel droplets) opens avenues for new cellular assays linking cell phenotypes to genotypes at the single-cell level. In this review, we discuss the capabilities and applications of droplet flow cytometry (DFC). This unique technique uses standard commercially available flow cytometry instruments to characterise or select individual microdroplets containing single cells of interest. We explore current challenges associated with DFC and present our visions for future development.
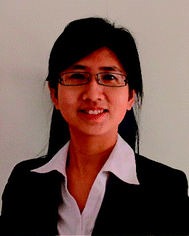 Ming Li | Dr Ming Li is currently a Senior Lecturer in the School of Engineering at Macquarie University in Sydney, Australia. She competed her PhD in Mechanical Engineering at the University of Wollongong, Australia in 2013. She was a postdoctoral research fellow in the Department of Bioengineering and Department of Electrical Engineering at the University of California, Los Angeles, USA from 2014 to 2017, and Department of Electrical and Computer Engineering at the University of Houston, USA from 2013 to 2014. Her research interests include microfluidics, plasmonic biosensors, lab-on-a-chip devices and cytometry. |
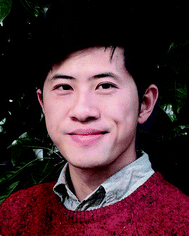 Hangrui Liu | Mr Hangrui Liu received a BSc and a MSc in biotechnology from China Agricultural University in 2014 and 2017, respectively. In 2015–2016, he worked as a visiting scholar in the Australian Institute for Bioengineering and Nanotechnology, University of Queensland. He is currently a PhD candidate in the Department of Physics and Astronomy, ARC Centre for Nanoscale BioPhotonics, Macquarie University, Sydney, Australia, funded by the International Macquarie Research Excellence Scholarship. His research interests lie in the development of biomedical microdevices and biosensors for single-cell biology and medical applications. |
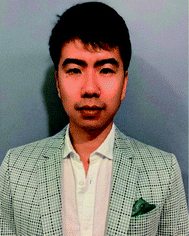 Siyuan Zhuang | Mr Siyuan Zhuang received a Master of Philosophy in Engineering with excellent project thesis outcome from University of New South Wales, Australia. He is currently employed as a professional research staff at Macquarie University and his research focuses on the development of integrated microfluidic platform technologies for single-cell analysis. |
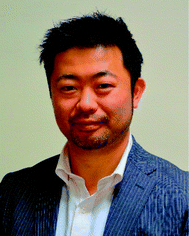 Keisuke Goda | Dr Keisuke Goda is a Professor in the Department of Chemistry at the University of Tokyo, an adjunct professor in the Department of Bioengineering at the University of California, Los Angeles, and an adjunct professor in the Institute of Technological Sciences at Wuhan University. He obtained a BS degree from University of California, Berkeley in 2001 and a PhD degree from Massachusetts Institute of Technology in 2007, both in physics. In 2012, he joined the University of Tokyo as a professor. His research group focuses on the development of serendipity-enabling technologies to achieve Louis Pasteur's statement “Chance favours the prepared mind”. |
1. Introduction
Single-cell analysis plays an essential role in revealing the differences of individual cells in structure and function.1,2 The adaption of single-cell techniques has highlighted critical cell-to-cell heterogeneity, identified rare sub-populations of functional importance and discovered unique characteristics of individual cells.3,4 Flow cytometry (FC) has been widely used as a versatile and powerful technique to interrogate single cells, offering automated, quantitative, high-throughput and multi-parameter characterisation of cell phenotypes and activities.5,6 In this technique, individual cells suspended in a fluid flow are directed to pass through a laser beam one by one and then measured based on the resulting scatter or emission of light energy from fluorescence molecules. By adding cell sorting functionality, fluorescence-activated cell sorting (FACS, a specialised type of FC)7,8 can selectively isolate individual cells of particular interest from heterogeneous populations into separate containers (e.g., tubes, well plates or other collection containers), allowing subsequent experiments on the selected cells. Single-cell analysis by FC (including FACS) has enabled significant advances in life and biomedical sciences, clinical diagnosis and drug development.9–11
However, FC-based single-cell analysis is exclusively limited to readouts from fluorescent molecules tagged either within the cells or on the cell surface. The attributes of individual cells are directly quantified and assessed by the light emitted by the fluorophore, which indicates the number of antibodies present within or on the cell. Unfortunately, this largely excludes important extracellular biomarkers and cell phenotypes and activities that are spatially and temporally important, such as secreted proteins, extracellular metabolite production, and proliferation. For example, the proteins secreted by cancer cells (i.e., secretomes) could represent putative tumour biomarkers or therapeutic targets.12 Metabolites produced by industrial microorganisms have great values in nutrition, sustainable agriculture and healthcare sectors;13 real-time cell proliferation assays are essential in drug discovery and clinical evaluation.14,15 Therefore, there is a need to develop strategies that enable FC to make comprehensive measurements of cells, including extracellular, spatial and temporal biomarkers of biological, industrial and clinical significance.
Droplet microfluidics16,17 has emerged as an incredible tool in this effort, which can compartmentalise individual cells in pico-litre microdroplets containing a highly reproducible volume of a reaction mixture. Unlike conventional water-in-oil (W/O) droplets, two main architectures of droplets suspended in aqueous solutions are compatible with FC: water-in-oil-in-water (W/O/W)18 double emulsion (DE) droplets and hydrogel droplets.19 The pairing of FC and droplets allows a complete characterisation of individual cells beyond the conventional surface and intracellular biomarkers, featuring high throughput, sensitivity and dynamic range, and low cost. Moreover, it enables tandem genomic, epigenomic, or transcriptomic analyses on isolated cells,20 allowing integrative single-cell analysis techniques. This integrated approach has helped a wide range of discoveries, including directed evolution of enzymes and proteins,21–24 probing cellular heterogeneity in response to drug treatment,25,26 recognition of rare cells in microbial communities,25,27–30 determination of antibiotic resistance genes,31,32 and identification of biomarkers linked to diseases.33,34
Although fluorescence-activated droplet sorting (FADS)35,36 and other variants of the technique (e.g., sequentially addressable dielectrophoretic array (SADA)37,38 and printed droplet microfluidics (PDM)39) allow on-chip screening and sorting of W/O droplets based on fluorescent readouts of in-droplet assays, the high complexity of custom devices and instruments involved limit their widespread adoptions.35,40 Moreover, they can only simultaneously measure 1 or 2 fluorescent parameters and requires relatively slow rates (i.e., 0.1–2 kHz, two orders of magnitude slower than FACS) for high-accuracy sorting.40 Besides fluorescence, other optical detection techniques, such as adsorption spectroscopy41 and Raman spectroscopy,42 have been applied to characterise and select droplets encapsulated with individual cells in a microfluidic chip. However, these techniques suffer from low specificity (adsorption spectroscopy) and low sensitivity (Raman spectroscopy), making them unpopular in high-throughput droplet screening workflows.
The article reviews the current achievements in use of FC (including FACS) to screen and isolate microdroplets encapsulated with single cells of interest. It should be noted that we do not highlight advances in FADS and other techniques for screening and sorting of cell-laden W/O droplets on a microfluidic chip. As shown in Fig. 1, different types of single cells (e.g., mammalian, bacterial and yeast cells) are encapsulated individually within microdroplets (either W/O/W DE droplets or hydrogel droplets), which can be screened and sorted by commercial FC machines, and further analysed at the downstream (e.g., sequencing). This integrated technology, so called droplet flow cytometry (DFC), allows a variety of biochemical assays at the single-cell level, such as single-cell cultivation, molecular evolution, single-cell detection and cell-to-cell interactions, due to droplet monodispersity, cell compartmentalisation and high-throughput processing. Also, this opens avenues for broad applications in the fields of drug discovery, metabolic engineering, medical diagnosis and cell biology.
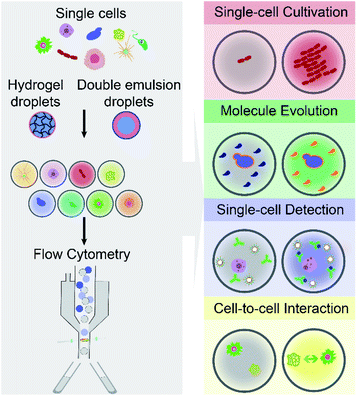 |
| Fig. 1 Droplet flow cytometry enables a wide range of high-throughput single-cell analysis, by integrating emulsion microdroplets (e.g., water-in-oil-water double emulsion and hydrogel droplets) with commercially available flow cytometry. | |
Specifically, this review is composed of the following sections. First, Section 2 introduces two main architectures of emulsion microdroplets compatible with FC, namely W/O/W and hydrogel droplets. Next, Section 3 summarised the main capabilities of integrating emulsion microdroplets and FC techniques (so-called DFC) for high-throughput single-cell analysis. Also, Section 4 discusses critical field applications of DFC. Furthermore, Section 5 discusses current challenges confronted by DFC with an eye towards future development. Finally, Section 6 concludes the review.
2. Architectures of emulsion microdroplets that are compatible with flow cytometry
An FC system is typically equipped with a fluidic system for cell handling, consisting of sheath fluid (e.g., a buffered saline solution) to deliver and focus cells one by one in a narrow uniform stream. It enables cells to be equally illuminated, detected and measured as they pass through the laser intercept or interrogation point. Since FC requires cells to be suspended in an aqueous sheath fluid, it is not compatible with conventional W/O droplets having an insulating oil surrounding the aqueous core. Fortuitously, two main formats of droplets suspended in aqueous solutions, W/O/W DE droplets and hydrogel droplets, provide alternate droplet architectures compatible with FC. Individual cells can be encapsulated, cultured, subjected to biochemical assays in droplets; the cell-laden microdroplets can be detected as individual events by the cytometers and then screened or selected. Flow cytometric analysis of single-cell laden microdroplets allows a detailed study of cellular characteristics at the single-cell level by combining high throughput and rapid analysis with a relatively low reagent consumption and a high dynamic detection range.
2.1 Water-in-oil-in-water (W/O/W) double emulsion (DE) droplets
Conventional emulsion droplets can be either water-in-oil (W/O) or oil-in-water (O/W) single emulsions. There also can be more complex systems, such as W/O/W DE droplets, in which a W/O emulsion is dispersed in a second continuous aqueous phase.43,44 Therefore, W/O/W DEs can be viewed as two aqueous phases separated by a thin oil layer, stabilised by a monolayer of molecules of emulsifier or emulsifying agents at the interface between water and oil. Wettability plays a vital role in the types of emulsion droplets generated, as hydrophobic and hydrophilic surfaces are required to generate W/O and O/W emulsions, respectively.45–47 By selectively controlling the wettability of microchannels, microfluidic devices enabled a hierarchical generation of monodisperse W/O/W DEs encapsulated with individual cells.47–50
There are two main approaches to generate W/O/W DEs: the two-step batch method and the one-step continuous flow method using microfluidic devices.51 In the first approach, water phase and oil phase are mixed by stirring to form W/O droplets, which can then be encapsulated by another water phase via constantly adding more water under the stirring condition. However, DE droplets produced by this approach have large variations in size.52 The one-step continuous flow method is more widely used for single-cell encapsulation. DE production by this approach always requires a relatively high rate of oil phase flow to cut water phase with a low rate to generate W/O droplets, and thereafter a second aqueous flow with a much higher rate is used to cut the oil-water layer flow. Unlike the batch method, it ensures that microdroplets can be consecutively made with a uniform size.
In the DE, the aqueous internal phase can be composed of a reaction mixture (e.g., substrates, buffers, fluorescent dyes and antibodies), cell suspensions or a gene library, providing a suitable environment for different cellular assays.49,53,54 The oil shell is bioinert and non-adhesive to hydrophilic activities and cells. The usage of fluorinated oil (e.g., HFE-7500 and FC-40) acting as a selective barrier can reduce hydrophilic mass transfer and communication between DEs.49,55,56 The aqueous external phase allows the suspension of DEs in nutrient medium for long-term cell culture and the compatibility with commercial cytometers for high-throughput assays18,49,57,58 (with the sorting speed >107 events per hour31,32,56).
The integration of FACS and W/O/W DE droplets have already found various applications, such as analysis of cell-secreted molecules and enzymatic turnover. Moreover, the isolated cells from heterogeneous populations allow downstream bioinformatics analysis (e.g., tandem genomic, epigenomic and transcriptomic analyses) of the same target, revealing the biological functions of massive genetic pathways.48–50
2.2 Hydrogel droplets
Hydrogels are three-dimensional cross-linked hydrophilic polymer networks that are capable of swelling or de-swelling reversibly in water. By using monomer or polymer solutions as the aqueous dispersed phase and oil with a surfactant as the continuous phase, hydrogel droplets in oil phase can rapidly generate by either laboratory-customised 2D microfluidic devices59 or 3D-printed microdroplet generators60 for various biomedical assays. Unlike W/O droplets, the generated hydrogel droplets can be transferred from an oil suspension into an aqueous solution (e.g., buffer or cell culture medium) after gelation for use in FC. The hydrogel microdroplets can offer a biologically friendly environment (mimic the extracellular environment by a three-dimensional matrix for cell culture). A microcapsule remains the activities of living cells61–65 and their genes,66–69 secreted proteins70–73 and other ingredients (e.g., drugs74–77). Due to their biocompatibility, mechanical and chemical stability in aqueous media, sufficient permeability of porous structure and tunable polymer properties, hydrogels (both natural and synthetic) have been widely used in droplet microfluidics for single-cell assays.
Natural hydrogels used for emulsion microdroplets are either polysaccharides (e.g., agarose, alginate, and chitosan) or proteins (e.g., collagen and gelatin). For example, agarose is a linear neutral polysaccharide extracted from specific red seaweed, which undergoes sol–gel transition depending on temperature.78 Alginate is an anionic polysaccharide typically obtained from brown seaweed and bacteria, which chelates with alkaline earth metal ions, such as calcium (Ca2+), barium (Ba2+) and strontium (Sr2+) to form hydrogels. Gelatin is a protein derived from collagen, gels via a cold-setting mechanism and remains gel-like upon heating up to physiological temperature. It allows the liquid form to remain during the cultivation period of cells (i.e., at 20–27 °C) and cell recovery under mild conditions (i.e., at 35 °C) without cell damage.79
Besides, synthetic hydrogels, such as poly(ethylene glycol), poly(acrylic acid), poly(vinyl alcohol), poly(acrylamide) and their derivatives, offer desirable, controllable and reproducible properties (e.g., molecular weights, structures and crosslinking density), which translate into tunable chemical, mechanical, and degradation properties of hydrogels. Typically, the aqueous monomer solutions are mixed with initiator and cross-linker, followed by polymerisation or crosslinking via different methods, such as photoirradiation. For example, poly(ethylene glycol) (PEG) can be chemically modified to generate ultraviolet (UV)-sensitive PEG-diacrylate that allows photoinitiated polymerisation.80,81
3. Capabilities
By encapsulating individual cells from a heterogeneous population within monodisperse microdroplets (e.g., W/O/W DE and hydrogel droplets) and analysing cell-laden microdroplets as individual events by FC (including FACS), DFC has enabled new functionalities and diversified applications for single-cell analysis. This section aims to summarise and discuss four main capabilities of DFC, namely single-cell cultivation, molecular evolution, single-cell detection, and cell-to-cell interaction (see Fig. 1), which allow a broad range of applications in biological, biomedical and environmental fields.
3.1 Single-cell cultivation
Microdroplets can act as miniaturised bioreactors to facilitate cell-based biological studies. This is because they mimic the micro-scale environment where biochemical events transpire and significantly enhance the efficiency and sensitivity of high-throughput cellular studies by reducing reagent consumption and improving detection signals.82,83 Flow cytometric analysis of single cells encapsulated in microdroplets was reported as a powerful tool for high-throughput screening of cell growth kinetics and metabolite accumulation.31,32,56 Y. Zhang et al.56 proposed flow cytometric characterisation of bacterial growth in DE droplets acting as programmable bioreactors. Unlike single emulsion droplets that are incapable of continuous supply of nutrient molecules, the oil shell in the DEs has demonstrated the capability to allow selective transport of small nutrient molecules and chemical inducers into the aqueous core. This study demonstrated the capability of tracking the growth of individual bacterial cells both qualitatively and quantitatively, where DEs enable more control over microbial cell growth conditions. Moreover, it shows the potential of using DEs for the study of complex biological processes requiring communication with external environments. M. Li et al.79 quantitatively tracked the growth and metabolite (i.e., chlorophyll and lipid) accumulation of two microalgal species, Euglena gracilis (E. gracilis) and Chlamydomonas reinhardtii (C. reinhardtii), in gelatin hydrogel microdroplets using FACS (see Fig. 2A). The porous hydrogel structures enabled the infusion of fluorescent reporter molecules into the hydrogel matrices, and cells exhibiting desired properties (e.g., high biomass and metabolite production) can be recovered after sorting for re-culture. In comparison to previous works, this research enables quantitative tracking of not only the growth of individual cells but also their metabolite production. The proposed technology can be easily integrated into directed cellular evolution pipelines for microbial strain improvement.
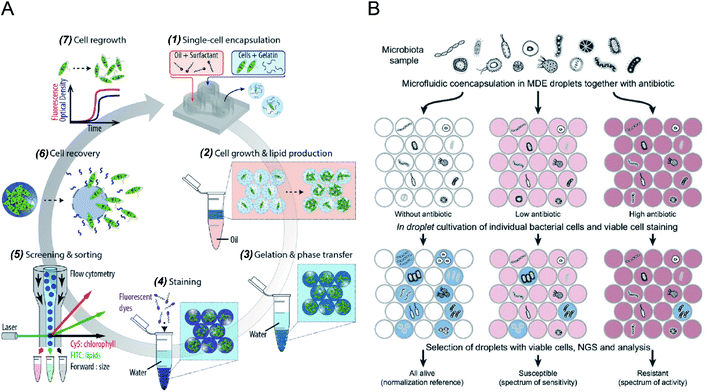 |
| Fig. 2 Droplet flow cytometry for single-cell cultivation. (A) Schematic workflow of screening and selecting microalgal cells with high biomass and lipid production rate. (1) Encapsulation of single microalgal cells within gelatin droplets, (2) cultivation and metabolite accumulation of single cells, (3) transfer of cell-laden hydrogel droplets from oil into an aqueous phase after gelation, (4) staining of target metabolites in hydrogel beads, (5) screening and sorting of hydrogel beads containing a high level of metabolites, (6) recovery of cells from the hydrogel beads, (7) regrowth of released cells, which can be reintroduced into iterative selection. Adapted with permission from ref. 79. Copyright 2018 John Wiley & Sons, Inc. (B) Schematic diagram of the activity/sensitivity spectrum assessment of a highly heterogeneous bacteria population (microbiota) using DEs. Individual cells from microbital samples were encapsulated in DEs together with different concentrations of the antibiotic, amicoumacin A (Ami). After cultivation, DEs were stained for metabolic activity, selected by FACS, and analysed by next-generation sequencing (NGS) and bioinformatics. Adapted with permission from ref. 31. Copyright 2018 National Academy of Sciences. | |
The integration of emulsion microdroplets with FACS has also enabled antibiotic susceptibility testing, the discovery of antibiotic resistance genes and the quantification of microbiome diversity. Y. J. Eun et al.84 encapsulated E. coli cells in agarose droplets and determined the minimum inhibitory concentration (MIC) of rifampicin using FACS. Spontaneous mutants resistant to antibiotic were isolated and further characterized by DNA sequencing, which identified β-subunit of RNA polymerase, RpoB, as the target. S. S. Terekhov et al.31 reported the culture of individual cells from a heterogeneous bacterial population in DE droplets to investigate the efficacy of an antibiotic, amicoumacin A (Ami), toward different microbiomes (see Fig. 2B). Unlike bulk cultures where fast-growing species can quickly dominate a population, single-cell cultivation in droplet compartments allows researchers to assess minor microbiota species representing <0.1% of the initial population. Later, potential antibiotic producers were selected using FACS, and a unique mechanism of self-resistance was discovered using proteomics and heterologous expression. This outcome shows that DFC could be efficiently applied to analyse complex microbial communities, and potentially expanded to functional profiling of eukaryotic cells.
In addition to microorganisms (e.g., bacterial and microalgal cells), mammalian cells have been successfully encapsulated and cultured in DE droplets for high-throughput screening. K. K. Brower et al.18 performed the phenotyping of individual cells from four mammalian cells lines, including mouse embryonic stem cells, human T lymphocytes, mouse macrophage cells, human embryonic kidney cells, and mouse embryonic fibroblasts having different morphologies and sizes (5–20 μm), in DEs (with an inner diameter of 35 μm). Using a live-cell labelling dye, Calcein Blue AM, as an indicator of cell-laden DEs, FACS measurement results successfully discriminated empty droplets from those containing cells, which showed good agreement with theoretical predictions by Poisson distribution. This high-throughput screening of DEs encapsulated with different types of mammalian cells opens up new avenues for various biomedical applications, such as disease diagnostics, drug discovery, and rare mutation detection.
3.2 Molecular evolution
Microbial production of industrial enzymes and other value-added bioproducts is attractive due to their advantages compared to conventional chemical syntheses, such as sustainability, reduction in environmental pollution, and cost-effectiveness.6 Although agar plate screening and microtiter plate assays enable automatic screening of enzymatic activities, they are costly and low-throughput, limiting the number of strain variants that can be evaluated (typically in the range of 103–104).53,54,85 Single-cell FACS has already demonstrated its capability to select individual cells exhibiting desired properties from a heterogeneous cell population, significantly increasing the screening throughput (with sorting speed >107 events per hour).86 However, FACS for enzyme selection is limited to the cases where signalling molecules have restricted diffusion out of the cells or can be entrapped on the cell surface or antibody-conjugated microbeads. This limitation can be overcome by encapsulating single cells within emulsion microdroplets, which restrict the diffusion of products by compartmentalization, and the fluorescence intensity of the whole droplet can be detected by FC, which is also accurately correlated with the activity of encapsulated individual cells.
A. Aharoni et al.54 first reported using the DE-FACS technique and achieved high-throughput screening of enzyme libraries (see Fig. 3A). A variant gene library was transformed and cloned into E. coli, and the encoded protein, serum paraoxonase (PON1), was allowed to translate in the cytoplasm or on the surface of the E. coli cells. After encapsulation and cultivation of individual E. coli cells in DEs, compartments containing the fluorescent products, encapsulated cell variants, and the gene encoding enzyme were sorted by FACS. The isolated PON1 variants showed 100 fold improvements in TBLase catalytic efficiency. These works demonstrated the use of DE-FACS for directed enzyme evolution by directly monitoring the actual levels of endogenous cellular enzymes (rather expression levels of a reporter protein). Moreover, it shows the capability to identify rare phenotypes and genotypes for analysing large populations at a single-cell level.
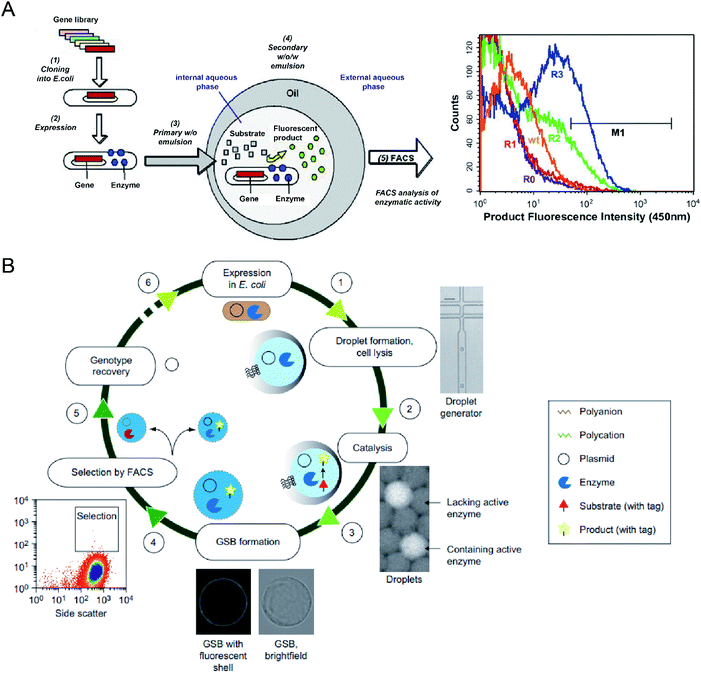 |
| Fig. 3 Droplet flow cytometry for molecular evolution. (A) Schematic workflow of directed enzyme evolution by screening and selecting positive DE droplets via FACS. Left: (1) a variant gene library was transformed and cloned into E. coli, (2) the encoded proteins translated within E. coli, (3) encapsulation of single cells in the W/O emulsion droplets, (4) addition of fluorogenic substrates through the oil phase and the formation of W/O/W DE droplets. Cells with functional enzymes can convert the non-fluorescence substrates into fluorescent products entrapped in the internal core, (5) analysis of enzymatic activity across the DE droplet populations via FACS. Right: FACS results for DE droplets containing E. coli expressing wide-type (wt) enzyme, unselected library (R0) and the library after one (R1), two (R2) and three (R3) rounds of sorting based on gate M1. Adapted with permission from ref. 54. Copyright 2006 Cell Press. (B) Schematic workflow of directed enzyme evolution using gel-shell beads (GSBs) and FACS. (1) Encapsulation of single E. coli cells expressing target enzyme in single emulsions and cell lysis to liberate the enzyme and its coding plasmid, (2) release of a fluorescent product by catalysis, (3) formation of GSB to entrap products, (4) high-throughput screening and sorting of GSB containing catalytically active hits via FACS, (5) recovery of variants with desired phenotypes, (6) iterative rounds of selection. Adapted with permission from ref. 93. Copyright 2014 Nature Publishing Group. | |
To date, DE-FACS has been widely used for the directed evolution of different types of enzymes, including thiolactonase,54 β-glucosidase,23 arysulfatase50, G-type nerve agent hydrolase,24 protease,22 cutinase,87 cellulase,88 glucose oxidase,89 esterase,90,91 and polymerase,92 expressed in different microbial strains, such as S. cerevisiae, E. coli and Bacillus subtilis (B. subtilis). Moreover, hydrogel droplets with either core–shell (e.g., agarose-alginate and polyacrylamide-agarose) or single emulsion (e.g., PEG and alginate) structures have enabled the directed evolution of phosphotriesterase,93 hydrolytic enzymes94 for high-throughput cell cultivation and enzymatic analysis95 by integrating with FACS. For example, M. Fischlechner et al.93 utilised gel-shell microbeads with a polyelectrolyte shell to encapsulate an enzyme, its encoding DNA and fluorescent reaction product. FACS was able to identify active clones within the compartments at rates of higher than 107 beads per hour (see Fig. 3B). This work allows the establishment of gel-shell microbeads as biomimetic compartments in which the content can be modified by directed evolution. The presence of the hydrogel shell allows more effective retention of target molecules (e.g., cell-secreted molecules and cleaved products from the substrate) within the droplet compartments.
Besides the directed evolution of enzymes, microorganisms exhibiting high yields of other industrially essential metabolites, e.g., organic acids and vitamins, have also been screened and selected using DE-FACS. J. M. Wagner et al.96 successfully isolated Yarrowia lipolytica (Y. lipolytica) mutants, resulting in a 54 fold increase in Riboflavin (vitamin B2) production parent strain. Since Riboflavin is an innate fluorescent product, no extra fluorescent substrates were used. Also, the isolated mutants can produce more extracellular products and the total amount of products than those separated by single-cell FACS, as confirmed by absorbance assays and high-performance liquid chromatography (HPLC). Similarly, X. D. Zhu et al.97 selected a Bacillus coagulans (B. coagulans) mutant yielding 52% higher lactic acid production than its parent strain. A pH-sensitive fluorescent sensor was adopted to indicate the level of lactic acid production inside the DEs, allowing the selection of DEs containing high-yielding mutants based on fluorescence.
DE-FACS has been widely used for directed molecular evolution by isolating single cells exhibiting a desirable phenotype. By combing with sequencing of the isolated variants, it provides valuable insights into the underlying mechanisms of molecular evolution. It is also obvious that this technology can be applied to other areas where analysing and sorting single cells in a high-throughput manner is needed, such as functional genomics and advanced protein engineering.
3.3 Single-cell detection
Sensitive and selective detection of single molecules (e.g., DNA, RNA and proteins) and single cells are essential for probing cellular heterogeneity and discovering the unbiased diversity of cells in biology, chemistry and biomedicine. However, due to the limited initial numbers of single cells, traditional single-cell sequencing approaches are time-consuming and low-throughput. In contrast, droplet microfluidics is a promising technique for encapsulating and processing individual cells for whole-transcriptome or genomic analysis in a massively parallel manner with minimal reagent consumption. By taking advantage of FC (and FACS), ultra-high throughput and multi-parameter genetic analysis of a large heterogeneous population of cells at the single-cell level can be achieved to discover stochastic variations in a complex biological system.
The integration of agarose microdroplets and FACS allows the identification and sorting of rare pathogens using polymerase chain reaction (PCR) signals from individual cells. A notorious pathogen causing many food-borne illnesses, E. coli O157:H7, was used as a target analyte, and the typical E. coli K12 was used as background noise.27 Massively parallel single-molecule PCR, singleplex and multiplex single-cell PCR were achieved in agarose droplets, and FACS determined the fluorescence of droplets (see Fig. 4A). This technique enabled the sensitive and quantitative analysis of single E. coli O157:H7 cells in the high background of 100
000 excess normal E. coli K12 cells. A similar work using agarose microdroplets for single-molecule Reverse Transcription Polymerase Chain Reaction (RT-PCR) was reported by H. Zhang et al.28 Agarose droplets demonstrated the capability to carry RT-PCR products after amplification at the single-molecule level. As a result, single-cell transcriptome analysis revealed a clear difference in the expression level of a cancer biomarker gene (i.e., EpCAM) between different types of cancer cells (Kato III and MDA-MB-231 cells) at the single-cell level.
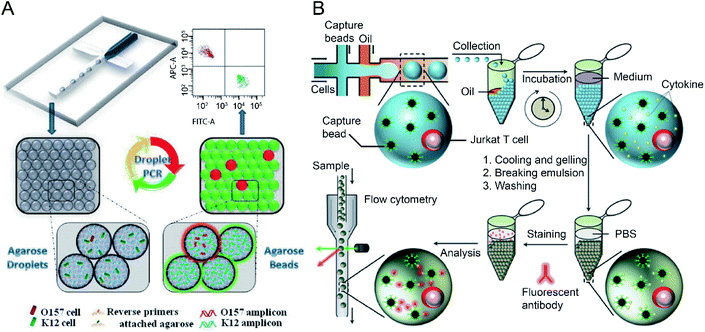 |
| Fig. 4 Droplet flow cytometry for single-cell detection. (A) Schematic diagram of rare pathogen detection by droplet-based PCR using agarose droplets and FACS. The rare pathogens (O157) and high-density normal bacteria (K12) are co-encapsulated into agarose droplets for PCR amplification. The PCR reagent mixture including two forwards primers labelled fluorescent dyes and two specific reverse primers specific for K12 and O157, respectively, were covalently conjugated to agarose. After PCR and cooling down, the agarose beads are analysed by flow cytometry for the detection of O157 cells. Adapted with permission from ref. 27. Copyright 2012 Royal Society of Chemistry. (B) Schematic workflow of the detection of cytokines secreted by single cells using agarose droplets and FC. Single Jurkat T cells were encapsulated within agarose droplets together with functionalized cytokine-capture nanobeads. After incubation, gelation, demulsification and washing, agarose beads were stained with fluorescent antibodies which can bind to the secreted cytokines, and high-throughput screening of cytokines secreted by single cells were performed by flow cytometry. Adapted with permission from ref. 25. Copyright 2013 Royal Society of Chemistry. | |
The detection of a single gene of individual microbial genomes from a mixture of microbial cells was achieved using hydrogel droplets with core–shell structures.29 Polyacrylamide droplets were used to encapsulate individual microbial cells and trap their genomes after cell lysis, which were further converted into agarose pico-reactors for subsequent multiple displacement amplification (MDA) reaction. After PCR, the polyacrylamide–agarose hydrogel droplets containing genomic DNA with target genes were fluorescently labelled with SYBR Green and analysed by FC. E. coli XL1 genomes containing a tetracycline resistance gene present 0.1% of E. coli MC1061 genomes without the tetracycline resistance gene can be differentiated.
The profiling of the cellular heterogeneity in cytokine secretion by cancer cells12,98 and immune cells26 at the single-cell level has been demonstrated. V. Turcanu et al.30 encapsulated lymphocytes in agarose microdroplets, which were functionalized with avidin to capture biotin-labelled cytokine-specific antibodies. Cytokines secreted by the encapsulated cells can be detected using a second fluorescent antibody specific to the cytokine. The analysis of several key cytokines, such as interleukin (IL)-4, transforming growth factor (TGF)-β, interferon-γ (IFN-γ) and IL-10 secreted by human peripheral blood mononuclear cells (PBMCs) and a rat keratinocyte cell line transfected with the human TGF-β gene was achieved. Also, polystyrene beads functionalized with specific antibodies have been used to capture cytokines secreted by immune cells entrapped in the droplet compartments. V. Chokkalingam et al.25 encapsulated single activated Jurkat Tet cells in monodisperse agarose droplets with functionalized cytokine-capture nanobeads. After incubation and phase transfer, the agarose beads were stained with fluorophore-labelled antibodies that bind to the cytokines, allowing flow cytometric detection of secreted cytokines (IL-2, IFN-γ, TNF-α) from single Jurkat T cells (see Fig. 4B). Besides hydrogel functionalisation and use of cytokine-capture nanobeads.
In addition to gene expression and cytokine, proteins,98 metabolites,99 and exosomes100 can be used as biomarkers for single-cell detection. For example, X. Yang et al.101 performed on-cell target detection and phenotypic probing of live cancer cells at the single-cell level by integrating droplet compartments with a fluorescence microscope. A biomarker-designated enzymatic fluorescent assay was carried out within the droplets, which was based on a DNA–antibody conjugate to the surface of live cancer cells. This approach shows great potential for the detection of different biomarkers at the single-cell level by modifying the antibody–DNA constructs with other antibodies of interest.
The single-cell agarose droplet flow cytometry method has tremendous implications in detecting rare pathogenic cells, analysing single-cell protein secretion and profiling of cellular heterogeneity at the single-molecule level and enumerating rare cancer cells, and shows great potential for numerous applications (e.g., environmental science, drug discovery, diagnostics, cancer research, regenerative medicine, and synthetic biology).
3.4 Cell-to-cell interaction
Microbial interactions play a pivotal role in the dynamics and functions of microbial communities. However, it is difficult to reveal this intertwined phenomenon due to limitations in parallel culture and absolute abundance quantification of community members across environments and replicates.102 DFC has enabled rapid and quantitative investigation of the interaction networks between community members by encapsulating microbial cells into the micro-droplets that are screened and selected in parallel.
S. S. Terekhov et al.32 investigated different types of bacterial cell-to-cell interactions using DE-FACS (see Fig. 5A). A common pathogen, Staphylococcus aureus (S. aureus), was co-cultured with a mate (E. coli), which led to the growth of both bacteria in droplets, and an antibiotic producer, Streptomyces venezuelae (S. venezuelae), which inhibited the growth of S. aureus as a killer. Since all the bacteria used in the experiment have different fluorescence reporters, killers and mates can be distinguished, and droplets encapsulating with killers can be selected. This technique was then used to identify rare S. aureus killers from human oral microbiota by selecting the droplets containing a high concentration of killers and a low concentration of S. aureus. Slow-growing oral microbiota species that inhibit the growth of S. aureus were isolated, and genera associating with inhibitory activity was revealed. Using the same platform, S. S. Terekhov et al.31 performed environmental microbiota communities profiling (i.e., a Siberian bear oral microbial community) to discover secondary microbial metabolites. The target pathogen, S. aureus, was co-encapsulated with individual cells from the heterogeneous community to identify rare antimicrobial strains. As a result, bacterial clones with inhibitory activity, especially ‘unculturable bacteria’ that cannot be recognized by standard bulk methods, were selected and identified.
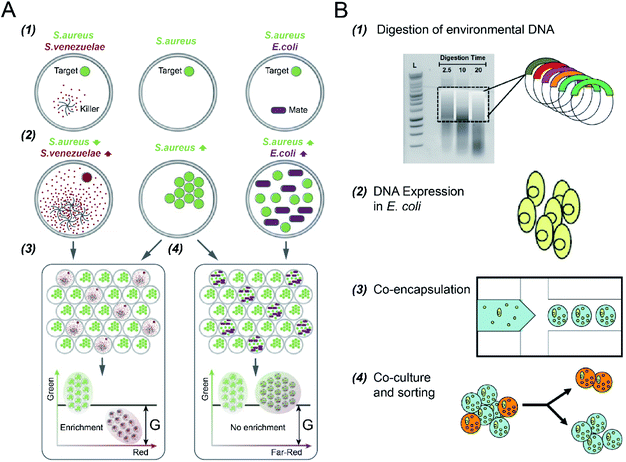 |
| Fig. 5 Droplet flow cytometry for cell-to-cell interaction. (A) Screening of bacteria inhibiting S. aureus growth using DE-FACS. (1) Target S. aureus cells with a GFP reporter were encapsulated with either antibiotic producer, S. venezuelae (secreting red fluorescent metabolites) or mate E. coli (with a far-red fluorescent reporter). (2) The inhibition of S. aureus with the growth of S. venezuelae, and the growth of both E. coli and S. aureus, which yielded different combinations of fluorescent signals. (3 and 4) Selection of DE droplets with the lowest fluorescence level caused the enrichment of killers S. venezuelae (3) rather than mates E. coli (4). Adapted with permission from ref. 32. Copyright 2017 National Academy of Sciences. (B) The workflow of high-throughput screening and selection of the co-culture of recombinant E. coli and S. aureus for antibiotic drug discovery via the integration of agarose droplets and FACS. (1) environmental DNA was subjected to a limited DNasel digestion, (2) metagenomic DNA was cloned and then transferred into E. coli, (3) individual recombinant E. coli were co-encapsulated with live S. aureus (small yellow spheres), (4) microdroplets were labelled with a fluorogenic viability probe and then selected by FACS to isolate E. coli secreting bacterial natural products. Adapted with permission from ref. 33. Copyright 2013 John Wiley & Sons, Inc. | |
In addition to DEs, hydrogel (agarose) microdroplets have also been used to uncover yeast–bacterium interactions for antibiotic drug discovery,33 where bacterial pathogens were co-cultured with recombinant host microorganisms capable of secreting biocatalytic antibiotics and/or secondary metabolites (see Fig. 5B). S. cerevisiae strains, including both the positive control (with the secretion of bacteriolytic enzyme lysostaphin) and the negative control (with the secretion of inactive lytic hydrolase enzyme), were co-encapsulated with a target pathogen S. aureus, respectively. Following host S. cerevisiae growth and protein induction, agarose microdroplets were stained with a fluorogenic viability probe (SyTox Orange) that selectively stains killed target pathogens, and then analysed by FACS. Rare positive clones producing a lytic hydrolase specific for S. aureus can be isolated with an enrichment factor of up to 10
000 fold relative to the initial mixture.
Moreover, the interactions between microalgal and bacterial cells have been investigated. J. Ohan et al.34 utilised agarose gel droplets to co-encapsulate individual bacterial cells from a complex microbial community with Chlorella sorokiniana (C. sorokiniana), a candidate algal strain for biofuel production. Using FACS, viable bacteria species exhibiting the desired phenotype (i.e., improved algal growth rate) were isolated and recovered. Researchers have also reported the use of DFC for the screening of co-cultures of mammalian cells and yeast cells. Individual yeast secretors (e.g., S. cerevisiae103 and Pichia pastoris104) and mammalian reporter cells were co-encapsulated and cultivated in agarose microdroplets, which is followed by FACS to select clones secreting bioactive recombinant proteins. These studies demonstrated the feasibility of DFC for screening and isolation of protein variants produced by microbes, which would enable and accelerate the discovery of new functional biologics, such as cytokines, antibodies, and soluble receptors.
Using the same technique, E. Tumarkin et al.105 uncovered mammalian cell interaction networks. MBA2 cell line (secreting interleukin-3 (IL-3)) and the human megakaryoblastic leukemia cell line, M07e (depending on IL-3 for proliferation and survival), were co-encapsulated within agarose hydrogel droplets at different ratios. Flow cytometric analysis results showed that local paracrine secretion could modulate the viability of the factor-dependent M07e cells. Besides, IL-3 secreting MBA2 cells were co-encapsulated with umbilical cord blood (UCB) cells to determine the impact of IL-3 on a heterogeneous cell population, which revealed subpopulations that are primarily dependent on locally delivered IL-3 (i.e., CD14 + cells).
Mammalian cell-to-cell interactions are crucial in cell development, tissue and organ homeostasis, and immune interactions in disease.106,107 Researchers have achieved in vitro co-culture of different types of mammalian cells to investigate the underlying metabolic processes and interactions.108 Unfortunately, despite the biological and biomedical significance of mammalian cellular interactions, there are still quite limited reports in literature regarding the use of the DFC in the study of mammalian cell communications. With further advances in droplet compartments and cell co-culture models, we can expect that wider communities will adopt DFC for cell-to-cell interaction analysis.
4. Applications
By pairing FC with emulsion droplets (i.e., W/O/W DE and hydrogel droplets), it enables the screening and isolation of microdroplets encapsulated with rare cells exhibiting properties of interest and characterization of unexpected cell-to-cell heterogeneity within the population. Table 1 summarised the capabilities of DFC for high-throughput single-cell analysis. This integrated technology is critical for various applications, including but not limited to drug screening, medical diagnosis, metabolic engineering and cell biology.
Table 1 Summary of capabilities of droplet flow cytometry for high-throughput single-cell analysis
Microdroplet |
Target cell |
Application |
Single-cell cultivation |
W/O/W |
E. coli |
Cell culture (from proliferation to death)56 |
W/O/W |
Oral microbiota of Siberian bear |
Cell culture (with antibiotics)31 |
Human fecal microbiota from patient and healthy donors |
W/O/W |
Mouse embryonic stem cells (E14), macrophage cells (LM-1) and embryonic fibroblasts (NIH 3T3); human T lymphocytes (Jurkat) and embryonic kidney cells (HEK 239T) |
Screening of cell encapsulation ratios for different types of cells18 |
Gelatin |
E. gracilis, C. reinhardtii |
Chlorophyll and lipid accumulation79 |
Agarose |
E. coli |
MIC of rifampicin determination; mutant isolation84 |
![[thin space (1/6-em)]](https://www.rsc.org/images/entities/char_2009.gif) |
Molecular evolution |
W/O/W |
E. coli |
Thiolactonases (100 fold increase)54 |
Arysulfatase50 |
β-Glucosidase (∼2 fold increase for lactose)23 |
G-type nerve agent hydrolase (104-fold increase)24 |
Cutinase (8 fold increase)87 |
Esterase (2 fold increase)90 |
Polymerase (1200 fold increase)92 |
W/O/W |
B. subtilis |
Protease (1.6 fold increase)22 |
W/O/W |
S. cerevisiae |
Endoglucanase-II cellulase (20 fold increase)88 |
Glucose oxidase (5.8 fold increase)89 |
Agarose-alginate polyelectrolyte |
E. coli |
Phosphotriesterase (20 fold increase)93 |
PEG |
E. coli |
Hydrolytic enzyme (3 fold increase)94 |
W/O/W |
Y. lipolytica |
Riboflavin (54 fold increase)96 |
W/O/W |
B. coagulans |
Lactic acid (52% more effective)97 |
![[thin space (1/6-em)]](https://www.rsc.org/images/entities/char_2009.gif) |
Single-cell detection |
Agarose |
E. coli (O175:H7 and K12) |
Detection of O157:H7 (0.0001%)27 |
Agarose |
Human gastric carcinoma cells (Kato III) and breast cancer cells (MDA-MB-231) |
Differentiation in gene expression level of a cancer biomarker (EpCAM)28 |
Polyacrylamide-agarose |
E. coli XL1 |
Differentiation of a tetracycline resistance gene29 |
Agarose |
T lymphocytes |
Secretion of cytokines: IFN-γ, IL-4, IL-10 or TGF-β30 |
Secretion of cytokines: IL-2, IFN-γ, TNF-α25 |
![[thin space (1/6-em)]](https://www.rsc.org/images/entities/char_2009.gif) |
Cell-to-cell interaction |
W/O/W |
S. aureu + microbes |
Antibiotic producer discovery32 |
S. aureus + S. venezuelae |
S. aureus + E. coli |
W/O/W |
Oral microbiota of Siberian bear + S. aureus |
Antibiotic Ami discovery31 |
Agarose |
S. cerevisiae + S. aureus |
Lytic hydrolase producer for S. aureus33 |
Agarose |
C. sorokiniana + Pseudomonas spp. |
Viable bacteria improving algal growth34 |
Agarose |
Stromal cells (MBA2) + leukemia cells (M07e) |
Impacts on M07e cells survival105 |
Agarose |
S. cerevisiae + murine Ba/F3 reporter cells |
Cytokine secretion by S. cerevisiae libraries103 |
Agarose |
P. pastoris + cancer cells (A431) |
Antibody secretion by P. pastoris libraries104 |
4.1 Drug discovery
Due to the emergence and spread of drug resistance and new diseases, there is an urgent need for new drug development. An effective technique for high-throughput drug screening plays a critical role in finding a new drug against the target of particular diseases, where diverse compound libraries are tested under different conditions in parallel with the drug target.109 Cell-based assays are always involved in this process, which provides substantive information on various cellular responses upon compound exposure, reducing the need for animal models. DFC allows high-throughput monitoring and screening of cultures of isolated single cells (e.g., bacteria cells) within droplet compartments in response to a specific drug or a combination of multiple drugs. This reveals a heterogenic reaction that cannot be obtained by traditional drug screening methods (which are based on the average of the entire population). In addition, the use of emulsion droplets enables the analysis of a single cell's anti-drug response (e.g., antibiotic resistance31,32,84). Microdroplets are also used to analyse the drug response of a cancer cell line with different phenotypes, showing a clear distinction in drug response as a function of a specific phenotype. This ability to reveal different cell line responses to the same compound allows scientists to gain important information for new drug discovery and development.
4.2 Medical diagnosis
The presence and the stage of a particular disease can be indicated by sensitive and reliable detection of specific biomarkers, bacteria and viruses. For example, the proteins secreted by cancer cells (i.e., secretomes) are a fundamental source of biomarkers.98 Although conventional immunoassays, in particular, enzyme-linked immunosorbent assay (ELISA)110,111 have been widely used to quantify protein biomarkers in bulk, the detectable concentrations of proteins are limited to generally above the picomolar range, making them unable to detect cancer biomarkers that exist in biological fluids at concentrations in the range of 10−12 to 10−16 M.112 Moreover, it is quite challenging to perform characterisation of immune responses in many cases, as it requires the isolation of individual immune cells. For instance, a diversity of T cells can be produced by human immune system, but only a fraction of them are capable of being recognised and distinguished from those infected and mutant cells. Therefore, DFC has been applied to detect low-abundance proteins secreted from single cells and to quantify single-cell immune signatures.113 Tumour heterogeneity can confound the detection and diagnosis of cancer patients, which has led to high-throughput single-cell analysis platforms to aid in the determination of cancer malignancy.114–117 Also, co-encapsulation of distinct cell types in microdroplets holds great potential for future cancer research by probing heterogeneous cell–cell interactions (e.g., cancer-immune cell interactions in cancer surveillance).
4.3 Metabolic engineering
Due to the limited knowledge of complicated cellular networks, directed evolution has played an essential role in improving the performance and functionality of industrially important microbes.118 High-throughput and cost-effective screening tools are required in the selection of super variants. Emulsion droplets provide compartmentalization of individual cells from a massive variant library containing genetic diversity. Each cell-laden droplet in aqueous suspension can be considered a distinct event in the cytometers. Selection of improved variants from the library can be carried out based on the level of phenotypes (e.g., secreted protein production), allowing to establish the linkages of phenotypes and genotypes for directed evolution of functional proteins. This mimics the process of natural evolution in a laboratory setting, which relies on iterative cycles of genetic diversity generation and phenotypic selection to isolate evolved industrial strains with desired traits. DFC has been providing an efficient screening avenue to improve microbial strains for enhanced enzymatic activity, and improved production of value-added bioproducts (e.g., vitamins and organic acids).96
4.4 Cell biology
DFC opens new opportunities in cell biology by improving our understanding of how the behaviours of individual cells shape biological processes. Microbial cells from a complex community can be randomly encapsulated into microdroplets that can be used as miniaturized compartments and screened or sorted in parallel by flow cytometers to study how the community members interact. This high-throughput approach can rapidly resolve microbial interaction networks across different initial community states, population sizes, and environments, enabling a better understanding of the critical parameters shaping the structures and functions of microbial communities.102 For example, co-encapsulation of two bacterial strains allows for discrimination between viable and non-viable cells, identifying the conditions that support the growth of unculturable cells and improved understanding of mechanisms underlying bacterial inhibitory activity.31 Moreover, co-culture of a microalgal strain with a pool of environmentally sourced bacterial cells allows the isolation of the ones that can induce a desired phenotype (i.e., improvement in algae growth rate).34
5. Current challenges and perspectives
As discussed above, the ability to screen and select microdroplets encapsulated with single cells by FC brings in new possibilities in various fields. Compared with traditional well plate assays, this integrated technique has impressive advantages, such as high throughput and low cost. However, there are still issues that limit DFC as a common technique for high-throughput single-cell analysis.
5.1 Molecule leakage
The first area that needs improvement is the transport and leakage of the molecules encapsulated within emulsion droplets. To ensure accurate and effective in-drop assays, it is essential to maintain all the contents within the droplet compartments during the process (e.g., incubation, relocation and manipulation) without any cross-contamination or analyte loss.22 Unfortunately, there is a possible leakage of small molecules from the droplets into the surrounding oil and neighbouring droplets, which may be due to the hydrophobicity and solubility of fluorophores, formation, and transport micellular or vascular structures, or media pH.50 This results in the reduction of sensitivity and resolution of biomechanical assays performed in microdroplets.
Although several approaches have been reported to increase the containment of small molecules within droplets, such as the use of fluorinated oils,119,120 decrease in the concentration of surfactants,121 and addition of bovine serum albumin (BSA) or sugar additives into the inner aqueous phase,122 some fluorophores of widely used substrates are hydrophobic and suffer rapid diffusion or exchange between droplets. One interesting approach is nanoparticles that can interface with target hydrophobic molecules to prevent their leakage.123
Another approach that may circumvent this limitation is the chemical or enzymatic modification of the hydrophobic products into hydrophilic, charged molecules that cannot diffuse into the oil shell.55 There is still a need for further development of new fluorescence dyes and metabolic biomarkers that can be confined within the droplets and new biochemical technologies that can prevent the leakage of small molecules from droplets.
5.2 Access to droplet microfluidic technology
The second important challenge is the restricted access to droplet microfluidic technology. Currently, microfluidic systems are mainly designed, fabricated and operated by specialized engineering laboratories, and the applications in life and biomedical sciences achieved are based on the collaborations with engineering research groups. Therefore, more efforts should be put into commercialising simple and cost-effective instruments and surfactants to facilitate wider adoptions, allowing users without expertise in microfluidics to carry out the wide range of applications summarised above.
We note that a few examples of droplet microfluidic technology have been successfully commercialized, e.g., DropSeq and InDrops by 10× Genomics, which enables single-molecule detection by measuring thousands of independent amplification events within a single sample. Moreover, there are many companies provide a wide catalogue of standard microfluidic chips for various applications, and help with design, prototyping and fabrication of full custom microfluidic chips that meet specific needs. It would be helpful to push some relatively basic instruments for individual applications (e.g., enzymatic assays and PCR) into the market. Also, it looks great to make the technology instruments capable of integration with the multi-well plate format, so that highly parallelized downstream procedures can be carried out with existing liquid handling robots. We foresee that the accessibility of basic microfluidic technology will be expanded to non-specialists in the broader research and clinical communities.
5.3 Optical interrogation
Another challenge is that the screening and analysis of droplet bioreactors have mainly relied on the detection of fluorescent products. Although fluorescent labelling allows multiple readouts simultaneously with high sensitivity, it is often challenging to incorporate them into biochemical assays. The native target is not fluorescently active, and adding a fluorescent dye may cause cellular cytotoxicity, photobleaching, and interface with cellular metabolism.124 Also, there are limited fluorescent dyes available to probe complex cell activities in microdroplets. Therefore, developing a label-free optical detection technology for sensitive detection and characterization of droplet bioreactors would be of great promise by expanding the available assays in droplet screening and sorting.
Different optical techniques have been used to analyse droplets beyond fluorescence detection. For example, absorbance and mass-based detection41,125 has been used for enzymatic activities in droplets, but it suffers from relatively low specificity. Although Raman spectroscopy has been applied to quantify single-cell metabolite and enzyme production in microdroplets,42,126 the relatively high level of noise and low sensitivity limit its comprehensive implementation in high-throughput assays. We note that recent advances in coherent Raman spectroscopy127–130 have enabled high-throughput (i.e., 2 kHz) and label-free analysis of heterogeneous populations at the single-cell level, which holds great promise for high-accuracy cell classification.
Moreover, imaging flow cytometry (IFC) has become an attractive tool for single-cell analysis in diverse biomedical fields, and a two-dimensional image signal has been employed for real-time classification and sorting of droplets encapsulating with cancer131,132 and planktonic cells.133 Combining with high-speed image-based sorting,134–139 this will eventually be used in the process of isolating individual cells exhibiting properties of interest. With further advances in sensitivity and specificity of label-free optical detection techniques, we expect that droplet encapsulation and compartmentalization will expand into a more versatile platform technology for high-throughput single-cell analysis.
6. Conclusions
The ability to encapsulate, cultivate, monitor and select single cells in a large number of droplet bioreactors provides new opportunities in tackling numerous problems in fundamental and applied biology and health. New toolsets and new fluorescent toolsets may deliver breakthroughs in tackling the challenges regarding transport and leakage of small molecules in droplets. The availability of droplet technology has a chance to increase via collaborations between engineers and biologists rapidly by commercializing standard instruments and chemicals (e.g., surfactants) for droplet generation and manipulation. We envision that further development of droplet systems and optical detection techniques should allow DFC to expand into a more typical and versatile platform technology for high-throughput single-cell analysis.
Conflicts of interest
There are no conflicts to declare.
Acknowledgements
This work was supported by the Australian Research Council (ARC) Discovery Project (DP200102269), JSPS Core-to-Core Program, White Rock Foundation and Macquarie University Research Seeding Grant. H. Liu was supported by International Macquarie University Research Excellence Scholarships (iMQRES) for graduate study.
References
- F. S. O. Fritzsch, C. Dusny, O. Frick and A. Schmid, Annu. Rev. Chem. Biomol. Eng., 2012, 3, 129–155 CrossRef CAS
. - G.-C. Yuan, L. Cai, M. Elowitz, T. Enver, G. Fan, G. Guo, R. Irizarry, P. Kharchenko, J. Kim, S. Orkin, J. Quackenbush, A. Saadatpour, T. Schroeder, R. Shivdasani and I. Tirosh, Genome Biol., 2017, 18, 84 CrossRef PubMed
. - H. Andersson and A. Van Den Berg, Curr. Opin. Biotechnol., 2004, 15, 44–49 CrossRef CAS PubMed
. - D. Ryan, K. Ren and H. Wu, Biomicrofluidics, 2011, 5, 021501 CrossRef PubMed
. - A. Cossarizza, H. D. Chang, A. Radbruch, A. Acs, D. Adam, S. Adam-Klages, W. W. Agace, N. Aghaeepour, M. Akdis, M. Allez, L. N. Almeida, G. Alvisi, G. Anderson, I. Andrä, F. Annunziato, A. Anselmo, P. Bacher, C. T. Baldari, S. Bari, V. Barnaba, J. Barros-Martins, L. Battistini, W. Bauer, S. Baumgart, N. Baumgarth, D. Baumjohann, B. Baying, M. Bebawy, B. Becher, W. Beisker, V. Benes, R. Beyaert, A. Blanco, D. A. Boardman, C. Bogdan, J. G. Borger, G. Borsellino, P. E. Boulais, J. A. Bradford, D. Brenner, R. R. Brinkman, A. E. S. Brooks, D. H. Busch, M. Büscher, T. P. Bushnell, F. Calzetti, G. Cameron, I. Cammarata, X. Cao, S. L. Cardell, S. Casola, M. A. Cassatella, A. Cavani, A. Celada, L. Chatenoud, P. K. Chattopadhyay, S. Chow, E. Christakou, L. Čičin-Šain, M. Clerici, F. S. Colombo, L. Cook, A. Cooke, A. M. Cooper, A. J. Corbett, A. Cosma, L. Cosmi, P. G. Coulie, A. Cumano, L. Cvetkovic, V. D. Dang, C. Dang-Heine, M. S. Davey, D. Davies, S. De Biasi, G. Del Zotto, G. V. Dela Cruz, M. Delacher, S. Della Bella, P. Dellabona, G. Deniz, M. Dessing, J. P. Di Santo, A. Diefenbach, F. Dieli, A. Dolf, T. Dörner, R. J. Dress, D. Dudziak, M. Dustin, C. A. Dutertre, F. Ebner, S. B. G. Eckle, M. Edinger, P. Eede, G. R. A. Ehrhardt, M. Eich, P. Engel, B. Engelhardt, A. Erdei, C. Esser, B. Everts, M. Evrard, C. S. Falk, T. A. Fehniger, M. Felipo-Benavent, H. Ferry, M. Feuerer, A. Filby, K. Filkor, S. Fillatreau, M. Follo, I. Förster, J. Foster, G. A. Foulds, B. Frehse, P. S. Frenette, S. Frischbutter, W. Fritzsche, D. W. Galbraith, A. Gangaev, N. Garbi, B. Gaudilliere, R. T. Gazzinelli, J. Geginat, W. Gerner, N. A. Gherardin, K. Ghoreschi, L. Gibellini, F. Ginhoux, K. Goda, D. I. Godfrey, C. Goettlinger, J. M. González-Navajas, C. S. Goodyear, A. Gori, J. L. Grogan, D. Grummitt, A. Grützkau, C. Haftmann, J. Hahn, H. Hammad, G. Hämmerling, L. Hansmann, G. Hansson, C. M. Harpur, S. Hartmann, A. Hauser, A. E. Hauser, D. L. Haviland, D. Hedley, D. C. Hernández, G. Herrera, M. Herrmann, C. Hess, T. Höfer, P. Hoffmann, K. Hogquist, T. Holland, T. Höllt, R. Holmdahl, P. Hombrink, J. P. Houston, B. F. Hoyer, B. Huang, F. P. Huang, J. E. Huber, J. Huehn, M. Hundemer, C. A. Hunter, W. Y. K. Hwang, A. Iannone, F. Ingelfinger, S. M. Ivison, H. M. Jäck, P. K. Jani, B. Jávega, S. Jonjic, T. Kaiser, T. Kalina, T. Kamradt, S. H. E. Kaufmann, B. Keller, S. L. C. Ketelaars, A. Khalilnezhad, S. Khan, J. Kisielow, P. Klenerman, J. Knopf, H. F. Koay, K. Kobow, J. K. Kolls, W. T. Kong, M. Kopf, T. Korn, K. Kriegsmann, H. Kristyanto, T. Kroneis, A. Krueger, J. Kühne, C. Kukat, D. Kunkel, H. Kunze-Schumacher, T. Kurosaki, C. Kurts, P. Kvistborg, I. Kwok, J. Landry, O. Lantz, P. Lanuti, F. LaRosa, A. Lehuen, S. LeibundGut-Landmann, M. D. Leipold, L. Y. T. Leung, M. K. Levings, A. C. Lino, F. Liotta, V. Litwin, Y. Liu, H. G. Ljunggren, M. Lohoff, G. Lombardi, L. Lopez, M. López-Botet, A. E. Lovett-Racke, E. Lubberts, H. Luche, B. Ludewig, E. Lugli, S. Lunemann, H. T. Maecker, L. Maggi, O. Maguire, F. Mair, K. H. Mair, A. Mantovani, R. A. Manz, A. J. Marshall, A. Martínez-Romero, G. Martrus, I. Marventano, W. Maslinski, G. Matarese, A. V. Mattioli, C. Maueröder, A. Mazzoni, J. McCluskey, M. McGrath, H. M. McGuire, I. B. McInnes, H. E. Mei, F. Melchers, S. Melzer, D. Mielenz, S. D. Miller, K. H. G. Mills, H. Minderman, J. Mjösberg, J. Moore, B. Moran, L. Moretta, T. R. Mosmann, S. Müller, G. Multhoff, L. E. Muñoz, C. Münz, T. Nakayama, M. Nasi, K. Neumann, L. G. Ng, A. Niedobitek, S. Nourshargh, G. Núñez, J. E. O'Connor, A. Ochel, A. Oja, D. Ordonez, A. Orfao, E. Orlowski-Oliver, W. Ouyang, A. Oxenius, R. Palankar, I. Panse, K. Pattanapanyasat, M. Paulsen, D. Pavlinic, L. Penter, P. Peterson, C. Peth, J. Petriz, F. Piancone, W. F. Pickl, S. Piconese, M. Pinti, A. G. Pockley, M. J. Podolska, Z. Poon, K. Pracht, I. Prinz, C. E. M. Pucillo, S. A. Quataert, L. Quatrini, K. M. Quinn, H. Radbruch, T. R. D. J. Radstake, S. Rahmig, H. P. Rahn, B. Rajwa, G. Ravichandran, Y. Raz, J. A. Rebhahn, D. Recktenwald, D. Reimer, C. Reis e Sousa, E. B. M. Remmerswaal, L. Richter, L. G. Rico, A. Riddell, A. M. Rieger, J. P. Robinson, C. Romagnani, A. Rubartelli, J. Ruland, A. Saalmüller, Y. Saeys, T. Saito, S. Sakaguchi, F. Sala-de-Oyanguren, Y. Samstag, S. Sanderson, I. Sandrock, A. Santoni, R. B. Sanz, M. Saresella, C. Sautes-Fridman, B. Sawitzki, L. Schadt, A. Scheffold, H. U. Scherer, M. Schiemann, F. A. Schildberg, E. Schimisky, A. Schlitzer, J. Schlosser, S. Schmid, S. Schmitt, K. Schober, D. Schraivogel, W. Schuh, T. Schüler, R. Schulte, A. R. Schulz, S. R. Schulz, C. Scottá, D. Scott-Algara, D. P. Sester, T. V. Shankey, B. Silva-Santos, A. K. Simon, K. M. Sitnik, S. Sozzani, D. E. Speiser, J. Spidlen, A. Stahlberg, A. M. Stall, N. Stanley, R. Stark, C. Stehle, T. Steinmetz, H. Stockinger, Y. Takahama, K. Takeda, L. Tan, A. Tárnok, G. Tiegs, G. Toldi, J. Tornack, E. Traggiai, M. Trebak, T. I. M. Tree, J. Trotter, J. Trowsdale, M. Tsoumakidou, H. Ulrich, S. Urbanczyk, W. van de Veen, M. van den Broek, E. van der Pol, S. Van Gassen, G. Van Isterdael, R. A. W. van Lier, M. Veldhoen, S. Vento-Asturias, P. Vieira, D. Voehringer, H. D. Volk, A. von Borstel, K. von Volkmann, A. Waisman, R. V. Walker, P. K. Wallace, S. A. Wang, X. M. Wang, M. D. Ward, K. A. Ward-Hartstonge, K. Warnatz, G. Warnes, S. Warth, C. Waskow, J. V. Watson, C. Watzl, L. Wegener, T. Weisenburger, A. Wiedemann, J. Wienands, A. Wilharm, R. J. Wilkinson, G. Willimsky, J. B. Wing, R. Winkelmann, T. H. Winkler, O. F. Wirz, A. Wong, P. Wurst, J. H. M. Yang, J. Yang, M. Yazdanbakhsh, L. Yu, A. Yue, H. Zhang, Y. Zhao, S. M. Ziegler, C. Zielinski, J. Zimmermann and A. Zychlinsky, Eur. J. Immunol., 2019, 49, 1457–1973 CrossRef CAS PubMed
. - Y. Saeys, S. Van Gassen and B. N. Lambrecht, Nat. Rev. Immunol., 2016, 16, 449–462 CrossRef CAS PubMed
. - E. Lugli, M. Roederer and A. Cossarizza, Cytometry, Part A, 2010, 77, 705–713 CrossRef PubMed
. - W. A. Bonner, H. R. Hulett, R. G. Sweet and L. A. Herzenberg, Rev. Sci. Instrum., 1972, 43, 404–409 CrossRef CAS PubMed
. - S. C. De Rosa, L. A. Herzenberg, L. A. Herzenberg and M. Roederer, Nat. Med., 2001, 7, 245–248 CrossRef CAS PubMed
. - S. P. Perfetto, P. K. Chattopadhyay and M. Roederer, Nat. Rev. Immunol., 2004, 4, 648–655 CrossRef CAS PubMed
. - S. Picelli, Å. K. Björklund, O. R. Faridani, S. Sagasser, G. Winberg and R. Sandberg, Nat. Methods, 2013, 10, 1096–1100 CrossRef CAS PubMed
. - G. S. Karagiannis, M. P. Pavlou and E. P. Diamandis, Mol. Oncol., 2010, 4, 496–510 CrossRef CAS PubMed
. - R. Singh, M. Kumar, A. Mittal and P. K. Mehta, 3 Biotech, 2017, 7, 15 CrossRef PubMed
. - G. Damia and M. D'Incalci, Eur. J. Cancer, 2009, 45, 2768–2781 CrossRef CAS PubMed
. - A. Adan, Y. Kiraz and Y. Baran, Curr. Pharm. Biotechnol., 2016, 17, 1213–1221 CAS
. - H. N. Joensson and H. Andersson Svahn, Angew. Chem., Int. Ed., 2012, 51, 12176–12192 CrossRef CAS PubMed
. - A. Rakszewska, J. Tel, V. Chokkalingam and W. T. S. Huck, NPG Asia Mater., 2014, 6, 133 CrossRef
. - K. K. Brower, M. Khariton, P. H. Suzuki, C. Still, G. Kim, S. G. K. Calhoun, L. S. Qi, B. Wang and P. M. Fordyce, Anal. Chem., 2020, 92, 13262–13270 CrossRef CAS PubMed
. - Z. Zhu and C. J. Yang, Acc. Chem. Res., 2017, 50, 22–31 CrossRef CAS PubMed
. - T. Stuart and R. Satija, Nat. Rev. Genet., 2019, 20, 257–272 CrossRef CAS PubMed
. - R. Vanella, D. T. Ta and M. A. Nash, Biotechnol. Bioeng., 2019, 116, 1878–1886 CrossRef CAS PubMed
. - R. Tu, R. Martinez, R. Prodanovic, M. Klein and U. Schwaneberg, J. Biomol. Screening, 2011, 16, 285–294 CrossRef CAS PubMed
. - E. Hardiman, M. Gibbs, R. Reeves and P. Bergquist, Appl. Biochem. Biotechnol., 2010, 161, 301–312 CrossRef CAS PubMed
. - R. D. Gupta, M. Goldsmith, Y. Ashani, Y. Simo, G. Mullokandov, H. Bar, M. Ben-David, H. Leader, R. Margalit, I. Silman, J. L. Sussman and D. S. Tawfik, Nat. Chem. Biol., 2011, 7, 120–125 CrossRef CAS PubMed
. - V. Chokkalingam, J. Tel, F. Wimmers, X. Liu, S. Semenov, J. Thiele, C. G. Figdor and W. T. S. Huck, Lab Chip, 2013, 13, 4740–4744 RSC
. - L. Qiu, F. Wimmers, J. Weiden, H. A. Heus, J. Tel and C. G. Figdor, Chem. Commun., 2017, 53, 8066–8069 RSC
. - Z. Zhu, W. Zhang, X. Leng, M. Zhang, Z. Guan, J. Lu and C. J. Yang, Lab Chip, 2012, 12, 3907–3913 RSC
. - H. Zhang, G. Jenkins, Y. Zou, Z. Zhu and C. J. Yang, Anal. Chem., 2012, 84, 3599–3606 CrossRef CAS PubMed
. - M. V. Tamminen and M. P. J. Virta, Front. Microb., 2015, 6, 195 Search PubMed
. - V. Turcanu and N. A. Williams, Nat. Med., 2001, 7, 373–376 CrossRef CAS PubMed
. - S. S. Terekhov, I. V. Smirnov, M. V. Malakhova, A. E. Samoilov, A. I. Manolo, A. S. Nazarov, D. V. Danilov, S. A. Dubiley, I. A. Osterman, M. P. Rubtsova, E. S. Kostryukova, R. H. Ziganshin, M. A. Kornienko, A. A. Vanyushkina, O. N. Bukato, E. N. Ilina, V. V. Vlasov, K. V. Severinov, A. G. Gabibov and S. Altmani, Proc. Natl. Acad. Sci. U. S. A., 2018, 115, 9551–9556 CrossRef CAS PubMed
. - S. S. Terekhov, I. V. Smirnov, A. V. Stepanova, T. V. Bobik, Y. A. Mokrushina, N. A. Ponomarenko, A. A. Belogurov, M. P. Rubtsova, O. V. Kartseva, M. O. Gomzikova, A. A. Moskovtsev, A. S. Bukatin, M. V. Dubina, E. S. Kostryukova, V. V. Babenko, M. T. Vakhitova, A. I. Manolov, M. V. Malakhova, M. A. Kornienko, A. V. Tyakht, A. A. Vanyushkina, E. N. Ilina, P. Masson, A. G. Gabibov and S. Altman, Proc. Natl. Acad. Sci. U. S. A., 2017, 114, 2550–2555 CrossRef CAS PubMed
. - T. C. Scanlon, S. M. Dostal and K. E. Griswold, Biotechnol. Bioeng., 2014, 111, 232–243 CrossRef CAS PubMed
. - J. Ohan, B. Pelle, P. Nath, J.-H. Huang, B. Hovde, M. Vuyisich, A. E. Dichosa and S. R. Starkenburg, BioTechniques, 2019, 66, 218–224 CrossRef CAS PubMed
. - J. C. Baret, O. J. Miller, V. Taly, M. Ryckelynck, A. El-Harrak, L. Frenz, C. Rick, M. L. Samuels, J. B. Hutchison, J. J. Agresti, D. R. Link, D. A. Weitz and A. D. Griffiths, Lab Chip, 2009, 9, 1850–1858 RSC
. - D. Vallejo, A. Nikoomanzar, B. M. Paegel and J. C. Chaput, ACS Synth. Biol., 2019, 8, 1430–1440 CrossRef CAS PubMed
. - A. Isozaki, A. Isozaki, Y. Nakagawa, M. H. Loo, Y. Shibata, N. Tanaka, D. L. Setyaningrum, J. W. Park, Y. Shirasaki, H. Mikami, D. Huang, H. Tsoi, C. T. Riche, T. Ota, H. Miwa, Y. Kanda, T. Ito, T. Ito, K. Yamada, O. Iwata, K. Suzuki, S. Ohnuki, Y. Ohya, Y. Ohya, Y. Kato, T. Hasunuma, T. Hasunuma, S. Matsusaka, M. Yamagishi, M. Yazawa, S. Uemura, K. Nagasawa, H. Watarai, H. Watarai, D. Di Carlo, K. Goda, K. Goda, K. Goda and K. Goda, Sci. Adv., 2020, 6, eaba6712 CrossRef CAS PubMed
. - A. Isozaki, D. Huang, Y. Nakagawa and K. Goda, Microfluid. Nanofluid., 2021, 25, 32 CrossRef
. - R. H. Cole, S. Y. Tang, C. A. Siltanen, P. Shahi, J. Q. Zhang, S. Poust, Z. J. Gartner and A. R. Abate, Proc. Natl. Acad. Sci. U. S. A., 2017, 114, 8728–8733 CrossRef CAS PubMed
. - A. Sciambi and A. R. Abate, Lab Chip, 2015, 15, 47–51 RSC
. - F. Gielen, R. Hours, S. Emond, M. Fischlechner, U. Schell and F. Hollfelder, Proc. Natl. Acad. Sci. U. S. A., 2016, 113, E7383–E7389 CrossRef CAS PubMed
. - X. Wang, L. Ren, Y. Su, Y. Ji, Y. Liu, C. Li, X. Li, Y. Zhang, W. Wang, Q. Hu, D. Han, J. Xu and B. Ma, Anal. Chem., 2017, 89, 12569–12577 CrossRef CAS PubMed
. - A. T. Florence and D. Whitehill, Int. J. Pharm., 1982, 11, 277–308 CrossRef CAS
. - N. Garti, Colloids Surf., A, 1997, 123–124, 233–246 CrossRef CAS
. - R. T. Davies, D. Kim and J. Park, J. Micromech. Microeng., 2012, 22, 055003 CrossRef
. - Z. Bai, B. Wang, H. Chen and M. Wang, Sens. Actuators, B, 2015, 215, 330–336 CrossRef CAS
. - S. C. Kim, D. J. Sukovich and A. R. Abate, Lab Chip, 2015, 15, 3163–3169 RSC
. - K. K. Brower, M. Khariton, P. H. Suzuki, C. Still, G. Kim, S. G. K. K. Calhoun, L. S. Qi, B. Wang and P. M. Fordyce, Anal. Chem., 2020, 92, 13262–13270 CrossRef CAS PubMed
. - K. K. Brower, K. K. Brower, C. Carswell-Crumpton, S. Klemm, B. Cruz, G. Kim, S. G. K. Calhoun, L. Nichols, P. M. Fordyce, P. M. Fordyce, P. M. Fordyce and P. M. Fordyce, Lab Chip, 2020, 20, 2062–2074 RSC
. - A. Zinchenko, S. R. A. A. Devenish, B. Kintses, P.-Y. Y. Colin, M. Fischlechner and F. Hollfelder, Anal. Chem., 2014, 86, 2526–2533 CrossRef CAS PubMed
. - E. Tumarkin, L. Tzadu, E. Csaszar, M. Seo, H. Zhang, A. Lee, R. Peerani, K. Purpura, P. W. Zandstra and E. Kumacheva, Integr. Biol., 2011, 3, 653 CrossRef CAS PubMed
. - T. C. Scanlon, S. M. Dostal and K. E. Griswold, Biotechnol. Bioeng., 2014, 111, 232–243 CrossRef CAS PubMed
. - E. Mastrobattista, V. Taly, E. Chanudet, P. Treacy, B. T. Kelly and A. D. Griffiths, Chem. Biol., 2005, 12, 1291–1300 CrossRef CAS PubMed
. - A. Aharoni, G. Amitai, K. Bernath, S. Magdassi and D. S. Tawfik, Chem. Biol., 2005, 12, 1281–1289 CrossRef CAS PubMed
. - F. Ma, M. Fischer, Y. Han, S. G. Withers, Y. Feng and G. Y. Yang, Anal. Chem., 2016, 88, 8587–8595 CrossRef CAS PubMed
. - Y. Zhang, Y. P. Ho, Y. L. Chiu, H. F. Chan, B. Chlebina, T. Schuhmann, L. You and K. W. Leong, Biomaterials, 2013, 34, 4564–4572 CrossRef CAS PubMed
. - K. Bernath, M. Hai, E. Mastrobattista, A. D. Griffiths, S. Magdassi and D. S. Tawfik, Anal. Biochem., 2004, 325, 151–157 CrossRef CAS PubMed
. - D. J. Sukovich, S. C. Kim, N. Ahmed and A. R. Abate, Analyst, 2017, 142, 4618–4622 RSC
. - J. Ohan, B. Pelle, P. Nath, J.-H. Huang, B. Hovde, M. Vuyisich, A. E. Dichosa and S. R. Starkenburg, BioTechniques, 2019, 66, 218–224 CrossRef CAS PubMed
. - C. T. Riche, E. J. Roberts, M. Gupta, R. L. Brutchey and N. Malmstadt, Nat. Commun., 2016, 7, 1–7 Search PubMed
. - M. A. J. Mazumder, N. A. D. Burke, F. Shen, M. A. Potter and H. D. H. Stöver, Biomacromolecules, 2009, 10, 1365–1373 CrossRef CAS PubMed
. - S. A. Hamad, A. F. K. Dyab, S. D. Stoyanov and V. N. Paunov, J. Mater. Chem., 2011, 21, 18018–18023 RSC
. - T. Trongsatitkul and B. M. Budhlall, Langmuir, 2011, 27, 13468–13480 CrossRef CAS PubMed
. - T. Rossow, J. A. Heyman, A. J. Ehrlicher, A. Langhoff, D. A. Weitz, R. Haag and S. Seiffert, J. Am. Chem. Soc., 2012, 134, 4983–4989 CrossRef CAS PubMed
. - P. H. R. Keen, N. K. H. Slater and A. F. Routh, Langmuir, 2014, 30, 1939–1948 CrossRef CAS PubMed
. - E. Walter, K. Moelling, J. Pavlovic and H. P. Merkle, J. Controlled Release, 1999, 61, 361–374 CrossRef CAS PubMed
. - D. Wang, D. R. Robinson, G. S. Kwon and J. Samuel, J. Controlled Release, 1999, 57, 9–18 CrossRef CAS PubMed
. - E. Walter, D. Dreher, M. Kok, L. Thiele, S. G. Kiama, P. Gehr and H. P. Merkle, J. Controlled Release, 2001, 76, 149–168 CrossRef CAS PubMed
. - C. Wang, Q. Ge, D. Ting, D. Nguyen, H. R. Shen, J. Chen, H. N. Eisen, J. Heller, R. Langer and D. Putnam, Nat. Mater., 2004, 3, 190–196 CrossRef CAS PubMed
. - Y. Y. Yang, T. S. Chung, X. L. Bai and W. K. Chan, Chem. Eng. Sci., 2000, 55, 2223–2236 CrossRef CAS
. - N. G. Balabushevitch, G. B. Sukhorukov, N. A. Moroz, D. V. Volodkin, N. I. Larionova, E. Donath and H. Mohwald, Biotechnol. Bioeng., 2001, 76, 207–213 CrossRef CAS PubMed
. - N. Elvassore, A. Bertucco and P. Caliceti, Ind. Eng. Chem. Res., 2001, 40, 795–800 CrossRef CAS
. - D. A. Christian, S. Cai, D. M. Bowen, Y. Kim, J. D. Pajerowski and D. E. Discher, Eur. J. Pharm. Biopharm., 2009, 71, 463–474 CrossRef CAS PubMed
. - S. Bin Wang, F. H. Xu, H. S. He and L. J. Weng, Macromol. Biosci., 2005, 5, 408–414 CrossRef PubMed
. - C. Wang, W. Ye, Y. Zheng, X. Liu and Z. Tong, Int. J. Pharm., 2007, 338, 165–173 CrossRef CAS PubMed
. - W. Song, Q. He, H. Möhwald, Y. Yang and J. Li, J. Controlled Release, 2009, 139, 160–166 CrossRef CAS PubMed
. - Q. Zhao, B. Han, Z. Wang, C. Gao, C. Peng and J. Shen, Nanomed. Cancer, 2017, 3, 317–345 Search PubMed
. - M. Rinaudo, Polym. Int., 2008, 57, 397–430 CrossRef CAS
. - M. Li, M. van Zee, C. T. Riche, B. Tofig, S. D. Gallaher, S. S. Merchant, R. Damoiseaux, K. Goda and D. Di Carlo, Small, 2018, 14, 1803315 CrossRef PubMed
. - A. Khademhosseini, J. Yeh, S. Jon, G. Eng, K. Y. Suh, J. A. Burdick and R. Langer, Lab Chip, 2004, 4, 425–430 RSC
. - D. L. Hern and J. A. Hubbell, J. Biomed. Mater. Res., 1998, 39, 266–276 CrossRef CAS PubMed
. - A. D. Griffiths and D. S. Tawfik, Trends Biotechnol., 2006, 24, 395–402 CrossRef CAS PubMed
. - R. K. Shah, H. C. Shum, A. C. Rowat, D. Lee, J. J. Agresti, A. S. Utada, L. Y. Chu, J. W. Kim, A. Fernandez-Nieves, C. J. Martinez and D. A. Weitz, Mater. Today, 2008, 11, 18–27 CrossRef CAS
. - Y. J. J. Eun, A. S. Utada, M. F. Copeland, S. Takeuchi and D. B. Weibel, ACS Chem. Biol., 2011, 6, 260–266 CrossRef CAS PubMed
. - A. Aharoni, A. D. Griffiths and D. S. Tawfik, Curr. Opin. Chem. Biol., 2005, 9, 210–216 CrossRef CAS PubMed
. - G. Georgiou, Adv. Protein Chem., 2001, 55, 293–315 CrossRef
. - Y. Hwang, Biotechnol. Bioprocess Eng., 2012, 17, 500–505 CrossRef
. - R. Ostafe, R. Prodanovic, U. Commandeur and R. Fischer, Anal. Biochem., 2013, 435, 93–98 CrossRef CAS PubMed
. - R. Ostafe, R. Prodanovic, J. Nazor and R. Fischer, Chem. Biol., 2014, 21, 414–421 CrossRef CAS PubMed
. - F. Ma, Y. Xie, C. Huang, Y. Feng and G. Yang, PLoS One, 2014, 9, e89785 CrossRef PubMed
. - F. Ma, T. Guo, Y. Zhang, X. Bai, C. Li, Z. Lu, X. Deng, D. Li, K. Kurabayashi and G. Yang, Environ. Microbiol., 2021, 23, 996–1008 CrossRef CAS PubMed
. - A. C. Larsen, M. R. Dunn, A. Hatch, S. P. Sau, C. Youngbull and J. C. Chaput, Nat. Commun., 2016, 7, 1–9 Search PubMed
. - M. Fischlechner, Y. Schaerli, M. F. Mohamed, S. Patil, C. Abell and F. Hollfelder, Nat. Chem., 2014, 6, 791–796 CrossRef CAS PubMed
. - C. Pitzler, G. Wirtz, L. Vojcic, S. Hiltl, A. Böker, R. Martinez and U. Schwaneberg, Chem. Biol., 2014, 21, 1733–1742 CrossRef CAS PubMed
. - S. Di Girolamo, C. Puorger and G. Lipps, Microb. Cell Fact., 2020, 19, 1–13 CrossRef PubMed
. - J. M. Wagner, L. Liu, S. F. Yuan, M. V. Venkataraman, A. R. Abate and H. S. Alper, Metab. Eng., 2018, 47, 346–356 CrossRef CAS PubMed
. - X. D. Zhu, X. Shi, S. W. Wang, J. Chu, W. H. Zhu, B. C. Ye, P. Zuo and Y.
H. Wang, RSC Adv., 2019, 9, 4507–4513 RSC
. - M. Stastna and J. E. Van Eyk, Proteomics, 2012, 12, 722–735 CrossRef CAS PubMed
. - C. L. Sawyers, Nature, 2008, 452, 548–552 CrossRef CAS PubMed
. - D. Duijvesz, T. Luider, C. H. Bangma and G. Jenster, Eur. Urol., 2011, 59, 823–831 CrossRef CAS PubMed
. - X. Yang, W. Liu, D. C.-H. Chan, S. U. Ahmed, H. Wang, Z. Wang, C. R. Nemr and S. O. Kelley, J. Am. Chem. Soc., 2020, 142, 14805–14809 CrossRef CAS PubMed
. - R. H. Hsu, R. L. Clark, J. W. Tan, J. C. Ahn, S. Gupta, P. A. Romero and O. S. Venturelli, Cell Syst., 2019, 9, 229–242 CrossRef CAS PubMed
. - D. Yanakieva, A. Elter, J. Bratsch, K. Friedrich, S. Becker and H. Kolmar, Sci. Rep., 2020, 10, 1–13 CrossRef PubMed
. - Y. Fang, T. H. Chu, M. E. Ackerman and K. E. Griswold, mAbs, 2017, 9, 1253–1261 CrossRef CAS PubMed
. - E. Tumarkin, L. Tzadu, E. Csaszar, M. Seo, H. Zhang, A. Lee, R. Peerani, K. Purpura, P. W. Zandstra and E. Kumacheva, Integr. Biol., 2011, 3, 653 CrossRef CAS PubMed
. - E. Armingol, A. Officer, O. Harismendy and N. E. Lewis, Nat. Rev. Genet., 2021, 22, 71–88 CrossRef CAS PubMed
. - T. Nakahara, H. Uchi, A. M. Lesokhin, F. Avogadri, G. A. Rizzuto, D. Hirschhorn-Cymerman, K. S. Panageas, T. Merghoub, J. D. Wolchok and A. N. Houghton, Blood, 2010, 115, 4384–4392 CrossRef CAS PubMed
. - I. N. Druzhkova, M. V. Shirmanova, M. M. Lukina, V. V. Dudenkova, N. M. Mishina and E. V. Zagaynova, Cell Cycle, 2016, 15, 1257–1266 CrossRef CAS PubMed
. - J. Bajorath, Nat. Rev. Drug Discovery, 2002, 1, 882–894 CrossRef CAS PubMed
. - A. Gonzalez-Pujana, K. H. Vining, D. K. Y. Zhang, E. Santos-Vizcaino, M. Igartua, R. M. Hernandez and D. J. Mooney, Biomaterials, 2020, 257, 120266 CrossRef CAS PubMed
. - X. Yao, Y. Liu, J. Gao, L. Yang, D. Mao, C. Stefanitsch, Y. Li, J. Zhang, L. Ou, D. Kong, Q. Zhao and Z. Li, Biomaterials, 2015, 60, 130–140 CrossRef CAS PubMed
. - M. Baker, Nat. Methods, 2012, 9, 541–544 CrossRef CAS
. - B. J. Hindson, K. D. Ness, D. A. Masquelier, P. Belgrader, N. J. Heredia, A. J. Makarewicz, I. J. Bright, M. Y. Lucero, A. L. Hiddessen, T. C. Legler, T. K. Kitano, M. R. Hodel, J. F. Petersen, P. W. Wyatt, E. R. Steenblock, P. H. Shah, L. J. Bousse, C. B. Troup, J. C. Mellen, D. K. Wittmann, N. G. Erndt, T. H. Cauley, R. T. Koehler, A. P. So, S. Dube, K. A. Rose, L. Montesclaros, S. Wang, D. P. Stumbo, S. P. Hodges, S. Romine, F. P. Milanovich, H. E. White, J. F. Regan, G. A. Karlin-Neumann, C. M. Hindson, S. Saxonov and B. W. Colston, Anal. Chem., 2011, 83, 8604–8610 CrossRef CAS PubMed
. - T. Takahama, K. Sakai, M. Takeda, K. Azuma, T. Hida, M. Hirabayashi, T. Oguri, H. Tanaka, N. Ebi, T. Sawa, A. Bessho, M. Tachihara, H. Akamatsu, S. Bandoh, D. Himeji, T. Ohira, M. Shimokawa, Y. Nakanishi, K. Nakagawa and K. Nishio, Oncotarget, 2016, 7, 58492–58499 CrossRef PubMed
. - K. S. Thress, R. Brant, T. H. Carr, S. Dearden, S. Jenkins, H. Brown, T. Hammett, M. Cantarini and J. C. Barrett, Lung Cancer, 2015, 90, 509–515 CrossRef PubMed
. - N. J. Heredia, P. Belgrader, S. Wang, R. Koehler, J. Regan, A. M. Cosman, S. Saxonov, B. Hindson, S. C. Tanner, A. S. Brown and G. Karlin-Neumann, Methods, 2013, 59, S20–S23 CrossRef CAS PubMed
. - J. H. van Ginkel, M. M. H. Huibers, R. J. J. van Es, R. de Bree and S. M. Willems, BMC Cancer, 2017, 17, 428 CrossRef PubMed
. - M. Wang, T. Si and H. Zhao, Bioresour. Technol., 2012, 115, 117–125 CrossRef CAS PubMed
. - C. Holtze, A. C. Rowat, J. J. Agresti, J. B. Hutchison, F. E. Angilè, C. H. J. Schmitz, S. Köster, H. Duan, K. J. Humphry, R. A. Scanga, J. S. Johnson, D. Pisignano and D. A. Weitz, Lab Chip, 2008, 8, 1632–1639 RSC
. - J. C. Baret, Lab Chip, 2012, 12, 422–433 RSC
. - J. Wu and G. H. Ma, Small, 2016, 12, 4633–4648 CrossRef CAS PubMed
. - B. Müller-Hill and J. Kania, Nature, 1974, 249, 561–563 CrossRef PubMed
. - K. Yin, X. Zeng, X. Liang, H. Wei, H. Zeng, W. Qi, W. Ruan, Y. Song, C. Yang and Z. Zhu, Sci. China: Chem., 2020, 63, 1507–1514 CrossRef CAS
. - J. W. Lichtman and J. A. Conchello, Nat. Methods, 2005, 2, 910–919 CrossRef CAS PubMed
. - D. A. Holland-Moritz, M. K. Wismer, B. F. Mann, I. Farasat, P. Devine, E. D. Guetschow, I. Mangion, C. J. Welch, J. C. Moore, S. Sun and R. T. Kennedy, Angew. Chem., Int. Ed., 2020, 59, 4470–4477 CrossRef CAS PubMed
. - X. Wang, Y. Xin, L. Ren, Z. Sun, P. Zhu, Y. Ji, C. Li, J. Xu and B. Ma, Sci. Adv., 2020, 6, 3521–3528 CrossRef PubMed
. - N. Nitta, T. Iino, A. Isozaki, M. Yamagishi, Y. Kitahama, S. Sakuma, Y. Suzuki, H. Tezuka, M. Oikawa, F. Arai, T. Asai, D. Deng, H. Fukuzawa, M. Hase, T. Hasunuma, T. Hayakawa, K. Hiraki, K. Hiramatsu, Y. Hoshino, M. Inaba, Y. Inoue, T. Ito, M. Kajikawa, H. Karakawa, Y. Kasai, Y. Kato, H. Kobayashi, C. Lei, S. Matsusaka, H. Mikami, A. Nakagawa, K. Numata, T. Ota, T. Sekiya, K. Shiba, Y. Shirasaki, N. Suzuki, S. Tanaka, S. Ueno, H. Watarai, T. Yamano, M. Yazawa, Y. Yonamine, D. Di Carlo, Y. Hosokawa, S. Uemura, T. Sugimura, Y. Ozeki and K. Goda, Nat. Commun., 2020, 11, 1–16 Search PubMed
. - K. Hiramatsu, K. Yamada, M. Lindley, K. Suzuki and K. Goda, Biomed. Opt. Express, 2020, 11, 1752 CrossRef CAS PubMed
. - K. Hiramatsu, T. Ideguchi, Y. Yonamine, S. W. Lee, Y. Luo, K. Hashimoto, T. Ito, M. Hase, J. W. Park, Y. Kasai, S. Sakuma, T. Hayakawa, F. Arai, Y. Hoshino and K. Goda, Sci. Adv., 2019, 5, 241–257 Search PubMed
. - Y. Suzuki, K. Kobayashi, Y. Wakisaka, D. Deng, S. Tanaka, C. J. Huang, C. Lei, C. W. Sun, H. Liu, Y. Fujiwaki, S. Lee, A. Isozaki, Y. Kasai, T. Hayakawa, S. Sakuma, F. Arai, K. Koizumi, H. Tezuka, M. Inaba, K. Hiraki, T. Ito, M. Hase, S. Matsusaka, K. Shiba, K. Suga, M. Nishikawa, M. Jona, Y. Yatomi, Y. Yalikun, Y. Tanaka, T. Sugimura, N. Nitta, K. Goda and Y. Ozeki, Proc. Natl. Acad. Sci. U. S. A., 2019, 116, 15842–15848 CrossRef CAS PubMed
. - V. Anagnostidis, B. Sherlock, J. Metz, P. Mair, F. Hollfelder and F. Gielen, Lab Chip, 2020, 20, 889–900 RSC
. - M. Sesen and G. Whyte, Sci. Rep., 2020, 10, 1–14 CrossRef PubMed
. - M. Girault, H. Kim, H. Arakawa, K. Matsuura, M. Odaka, A. Hattori, H. Terazono and K. Yasuda, Sci. Rep., 2017, 7, 1–10 CrossRef PubMed
. - A. Isozaki, H. Mikami, K. Hiramatsu, S. Sakuma, Y. Kasai, T. Iino, T. Yamano, A. Yasumoto, Y. Oguchi, N. Suzuki, Y. Shirasaki, T. Endo, T. Ito, K. Hiraki, M. Yamada, S. Matsusaka, T. Hayakawa, H. Fukuzawa, Y. Yatomi, F. Arai, D. Di Carlo, A. Nakagawa, Y. Hoshino, Y. Hosokawa, S. Uemura, T. Sugimura, Y. Ozeki, N. Nitta and K. Goda, Nat. Protoc., 2019, 14, 2370–2415 CrossRef CAS PubMed
. - N. Nitta, T. Sugimura, A. Isozaki, H. Mikami, K. Hiraki, S. Sakuma, T. Iino, F. Arai, T. Endo, Y. Fujiwaki, H. Fukuzawa, M. Hase, T. Hayakawa, K. Hiramatsu, Y. Hoshino, M. Inaba, T. Ito, H. Karakawa, Y. Kasai, K. Koizumi, S. W. Lee, C. Lei, M. Li, T. Maeno, S. Matsusaka, D. Murakami, A. Nakagawa, Y. Oguchi, M. Oikawa, T. Ota, K. Shiba, H. Shintaku, Y. Shirasaki, K. Suga, Y. Suzuki, N. Suzuki, Y. Tanaka, H. Tezuka, C. Toyokawa, Y. Yalikun, M. Yamada, M. Yamagishi, T. Yamano, A. Yasumoto, Y. Yatomi, M. Yazawa, D. Di Carlo, Y. Hosokawa, S. Uemura, Y. Ozeki and K. Goda, Cell, 2018, 175, 266–276 CrossRef CAS PubMed
. - H. Mikami, M. Kawaguchi, C. J. Huang, H. Matsumura, T. Sugimura, K. Huang, C. Lei, S. Ueno, T. Miura, T. Ito, K. Nagasawa, T. Maeno, H. Watarai, M. Yamagishi, S. Uemura, S. Ohnuki, Y. Ohya, H. Kurokawa, S. Matsusaka, C. W. Sun, Y. Ozeki and K. Goda, Nat. Commun., 2020, 11, 1–11 Search PubMed
. - A. Isozaki, H. Mikami, H. Tezuka, H. Matsumura, K. Huang, M. Akamine, K. Hiramatsu, T. Iino, T. Ito, H. Karakawa, Y. Kasai, Y. Li, Y. Nakagawa, S. Ohnuki, T. Ota, Y. Qian, S. Sakuma, T. Sekiya, Y. Shirasaki, N. Suzuki, E. Tayyabi, T. Wakamiya, M. Xu, M. Yamagishi, H. Yan, Q. Yu, S. Yan, D. Yuan, W. Zhang, Y. Zhao, F. Arai, R. E. Campbell, C. Danelon, D. Di Carlo, K. Hiraki, Y. Hoshino, Y. Hosokawa, M. Inaba, A. Nakagawa, Y. Ohya, M. Oikawa, S. Uemura, Y. Ozeki, T. Sugimura, N. Nitta and K. Goda, Lab Chip, 2020, 20, 2263–2273 RSC
. - Y. Zhou, A. Isozaki, A. Yasumoto, T. H. Xiao, Y. Yatomi, C. Lei and K. Goda, Trends Biotechnol., 2021 DOI:10.1016/j.tibtech.2020.12.012
. - A. Isozaki, J. Harmon, Y. Zhou, S. Li, Y. Nakagawa, M. Hayashi, H. Mikami, C. Lei and K. Goda, Lab Chip, 2020, 20, 3074–3090 RSC
.
|
This journal is © The Royal Society of Chemistry 2021 |