DOI:
10.1039/D1RA00127B
(Paper)
RSC Adv., 2021,
11, 8718-8729
Insight into the origin of SARS-CoV-2 through structural analysis of receptor recognition: a molecular simulation study†
Received
7th January 2021
, Accepted 17th February 2021
First published on 25th February 2021
Abstract
Bats and pangolins are considered to be potential hosts of the new coronavirus SARS-CoV-2, based on its genome similarity to coronaviruses of these species (Bat-CoV-RaTG13 and Pangolin-CoV). The receptor-binding domain (RBD), a functional component of the spike protein, is responsible for binding of SARS-CoV-2 by human ACE2 receptors and is also key to cross-species viral transmission. We performed molecular dynamics (MD) simulations using structures of hACE2 in complex with the RBD of SARS-CoV-2, SARS-CoV, Pangolin-CoV and Bat-CoV-RaTG13, respectively. By analyzing the hydrogen-bonding network at the RBD–hACE2 interface and estimating the binding free energies between RBD and hACE2, we found Pangolin-CoV bound hACE2 in a similar state as did SARS-CoV-2, and both of them bound hACE2 more strongly than did Bat-CoV-RaTG13 or SARS-CoV. We further identified two major adaptation mutations of SARS-CoV-2-RBD, which may have significant roles in regulating the recognition and binding between RBD and hACE2. Our results add to existing evidence that Pangolins have the potential to act as an intermediate host for SARS-CoV-2, and provide guidance for future design of antiviral drugs and vaccines.
1. Introduction
An outbreak of serious pneumonia was reported in Wuhan, China, on 30 December 2019. The pneumonia was caused by a novel coronavirus (severe acute respiratory syndrome coronavirus 2, SARS-CoV-2, once named 2019-nCoV). The World Health Organization has declared the virus outbreak to be a public health emergency of international concern. Despite great efforts to control the spread of SARS-CoV-2, there is currently no targeted direct treatment and/or prevention method for coronaviruses. Therefore, understanding the virology of coronaviruses and controlling their spread is important to human health and social stability.
Coronaviruses were so named because the negatively stained specimens of their particles show a corona or crown shape.1,2 They belong to the order Nidovirales and suborder Coronaviridae and can be classified into four genera: α-CoV, β-CoV, γ-CoV, and δ-CoV;1 α-CoV and β-CoV can infect humans, whereas γ-CoV and δ-CoV are highly pathogenic to livestock.3 Like SARS-CoV and MERS-CoV, SARS-CoV-2 belongs to the β-CoV genus and has a single-stranded RNA genome. The genome of SARS-CoV-2 is about 29.8 kb,4 it encodes a series of non-structural proteins involved in replication and transcription, and four essential structural proteins: the spike (S) glycoprotein, the envelope (E) protein, the membrane (M) protein, and the nucleocapsid (N) protein.1,2,5
Entry of coronaviruses into host cells is mediated by the transmembrane S glycoprotein, which forms homotrimers protruding from the viral surface.6 Thus, the S protein is the main target of neutralizing antibodies upon infection, and is the focus of therapeutic and vaccine design.7 It also determines the host range and tissue tropism of the virus.7,8 Structurally, the S protein has three main parts (Fig. S1†): a large ectodomain, a single-pass transmembrane anchor, and a short intracellular tail. The ectodomain comprises two functional subunits: the S1 subunit, which is responsible for binding to the host cell receptor, and the S2 subunit, which controls fusion of the viral and cellular membranes.7,9 The coronavirus first binds to the receptor on the host cell surface via S1 and is then primed by cellular proteases. This entails S protein cleavage at the S1/S2 site, which allows fusion of viral and cellular membranes, a process driven by S2.6,8 Therefore, receptor binding is a critical initial step for coronavirus entry into target cells. Different coronaviruses use different receptor-binding domains (RBDs) within S1 to recognize distinct entry receptors. SARS-CoV-2 interacts directly with angiotensin-converting enzyme 2 (ACE2) to enter host cells, via the same RBD as SARS-CoV.10,11 In vitro binding measurements showed that the SARS-CoV-2 S protein binds to human ACE2 (hACE2) with an affinity in the low-nM range, which is ∼10-20-fold higher than that of the SARS-CoV S protein.7,12 Tight binding to hACE2 could partially explain the efficient transmission of SARS-CoV-2 in humans. These studies indicate that the RBD is the key functional component of the S1 subunit that is responsible for binding of SARS-CoV-2 by ACE2.
Much more information can be gained owing to the mutational nature of the S protein. In particular, the ability of RBD to bind to receptors of different species is a prerequisite for inter-species transmission. Its amino acid sequence and protein structure may provide information about hosts in the process of infection. In the early stages of this epidemic, full-length genomic sequences of SARS-CoV-2 were obtained from five patients; they were almost identical and had 79.5% genome homology with SARS-CoV.13 SARS-CoV-2 was highly similar throughout its genome to a bat coronavirus RaTG13 (Bat-CoV-RaTG13), with an overall genome sequence identity of 96.2%.13 The results of phylogenetic analysis of the complete viral genome also support the conclusion that SARS-CoV-2 may be derived from bats.14–16 However, the binding affinity of Bat-CoV-RaTG13 toward hACE2 is about 1000-fold lower than that of the SARS-CoV-2, indicating that Bat-CoV-RaTG13 would be unlikely to infect humans directly.17 In a new coronavirus found in Malaysian pangolins (Pangolin-CoV) seized in Guangdong, the E, M, N, and S proteins had 100%, 98.2%, 96.7%, and 90.4% amino acid homology with SARS-CoV-2.18 Notably, the amino acid phylogenetic tree showed that the S1 protein of Pangolin-CoV was more closely related to that of SARS-CoV-2 than to that of Bat-CoV-RaTG13.19 This finding highlights some intrinsic sequence and structural differences among the Pangolin-CoV, Bat-CoV-RaTG13, and SARS-CoV-2 RBDs.18,20 In addition, comparative genomic analysis suggests that SARS-CoV-2 might have originated from the recombination of a Pangolin-CoV-like virus with a Bat-CoV-RaTG13-like virus.18 Therefore, pangolin has the potential to act as an intermediate host of SARS-CoV-2, although this has yet to be confirmed.
The crystal structure of SARS-CoV-2 RBD complexed with hACE2, and the cryo-electron microscopy (cryo-EM) structure of Bat-CoV-RaTG13 RBD, have been resolved very recently,11,17,21,22 revealing high structural similarity between the SARS-CoV-2, Bat-CoV-RaTG13 and SARS-CoV RBDs.12,23 Based on this foundation, several open questions can be further explored: first, why Bat-CoV-RaTG13 has a much lower binding affinity to hACE2 than SARS-CoV-2; second, how Pangolin-CoV overcomes major species barriers to gain the ability to infect humans; and, third, why SARS-CoV-2 is more infectious than SARS-CoV. Extensive investigation of the molecular mechanism of the binding between the RBD protein and the hACE2 receptor may help provide answers to these questions. To this end, molecular dynamics (MD) simulations can be fruitfully used to demonstrate dynamical features at an atomic level.24–26 For instance, He et al.24 studied the molecular mechanisms of human infection with SARS-CoV-2 and SARS-CoV by MD simulations. By calculating binding free energies, they found that SARS-CoV-2 binds ACE2 with a higher affinity than SARS-CoV, indicating that SARS-CoV-2 may be more infectious than SARS-CoV. Similarly, Spinello et al.25 performed μs-long MD simulations to explain the higher affinity of SARS-CoV-2 toward ACE2 as compared to SARS-CoV through binding free energy and hydrogen-bonding network analyses. Additionally, Wang et al.26 performed MD simulations of binary complexes of the RBD of SARS-CoV-2 and SARS-CoV with the receptor ACE2 and the antibody 80R. They found that both electrostatic complementarity and hydrophobic interactions are critical to enhancing receptor binding for the RBD of SARS-CoV-2. However, more findings about receptor binding for the RBD of Pangolin-CoV and Bat-CoV-RaTG13 toward ACH2 are still waiting to be explored.
In this study, we constructed four models: (1) SARS-CoV-2 RBD–hACE2 complex, (2) Pangolin-CoV RBD–hACE2 complex, (3) Bat-CoV-RaTG13 RBD–hACE2 complex, and (4) SARS-CoV RBD–hACE2 complex. Models (1) and (4) were derived from their respective crystal structures. In the model (2), the Pangolin-CoV RBD was a homology model constructed using the SARS-CoV-2 RBD as a template. In the model (3), the Bat-CoV-RaTG13 RBD was derived from cryo-EM structure. These complexes were subjected to four long and independent MD simulations with a total of 4 μs sampling.
By comparing the overall conformational changes of these four complexes, and analyzing the binding free energies and differences in key residues at the RBD–hACE2 interface, the following conclusions, which are consistent with previous studies,7,11,12,21,24–26 were drawn. (1) The binding affinity of Bat-CoV-RaTG13 toward hACE2 is weak, indicating that it may not infect humans directly. (2) Pangolin-CoV and SARS-CoV-2 have similar hydrogen-bonding network and binding free energies toward hACE2, suggesting that pangolins have the potential to act as an intermediate host for SARS-CoV-2. (3) SARS-CoV-2 RBD binds to hACE2 more tightly than does SARS-CoV RBD, which may explain its greater infectivity. The present study provides insights that will be beneficial for the development of vaccines and drugs against SARS-CoV-2.
2. Computational methods
2.1 Amino acid sequence alignment
The amino acid sequences of the S proteins of SARS-CoV-2, Pangolin-CoV, Bat-CoV-RaTG13, and SARS-CoV were obtained from NCBI (accession numbers MN908947, MT084071, MN996532, and AY274119). CLUSTALW27 was used to perform multiple sequence alignments. BLASTp28 was used to calculate the identities of the S protein and the RBD of the four coronaviruses.
2.2 Structural modeling for MD simulations
Four systems were built for the MD simulations: SARS-CoV-2, Pangolin-CoV, Bat-CoV-RaTG13, and SARS-CoV. In the SARS-CoV-2 and SARS-CoV systems, the starting structures were derived from crystal structures of the respective RBD–hACE2 complexes (PDB: 6M0J and 2AJF).21,23 Missing residues were added using SWISS-MODEL.29 In the Pangolin-CoV system, homology model of the RBD structure were constructed using the I-TASSER server.30 The crystal structure of the RBD of SARS-CoV-2 was used as a template. In the Bat-CoV-RaTG13 system, the RBD structure was derived from cryo-EM structure (PDB: 6ZGF).17 Then the RBD of Pangolin-CoV and Bat-CoV-RaTG13 were superimposed onto the RBD of SARS-CoV-2 to obtain complexes with hACE2 as starting structures, respectively. The complex of each system was prepared using the Leap module of the AMBER18 package31 with the AMBER FF14SB force field.32 Cysteine residues were deprotonated if they formed disulfide bonds. The complexes were solvated in a hexagonal explicit water box under periodic boundary conditions using TIP3P water33 and neutralized using 0.15 M NaCl. The distance between the edge of the box and the closest atom of the complex was 12 Å. The box sizes of SARS-CoV-2, Pangolin-CoV, Bat-CoV-RaTG13 and SARS-CoV were 1343245.3, 1366144.8, 1383729.7 and 1345510.5 Å3 with 33
630, 33
901, 34
318 and 33
432 TIP3P water molecules, respectively.
The AMBER18 software package31 was used to perform the MD simulations. First, for the Pangolin-CoV and Bat-CoV-RaTG13 systems, the mutated residues in the modeled RBD structures were performed an extra 10
000-step minimization with other residues constrained. Then with the constraint force removed, for each solvated system, a 10
000-step energy minimization was performed, followed by a combined equilibration process with a 500 ps constant volume ensemble to heat the system from 0 to 300 K, and a 500 ps constant pressure ensemble at a pressure of 1 bar. The Langevin thermostat34 and Berendsen barostat35 were used for temperature and pressure control, respectively. During equilibration, a force constant of 20 kcal mol−1 Å−2 was applied to the complex as a harmonic constraint. Then, a 1 μs MD simulation of each system was performed in a constant pressure ensemble at 300 K with the constraint released. The time step was set to 2 fs. The SHAKE algorithm36 was used to restrain all the bond lengths involving hydrogen atoms. The cut-off value of the van der Waals interactions was set to 10 Å. The particle mesh Ewald method37 was used to calculate long-range electrostatic contributions.
2.3 Analysis of the simulations
2.3.1 Coordinate analyses. The cpptraj module38 of AmberTools18 was used to perform a series of analyses including calculation of root mean square deviation (RMSD), distance and angle measurements, solvent accessibility surface area (SASA), dynamic cross-correlation maps (DCCM) and hydrogen bonds between the RBD and hACE2. As the residue index was not identical among the simulated systems, SARS-CoV-2 was taken as an example for brevity. The distance D1 was measured based on the centroids of residues 486–502 of RBD and residues 24–53 of hACE2. The angle A1 was measured based on the centroids of residues 395–398 of RBD, residues 492–494 of RBD and residues 548–560 of hACE2. SASA was calculated based on the residues at the hACE2–RBD interface including S19, Q24, A25, T27, F28, L29, D30, K31, H34, E35, E37, D38, Y41, Q42, L45, L79, M82, Y83, Q325, G326, N330, G352, K353, G354, D355, R357, A386 and R393 of hACE2, and, R403, K417, V445, G446, G447, Y449, Y453, L455, F456, Y473, A475, G476, S477, E484, G485, F486, N487, Y489, F490, Q493, S494, Y495, G496, F497, Q498, P499, T500, N501, G502, V503 and Y505 of RBD. These residues were also selected to calculate the dynamic cross-correlations map (DCCM) of the last 200 ns of the MD simulation based on mutual information between all Cα atoms. For the other simulated systems, residues at the same positions were employed for the analyses above. The averaged final conformation for each system was derived from the centroid cluster analysis based on the RMSD of the last 200 ns of the MD simulation.
2.3.2 Potential of mean force. The potential of mean force (PMF) of each system was calculated along with distance D1 and angle A1 throughout the MD simulation, and depicted the conformational changes due to the adequate sampling applied. The energy landscape was calculated using the equation |
ΔG(x,y) = kBT ln g(xy),
| (1) |
where kB is the Boltzmann constant, T is the simulation temperature, and g(x,y) is the normalized joint probability distribution. The minimum energy was set to zero.
2.3.3 Binding free energy. The binding free energy between RBD and hACE2 for each system was calculated by the MMGBSA method, which combines molecular mechanics, the generalized Born equations, and surface accessible calculations.39–41 In this work, RBD and hACE2 were considered as ligand and receptor, respectively. The time evolution of the binding free energy of each system was calculated by snapshots with a 1 ns interval during the MD simulations. The averaged binding free energy and the residue decomposition were calculated using snapshots from the final 200 ns trajectory. All parameters were set to their default values in the calculations. As we were mainly interested in the differences in the binding free energies, the entropic contribution of the free energy was not taken into account as suggested in the previous work.42
3. Results
3.1 Sequence features of SARS-CoV-2 S-RBD
The amino acid sequences of the S proteins of SARS-CoV-2, Pangolin-CoV, Bat-CoV-RaTG13 and SARS-CoV were aligned to compare their differences. Pangolin-CoV, Bat-CoV-RaTG13, and SARS-CoV had 90.4%, 97.4%, and 76.0% amino acid identity with SARS-CoV-2 in their S protein genes, and 97.2%, 89.0%, and 72.4% amino acid identity in their RBD genes, respectively (Table 1). Fig. 1 shows the amino acid sequence alignment of the RBD of the SARS-CoV-2 with those of the other three coronaviruses. The residues at the RBD–hACE2 interface are labeled by black triangles. Among them five critical residues, R403, K417, Q493, S494, and Q498 (SARS-CoV-2 numbering) were labeled by black boxes. They were identified by comparing the hydrogen-bonding network and calculating the binding free energy between RBD and hACE2 from the simulated systems that will be discussed in the following sections of 3.4 and 3.5.
Table 1 Amino acid sequence identities of the RBD of SARS-CoV-2 with Pangolin-CoV, Bat-CoV-RaTG13, and SARS-CoV S protein and RBD genes
Identity |
Pangolin-CoV |
Bat-CoV-RaTG13 |
SARS-CoV |
S protein |
90.4% |
97.4% |
76.0% |
RBD |
97.2% |
89.0% |
72.4% |
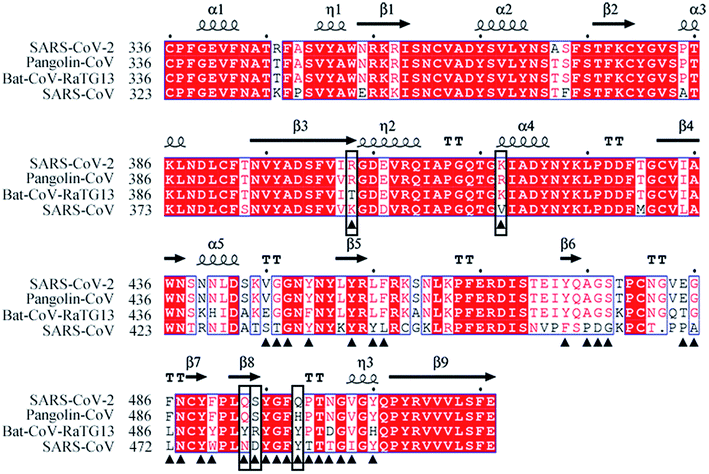 |
| Fig. 1 Amino acid sequence alignments of the RBD of SARS-CoV-2 with Pangolin-CoV, Bat-CoV-RaTG13, and SARS-CoV. Residues at the RBD–hACE2 interface are labeled by black triangles. Five critical residues are indicated by black boxes. | |
3.2 Overall conformational change during MD simulations
To determine the effectiveness of the recognition and the stability of the binding between the CoV RBDs and hACE2, four models were built and used in 1 μs MD simulations: (1) SARS-CoV-2 RBD–hACE2 complex, (2) Pangolin-CoV RBD–hACE2 complex, (3) Bat-CoV-RaTG13 RBD–hACE2 complex, and (4) SARS-CoV RBD–hACE2 complex. Coordinate analyses were used to determine the structural stability of each complex. Time evolutions of RMSD values (Fig. 2A) showed that the complex was stable in the SARS-CoV-2, Pangolin-CoV, and SARS-CoV systems, with the values remaining in a range of 2.5–3.5 Å during almost the whole trajectory. In the Bat-CoV-RaTG13 system, the RMSD showed an ascent from 2.5 to 3.5 Å after ∼100 ns of simulation and a further ascent to 4.5 Å after ∼600 ns. However, both the RBD of Bat-CoV-RaTG13 and the hACE2 were stable from their respective RMSD analysis (Fig. S2†). It is indicated that the complex underwent a marked conformational change. Fig. 2B–D shows the structural superimpositions of the averaged final conformation of SARS-CoV-2 with those of Pangolin-CoV, Bat-CoV-RaTG13, and SARS-CoV, respectively. The RBDs of SARS-CoV-2 and Pangolin-CoV still showed high structural similarity after the 1 μs MD simulation. Although there were a few differences in the loop region, the RBDs of SARS-CoV-2 and SARS-CoV also exhibited good alignment. However, the RBD of Bat-CoV-RaTG13 showed an obvious displacement from that of SARS-CoV-2.
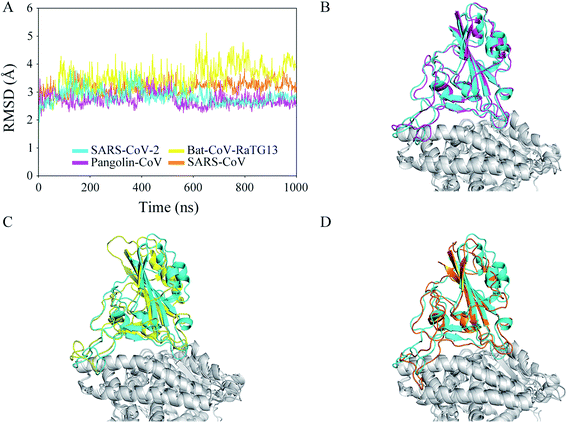 |
| Fig. 2 Structural deviation during MD simulations for the four systems. (A) Time evolutions of RMSD. SARS-CoV-2, Pangolin-CoV, Bat-CoV-RaTG13, and SARS-CoV are shown in cyan, magenta, yellow, and orange, respectively. The same scheme is used in the following figures unless otherwise specified. (B–D) Superpositions of averaged final conformations. Only the RBD is colored for comparison purposes. The RBD of SARS-CoV-2, Pangolin-CoV, Bat-CoV-RaTG13 and SARS-CoV are shown in cyan, magenta, yellow, and orange, respectively. | |
To explore the geometrical changes at the interface of RBD and hACE2, especially for the Bat-CoV-RaTG13 system, the distance D1 as defined in Fig. 3A was measured. The time evolutions of distance D1 (Fig. 3B) showed that in the SARS-CoV-2, Pangolin-CoV, and SARS-CoV systems, the D1 values remained steady, with average values of 11.18, 11.17, and 10.86 Å, respectively. These values were comparable to the initial value of ∼11.00 Å. In the Bat-CoV-RaTG13 system, the value dramatically increased after ∼100 ns, leading to an average D1 value of 12.74 Å; this was much larger than those of the other systems. Besides, another geometrical descriptor, the angle A1 between RBD and hACE2 as defined in Fig. 3C was measured. The results (Fig. 3D) exhibited similar trends to those of RMSD and distance D1 (Fig. 2A and 3B). The angle A1 showed a deep descent with an approximate range of 75–85° after 100 ns of the MD simulation in the Bat-CoV-RaTG13 system, whereas they remained steady with an approximate range of 85–95° in the other three simulated systems in the whole trajectories.
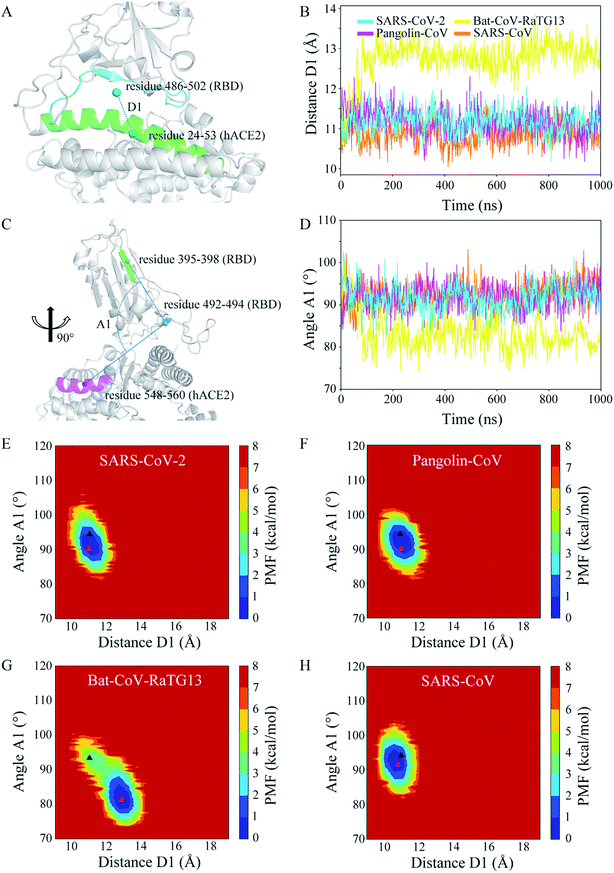 |
| Fig. 3 Conformational descriptors characterizing the dynamics of the structure of RBD complexed with hACE2 during MD simulations for the four systems. (A) Definition of distance D1 in the structure of the SARS-CoV RBD complexed with hACE2. Residues 486-502 of the SARS-CoV-2 RBD are shown in cyan. Residues 24–53 of hACE2 are shown in green. (B) Time evolutions of distance D1 between RBD and hACE2. (C) Definition of angle A1 in the structure of the SARS-CoV RBD complexed with hACE2. Residues 395–398 and 492–494 of the SARS-CoV-2 RBD are shown in green and cyan, respectively. Residues 548–560 of hACE2 are shown in magenta. (D) Time evolutions of distance D1 between RBD and hACE2. (E–H) PMF calculated for distance D1 vs. angle A1 for SARS-CoV-2, Pangolin-CoV, Bat-CoV-RaTG13 and SARS-CoV, respectively. The initial and averaged final states for each system are labeled by the black and red triangles, respectively. | |
The conformational change in the Bat-CoV-RaTG13 system could be monitored by the PMF map which depicts an energy landscape based on the large sampling space. In order to obtain a two-dimensional energy landscape map, distance D1 and angle A1 were chosen as the reaction coordinates. The initial and averaged final conformation in each system are labeled by the black and red triangles in Fig. 3E–H, respectively. From the analyses of the PMF maps, each of the SARS-CoV-2, Pangolin-CoV, and SARS-CoV systems, was concluded to adopt a uniform conformational state, indicative of a stable RBD–hACE2 interface. However, the Bat-CoV-RaTG13 system was concluded to a distinct conformational state from the initial conformation. Thus, the PMF map clearly suggested the complex underwent a large conformational change with an undesired recognition of RBD toward hACE2.
3.3 Correlated motions of the interfacial residues
To better decrypt the conformational change, the dynamic cross-correlation maps (DCCM) of the interfacial residues for each of system was performed. Inspection of the DCCM gave out a striking contrast between the SARS-CoV-2 and Bat-CoV-RaTG13 systems (Fig. 4A and B). And the Pangolin and SARS-CoV systems exhibited the similar correlated motions as compared to the SARS-CoV-2 systems (Fig. S3†). In Fig. 4, two distinct correlated motions between two systems were highlighted by the black and red boxes, respectively. They were residues 449 of RBD and residues 37–38, 352–355 of hACE2, and residue 484 of RBD and residues 27–31 of hACE2. Apparently, residue motions at both the two regions exhibited a general decrease in residue–residue correlations in Bat-CoV-RaTG13. It is inferred that these negative residue correlations were derived from the conformational change that was depicted by the two geometrical descriptors D1 and A1 analyzed above. Compared with SARS-CoV-2, the regions where F449 and T484 were located moved away from hACE2 obviously (Fig. 4C and D). In this case, during the last 200 ns of MD simulation, the averaged solvent accessibility surface area (SASA) of the interfacial residues was 2313.32 Å2 in the Bat-CoV-RaTG13 system due to the unstable RBD–hACE2 interface; by contrast, the averaged SASA in the SARS-CoV-2 system was 1950.42 Å2 (Table S1†). For Bat-CoV-RaTG13, the increased SASA was detrimental to the RBD binding toward hACE2. In addition, the numbers of hydrogen bonds between the RBDs and hACE2 were calculated to determine the structural stability. The time evolutions showed that the structure of the SARS-CoV-2 RBD with hACE2 had the most hydrogen bonds of all the systems, whereas the structure of Bat-CoV-RaTG13 RBD with hACE2 had the fewest (Fig. S4†).
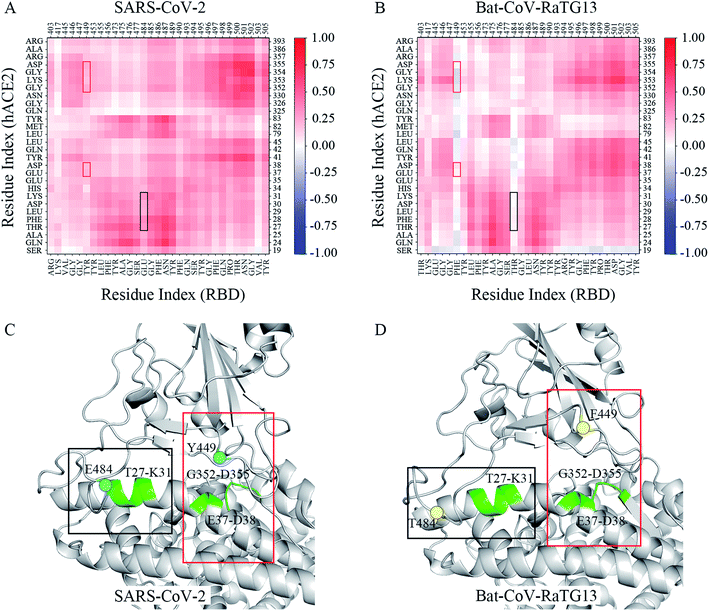 |
| Fig. 4 Correlated motions for the residues at the RBD–hACE2 interface. Dynamic cross-correlation maps for the (A) SARS-CoV-2 and (B) Bat-CoV-RaTG13 systems. The color scale is shown on the right changing from red (highly positive correlations) to blue (highly negative correlations). Two distinct correlated motions between the (C) SARS-CoV-2 and (D) Bat-CoV-RaTG13 systems are highlighted in the black and red boxes, respectively. | |
3.4 Key residues at the RBD–hACE2 interface
A previous structural analysis revealed that there are two positions at the RBD–hACE2 interface (hereafter referred to as P1 and P2) where the residue interactions may determine the major species barriers between humans and civets for SARS-CoV infections.43 It is worth discussing that whether the residue interactions at the two positions may affect the recognition process of RBD to hACE2 for different coronavirus subtypes. Fig. 5 shows the distinct hydrogen-bonding networks at the P1 and P2 positions for the four systems. Table 2 shows the occupancy of the hydrogen bonds. At the P1 position (Fig. 5A and B), two key residues, K417 and Q493 on the SARS-CoV-2 RBD, had intricate structural relationships with residues D30, K31, and E35 on hACE2. The occupancies of the K417-D30, Q493-K31, and Q493-E35 hydrogen bonds in the RBD–hACE2 complex were 67.24%, 35.01%, and 56.00%, respectively (Table 2). In the Pangolin-CoV system, residues 417 and 493 were arginine and glutamine, leading to similar interactions with residues D30, K31, and E35 on hACE2, respectively. The occupancies of the R417-D30, Q493-K31, and Q493-E35 hydrogen bonds were 77.50%, 38.41%, and 71.75%, respectively (Table 2). In the Bat-CoV-RaTG13 system, residue 417 remained lysine; however, residue 493 was a tyrosine, and its side chain could not form a hydrogen bond with K31 on hACE2 (Table 2). The substitution of residue 493 from glutamine to tyrosine resulted in a weakened interaction between the RBD and hACE2 and affected the formation of hydrogen bonds K417-D30 and Y493-E35, which had low occupancies of 48.86% and 1.50%, respectively (Table 2). In the SARS-CoV system, residue 404 (corresponding to residue 417 on SARS-CoV-2 RBD) was valine; the sidechain of V404 could not form a hydrogen bond or a salt bridge with D30 on hACE2. Residue 479 (corresponding to residue 493 on SARS-CoV-2 RBD) was asparagine. Compared with Q493, the shorter side chain of N479 limited it to form long-lived hydrogen bonds with K31 and E35 on hACE2 with a suitable orientation at the same time. Thus, the occupancy of hydrogen bond N479–K31 was much higher than that of N479-E35 during the MD simulations (58.34% vs. 8.28%, Table 2). In addition, the hydrogen bond between K31 and E35 on hACE2 was broken in this binding state, leaving a free positive charge and destabilizing the interface.
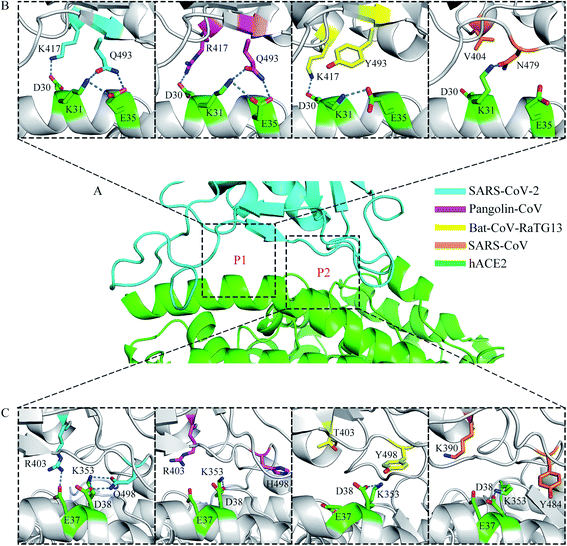 |
| Fig. 5 Distinct hydrogen-bonding networks for the four systems. (A) Structure of SARS-CoV-2 RBD (cyan) complexed with hACE2 (green). P1 and P2 positions are labeled. (B) The hydrogen-bonding networks at the P1 position for the four systems. Hydrogen bonds are depicted as dashed lines. (C) The hydrogen-bonding networks at the P2 position for the four systems. | |
Table 2 Occupancies of hydrogen bonds at the P1 and P2 positions during MD simulations
RBD–hACE2 |
SARS-CoV-2 |
Pangolin-CoV |
Bat-CoV-RaTG13 |
SARS-CoV |
K417/R417/K417/V404-D30 |
67.24% |
77.50% |
48.86% |
0 |
Q493/Q493/Y493/N479-K31 |
35.01% |
38.41% |
0 |
58.34% |
Q493/Q493/Y493/N479-E35 |
56.00% |
71.75% |
1.50% |
8.28% |
R403/R403/T403/K390-E37 |
27.94% |
5.19% |
0 |
0 |
Q498/H498/Y498/Y484-D38 |
58.21% |
21.30% |
24.17% |
2.02% |
Q498/H498/Y498/Y484-K353 |
66.72% |
0 |
0 |
0 |
At the P2 position (Fig. 5A and C), another two key residues, R403 and Q498 on the SARS-CoV-2 RBD, showed distinctly different interaction modes among the four systems. In the structure of SARS-CoV-2 RBD with hACE2, residue R403 formed a hydrogen bond with E37, with an occupancy of 27.94% (Table 2). However, in the Pangolin-CoV system, although residue 403 remained arginine, hydrogen bond R403-E37 had a short lifetime and a low occupancy of 5.19% (Table 2). A similar network was observed in the SARS-CoV system, where K390 did not form a hydrogen bond with E37 on hACE2 during the MD simulations (Table 2). In the Bat-CoV-RaTG13 system, residue 403 was threonine, and its sidechain was too short to form a hydrogen bond with E37 on hACE2 (Table 2).
The other residue, Q498, on the SARS-CoV-2 RBD formed hydrogen bonds with both D38 and K353 on hACE2. The occupancies of the hydrogen bonds Q498–D38 and Q498–K353 were 58.21% and 66.72%, respectively (Table 2). However, compared with Q498, residues H498, Y498, and Y484 at the corresponding sites on the Pangolin-CoV, Bat-CoV-RaTG13, and SARS-CoV RBDs could not form hydrogen bonds with K353 on hACE2 but only with D38, with low occupancies of 21.30%, 24.17%, and 2.02%, respectively (Table 2). This weakened interaction destabilized the RBD–hACE2 interface and may have affected the formation of the hydrogen bond R403-E37 in the Pangolin-CoV system and K390-E37 in the SARS-CoV system.
3.5 Energetic analyses between RBD and hACE2
The binding free energy and residue free energy decomposition between the RBD and hACE2 for each system were calculated to detect the interaction at the interface of the complex in terms of energy (Fig. 6). Fig. 6A shows the time evolutions of the binding free energies and their distributions during the MD simulations. The binding free energy of the Bat-CoV-RaTG13 RBD complexed with hACE2 increased during the simulation, even reaching a positive charge value. By contrast, the binding free energies in the other three systems showed lower fluctuations and narrower distributions. For comparison purposes, the average and standard deviation of the binding free energy during the last 200 ns of the MD simulation were calculated for each system (Table 3). As expected, the SARS-CoV-2 RBD had the most negative binding free energy (−47.59 kcal mol−1) toward hACE2, indicating the most favorable RBD–hACE2 recognition among the four systems. The Pangolin-CoV system had an approximate binding free energy of −39.83 kcal mol−1. The binding free energy between the Bat-CoV-RaTG13 RBD and hACE2 was −16.24 kcal mol−1, indicating an unstable interface and inefficient recognition between RBD and hACE2. Compared with SARS-CoV-2, the SARS-CoV RBD had a higher negative binding free energy (−27.44 kcal mol−1) toward hACE2, suggesting lower binding affinity.
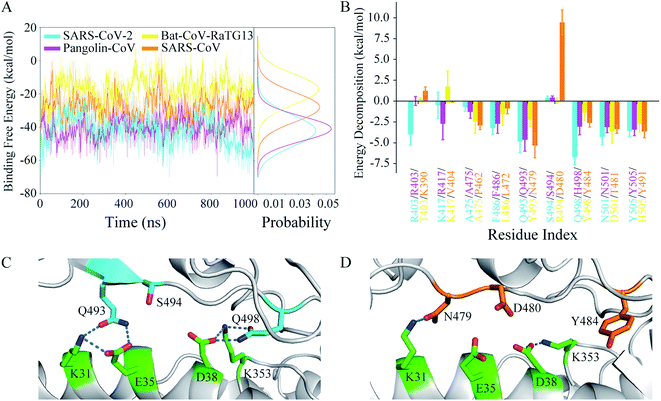 |
| Fig. 6 Energetic analyses characterizing the binding of RBD toward hACE2. (A) Time evolutions of the binding free energies of RBD toward hACE2 and their distributions for the four systems. (B) Decomposed binding free energies of several residues at the RBD–hACE2 interface. Residues shown are those for which the absolute value of the decomposed binding free energies at the corresponding position in any one of the simulated systems was more than 2.5 kcal mol−1. (C) Structural detail of S494 on SARS-CoV-2 RBD. (D) Structural detail of D480 on SARS-CoV RBD. | |
Table 3 Binding free energies of RBD toward hACE2 based on the last 200 ns of MD simulations for the four systemsa
RBD–hACE2 |
SARS-CoV-2 |
Pangolin-CoV |
Bat-CoV-RaTG13 |
SARS-CoV |
All binding free energies are in kcal mol−1. |
Binding free energy |
−47.59 ± 8.74 |
−39.83 ± 7.59 |
−16.24 ± 7.13 |
−27.44 ± 7.05 |
The differences in binding free energy could be detected by residue decomposition calculations. As shown in Fig. 6B, key residues were defined as those for which the absolute value of the decomposed binding free energy at the corresponding position in any of the simulated systems was more than 2.5 kcal mol−1. The decomposed contributions of all residues at the interface of RBD and hACE2 are shown in Table S2.† The residues involved in the hydrogen bonds discussed above made great contributions to the RBD–hACE2 binding, for instance, Q493 and Q498 in the SARS-CoV-2 system. The decomposed binding free energies of these two residues were −4.87 and −6.75 kcal mol−1, respectively. In the Pangolin-CoV system, Q493 also played an important part in the binding, with a similar contribution (−4.66 kcal mol−1). Although H498 did not form stable hydrogen bonds with residues of hACE2 during the MD simulation, its hydrophobic property could enhance the binding at the protein–protein interface. Thus, H498 showed a weaker but tolerable binding free energy toward hACE2 (−3.06 kcal mol−1). In the Bat-CoV-RaTG13 system, both Y493 and Y498 exhibited unfavorable protein–protein recognition, with high binding free energies of −2.23 and −1.37 kcal mol−1, respectively. Combined with the hydrogen-bonding network analysis above, a glutamine residue of RBD for both the P1 and P2 position exhibited much more rationality as a result of evolution than a histidine or tyrosine residue did. Due to its polar property, the glutamine sidechain could be both a hydrogen-bonding donor and acceptor, in favor of stabilizing the interface and enhancing the acceptor binding by interacting with the interfacial residues of hACE2. However, it seems that without strong hydrogen bonding, the hydrophobic interactions of the two tyrosine residues were insufficient to stabilize the RBD–hACE2 interface in the Bat-CoV-RaTG13 system (Fig. 5B and C). The destabilized interface led to the increased SASA (Table S1†). Naturally, it is energetically detrimental to the hydrophobic sidechain to be exposed to the solvent, which in turn further destabilized the interface. Thus, this explains why the Bat-CoV-RaTG13 RBD had a much higher total binding free energy toward hACE2 compared with the other models.
In the SARS-CoV system, the decomposed binding free energies of N479 and Y484 were −5.34 and −2.62 kcal mol−1, respectively, indicating acceptable contributions to the RBD–hACE2 binding. However, D480 of the SARS-CoV RBD had a much more positive binding free energy than S494 of the SARS-CoV-2 RBD (0.37 vs. 9.43 kcal mol−1). The high positive charge values indicate that these residues were very disadvantageous to SARS-CoV RBD–hACE2 binding, which was confirmed by detailed structure analysis (Fig. 6C and D). In the structure of SARS-CoV-2 RBD with hACE2, two key residues, Q493 and Q498, formed hydrogen bonds with K31, E35, D38, and K353 of ACE2, respectively. The negative charges of E35 and D38 on hACE2 were effectively neutralized. Besides, the sidechain hydroxyl group of S494 on RBD was a hydrogen-bonding donor, which could form a hydrogen bond with E35 or D38 on hACE2 if they were close. However, in the SARS-CoV RBD complex with hACE2, only N479 formed a long-lasting hydrogen bond with K31 on hACE2 at the corresponding sites (Table 2). The negative charges of E35 and D38 on hACE2 not only could not be neutralized by partners from the SARS-CoV RBD but were also electrically repulsed by the negative charge of D480 on the SARS-CoV RBD. In this situation, D480 had a large negative influence on the binding of the SARS-CoV RBD to hACE2. Thus, SARS-CoV has less favorable RBD–hACE2 recognition than SARS-CoV-2.
4. Discussion
4.1 The critical determinant of human viral infections
The RBD is responsible for binding to host cell surface receptors. Both SARS-CoV-2 and SARS-CoV interact with hACE2 directly to enter human target cells. Certain key residues are critical determinants of the recognition process between the RBD and hACE2. By multiple amino acid sequence alignments and hydrogen-bonding analyses after MD simulations, five residues, R403, K417, Q493, S494, and Q498 on the SARS-CoV-2 RBD were identified as potentially having significant roles in human viral infection (Fig. 1, 5 and Table 2). Among these residues, Q493 and Q498, which were also discussed in the study of Spinello et al.,25 were especially important in forming hydrogen bonds with K31, E35, D38, and K353 of hACE2. The former has a major role in determining whether the RBD can bind with hACE2, whereas the latter is likely to determine the binding affinity.43–45 SARS-CoV-2 undergoes major adaptation mutations at residues 493 and 498 on its RBD compared with Bat-CoV-RaTG13 and Pangolin-CoV. The MD simulations show that glutamine may be the best candidate to form hydrogen bonds with a lysine and glutamate (K31 and E35 of hACE2) or an aspartate and lysine (D38 and K353 of hACE2) pair simultaneously to avoid free charges embedded in a hydrophobic environment at the interface of RBD and hACE2. Both Q493 and Q498 make significant contributions to the binding of RBD toward hACE2. Besides, their stable bindings further enhance other interactions, including K417 of RBD with D30 of hACE2, and R403 of RBD with E37 of hACE2.
4.2 Bat-CoV-RaTG13 RBD may not effectively recognize hACE2
In this work, starting with strongly binding RBD–hACE2 complexes, all the systems had stable hydrogen-bonding networks at the interface during MD simulations except Bat-CoV-RaTG13, in which the complex underwent a large conformational change. In the Bat-CoV-RaTG13 system, the unstable interface enlarged the gap and distorted the angle between RBD and hACE2, leading to a larger SASA of RBD and a higher total binding free energy of RBD toward hACE2. In this case, the complex had a trend of dissociation. Although the RBD genes of Bat-CoV-RaTG13 and SARS-CoV-2 had a high amino acid identity of 89.0%, the residues at the RBD–hACE2 interface showed several significant differences. Among them the key residues Y493 and Y498 of the Bat-CoV-RaTG13 RBD could not interact with the key residues K31, E35, D38, and K353 of hACE2 well (Fig. 5 and Table 2). The unmatched interactions at the interface had a negative effect on the recognition of RBD to hACE2. These results are consistent with speculation that the RBD of Bat-CoV-RaTG13 cannot recognize hACE2 receptors.17,18,20 Thus, without an intermediate host, the Bat-CoV-RaTG13 may not infect humans directly.
4.3 A potential intermediate host for SARS-CoV-2
Multiple amino acid sequence alignments showed that Pangolin-CoV had a lower amino acid identity (89.0%) with SARS-CoV-2 in the S protein genes compared with Bat-CoV-RaTG13; however, it had a higher amino acid identity of 97.2% with SARS-CoV-2 in the RBD genes. It has been suggested based on sequence alignments that SARS-CoV-2 may have recombined from a Pangolin-CoV-like virus and a Bat-CoV-RaTG13-like virus.18,20 Residues 493 and 498 of Pangolin-CoV RBD are glutamine and histidine, respectively. Although the mutation from Bat-Y498 to Pangolin-H498 of CoV-RBD still did not enable stable hydrogen bonds to form with D38 and K353 of hACE2, the sidechain of H498 embedded at the stable interface was energetically advantageous to hydrophobic binding in this case. Meantime, the mutation from Bat-Y493 to Pangolin-Q493 of RBD greatly increased the binding affinity to hACE2 by enabling direct interaction with K31 and E35 of hACE2 (Fig. 5 and Table 2). The total binding free energy of RBD toward hACE2 decreased to −39.83 kcal mol−1 (Table 3). These results indicate that Pangolin-CoV may overcome major species barriers and have the ability to infect humans owing to the significant residue Q493, enabling pangolins to act as potential intermediate hosts of SARS-CoV-2.
4.4 SARS-CoV-2 RBD has higher binding affinity than SARS-CoV RBD toward hACE2
The results of the biophysical experiments indicate that the SARS-CoV-2 S protein binds to hACE2 with 10- to 20-fold higher affinity than the SARS-CoV S protein.12 This difference can be explained in terms of both structure and energy. The two major adaptation mutations of SARS-CoV RBD are D479 and Y484, respectively. The former is similar to the Q493 of SARS-CoV-2 RBD, whereas the latter makes few contributions to the binding comparing with Q498 of SARS-CoV-2 RBD does (Fig. 6B). Another key residue, 480 of SARS-CoV RBD, which is an aspartic with a free negative charge, had a large negative influence on the binding, with a decomposed binding free energy of 9.43 kcal mol−1. During the MD simulations, it interacted with E35 and D38 of hACE2, whose negative charges could not be neutralized by residues N479 and Y484 of the SARS-CoV RBD. These conflictive free charges induced strong electric repulsions, which are energetically detrimental to the binding of RBD toward hACE2. These disadvantages led to a binding free energy of −27.44 kcal mol−1 for the SARS-CoV RBD toward hACE2. The approximate 20 kcal mol−1 difference were also predicted in other two MD simulation studies.24,25 In contrast to SARS-CoV, the residues Q493 and Q498 of the SARS-CoV-2 RBD could effectively neutralize the negative charges of E35 and D38 of hACE2 through forming stable hydrogen bonds. Moreover, SARS-CoV-2, unlike SARS-CoV, contains a mutation at residue 494 from aspartic to serine, which removes a negative charge. Thus, owing to the neutralization or removal of such free charges at the RBD–hACE2 interface, the SARS-CoV-2 RBD has enhanced affinity for hACE2 compared with SARS-CoV and may thus more effectively infect humans.
5. Conclusions
In conclusion, we carried out four independent 1 μs MD simulations to investigate the molecular mechanism of the binding between different species of RBD proteins and the hACE2 receptor. By analyzing the hydrogen-bonding network at the RBD–hACE2 interface and estimating the binding free energies between RBD and hACE2, we found Pangolin-CoV and SARS-CoV-2 had similar networks and binding free energies toward hACE2, while binding affinities of Bat-CoV-RaTG13 and SARS-CoV toward hACE2 were weak, suggesting that pangolins have the potential to act as an intermediate host for SARS-CoV-2. We further identified five key residues, R403, K417, Q493, S494, and Q498 (SARS-CoV-2 numbering), which may have significant roles in regulating the recognition and binding between RBD and hACE2. Among these residues, Q493 and Q498 are the sites of two major adaptation mutations that affect the binding capacity of RBD toward hACE2. The results of the present study may provide targets for drug design and vaccine development against SARS-CoV-2.
Conflicts of interest
There are no conflicts of interest to declare.
Acknowledgements
We thank grant supports from the Natural Science Foundation of China 31870737 and 31521002, Chinese Ministry of Science and Technology 2018YFA0107004 and 2019YFA0508902, Tianjin Funds for Distinguished Young Scientists 17JCJQJC45900, and Fundamental Research Funds for the Central Universities of Nankai University 735-63201233.
References
- R. W. Susan and L. L. Julian, Adv. Virus Res., 2011, 81, 85–164 CrossRef.
- S. Su, G. Wong, W. Shi, J. Liu, A. C. Lai, J. Zhou, W. Liu, Y. Bi and G. F. Gao, Trends Microbiol., 2016, 24, 490–502 CrossRef CAS.
- N. Zhu, D. Zhang, W. Wang, X. Li, B. Yang, J. Song, X. Zhao, B. Huang, W. Shi, R. Lu, P. Niu, F. Zhan, X. Ma, D. Wang, W. Xu, G. Wu, G. F. Gao and W. Tan, N. Engl. J. Med., 2020, 382, 727–733 CrossRef CAS.
- R. A. Khailany, M. Safdar and M. Ozaslan, Gene Rep., 2020, 19, 100682–100687 CrossRef.
- H. K. H. Luk, X. Li, J. Fung, S. K. P. Lau and P. C. Y. Woo, Infect., Genet. Evol., 2019, 71, 21–30 CrossRef CAS.
- A. C. Walls, M. A. Tortorici, J. Snijder, X. Xiong, B. J. Bosch, F. A. Rey and D. Veesler, Proc. Natl. Acad. Sci. U. S. A., 2017, 114, 11157–11162 CrossRef.
- A. C. Walls, Y. J. Park, M. A. Tortorici, A. Wall, A. T. McGuire and D. Veesler, Cell, 2020, 181, 281–292 CrossRef CAS.
- A. C. Walls, M. A. Tortorici, B. J. Bosch, B. Frenz, P. J. M. Rottier, F. DiMaio, F. A. Rey and D. Veesler, Nature, 2016, 531, 114–117 CrossRef CAS.
- W. Song, M. Gui, X. Wang and Y. Xiang, PLoS Pathog., 2018, 14, 1–19 Search PubMed.
- M. Hoffmann, H. Kleine-Weber, S. Schroeder, N. Krüger, T. Herrler, S. Erichsen, T. S. Schiergens, r. G. Herrle, N. H. Wu, A. Nitsche, M. A. Müller, C. Drosten and S. Pöhlmann, Cell, 2020, 181, 271–280 CrossRef CAS.
- Q. Wang, Y. Zhang, L. Wu, S. Niu, C. Song, Z. Zhang, G. Lu, C. Qiao, Y. Hu, K. Y. Yuen, Q. Wang, H. Zhou, J. Yan and J. Qi, Cell, 2020, 181, 894–904 CrossRef CAS.
- W. Daniel, N. S. Wang, S. C. Kizzmekia, A. G. Jory, H. Ching-Lin, A. Olubukola, S. G. Barney and S. M. Jason, Science, 2020, 367, 1260–1263 CrossRef.
- P. Zhou, X. L. Yang, X. G. Wang, B. Hu, L. Zhang, W. Zhang, H. R. Si, Y. Zhu, B. Li, C. L. Huang, H. D. Chen, J. Chen, Y. Luo, H. Guo, R. D. Jiang, M. Q. Liu, Y. Chen, X. R. Shen, X. Wang, X. S. Zheng, K. Zhao, Q. J. Chen, F. Deng, L. L. Liu, B. Yan, F. X. Zhan, Y. Y. Wang, G. F. Xiao and Z. L. Shi, Nature, 2020, 5, e00160–20 Search PubMed.
- F. Wu, S. Zhao, B. Yu, Y. M. Chen, W. Wang, Z. G. Song, Y. Hu, Z. W. Tao, J. H. Tian, Y. Y. Pei, M. L. Yuan, Y. L. Zhang, F. H. Dai, Y. Liu, Q. M. Wang, J. J. Zheng, L. Xu, E. C. Holmes and Y. Z. Zhang, Nature, 2020, 579, 265–269 CrossRef CAS.
- B. Domenico, G. Marta, C. Alessandra, S. Silvia, A. Silvia and C. Massimo, J. Med. Virol., 2020, 92, 455–459 CrossRef.
- G. S. Randhawa, M. P. M. Soltysiak, H. El Roz, C. P. E. de Souza, K. A. Hill and L. Kari, PLoS One, 2020, 15, 1–24 CrossRef.
- A. G. Wrobel, D. J. Benton, P. Xu, C. Roustan, S. R. Martin, P. B. Rosenthal, J. J. Skehel and S. J. Gamblin, Nat. Struct. Mol. Biol., 2020, 27, 763–767 CrossRef CAS.
- K. Xiao, J. Zhai, Y. Feng, N. Zhou, X. Zhang, J. J. Zou, N. Li, Y. Guo, X. Li, X. Shen, Z. Zhang, F. Shu, W. Huang, Y. Li, Z. Zhang, R. A. Chen, Y. J. Wu, S. M. Peng, M. Huang, W. J. Xie, Q. H. Cai, F. H. Hou, Y. Liu, W. Chen, L. Xiao and Y. Shen, Nature, 2020, 583, 286–289 CrossRef CAS.
- T. Zhang, Q. Wu and Z. Zhang, Curr. Biol., 2020, 30, 1346–1351 CrossRef CAS.
- T. T. Lam, M. H. Shum, H. Zhu, Y. G. Tong, X. B. Ni, Y. S. Liao, W. Wei, W. Y. Cheung, W. J. Li, L. F. Li, G. M. Leung, E. C. Holmes, Y. L. Hu, Y. Guan and W. C. Cao, Nature, 2020, 583, 282–285 CrossRef CAS.
- J. Lan, J. Ge, J. Yu, S. Shan, H. Zhou, S. Fan, Q. Zhang, X. Shi, Q. Wang, L. Zhang and X. Wang, Nature, 2020, 581, 215–220 CrossRef CAS.
- J. Shang, G. Ye, K. Shi, Y. Wan, C. Luo, H. Aihara, Q. Geng, A. Auerbach and F. Li, Nature, 2020, 581, 221–224 CrossRef CAS.
- F. Li, W. Li, M. Farzan and S. C. Harrison, Science, 2005, 309, 1864–1868 CrossRef CAS.
- J. He, H. Tao, Y. Yan, S. Y. Huang and Y. Xiao, Viruses, 2020, 12, 428–430 CrossRef CAS.
- A. Spinello, A. Saltalamacchia and A. Magistrato, J. Phys. Chem. Lett., 2020, 11, 4785–4790 CrossRef CAS.
- Y. Wang, M. Liu and J. Gao, Proc. Natl. Acad. Sci. U. S. A., 2020, 117, 13967–13974 CrossRef CAS.
- M. Fábio, M. P. Young, L. Joon, B. Nicola, G. Tamer, M. Nandana, B. Prasad, R. N. T. Adrian, C. P. Simon, D. F. Robert and L. Rodrigo, Nucleic Acids Res., 2019, 47, 636–641 CrossRef.
- F. A. Stephen, L. M. Thomas, A. S. Alejandro, J. H. Zhang, Z. Zhang, M. Webb and J. L. David, Nucleic Acids Res., 1997, 25, 3389–3402 CrossRef.
- A. Waterhouse, M. Bertoni, S. Bienert, G. Studer, G. Tauriello, R. Gumienny, F. T. Heer, T. A. P. de Beer, C. Rempfer, L. Bordoli, R. Lepore and T. Schwede, Nucleic Acids Res., 2018, 46, 296–303 CrossRef.
- Y. Zhang, Bioinformatics, 2008, 9, 1–8 Search PubMed.
- J. Wang, R. M. Wolf, J. W. Caldwell, P. A. Kollma and D. A. Case, Amber 18, 2018 Search PubMed.
- A. M. James, M. Carmenza, K. Koushik, W. Lauren, E. H. Kevin and S. Carlos, J. Chem. Theory Comput., 2015, 11, 3696–3713 CrossRef.
- L. J. William, C. Jayaraman, D. M. Jeffry, W. I. Roger and L. K. Michael, J. Chem. Phys., 1983, 79, 926–935 CrossRef.
- W. P. Richard, R. B. Bernard and S. Attila, Mol. Phys., 1988, 65, 1409–1419 CrossRef.
- H. J. C. Berendsen, J. P. M. Postma, W. F. van Gunsteren, A. DiNola and J. R. Haak, J. Chem. Phys., 1984, 81, 3684–3690 CrossRef CAS.
- J. P. Ryckaert, G. Ciccotti and H. J. C. Berendsen, J. Comput. Phys., 1977, 23, 327–341 CrossRef CAS.
- T. Darden, D. York and L. Pedersen, J. Chem. Phys., 1993, 98, 10089–10092 CrossRef CAS.
- D. R. Roe and T. R. Cheatham, J. Chem. Theory Comput., 2013, 9, 3084–3095 CrossRef CAS.
- L. T. Chong, Y. Duan, L. Wang and I. Massova, Proc. Natl. Acad. Sci. U. S. A., 1999, 96, 14330–14335 CrossRef CAS.
- K. Peter, Chem. Rev., 1993, 93, 2395–2417 CrossRef.
- P. A. Kollman, I. Massova, C. Reyes, B. Kuhn, S. Huo, L. Chong, M. Lee, T. Lee, Y. Duan, W. Wang, O. Donini, P. Cieplak, J. Srinivasan, D. A. Case and T. E. Cheatham, Acc. Chem. Res., 2000, 33, 889–897 CrossRef CAS.
- S. Genheden and U. Ryde, Expert Opin. Drug Discovery, 2015, 10, 449–461 CrossRef CAS.
- W. H. Li, S. Wong, F. Li, H. K. Jens, I. C. Huang, H. Choe and M. Farzan, J. Virol., 2006, 80, 4211–4219 CrossRef CAS.
- F. Li, J. Virol., 2008, 82, 6984–6991 CrossRef CAS.
- W. Li, C. Zhang, J. Sui, J. H. Kuhn, M. J. Moore, S. Luo, S. K. Wong, I. C. Huang, K. Xu, N. Vasilieva, A. Murakami, Y. He, W. A. Marasco, Y. Guan, H. Choe and M. Farzan, EMBO J., 2005, 24, 1634–1643 CrossRef CAS.
Footnote |
† Electronic supplementary information (ESI) available. See DOI: 10.1039/d1ra00127b |
|
This journal is © The Royal Society of Chemistry 2021 |
Click here to see how this site uses Cookies. View our privacy policy here.