DOI:
10.1039/D0RA10106K
(Paper)
RSC Adv., 2021,
11, 9315-9326
Considering both small and large scale motions of vascular endothelial growth factor (VEGF) is crucial for reliably predicting its binding affinities to DNA aptamers†
Received
30th November 2020
, Accepted 23rd February 2021
First published on 1st March 2021
Abstract
Vascular endothelial growth factor 165 (VEGF165), a predominant isoform of VEGF signal proteins, is an ideal target for developing drugs against various diseases. It is composed of a heparin binding domain (HBD) and a receptor binding domain (RBD), which are connected by a flexible linker. Among the two domains, RBD is utilized in binding with the signal transduction protein, the VEGF receptor (VEGFR). None the less for its pharmaceutical importance, structure-based studies for developing drugs has been severely hindered by the lack of its whole structure determination, mainly owing to the existence of the flexible linker. Fortunately, the utilization of computer simulation methods can offer a possibility to circumvent this difficult issue. Here, we employ ensemble docking in combination with the anisotropic network model analysis to examine the interactions between DNA aptamers and VEGF165. We model three-dimensional structures of aptamer variants based on their sequence information and perform docking calculations with the whole VEGF165 structure. Indeed, we show that we can closely reproduce the experimental binding affinity order among different DNA aptamer variants by inclusively considering the flexible nature of VEGF. In addition, we address how DNA aptamer that binds to HBD of VEGF165 impedes the interaction between VEGFR and VEGF165 through RBD, even though HBD and RBD are rather distant. The present study illustrates that the flexible docking scheme employed here can be applied to tricky cases that involve flexible proteins with undetermined structures, toward effectively predicting ligand binding affinities to such proteins.
Introduction
VEGF is a signal protein that is known to regulate angiogenesis in physiologically important events such as fetal development and wound repair.1,2 It is also implicated in pathologic disorders associated with angiogenesis such as tumor growth.3–7 VEGF has many isoforms, and one of them known as VEGF165 is primarily responsible for ocular neovascularization, which often results in a serious eye disease such as age-related macular degeneration (AMD).8–11 Since AMD can cause severe loss of vision, eventually leading to blindness, many efforts have been made to cure this disease. Naturally, VEGF165 has been considered as an ideal pharmaceutical target in many of those efforts.
VEGF165 is composed of the heparin binding domain (HBD) and the receptor binding domain (RBD) which are connected by a flexible linker.8 The structure of each domain has been determined, but mainly due to the flexible linker, the whole VEGF165 structure has not been determined. The correct structural information on a target protein to start with is crucial for computational pharmaceutical studies such as docking, but proteins with undetermined structural parts due to flexible regions render the computation quite tricky. Therefore, despite the potential as a promising drug target against AMD, no docking study considering the whole structure of VEGF165 has been carried out, except one recent study that examined the interaction between VEGF165 and heparin.12 Even in that study, the flexibility of VEGF165 was not taken into account during docking.
Experimentally, aptamers are considered as important drug candidates against AMD. Aptamers are single stranded oligonucleotides that bind to target molecules with high specificity and affinity. They are generated through the systematic evolution of ligands by exponential enrichment (SELEX) process, during which aptamers are screened from large and randomized nucleic acid libraries based on their affinity to a specific target molecule.13,14 Pegaptanib was the first of its kind approved by Food and Drug Administration (FDA) of the United States for treating AMD.11 Since it exhibits affinity only to VEGF165 and not to other VEGF isoforms, it is ideal for the treatment.15 Recently, another VEGF165 binding aptamer patented in 1998 (ref. 16) was studied by considering its variants toward characterizing their relative binding affinities.17 The variants were created by extension and substitution of the original aptamer, and their relative affinities were evaluated based on kinetics and competitive binding analyses. The biophysical characterization of these interactions provides a testbed to check whether a more advanced docking scheme can reproduce experimental observables even for a tricky system with an undetermined structure like VEGF165. Of course, through the characterization, we can also access new insights toward designing new drug candidates against AMD.
Here, in this regard, we carry out docking simulations between VEGF165 and the reported DNA aptamer variants to test whether the experimentally measured affinity order can be reproduced by an advanced docking scheme. Based on molecular dynamics (MD) simulations in conjunction with docking, we also address the puzzling issue that DNA aptamers bind only to HBD but still inhibit the binding between VEGF165 and VEGF receptor (VEGFR), even though the binding takes place via the epitopes in RBD which are rather far from HBD. We expect that our scheme can be a useful tactic for handling flexible protein binding and that the additional findings will provide a clue in designing new inhibitors targeting VEGF165.
Results and discussion
VEGF165 exists as a homodimer, and each monomer is composed of HBD and RBD that are connected by a flexible linker. When it binds to the extracellular domains D2 and D3 of VEGFR, it promotes the reorientation of the VEGFR dimer, leading to a conformation suitable for activating the receptor tyrosine kinase (RTK) in the intracellular domain (Fig. 1).18 As already discussed, the flexible nature of VEGF165 has rendered the determination of its whole structure impossible. Especially, docking with the flexible HBD has not been carried out yet to the best of our knowledge. To shed light on this issue, by employing docking and MD simulations, we examined the binding between DNA aptamer variants and VEGF165 and inspected how this binding impedes the interaction between VEGF and VEGFR. We used macromolecular docking program DOT2 (ref. 19 and 20) for all docking calculations because of its excellence in describing protein–DNA interactions. A very recent study has also employed DOT2 for dockings between transmembrane protein and RNA aptamers and succeeded in discovering aptamer variants with improved binding affinities.21
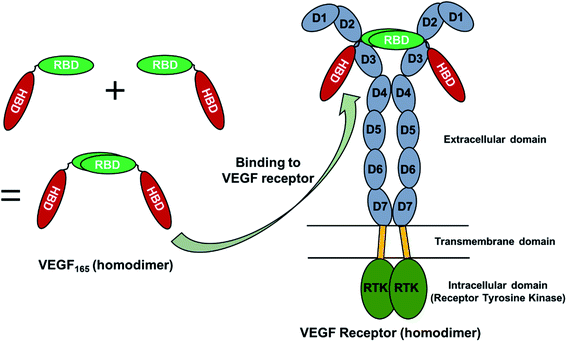 |
| Fig. 1 Schematic representation of the structural aspects of VEGF165 and its complex with VEGFR. | |
We attempted docking calculations of aptamers with VEGF165, starting from the experimental results reported by Potty et al.17 They characterized biophysical properties of a DNA aptamer 5′-CCGTCTTCCAGACAAGAGTGCAGGG-3′ (referred to as the “initial” aptamer hereafter) and its variants on their binding to VEGF165. The variants were synthesized by elongation (“0C” and “extended”) or point mutations (A14G, G16A, and G18A). Their schematic two-dimensional structures are shown in Fig. 2. They measured dissociation constants for the initial aptamer and its elongated variants, and obtained the relative binding affinities of the point mutants.17 The aptamer binding affinities to VEGF165 were measured to be in the order of extended, 0C, initial, A14G, and G16A/G18A, with G16A and G18A exhibiting comparable affinities. We built the structures of these aptamer variants based on their sequences, and the details can be found in the last section (Computational details).
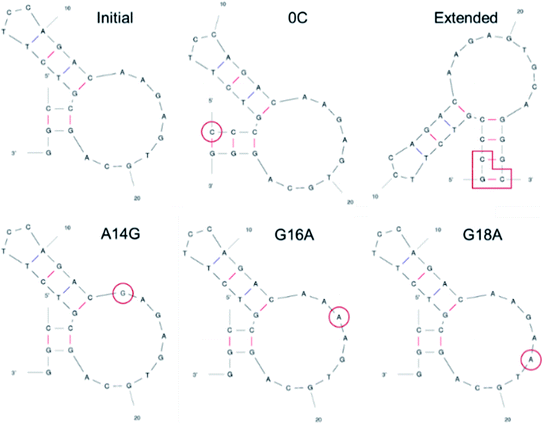 |
| Fig. 2 Two-dimensional structures of DNA aptamers adopted in this work. The structures were predicted by mfold (ref. 22). The elongated or mutated residues are marked in red. | |
Because there is no experimental VEGF165 structure, the relative pose of HBD with respect to RBD is not fixed at equilibrium and will actually keep moving. This also suggests that the corresponding potential energy minimum for the motion of HBD will be very shallow or even flat in reference to the thermal energy. Therefore, diverse VEGF165 conformations should be allowed at equilibrium. Although there is no information regarding the whole VEGF165 structure, RBD X-ray crystal structure and HBD NMR structure are available (PDB ID's: 2VPF23 and 1VGH,24 respectively). Because the NMR structure contains 20 conformations, the same number of structures for the whole VEGF165 are possible when concatenating the two domains (Fig. S1 in ESI†). The six aptamers (Fig. 2) that we created earlier were docked to these twenty VEGF165 structures. The best docking energies of these twenty VEGF165 to each aptamer are presented in Fig. 3. The results show that the docking scores with respect to the twenty VEGF165 structures are merely inconsistent with the experimental binding affinity order, extended > 0C > initial > A14G > G16A ≈ G18A.17 These poor agreements are likely due to the flexibility of the protein that we did not include in our computations. Please note that, instead of computing binding affinities with the binding energies based on free energy calculations, we used docking scores here as a metric for predicting the binding affinity order. This was because conventional free energy methods require extensive conformational sampling, which will be prohibitively costly due to the multiple pose binding nature between VEGF165 and DNA aptamers. Namely, in more conventional docking, the best pose tends to be predicted because a specific binding site normally exists for each ligand, and a free energy calculation method can be subsequently applied to the best pose toward computing the binding affinity. However, we could not find such a binding site for the DNA aptamer in HBD of VEGF, as multiple pose binding occurred. Therefore, if a free energy calculation was pursued, it would have cost an immense amount of computational time. In fact, the multiple pose binding nature between HBD of VEGF and its ligand driven by electrostatic interactions has also been reported previously.12 In addition, we note that binding affinities have been reasonably reproduced by docking scores without actual free energy calculations.25,26
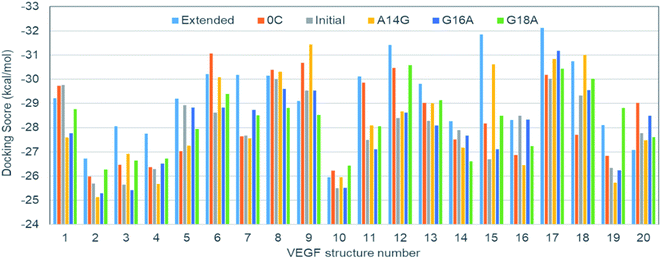 |
| Fig. 3 Docking scores of DNA aptamer variants to the twenty VEGF165 structures. | |
Thus, it is clear that we have to somehow include the flexible nature of the protein for our computations. As a matter of fact, properly considering various protein flexibilities has been a challenging task not just in free energy calculations but also in any computations including docking. Although the thermal fluctuations of the backbone and the surface residues can be taken into account to some degree by adopting ensemble docking,27,28 large scale motions such as HBD movement in VEGF165 will be very expensive to sample statistically. To circumvent this issue, we carried out the anisotropic network model (ANM) analysis for the twenty concatenated VEGF165 conformations created earlier. This will enable us to examine the principal movements based on the simplified normal mode analysis.29–31 Among the computed normal modes, we focused on the lowest frequency one because that will be the lowest energy vibration whose motion is energetically most favored. Furthermore, regardless of the conformation identified from the twenty possibilities used for the ANM analysis, the lowest frequency normal mode motion always appeared similar. In fact, the motion could be described as the scissoring bending vibration by the two HBD units, resulting in oscillations of the distance between the two units (Fig. 4A).
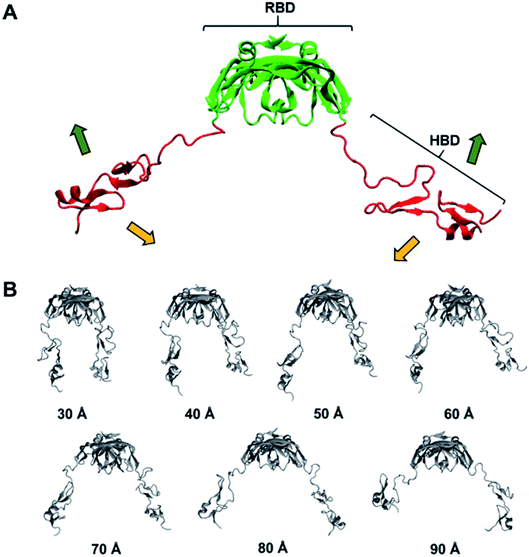 |
| Fig. 4 (A) Lowest frequency normal mode obtained from ANM. (B) Seven VEGF165 conformations generated from biased MD simulations. | |
In order to reflect the effect of this lowest frequency normal mode in docking, we generated several different VEGF165 conformations by varying the distance between the HBD units starting from the one that bears largely separated HBD units (“Structure 11” in Fig. S1†). For that, we carried out biased MD simulations with a restraint on the distance between the two units in the range of 30–90 Å at an interval of 10 Å, eventually extracting seven different VEGF conformations at as many inter-unit distances (Fig. 4B). In principle, computing a free energy profile along the inter-HBD distance can also be considered, but our system in combination with a large number of explicit water molecules is excessively large for conducting the required sampling. Instead, we then performed docking simulations with the six DNA aptamer variants to these seven VEGF165 conformations, yielding a total of 42 docking calculations. The lowest energy from each docking and the average from the seven VEGF165 conformations are plotted in Fig. 5A. Even after considering the VEGF165 conformational flexibility from the lowest frequency normal mode, the predicted binding affinity order from docking simulations is still inconsistent with the experimental results. In addition, almost no correlations between the docking scores and the logarithms of the dissociation constants were found (Fig. 5B) for the three variants (initial, 0C, and extended) whose dissociation constants are available from experiments.
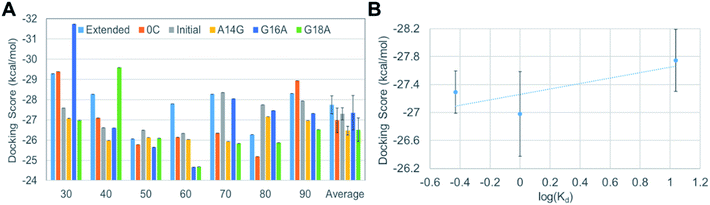 |
| Fig. 5 (A) Docking scores of DNA aptamer variants to the seven VEGF165 structures generated along the lowest frequency normal mode. (B) Correlation between the computed docking scores and the experimental dissociation constants. Error bars represent statistical standard errors. | |
Although we did not observe a satisfactory trend yet, meanwhile, we found that all DNA aptamer variants bound only to HBD in these 42 docking simulations. In fact, this binding specificity is in line with a previous experimental observation on a well-known DNA aptamer, Macugen.32 This correct aspect has motivated us to perform new docking simulations between the DNA aptamers and the HBD. Because the computational time for docking can be significantly reduced with this approach, at this time, we decided to carry out ensemble docking toward considering small-scale motions of the protein. Toward this end, we generated 50 snapshots of HBD using MD simulations. We then performed docking of the six DNA aptamers with the 50 HBD snapshots, obtaining 300 docking results in total. The energies computed from these dockings are shown in the leftmost part of Fig. 6A. Now the results reproduce the experimental binding affinity order well, except one slightly outlying mutant A14G. The correlation between docking scores and dissociation constants is also improved remarkably, although it is not yet quantitatively agreeing (Fig. 6B).
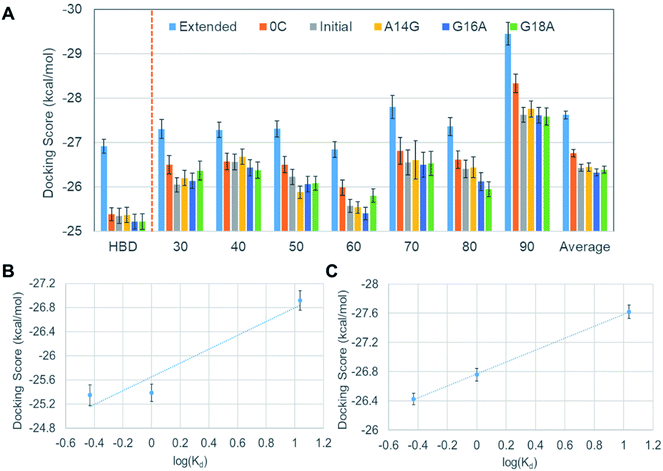 |
| Fig. 6 (A) Average docking scores of the DNA aptamer variants to HBD and various VEGF165 structures, (B) the correlation between docking scores and the dissociation constants obtained after considering small-scale fluctuations in HBD with 50 MD-sampled conformations, and (C) the same correlation after considering all 350 VEGF165 structures. Error bars represent statistical standard errors. | |
Encouraged by the fact that the docking with HBD ensemble after considering small-scale motions showed noticeable improvements in predicting both binding affinity order and the energy correlation, we decided to further consider both the large and the small-scale motions simultaneously. To this end, 50 snapshots for all seven separations by the lowest frequency normal mode were taken into account, together with the six aptamers. This resulted in a total of 2100 docking results (i.e. 350 VEGF165 snapshots and six DNA aptamers). The results in Fig. 6A show that the averages from these are now in good agreement with the experimental binding affinity order except the slight affinity overestimation for A14G in comparison with the initial aptamer. Of course, the energy “resolution” of the docking result will not be good enough to discriminate the small affinity difference between the initial aptamer and A14G. Even still, the averages from 350 dockings at each distance (30 to 90 Å) predict significantly better binding affinity order compared to the single docking results shown in Fig. 3. This again substantiates the importance of ensemble docking. In addition, an almost perfect correlation between the docking scores and the experimental dissociation constants is observed at this time (Fig. 6C). Considering that this correlation is a large improvement over the ensemble docking results that involved only one HBD (Fig. 6B), we can infer that the whole structure of VEGF165 should be taken into account toward docking for a more reliable prediction of the binding affinity order. In fact, an earlier electrostatic potential isosurface analysis has shown that HBD is predominated by positive surface charges.12 Therefore, one HBD unit will likely influence the binding of a negatively charged DNA aptamer on the other HBD unit. This explains the necessity of adopting the whole VEGF165 for a reliable prediction. In addition, it is noteworthy that DNA aptamers bind to the HBD surface without any specificity, indicating that the binding is mainly driven by electrostatic interactions rather than shape complementarity. Such a multiple pose binding nature of HBD has been also reported previously against heparin ligand.12
Although we closely reproduced the binding affinity order between VEGF165 and DNA aptamers based on docking scores, there are still two issues to be clarified in order to substantiate the validity of our docking scheme. One is the validity of considering only lowest frequency normal mode in generating large-scale motions of VEGF165, and the other is the validity of examining only one initial structure (Structure 11 in Fig. S1†) among the twenty. To address the first issue, we additionally tested VEGF165 conformations based on the linear combination of six lowest frequency normal modes by using the NMSim web server.33,34 We generated 350 VEGF165 structures, which is the same number that we used for docking when considering only the lowest frequency normal mode. Since the number of VEGF structures for docking is identical, we can directly compare the results from our earlier docking scheme and this new one with NMSim. The docking results are shown in the leftmost part of Fig. 7A, together with the corresponding correlation given in Fig. 7B. Although we included as many as six normal modes, the results changed only marginally compared to the matching average from using one mode given in Fig. 6A. To address the second issue, we picked another VEGF165 structure (“Structure 17” in Fig. S1†), which is the most different from Structure 11 in terms of the root-mean-squared distance (RMSD). We then generated seven VEGF165 conformations along the distance between the HBD units (Fig. S2†). With these conformations, we repeated the same calculations as with Structure 11, resulting in a total of 2100 docking scores (Fig. 7A and C). The results indeed show similar improvements in binding affinity prediction as with Structure 11. This surely indicates that our docking scheme will work well regardless of which initial structure we choose.
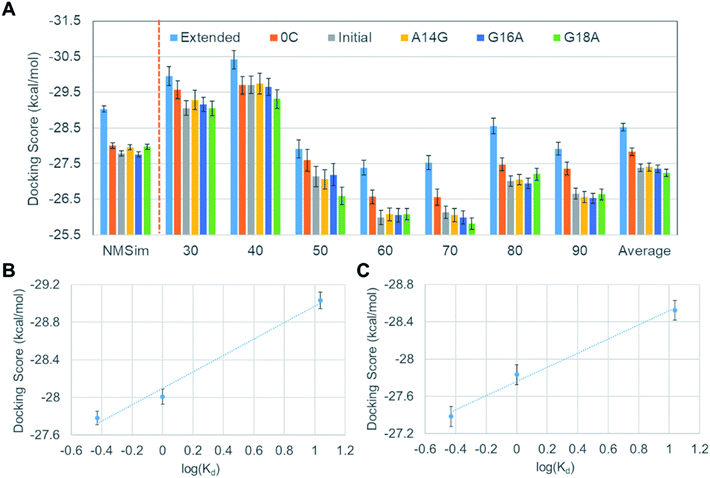 |
| Fig. 7 (A) Average docking scores of the DNA aptamer variants to VEGF165 structures from NMSim and to additional structures derived from Structure 17, (B) the correlation between docking scores and the dissociation constants with NMSim, and (C) the same correlation for the docking results with Structure 17. Error bars represent statistical standard errors. | |
As described earlier, from our docking, we observed that all DNA aptamers interact with HBD only. An earlier study also reported this binding specificity of DNA aptamers.32 On the other hand, VEGFR binds to VEGF165 through its RBD, which is rather distant from HBD. Thus, the fact that aptamer binding on HBD can impede the VEGFR–VEGF165 binding may be puzzling. The answer to this puzzle is actually intriguing and may provide a new insight into the development of new VEGF/VEGFR antagonists to inhibit angiogenesis. This is also notable, as many researchers have tried designing new antagonist peptides by targeting on RBD.35–42 In order to address this, we performed MD simulations of the initial aptamer bound on VEGF165 without fixing any atoms in space. For VEGF165, we chose the conformation with the inter-HBD distance value of 30 Å as it would reflect the fact better that the negatively charged DNA aptamer interacts with both HBD units with positive surface charges.12 We performed the trajectory simulation until the RMSD from the initial structure converged (40 ns, Fig. S3†). As expected, during the simulation the DNA aptamer attached itself to the other HBD, and this additional binding caused the two HBD unit to twist somewhat. This twist in turn triggered one of the HBD units to strongly interact with the L1 loop of RBD (Fig. 8). Indeed, according to an X-ray crystal structure,43 the L1 loop of RBD is known to interact with the D3 domain of VEGFR1 when they bind together. Especially, the Glu44 residue of RBD was shown to interact with Gln263 of D3 in VEGFR1. Interestingly, in the complex of VEGF165 with DNA aptamer from the MD simulation, this Glu44 of RBD formed a strong hydrogen bond with Arg124 of HBD. Moreover, seven additional hydrogen bonds were observed between HBD and RBD besides the hydrogen bond of Glu44 with Arg124. Consequently, these strong interactions between HBD and RBD are expected to effectively block the interaction between RBD and VEGFR1.
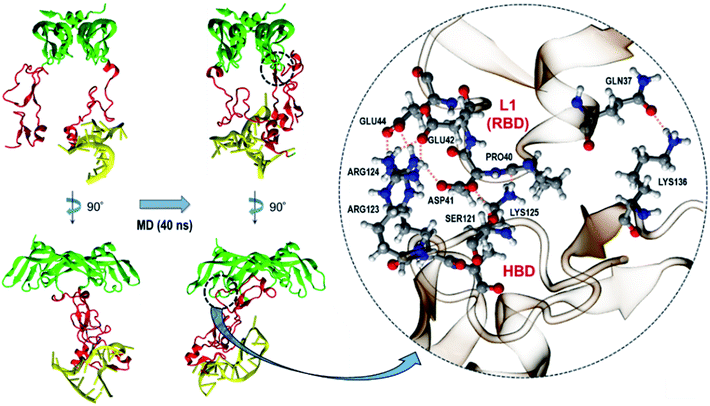 |
| Fig. 8 Conformational change of VEGF165 upon binding to a DNA aptamer. HBD, RBD, and DNA aptamer are colored in red, green, and yellow, respectively. The interacting residues between HBD and RBD are shown in the circled inset. | |
Based on the equilibrated structure of the VEGF165–DNA aptamer complex obtained from the MD simulations, we further examined how much this binding spatially interferes with the interaction between VEGF165 and VEGFR. To this end, we adopted the X-ray crystal structure of a complex between RBD of VEGF165 and VEGFR1 (PDB ID: 5T89),43 and overlaid our structure of VEGF165–DNA aptamer complex on it. The overlay was performed after aligning the structures with respect to the RBD units from our simulation result and the crystallography data. For comparison, we also overlaid free VEGF165 with closed and opened HBD units, respectively represented by the conformations at 30 and 90 Å inter-HBD separations displayed in Fig. 4B. We focused our attention especially on the change in HBD–VEGFR overlap induced by the introduction of the DNA aptamer binding to VEGF165. The overlaid structures are shown in Fig. 9. With free VEGF165, there is no significant spatial overlaps observed regardless of how widely the HBD pair opens. The observed overlaps are indeed quite small such that they can be avoided with the natural protein flexibility. What is noteworthy here is that, although a previous computational study predicted that VEGF165 with closed HBDs would bind to VEGFR more preferably than VEGF165 with opened HBDs,12 our results are not in line with that prediction. On the contrary, in our results, VEGF165 with closed HBDs (Fig. 9A) exhibits more spatial overlap with VEGFR1 than the one with the opened pair (Fig. 9B). Because VEGFR2 was used for the modeling in that previous study, the inconsistency may have arisen from the structural differences between VEGFR1 and VEGFR2. At least, the binding of VEGF165 with opened HBDs to VEGFR1 is not disfavored according to our results. In the case of the binding between the VEGF165–DNA aptamer complex and VEGFR1, the aptamer-bound HBD completely overlaps with the D3 domain of VEGFR1, showing far larger spatial clash compared to the case with the free VEGF165 (Fig. 9C). This again confirms that the native interaction between VEGF165 and VEGFR1 will be severely hindered when the DNA aptamer is bound to VEGF165.
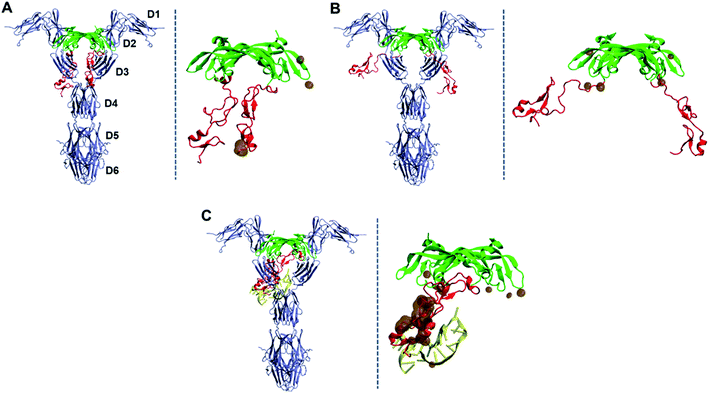 |
| Fig. 9 Hypothetical binding of VEGF165 to VEGFR1 based on the overlay of the simulated structures of VEGF165 on the X-ray crystal structure of RBD–VEGFR1 complex. (A) VEGF165–VEGFR1 without a DNA aptamer at 30 Å of inter-HBD separation. (B) VEGF165–VEGFR1 without a DNA aptamer at 90 Å of inter-HBD separation. (C) VEGF165–VEGFR1 with the initial aptamer bound to the HBD domain. The overlapping heavy atoms of VEGF165, whose distance to any atom in VEGFR is shorter than 1.5 Å, are also shown with brown surfaces in each panel. The domain names of VEGFR1 are also marked in (A). DNA aptamer, HBD, RBD, and VEGFR1 are colored in yellow, red, green, and blue, respectively. | |
Conclusions
Due to its direct involvement in angiogenesis, VEGF165 has been a main target of many designed molecules that aim for inhibiting angiogenesis. Nevertheless, the flexible nature of HBD has rendered various structure-based analyses of VEGF165 very difficult. To make a step forward in this challenging task, we examined the binding of DNA aptamers to HBD through docking and show how this binding can eventually impede the interaction between VEGF165 and VEGFR. We found that ensemble docking with considerations on the backbone and side chain flexibility is crucial for correctly predicting the binding affinity order among different aptamer variants. In addition to small-scale motions, we found that properly considering a large domain motion of VEGF165 as obtained with the ANM analysis is essential in docking toward correctly reproducing the correlation between docking scores and dissociation constants. Once a DNA aptamer binds to one of the HBD units in VEGF165, it subsequently attaches itself to the other HBD. This in turn causes a strong interaction of HBD with the L1 loop of RBD through a number of hydrogen bonds. Because the L1 loop of RBD is a binding site for VEGFR, this will eventually lead to the inhibition of the interaction between VEGF165 and VEGFR. The present work demonstrates that each of two flexible HBD units of VEGF165 affects the aptamer binding as well as the interaction between VEGF165 and VEGFR. This may provide an important design rule to devise new antagonist molecules against VEGF165 to control angiogenesis.
Computational details
DNA aptamer modeling
Because the 3D structure of the aptamer variants are not available, we modeled each structure based on the sequence information. For this, we referred to a previously suggested approach44 and used mfold web server22 to generate the 2D structures of the aptamers using their sequences as inputs. When we tried an alternative, RNA structure web server,45 to see the robustness of the prediction by mfold, we obtained exactly the same 2D DNA structures. Predicting the 3D DNA structure from such 2D information is not practically possible, and we built an equivalent 3D structure of an RNA sequence using the RNA composer46,47 first and then subsequently converted it into DNA by manually modifying corresponding atoms. We also tried other alternatives in predicting the 3D RNA structures, namely, 3dRNA,48 SimRNA,49 and MC-Sym.50 However, they gave either energetically unstable structures or even abnormal structures with extremely elongated covalent bonds. Therefore, we decided to use RNA composer for this step. After this, we refined each structure by an energy minimization for 500 steps with the ff14SB AMBER force field parameter set51 and the generalized Born solvent model.52
VEGF165 modeling
In order to build the whole VEGF165 structure, we used RBD taken from an X-ray crystal structure (PDB ID: 2VPF)23 and HBD from an NMR structure (PDB ID: 1VGH).24 Because two amino acids, Asp109 and Arg110, in a flexible linker connecting RBD and HBD are missing in both 2VPF and 1VGH, we first built a peptide chain Lys108–Asp109–Arg110–Ala111 using Avogadro.53 This chain was used as a bridge to connect Lys108 of RBD and Ala111 of HBD. Similarly to the DNA aptamer case, the concatenated VEGF165 structure was further refined by 500 steps of minimization with the ff14SB51 force field and the generalized Born solvent model.52 The ANM analysis of VEGF165 was performed using ANM web server 2.0.31
MD simulations
The modeled structures were first solvated in a truncated octahedral TIP3P water box54 with the minimum distance of 10 Å from any atom in the solute to the edge of the water box. The solvated structures were subsequently neutralized by adding counter ions and minimized for 500 steps to avoid any possible steric clashes. The minimized structures were then gradually heated up to 300 K with positional restraints on the solute. These restraints were removed gradually, and the structures were equilibrated for 2 ns prior to any further calculations. Periodic boundary condition was employed for all MD simulations, and the cut-off distance of 8 Å was used for non-bonded interactions. Particle Mesh Ewald (PME) summation was employed for handling long range electrostatic interactions55 while the continuum model was adopted for treating long range van der Waals interactions.56 The time step of 2 fs was utilized, and the SHAKE algorithm was used to fix the bonds including hydrogen atoms.57 To keep the temperature constant during MD simulations, the Langevin thermostat was employed, and the pressure was kept at 1 bar by using Berendsen's weak-coupling algorithm.58 In order to generate seven discrete conformations of VEGF165 along the distance between the two HBD units, we performed MD simulations with a biasing potential represented by a force constant of 40 kcal mol−1 Å−2. The 50 snapshots to carry out ensemble docking were extracted from each biased trajectory (10 ns) with the different inter-HBD distances. All MD simulations were carried out using AMBER 15.56
Docking with DOT2
All dockings performed in this work used the DOT2 software. DOT2 is a docking program for rigid-body docking between two macromolecules.19,20 The notable strength of DOT2 is its capability of computing the electrostatic potential of molecules thoroughly based on Poisson–Boltzmann methods,59,60 so it is particularly superior in the estimation of electrostatic interactions between highly polar molecules such as protein–DNA binding.61,62 Due to this advantage, DOT2 has been employed for docking for various protein–DNA interactions, such as DNA–DNA repair enzymes,63–66 the nucleosome,67 and viral DNA-integrase.68,69 DOT2 calculates intermolecular energies as the sum of electrostatic and van der Waals (VDW) energy terms. We used the AMBER library (uhbd.amber84.prot.dna.rlb)70 as the residue library to assign partial atomic charges for both VEGF165 and DNA aptamer. The grid points with the spacing of 1 Å were generated for VEGF165, and the electrostatic potential of VEGF165 was computed for each grid by an outside program Adaptive Poisson–Boltzmann Solver (APBS) based on the linearized Poisson–Boltzmann equation.71 To compute the VDW interaction energies, the shape of VEGF165 was determined first by its volume surrounded by a 3 Å thick layer using MSMS,72 and then each heavy atom of the DNA aptamer inside this layer was computed to contribute 0.1 kcal mol−1 to the VDW interaction energy. At each grid, the interaction energy between VEGF165 and the aptamer was calculated for 54
000 evenly spaced orientations (6° spacing) of the DNA aptamer under the potential of VEGF165 at 300 K. During docking calculations, the atoms of the DNA aptamer were not allowed to spatially overlap with VEGF165 within the distance of their VDW radii.
Conflicts of interest
There are no conflicts to declare.
Acknowledgements
This work was financially supported by the Creative Materials Discovery Program (Grant 2018M3D1A1058813) through National Research Foundation, funded by Ministry of Science and ICT of Korea.
References
- W. Risau, Mechanisms of angiogenesis, Nature, 1997, 386, 671–674 CrossRef CAS.
- P. Carmeliet, Angiogenesis in life, disease and medicine, Nature, 2005, 438, 932–936 CrossRef CAS.
- D. Hanahan and R. A. Weinberg, Hallmarks of cancer: the next generation, Cell, 2011, 144, 646–674 CrossRef CAS.
- G. Azizi, R. Boghozian and A. Mirshafiey, The potential role of angiogenic factors in rheumatoid arthritis, Int. J. Rheum. Dis., 2014, 17, 369–383 CrossRef CAS.
- J. W. Miller, J. Le Couter, E. C. Strauss and N. Ferrara, Vascular endothelial growth factor a in intraocular vascular disease, Ophthalmology, 2013, 120, 106–114 CrossRef.
- I. D. Pousa, J. Maté and J. P. Gisbert, Angiogenesis in inflammatory bowel disease, Eur. J. Clin. Invest., 2008, 38, 73–81 CrossRef CAS.
- N. Ved, R. P. Hulse, S. M. Bestall, L. F. Donaldson, J. W. Bainbridge and D. O. Bates, Vascular endothelial growth factor-A165b ameliorates outer-retinal barrier and vascular dysfunction in the diabetic retina, Clin. Sci., 1979, 131, 1225–1243 CrossRef.
- N. Ferrara, H.-P. Gerber and J. LeCouter, The biology of VEGF and its receptors, Nat. Med., 2003, 9, 669–676 CrossRef CAS.
- A. N. Witmer, G. F. J. M. Vrensen, C. J. F. Van Noorden and R. O. Schlingemann, Vascular endothelial growth factors and angiogenesis in eye disease, Prog. Retinal Eye Res., 2003, 22, 1–29 CrossRef CAS.
- S. Ishida, T. Usui, K. Yamashiro, Y. Kaji, S. Amano, Y. Ogura, T. Hida, Y. Oguchi, J. Ambati, J. W. Miller, E. S. Gragoudas, Y.-S. Ng, P. A. D'Amore, D. T. Shima and A. P. Adamis, VEGF164-mediated inflammation is required for pathological, but not physiological, ischemia-induced retinal neovascularization, J. Exp. Med., 2003, 198, 483–489 CrossRef CAS.
- E. W. M. Ng and A. P. Adamis, Targeting angiogenesis, the underlying disorder in neovascular age-related macular degeneration, Can. J. Ophthalmol., 2005, 40, 352–368 CrossRef.
- U. Uciechowska-Kaczmarzyk, S. Babik, F. Zsila, K. K. Bojarski, T. Beke-Somfai and S. A. Samsonov, Molecular dynamics-based model of VEGF-A and its heparin interactions, J. Mol. Graphics Modell., 2018, 82, 157–166 CrossRef CAS.
- C. Tuerk and L. Gold, Systematic evolution of ligands by exponential enrichment: RNA ligands to bacteriophage T4 DNA polymerase, Science, 1990, 249, 505–510 CrossRef CAS.
- S. M. Nimjee, C. P. Rusconi and B. A. Sullenger, Aptamers: an emerging class of therapeutics, Annu. Rev. Med., 2005, 56, 555–583 CrossRef CAS.
- J. Ruckman, L. S. Green, J. Beeson, S. Waugh, W. L. Gillette, D. D. Henninger, L. Claesson-Welsh and N. Janjić, 2′-Fluoropyrimidine RNA-based aptamers to the 165-amino acid form of vascular endothelial growth factor (VEGF165). Inhibition of receptor binding and VEGF-induced vascular permeability through interactions requiring the exon 7-encoded domain, J. Biol. Chem., 1998, 273, 20556–20567 CrossRef CAS.
- L. Gold and N. Janjic, US Pat., 669625, 1998 Search PubMed.
- A. S. R. Potty, K. Kourentzi, H. Fang, G. W. Jackson, X. Zhang, G. B. Legge and R. C. Willson, Biophysical characterization of DNA aptamer interactions with vascular endothelial growth factor, Biopolymers, 2009, 91, 145–156 CrossRef CAS.
- S. Sarabipour, K. Ballmer-Hofer and K. Hristova, VEGFR-2 conformational switch in response to ligand binding, eLife, 2016, 5, e13876 CrossRef.
- J. G. Mandell, V. A. Roberts, M. E. Pique, V. Kotlovyi, J. C. Mitchell, E. Nelson, I. Tsigelny and L. F. Ten Eyck, Protein docking using continuum electrostatics and geometric fit, Protein Eng., Des. Sel., 2001, 14, 105–113 CrossRef CAS.
- V. A. Roberts, E. E. Thompson, M. E. Pique, M. S. Perez and L. F. Ten Eyck, DOT2: macromolecular docking with improved biophysical models, J. Comput. Chem., 2013, 34, 1743–1758 CrossRef CAS.
- D. R. Bell, J. K. Weber, W. Yin, T. Huynh, W. Duan and R. Zhou, In silico design and validation of high-affinity RNA aptamers targeting epithelial cellular adhesion molecule dimers, Proc. Natl. Acad. Sci. U. S. A., 2020, 117, 8486–8493 CrossRef CAS.
- M. Zuker, Mfold web server for nucleic acid folding and hybridization prediction, Nucleic Acids Res., 2003, 31, 3406–3415 CrossRef CAS.
- Y. A. Muller, H. W. Christinger, B. A. Keyt and A. M. de Vos, The crystal structure of vascular endothelial growth factor (VEGF) refined to 1.93 a resolution: multiple copy flexibility and receptor binding, Structure, 1993, 5, 1325–1338 CrossRef.
- W. J. Fairbrother, M. A. Champe, H. W. Christinger, B. A. Keyt and M. A. Starovasnik, Solution structure of the heparin-binding domain of vascular endothelial growth factor, Structure, 1998, 6, 637–648 CrossRef CAS.
- M. L. Verdonk, J. C. Cole, M. J. Hartshorn, C. W. Murray and R. D. Taylor, Improved protein–ligand docking using GOLD, Proteins, 2003, 52, 609–623 CrossRef CAS.
- M. M. Gromiha, K. Yugandhar and S. Jemimah, Protein–protein interactions: scoring schemes and binding affinity, Curr. Opin. Struct. Biol., 2017, 44, 31–38 CrossRef CAS.
- H. A. Carlson, K. M. Masukawa and J. A. McCammon, Method for Including the Dynamic Fluctuations of a Protein in Computer-Aided Drug Design, J. Phys. Chem. A, 1999, 103, 10213–10219 CrossRef CAS.
- R. E. Amaro, J. Baudry, J. Chodera, Ö. Demir, J. A. McCammon, Y. Miao and J. C. Smith, Ensemble Docking in Drug Discovery, Biophys. J., 2018, 114, 2271–2278 CrossRef CAS.
- A. R. Atilgan, S. R. Durell, R. L. Jernigan, M. C. Demirel, O. Keskin and I. Bahar, Anisotropy of fluctuation dynamics of proteins with an elastic network model, Biophys. J., 2001, 80, 505–515 CrossRef CAS.
- P. Doruker, A. R. Atilgan and I. Bahar, Dynamics of proteins predicted by molecular dynamics simulations and analytical approaches: application to α-amylase inhibitor, Proteins: Struct., Funct., Bioinf., 2000, 40, 512–524 CrossRef CAS.
- E. Eyal, G. Lum and I. Bahar, The anisotropic network model web server at 2015 (ANM 2.0), Bioinformatics, 2015, 31, 1487–1489 CrossRef CAS.
- J.-H. Lee, M. D. Canny, A. De Erkenez, D. Krilleke, Y.-S. Ng, D. T. Shima, A. Pardi and F. Jucker, A therapeutic aptamer inhibits angiogenesis by specifically targeting the heparin binding domain of VEGF165, Proc. Natl. Acad. Sci. U. S. A., 2005, 102, 18902–18907 CrossRef CAS.
- A. Ahmed, F. Rippmann, G. Barnickel and H. Gohlke, A normal mode-based geometric simulation approach for exploring biologically relevant conformational transitions in proteins, J. Chem. Inf. Model., 2011, 51, 1604–1622 CrossRef CAS.
- D. M. Krüger, A. Ahmed and H. Gohlke, NMSim web server: integrated approach for normal mode-based geometric simulations of biologically relevant conformational transitions in proteins, Nucleic Acids Res., 2012, 40, W310–W316 CrossRef.
- V. Goncalves, B. Gautier, P. Coric, S. Bouaziz, C. Lenoir, C. Garbay, M. Vidal and N. Inguimbert, Rational Design, Structure, and Biological Evaluation of Cyclic Peptides Mimicking the Vascular Endothelial Growth Factor, J. Med. Chem., 2007, 50, 5135–5146 CrossRef CAS.
- B. Gautier, V. Goncalves, D. Diana, R. Di Stasi, F. Teillet, C. Lenoir, F. Huguenot, C. Garbay, R. Fattorusso, L. D. D'Andrea, M. Vidal and N. Inguimbert, Biochemical and Structural Analysis of the Binding Determinants of a Vascular Endothelial Growth Factor Receptor Peptidic Antagonist, J. Med. Chem., 2010, 53, 4428–4440 CrossRef CAS.
- A. Basile, A. Del Gatto, D. Diana, R. Di Stasi, A. Falco, M. Festa, A. Rosati, A. Barbieri, R. Franco, C. Arra, C. Pedone, R. Fattorusso, M. C. Turco and L. D. D'Andrea, Characterization of a Designed Vascular Endothelial Growth Factor Receptor Antagonist Helical Peptide with Antiangiogenic Activity in Vivo, J. Med. Chem., 2011, 54, 1391–1400 CrossRef CAS.
- L. Wang, N. Gagey-Eilstein, S. Broussy, M. Reille-Seroussi, F. Huguenot, M. Vidal and W.-Q. Liu, Design and synthesis of C-terminal modified cyclic peptides as VEGFR1 antagonists, Materials, 2014, 19, 15391–15407 Search PubMed.
- L. De Rosa, F. Finetti, D. Diana, R. Di Stasi, S. Auriemma, A. Romanelli, R. Fattorusso, M. Ziche, L. Morbidelli and L. D. D'Andrea, Miniaturizing VEGF: Peptides mimicking the discontinuous VEGF receptor-binding site modulate the angiogenic response, Sci. Rep., 2016, 6, 31295 CrossRef CAS.
- L. Wang, L. Zhou, M. Reille-Seroussi, N. Gagey-Eilstein, S. Broussy, T. Zhang, L. Ji, M. Vidal and W.-Q. Liu, Identification of Peptidic Antagonists of Vascular Endothelial Growth Factor Receptor 1 by Scanning the Binding Epitopes of Its Ligands, J. Med. Chem., 2017, 60, 6598–6606 CrossRef CAS.
- A. Sadremomtaz, K. Mansouri, G. Alemzadeh, M. Safa, A. E. Rastaghi and S. M. Asghari, Dual blockade of VEGFR1 and VEGFR2 by a novel peptide abrogates VEGF-driven angiogenesis, tumor growth, and metastasis through PI3K/AKT and MAPK/ERK1/2 pathway, Biochim. Biophys. Acta, Gen. Subj., 2018, 1862, 2688–2700 CrossRef CAS.
- M. F. Behelgardi, S. Zahri, F. Mashayekhi, K. Mansouri and S. M. Asghari, A peptide mimicking the binding sites of VEGF-A and VEGF-B inhibits VEGFR-1/-2 driven angiogenesis, tumor growth and metastasis, Sci. Rep., 2018, 8, 17924 CrossRef.
- S. Markovic-Mueller, E. Stuttfeld, M. Asthana, T. Weinert, S. Bliven, K. N. Goldie, K. Kisko, G. Capitani and K. Ballmer-Hofer, Structure of the Full-length VEGFR-1 Extracellular Domain in Complex with VEGF-A, Structure, 1993, 25, 341–352 CrossRef.
- I. Jeddi and L. Saiz, Three-dimensional modeling of single stranded DNA hairpins for aptamer-based biosensors, Sci. Rep., 2017, 7, 1178 CrossRef.
- J. S. Reuter and D. H. Mathews, RNAstructure: software for RNA secondary structure prediction and analysis, BMC Bioinf., 2010, 11, 129 CrossRef.
- M. Popenda, M. Szachniuk, M. Antczak, K. J. Purzycka, P. Lukasiak, N. Bartol, J. Blazewicz and R. W. Adamiak, Automated 3D structure composition for large RNAs, Nucleic Acids Res., 2012, 40, e112 CrossRef CAS.
- M. Antczak, M. Popenda, T. Zok, J. Sarzynska, T. Ratajczak, K. Tomczyk, R. W. Adamiak and M. Szachniuk, New functionality of RNAComposer: an application to shape the axis of miR160 precursor structure, Acta Biochim. Pol., 2016, 63, 737–744 CAS.
- J. Wang, K. Mao, Y. Zhao, C. Zeng, J. Xiang, Y. Zhang and Y. Xiao, Optimization of RNA 3D structure prediction using evolutionary restraints of nucleotide–nucleotide interactions from direct coupling analysis, Nucleic Acids Res., 2017, 45, 6299–6309 CrossRef CAS.
- M. Magnus, M. J. Boniecki, W. Dawson and J. M. Bujnicki, SimRNAweb: a web server for RNA 3D structure modeling with optional restraints, Nucleic Acids Res., 2016, 44, W315–W319 CrossRef CAS.
- M. Parisien and F. Major, The MC-Fold and MC-Sym pipeline infers RNA structure from sequence data, Nature, 2008, 452, 51–55 CrossRef CAS.
- J. A. Maier, C. Martinez, K. Kasavajhala, L. Wickstrom, K. E. Hauser and C. Simmerling, ff14SB: Improving the Accuracy of Protein Side Chain and Backbone Parameters from ff99SB, J. Chem. Theory Comput., 2015, 11, 3696–3713 CrossRef CAS.
- W. C. Still, A. Tempczyk, R. C. Hawley and T. Hendrickson, Semianalytical treatment of solvation for molecular mechanics and dynamics, J. Am. Chem. Soc., 1990, 112, 6127–6129 CrossRef CAS.
- M. D. Hanwell, D. E. Curtis, D. C. Lonie, T. Vandermeersch, E. Zurek and G. R. Hutchison, Avogadro: an advanced semantic chemical editor, visualization, and analysis platform, J. Cheminf., 2012, 4, 17 CAS.
- W. L. Jorgensen, J. Chandrasekhar, J. D. Madura, R. W. Impey and M. L. Klein, Comparison of Simple Potential Functions for Simulating Liquid Water, J. Chem. Phys., 1983, 79, 926 CrossRef CAS.
- U. Essmann, L. Perera, M. L. Berkowitz, T. Darden, H. Lee and L. G. Pedersen, A smooth particle mesh Ewald method, J. Chem. Phys., 1995, 103, 8577–8593 CrossRef CAS.
- D. Case, J. Berryman, R. Betz, D. Cerutti, T. Cheatham III, T. Darden, R. Duke, T. Giese, H. Gohlke, A. Goetz, N. Homeyer, S. Izadi, P. Janowski, J. Kaus, A. Kovalenko, T. Lee, S. LeGrand, P. Li, T. Luchko, R. Luo, B. Madej, K. Merz, G. Monard, P. Needham, H. Nguyen, H. Nguyen, I. Omelyan, A. Onufriev, D. Roe, A. Roitberg, R. Solomon-Ferrer, C. Simmerling, W. Smith, J. Swails, R. Walker, J. Wang, R. Wolf, X. Wu, D. York and P. Kollman, AMBER 2015, University of California, San Francisco, 2015 Search PubMed.
- J.-P. Ryckaert, G. Ciccotti and H. J. C. Berendsen, Numerical integration of the cartesian equations of motion of a system with constraints: molecular dynamics of n-alkanes, J. Comput. Phys., 1977, 23, 327–341 CrossRef CAS.
- H. J. C. Berendsen, J. P. M. Postma, W. F. van Gunsteren, A. DiNola and J. R. Haak, Molecular dynamics with coupling to an external bath, J. Chem. Phys., 1984, 81, 3684–3690 CrossRef CAS.
- M. Gouy, Sur la constitution de la charge électrique à la surface d'un électrolyte, J. Phys. Theor. Appl., 1910, 9, 457–468 CrossRef.
- D. L. Chapman, LI. A contribution to the theory of electrocapillarity, London, Edinburgh Dublin Philos. Mag. J. Sci., 1913, 25, 475–481 CrossRef.
- V. A. Roberts, D. A. Case and V. Tsui, Predicting interactions of winged-helix transcription factors with DNA, Proteins: Struct., Funct., Bioinf., 2004, 57, 172–187 CrossRef CAS.
- V. A. Roberts, M. E. Pique, L. F. Ten Eyck and S. Li, Predicting protein–DNA interactions by full search computational docking, Proteins, 2013, 81, 2106–2118 CrossRef CAS.
- K. P. Hopfner, A. Karcher, L. Craig, T. T. Woo, J. P. Carney and J. A. Tainer, Structural biochemistry and interaction architecture of the DNA double-strand break repair Mre11 nuclease and Rad50-ATPase, Cell, 2001, 105, 473–485 CrossRef CAS.
- L. Fan, J. O. Fuss, Q. J. Cheng, A. S. Arvai, M. Hammel, V. A. Roberts, P. K. Cooper and J. A. Tainer, XPD helicase structures and activities: insights into the cancer and aging phenotypes from XPD mutations, Cell, 2008, 133, 789–800 CrossRef CAS.
- M. Hammel, M. Rey, Y. Yu, R. S. Mani, S. Classen, M. Liu, M. E. Pique, S. Fang, B. L. Mahaney, M. Weinfeld, D. C. Schriemer, S. P. Lees-Miller and J. A. Tainer, XRCC4 Protein Interactions with XRCC4-like Factor (XLF) Create an Extended Grooved Scaffold for DNA Ligation and Double Strand Break Repair, J. Biol. Chem., 2011, 286, 32638–32650 CrossRef CAS.
- V. A. Roberts, M. E. Pique, S. Hsu, S. Li, G. Slupphaug, R. P. Rambo, J. W. Jamison, T. Liu, J. H. Lee, J. A. Tainer, L. F. Ten Eyck and V. L. Woods, Combining H/D exchange mass spectroscopy and computational docking reveals extended DNA-binding surface on uracil–DNA glycosylase, Nucleic Acids Res., 2012, 40, 6070–6081 CrossRef CAS.
- L. Fan and V. A. Roberts, Complex of linker histone H5 with the nucleosome and its implications for chromatin packing, Proc. Natl. Acad. Sci. U. S. A., 2006, 103, 8384–8389 CrossRef CAS.
- A. A. Adesokan, V. A. Roberts, K. W. Lee, R. D. Lins and J. M. Briggs, Prediction of HIV-1 integrase/viral DNA interactions in the catalytic domain by fast molecular docking, J. Med. Chem., 2004, 47, 821–828 CrossRef CAS.
- V. A. Roberts, C-Terminal Domain of Integrase Binds between the Two Active Sites, J. Chem. Theory Comput., 2015, 11, 4500–4511 CrossRef CAS.
- S. J. Weiner, P. A. Kollman, D. A. Case, U. C. Singh, C. Ghio, G. Alagona, S. Profeta and P. Weiner, A new force field for molecular mechanical simulation of nucleic acids and proteins, J. Am. Chem. Soc., 1984, 106, 765–784 CrossRef CAS.
- N. A. Baker, D. Sept, S. Joseph, M. J. Holst and J. A. McCammon, Electrostatics of nanosystems: application to microtubules and the ribosome, Proc. Natl. Acad. Sci. U. S. A., 2001, 98, 10037–10041 CrossRef CAS.
- M. F. Sanner, A. J. Olson and J. C. Spehner, Reduced surface: an efficient way to compute molecular surfaces, Biopolymers, 1996, 38, 305–320 CrossRef CAS.
Footnote |
† Electronic supplementary information (ESI) available. See DOI: 10.1039/d0ra10106k |
|
This journal is © The Royal Society of Chemistry 2021 |