DOI:
10.1039/D0RA09123E
(Paper)
RSC Adv., 2021,
11, 3816-3826
In silico design of peptides with binding to the receptor binding domain (RBD) of the SARS-CoV-2 and their utility in bio-sensor development for SARS-CoV-2 detection†
Received
26th October 2020
, Accepted 13th January 2021
First published on 19th January 2021
Abstract
The severe acute respiratory syndrome coronavirus 2 (SARS-CoV-2) has infected millions of people across the globe and created not only a health emergency but also a financial crisis. This virus attacks the angiotensin-converting enzyme 2 (ACE2) receptor situated on the surface of the host cell membrane. The spike protein of the virus binds to this receptor which is a critical step in infection. A molecule which can specifically stop this binding could be a potential therapeutic agent. In this study, we have tested 12 potential peptides which can bind to the receptor binding domain (RBD) of the spike protein of the virus and thus can potentially inhibit the binding of the latter on ACE2 receptors. These peptides are screened based on their binding with the RBD of the spike protein and aqueous stability, obtained using several atomistic molecular dynamic simulations. The potential of mean force calculation of peptides confirmed their binding to the RBD of the spike protein. Furthermore, two potential peptides were tested for use in a biosensing application for SARS-CoV-2 detection. Two types of biosensing platforms, a graphene sheet and a carbon nano tube (CNT) were tested. The peptides were modified in order to functionalize the graphene and CNT. Based on the interaction between the substrate, peptide and spike protein, the utility of the screened peptide for a given bio sensing platform is discussed and recommended.
1 Introduction
The severe acute respiratory syndrome coronavirus 2 (SARS-Cov-2), also known as novel coronavirus 2019 (2019-nCov), outbreak was declared a global pandemic by the World Health Organization on March 11, 2020.1 The genome sequences of 2019-nCov are almost identical to those of the severe acute respiratory syndrome (SARS)2 virus of 2003 and share ∼79 percent of sequence identity.3 At the whole genome level, 2019-nCov was found to be 96 percent identical to bat coronavirus and pairwise protein sequence analysis confirmed that this virus belongs to the SARS-CoV family.3,4 Recent experimental studies have confirmed that both SARS-CoV5,6 and SARS-CoV-2 (ref. 7–9) specifically bind to the human angiotensin-converting enzyme 2 (ACE2) receptor of the host cell. However, SARS-Cov-2 is more contagious as compared to SARS-CoV and spreads faster.10,11
SARS-CoV-2 is an enveloped spherical shape virus having a diameter of 50–100 nm. The viral RNA is protected inside a lipid membrane envelope derived from the host cell.12,13 The virus has four main structural proteins namely, spike (S), membrane (M), envelope (E), and nucleocapsid (N) proteins. These proteins facilitate several critical function such as binding of the virus to the host cell receptors, fusion of virus envelop to host cell, fusion of viral RNA inside the host cell and replication of the RNA inside the host cell.14 The SARS-CoV-2 uses the human ACE2 receptor for binding on the host cell and the serine protease TMPRSS2 (provided by the host cell) for priming the S-protein which is critical for fusion of virus to the host cell membrane.9
The S-protein plays a dual role, for being responsible for virus attachment to the host cell and for the fusion of the viral RNA inside the host cell membrane.15 The S-protein has two subdomains S1 and S2. Fragment of S2, called receptor binding domain (RBD), undergoes conformational change in order to bind to the ACE2.15–17 Once bounded, S1 domain further helps in the fusion of the membrane envelope of the virus to the host cell membrane.16,17 The molecular structure of the binding domain, obtained at angstrom resolution, has been reported recently.15,17 The knowledge of the binding domain structure can be leveraged to design functional molecules/sequences that can selectively bind to the RBD. Several strategies are being explored to target (or block) any of these structural proteins to stop any of the processes such as binding of virus to host cell, fusion of virus envelope with host cell, fusion of viral RNA inside host cell and replication of viral RNA inside the infected host cell.18 In this study, we have targeted the blocking of RBD from binding to ACE2 using peptides as potential inhibitors.
Recently, Wu et al.14 used 3CLpro, S-protein, RdRP and PLpro as targets and used molecular docking technique to screen several drug databases. The hesperidin was shown to fit into the shallow pocket on the surface of RBD of SARS-CoV-2. Han et al.19 have reported few peptides designed using crystal structure of ACE2 and RBD of SARS-CoV-2 (PDB ID: 6M17).16 However, reported peptides were not tested in aqueous conditions to check their stability. It has been reported recently that crystal structure studied by Han et al.,19 had more steric clashes as compared to the latter on structure obtained by X-ray technique (PDB ID: 6M0J17). Huang et al.20 designed several peptides taking 6M0J as a template using EvoEF2 (ref. 21) and EvoDesign22 method. The peptides were designed by inducing mutation in a linear scaffold (31-mer) created by linking two fragments grafted from human ACE2 receptor (amino acid sequence 22–44 and 351–357) with a linker glycine.20 However, no detailed molecular simulation study or experimental study was done to confirm their role as a RBD–ACE2 binding inhibitors. These peptides were designed in vacuum and no solvation effect was captured to check whether these peptides will hold their secondary structure stable in the accompanying solvent.
The peptides suggested by Huang et al. looked promising based on energetics. However, wet lab experiments yet to be done to confirm their potential. To speed up the process, we tested ten most promising candidates suggested by them using atomistic simulations. The binding ability of these peptides is compared with the two natives like peptides derived from structure of RBD–ACE2 complex (PDB ID: 6M0J). At first, peptides were shortlisted based on their binding to the RBD domain of the S-protein. The binding was determined based on interaction energy, hydrogen bonding capacity and root mean square displacement calculations. Later, the stability of these peptides was checked in aqueous solution. Based on their binding stability both with RBD and in aqueous solution, among these twelve, eight most promising candidates were shortlisted. Further, the binding between these eight peptides and RBD is studied in detail by carrying out potential of mean force calculations using umbrella sampling simulations. Among these eight, two most promising peptides were identified.
These top two peptides were tested for their use in a biosensor for SARS-CoV-2 detection. In this study, two types of biosensing platforms – a graphene sheet and a CNT, were tested for their effectiveness as a sensor-support structure. We found that, two peptides could be potential molecules for SARS-CoV-2 detection. The complex interaction between peptides, graphene or CNT and spike proteins were captured using several MD simulations and discussed in further sections.
2 Protocol: peptide design, screening and possible use for detection
A detailed protocol for peptide design and its possible use for SARS-CoV-2 detection is shown in the Fig. 1. The peptides were screened and selected based on their binding with the receptor binding domain (RBD) and stability in aqueous environment. The promising peptides were further tested for sensing application. The graphene and CNT were functionalized with the peptides using 1-pyrenebutanoic acid succinimidyl ester (PBSE) linker. The stable functionalized graphene and CNT systems were further simulated in presence of RBD of spike protein. Based on the binding of RBD of spike on peptides bounded to either graphene or CNT were recommended for sensing application.
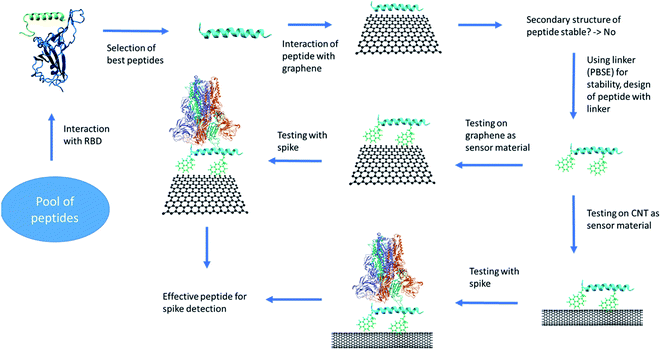 |
| Fig. 1 The protocol of the peptide design and testing for its usage as a sensor. The images are rendered using VMD software. | |
3 Results & discussion
3.1 In silico design of peptides
3.1.1 MD simulation of RBD and peptide domain (PD) of ACE2 complex (PDB ID: 6M0J). At first, we performed MD simulation of RBD–PD complex. The system was simulated in NPT ensemble for 200 ns. The molecules were modelled using CHARMM force field.23–26 The close inspection of RBD–PD complex showed that two long and one short alpha helix and one beta sheet with accompanied turn of ACE2 receptor, mainly interacted with the RBD (Fig. 2). In total, eight hydrogen bonds formed between the RBD and PD complex (Fig. S1†). The evolution of the potential energy, root mean square displacement (RMSD) of RBD and PD, and density confirms the stability of the RBD–PD complex (Fig. S1†) and confirm the equilibration of the system. Experimentally, Shang et al.27 have also shown that RBD of SARS-CoV-2 exhibits much higher affinity as compared to RBD of SARS-CoV.
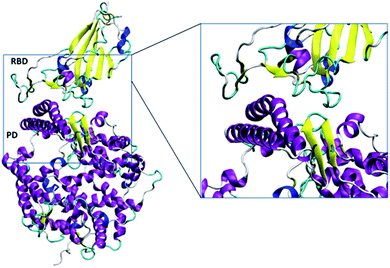 |
| Fig. 2 The simulated RBD–PD complex. Two long and one short alpha helices (purple) and beta sheet (yellow) with accompanied turn (cyan) of ACE2 mainly interacted with the RBD. The snapshots were created using VMD.28 | |
Based on above understanding, a peptide having similar or better binding as compared to PD on RBD can serve as a potential therapeutic/inhibitor candidate. Here, we have tested 12 peptides for their possible therapeutic applications (Table 1). The first 10 peptides were taken from recent study of Huang et al.20 where several peptides were suggested based on protein design scoring function. The remaining 2 peptides, peptide 11 (residue 22 to 52) and peptide 12 (residue 21 to 43) were taken from the alpha helix part of ACE2, that interacts with RBD of S-protein.
Table 1 The amino acid sequence of peptides tested in this study
Peptide name |
One letter amino acid sequence of peptides |
Peptide 1 |
EQEERIQQDKRKNEQEDKRYQRYGRGKGHQP |
Peptide 2 |
EQQQRIQEDQYRNDWEDEEYQRKGRGKGHQP |
Peptide 3 |
EQQQRIQQDQDSNDREDKEYQKRGKGKGHNH |
Peptide 4 |
QEEQKIQEDQRRNDKEHKRKQRYGRGCGKQN |
Peptide 5 |
EQEERIQRDKRKNEKEHEEKQRRGRGCGKQN |
Peptide 6 |
EQEERIQQDKRKNENEDKRYQRYGRGKGHQP |
Peptide 7 |
EQEERIQQDKRKNEWEDQYYQKYGQGKGHQP |
Peptide 8 |
EQEERIQRDQYKNDYEDEEYQRKGRGKGHQP |
Peptide 9 |
EQEQRIQQDKRSNEQEDKRYQREGKGKGHNN |
Peptide 10 |
EQEERIQQDQRKNDKEDQRYQREGKGKGHNH |
Peptide 11 |
EEQAKTFLDKFNHEAEDLFYQSSGLGKGDFR |
Peptide 12 |
IEEQAKTFLDKFNHEAEDLFYQS |
3.1.2 Binding of the peptide with RBD. The binding of each peptide with RBD was checked in the aqueous condition. These peptides were complexed with RBD and simulated for 100 ns under NPT ensemble. The snapshots of each system at the end of the simulation are shown in Fig. 3. Each of the peptide remained bound to the RBD and did not deviate much from their initial configuration. The RMSD for each peptide and RBD domain was calculated and shown in Fig. S2.† The RMSD was calculated for backbone atoms of both RBD and peptides. The initial energy minimized structure of each system was used as a reference structure. On an average, all peptides showed RMSD less than 0.6 nm. The peptide 4 and peptide 8 had higher deviation from their initial configuration as compared to other peptides. The RBD in each complex showed RMSD of less than 0.2 nm except peptide 12 system. It could be due to the small size of the peptide 12, which is not able to bind completely on to the RBD domain. The binding between the peptide and RBD is also a function of surface in contact, since the peptide 12 is a shorter peptide as compared to other peptides, the interaction between its amino acids and RBD is less. This was also reflected in the hydrogen bonding of the peptide 12, that will be discussed in the upcoming section.
 |
| Fig. 3 The snapshot of each peptide–RBD complex obtained in the end of 100 ns NPT simulation. The peptide and RBD are shown in green and blue color respectively. | |
To gain more structural insight at individual amino acid level, the secondary structure of each peptide was calculated using the DSSP program.29 The peptides were observed in various conformations namely coil, B-bridge, bend, turn, alpha helix, 5-helix and 3 helix, as can easily be seen in Fig. 4. The amino acids are marked sequentially from N-terminus (residue 1) to C-terminus (residue 31). Initial configuration of peptides had first 23 amino acids in alpha-helix form and the remaining eight amino acids (tail at C-terminus) were in the randomly oriented chain. The tail amino acid of each peptides has gone into various structural transformation (coil, bend and turn) as shown in Fig. 4 and S3.† In peptides 1, 5, 8, 9 and 12, the alpha helix residues were also observed in either bend or turn configurations.
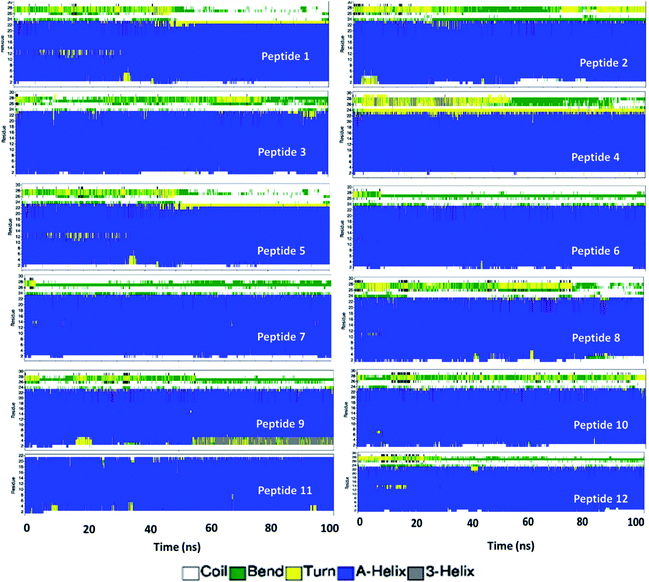 |
| Fig. 4 Evolution of secondary structure of amino acid residue in each peptide calculated using DSSP program. | |
3.1.3 Stability of the peptides in aqueous solution. The stability of the peptides in accompanying solvent environment is one of the important parameters while designing the peptides. The shape (secondary structure) of the peptide is important for its binding to the RBD. Each peptide system was simulated for 100 ns in aqueous condition. The structure of the all twelve peptides is given in the Fig. 5.
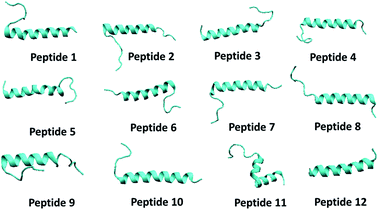 |
| Fig. 5 The snapshot of secondary structure of each peptide molecule obtained in the end of 100 ns NPT simulation. | |
On an average, the peptides were stable and conserved their helical state. The RMSD profile for each peptide is shown in Fig. S4.† Overall, the RMSD values are less than 0.8 nm for each peptide except peptide 5 which experienced several structural transitions. The RMSD values for each peptide in aqueous solution were found to be higher than that of RMSD values obtained in RBD–PD complex. The alpha helical form of all peptides remained stable during the simulation (Fig. S5†). In few cases (4, 5, 9 and 11) the amino acids on both ends (N terminus and C terminus) have gone into random coil configuration (Fig. S5 and S6†). Overall conformational changes observed in each of the peptide region are summarized in Table S1.† The peptides 1, 6 and 7 had minimal conformational changes in middle region, the region mostly remained in the alpha helical form.
3.1.4 Screening of peptides. In previous sections, we have discussed the stability of peptides when (a) they were bound to the RBD domain of the S-protein and (b) in aqueous condition. We have also shown that in both cases peptides behaved differently (Fig. S2 and S4†). In order to choose a potential candidate, several points such as stability of the peptide and their average RMSD (Fig. 7a), interaction energy (Fig. 7b), distance between RDB and peptides (Fig. 7c) and hydrogen bonding capacity (Fig. 7c) must be considered. Based on average RMSD values, peptides 1, 3, and 6 are promising candidates, however peptide 3 had lesser hydrogen bonding capacity. Based on interaction energy peptides 1, 4, and 6 are promising candidate but peptide 4 had very less hydrogen bonding capacity with RBD of S-protein and much higher average RMSD value in bound state with RBD as compared to free state. Based on average distance between the center of mass of peptide and RBD, peptides 5, 11, and 12 are most suitable peptides, however all of them had higher average RMSD values. Even peptide 12 had vary less hydrogen bonding capability. Based on hydrogen bonding capacity peptides 2, 5, 7 and 11 are most promising candidates but peptides 2, 5 and 11 have higher RMSD values. The detailed description about the key amino acids participating in the hydrogen bonding is given in the ESI† (Fig. 6 and Table 2). We found that, LYS48 and CYS48 formed strong hydrogen bonding from the peptide side. The key amino acid that participated from the RBD side are LYS417 and GLY502. The strength of the hydrogen bond is defined based on the occupancy. Thus, considering all factors, peptides except 3, 4, 11 and 12 are likely to be most promising candidates.
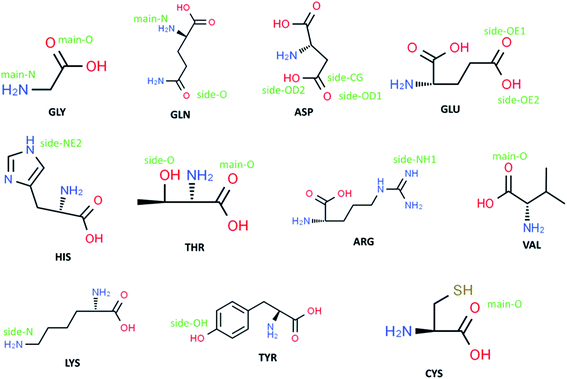 |
| Fig. 6 The key amino acids participated in the hydrogen bonding. The key atoms, that shared hydrogen bonds are shown. | |
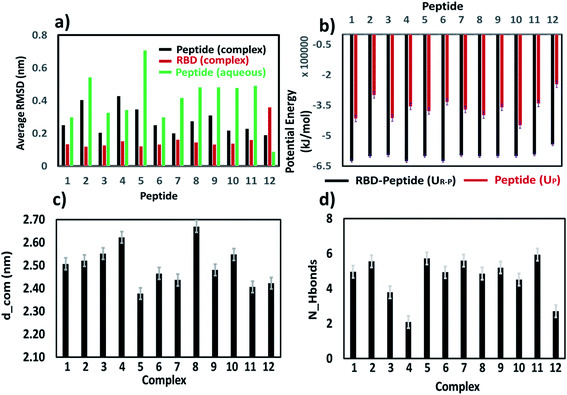 |
| Fig. 7 (a) Average RMSD for peptide and RBD in RBD–PD complex system, and for lone peptide in aqueous solution. (b) Average potential energy of each peptide–RBD (UR–P) and peptide (UP) in aqueous system. (c) Average distance (d_com) between the center of mass of the peptide and center of mass of the RBD in RBD–PD complex systems. (d) Average number of hydrogen bonds (N_H bonds) between each peptide and RBD calculated in RBD–PD complex systems. | |
Table 2 The amino acid sequence of peptide 6 and 7. The amino acids glutamic acid (E) and lysine (K) are highlighted in red where linker was attached. See Fig. S8, for detailed information about attachment
Peptide |
Amino acid sequence |
Peptide 6 with one linker (peptide 61) |
EQEERIQQDKRKNENEDKRYQRYGRGKGHQP |
Peptide 7 with one linker (peptide 71) |
EQEERIQQDKRKNEWEDQYYQKYGQGKGHQP |
Peptide 6 with two linkers (peptide 62) |
EQEERIQQDKRKNENEDKRYQRYGRGKGHQP |
Peptide 7 with two linkers (peptide 72) |
EQEERIQQDKRKNEWEDQYYQKYGQGKGHQP |
3.1.5 Potential of mean force calculation. To determine the best peptide, we did potential of mean force calculation using umbrella sampling simulations. The details of the simulations can be found in the Method and system section. The results are shown in the Fig. 8. The corresponding convergence of the PMF profiles is shown in the Fig. S7 (ESI†). The peptide 1, peptide 8 and peptide 10 have binding energy lesser then 20 kJ mol−1. The peptide 2, peptide 6, peptide 7 and peptide 9 have high binding energy.
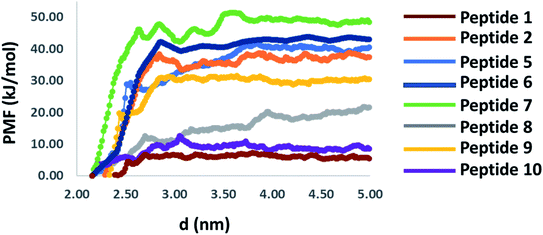 |
| Fig. 8 Potential of mean force profile of binding of each peptide to the RBD domain of the spike protein calculated by umbrella sampling simulation. | |
The peak position of the PMF profile for peptides were at the similar position. Overall, based on stability of the peptides in aqueous condition, binding with RBD domain and potential of mean force calculation, it could be concluded that each of the peptides binds to the RBD domain of the S-protein, among which 6 and 7 are could be promising candidates. Considering the native like peptides, peptide 11, taken from the ACE2 receptor binding domain, exhibits structural change in aqueous condition. The peptide 12, is stable in aqueous condition but could not bind properly to the RBD of S-protein due to its smaller size, as was clear from the hydrogen bonding. The mutated peptides (1 to 10) showed good binding with RBD of S-protein, however many of them also exhibited different structural conformations in aqueous solution. Hence, just based on any of the single parameter such as RMSD, secondary structure conformation, hydrogen bonding capacity, amino acid compositions, interaction energy, the utility of peptides cannot be judged. Hence, all these parameters along with the PMF profiles should be considered for designing the peptides.
3.2 Detection of spike protein using functionalized graphene and CNT
In this section, we would be discussing the use of screened peptides for possible detection of SARS-CoV-2 by exploring their binding affinity to the spike protein of the virus. The functionalized (by peptide using PBASE linker) graphene and CNT were explored as platform for the current study.
3.2.1 Peptide stabilization on graphene and CNT. One of the crucial and challenging part was to functionalize either the graphene or CNT without inducing significant change in the peptide secondary structure. In order to check the stability of the peptide on graphene surface, peptide 6 and peptide 7 were simulated along with graphene surface in NPT ensemble for 100 ns. The details of computational and force field parameters are provided in the method and system section. It was found that, both peptides – peptide 6 and 7, deformed considerably when interacted with the graphene surface (Fig. 9a). The secondary structure of the peptide changed significantly which mainly governs the function of the peptide or proteins in general. Similar findings related to the deformation of proteins in presence of graphene has been reported earlier.30,31 The hydrophobic surface of the graphene selectively interacts with the specific amino acids of the protein leading to the change in the secondary structure. Malaspina et al.30 recently showed that, unlike cellulose, which has hydrogen bonding capacity, the graphene surface considerably deforms the S protein of the SARS-COV-2. The study by Wei et al.31 shows that, by identifying key amino acids interacting with the graphene, proteins can be systemically redesigned for better conformational stability and desirable orientation on graphene. In our case, in order to stabilize the peptide on the graphene and CNT surface, a linker PBSE was used. The PBSE has been shown to use in several bio-sensing application earlier.32–34
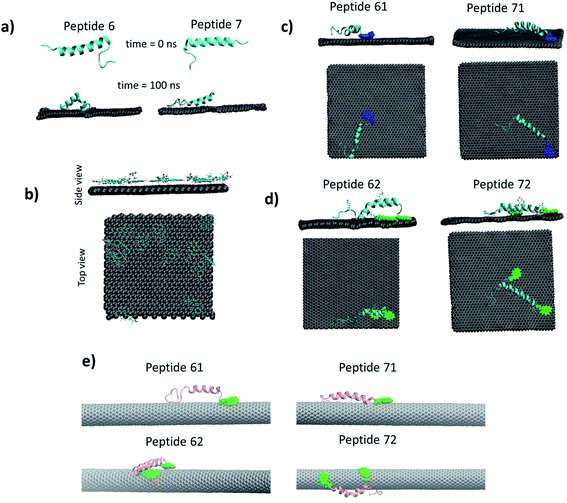 |
| Fig. 9 (a) Peptides interaction with graphene sheet. (b) Interaction of PBSE with graphene surface, they formed a monolayer on the surface. (c) Interaction of peptide 61 and 71 with the graphene sheet. (d) Interaction of peptide 62 and 72 with the graphene sheet. The PBSE is shown in blue and green color in one and two linker systems respectively. (e) Interaction of peptide 61, 71, 62 and 72 with CNT. | |
To understand the interaction of the PBSE with graphene surface, we simulated a system of fifteen PBSE molecules kept on the graphene surface under aqueous conditions. The PBSE molecules formed monolayer on the graphene surface (see Fig. 9b). They mainly interacted with four aromatic rings through π–π interactions. The interaction between aromatic rings of PBSE molecule and graphene sheet has been shown earlier and our observation of forming monolayer is line with this study.35
The peptides 6 and 7 were modified by attaching a PBSE molecule at the first amino acid (glutamic acid) of the peptides (Fig. S8†). The modified peptide 6 and peptide 7 are termed as peptide 61 and peptide 71 respectively. These peptides were tested on graphene sheet by simulating for 100 ns in NPT ensemble. It was found that both peptides lost their secondary structure significantly (see Fig. 9c). Single linker molecule was not enough for keeping the peptides structure stable, hence one more linker added to the peptide. It has been shown earlier33 that PBSE can bind with side chain of the lysine amino acid (Fig. S8†). The peptide 61 and 71 were further modified by adding PBSE molecule at the 18th and 22nd lysine molecule (K) respectively. The corresponding peptides are referred as peptide 62 and peptide 72. On simulating peptide 62 and 72 on graphene sheet, the peptide 72 was found to be more stable as compared to the peptide 62 as can be seen from Fig. 7d.
We have also explored the possibility of the CNT and carried out simulations with peptide 61, peptide 62, peptide 71 and peptide 72 with CNT (Fig. 7e). It was found that, the RMSD of the peptide was below 0.2 nm before binding to the CNT, on the binding, the RMSD increased by 3–4 times (Fig. S9, ESI†). The secondary structure of the peptide changed on binding that is reflected in the increased RMSD of the peptides. Only peptide 62 showed least deviation in the RMSD (Fig. S9†). Visual inspection of the trajectory confirmed the stability of the peptide 62 on the CNT surface.
3.2.2 Binding of spike protein in using functionalized graphene and CNT. Finally, we studied the interaction of RBD with functionalized graphene and CNT systems. The simulations of graphene functionalized with peptide 72 and CNT functionalized with peptide 62 were performed in presence of RBD of spike protein. Three different initial configurations of systems were simulated for former case. In the first system, the RBD was placed at around 4–5 nm (z direction) from the functionalized graphene surface. In the second system, the bounded state of peptide 72 and RBD was directly used on the graphene surface. In the third system, like system 1, RBD was placed on the top of graphene surface at distance of 0.7 nm. This system was generated by taking the final configuration of the system 2 and pulling the RBD away from the peptide 72 by 0.7 nm. The snapshot of final configurations after 100 ns simulation run for each system is shown in the Fig. 10a. From the analysis of the trajectory, we found that, for system 1, RBD directly interacted with the graphene surface and lost its secondary structure significantly (Fig. S10†). The peptide 72's movement on the surface of the graphene found to be quicker as compared to the RBD. Hence, perfect binding between the RBD and peptide 72 found to be difficult to occur. Both, peptide 72 and RBD interacted, but for very short period. We found that such interaction is difficult to capture through few hundred nano second simulation at atomistic scale, considering the large degree of freedom for the movement. For the system 2, we found that the peptide 72-RBD complex is intact and moves laterally on the surface of the graphene. It showed significant binding with the graphene. It confirms that, whenever such a complex form by perfect binding of the RBD on the peptide 72, they will be stable. For the system 3, it was observed that, the RBD, which is at the distance of 0.7 nm away from the functionalized graphene surface, binds to the peptide 72. It is important to note that, the initial configuration for this system was well equilibrated by keeping the restrains on the RBD, peptide 72 and graphene. In summary, we studied three important stages of binding, one in which RBD and peptide are far apart (system 1), when they perfect bind to each other (system 2), and intermediate level, in which RBD was about to bind to the peptide 72. In all three systems, RMSD of the peptide 72 remained low (below 0.3 nm) showing the stability of the peptide 72.
 |
| Fig. 10 (a) Final configuration of the systems simulated for 100 ns. System 1: peptide 72 closely interacting with the graphene, the RBD is placed 4–5 nm away from it. System 2: peptide 72 – RBD complex interacting with graphene. System 3: peptide 72 interacting with graphene, the RBD is kept at 0.7 nm away from the peptide. An intermediate configuration. (b) Final configuration of the systems simulated for 100 ns. System 1: peptide 62 closely interacting with the graphene, the RBD is placed 4–5 nm away from it. System 2: peptide 62 – RBD complex interacting with graphene. | |
In case of CNT, we studied two systems. In first case, the RBD was kept closer to the already bounded peptide 62 to the CNT. In the second case, the RBD was kept slightly closer to the CNT functionalized with peptide 6 (Fig. 10b). The changes in the RMSD for both systems are given in Fig. S11.† We found that, again, in first system, the RBD and peptide motion was random, they interacted for short period of time, otherwise their motions was independent of each other. The peptides move quickly on the surface of the CNT, whereas RBD is comparatively, slow in movement. For the second system, the peptide–RBD complex found to be stable on the surface of the CNT. Signifying that, whenever such a complex form, it will be stable for longer time.
4 Conclusion
The SARS-CoV-2 and SARS-CoV share ∼96% similarity in their genomes sequence and both utilizes ACE2 receptor present on host cell for entering inside the cell membrane. The SARS-CoV-2 have several proteins (Mpr0, Nsp1 etc.) which regulate several critical tasks such as the fusion of virus to cell membrane, translation and transcription of viral RNA to name few. Each of these proteins are possible targets for developing effective therapeutics.
In this study, at first, we have tested 12 potential peptides which could selectively bind to the RBD of the S-protein and possibly can block the binding of the S-protein on the ACE2 receptor. At first, we have shown that each peptide (except peptide 12) can easily bind to the RBD domain of the S-protein. The extent of binding and stability was checked based on hydrogen bonding capability and RMSD calculation. Later, the stability of these peptide was checked in the aqueous solution. The native peptide 11 which had good affinity with RBD exhibited undesired conformation changes thereby making it less suitable. Another native peptide 12 was stable in the aqueous solution but showed very little affinity with RBD domains. The mutated peptides, peptide 1 to peptide 10, showed good affinity but most of them lost some of their alpha helical structure in solution. Overall, peptides 6 and 7 showed both good affinity with RBD and aqueous stability.
Further, these peptides were explored for their possible use in the detection of the COVID-19, where functionalization of nanomaterials (graphene and CNT) with peptides could increase their specificity.36 The stabilization of the peptides on both graphene and CNT surface was challenging and achieved by linking the PBSE on the peptides at designated places. For a proper stabilization, two PBSE linkers were necessary and were attached on C terminus glutamic acid and lysine amino acid. The peptide 62 and peptide 72 were found to be suitable for functionalizing the CNT and graphene respectively. Both functionalized CNT and graphene were able to bound the RBD of spike protein.
In summary, these in silico designed peptides could be further tested in in vitro and in vivo experiments for their potential therapeutic application. The native peptides, peptide 11 and peptide 12 are made up of higher amount of hydrophobic amino acid and hence, may be difficult to synthesize. On the other hand, mutated peptides with higher amount of acidic or basic amino acid residues would be comparatively easy to synthesize (Fig. S12†). The screened peptides also have potential for functionalizing the nanomaterials and possible use in detection of the spike protein of the virus. We have tested these possibilities through in silico route and needs to be confirmed with further in vitro experimentation.
5 Method and system
The crystal structure of PD–RBD complex was taken from the from the Huang paper.20 The native like peptides (peptide 11 and 12) are taken from the crystal structure of the PD–RBD complex (6M0J). All simulations were performed using GROMACS 2018 software.37–39 The peptides and RBD of S-protein were modelled using the CHARMM36 force field.40 The TIP3P model was used for the water. Each peptide and complex bounded were energy minimized in vacuum. The structures were solvated with water and ions were inserted to maintain the salt concentration at 150 mM. The systems were energy minimized and further subjected to NVT (5 ns) and NPT (5 ns) equilibration by keeping peptide and complex restrained at their position. These systems were further simulated for 100 ns in production run in NPT (T = 305 K, P = 1 bar) ensemble. In the equilibration move, the temperature and pressure were controlled using the Berendsen thermostat and barostat with a time constant of 1 ps and 5 ps respectively. The pressure was coupled isotopically with the isothermal compressibility of 4.5 × 10−5 bar−1. In the production run, temperature and pressure were controlled by Nose–Hover thermostat and Parrinello–Rahman barostat with a time constant of 2 ps and 5 ps, respectively. The simulation trajectory was saved after every 20 ps. The Particle Mesh Ewald (PME) method was used for the treatment of the long-range electrostatic interactions. All bonds of the proteins & peptides were constrained using LINCS algorithm whereas SETTLE algorithm was used for water molecule.
The umbrella sampling simulations were run for two systems. The initial configurations were generated using system simulated for 100 ns NPT production run. The peptides were slowly pulled from the RBD domain at a constant speed of 0.001 nm ps−1. Whenever the distance between the center of the mass of RBD and peptide changed by 0.1 nm, that configuration was kept for further simulations. The force constant of 1000 kJ mol−1 nm−2 was used to keep the peptide restrained at their respective position. Each system was simulated for 5 ns and last 4 ns run was used for the potential of mean force calculation. The forces were saved at every 10 steps. The potential of mean force was generated using the weighted histogram analysis method (WHAM).41
The system of peptide in water on the surface of graphene sheet (single monolayer) is simulated at 300 K and 1 bar pressure using CHARMM36 force field. The system is created using GROMACS tools and energy minimized using steepest decent algorithm. The NVT simulation is done for 5 nano seconds, where restraints were put on the graphene layer. By keeping the same restraints, system is simulated under NPT condition for 100 ns. The properties are calculated from the 1000 configurations saved during the simulation. Similar simulation approach is used for the CNT as well.
Author contributions
Both Yogesh Badhe and Rakesh Gupta have contributed equally.
Funding
This research was funded by Tata Consultancy Services (TCS), CTO organization. Grant number, 1009292.
Conflicts of interest
There are no conflicts to declare.
Acknowledgements
Authors would like to thank: Mr K Ananth Krishnan, Corporate Technology Officer, Tata Consultancy Services and Dr Gautam Shroff, Head of Research, Tata Consultancy Services for their constant encouragement and support during this project.
References
- D. Cucinotta and M. Vanelli, WHO declares COVID-19 a pandemic, Acta Bio Med. Atenei Parmensis, 2020, 91(1), 157–160 Search PubMed.
- P. Zhou, X.-L. Yang, X.-G. Wang, B. Hu, L. Zhang, W. Zhang, H.-R. Si, Y. Zhu, B. Li and C.-L. Huang, A pneumonia outbreak associated with a new coronavirus of probable bat origin, Nature, 2020, 579(7798), 270–273 CrossRef CAS.
- R. Lu, X. Zhao, J. Li, P. Niu, B. Yang, H. Wu, W. Wang, H. Song, B. Huang and N. Zhu, Genomic characterisation and epidemiology of 2019 novel coronavirus: implications for virus origins and receptor binding, Lancet, 2020, 395(10224), 565–574 CrossRef CAS.
- W. Li, Z. Shi, M. Yu, W. Ren, C. Smith, J. H. Epstein, H. Wang, G. Crameri, Z. Hu and H. Zhang, Bats are natural reservoirs of SARS-like coronaviruses, Science, 2005, 310(5748), 676–679 CrossRef CAS.
- W. Li, M. J. Moore, N. Vasilieva, J. Sui, S. K. Wong, M. A. Berne, M. Somasundaran, J. L. Sullivan, K. Luzuriaga and T. C. Greenough, Angiotensin-converting enzyme 2 is a functional receptor for the SARS coronavirus, Nature, 2003, 426(6965), 450–454 CrossRef CAS.
- S. K. Wong, W. Li, M. J. Moore, H. Choe and M. Farzan, A 193-amino acid fragment of the SARS coronavirus S protein efficiently binds angiotensin-converting enzyme 2, J. Biol. Chem., 2004, 279(5), 3197–3201 CrossRef CAS.
- Q. Wang, Y. Zhang, L. Wu, S. Niu, C. Song, Z. Zhang, G. Lu, C. Qiao, Y. Hu and K.-Y. Yuen, Structural and functional basis of SARS-CoV-2 entry by using human ACE2, Cell, 2020, 181(4), 894–904 CrossRef CAS.
- H. Zhang, J. M. Penninger, Y. Li, N. Zhong and A. S. Slutsky, Angiotensin-converting enzyme 2 (ACE2) as a SARS-CoV-2 receptor: molecular mechanisms and potential therapeutic target, Intensive Care Med., 2020, 1–5 Search PubMed.
- M. Hoffmann, H. Kleine-Weber, S. Schroeder, N. Krüger, T. Herrler, S. Erichsen, T. S. Schiergens, G. Herrler, N.-H. Wu and A. Nitsche, SARS-CoV-2 cell entry depends on ACE2 and TMPRSS2 and is blocked by a clinically proven protease inhibitor, Cell, 2020, 181(2), 271–280 CrossRef CAS.
- Z. Wu and J. M. McGoogan, Characteristics of and important lessons from the coronavirus disease 2019 (COVID-19) outbreak in China: summary of a report of 72 314 cases from the Chinese Center for Disease Control and Prevention, JAMA, 2020, 323(13), 1239–1242 CrossRef CAS.
- N. Chen, M. Zhou, X. Dong, J. Qu, F. Gong, Y. Han, Y. Qiu, J. Wang, Y. Liu and Y. Wei, Epidemiological and clinical characteristics of 99 cases of 2019 novel coronavirus pneumonia in Wuhan, China: a descriptive study, Lancet, 2020, 395(10223), 507–513 CrossRef CAS.
- F. Li, Structure, function, and evolution of coronavirus spike proteins, Annu. Rev. Virol., 2016, 3, 237–261 CrossRef CAS.
- M. Cascella, M. Rajnik, A. Cuomo, S. C. Dulebohn and R. Di Napoli, Features, evaluation and treatment coronavirus (COVID-19), in StatPearls, StatPearls Publishing, 2020 Search PubMed.
- C. Wu, Y. Liu, Y. Yang, P. Zhang, W. Zhong, Y. Wang, Q. Wang, Y. Xu, M. Li and X. Li, Analysis of therapeutic targets for SARS-CoV-2 and discovery of potential drugs by computational methods, Acta Pharm. Sin. B, 2020, 10(5), 766–788 CrossRef CAS.
- D. Wrapp, N. Wang, K. S. Corbett, J. A. Goldsmith, C.-L. Hsieh, O. Abiona, B. S. Graham and J. S. McLellan, Cryo-EM structure of the 2019-nCoV spike in the prefusion conformation, Science, 2020, 367(6483), 1260–1263 CrossRef CAS.
- R. Yan, Y. Zhang, Y. Li, L. Xia, Y. Guo and Q. Zhou, Structural basis for the recognition of SARS-CoV-2 by full-length human ACE2, Science, 2020, 367(6485), 1444–1448 CrossRef CAS.
- J. Lan, J. Ge, J. Yu, S. Shan, H. Zhou, S. Fan, Q. Zhang, X. Shi, Q. Wang and L. Zhang, Structure of the SARS-CoV-2 spike receptor-binding domain bound to the ACE2 receptor, Nature, 2020, 581(7807), 215–220 CrossRef CAS.
- G. J. Soufi, A. Hekmatnia, M. Nasrollahzadeh, N. Shafiei, M. Sajjadi, P. Iravani, S. Fallah, S. Iravani and R. S. Varma, SARS-CoV-2 (COVID-19): New Discoveries and Current Challenges, Appl. Sci., 2020, 10(10), 3641 CrossRef CAS.
- Y. Han and P. Král, Computational Design of ACE2-Based Peptide Inhibitors of SARS-CoV-2, ACS Nano, 2020, 14(4), 5143–5147 CrossRef CAS.
- X. Huang, R. Pearce and Y. Zhang, De novo design of protein peptides to block association of the SARS-CoV-2 spike protein with human ACE2, Aging, 2020, 12(12), 11263–11276 CrossRef CAS.
- X. Huang, R. Pearce and Y. Zhang, EvoEF2: accurate and fast energy function for computational protein design, Bioinformatics, 2020, 36(4), 1135–1142 CrossRef CAS.
- R. Pearce, X. Huang, D. Setiawan and Y. Zhang, EvoDesign: designing protein–protein binding interactions using evolutionary interface profiles in conjunction with an optimized physical energy function, J. Mol. Biol., 2019, 431(13), 2467–2476 CrossRef CAS.
- A. D. MacKerell, D. Bashford, M. Bellott, R. L. Dunbrack, J. D. Evanseck, M. J. Field, S. Fischer, J. Gao, H. Guo, S. Ha, D. Joseph-McCarthy, L. Kuchnir, K. Kuczera, F. T. K. Lau, C. Mattos, S. Michnick, T. Ngo, D. T. Nguyen, B. Prodhom, W. E. Reiher, B. Roux, M. Schlenkrich, J. C. Smith, R. Stote, J. Straub, M. Watanabe, J. Wiórkiewicz-Kuczera, D. Yin and M. Karplus, All-atom empirical potential for molecular modeling and dynamics studies of proteins, J. Phys. Chem. B, 1998, 102(18), 3586–3616 CrossRef CAS.
- A. D. Mackerell, M. Feig and C. L. Brooks, Extending the treatment of backbone energetics in protein force fields: limitations of gas-phase quantum mechanics in reproducing protein conformational distributions in molecular dynamics simulations, J. Comput. Chem., 2004, 25(11), 1400–1415 CrossRef CAS.
- R. B. Best, X. Zhu, J. Shim, P. E. M. Lopes, J. Mittal, M. Feig and A. D. MacKerell, Optimization of the Additive CHARMM All-Atom Protein Force Field Targeting Improved Sampling of the Backbone ϕ, ψ and Side-Chain χ1 and χ2 Dihedral Angles, J. Chem. Theory Comput., 2012, 8(9), 3257–3273 CrossRef CAS.
- J. Huang, S. Rauscher, G. Nawrocki, T. Ran, M. Feig, B. L. d. Groot, H. Grubmüller and A. D. MacKerell, CHARMM36m: An improved force field for folded and intrinsically disordered proteins, Nat. Methods, 2017, 14(1), 71–73 CrossRef CAS.
- J. Shang, G. Ye, K. Shi, Y. Wan, C. Luo, H. Aihara, Q. Geng, A. Auerbach and F. Li, Structural basis of receptor recognition by SARS-CoV-2, Nature, 2020, 1–4 Search PubMed.
- W. Humphrey, A. Dalke and K. Schulten, VMD: visual molecular dynamics, J. Mol. Graphics, 1996, 14(1), 33–38 CrossRef CAS.
- W. Kabsch and C. Sander, Dictionary of protein secondary structure: pattern recognition of hydrogen-bonded and geometrical features, Biopolymers, 1983, 22(12), 2577–2637 CrossRef CAS.
- D. C. Malaspina and J. Faraudo, Computer simulations of the interaction between SARS-CoV-2 spike glycoprotein and different surfaces, Biointerphases, 2020, 15(5), 51008 CrossRef CAS.
- S. Wei, X. Zou, J. Tian, H. Huang, W. Guo and Z. Chen, Control of Protein Conformation and Orientation on Graphene, J. Am. Chem. Soc., 2019, 141(51), 20335–20343 CrossRef CAS.
- D. K. H. Tsang, T. J. Lieberthal, C. Watts, I. E. Dunlop, S. Ramadan, A. E. d. R. Hernandez and N. Klein, Chemically Functionalised Graphene FET Biosensor for the Label-free Sensing of Exosomes, Sci. Rep., 2019, 9(1), 13946 CrossRef.
- V. A. Karachevtsev, S. G. Stepanian, A. Y. Glamazda, M. V. Karachevtsev, V. V. Eremenko, O. S. Lytvyn and L. Adamowicz, Noncovalent Interaction of Single-Walled Carbon Nanotubes with 1-Pyrenebutanoic Acid Succinimide Ester and Glucose oxidase, J. Phys. Chem. C, 2011, 115(43), 21072–21082 CrossRef CAS.
- G. Seo, G. Lee, M. J. Kim, S.-H. Baek, M. Choi, K. B. Ku, C.-S. Lee, S. Jun, D. Park, H. G. Kim, S.-J. Kim, J.-O. Lee, B. T. Kim, E. C. Park and S. I. Kim, Rapid Detection of COVID-19 Causative Virus (SARS-CoV-2) in Human Nasopharyngeal Swab Specimens Using Field-Effect Transistor-Based Biosensor, ACS Nano, 2020, 14(4), 5135–5142 CrossRef CAS.
- J. Tian, P.-X. Yuan, D. Shan, S.-N. Ding, G.-Y. Zhang and X.-J. Zhang, Biosensing platform based on graphene oxide via self-assembly induced by synergic interactions, Anal. Biochem., 2014, 460, 16–21 CrossRef CAS.
- P. Moitra, M. Alafeef, K. Dighe, M. Frieman and D. Pan, Selective Naked-Eye Detection of SARS-CoV-2 Mediated by N Gene Targeted Antisense Oligonucleotide Capped Plasmonic Nanoparticles, ACS Nano, 2020, 14(6), 7617–7627 CrossRef CAS.
- B. Hess, C. Kutzner, D. Van Der Spoel and E. Lindahl, GROMACS 4: algorithms for highly efficient, load-balanced, and scalable molecular simulation, J. Chem. Theory Comput., 2008, 4(3), 435–447 CrossRef CAS.
- S. Pronk, S. Páll, R. Schulz, P. Larsson, P. Bjelkmar, R. Apostolov, M. R. Shirts, J. C. Smith, P. M. Kasson and D. van der Spoel, GROMACS 4.5: a high-throughput and highly parallel open source molecular simulation toolkit, Bioinformatics, 2013, 29(7), 845–854 CrossRef CAS.
- M. J. Abraham, T. Murtola, R. Schulz, S. Páll, J. C. Smith, B. Hess and E. Lindahl, GROMACS: High performance molecular simulations through multi-level parallelism from laptops to supercomputers, SoftwareX, 2015, 1, 19–25 CrossRef.
- J. Huang and A. D. MacKerell Jr, CHARMM36 all-atom additive protein force field: validation based on comparison to NMR data, J. Comput. Chem., 2013, 34(25), 2135–2145 CrossRef CAS.
- S. Kumar, J. M. Rosenberg, D. Bouzida, R. H. Swendsen and P. A. Kollman, The weighted histogram analysis method for free-energy calculations on biomolecules. I. The method, J. Comput. Chem., 1992, 13(8), 1011–1021 CrossRef CAS.
Footnote |
† Electronic supplementary information (ESI) available. See DOI: 10.1039/d0ra09123e |
|
This journal is © The Royal Society of Chemistry 2021 |