DOI:
10.1039/D0RA09059J
(Paper)
RSC Adv., 2021,
11, 147-159
Computational identification of potential chemoprophylactic agents according to dynamic behavior of peroxisome proliferator-activated receptor gamma†
Received
23rd October 2020
, Accepted 12th December 2020
First published on 22nd December 2020
Abstract
Peroxisome proliferator-activated receptor gamma (PPARγ) is an attractive target for chemoprevention of lung carcinoma, however its highly dynamic nature has plagued drug development for decades, with difficulties in receptor modeling for structure-based design. In this work, an integrated receptor-based virtual screening (VS) strategy was applied to identify PPARγ agonists as chemoprophylactic agents by using extensive docking and conformational sampling methods. Our results showed that the conformational plasticity of PPARγ, especially the H2 & S245 loop, H2′ & Ω loop and AF-2 surface, is markedly affected by binding of full/partial agonists. To fully take the dynamic behavior of PPARγ into account, the VS approach effectively sorts out five commercial agents with reported antineoplastic properties. Among them, ZINC03775146 (gusperimus) and ZINC14087743 (miltefosine) might be novel PPARγ agonists with the potential for chemoprophylaxis, that simultaneously take part in a flexible switch of the AF-2 surface and state change of the Ω loop. Furthermore, the dynamic structural coupling between the H2 & S245 and H2′ & Ω loops offers enticing hope for PPARγ-targeted therapeutics, by blocking kinase accessibility to PPARγ. These results might aid the development of chemopreventive drugs, and the integrated VS strategy could be conducive to drug design for highly flexible biomacromolecules.
1. Introduction
Peroxisome proliferator-activated receptor gamma (PPARγ), a ligand-activated transcription factor of the nuclear receptor (NR) superfamily, directly binds to and regulates the target genes involved in lipid and carbohydrate metabolism, cell proliferation, apoptosis, and angiogenesis. Recent preclinical study and epidemiological analysis postulate an important role for PPARγ in carcinogenesis,1,2 and PPARγ agonists thiazolidinediones (TZDs, e.g. rosiglitazone, RSG) possess antiproliferative effects and stimulate apoptosis in lung carcinoma cell lines, especially non-small-cell lung cancer (NSCLC).3,4 More interestingly, the cancer-preventing effects of several natural products are invariably associated with activation of PPARγ.5–7 Existing evidence intensively indicates the latency of PPARγ agonists in lung cancer chemoprevention, and stimulates a viable pursuit in chemoprophylactic agents.8,9
PPARγ ligand-binding domain (PPARγ-LBD), which is necessary and sufficient for the transcriptional activation, is composed of 13 α-helices (H1–H12 and H2′), and includes a Y-shaped internal ligand-binding pocket (LBP) and an activation function-2 surface (AF-2, formed by H3-5 and H12, see Fig. 1).10,11 The binding of endogenous and synthetic agonists to LBP causes conformational alterations in the AF-2 region to promote the displacement of a corepressor and the recruitment of a transcriptional coactivator, resulting in the activation and differentiation of gene expression.10 Meanwhile, the β-sheet (surrounded by H2, H3 and H2′) and Ω loop can be mediators in the cyclin-dependent kinase 5 (Cdk5)-mediated PPARγ phosphorylation at Ser245, which leads to the dysregulated expression of target genes.11 Aiming at these conformational alterations, numerous efforts have been made to explore selective full/partial agonists with distinct binding profiles.11,12 Full agonists (e.g. RSG) in general favor direct contacts with AF-2,13 while partial agonists (e.g. (2S)-2-(biphenyl-4-yloxy)-3-phenylpropanoic acid, LRG) occasionally stabilize the β-sheet/Ω loop (see Fig. 1).13,14 Unfortunately, PPARγ-LBD is a highly dynamic entity with multiple low-energy conformations and ligand-dependent conformational dynamics, especially the AF-2, β-sheet and Ω loop regions (Fig. 1).11,15,16 The highly structurally dynamic nature adds to not only the difficulties in advanced understanding of PPARγ function, but also hinders the rational discovery of PPARγ agonists.15,16
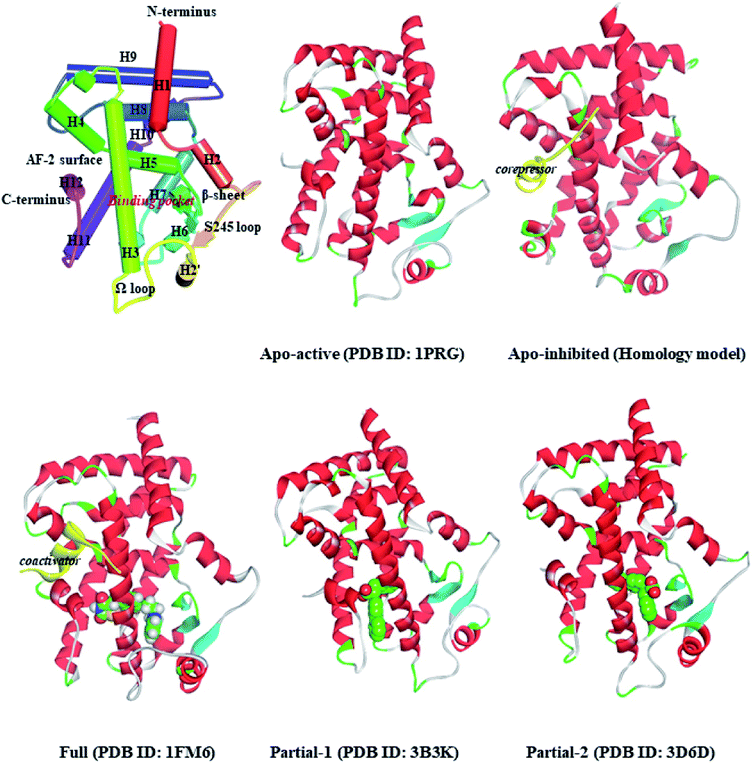 |
| Fig. 1 Ribbon diagrams of PPARγ-LBD and five structures used in the simulations: Apo-active, apo-active form of PPARγ-LBD, Apo-inhibited, apo-inhibition form of PPARγ-LBD with corepressor peptide (in yellow), Full, active form of PPARγ-LBD with full agonist rosiglitazone (RSG) and coactivator peptide (in yellow), Partial-1, active form of PPARγ-LBD with partial agonist LRGS (S-enantiomer of LRG), Partial-2, active form of PPARγ-LBD with partial agonist LRGR (R-enantiomer of LRG). | |
Nowadays, receptor-based virtual screening (RBVS) is routinely implemented in drug discovery to increase the efficiency of the development pipeline.17 It not only allows successful predictions (e.g. antivirals for influenza and COVID-19) at the leading edge of a battle against a pandemic, but also provides a better understanding about the complexity of living systems.18–22 Among the sequential stages of RBVS, the initial receptor structures have become a prerequisite for success, with a significant practical problem to determine the major physiological states or important transition states, especially for receptors with highly dynamic behavior.23–25 To address this issue, a variety of algorithms and strategies have been developed for modeling receptor dynamics and minimizing disturbance of false positives, such as Markov state models (MSMs) and ensemble docking.25–28
In our previous works,9,29 we applied an ensemble-based docking method to determine the binding profiles between ligands and PPARγ with the ideal computational costs. The outcomes aroused our interest to integrate and extend existing flexible docking and conformational sampling methods to identify novel agents based on the dynamic behavior of PPARγ. In this work, an integrated RBVS strategy (Scheme 1) was proposed to identify PPARγ agonists as cancer chemopreventive agents and their binding profiles, with the necessary flexibility to PPARγ-LBD, desirable chemical space of drug-like compound, and more cost-effective. The dynamic behavior of PPARγ-LBD (especially the AF-2, β-sheet and Ω loop regions) was firstly studied on a set of five representative structures by explicitly solvated molecular dynamics (MD) simulations, consisting of four crystal structures and a homology model (Fig. 1). Then, VS experiments were carried out against the ZINC library,30 utilizing the multiple conformations exacted from the MD simulations. Ultimately, top ten compounds were selected for further MD simulations to clarify their binding profiles and interaction mechanisms. The data presented here showed that the remarkable flexibility of AF-2, β-sheet and Ω loop regions results in correspondingly different conformations of PPARγ, and five of selected compounds have been reported to the anti-tumor effects. Our results could be of value in the development of PPARγ agonists with the chemopreventive effects, and the integrated VS strategy is expected to contribute to rational drug discovery of biomacromolecules with high dynamics.
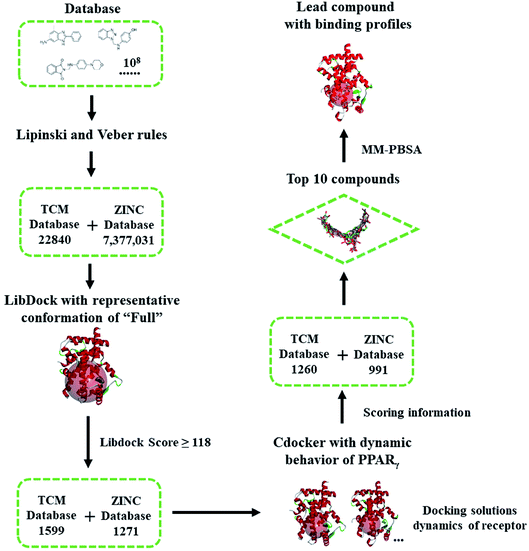 |
| Scheme 1 Flowchart of virtual screening based on the dynamic behavior of PPARγ-LBD. The compounds were firstly filtered by rules formulated by Lipinski and Veber, then evaluated (LibDock) against a representative conformation of Full, with a 15.0 Å binding sites sphere. In terms of cDocker algorithm, representative conformations of Full and Partial-1 were used with a 10.0 Å binding site sphere (conformation selection, see Fig. 2–4 and main text). | |
2. Materials and methods
2.1. Representative structure setup
The coordinates of apo-active form (Apo-active, 1PRG31), active form with full agonist rosiglitazone (RSG) and coactivator peptide (Full, 1FM6 (ref. 32)), active form with partial agonist S-enantiomer of LRG (Partial-1, 3B3K33), and active form with partial agonist R-enantiomer of LRG (Partial-2, 3D6D33) were retrieved from RCSB Protein Data Bank. The apo-inhibition form with corepressor peptide (Apo-inhibited) was modelled using the MODELER module,34,35 with the templates of PPARα-LBD (1KKQ)36 and 1PRG,31 and then evaluated by Profile-3D module35 and Procheck program.37 Note that the differences between the five structures are shown in Fig. 1 and ESI Fig. 1.† All the hetero-atoms of non-protein parts were removed, and missing hydrogen atoms were added using Discovery Studio,35 based on the expected charge distributions of amino acids at neutral pH.
The energy minimizations were performed with the Charmm27 force field,38 until converged to 0.01 kcal mol−1 Å−1. The explicit solvent MD simulations were conducted using GROMACS5.1.4 (ref. 39) and the Charmm27 force field.38 All investigated systems are summarized in Table 1, and the details of simulation setup are in agreement with our previous work.9,20,29 In brief, each system was solvated in a cubic box of SPC/E (simple-point-charge) water molecules extending at least 9.0 Å from any solute atom. Na+ counter-anions were placed to neutralize the system. To mimic physiological conditions, the NPT ensemble was applied at constant pressure (1 atm) and 300 K.40 Particle-Mesh Ewald (PME) method41 and LINCS algorithm42 were applied to handle long-range electrostatics and constrain all covalent bonds. The cutoff radii for coulomb and van der Waals interactions were set to 8.0 Å. Free dynamics were performed using a 2.0 fs time step, and coordinates were collected every 10.0 ps. The representative conformation of each system was chosen using the g_cluster tool implemented in GROMACS.43
Table 1 Summary of each simulation and corresponding MD time-scalea,b
Simulation |
System setup |
Time (ns) |
Fig. 1 shows the corresponding structures. All simulations include SPC/E water box, which extends at least 9.0 Å from any solute atom. |
Apo-active |
Apo-active PPARγ-LBD (PDB source: 1PRG chain A) |
100 |
Apo-inhibited |
Apo-inhibited PPARγ-LBD with corepressor (homology model) |
100 |
Full |
Active PPARγ-LBD with rosiglitazone and coactivator (PDB source: 1FM6) |
100 |
Partial-1 |
Active PPARγ-LBD with partial agonist LRGS (PDB source: 3B3K) |
100 |
Partial-2 |
Active PPARγ-LBD with partial agonist LRGR (PDB source: 3D6D) |
100 |
Apo-activeR |
Apo-active PPARγ-LBD (PDB source: 1PRG chain A) |
600 |
FullR (triplicate) |
Active PPARγ-LBD with rosiglitazone and coactivator (PDB source: 1FM6, repeated for three times) |
600 |
FullNP |
Active PPARγ-LBD with rosiglitazone (PDB source: 1FM6) |
600 |
2.2. Virtual screening with representative structure
The ‘Drugs-Now’ and ‘TCM Database @ Taiwan’ subsets of ZINC database (downloaded on 28 August 2018)30 were used in virtual screening, and firstly filtered by rules formulated by Lipinski (rule of five)44 and Veber.45 The selected compounds were then treated with ‘Prepare Ligands’ and ‘Minimize Ligands’ tools35 to handle the geometries and partial atomic charges, especially correct ionization and low-energy conformers (converged to 0.001 kcal mol−1 Å−1). Virtual screening was performed via two programs, LibDock46 and cDocker.47 The former is used for shape-based fast filtering, and the latter for semi-flexible fine screening. In our virtual screening protocol (see the flowchart of Scheme 1), the compounds were firstly evaluated (LibDock) against the MD-generated representative conformation of Full, with a 15.0 Å binding site sphere. The compounds with LibDock scores larger than those of full agonist RSG and partial agonist LRGS (≥118), were selected for the second filter. In terms of cDocker algorithm, MD-generated representative conformations of Full and Partial-1 were used, and the binding site was assigned with a sphere of 10.0 Å. The binding poses of best ten compounds were selected and further refined by 100.0 ns MD simulations. The details of screening parameters can be found in the ESI.†
2.3. Free energy calculation and geometric analysis
Binding free energies (ΔGbind) were evaluated by the molecular mechanics generalized Born surface area method (g_mmpbsa),48 which has been extensively used to expound the interactions between ligands and protein binding pockets.9,29 Details of parameters are similar to those performed the previous works,9,29 and in the ESI.† All values were calculated in averages over 200 snapshots evenly extracted from the 60–100 ns MD trajectories.
Apart from standard methods, secondary structures, volumes and binding pocket size were separately determined by the defined secondary structure of proteins (DSSP) method (do_dssp),49 Discovery Studio client35 and Fpocket program.50 Principal component analysis (PCA) and dynamics cross-correlation matrices (DCCM) were calculated using Bio3D package51 and GROMACS implemented tools.39 Structural plotting and visualization were accomplished by Discovery Studio client.35 Details of these analytical approaches can be found in the ESI.†
3. Results and discussion
3.1. Representative conformations and essential dynamics of PPARγ
First of all, dynamic behavior of PPARγ-LBD (especially the AF-2, β-sheet and Ω loop regions) was studied with the four crystal structures (Apo-active, Full, Partial-1 and Partial-2) and one homology model structure (Apo-inhibited). Their structural differences have been shown in Fig. 1 and ESI Fig. 1,† and system setup of each MD simulation can refer to Table 1. The equilibration reliabilities were verified by monitoring the time evolution of structural parameters,52 and each PPARγ-LBD structure (except Full) was well-behaved during the 100 ns MD simulations (ESI Fig. 2†), consistent with previous atomistic MD simulations of PPARγ-LBD with agonists.9,29 As shown in Fig. 2, the representative conformations, extracted by RMSD clustering method, represent obvious structural differences. The representative conformation of Full has the maximal volume and pocket size with the values of ∼33
937 and ∼1200 Å3, while those of Partial-1 are moderate (∼25
712 and ∼909 Å3) with the predictably different ligand-binding manner. While the volumes (pocket sizes) of Apo-active, Apo-inhibited and Partial-2 are ∼28
094 (∼993), ∼23
530 (∼832) and ∼24
895 (∼880) Å3. To further explore the structural adjustments, the root mean square fluctuations (RMSF) and principal component analysis (PCA) analyses were separately performed with the 60–100 ns MD trajectories using the GROMACS implemented tools39 and Bio3D package.51 According to the analysis results (Fig. 3 and 4), it was not difficult to observe that Full, Partial-1 and Partial-2 exhibit dramatic fluctuations of S245 loop (residues 237–253), H2′ & Ω loop (residues 250–266 and residues 263–278) and H12 (residues 465–477). In addition, the overall correlated motions of Full, Partial-1 and Partial-2 are substantially dramatic, and their structures require a relatively long period to adjust and equilibrate, in which the AF-2, β-sheet and Ω loop regions occupy the accessible conformations for the recruitment of co-regulator and agonist (Fig. 2–4), in accord with previous NMR and MD results.10,15
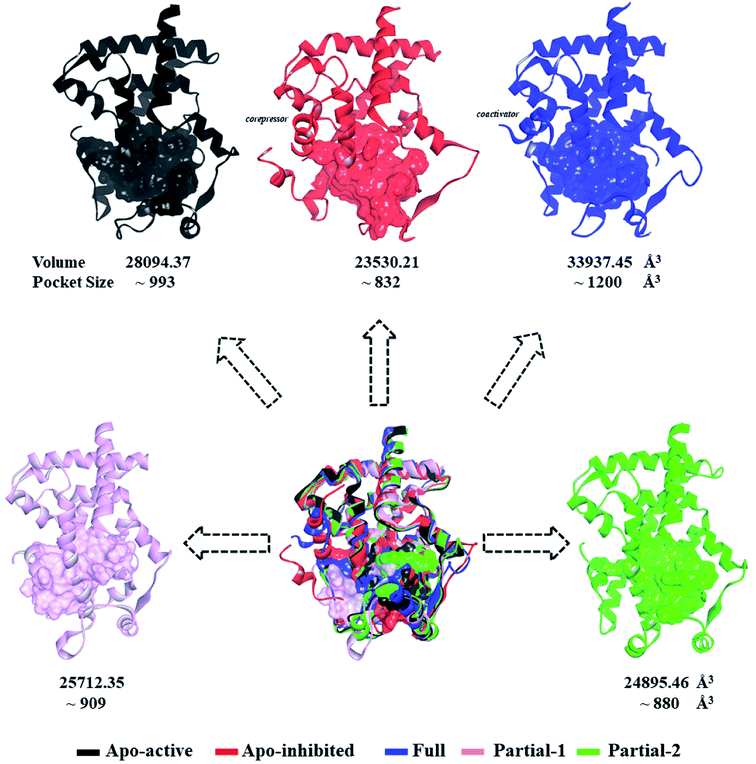 |
| Fig. 2 Superimposition of various representative PPARγ-LBD conformations which were derived from simulation trajectories by using root mean-square difference (RMSD) clustering. The volumes and pocket sizes were separately determined by Discovery Studio client35 and Fpocket program.50 | |
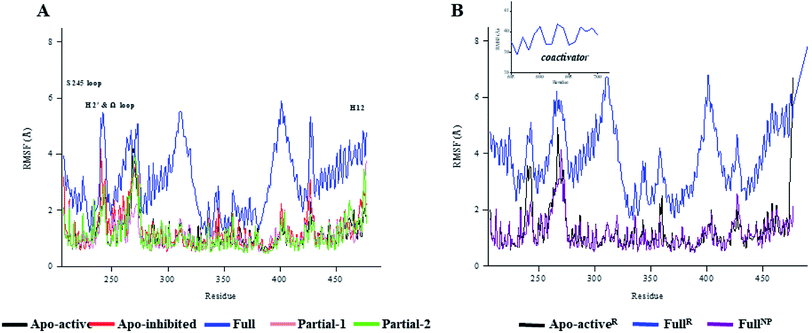 |
| Fig. 3 The root-mean-square fluctuations (RMSF) per residue of various PPARγ-LBD structures over (A) 100 ns and (B) 600 ns MD trajectories. The values of FullR were averaged based on three independent 600 ns MD trajectories. H2′ & Ω loop (residues 250–266 and residues 263–278), S245 loop (residues 237–253) and H12 (residues 465–477). | |
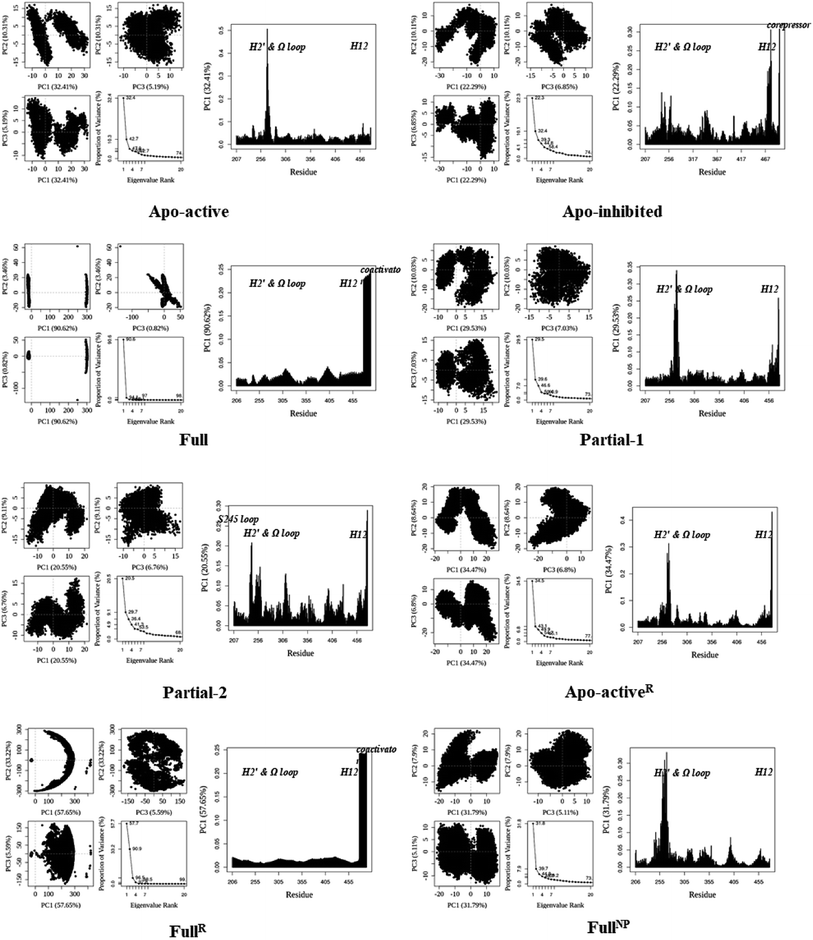 |
| Fig. 4 Analysis of various PPARγ-LBD structures from MD simulations. Projection of snapshots onto the principal planes defined by the three most significant PCs (PC1, PC2 and PC3) via Bio3D package.51 The magnitude of each eigenvalue is expressed as the percentage of the total variance (mean-square fluctuation) captured by the corresponding eigenvector. Eigenvalue rank was obtained from diagonalization of the atomic displacement correlation matrix of Cα atom coordinates. The analyses of Apo-activeR, FullR and FullNP were from 600 ns MD simulations, while others from 100 ns MD simulations (see ESI Fig. 2 and 3,† and main text). | |
The dynamic features of apo-active PPARγ-LBD (Apo-activeR), active PPARγ-LBD with RSG and coactivator (FullR), as well as active PPARγ-LBD with RSG (FullNP) were further validated throughout the extra 600 ns MD simulations (Table 1 and ESI Fig. 3†). Note that the analyses of FullR were performed over three replicate simulations to ensure the consistency of the individual simulations. The above-described motion patterns all reappeared, with more speculative locations of coactivator peptide within FullR, viewed by RMSF and PCA results (Figure 3, 4, and ESI Fig. 4†). Structural fluctuates from our atomistic MD simulations reveal that the bound full/partial agonist (e.g. RSG/LRG) could consolidate the PPARγ-LBD conformations into active state, which favors coactivator binding (activation). While the H2′ & Ω loop, S245 loop and AF-2 surface (especially H12) are much perturbed during the agonist-interaction process (Fig. 2–4), in agreement with above descriptions.10,15 Though the dynamic nature of PPARγ-LBD is restricted to favor coactivator binding with the present of RSG (FullNP vs. Apo-activeR), the binding stability of coactivator peptide with PPARγ-LBD is less than previous affirmative expectations (FullR vs. FullNP).13,53 It means that current full/partial agonists cannot fully facilitate the PPARγ activation in modulating target gene expression, and may be attributed to the insufficient consideration of PPARγ-LBD dynamic behavior in rational design.16,54 In fact, most of agonists were explored based on the steady-states of Apo-active, Full and Partial-2, with rarely considering the structural fluctuation of β-sheet and Ω loop regions (e.g. motion patterns of Full and Partial-1).55–57 Thereby the representative MD-derived conformations of Full and Partial-1 were adopted in the subsequent VS process to fully take the structural dynamics of AF-2, β-sheet and Ω loop regions, because of the dramatic motions (e.g. pocket size, structural fluctuation and ligand-binding manner, see Fig. 2–4).
3.2. Docking hits with representative conformations of PPARγ
Highly efficient and robust RBVS is a kind of art which aims at the achievement of balance between accurate optimization of ligand and receptor geometries, ligand–receptor complex representation and computational costs.58 To take distinct ligand geometries, dynamic ensembles of PPARγ-LBD and effective efficiency into account in the docking procedures, an integrated VS platform (see Scheme 1) was applied to accurately predict the binding poses of candidates. The Lipinski (rule of five)44 and Veber45 rules quickly narrow the candidates (∼108) down to 22
840 (TCM) and 7
377
031 (ZINC) compounds, with reasonable drug-like physicochemical properties. Site feature docking (LibDock46) ulteriorly picks out 1599 (TCM) and 1271 (ZINC) compounds to form favorable binding interactions, using the protein site feature of MD-generated conformation of Full which has the maximal internal pocket size. Note that this motion not only ensures the chemical space of filtered compounds, but also successfully sorts out positive controls RSG and LRGS, with the LibDock score of ∼118. In terms of cDocker algorithm,47 MD-generated conformations of Full and Partial-1 present the representative dynamic features, and the predicted binding poses of RSG and LRGS with Full and Partial-1 are in a manner consistent with the crystallographic poses (1FM6 and 3B3K), with the cDocker interaction energies (Eint) being −61.29 and −66.62 kcal mol−1, respectively (ESI Table 1†). As is evident, 1260 (TCM) and 991 (ZINC) compounds selectively bind with Full and Partial-1 (Scheme 1), and the binding patterns of evaluated top ten compounds seem separately to be comparable to those of RSG and LRGS (ESI Fig. 5†). Among the top ten compounds, compound ZINC15120682 and ZINC85568445 are the natural products (TCM), and other eight compounds are commercial agents, with one of them (ZINC12381030) being the proved PPAR agonist (ESI Scheme 1†).30 More important, several experimental studies have shown the five commercial agents ZINC03775146 (gusperimus),59 ZINC03831462 (sofalcone),60 ZINC14087743 (miltefosine),61 ZINC17719775 (ascorbyl palmitate)62 and ZINC58581064 (dolutegravir)63 to have the miscellaneous antineoplastic activities, especially hindering the signal transduction of cellular proliferation. The binding properties will be further discussed in the following section.
3.3. Binding features of compounds predicted by MM–PBSA
As shown in ESI Fig. 6 and 7,† each docked complex reached the fundamental convergence after ∼40 ns, indicated by the time evolutions of backbone-atom and ligand positional RMSDs, and interaction energy profiles (short-range energy components).64,65 It reveals that a 100 ns simulation is sufficient for the structural relax, and relatively stable MD trajectories (60–100 ns) are subsequently selected for further analyses of energy and geometry.9,29 In accord with our simulations, ZINC03775146 (gusperimus), ZINC03831462 (sofalcone), ZINC14087743 (miltefosine) and ZINC17719775 (ascorbyl palmitate) are preferred agonists, with the average binding free energies (average between values of Full and Partial-1, ΔGbind) being −109.04, −8.12, −22.94 and −34.91 kcal mol−1, respectively (Table 2). Note that the ΔGbind values of RSG and LRGS with PPARγ-LBD are −40.04 and −43.23 kcal mol−1, respectively. van der Waals components (ΔEvdw + ΔGsur) primarily drive the binding processes of the four compounds, with the contributions over −48 kcal mol−1, which is consistent with previous MD simulations of PPARγ-LBD with agonists (ESI Tables 2 and 3†).9,29 In contrast to current agonist (e.g. RSG/LRG), the four compounds seem to induce more favorable coactivator binding, with the possibly preferable PPARγ activation (Fig. 5 and ESI Fig. 5–7†).
Table 2 Docking results of top ten compounds (binding free energies, ΔGbind)a
# |
ZINC no. |
Vendors |
Catalog number |
Full |
Partial-1 |
Average |
Energy units in kcal mol−1, obtained from the equilibrium structures. Rosiglitazone, control for full agonist. S-(2S)-2-(Biphenyl-4-yloxy)-3-phenylpropanoic acid, control for partial agonist. |
1 |
ZINC03775146 |
AK Scientific |
K529 |
−124.17 |
−93.90 |
−109.04 |
2 |
ZINC03831462 |
3B Scientific Corporation |
3B2-0795 |
−4.16 |
−12.09 |
−8.12 |
3 |
ZINC15120682 |
— |
— |
−0.92 |
15.31 |
7.20 |
4 |
ZINC12381030 |
Vitas-M |
STK170412 |
14.60 |
20.74 |
17.67 |
5 |
ZINC14087743 |
BioSynth |
M-7200 |
−24.58 |
−21.29 |
−22.94 |
6 |
ZINC03874917 |
3B Scientific Corporation |
3B3-013268 |
18.02 |
29.13 |
23.58 |
7 |
ZINC17719775 |
Acros Organics |
44 948 |
−29.52 |
−40.29 |
−34.91 |
8 |
ZINC03874915 |
3B Scientific Corporation |
3B3-013268 |
35.25 |
36.13 |
35.69 |
9 |
ZINC58581064 |
AK Scientific |
X7595 |
6.69 |
9.12 |
7.91 |
10 |
ZINC85569445 |
— |
— |
24.85 |
29.74 |
27.30 |
RSGb |
Vitas-M |
STL350047 |
−40.04 |
— |
−40.04 |
LRGSc |
— |
— |
— |
−43.23 |
−43.23 |
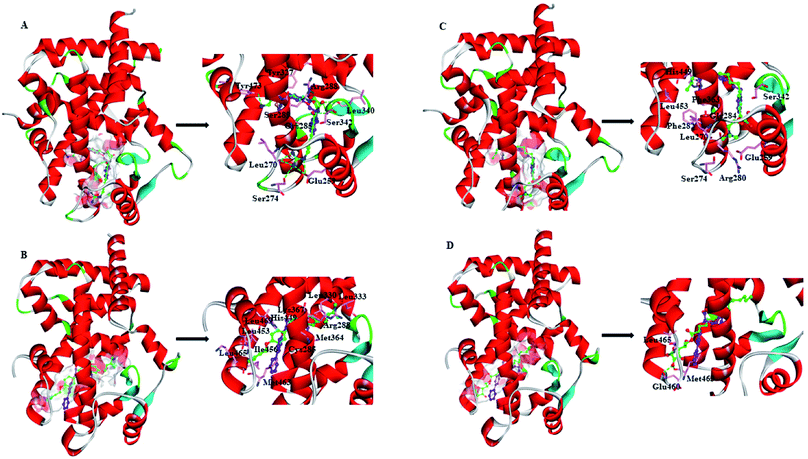 |
| Fig. 5 Views of the preferred binding modes of selected compounds with the active-site residues of PPARγ-LBD: (A) ZINC03775146–Full, (B) ZINC03831462–Partial-1, (C) ZINC14087743–Full and (D) ZINC17719775–Partial-1. Key residues are represented by stick models, with C atoms in pink. Compounds are represented by ball and stick models. The O, N, C, S atoms are colored in red, blue, green and dark yellow, respectively. The important H-bonds (or electrostatic interactions) are labeled in the dashed black lines. For comparison, cocrystallized orientation of rosiglitazone (RSG) and S-(2S)-2-(biphenyl-4-yloxy)-3-phenylpropanoic acid (LRGS) within Full and Partial-1 are shown in ball and stick models, with C atoms in purple. | |
ZINC03775146 (gusperimus) is a conventional immunosuppressor drug with the antitumor activities.59 It can enter and fill the ligand-binding pocket (LBP) of PPARγ-LBD (Full), with the binding pose resembling that of full agonist RSG (Fig. 5A). Guanidino group of ZINC03775146 has electrostatic (H-bonding) interactions with residue Glu259 of H2′, residues Leu270 and Ser274 of Ω loop. N-(Hydroxymethyl)acetamide group of ZINC03775146 occupies the portion of the large two-lobe entry of the pocket,13,66 where residue Arg288 of H3, residues Leu340 and Ser342 of β-sheet region are in electrostatic contacts. ZINC03775146 also has H-bonding interactions with residue Ser289 of H3 and residue Tyr473 of H12 (Fig. 5A). In addition, the alkyl portion of ZINC03775146 has hydrophobic stacking with residues Cys285 (H3) and Tyr327 (H4/5). ZINC03831462 (sofalcone)60 normally treats gastric ulcer and chronic gastritis, and upregulates the nuclear factor (erythroid-derived 2)-like 2/heme oxygenase-1 pathway. Its binding with Partial-1 is close to the case of partial agonist LRGS (Fig. 5B). ZINC03831462 demonstrates hydrophobic interactions with residues Cys285 and Arg288 of H3, residues Leu330 and Leu333 of H4/H5, residues Met364 and Lys367 of H7, residues His449, Leu453, Ile456 and Lys457 of H11, as well as residue Leu469 of H12. The result agrees with a previous proposal that PPARγ partial agonists interact with residues inside the region between H3 and β-sheet (Fig. 1), thereby promoting the stabilization of β-sheet surface.67,68 ZINC14087743 (miltefosine)61 is an oral drug used for the treatment of visceral leishmaniasis, can inhibit phosphatidylinositol-3-kinases (PI3K)/protein-serine–threonine kinase (Akt) activity in the cancer cell lines A431 and HeLa. It occupies the canonical binding pocket of PPARγ-LBD, with the binding pose resembling those of RSG and ZINC03775146 (Fig. 5C and ESI Fig. 5†). Residues Arg280, Phe282 and Gly284 of H3, residue Phe363 of H7, residues His449 and Leu453 of H11, residue Glu259 of H2′, residues Leu270 and Ser274 of Ω loop, as well as residue Ser342 of β-sheet region are in hydrophobic contacts within the ZINC14087743–Full complex. The binding of ZINC17719775 (ascorbyl palmitate, an antioxidant)62 with Partial-1 is close to the cases of LRGS and ZINC03831462 (Fig. 5D). However, only residues Glu460, Met463 and Leu465 of AF-2 (portion of H11 and H12) are in hydrophobic interactions with ZINC17719775.
3.4. Insights about structural movements of PPARγ and chemoprophylactic agents
In general, full agonists (e.g. RSG) adopt a common horseshoe conformation centered about H3 to stabilize the AF2 surface (Fig. 1), and have the extensive hydrogen bond network with residues Ser289, His323, His449 and Tyr473.13 Instead, partial agonists (e.g. LRG) bind in the part of LBP with a hydrophobic manner (e.g. residue Cys285) and stabilize the β-sheet (e.g. residue Ser342)/Ω loop.13,14 Our MD results indicated that the top four compounds are located near the canonical LBP of PPARγ-LBD, associated with the stabilization of AF-2 (e.g. residue Leu453 of H11, and residues Leu465, Leu469 and Tyr473 of H12, Fig. 5 and ESI Fig. 5†). This motion is similar as the cases of current full/partial agonists, while shows relatively few H-bonding interactions with H12 (Fig. 5).10,15 The four compounds take a middle-of-the-road approach, and supplement the hydrophobic (H-bonding) interactions with few important residues of H3 (e.g. residue Cys285 and Arg288), β-sheet (residue Ser342) and Ω loop (e.g. residues Leu270 and Ser274) regions, especially ZINC03775146 (gusperimus) and ZINC14087743 (miltefosine). For all this, the residue fluctuations of H12 remain relatively stable, however those in the ZINC03775146/ZINC14087743 bound complexes have much larger changes (Fig. 5, ESI Fig. 5 and 6†). It indicates that H12 of the four bound complexes occupies more stability (less sub-ensembles), seemed to correlate positively with agonist activity. For instance, RSG holds the H12 in narrow energy well with rare structural exchange, and has the substantial IC50 value.15,33
To further explore the influence of the four compounds on the structural movements of PPARγ-LBD, the large amplitude conformational changes and secondary structure alterations were monitored during the 100.0 ns MD simulations (Fig. 6 and 7). H2 & S245 loop and H2′ & Ω loop in ZINC03775146–Full and ZINC14087743–Full exhibit a much larger fluctuation than those in the other two bound complexes, especially residues Thr238 and Gly239 of H2, residues Ser245 and Pro246 of S245 loop, and residues Leu270 and Ser274 of Ω loop (Fig. 6 and 7). These results intensely indicate that H2 and H2′ of Full with the present of ZINC03775146 (gusperimus)/ZINC14087743 (miltefosine) turn the conformational alteration process over to the configuration adjustment of Ω loop (adjacent to H2′), to achieve an optimal fit of compound with the PPARγ-LBD (Fig. 5 and ESI Fig. 5†). This observation agrees with the previous points that some partial agonists likely induce a closed state of the Ω loop, to inhibit the PPARγ phosphorylation induced by Cdk5.57,68 The differences in dynamic cross-correlation maps (DCCM) between the four docked complexes further support the altered motions of the S245 and Ω loops upon binding of the corresponding compound (ESI Fig. 8†). So in conclusion, the binding of selective compound (at least ZINC03775146, gusperimus) induces the conformational adjustments of the PPARγ-LBD (e.g. H12 and Ω loop regions), associated with the correlated motions (shared community) between H2 & S245 loop and H2′ & Ω loop regions, that analogously viewed by the conformational dynamics of allosteric proteins.54 Consequently, the four compounds could be looked as a new class of agonist, in view of AF-2 surface's flexible switch (full agonist-like binding model) and Ω loop's state change (partial agonist-like binding model) simultaneously (Fig. 1 and 5). More importantly, the middle-of-the-road approach of ZINC03775146 (gusperimus) and ZINC14087743 (miltefosine) with PPARγ gives conceivable clues to explain the relevance of them in inhibiting and blocking the signal transduction of cellular proliferation, as well as the inhibitions of cancer cells.59,61 Thereby ZINC03775146 (gusperimus) and ZINC14087743 (miltefosine) may be preferred PPARγ agonists, and lead candidate agents for the lung cancer chemoprevention.
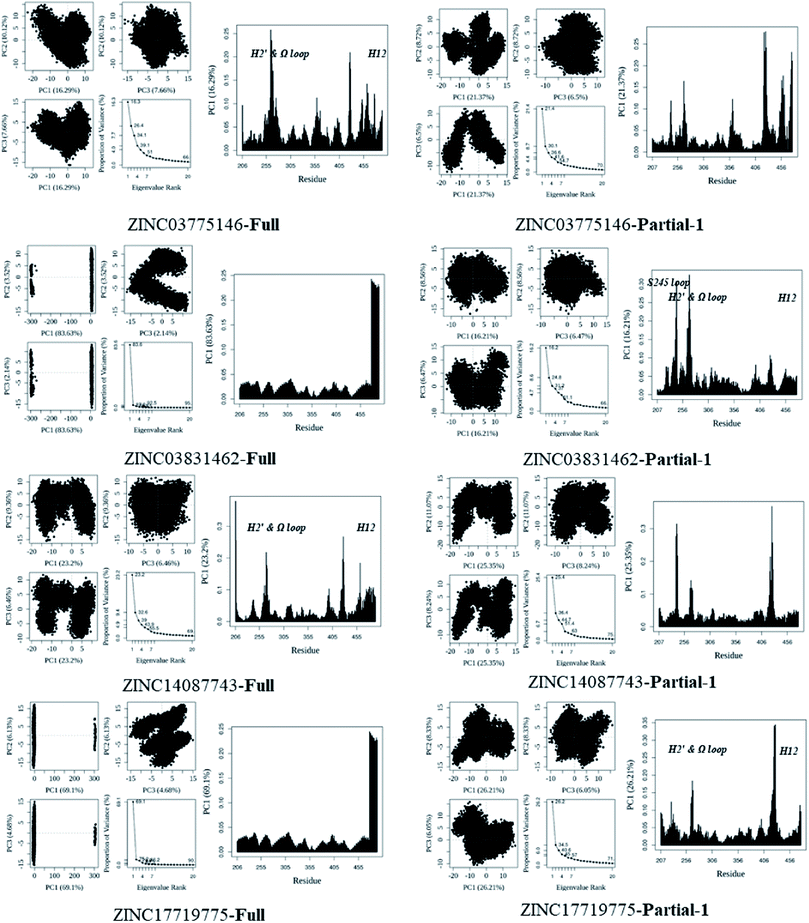 |
| Fig. 6 Analysis of various docked complexes from MD simulations. Projection of snapshots onto the principal planes defined by the three most significant PCs (PC1, PC2 and PC3) via Bio3D package.51 The magnitude of each eigenvalue is expressed as the percentage of the total variance (mean-square fluctuation) captured by the corresponding eigenvector. Eigenvalue rank was obtained from diagonalization of the atomic displacement correlation matrix of Cα atom coordinates. The analyses were from 100 ns MD simulations (see ESI Fig. 6 and 7,† and main text). | |
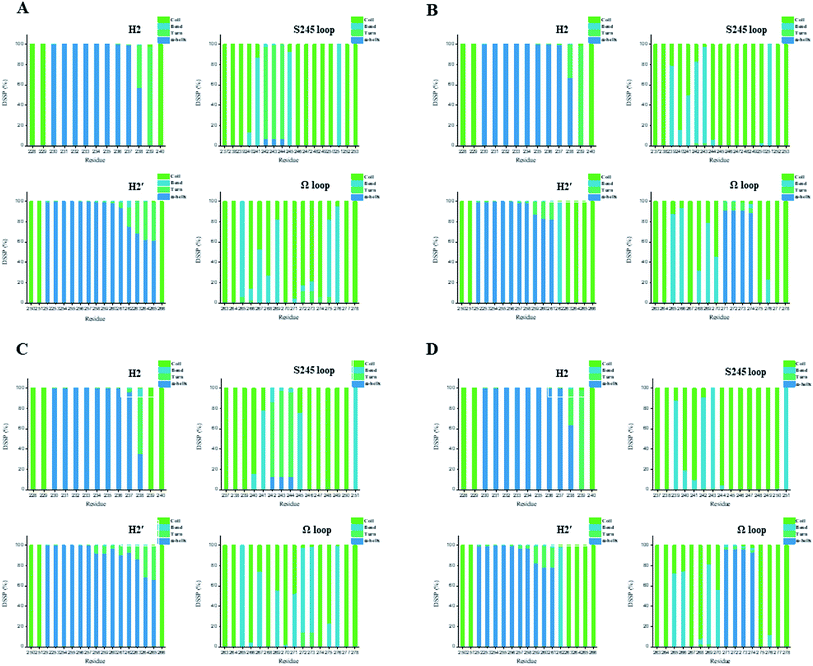 |
| Fig. 7 Secondary structures of H2 & S245 loop (resides 228–240 and residues 237–253) and H2′ & Ω loop (residues 250–266 and residues 263–278) in the (A) ZINC03775146–Full, (B) ZINC03831462–Partial-1, (C) ZINC14087743–Full and (D) ZINC17719775–Partial-1 complexes. Content rate of turn, α-helix, bend and coil conformations of the individual residues was calculated through the DSSP tool implemented in GROMACS.49 | |
In this study, the acquired binding profiles of top four compounds satisfy some general features, including proper orientation of the protein–substrate interface (AF-2 surface stabilization) and compatibility with the representative of heterodimer PPARγ-RXRα bound to a DNA stretch.10,15,31 The predicted binding poses of ZINC03775146 (gusperimus) and ZINC14087743 (miltefosine) also reproduce the adequate structural arrangement for the phosphorylation reaction at the catalytic site (S245 loop), with the necessary flexibility to the β-sheet and Ω loop regions.11 In addition to the conventional structural adjustments (e.g. AF-2), the collaborative structural alterations of H2 & S245 loop and H2′ & Ω loop regions should play an important role in the interaction of Cdk5 with the nuclear receptor, and then affect the phosphorylation of residue Ser245.68 It means that a structural coupling between H2 & S245 loop and H2′ & Ω loop regions controls PPARγ/Cdk5 axis, and PPARγ ligands break the collaborative structural alterations may also hold promise for the drug design, not just on the stabilizations of β-sheets and Ω-loop regions.11 It is consistent with recent NMR and hydrogen–deuterium exchange (HDX) results that the salt-bridge disruption involving Ω loop and H3 switch are expected to destabilize the AF2 surface.16 From there, the hydrophobic groups (e.g., biphenyl group) might contribute a lot to the ligand binding with the region and benefit a more effective regulator, on basis of the steric and hydrophobicity/hydrophilicity characteristics of the region between H3, Ω loop and β-sheet of PPARγ-LBD (Fig. 1 and 5).15,57
4. Conclusion
PPARγ plays a key role in the control of several cellular signaling and gene expression, and is an attractive target for the lung cancer chemoprevention.3,4 Unfortunately the highly structural dynamic nature of PPARγ-LBD adds to not only the difficulties in basic understanding of PPARγ function, but also hinders the rational drug design.15 To solve the problem, we presented an integrated VS platform (details in Scheme 1) to identify the PPARγ agonists and the binding profiles, with the dynamic behavior of PPARγ.
Through the analyses of ligand-binding pocket (LBP) volume and correlated motions of AF-2, β-sheet and Ω loop regions, it was found that the conformational plasticity of PPARγ is markedly affected by binding of selective agonist (e.g. RSG/LRG), and the intrinsically dynamic behavior of PPARγ should be carefully considered in the virtual screening, with multiple acceptable conformations (e.g. MD-generated conformations of Full and Partial-1). The integrated VS platform could quickly sort out favorable compounds (e.g. ZINC03775146 (gusperimus) and ZINC14087743 (miltefosine)) and determine their binding profiles, with the considerable balance between accuracies and computational costs. In addition, the intrinsically dynamic behavior of PPARγ aroused a therapeutic window for the regulation of target genes via PPARγ, using a ligand that blocks kinase accessibility to PPARγ Ser245, and offered hope for resurrecting PPARγ-targeted therapeutics to lung cancer chemoprevention.
The recent unified view of drug screening emphasizes that the appropriate selection of the initial structure will determine the success of rational drug design.69,70 In effect, a considerable proportion of receptors are discovered without the presence of endogenous substrates or synthetic ligands. The allosterically perturbed signals transmit from apo state to ligand bound state (or transition state) can affect the functional activities of receptors. As a result, the presented integrated VS strategy should be of value in the rational drug screening, especially aiming at biomacromolecules with considerable flexibilities or protein–protein association.
Conflicts of interest
The authors have declared that no competing interests exist.
Acknowledgements
The authors wish to thank Professor R. J. Cao for the useful suggestions. This research was supported by the National Natural Science Foundation of China (No. 11774279, 11774280), Fundamental Research Funds for the Central Universities (xzy032020038), China Postdoctoral Science Foundation (2017M613147), Natural Science Basic Research Plan in Shaanxi Province of China (2019JQ-603) and Shaanxi Province Postdoctoral Science Foundation (2017BSHYDZZ44).
References
- V. G. Keshamouni, R. C. Reddy, D. A. Arenberg, B. Joel, V. J. Thannickal, G. P. Kalemkerian and T. J. Standiford, Oncogene, 2004, 23, 100–108 CrossRef CAS.
- J. M. Peters, Y. M. Shah and F. J. Gonzalez, Nat. Rev. Cancer, 2012, 12, 181–195 CrossRef CAS.
- T. H. Chang and E. Szabo, Cancer Res., 2000, 60, 1129–1138 CAS.
- R. L. Keith and Y. E. Miller, Nat. Rev. Clin. Oncol., 2013, 10, 334–343 CrossRef CAS.
- H. Lapillonne, M. Konopleva, T. Tsao, D. Gold, T. McQueen, R. L. Sutherland, T. Madden and M. Andreeff, Cancer Res., 2003, 63, 5926–5939 CAS.
- Z. C. Dang, V. Audinot, S. E. Papapoulos, J. A. Boutin and C. W. Lowik, J. Biol. Chem., 2003, 278, 962–967 CrossRef CAS.
- X. Zuo, Y. Wu, J. S. Morris, J. B. Stimmel, L. M. Leesnitzer, S. M. Fischer, S. M. Lippman and I. Shureiqi, Oncogene, 2006, 25, 1225–1241 CrossRef CAS.
- S. Prost, F. Relouzat, M. Spentchian, Y. Ouzegdouh, J. Saliba, G. Massonnet, J. P. Beressi, E. Verhoeyen, V. Raggueneau, B. Maneglier, S. Castaigne, C. Chomienne, S. Chretien, P. Rousselot and P. Leboulch, Nature, 2015, 525, 380–383 CrossRef CAS.
- J. Xia, L. Yang, L. Dong, M. Niu, S. Zhang, Z. Yang, G. Wumaier, Y. Li, X. Wei, Y. Gong, N. Zhu and S. Li, Front. Pharmacol., 2018, 9, 134 CrossRef.
- T. S. Hughes, P. K. Giri, I. M. de Vera, D. P. Marciano, D. S. Kuruvilla, Y. Shin, A. L. Blayo, T. M. Kamenecka, T. P. Burris, P. R. Griffin and D. J. Kojetin, Nat. Commun., 2014, 5, 3571 CrossRef.
- A. S. Banks, F. E. McAllister, J. P. Camporez, P. J. Zushin, M. J. Jurczak, D. Laznik-Bogoslavski, G. I. Shulman, S. P. Gygi and B. M. Spiegelman, Nature, 2015, 517, 391–395 CrossRef CAS.
- P. Tontonoz and B. M. Spiegelman, Annu. Rev. Biochem., 2008, 77, 289–312 CrossRef CAS.
- A. Farce, N. Renault and P. Chavatte, Curr. Med. Chem., 2009, 16, 1768–1789 CrossRef CAS.
- A. J. Kroker and J. B. Bruning, PPAR Res., 2015, 2015, 816856 CrossRef.
- I. M. Chrisman, M. D. Nemetchek, I. M. S. de Vera, J. Shang, Z. Heidari, Y. Long, H. Reyes-Caballero, R. Galindo-Murillo, T. E. Cheatham 3rd, A. L. Blayo, Y. Shin, J. Fuhrmann, P. R. Griffin, T. M. Kamenecka, D. J. Kojetin and T. S. Hughes, Nat. Commun., 2018, 9, 1794 CrossRef.
- Z. Heidari, I. M. Chrisman, M. D. Nemetchek, S. J. Novick, A. L. Blayo, T. Patton, D. E. Mendes, P. Diaz, T. M. Kamenecka, P. R. Griffin and T. S. Hughes, Nat. Commun., 2019, 10, 5825 CrossRef CAS.
- Y. Tanrikulu and G. Schneider, Nat. Rev. Drug Discovery, 2008, 7, 667–677 CrossRef CAS.
- K. J. Kohlhoff, D. Shukla, M. Lawrenz, G. R. Bowman, D. E. Konerding, D. Belov, R. B. Altman and V. S. Pande, Nat. Chem., 2014, 6, 15–21 CrossRef CAS.
- J. Lyu, S. Wang, T. E. Balius, I. Singh, A. Levit, Y. S. Moroz, M. J. O'Meara, T. Che, E. Algaa, K. Tolmachova, A. A. Tolmachev, B. K. Shoichet, B. L. Roth and J. J. Irwin, Nature, 2019, 566, 224–229 CrossRef CAS.
- Z. Li, S. Chen, C. Gao, Z. Yang, K. C. Shih, Z. Kochovski, G. Yang, L. Gou, M. P. Nieh, M. Jiang, L. Zhang and G. Chen, J. Am. Chem. Soc., 2019, 141, 19448–19457 CrossRef CAS.
- Z.-W. Yang, Y.-Z. Zhao, Y.-J. Zang, H. Wang, X. Zhu, L.-J. Meng, X.-H. Yuan, L. Zhang and S.-L. Zhang, Chin. Phys. Lett., 2020, 37, 058701 CrossRef CAS.
- Y. Han and P. Kral, ACS Nano, 2020, 14, 5143–5147 CrossRef CAS.
- R. E. Amaro and W. W. Li, Curr. Top. Med. Chem., 2010, 10, 3–13 CrossRef CAS.
- F. Spyrakis and C. N. Cavasotto, Arch. Biochem. Biophys., 2015, 583, 105–119 CrossRef CAS.
- W. Evangelista Falcon, S. R. Ellingson, J. C. Smith and J. Baudry, J. Phys. Chem. B, 2019, 123, 5189–5195 CrossRef CAS.
- R. E. Amaro, J. Baudry, J. Chodera, O. Demir, J. A. McCammon, Y. Miao and J. C. Smith, Biophys. J., 2018, 114, 2271–2278 CrossRef CAS.
- C. F. Wong, J. Theor. Comput. Chem., 2019, 18, 1920001 CrossRef CAS.
- S. M. Linker, A. Magarkar, J. Kofinger, G. Hummer and D. Seeliger, J. Chem. Theory Comput., 2019, 15, 4974–4981 CrossRef CAS.
- Z. Yang, Y. Zhao, D. Hao, S. Ren, X. Yuan, L. Meng and S. Zhang, J. Biomol. Struct. Dyn., 2020, 38, 1918–1926 CrossRef CAS.
- J. J. Irwin, T. Sterling, M. M. Mysinger, E. S. Bolstad and R. G. Coleman, J. Chem. Inf. Model., 2012, 52, 1757–1768 CrossRef CAS.
- R. T. Nolte, G. B. Wisely, S. Westin, J. E. Cobb, M. H. Lambert, R. Kurokawa, M. G. Rosenfeld, T. M. Willson, C. K. Glass and M. V. Milburn, Nature, 1998, 395, 137–143 CrossRef CAS.
- R. T. Gampe Jr, V. G. Montana, M. H. Lambert, A. B. Miller, R. K. Bledsoe, M. V. Milburn, S. A. Kliewer, T. M. Willson and H. E. Xu, Mol. Cell, 2000, 5, 545–555 CrossRef CAS.
- R. Montanari, F. Saccoccia, E. Scotti, M. Crestani, C. Godio, F. Gilardi, F. Loiodice, G. Fracchiolla, A. Laghezza, P. Tortorella, A. Lavecchia, E. Novellino, F. Mazza, M. Aschi and G. Pochetti, J. Med. Chem., 2008, 51, 7768–7776 CrossRef CAS.
- P. Larsson, B. Wallner, E. Lindahl and A. Elofsson, Protein Sci., 2008, 17, 990–1002 CrossRef CAS.
- Accelrys, Discovery Studio 3.1, http://accelrys.com Search PubMed.
- H. E. Xu, T. B. Stanley, V. G. Montana, M. H. Lambert, B. G. Shearer, J. E. Cobb, D. D. McKee, C. M. Galardi, K. D. Plunket, R. T. Nolte, D. J. Parks, J. T. Moore, S. A. Kliewer, T. M. Willson and J. B. Stimmel, Nature, 2002, 415, 813–817 CrossRef CAS.
- R. A. Laskowski, J. A. Rullmannn, M. W. MacArthur, R. Kaptein and J. M. Thornton, J. Biomol. NMR, 1996, 8, 477–486 CrossRef CAS.
- B. R. Brooks, C. L. Brooks 3rd, A. D. Mackerell Jr, L. Nilsson, R. J. Petrella, B. Roux, Y. Won, G. Archontis, C. Bartels, S. Boresch, A. Caflisch, L. Caves, Q. Cui, A. R. Dinner, M. Feig, S. Fischer, J. Gao, M. Hodoscek, W. Im, K. Kuczera, T. Lazaridis, J. Ma, V. Ovchinnikov, E. Paci, R. W. Pastor, C. B. Post, J. Z. Pu, M. Schaefer, B. Tidor, R. M. Venable, H. L. Woodcock, X. Wu, W. Yang, D. M. York and M. Karplus, J. Comput. Chem., 2009, 30, 1545–1614 CrossRef CAS.
- M. J. Abraham, T. Murtola, R. Schulz, S. Páll, J. C. Smith, B. Hess and E. Lindahl, Softwarex, 2015, 1–2, 19–25 CrossRef.
- H. J. C. Berendsen, J. P. M. Postma, W. F. Vangunsteren, A. Dinola and J. R. Haak, J. Chem. Phys., 1984, 81, 3684–3690 CrossRef CAS.
- T. Darden, D. York and L. Pedersen, J. Chem. Phys., 1993, 98, 10089–10092 CrossRef CAS.
- B. Hess, H. Bekker, H. J. C. Berendsen and J. G. E. M. Fraaije, J. Comput. Chem., 1997, 18, 1463–1472 CrossRef CAS.
- X. Daura, K. Gademann, B. Jaun, D. Seebach, W. F. van Gunsteren and A. E. Mark, Angew. Chem., Int. Ed., 1999, 38, 236–240 CrossRef CAS.
- C. A. Lipinski, F. Lombardo, B. W. Dominy and P. J. Feeney, Adv. Drug Delivery Rev., 1997, 23, 3–25 CrossRef CAS.
- D. F. Veber, S. R. Johnson, H. Y. Cheng, B. R. Smith, K. W. Ward and K. D. Kopple, J. Med. Chem., 2002, 45, 2615–2623 CrossRef CAS.
- S. N. Rao, M. S. Head, A. Kulkarni and J. M. LaLonde, J. Chem. Inf. Model., 2007, 47, 2159–2171 CrossRef CAS.
- G. Wu, D. H. Robertson, C. L. Brooks 3rd and M. Vieth, J. Comput. Chem., 2003, 24, 1549–1562 CrossRef CAS.
- R. Kumari, R. Kumar, C. Open Source Drug Discovery and A. Lynn, J. Chem. Inf. Model., 2014, 54, 1951–1962 CrossRef CAS.
- W. Kabsch and C. Sander, Biopolymers, 1983, 22, 2577–2637 CrossRef CAS.
- P. Schmidtke, V. Le Guilloux, J. Maupetit and P. Tuffery, Nucleic Acids Res., 2010, 38, W582–W589 CrossRef CAS.
- B. J. Grant, A. P. Rodrigues, K. M. ElSawy, J. A. McCammon and L. S. Caves, Bioinformatics, 2006, 22, 2695–2696 CrossRef CAS.
- A. Grossfield and D. M. Zuckerman, Annu. Rep. Comput. Chem., 2009, 5, 23–48 CrossRef CAS.
- V. Chandra, P. Huang, Y. Hamuro, S. Raghuram, Y. Wang, T. P. Burris and F. Rastinejad, Nature, 2008, 456, 350–356 CrossRef.
- S. Lu, Q. Shen and J. Zhang, Acc. Chem. Res., 2019, 52, 492–500 CrossRef CAS.
- D. Vidovic, S. A. Busby, P. R. Griffin and S. C. Schurer, ChemMedChem, 2011, 6, 94–103 CrossRef CAS.
- N. Fresno, M. Macias-Gonzalez, A. Torres-Zaguirre, M. Romero-Cuevas, P. Sanz-Camacho, J. Elguero, F. J. Pavon, F. Rodriguez de Fonseca, P. Goya and R. Perez-Fernandez, J. Med. Chem., 2015, 58, 6639–6652 CrossRef CAS.
- A. Laghezza, L. Piemontese, C. Cerchia, R. Montanari, D. Capelli, M. Giudici, M. Crestani, P. Tortorella, F. Peiretti, G. Pochetti, A. Lavecchia and F. Loiodice, J. Med. Chem., 2018, 61, 8282–8298 CrossRef CAS.
- N. Brooijmans and I. D. Kuntz, Annu. Rev. Biophys. Biomol. Struct., 2003, 32, 335–373 CrossRef CAS.
- H. Wiendl and R. Hohlfeld, BioDrugs, 2002, 16, 183–200 CrossRef CAS.
- S. Shimakura, M. Kato, K. Saijo, T. Ohno, S. Matsuzaki, N. Tanaka, H. Fukutomi and C. R. Boland, Gastroenterology, 1995, 108, A537 Search PubMed.
- S. Clive and R. C. F. Leonard, Lancet, 1997, 349, 621–622 CrossRef CAS.
- Y. Zhang, D. Tong, D. Che, B. Pei, X. Xia, G. Yuan and X. Jin, Int. J. Nanomed., 2017, 12, 605–614 CrossRef CAS.
- A. Chrdle, Z. Jerhotova, M. Vacik, M. Linka and V. Chmelik, Int. J. STD AIDS, 2019, 30, 94–98 CrossRef.
- Z. Yang, F. Wu, X. Yuan, L. Zhang and S. Zhang, J. Mol. Graphics Modell., 2016, 65, 27–34 CrossRef CAS.
- Z. Yang, Y. Cao, D. Hao, X. Yuan, L. Zhang and S. Zhang, J. Biomol. Struct. Dyn., 2017, 1–14, DOI:10.1080/07391102.2017.1363661.
- R. R. Malapaka, S. Khoo, J. Zhang, J. H. Choi, X. E. Zhou, Y. Xu, Y. Gong, J. Li, E. L. Yong, M. J. Chalmers, L. Chang, J. H. Resau, P. R. Griffin, Y. E. Chen and H. E. Xu, J. Biol. Chem., 2012, 287, 183–195 CrossRef CAS.
- F. Fratev, J. Biomol. Struct. Dyn., 2017, 35, 476–485 CrossRef CAS.
- J. Y. Jang, M. Koh, H. Bae, D. R. An, H. N. Im, H. S. Kim, J. Y. Yoon, H. J. Yoon, B. W. Han, S. B. Park and S. W. Suh, Biochim. Biophys. Acta, Proteins Proteomics, 2017, 1865, 674–681 CrossRef CAS.
- C. F. Wong and J. A. McCammon, Annu. Rev. Pharmacol. Toxicol., 2003, 43, 31–45 CrossRef CAS.
- J. Garcia-Nafria and C. G. Tate, Annu. Rev. Pharmacol. Toxicol., 2020, 60, 51–71 CrossRef CAS.
Footnote |
† Electronic supplementary information (ESI) available. See DOI: 10.1039/d0ra09059j |
|
This journal is © The Royal Society of Chemistry 2021 |
Click here to see how this site uses Cookies. View our privacy policy here.