DOI:
10.1039/D0RA07035A
(Paper)
RSC Adv., 2021,
11, 11821-11843
The inhibition of Mpro, the primary protease of COVID-19, by Poria cocos and its active compounds: a network pharmacology and molecular docking study†
Received
17th August 2020
, Accepted 1st February 2021
First published on 25th March 2021
Abstract
Poria cocos is a traditional Chinese medicine (TCM) that can clear dampness, promote diuresis, and strengthen the spleen and stomach. Poria cocos has been detected in many TCM compounds that are used for COVID-19 intervention. However, the active ingredients and mechanisms associated with the effect of Poria cocos on COVID-19 remain unclear. In this paper, the active ingredients of Poria cocos, along with their potential targets related to COVID-19, were screened using TCMSP, GeneCards, and other databases, by means of network pharmacology. We then investigated the active components, potential targets, and interactions, that are associated with COVID-19 intervention. The primary protease of COVID-19, Mpro, is currently a key target in the design of potential inhibitors. Molecular docking techniques and molecular dynamics simulations demonstrated that the active components of Poria cocos could bind stably to the active site of Mpro with high levels of binding activity. Pachymic acid is based on a triterpene structure and was identified as the main component of Poria cocos; its triterpene active component has low binding energy with Mpro. The pachymic acid of Mpro activity was further characterized and the IC50 was determined to be 18.607 μmol L−1. Our results indicate that pachymic acid exhibits a certain inhibitory effect on the Mpro protease.
1 Introduction
Coronavirus disease 2019 (COVID-19), a viral pneumonia, is caused by severe acute respiratory syndrome coronavirus 2 (SARS-CoV-2) and has a complex pathological mechanism.1 It is a highly infectious virus that spread rapidly, resulting in a global pandemic. There are no specific drugs or vaccines against this virus that are available for clinical treatment. SARS-CoV-2 3CL hydrolase (Mpro) is the main protease in this novel form of Coronavirus and is critical for viral proteolytic maturation. The crystal structure of this protease (PDB ID: 6LU7) was previously determined by Rao Zihe and Yang Haitao from Shanghai University of Science and Technology. Therefore, Mpro is considered to be a key target for the development of a novel coronavirus inhibitor and may provide us with a basis for screening for active components against SARS-COV-2 virus.2 From the perspective of traditional Chinese medicine (TCM), COVID-19 belongs to the “cold and wet epidemic” category of epidemic disease. “Dampness” is a theoretical concept in traditional Chinese medicine (TCM). Dampness is also called “pathogenic dampness”. Dampness is divided into external dampness and internal dampness in TCM. External dampness can be considered as environmental dampness, which can enter the human body. Decreased immunity can then cause disease. Internal dampness refers to dysfunction of the spleen and liquid due to external dampness or other factors. Secondly, according to the understanding of dampness in Chinese medicine, the clinical manifestations of dampness include: a heavy head, swelling and pain in joints, sallow complexion, mental drowsiness, loss of appetite, vomiting, sputum in the throat and drooling, loose stool diarrhea, etc. In a previous study, Qing et al. reported that the body of the tongue in most patients with COVID-19 is relatively large, and that this is accompanied by thick, greasy, and sometimes curdy fur, thus exhibiting the characteristics of “Shi and Han3” (And the explanation of thick fur in traditional Chinese medicine is that “the fur is thickened and the color of the fur quality cannot be seen”. Greasy fur refers to the fur character with fine and dense lichen particles, which are close to the surface of the tongue and difficult to scrape off, and which are thick at the middle root of the tongue and thin at the edge of the tongue. Curdy fur is explained as “the fur character with large, loose and thick particles, shaped like bean curd residue piled on the tongue surface, which can be scraped away. Fur refers to a mosslike layer on the surface of the tongue. Fur character refers to the shape and quality of fur, including thick, moist and dry, etc.4,5). TCM may therefore play an important role in the clinical treatment of this disease, including “Qingfei Paidu Decoction” in the ancient Chinese book ‘Treatise on Febrile Fever’. This decoction can involve the optimization of multiple prescriptions and represents a TCM intervention that is liquid based. It has achieved good therapeutic effects when applied clinically. The ‘Treatise on Febrile Fever’ states t hat these formulas exert various effects, including sweating, diuretic and laxative effects, and settlement.6,7 These are the same actions as those exhibited by formula for diuresis and diffusing dampness.
Poria cocos, is associated with heart, lung, spleen, and kidney meridians, and acts as a damp-clearing drug in TCM. This medicine is the dried sclerotia of Poria cocos (Schw.) Wolf and can eliminate the moisture that accumulates inside the body and body cavity as an abnormal fluid (known as “Shi” in Chinese medicine).8 With the discovery of aquaporin (AQP), modern studies have shown that damp-clearing drugs are mainly used to regulate water metabolism in vivo and act via the transportation of AQP.9 Poria cocos is found in a number of TCM compounds that are used for COVID-19 intervention and may play an important role in the intervention of COVID-19.10–13 However, we have yet to ascertain the precise interventional effect of Poria cocos on COVID-19. Moreover, the specific molecules and molecular mechanisms involved have yet to be elucidated.
Based on the complex components of Poria cocos, it is difficult to accurately determine the effect of a single substance on COVID-19. Therefore, traditional experimental methods are associated with certain limitations when studying TCM. However, these unfortunate issues were eliminated following the discovery and application of network pharmacology as a research method over the last few years. This novel method connects a drug, target and disease, to establish a “drug-target-disease” interaction network. This method has replaced the traditional research strategy of “one drug and one target”.14–16 Therefore, in the present study, we used network pharmacology to predict the compounds and targets of Poria cocos that had potential intervention effects against COVID-19. We also screened active compounds using AQP4 and Mpro as receptor proteins for molecular docking.17 At the molecular level, we investigated how Poria cocos regulates the body from a variety of aspects and via multiple components, multiple targets, and multiple pathways. Our primary goal was to investigate whether the active ingredients of Poria cocos could inhibit Mpro, the main protease used by the COVID-19 virus. We also used molecular dynamics simulations,18 and in vitro experiments involving the inhibition of Mpro activity, to validate the results arising from network pharmacology and molecular docking. Our findings not only provide reference guidelines for further investigations of the specific pharmacological mechanism of Poria cocos but also provide scientific proof that this TCM can be used to treat COVID-19.
2 Reagents and instruments
2.1 Reagents
EDTA, tris(hydroxymethyl)methyl aminomethane THAM were purchased from Sigma Aldrich corporation, America; fluorogenic substrate (MCA-AVLQ↓SGFR-Lys(Dnp)-Lys-NH2) was purchased from Shanghai Sangon Biological Engineering Co., Ltd,Shanghai, China; luminescent screen was purchased from Guangzhou Jiate Biofiltration Co. Ltd,Guangzhou, China; the standard of pachymic acid was purchased from Chengdu Purifa Technology Development Co., Ltd, Sichuan Province, China.
2.2 Instruments
The EnVision multimode microorifice detector were obtained from Perkinelmer Instruments, INC.
3 Methods
3.1 Screening the active ingredients and targets of Poria cocos
We used “Herb name” to screen the Traditional Chinese Medicine Systems Pharmacology Database and Analysis Platform (TCMSP: http://tcmspw.com/)19 and retrieve the chemical composition of Poria cocos. According to the recommendations of the TCMSP database for screening effective compounds in Traditional Chinese medicine, we selected an oral bioavailability (OB) of ≥30% and a drug-like property (DL) of ≥0.18 as the criteria for screening the active components and targets of Poria cocos.19,20 We also used the PubChem Database (https://pubchem.ncbi.nlm.nih.gov/)21 to collect SMILES files for each active ingredient. The SMILES files were imported into the Swiss Target Prediction Database (http://www.swiss-targetprediction.ch), and the target species was set to “Homo Sapiens”. Targets identified by the TCMSP and Swiss Target Prediction databases were analyzed and repeated targets were eliminated. By doing this, we created a final list of active targets for Poria cocos. We then used the UniProtKB search function in the UniProt Database (https://www.uniprot.org/)22 to convert target protein names into standard gene names.
3.2 Screening targets related to COVID-19
To further understand the key targets and associated pathways that may permit potential intervention with COVID-19, we used “Novel Coronavirus Pneumonia”, “COVID-19”, and “SARS-COV-2”, as keywords to search for targets related to COVID-19 in the Human Gene Database (GeneCards; http://www.genecards.org/).23 In order to better explore the relationship between these targets, the disease-related targets and the active targets of Poria cocos were mapped to identify targets that represented the intersection. Cytoscape version 3.7.1 software24 was then used to construct an “active ingredient-target” network to study the potential mechanisms of COVID-19 intervention using the active ingredients and targets of Poria cocos.
3.3 Construction of a protein–protein interaction (PPI) network and annotation of target function pathways
Next, the search tool for the Retrieval of Interacting Genes/Proteins database (STRING, https://string-db.org/)25 was used to analyze the targets of the active ingredient and the intersection targets. The research species was set to “human species” to conduct protein–protein interaction analysis; results were derived in a TSV format and imported into Cytoscape version 3.7.1 software for visualization and processing. In addition, we performed gene ontology (GO) analysis and Kyoto Encyclopedia of Genes and Genomes (KEGG) pathway enrichment analysis on the targets by using the Metascape database (http://metascape.org/gp/)26 and STRING database. This allowed us to identify the role of target proteins with regards to function and signaling pathways and allowed us to explore the efficacy of Poria cocos as an interventional agent against COVID-19.
3.4 Molecular docking verification
Network pharmacology demonstrated that Poria cocos might exert an intervention effect on COVID-19. In order to further explore the molecular mechanisms of how Poria cocos might be used to treat the water infiltration and dampness associated with COVID-19 and verify whether network pharmacology could be used to screen active ingredients for their potential to act as anti-COVID-19 compounds, we screened 16 active compounds by molecular docking. These experiments focused on AQP4 (PDB ID: 3GD8) and Mpro, the main protease of COVID-19 (PDB ID: 6LU7). The active region of Mpro was determined by consulting the existing literature. Molecular docking was used to preliminarily explore whether the active compound of Poria cocos could bind to the active region of Mpro and thus inhibit the activity of this critical enzyme.2 AQP4 was selected as the object for molecular docking in these experiments because this protein exhibits relatively high permeability to water within the AQP family. Recent studies have shown that TCM cleats damp and promotes diuresis by acting on AQP4.9
First, we downloaded the three-dimensional structure of Mpro from the RCSB Protein Data Bank (PDB, https://www.rcsb.org/)27 as the receptor and stored this in a “PDB” format. Second, we used PyMOL software28 to preprocess Mpro and remove water molecules, hydrogenate the protein, and strip away the original ligand. AutoDock Tools 1.5.6 software was then used to calculate the charge of Mpro and add non-polar hydrogen. We then stored this modified version of the Mpro structure in a “PDBQT” format. The selected 16 active compounds were then used as ligands for docking with Mpro. The structure of the active compounds were first obtained from the PubChem Database and then imported into Chem3D software for structural optimization; once modified, these structures were saved in a “MOL2” format. Autodock Tools 1.5.6 Software was then used to add atomic charges to the active compound; this structure was saved in a “MOL2” format. Next, we confirmed the number of flexible bonds within the compound and saved this structure in a “PDBQT” format. The binding pocket area, where the original ligand of Mpro was located, was used as the active binding site; this allowed us to set the size and coordinates of the grid box; the output file was saved in a “GPF” format. Finally, AutoDock Vina29 was used for molecular docking experiments; docking results were displayed by PyMOL software and Protein-Ligand Interaction Profiler.30
3.5 Molecular dynamics simulation
Molecular docking is a reliable technique in many aspects but can only perform simulations by placing the receptor and ligand in a vacuum state; this creates spatial differences that are larger than the real scenario. The introduction of molecular dynamics simulations has given us the opportunity to ensure that these spatial relationships between the receptor and ligand are much more realistic. This tool also considers the influence of the solvent, protein flexibility, and conformational trends of the complex over time. In this study, GROMACS 5.1.1 software31 was used for all simulations of molecular dynamics. The optimal docking conformation identified by the previous molecular docking procedure was taken as the initial conformation to begin molecular dynamics simulations.
GMX pdb2gmx is a universal tool for generating only topology files for biomolecular fields of proteins, DNA, and RNA. GMX pdb2gmx was used to prepare the protein topology file, and the ligand topology file was obtained via the PRODRG online website. The complex was placed in a truncated dodecahedron and water molecules from the SPC model were added so that the atoms in the protein were a minimum distance of 1 Å from the edge of the water box. Antagonistic ions were then added to obtain a neutral system. Before conducting the molecular dynamics simulation, we ensured that the structure, the distance between the atoms, and the geometry, were appropriate. Under the influence of a GROMOS96 43A1 force field, the minimum conformation with a complex energy less than 10.0 kJ mol−1 was obtained by using the maximum descent method of 50
000 steps to minimize the energy. After limiting the position of the system, the system was then placed in a 100 ps NVT (isothermal constant volume) system and the speed rescale temperature controller was used to balance the temperature of the system and the 100 ps NPT (isothermal constant pressure) system. The Parrinello–Rahman algorithm was used to balance the pressure of the system. Finally, the whole system was kept at 300 K and 1 atmosphere for 10 ns dynamics simulation. After the dynamic simulation of 10 ns was completed, the g_MMPBSA tool32 in GROMACS was used to process the balanced trajectory of the complex and then the free energy of the complex was calculated using the MM-PBSA algorithm. The combined free energy of this method was then determined with the formula shown in eqn (1).33
|
ΔGBind = ΔEvdW + ΔEELE + ΔGPA + ΔGSA
| (1) |
In eqn (1), ΔEvdW represented the van der Waals force between the protein and the ligand, ΔEELE represented the electrostatic force between the protein and the ligand, ΔGPA represented the strength of the polar solvent in the system, and ΔGSA represented the non-polar solvation force of the system. In general, the smaller the binding energy of the system, the more stable the binding between the protein and the ligand.
3.6 Inhibition of SARS-COV-2 3CL hydrolytic enzyme activity by pachymic acid
Enzyme activity inhibition experiments have been reported in previous studies.34 We combined results from network pharmacology, molecular docking, and molecular dynamics simulation, and selected pachymic acid for enzymic inhibition experiments. Pachymic acid is based on a triterpene structure and was identified as the main component of Poria cocos; its triterpene active component has low binding energy with Mpro. In this study, the fluorescent substrate was MCA-AVLQ↓SGFR-Lys(Dnp)-Lys-NH2, in which ↓ represents the Mpro cleavage site and the reaction buffer (50 mmol L−1 Tris–HCl (pH 7.3), 1 mmol L−1 EDTA). The reaction buffer was used to prepare and dilute 1 mmol L−1 of pachymic acid and ergosterol peroxide and 4 μmol L−1 of Mpro mother solution. Dimethyl sulfoxide was used to prepare 400 mol L−1 of fluorescent substrate stock solution. The final concentration of 0 40 μmol L−1 pachymic acid was then added into a luminous plate and then a final concentration of 0.2 mol L−1 of Mpro was added. The samples were incubated at 30 °C for 2 min and then placed on ice for 5 min. Then, a polypeptide substrate with a final concentration of 20 mol L−1 was rapidly added and incubated at 30 °C for 5 s. The sample was immediately stimulated with an excitation light wavelength of 320 nm; this caused the emission of light at 405 nm for fluorescence detection. Three parallel tests were performed for each concentration. The inhibition of enzyme activity was defined by the formula shown in eqn (2). |
Inhibition% = 1 − Vi/V0
| (2) |
In eqn (2), Vi and V0 were the inhibited and uninhibited rates of the reaction, respectively. By linear fitting of the first 400 s in the time-fluorescence intensity curve of each concentration, we were able to ascertain the slope; this reflected the initial enzyme reaction rate for each concentration and allowed us to calculate the rate of inhibition associated with different concentrations of pachymic acid on Mpro. All experimental data were analyzed by GraphPad Prism 8 software.
4 Results
4.1 The active ingredients of Poria cocos and target prediction
The main components of Poria cocos were identified as triterpenes and polysaccharides. In total, TCMSP identified 34 chemical components in Poria cocos. Of these, there were 15 active components with an OB ≥ 30% and a DL ≥ 0.18. These 15 active components were mainly triterpenes. Pachyman (MOL ID: MOL000288) was identified as a polysaccharide component of Poria cocos, with an OB of 0.45% and a DL of 0.68. Although the OB value of pachyman was low, its DL value was high, thus indicating that pachyman should be included in the active compound range as a potential drug. The number of active ingredients of Poria cocos and their targets are shown in Fig. 1. The repeated targets in the active ingredients were combined and 273 targets were obtained.
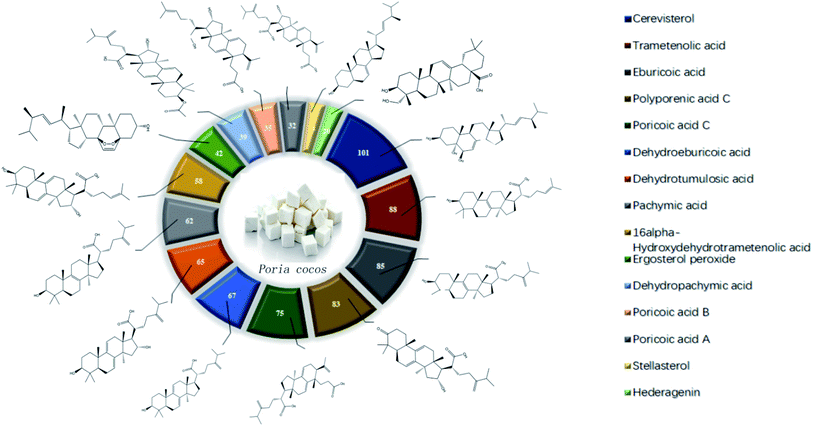 |
| Fig. 1 The active ingredients of Poria cocos and a target map. | |
4.2 The acquisition of COVID-19 related targets
Next, we searched the GeneCards database for “Novel Coronavirus Pneumonia”, “COVID-19” and “SARS-COV-2” with the species selection set to “Homo sapiens”; 660 relevant targets were identified. The targets identified for Poria cocos and COVID-19 were then uploaded to the OmicShare online platform (http://www.omicshare.com/tools/index.php/) to allow us to create a Venn diagram of drug and disease targets, as shown in Fig. 2. There were 32 intersection targets, as detailed in Table 1; these may represent potential targets for COVID-19 intervention with Poria cocos.
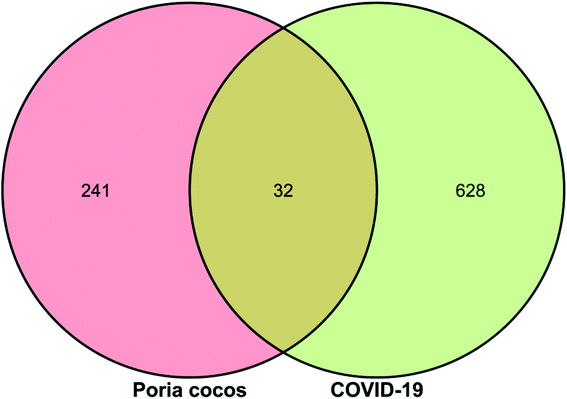 |
| Fig. 2 A Venn diagram showing targets for Poria cocos, COVID-19, and intersectional targets. | |
Table 1 Poria cocos and COVID-19 intersection target information table
Num |
Target name |
Gene name |
1 |
Prostaglandin-endoperoxide synthase 2 |
PTGS2 |
2 |
Tumor necrosis factor |
TNF |
3 |
Nitric oxide synthase 2 |
NOS2 |
4 |
Prostaglandin-endoperoxide synthase 1 |
PTGS1 |
5 |
Mitogen-activated protein kinase 3 |
MAPK3 |
6 |
Mitogen-activated protein kinase 8 |
MAPK8 |
7 |
Phosphatidylinositol-4,5-bisphosphate 3-kinase catalytic subunit alpha |
PIK3CA |
8 |
Phosphatidylinositol-4,5-bisphosphate 3-kinase catalytic subunit beta |
PIK3CB |
9 |
Phospholipase A2 group IVA |
PLA2G4A |
10 |
Peroxisome proliferator activated receptor gamma |
PPARG |
11 |
Protein kinase C alpha |
PRKCA |
12 |
Protein kinase C beta |
PRKCB |
13 |
Protein kinase C epsilon |
PRKCE |
14 |
Angiotensin-converting enzyme |
ACE |
15 |
C–C motif chemokine receptor 1 |
CCR1 |
16 |
CD81 molecule |
CD81 |
17 |
Cyclin dependent kinase 4 |
CDK4 |
18 |
Cathepsin L |
CTSL |
19 |
Epidermal growth factor receptor |
EGFR |
20 |
Coagulation factor X |
F10 |
21 |
Coagulation factor XI |
F11 |
22 |
Glucose-6-phosphate dehydrogenase |
G6PD |
23 |
Hematopoietic prostaglandin D synthase |
HPGDS |
24 |
Mitogen-activated protein kinase 14 |
MAPK14 |
25 |
NACHT, LRR and PYD domains-containing protein 3 |
NLRP3 |
26 |
Thrombin |
F2 |
27 |
EZH2/SUZ12/EED/RBBP7/RBBP4 |
EZH2 |
28 |
Inhibitor of nuclear factor kappa B kinase beta subunit |
IKBKB |
29 |
Glycogen synthase kinase-3 beta |
GSK3B |
30 |
FK506-binding protein 1A |
FKBP1A |
31 |
Inosine-5′-monophosphate dehydrogenase 2 |
IMPDH2 |
32 |
Sigma opioid receptor |
SIGMAR1 |
4.3 Construction and analysis of an “active ingredient-target” network
Cytoscape version 3.7.1 software was then used to establish a “active ingredient-target” network. Fig. 3 shows the correlation of 16 active components, 273 targets, and 32 intersection targets, of Poria cocos. In total, 287 nodes and 874 edges were included in this network. The square nodes represent active components, the triangle nodes represent intersection targets, and the circle nodes represent non-intersection targets. The larger the node shape of the active ingredient, the greater the degree of correlation between the active ingredient and the intersection target. Fig. 3 shows that the node shapes for ergosterol peroxide, eburicoic acid, cerevisterol, and trametenolic acid, were large, thus suggesting that these may be the key components of Poria cocos that could exert interventional effects on COVID-19. The “Network Analyzer” function was then used to analyze the topological parameters of the entire network. The mean number of degrees was 6.048, neighborhood connectivity (NC) was 67.757, closeness centrality (CC) was 0.331, and eccentricity was 4.156. Collectively, these results showed that multiple components in Poria cocos can act as multiple targets, thus reflecting the typical characteristics of TCM when treating disease.
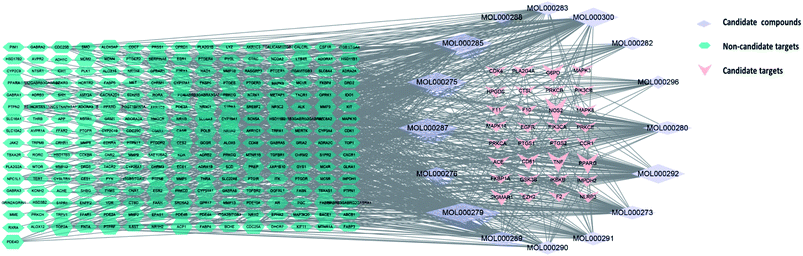 |
| Fig. 3 The active ingredient-target network for Poria cocos. | |
4.4 Construction of a core network of protein interactions
The STRING database identified 273 targets for the PPI network. Further mapping with COVID-19 disease targets yielded 32 intersection targets. Cytoscape version 3.7.1 software was then used to visualize the PPI network. After deleting individual targets, we obtained a final PPI network diagram of intersection targets, as shown in Fig. 4. This network diagram featured 30 nodes and 232 edges. The nodes represented proteins and the size and color of the nodes represent the “degree” value. The larger the shape and color of the nodes were, the larger the “degree” value and the more important the targets were. Each edge represented an interaction between proteins; the darker the line color, the larger the combined score. The mean nodal value of the target proteins was 7.73. All 15 target proteins exceeded the mean value: TNF, MAPK3, EGFR, PTGS2, MAPK8, PPAFG, MAPK14, HPGDS, PRKCA, PIK3CA, GSK3B, F3, NOS2, PLA2G4A, and PRKCB. The 15 target proteins contained in this network were of high importance, thus suggesting that these may represent key targets for the treatment of COVID-19.
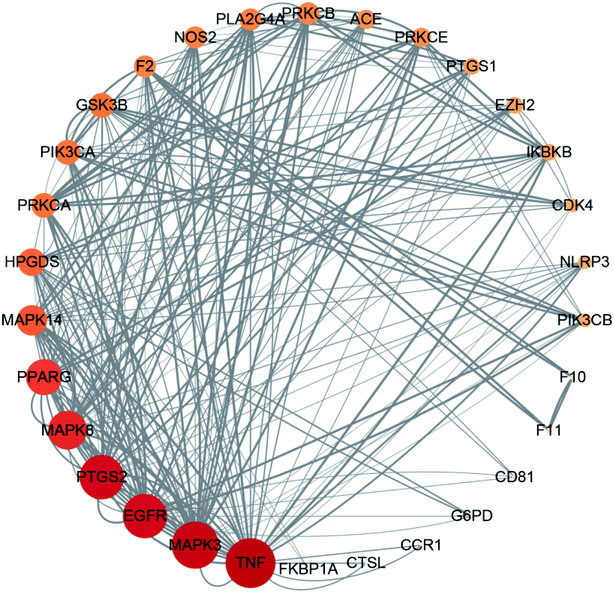 |
| Fig. 4 A network showing target interactions. | |
4.5 GO biological function annotation and KEGG pathway enrichment analysis
4.5.1 Analysis of the 273 targets. In total, 273 targets were imported into the STRING and Metascape databases for GO analysis and KEGG pathway enrichment analysis, as shown in Fig. 5. Based on the combination of KEGG results from two databases (P > 0.01), the pathways involved in the action of Poria cocos included calcium signaling pathways, the regulation of TRP channels, and hormone biosynthesis. This indicated that the beneficial effects of Poria cocos on clearing dampness and promoting diuresis may be related to ion transport, inflammation, and hormones. Poria cocos was shown to be involved in a range of biological processes, including G protein-coupled receptor signaling pathways, cellular calcium homeostasis, metal ion homeostasis, and the regulation of hormone levels. This reflected the fact that Poria cocos may regulate the G protein coupled receptor signaling pathway, the activity of various ion channels, and hormone levels, to balance fluid in vivo. The top 15 GO entries for biological processes (BPs), cellular components (CCs) and molecular functions (MFs) are shown in Fig. 5.
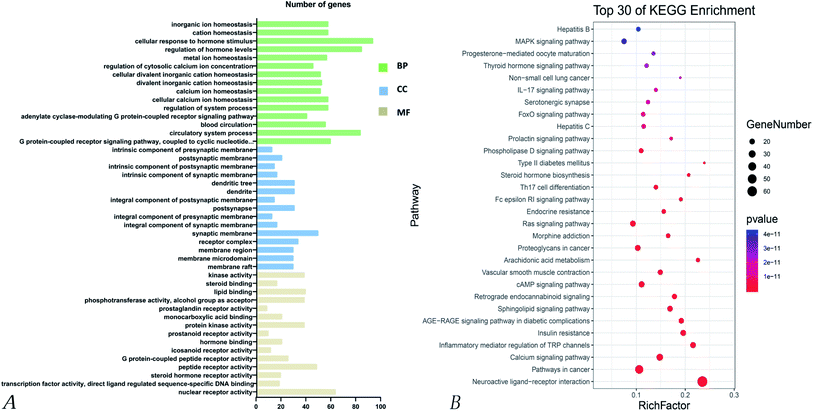 |
| Fig. 5 GO and KEGG enrichment analysis for the 273 targets of Poria cocos. (A) GO analysis for the 273 targets of Poria cocos. (B) KEGG enrichment analysis for the 273 targets of Poria cocos. | |
4.5.2 Analysis of the 32 intersection targets. GO enrichment analysis was performed on the 32 intersection targets using Metascape and STRING databases (P < 0.01). The top 10 GO entries for BP, CC, and MF, were plotted as bubble diagrams, as shown in Fig. 6. In terms of biological process, the targets for Poria cocos intervention in COVID-19 were mainly associated with response to wounding, peptidyl-serine phosphorylation, cellular response to biotic stimulus, regulation of the establishment of protein localization, and the response to lipopolysaccharide and other biological processes. In terms of cellular components, the intersection targets were involved in periodic regions of the cytoplasm, the side of the membrane, the external side of the plasma membrane, membrane rafts, membrane microdomains, membrane regions, transferase complex, and the transfer of phosphorus-containing groups. In terms of molecular function, the targets for Poria cocos intervention in COVID-19 were mainly associated with phosphotransferase activity, kinase activity, protein serine/threonine kinase activity, protein kinase activity, and drug binding. Collectively, these data suggested that the active ingredients of Poria cocos could exert TCM clinical efficacy by regulating various biological pathways, thus producing an interventional effect on COVID-19.
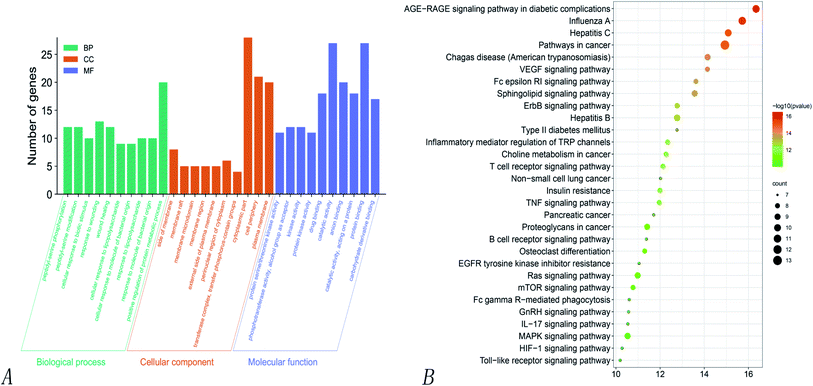 |
| Fig. 6 GO and KEGG enrichment analysis of the 24 intersection targets of Poria cocos. (A) GO analysis for the 273 targets of Poria cocos. (B) KEGG enrichment analysis for the 273 targets of Poria cocos. | |
KEGG pathway enrichment analyses of the 32 intersecting targets showed that (P > 0.01) the main signaling pathways involved the VEGF signaling pathway, the regulation of TRP channels by inflammatory mediators, non-small cell lung cancer, the toll-like receptor signaling pathway, the IL-17 signaling pathway, the MAPK signaling pathway, hepatitis C, hepatitis B, the ErbB signaling pathway, influenza A, the HIF-1 signaling pathway, the TNF signaling pathway, and the Ras signaling pathway. Of these, there were four pathways related to virus infection, namely hepatitis C, hepatitis B, influenza A, and Epstein–Barr virus infection, thus suggesting that Poria cocos can play an effective role in the disease caused by COVID-19. Fig. 6 shows the pathways where the main target was located.
4.6 Analysis of molecular docking
AutoDock Vina software was used to evaluate the ability of molecular ligands to bind to receptor proteins. The lower the combined energy, the better the conformational stability. When the binding energy was less than −5.0 kJ mol−1, the ligand and receptor were generally considered to bind well. The results of virtual molecular docking showed that 16 active compounds of Poria cocos exhibited good levels of binding activity with AQP4 and Mpro, as shown in Table 2. The main effective components of Poria cocos were triterpenes and polysaccharides. Therefore, the visualization of the interaction between AQP4 and Mpro and the compound with the optimal junction fraction of Poria cocos was identified, including ergosterol peroxide, pachymic acid, and pachyman.
Table 2 Docking results of active ingredients and some chemical drugs with SARS-CoV-2 3CL hydrolase
MOL ID |
Compound |
OB% |
DL |
Molecular formula |
Molecular weight (g mol−1) |
AQP4 binding affinity (kJ mol−1) |
Mpro binding affinity (kJ mol−1) |
MOL000273 |
16α-Hydroxydehydrotrametenolic acid |
30.93 |
0.81 |
C30H46O4 |
470.7 |
−7.4 |
−7.1 |
MOL000275 |
Trametenolic acid |
38.71 |
0.80 |
C30H48O3 |
456.7 |
−7.4 |
−6.5 |
MOL000276 |
Dehydropachymic acid |
35.11 |
0.81 |
C33H50O5 |
526.8 |
−6.8 |
−7.3 |
MOL000279 |
Cerevisterol |
37.96 |
0.77 |
C28H46O3 |
430.7 |
−8.0 |
−7.5 |
MOL000280 |
Dehydrotumulosic acid |
31.07 |
0.82 |
C31H48O4 |
484.7 |
−8.2 |
−7.2 |
MOL000282 |
Stellasterol |
43.51 |
0.72 |
C28H46O |
398.7 |
−8.1 |
−7.4 |
MOL000283 |
Ergosterol peroxide |
40.36 |
0.81 |
C28H44O3 |
428.6 |
−8.3 |
−8.0 |
MOL000285 |
Polyporenic acid C |
38.26 |
0.82 |
C31H46O4 |
482.7 |
−7.1 |
−7.1 |
MOL000287 |
Eburicoic acid |
38.70 |
0.81 |
C31H50O3 |
470.7 |
−7.0 |
−6.7 |
MOL000288 |
Pachyman |
0.45 |
0.68 |
C20H36O14 |
500.56 |
−7.0 |
−5.9 |
MOL000289 |
Pachymic acid |
33.63 |
0.81 |
C33H52O5 |
528.8 |
−6.7 |
−7.3 |
MOL000290 |
Poricoic acid A |
30.61 |
0.76 |
C31H46O5 |
498.7 |
−7.8 |
−6.8 |
MOL000291 |
Poricoic acid B |
30.52 |
0.75 |
C30H44O5 |
484.7 |
−7.2 |
−7.0 |
MOL000292 |
Poricoic acid C |
38.15 |
0.75 |
C31H46O4 |
482.7 |
−7.2 |
−6.5 |
MOL000296 |
Hederagenin |
36.91 |
0.75 |
C30H48O4 |
472.7 |
−7.1 |
−7.0 |
MOL000300 |
Dehydroeburicoic acid |
44.17 |
0.83 |
C31H48O3 |
468.7 |
−7.9 |
−6.8 |
— |
Ebselen |
— |
— |
C13H9NOSe |
274.2 |
— |
−6.8 |
4.6.1 Analysis of the interaction between the active compounds of Poria cocos and AQP4. In a previous study, HO and Huber studied AQP4 and its inhibitors and analyzed the active binding region of arylsulfonamides, an AQP4 inhibitor.35,36 These authors found that the presence of the ARG-216 amino acid residue determined the permeability of AQP4. Fig. 7 shows that the binding energies of ergosterol peroxide, pachymic acid, and pachyman, to AQP4 were −8.3 kJ mol−1, −6.7 kJ mol−1, and −7.0 kJ mol−1, respectively. All of these compounds interacted with ARG-216 and adjacent amino acid residues to form hydrogen bonds for stable binding. We speculate that the combination of binding effects between these compounds and AQP4 played a key role in regulating water molecules inside the cells, and provided the first body of evidence to suggest that Poria cocos can clear dampness and promote diuresis.
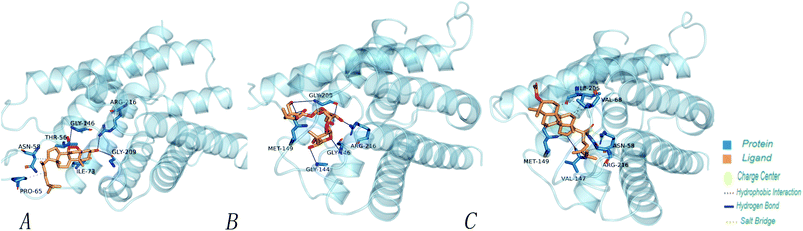 |
| Fig. 7 The minimum docking posture of three active drugs of Poria cocos at the AQP4 active site and the corresponding 3D interaction diagram of the docking posture. (A) Ergosterol peroxide (B) pachyman (C) pachymic acid. | |
4.6.2 Analysis of the interaction between the active compounds of Poria cocos and Mpro. Generally speaking, when the binding energy is less than −5.0 kJ mol−1, a ligand and receptor are generally considered to bind well. In a previous study, Zhenming used high throughput screening, enzymic activity inhibition, and qRT-PCR assays, to show that Ebselen, a key clinical and preclinical experimental drug, exhibited strong inhibitory effects upon Mpro activity, exerted antiviral effects, and had very low levels of cytotoxicity.4 Therefore, we use the docking score of Ebselen as a standard to determine whether the active compounds we used in our screening experiments could inhibit the activity of Mpro. As shown in Table 2, the binding capacity of Ebselen and Mpro was −6.8 kJ mol−1. The binding energies of 16 active compounds selected from Poria cocos and the active region of Mpro were all less than −5.0 kJ mol−1. These binding energies were similar to those of some currently used antiviral drugs, although the binding energies of a few active compounds exceeded −6.8 kJ mol−1.In order to more intuitively compare the affinity between active compounds of Poria cocos and Mpro, we used Ligplot+ v.2.2 software37 to display the relationship between active compounds of Poria cocos and amino acid residues. According to the 2D plot displayed by LigPlot, Thr25, Cys145, Gli143, His41, etc., were the main amino acids bound to active compounds of Poria cocos. The main forces between pachyman and Mpro were hydrogen bonds. Hydrogen bonds were formed between the hydroxyl group and carbonyl group of pachyman and several other amino acid residues in order to achieve stable binding, including HIS-41, ASN-142, GLU-166, and GLN-189. In general, the more rings a compound has, the more likely it is to fold, making the compound's spatial structure more suitable for entering the active pocket, or, in other words, a compound with more rings can provide more binding sites for interaction with the receptor. In terms of triterpenes in active compounds of Poria cocos, according to the number of rings, there are pentacyclic triterpenes, tetracyclic triterpenes and tricyclic triterpenes. Therefore, in terms of binding energy, pentacyclic triterpenes may have better binding energy with the receptor, but the specific binding site and its parent nuclear functional group substitution should be considered. If the receptor binding site itself is not suitable for the entry of the ring, it will lead to mutual repulsion, but multi-ring is not easy to combine. At the same time, substituent effect is also a factor to be considered, because different substituents will lead to the change of hydrogen bonding effect and hydrophobic effect, and eventually lead to the change of binding energy. Among the binding energies between active compounds of Poria cocos and Mpro, the binding energies of tricyclic triterpenes and Mpro were higher than those of other tetracyclic triterpenes and pentacyclic triterpenes.
Will tetracyclic triterpene 16alpha-hydroxydehydrotrametenolic acid (MOL000273) and pentacyclic triterpene hederagenin (MOL000296) comparison, MOL000273C16 and oxygen in the C30 can form two different oxygen atoms of hydrogen bonding, MOL000296C4 on the base of methanol is one atom of oxygen to form two hydrogen bonds. Therefore, the combination of the former and Mpro is better and the binding energy is lower. Moreover, tetracyclic triterpene ergosterol peroxide (MOL000283) and pentacyclic triterpene hederagenin (MOL000296) is compared, the 3 of the former can be on the same oxygen atoms to form two hydrogen bonds, also on the 19th, an oxygen atom can form hydrogen bond, due to the bridge and peroxide lipotropy mutual exclusion structure, the parent nucleus in the compound of oxygen ring located in the side, lipotropy conformation structure located at the side of the most stable, so the hydrogen bonding and hydrophobic action is strong, and MOL000296C4 on the base of methanol is a two oxygen atoms to form hydrogen bonds, at the same time, the former is C17 Mpro side chain and the receptor has the strong hydrophobic, and MOL000296C and D ring fused ring way different, Mpro after interaction with the D ring, probably because of the influence of the space steric interaction can not continue with the E ring. Therefore, the binding energy of MOL000283 is lower.
The contrast between tetracyclic triterpenes is mainly due to the influence of functional groups on the structure and amino acid residues. For example, dehydropachymic acid (MOL000276) is a dehydroid of pachymic acid (MOL000289). Both have the same binding energy with Mpro, and it can be seen from Table 3 that the sites and amino acid residues forming hydrogen bonds with Mpro are the same, indicating that hydrogenation of parent nuclear double bond may not affect their docking with Mpro. Furthermore, the comparison of dehydrotumulosic acid (MOL000280) and polyporenic acid C (MOL000285) shows that the hydroxyl group and carboxyl group of their structures form three hydrogen bonds with the amino group of HiS41, the amino group of Gly143 and the hydroxyl group of Leu141. The binding energy of MOL000285 and Mpro is slightly higher than that of MOL000280, possibly because the carbonyl group at C3 replaces the hydroxyl group, resulting in a slightly stronger hydrophobic effect than that of MOL000280.
Poricoic acid A (MOL000290), poricoic acid B (MOL000291) and poricoic acid C (MOL000292) were open ring types of tetracyclic triterpenes. Contrast between tricyclic triterpenes. Contrast between tricyclic triterpenes, the carboxyl group connected at position three forms two hydrogen bonds with the amino group of His41. The reason why MOL000291 and Mpro bind better than the other two may be that the hydroxyl group at position two forms hydrogen bonds with the hydroxyl group of Leu141. Our molecular docking result showed that the best Mpro binding activity of Poria cocos involved sterol triterpene (ergosterol peroxide), sterane triterpene (pachymic acid), and polysaccharides (pachyman). Fig. 8 shows a 3D visualization of the three active compounds.
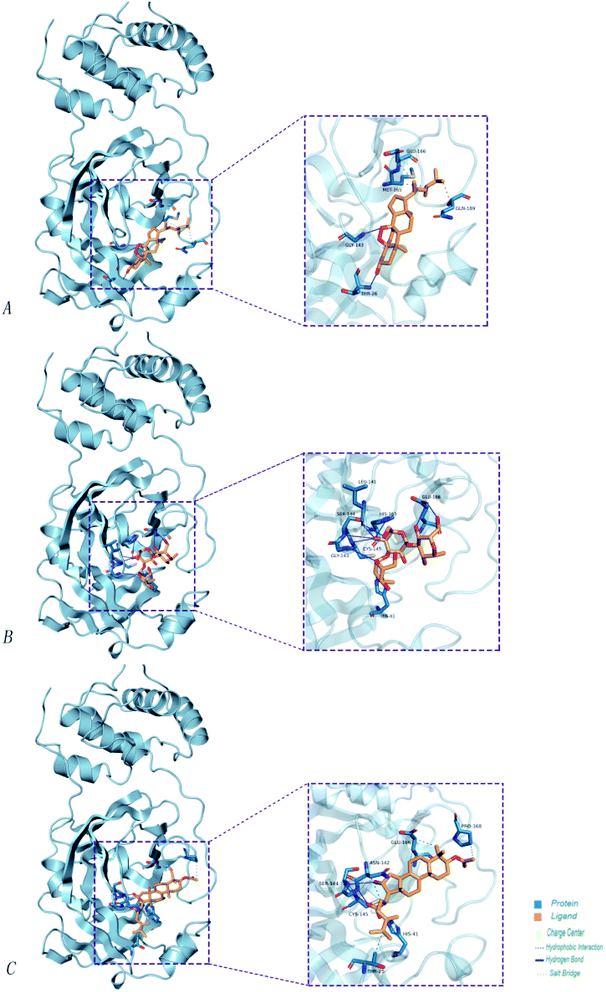 |
| Fig. 8 The minimum docking posture of three active drugs of Poria cocos and its corresponding docking posture 2D interaction diagram at the active site of Mpro. (A) Ergosterol peroxide (B) pachyman (C) pachymic acid. | |
4.7 Analysis of molecular dynamics simulation results
Our molecular docking result showed that the best Mpro binding activity of Poria cocos involved sterol triterpene (ergosterol peroxide), sterane triterpene (pachymic acid), and polysaccharides (pachyman). We extracted the optimal static conformation of these three compounds in the protein binding region. It is now commonly accepted that the spatial conformation of proteins is the basis of their functional activity, and when the conformation changes, the functional activity also changes. Molecular dynamics simulation can provide a virtual environment in which we can simulate the binding of proteins to small molecules and monitor their trajectory in a dynamic manner.
When analyzing a set of protein structures, RMSD (root-mean-square deviation) is usually used to measure the degree of deviation of all atoms on a skeleton with regards to atomic space coordinates. In order to compare the structural differences of the complexes before and after molecular dynamics simulation, the complexes were superposed and their RMSD values were calculated. Fig. 9(A) shows that after the binding of pachymic acid with Mpro, the RMSD value fluctuated between 0.2 and 0.25 nm after 2500 ps stabilized, and finally converged at approximately 0.2 nm. The RMSD value for ergosterol peroxide fluctuated between 0.2 and 0.3 nm after stabilizing at approximately 3000 ps. The RMSD of pachyman was stable at approximately 1000 ps and fluctuated from 0.20 to 0.35 nm to 9000 ps. These data indicated that pachyman was less active than pachymic acid and ergosterol peroxide. Furthermore, the range of fluctuation in the RMSD value of pachymic acid system was significantly lower than that of the other two compound systems, thus indicating that pachymic acid formed a relatively firm interaction in the active region of Mpro.
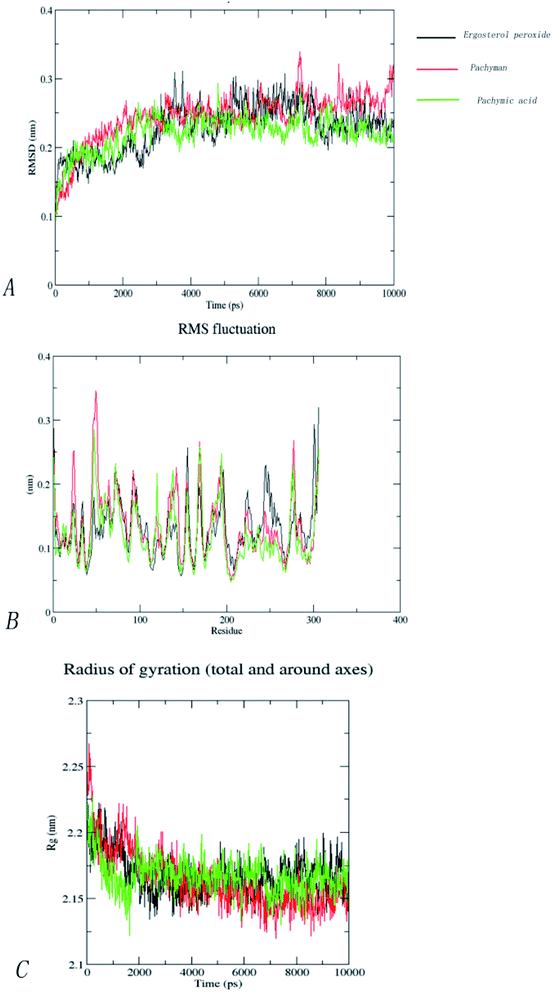 |
| Fig. 9 (A) RMSD of backbone of complexes with pachymic acid, ergosterol peroxide and pachyman. (B)RMSF of backbone of complexes with pachymic acid, ergosterol peroxide and pachyman. (C) Rg of backbone of complexes with pachymic acid, ergosterol peroxide and pachyman. | |
The RMSF (root-mean-square fluctuation) can be used to evaluate the impact of a compound on the flexibility of the amino acids within the receptor protein during molecular dynamics simulations, thus reflecting residual fluctuations in the entire system or among different systems. Fig. 9(B) shows that the trends for RMSF fluctuation in the three systems were very similar. The three systems showed fluctuations in amino acids 46 to 50 and 71 to 75 (RMSF < 0.3 nm). By investigating the amino acid sequence and location, we found that most of the fluctuating regions were located at the edge of the active region. The amino acids showing low levels of fluctuation in the three systems roughly coincided with the positions of the key amino acids, such as CYS145, HIS41, and GLY143. This effect may be due to stable hydrogen bonds or hydrophobic actions between the compound and the protein; collectively, these factors would have increased the stability of the complex. Analysis showed that the fluctuation of RMSF value in the amino acid residues in the three systems was less than 0.3 nm, thus indicating that the binding of the three compounds to proteins was relatively stable during the molecular dynamics simulations.
The radius of rotation (Rg), which depends on the relationship of atomic mass to the center of gravity in a given molecule, can be used to characterize the density of a protein structure. A larger Rg value indicates that the system expands during dynamic simulation. Fig. 9(C) clearly shows changes in the Rg within the three systems in the 10
000 ps molecular dynamics simulations. Furthermore, the Rg of the three systems gradually decreased to become stable and finally converged within the range of 2.15–2.20 nm. This indicated that the three compounds have little influence on the density of the protein structures.
In order to further analyze the stability of binding between small molecules and Mpro proteins, we used MM-PBSA to calculate the binding free energy of the complex system. According to the energies shown in Table 4 for the three composite systems, it was evident that the main van der Waals forces were conducive to the combination of small molecules and proteins. In contrast, the polar solvation forces of the system were not conducive to the combination of small molecules and proteins. According to Table 4, the positive binding capacity of pachyman indicated that the stability of binding between pachyman and Mpro was not as good as the other two compounds. The binding energy of the three compounds and Mpro protein was highest with ergosterol peroxide, followed by pachymic acid, and then pachyman. These findings were consistent with those arising from our molecular docking experiments.
Table 3 2D docking results of 16 active compounds of Poria cocos and Mpro were shown
|
MOL ID |
Compound |
Molecular structure |
The 2D interaction diagrams |
Pentacyclic triterpene |
MOL000296 |
Hederagenin |
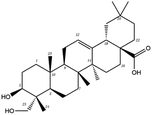 |
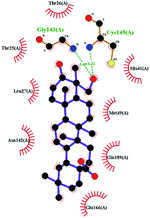 |
Tetracyclic triterpene |
MOL000273 |
16alpha-hydroxydehydrotrametenolic acid |
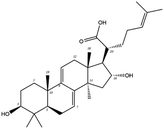 |
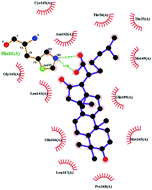 |
MOL000275 |
Trametenolic acid |
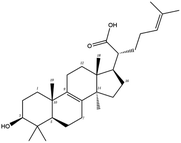 |
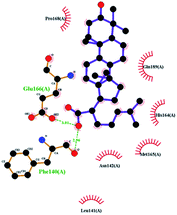 |
|
MOL000276 |
Dehydropachymic acid |
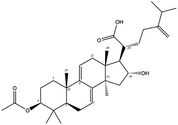 |
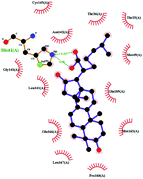 |
|
MOL000280 |
Dehydrotumulosic acid |
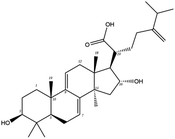 |
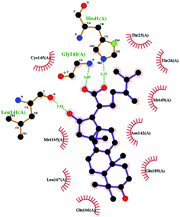 |
|
MOL000285 |
Polyporenic acid C |
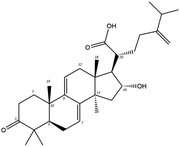 |
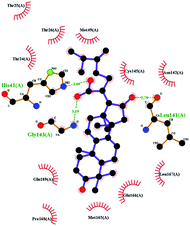 |
|
MOL000287 |
Eburicoic acid |
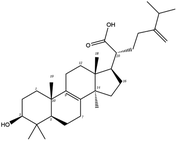 |
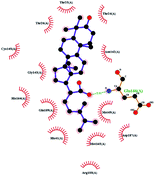 |
|
MOL000289 |
Pachymic acid |
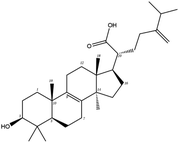 |
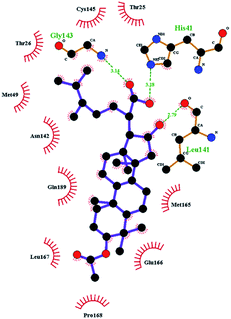 |
|
MOL000300 |
Dehydroeburicoic acid |
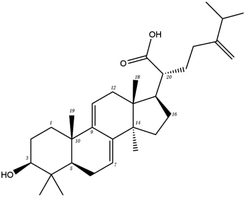 |
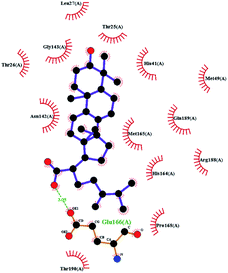 |
|
MOL000279 |
Cerevisterol |
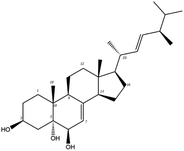 |
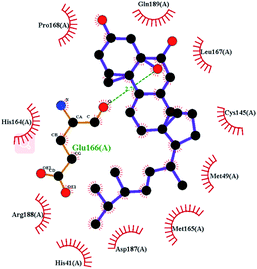 |
|
MOL000282 |
Stellasterol |
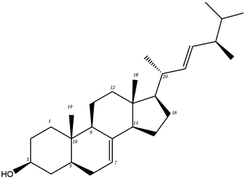 |
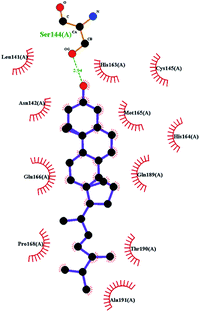 |
|
MOL000283 |
Ergosterol peroxide |
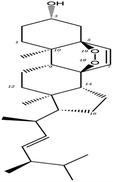 |
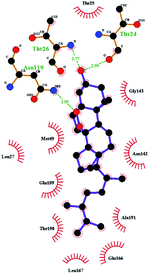 |
Tricyclic triterpenes |
MOL000290 |
Poricoic acid A |
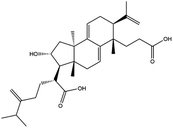 |
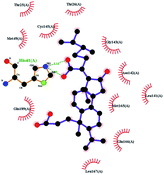 |
MOL000291 |
Poricoic acid B |
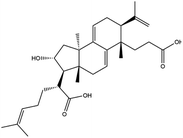 |
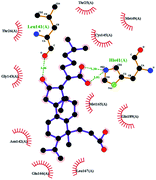 |
MOL000292 |
Poricoic acid C |
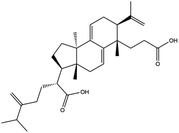 |
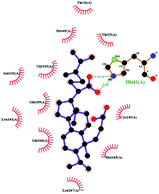 |
Polysaccharide |
MOL000288 |
Pachyman |
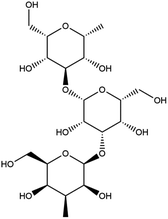 |
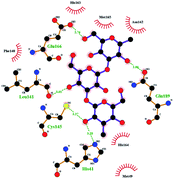 |
Table 4 MM-PBSA calculations of binding free energy for three selected complexes
MOL ID |
Compound |
ΔEvdW (kJ mol−1) |
ΔEELE (kJ mol−1) |
ΔGPA (kJ mol−1) |
ΔGSA (kJ mol−1) |
ΔGBind (kJ mol−1) |
MOL000283 |
Ergosterol peroxide |
−172.591 ± 16.230 |
−10.233 ± 6.135 |
56.609 ± 8.855 |
−16.702 ± 1.335 |
−142.917 ± 14.580 |
MOL000288 |
Pachyman |
−112.260 ± 21.247 |
30.854 ± 29.114 |
143.576 ± 38.069 |
348.632 ± 6.008 |
49.122 ± 20.777 |
MOL000289 |
Pachymic acid |
−213.345 ± 20.389 |
25.379 ± 40.160 |
194.958 ± 31.833 |
−20.572 ± 1.362 |
−13.581 ± 19.301 |
To better understand the contribution of important residues to the total binding energy, we used the MMPbsadecomp.py script to decompose the total binding energy of the system. Fig. 10 shows the contribution of amino acid residues in the binding pocket to the total binding energy. It was evident that several amino acid residues (THR-25, LEU-27, MET-49, MET-165 and GLN-189) have a greater impact on the binding energy of the ergosterol peroxide system. Other amino acid residues (ARG-40, GLU-47, ASP-48, ARG131, LYS-137, and GLU-166) had great influence on the binding energy of the other two systems. According to the molecular docking fraction and the binding free energy value after molecular dynamics simulation, it was evident that pachyman had the lowest inhibitory effect on Mpro activity among the three compounds. By comparing the data shown in Fig. 10, it was evident that the differences in binding energy between pachyman and the other two compounds were mainly present in certain sequences of the amino acids (25–27, 142–145, and 166–168) and the MET-49 residue. By combining with Fig. 8(B), it can be inferred that stable binding between small molecule compounds and the Mpro protein was reliant on certain amino acid regions (25–27, 142–145, and 166–168) and that these regions played critical roles in binding.
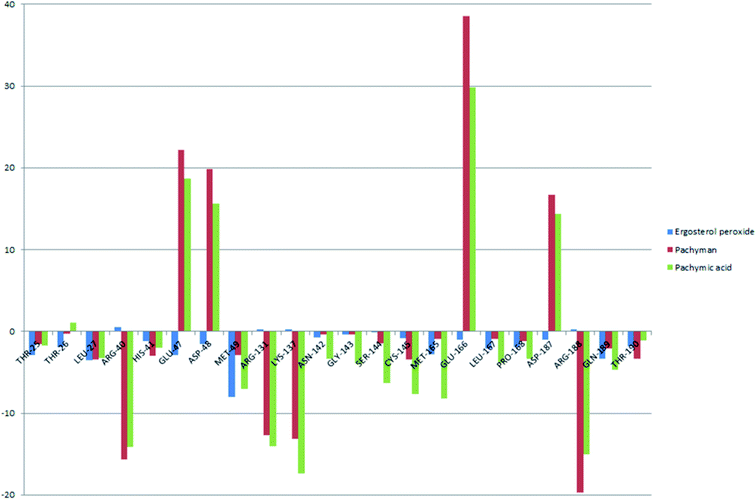 |
| Fig. 10 The energy contribution of partial residues after system binding energy decomposition (A) ergosterol peroxide (B) pachyman (C) pachymic acid. | |
4.8 The inhibition of SARS-COV-2 3CL hydrolytic enzyme activity by pachymic acid
Heterologous recombination expression and the purification of SARS-COV-2 3CL hydrolases have already been accomplished in other studies,38 as shown in Fig. 11. This aspect of the present research was carried out by Chen Shoudeng's research group in the Guangdong Key Laboratory of Biomedical Imaging, The Fifth Affiliated Hospital of Sun Yat-sen University. Fig. 11(A) shows the effect of different concentrations of pachymic acid on fluorescence intensity following addition of the enzyme reaction mixture. Changes of fluorescence intensity can reflect the inhibitory activity of the substance on the Mpro protein. The relationship between different concentrations of pachymic acid and SARS-COV-2 3CL hydrolase inhibition is demonstrated in Fig. 12(B); the IC50 was 18.607 μmol L−1.
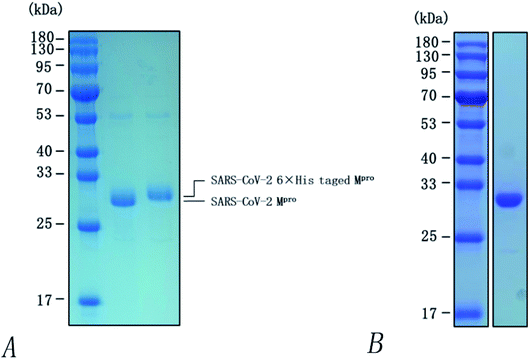 |
| Fig. 11 SDS-PAGE analysis of SARS-CoV-2 3CL hydrolase (Mpro). (A) Identification of Mpro by 12% SDS-PAGE. (1) marker, (2) Mpro-wt which cleavage by rhinovirus 3C-like protease, (3) Mpro after the cleavage of C-terminal His tag; (B) purification of Mpro by 14% SDS-PAGE. | |
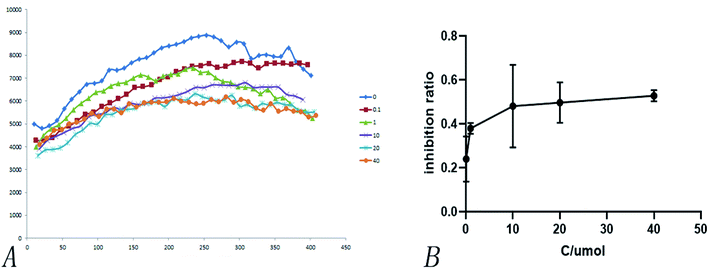 |
| Fig. 12 Inhibition of pachymic acid for SARS-CoV-2 3CL hydrolase. (A) Effect of different concentrations of pachymic acid on fluorescence intensity; (B) inhibitory activity of pachymic acid on SARS-CoV-2 3CL hydrolase. The inhibition rate was acquired by the calculation of initial rates calculated by fitting the straight-line portion of the curves (the first 400 second) in (A). | |
5 Discussion
Network pharmacology can be used to establish a “drug-target-disease” network by using multiple databases and allows us to understand the characteristics and molecular mechanisms associated with a key concept of TCM: that “one drug corresponds to multiple targets and one target corresponds to multiple drugs”.39 Poria cocos, a famous TCM that was first recorded in the Eastern Han Chinese book “Shen Nong Ben Cao Jing”, can exert numerous beneficial effects, including clearing damp, promoting diuresis, and strengthening the spleen and stomach. According to China's COVID-19 Diagnosis and Treatment Protocol (Trial; Seventh Edition), Poria cocos is found in many TCM treatment prescriptions, indicating that it may play an important role in COVID-19 intervention. Clinical evidence has shown that TCM has a positive effect on the treatment of patients with COVID-19.40 Poria cocos contains a number of active components that can be conducive to body functions by acting on multiple targets and related pathways.
Poria cocos belongs to the homologous substance of medicine and food.41 The main components of Poria cocos are polysaccharides, triterpenoid steranes and triterpenoid sterols, which have anti-inflammatory, anti-tumor, anti-oxidation and anti-aging activities as well as immunomodulant and other biological activities.42–46 Most of the active compounds screened out are triterpenes. Triterpenes have been found to exert a wide range of physiological activities, such as antiviral, antibacterial, anticancer, anti-inflammatory, and antipyretic effects. The main anti-inflammatory mechanism of pachymic acid involves reducing adhesion between white blood cells and microvascular endothelial cells. This compound then inhibits the excessive secretion of microvascular endothelial cells and prevents an excessive number of white blood cells from reaching the inflammatory site; consequently, pachymic acid exerts an anti-inflammatory effect.8 Pachymic acid and dehydropachymic acid also have the effect of promoting nerve extension, and can effectively clear the accumulation of Aβ1-42 in cells.47,48 The effect of eburicoic acid on LPS-induced RAW264.7 cell inflammation may arise by regulating the PI3K/Akt/mTOR/NF-KB signaling pathway to inhibit a number of inflammatory mediators (PGE2, COX-2, TNF-α, and IL-6).49 Eburicoic acid and dehydroeburicoic acid can also downregulate the activity of inflammatory factors and antioxidant enzymes and thus exert anti-inflammatory and antioxidant effects to protect liver tissues in mice with acute liver damage.50 Dehydrotumulosic acid has anti-inflammatory activity independent of glucocorticoid mechanism, and it can play an anti-tumor role by inhibiting the efflux of anticancer drugs, thus reversing cell resistance.51,52 Trametenolic acid also has anti-tumor effect, which plays a role by anti-proliferation and reversing cell drug resistance.53,54 Polyporenic acid C plays an anti-tumor role by inducing cell apoptosis by inhibiting PI3K/Akt signaling pathway and other ways.55 Hederagenin is also a triterpene compound. According to the current research on drug activity, hederagenin has anti-inflammatory, lipid-lowering, anti-depressant, anti-tumor and other physiological activities.56–60 Poricoic acid A, poricoic acid B and poricoic acid C are tetracyclic triterpenoids. Poricoic acid A and poricoic acid C mainly reduce renal fibrosis by regulating Wnt/β-catenin, AMPK and other signaling pathways.61–63 Poricoic acid A and poricoic acid B both have inhibitory effects on the growth of lung cancer cells and have significant cytotoxicity on human promyeloid leukemia cells.64,65 Pachyman is the main effective component of Poria cocos. Modern pharmacology shows that pachyman and its derivatives can enhance cellular immune response, while also exerting anti-tumor, antibacterial, antiviral, and anti-inflammatory effects.66,67 The selected sterol components of the active compound were ergosterol peroxide, stellasterol, and cerevisterol. Sterol compounds have anti-inflammatory, cholesterol-lowering, anti-tumor, anti-oxidation and other effects.68–70 Modern studies have shown that ergosterol peroxide has antibacterial, antioxidant, anti-tumor, and antiviral effects, and can block the activation of the downstream RIG-1 signaling pathway (P38 MAPK and NF-KB) to inhibit the pro-inflammatory response and apoptosis induced by influenza A virus.71 Cerevisterol can reduce the expression of inflammatory mediators and pro-inflammatory factors by modulating a series of signal cascades involving the MAPK/NF-KB/AP-1/Nrf2/Ho-1 axis to reduce the inflammatory response.72 Stellasterol, isolated from M. esculenta, achieves antioxidant effects by inhibiting the NF-kB pathway,68 and other studies have shown that stellasterol has potential as a hypoglycemic agent.73
In the present study, network pharmacology showed that Poria cocos may maintain internal water balance via G protein coupled receptor signaling pathways, regulating ion channel activity, and by regulating hormone levels. Furthermore, molecular docking studies involving AQP4 provided a preliminary verification of the molecular mechanisms underlying the efficacy of Poria cocos. In order to further understand the molecular mechanisms associated with COVID-19 intervention, the targets of Poria cocos were mapped with the targets of COVID-19 and the intersection target was enriched for analysis. KEGG enrichment analysis showed that multiple active components of Poria cocos might intervene with COVID-19 and that these interventions occurred via inflammation, immunity, and virus-related pathways.
We also generated a PPI network; this showed that MAPK3, TNF, and PTGS2, were predicted to be the most likely key targets for COVID-19 intervention. Pathway enrichment analysis showed that the MAPK pathway might be the dominant pathway. Recent literature published in the Lancet indicated that the development of COVID-19 was closely related to inflammatory storms. Inflammatory storm refers to a severe systemic inflammatory response characterized by a rapid increase in the number of pro-inflammatory cytokines (TNF-α, IL-6, etc.) over a short period of time.74 The prevention of inflammatory storms, and regulation of the immune response, are currently the main entry points for treating COVID-19. According to our KEGG enrichment results, the MAPK family is involved in a number of inflammatory and immune-related signaling pathways, including those featuring TNF, HIF-1, the T cell receptor, and the toll-like receptor. As one of the most important pathways of inflammatory factors, the MAPK signaling pathway plays an important role in inflammatory diseases of the lungs and other tissues related to respiration.75 In addition, human coronavirus (HCoVs) may regulate MAPK signaling pathway and other pathways.76 Previous research showed that it was possible to reduce the inflammatory response in mice exhibiting LPS-induced acute respiratory distress syndrome (ARDS) by inhibiting the phosphorylation of ERK and p38 MAPK and by reducing the secretion of TNF-α and other inflammatory factors in the lungs.77 Poria cocos may also regulate transcription factors, such as AP-1 and NF-B, by interfering with the MAPK signaling pathway, thereby regulating the TNF signaling pathway, the NF-KB pathway, and other downstream pathways, and by inhibiting the release of pro-inflammatory factors.76 In the present study, network pharmacology revealed that Poria cocos may exert effects on COVID-19 in a manner that features multiple components, targets, and pathways. However, network pharmacological data cannot reliably predict the effect of drugs when used to treat a disease. Mpro is the main protease of the SARS-COV-2 virus and is currently considered as an important target for designing inhibitors. Over recent years, molecular docking and molecular dynamics simulation techniques for computer-aided drug research have greatly reduced the cost of drug discovery.17,78 Based on our molecular docking and molecular dynamics simulations, we selected pachymic acid to measure the activity of the SARS-COV-2 3CL hydrolytic enzyme. Pachymic acid is based on a triterpene structure and represents the main component of Poria cocos; the triterpene active component of this compound exhibits low binding energy with Mpro. Our analyses showed that pachymic acid exhibits certain inhibitory effects on the SARS-CoV-2 3CL hydrolytic enzyme.
6 Conclusion
In the present study, we used network pharmacology to demonstrate that Poria cocos exerts effects on COVID-19 in a manner that features interactions between multiple components, targets, and pathways. As the main protease of COVID-19, Mpro represents a key target for the design of potential inhibitors. Through molecular docking, we learned that the selected active ingredients could bind in the active region of Mpro and that the docking score of most active ingredients was close to that of ebselen. These data provided a theoretical basis and reference value for clinical application and represented a major step forward for drug discovery projects featuring Poria cocos. However, network pharmacology and molecular docking can only play a predictive role and have certain limitations. We conducted molecular dynamics simulations, and enzyme activity verifications, and discovered that the active components of Poria cocos could inhibit the activity of the Mpro enzyme. However, the division of labor and the cooperative mechanisms by which different active compounds in Poria cocos can act against COVID-19 have yet to be elucidated. From a medical point of view, it is also possible that certain factors could influence the activity of these active compounds. For example, when extracting a compound, it is likely that temperature may affect its activity, interactions with other drugs, and pharmacokinetics. Consequently, the specific mechanisms of action related to these active compounds need to be investigated further.
Conflicts of interest
The authors declare that there are no conflicts of interests.
Acknowledgements
This work was funded by the 2020 Zhangzhengpu Expert Workstation in Pu'er City.
References
- T. Singhal, A Review of Coronavirus Disease-2019 (COVID-19), Indian J. Pediatr., 2020, 87(4), 281–286 CrossRef.
- Z. Jin, X. Du, Y. Xu, Y. Deng, M. Liu, Y. Zhao, B. Zhang, X. Li, L. Zhang, C. Peng, Y. Duan, J. Yu, L. Wang, K. Yang, F. Liu, R. Jiang, X. Yang, T. You, X. Liu, X. Yang, F. Bai, H. Liu, X. Liu, L. W. Guddat, W. Xu, G. Xiao, C. Qin, Z. Shi, H. Jiang, Z. Rao and H. Yang, Structure of Mpro from SARS-CoV-2 and discovery of its inhibitors, Nature, 2020, 582, 289–293 CrossRef CAS PubMed.
- M. Qing, C. Xiaodong, W. Bing, W. Yuguang and Z. Zhongde, Understanding and Thinking of Novel Coronavirus Pneumonia in Trditinal Chinese Medicine, J. Tradit. Chin. Med., 2020, 61(04), 286–288 Search PubMed.
- L. Peilu, Characteristics and main diseases of curdy fur, Shandong J. Tradit. Chin. Med., 2003, 10, 632 Search PubMed.
- Y. Guang, C. Guang and W. Jie, Connotation Interpretation and Clinical Application of Thick Greasy Tongue Coating, J. Tradit. Chin. Med., 2019, 60(06), 472–476 Search PubMed.
- L. Hui, Y. Wenxian, Z. Fulan, C. Li, Y. Sijin and M. Xiaoqin, Effect of Self-Developed Fuzhengbixie Formula and Qingfeipaidu Mixture on the Prevention and Treatment of the Coronavirus Disease 2019, China Pharm., 2020, 29(07), 9–11 Search PubMed.
- W. Fengxia, Z. Yun and L. Kechun, Prevention and control strategies of COVID-19 based on theory of principle,method and prescription of Treatise on Febrile Diseases, Shandong Sci., 2020, 33(02), 12–16 Search PubMed.
- C. Herong, W. Ruilin, G. Wenbo, W. Penglong and M. Tao, et al. Research advances in chemical components, pharmacological activities and clinical application of Poria cocos, Northwest Pharm. J., 2019, 34(05), 694–700 Search PubMed.
- W. Pengcheng, Z. Shan, W. Qiuhong and K. Haixue, Relationship between efficacy exertion of diuretic traditional Chinese medicines and aquaporin, China J. Chin. Mater. Med., 2015, 40(12), 2272–2277 Search PubMed.
- http://www.gov.cn/zhengce/zhengceku/2020-08/19/5535757/files/da89edf7cc9244fbb34ecf6c61df40bf.pdf.
- S. Jie, Z. Minxia, X. Shengyang and S. Caihua, Composition Regularities of Chinese Materia Medica Formulas for the Coronavirus Disease 2019, China Pharm., 2020, 29(06), 25–28 Search PubMed.
- W. Gang and J. Jingsong, Clinical Treatment to COVID-19 in Line with its Pathogenesis Evolution and Classic Formula, Jiangsu J. Tradit. Chin. Med., 2020, 52(04), 18–22 Search PubMed.
- Z. Huiyong, W. Wei, L. Jingnan, C. Zhi-hui and Z. Jingsheng, Analysis of pathogenesis clauses and prescriptions of “Treatise on differentiation and treatment of epidemic febrile disease” related to clinical symptoms of COVID-19, Chin. Tradit. Herb. Drugs, 2020, 51(06), 1455–1462 Search PubMed.
- A. L. Hopkins, Network pharmacology: the next paradigm in drug discovery, Nat. Chem. Biol., 2008, 4(2), 682–690 CrossRef CAS PubMed.
- H. Xu, L. Huang, P. Lu and H. Yang, [Application of ADME process in vivo in combination with network pharmacology in study of traditional Chinese medicine], Zhongguo Zhongyao Zazhi, 2012, 37(2), 142–145 Search PubMed.
- D. Xianchun, H. Shi, P. Daiyin, H. Lan, W. Xiaoli, W. Yongzhong and P. Lingyu, Application of network pharmacology in the study of traditional Chinese medicine formula, Chin. Pharmacol. Bull., 2020, 36(03), 303–308 Search PubMed.
- J. Fan, A. Fu and L. Zhang, Progress in molecular docking, Quant. Biol., 2019, 7(2), 83–89 CrossRef CAS.
- R. Salomon-Ferrer, D. A. Case and R. C. Walker, An overview of the Amber biomolecular simulation package, Wiley Interdiscip. Rev.: Comput. Mol. Sci., 2013, 3(2), 198–210 CAS.
- J. Ru, P. Li, J. Wang, W. Zhou, B. Li, C. Huang, P. Li, Z. Guo, W. Tao, Y. Yang, X. Xu, Y. Li, Y. Wang and L. Yang, TCMSP: a database of systems pharmacology for drug discovery from herbal medicines, J. Cheminf., 2014, 6(1), 13 Search PubMed.
- W. Tao, X. Xu, X. Wang, B. Li, Y. Wang, Y. Li and L. Yang, Network pharmacology-based prediction of the active ingredients and potential targets of Chinese herbal Radix Curcumae formula for application to cardiovascular disease, J Ethnopharmacol, 2013, 145(1), 1–10 CrossRef CAS.
- S. Kim, P. A. Thiessen, E. E. Bolton, J. Chen, G. Fu, A. Gindulyte, L. Han, J. He, S. He, B. A. Shoemaker, J. Wang, B. Yu, J. Zhang and S. H. Bryant, PubChem Substance and Compound databases, Nucleic Acids Res, 2016, 44, D1202–D1213 CrossRef CAS.
- The UniProt Consortium, UniProt: a worldwide hub of protein knowledge, Nucleic Acids Res, 2019, 47, D506–D515 CrossRef PubMed.
- G. Stelzer, N. Rosen, I. Plaschkes, S. Zimmerman, M. Twik, S. Fishilevich, T. I. Stein, R. Nudel, I. Lieder, Y. Mazor, S. Kaplan, D. Dahary, D. Warshawsky, Y. Guan-Golan, A. Kohn, N. Rappaport, M. Safran and D. Lancet, The GeneCards Suite: From Gene Data Mining to Disease Genome Sequence Analyses, Curr. Protoc. Bioinf., 2016, 54(1), 1.30.1–1.30.33 Search PubMed.
- P. Shannon, A. Markiel, O. Ozier, N. S. Baliga, J. T. Wang, D. Ramage, N. Amin, B. Schwikowski and T. Ideker, Cytoscape: a software environment for integrated models of biomolecular interaction networks, Genome Res, 2003, 13, 2498–2504 CrossRef CAS PubMed.
- C. von Mering, L. J. Jensen, B. Snel, S. D. Hooper, M. Krupp, M. Foglierini, N. Jouffre, M. A. Huynen and P. Bork, STRING: known and predicted protein-protein associations, integrated and transferred across organisms, Nucleic Acids Res, 2005, 33, D433–D437 CrossRef CAS PubMed.
- Y. Zhou, B. Zhou, L. Pache, M. Chang, A. Hadj Khodabakhshi, O. Tanaseichuk, C. Benner and S. K. Chanda, Metascape provides a biologist-oriented resource for the analysis of systems-level datasets, Nat. Commun., 2019, 10, 1523 CrossRef PubMed.
- H. Berman, K. Henrick and H. Nakamura, Announcing the worldwide Protein Data Bank, Nat. Struct. Biol., 2003, 10, 980 CrossRef CAS PubMed.
- S. Yuan, H. C. S. Chan, S. Filipek and H. Vogel, PyMOL and Inkscape Bridge the Data and the Data Visualization, Structure, 2016, 24, 2041–2042 CrossRef CAS PubMed.
- O. Trott and A. J. Olson, AutoDock Vina: improving the speed and accuracy of docking with a new scoring function, efficient optimization and multithreading, J. Comput. Chem., 2010, 31, 455–461 CAS.
- S. Salentin, S. Schreiber, V. J. Haupt, M. F. Adasme and M. Schroeder, PLIP: fully automated protein-ligand interaction profiler, Nucleic Acids Res., 2015, 43(W1), W443–W447 CrossRef CAS PubMed.
- P. V. Klimovich and D. L. Mobley, A Python tool to set up relative free energy calculations in GROMACS, J. Comput.-Aided Mol. Des., 2015, 29(11), 1007–1014 CrossRef CAS PubMed.
- R. Kumari and R. Kumar, Open Source Drug Discovery Consortium and A. Lynn, g_mmpbsa—A GROMACS Tool for High-Throughput MM-PBSA Calculations, J. Chem. Inf. Model., 2014, 54, 1951–1962 CrossRef CAS PubMed.
- M. Lv, S. Ma, Y. Tian, X. Zhang, W. Lv and H. Zhai, Computational studies on the binding mechanism between triazolone inhibitors and Chk1 by molecular docking and molecular dynamics, Mol. BioSyst., 2015, 11(1), 275–286 RSC.
- Z. Yunpeng, W. Peng, X. Boran, T. Yanting and W. Quan, Screening and Inhibition Kinetics of SARS Coronavirus Main Protease Inhibitors, China Biotechnol., 2016, 36(04), 35–42 Search PubMed.
- J. D. Ho, R. Yeh, A. Sandstrom, I. Chorny, W. E. C. Harries, R. A. Robbins, L. J. W. Miercke and R. M. Stroud, Crystal structure of human aquaporin 4 at 1.8 Å and its mechanism of conductance, Proc. Natl. Acad. Sci. U. S. A., 2009, 106, 7437–7442 CrossRef CAS PubMed.
- V. J. Huber, M. Tsujita, M. Yamazaki, K. Sakimura and T. Nakada, Identification of arylsulfonamides as Aquaporin 4 inhibitors, Bioorg, Med. Chem. Lett., 2007, 17, 1270–1273 CrossRef CAS PubMed.
- A. C. Wallace, R. A. Laskowski and J. M. Thornton, LIGPLOT: a program to generate schematic diagrams of protein-ligand interactions, Protein Eng., 1995, 8, 127–134 CrossRef CAS PubMed.
- Z. Danshui, C. Xiaoxue, W. Zhimin and N. Weiju, Potential active compounds of Liupao tea for prevention and treatment of COVID-19 based on network pharmacology and molecular docking, Journal of China Pharmaceutical University, 2020, 51(05), 556–567 Search PubMed.
- R. Zhang, X. Zhu, H. Bai and K. Ning, Network Pharmacology Databases for Traditional Chinese Medicine: Review and Assessment, Front. Pharmacol., 2019, 10, 123 CrossRef CAS.
- D. Zhang, B. Zhang, J.-T. Lv, R.-N. Sa, X.-M. Zhang and Z.-J. Lin, The clinical benefits of Chinese patent medicines against COVID-19 based on current evidence, Pharmacol. Res., 2020, 157, 104882 CrossRef CAS PubMed.
- http://www.nhc.gov.cn/zwgk/wtwj/201304/e33435ce0d894051b15490aa3219cdc4.shtml.
- S. Cheng, Y. Gui, S. Shen and W. Huang, The Antioxidant Activity of Triterpenes in the Peels of Poria cocos, Med. Plant, 2013, 4(08), 38–44 CAS.
- B.-F. Chu, H.-C. Lin, X.-W. Huang, H.-Y. Huang, C. P. Wu and M.-C. Kao, An ethanol extract of Poria cocos inhibits the proliferation of non-small cell lung cancer A549 cells via the mitochondria-mediated caspase activation pathway, J. Funct. Foods, 2016, 23, 614–627 CrossRef CAS.
- S. R. Lee, S. Lee, E. Moon, H.-J. Park, H. B. Park and K. H. Kim, Bioactivity-guided isolation of anti-inflammatory triterpenoids from the sclerotia of Poria cocos using LPS-stimulated Raw264.7 cells, Bioorg. Chem., 2017, 70, 94–99 CrossRef CAS PubMed.
- Y. Jiang and L. Fan, Evaluation of anticancer activities of Poria cocos ethanol extract in breast cancer: In vivo and in vitro, identification and mechanism, J. Ethnopharmacol., 2020, 257, 112851 CrossRef CAS PubMed.
- Z. Nian, L. Zhaoxing, L. Juan, L. Jing, D. Jiamu, D. Y. Lee. and L. Shunxiang, Advances in the Research of Constituents and Pharmacological Effects of Poria cocos (Schw.) Wolf, Modernization of Traditional Chinese Medicine and Materia Medica-World Science and Technology, 2019, 21(02), 220–233 Search PubMed.
- M. Yu, X. Xu, N. Jiang, W. Wei, F. Li, L. He and X. Luo, Dehydropachymic acid decreases bafilomycin A1 induced β-Amyloid accumulation in PC12 cells, J. Ethnopharmacol., 2017, 198, 167–173 CrossRef CAS.
- L. Guiqin, W. Xiaofeng and T. Wanxia, Studies on the chemical constituents and bioactivity of Poria cocos Wolf, J. Qiqihar Univ., Nat. Sci. Ed., 2015, 31(04), 50–54 Search PubMed.
- J. Wang, P. Zhang, H. He, X. Se, W. Sun, B. Chen, L. Zhang, X. Yan and K. Zou, Eburicoic acid from Laetiporus sulphureus (Bull.:Fr.) Murrill attenuates inflammatory responses through inhibiting LPS-induced activation of PI3K/Akt/mTOR/NF-κB pathways in RAW264.7 cells, Naunyn-Schmiedeberg's Arch. Pharmacol., 2017, 390(8), 845–856 CrossRef CAS PubMed.
- G.-J. Huang, J.-S. Deng, S.-S. Huang, C.-Y. Lee, W.-C. Hou, S.-Y. Wang, P.-J. Sung and Y.-H. Kuo, Hepatoprotective effects of eburicoic acid and dehydroeburicoic acid from Antrodia camphorata in a mouse model of acute hepatic injury, Food Chem., 2013, 141(3), 3020–3027 CrossRef CAS PubMed.
- E.-M. Giner, S. Máñez, M.-C. Recio, R.-M. Giner, M. Cerdá-Nicolás and J.-L. Ríos, In Vivo Studies on the Anti-Inflammatory Activity of Pachymic and Dehydrotumulosic Acids, Planta Med., 2000, 66(3), 221–227 CrossRef CAS PubMed.
- H. Shan, Z. Qinglin, X. Fengjun, L. Yuxin, C. Xiaochen and H. Yuan, Reversal of multidrug resistance of KBV200 cells by triterpenoids isolated from Poria cocos, Planta Med, 2012, 78, 428–433 CrossRef PubMed.
- J. Kim, S. C. Yang, A. Y. Hwang, H. Cho and K. T. Hwang, Composition of Triterpenoids in Inonotus obliquus and Their Anti-Proliferative Activity on Cancer Cell Lines, Molecules, 2020, 25, 4066 CrossRef CAS PubMed.
- Q. Zhang, J. Wang, H. He, H. Liu, X. Yan and K. Zou, Trametenolic Acid B Reverses Multidrug Resistance in Breast Cancer Cells Through Regulating the Expression Level of P-Glycoprotein, Phytother. Res., 2014, 28(7), 1037–1044 CrossRef CAS PubMed.
- H. Ling, L. Zhou, X. Jia, L. A. Gapter, R. Agarwal and K.-y. Ng, Polyporenic acid C induces caspase-8-mediated apoptosis in human lung cancer A549 cells, Mol. Carcinog., 2009, 48, 498–507 CrossRef CAS PubMed.
- G.-J. Kim, D. H. Song, H. S. Yoo, K.-H. Chung, K. J. Lee and J. H. An, Hederagenin Supplementation Alleviates the Pro-Inflammatory and Apoptotic Response to Alcohol in Rats, Nutrients, 2017, 9(1), 41 CrossRef PubMed.
- C. W. Lee, S. M. Park, R. Zhao, C. Lee, W. Chun, Y. Son, S. H. Kim, J. Y. Jung, K. H. Jegal, I. J. Cho, S. K. Ku, Y. W. Kim, S. A. Ju, S. C. Kim and W. G. An, Hederagenin, a major component of Clematis mandshurica Ruprecht root, attenuates inflammatory responses in RAW 264.7 cells and in mice, Int. Immunopharmacol., 2015, 29(2), 528–537 CrossRef CAS PubMed.
- L. Baofang, C. Yufang, X. Tian, G. Haibiao, Y. Xin and X. Jiangping, Pharmacodynamical evaluation of hederagenin as an antidepressant, Mil. Med. Sci., 2013, 37(04), 286–290 Search PubMed.
- L. Jiawei, L. Wanquan and Z. Meinan, Study On Lipid-lowering Activity Of Hederagenin In Vitro, J. Tradit. Chin. Med., 2019, 41(12), 3–6 Search PubMed.
- Z. Zhenxia, Z. Zhenmin and Z. Chengguang, Effects of hederagenin on proliferation, migration and invasion of prostate cancer DU145 cell, Pharmacol. Clin. Chin. Mater. Med., 2017, 33(01), 37–41 Search PubMed.
- D.-Q. Chen, Y.-N. Wang, N. D. Vaziri, L. Chen, H.-H. Hu and Y.-Y. Zhao, Poricoic acid A activates AMPK to attenuate fibroblast activation and abnormal extracellular matrix remodelling in renal fibrosis, Phytomedicine, 2020, 72, 153232 CrossRef CAS PubMed.
- D.-Q. Chen, X.-Q. Wu, L. Chen, H.-H. Hu, Y.-N. Wang and Y.-Y. Zhao, Poricoic acid A as a modulator of TPH-1 expression inhibits renal fibrosis via modulating protein stability of β-catenin and β-catenin-mediated transcription, Ther. Adv. Chronic Dis., 2020, 11, 2040622320962648 CAS.
- M. Wang, D. Q. Chen, L. Chen, G. Cao, H. Zhao, D. Liu, N. D. Vaziri, Y. Guo and Y. Y. Zhao, Novel inhibitors of the cellular renin-angiotensin system components, poricoic acids, target Smad3 phosphorylation and Wnt/β-catenin pathway against renal fibrosis, Br. J. Pharmacol., 2018, 175(13), 2689–2708 CrossRef CAS PubMed.
- H. Dong, P. Wu, R. Yan, Q. Xu, H. Li, F. Zhang, J. Li and B. Yang, Enrichment and separation of antitumor triterpene acids from the epidermis of Poria cocos by pH-zone-refining counter-current chromatography and conventional high-speed counter-current chromatography, J. Sep. Sci., 2015, 38(11), 1977–1982 CrossRef CAS PubMed.
- M. Ukiya, T. Akihisa, H. Tokuda, M. Hirano, M. Oshikubo, Y. Nobukuni, Y. Kimura, T. Tai, S. Kondo and H. Nishino, Inhibition of tumor-promoting effects by poricoic acids G and H and other lanostane-type triterpenes and cytotoxic activity of poricoic acids A and G from Poria cocos, J. Nat. Prod., 2002, 65, 462–465 CrossRef CAS PubMed.
- X. Jia, L. Ma, P. Li, M. Chen and C. He, Prospects of Poria cocos polysaccharides: Isolation process, structural features and bioactivities, Trends Food Sci. Technol., 2016, 54, 52–62 CrossRef CAS.
- Y. Wen, B. Jia and T. Peng, Research on Chemical Constituents and Pharmacological Action of Polysaccharide in Poria cocos, Med. Plant, 2014, 5(06), 51–54 CAS.
- K. Jeong-Ah, Antioxidant and NF-κB inhibitory constituents isolated from Morchella esculenta, Nat. Prod. Res., 2011, 25(15), 1412–1417 CrossRef.
- J. Shuo, Z. Fan, Z. Huan, X. Jianhua, Y. Lanlan and S. Mingyue, Biological Activity and Application of Food-derived Sterol Components, Sci. Technol. Food Ind., 2019, 40(08), 310–316 Search PubMed.
- X. Xiaoxia, W. Hongbo, Z. Jingwen, Y. Yanting, H. Junjie, L. Hongwei, Z. Feng and F. Fenghua, Inhibitory Effect of Ergosteroids G10-10A, an Ergosteroids Compound, on Human Gastric Cancer MKN45 Cells in vitro, J. Yantai Univ., Nat. Sci. and Eng. Ed., 2017, 30(03), 207–213 Search PubMed.
- B. Zhou, X. Liang, Q. Feng, J. Li, X. Pan, P. Xie, Z. Jiang and Z. Yang, Ergosterol peroxide suppresses influenza A virus-induced pro-inflammatory response and apoptosis by blocking RIG-I signaling, Eur. J. Pharmacol., 2019, 860, 172543 CrossRef CAS.
- M. B. Alam, N. S. Chowdhury, M. H. Sohrab, M. S. Rana, C. M. Hasan and S.-H. Lee, Cerevisterol Alleviates Inflammation via Suppression of MAPK/NF-κB/AP-1 and Activation of the Nrf2/HO-1 Signaling Cascade, Biomolecules, 2020, 10, 199 CrossRef CAS PubMed.
- K. Budipramana, J. Junaidin, K. R. Wirasutisna, Y. B. Pramana and S. Sukrasno, An Integrated In Silico and In Vitro Assays of Dipeptidyl Peptidase-4 and α-Glucosidase Inhibition by Stellasterol from Ganoderma australe, Sci. Pharm., 2019, 87(3), 21 CrossRef CAS.
- A. Zumla, D. S. Hui, E. I. Azhar, Z. A. Memish and M. Maeurer, Reducing mortality from 2019-nCoV: host-directed therapies should be an option, Lancet, 2020, 395(10224), E35–E36 CrossRef CAS.
- Z. Chen, D. Zhang, M. Li and B. Wang, Costunolide ameliorates lipoteichoic acid-induced acute lung injury via attenuating MAPK signaling pathway, Int. Immunopharmacol., 2018, 61, 283–289 CrossRef CAS PubMed.
- Y. X. Lim, Y. L. Ng, J. P. Tam and D. X. Liu, Human Coronaviruses: A Review of Virus–Host
Interactions, Disease, 2016, 4, 26 CrossRef PubMed.
- L.-p. Zhang, Y. Zhao, G.-j. Liu, D.-g. Yang, Y.-h. Dong and L.-h. Zhou, Glabridin attenuates lipopolysaccharide-induced acute lung injury by inhibiting p38MAPK/ERK signaling pathway, Oncotarget, 2017, 8, 18935–18942 CrossRef PubMed.
- L. S. Azevedo, F. P. Moraes, M. M. Xavier, E. O. Pantoja, B. Villavicencio, J. A. Finck, A. M. Proenca, K. B. Rocha and W. F. d. Azevedo, Recent Progress of Molecular Docking Simulations Applied to Development of Drugs, Curr. Bioinf., 2012, 7(4), 352–365 CrossRef CAS.
Footnotes |
† Electronic supplementary information (ESI) available. See DOI: 10.1039/d0ra07035a |
‡ These two authors contributed equally to this paper. |
|
This journal is © The Royal Society of Chemistry 2021 |
Click here to see how this site uses Cookies. View our privacy policy here.