DOI:
10.1039/D0NJ04216A
(Paper)
New J. Chem., 2021,
45, 373-382
Structural basis for tailor-made selective PI3K α/β inhibitors: a computational perspective†
Received
21st August 2020
, Accepted 6th November 2020
First published on 10th November 2020
Abstract
PI3K α and β are Class IA PI3K isoforms that share a highly homologous ATP binding site, differing only in a few residues around the binding site. They are ubiquitously expressed in various organs and play many different roles in terms of mutations in carcinomas and signaling in tumor growth. Their pan-inhibitors should theoretically be effective against cancers by offering a potentially broader spectrum of activity, however, clinical development of most panPI3K inhibitors has discontinued probably owing to the problematic toxicities. Therefore, it is crucial to clarify the structural basis of the selective inhibition mechanism towards PI3K α and β. Towards this end, comprehensive computational approaches including molecular docking, molecular dynamics simulations, mutagenesis, and DFT technologies were applied to reveal the different interaction modes between highly selective PI3Kα and PI3Kβ inhibitors. It was found that the VAL851 of PI3Kα and VAL848 of PI3Kβ remarkably affected their selectivity by forming hydrogen bonds with different molecular scaffolds. Moreover, a diverse amino acid, GLN859 of PI3K α and ASP856 of PI3Kβ greatly contributed to distinguishing the inhibitory selectivity between PI3Kα and β. Of note, PI3Kβ seemed to form more water bridges with the surrounding water molecules. Collectively, these data shed light in depicting the PI3Kα/β selective mechanisms, which guided the future strategy for a rational design of selective inhibitors towards PI3Kα/β.
1 Introduction
The phosphatidylinositol 3-kinase (PI3K) pathway is involved in a panoply of cellular responses, including survival, proliferation, protein synthesis, migration, and vesicular trafficking.1 Due to its frequent dysregulation in human cancers, it has attracted immense interest as a therapeutic target for cancer treatment.2,3 Typically, the PI3K family is divided into four different classes: Class I, II, III, and IV based on the primary structure, regulation, and in vitro lipid substrate specificity.4 By far, class I PI3K is the most extensively characterized family that is further subdivided into IA (activated by receptor tyrosine kinases) and IB (activated by G protein coupled receptors). In fact, the IA family is the subfamily that is most implicated in human cancers.5–7
PI3K IA includes heterodimers comprising a p85 regulatory subunit and a catalytic p110 subunit designated as p110α, β, or δ.1,8,9 Theoretically, their pan-inhibitors should be effective against cancers by offering a potentially broader spectrum of activity; however, clinical development of most panPI3K inhibitors has been discontinued probably owing to the problematic toxicities. For example, due to the critical role of PI3KIα in insulin signaling, PI3KIα inhibition is a main contributor to non-insulin-dependent diabetes, and hyperglycemia is being seen with PI3K inhibition, particularly PI3KIα inhibition.10 Indeed, hyperglycemia has been seen with the intravenous injection of the α/δ inhibitor copanlisib and the oral administration of the pan-inhibitor buparlisib. Accordingly, current interest in drug discovery is shifting to isotype-specific inhibition of PI3K, a promising strategy to maintain clinical efficacy and improve tolerance with lower overall toxicity.11,12
Typically, the tissue distribution reveals the expected activity and toxicity connected with pharmacological inhibition of different PI3K isoforms. However, in terms of the three PI3K IA isoforms, both PI3Kα and PI3Kβ are ubiquitously expressed in various organs, although PI3Kδ is highly expressed in hemopoietic cells.13 In particular, like most kinases,14–16 PI3Kα and β isoforms share a highly homologous ATP binding site, differing only in a few residues around the binding site17,18 with overlapping but non-redundant physiological effects.19–21 For example, PI3Kα plays an important role in glucose homeostasis and insulin signaling,20,22 whereas PI3Kβ is shown to regulate the activity of platelet integrin αIIbβ3 in the case of platelet adhesion and aggregation.23–25 Moreover, in terms of mutations in carcinomas, PI3Kα is essential for signaling and growth of tumors driven by PIK3CA gene mutations and amplification, while PI3Kβ is the main isoform that mediates the occurrence of PTEN-deficient tumors.26,27 Therefore, it is crucial to clarify the structural basis of the selective inhibition mechanism towards PI3Kα and β. Towards this end, multiple computational approaches were applied to reveal the structural basis for selective inhibition towards PI3Kα and PI3Kβ via comparing the different interaction modes between PI3Kα and PI3Kβ with their highly selective inhibitor A66 (compound 1) and TGX221 (compound 2) (Fig. 1), shedding light on better access for designing and developing novel selective PI3Kα/β inhibitors.
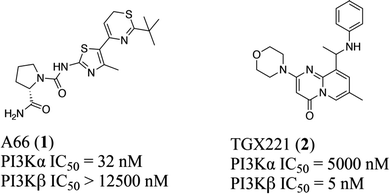 |
| Fig. 1 Structures of highly selective PI3Kα/β inhibitors. | |
2 Experimental section
2.1 Software
Various softwares including Schrödinger suite 2018-128 (Schrödinger, LLC, 2018), Gaussian 09W package,29 LigandScout 4.3,30 Discovery Studio program 3.0,31 PyMOL,32 and GraphPad Prism 8 were used in the study.
2.2 Protein and ligand preparation
The PI3K protein sequences were obtained from the UniprotKB database P42336 and P42338, respectively. Discovery Studio program was utilized for sequence alignment and PyMOL was employed for structural superposition. Crystal structures of PI3Kα (PDB code: 4JPS) and PI3Kβ (PDB code: 4BFR) were downloaded from the RCSB PDB database (http://www.rcsb.org/). The co-crystallized proteins were prepared in the Protein Preparation Wizard module in Maestro interface prior to docking in order to adjust the bond orders and the formal charges. Water molecules were removed, and hydrogen atoms were added to optimize the hydrogen bonds. Heteroatom ionization states were generated and optimized before minimizing with the OPLS_3e force field.33 All ligand structures were prepared using the LigPrep module in Schrödinger suite, and were protonated with the Epic module at pH 7.0 ± 2.0. The OPLS_3e force field was then employed to optimize the energy of the co-ligand and eliminate steric hindrance.
2.3 Molecular docking
The docking process was carried out using the XP (Extra-Precision) mode in the Glide module in Maestro 2018-1.34 Firstly, the docking grids were generated for two proteins using the Glide program. The co-ligands were defined as the centroid of the grid box with the slider set at 20 Å by default. The van der Waals radii of nonpolar receptor atoms in the grid box were set to 1.0, and the charge cutoff scaling factor was set to 0.25 Å using the OPLS_3e force field. Molecular docking validation was conducted by default settings with flexible ligand conformation generation. The co-ligands were re-docked into the grid box to reproduce the experimentally observed poses as well as to validate the suitability of the docking program by calculating the root-mean-square deviation (RMSD) values. Then, the PI3K inhibitors were docked with these validated parameters.35
2.4 Molecular dynamics simulations
The Desmond program was utilized to perform MD simulations on the complexes merged from the molecular docking.36 First of all, the solvent system was established using the SPC three-point explicit water solvent model. Herein, water molecules were placed at a minimum distance of 10 Å around the protein in each direction. In the meanwhile, 19 Na+ counterions were added to the 4JPS system, and 10 Na+ counterions were added to the 4BFR system to neutralize the system charge before minimizing the energy of the composite system under the OPLS_3e force field. The maximum number of iterations during the minimization process was set to 5000, and the convergence threshold was cut off at 1.0 kcal mol−1 Å−1. The Particle Mesh Ewald (PME) method was used to calculate the long-range electrostatic interaction with a gradient threshold of 25 kcal mol−1 Å−1 through the LBFGS algorithm. In addition, the time step was set to 100 ps, and the distance cut-off for long-range interaction was maintained at 10 Å. Also, no bonds were constrained during the simulation. The system adopted the Berendsen thermostat to increase the temperature from 0 to 300 K under 1.01325 bar within 100 ps. After a 2 ns system stabilization, a 100 ns generation phase of MD simulations was initiated, the energy was recorded every 1.0 ps and the orbital atomic coordinates were monitored every 100 ps. The statistical analysis of the data was performed, such as RMSD, root-mean-square fluctuation (RMSF), and ligand properties.
2.5 Binding free energy calculation
Molecular Mechanics/Generalized Born Surface Area (MM-GBSA) program in the Prime module was utilized to predict the binding modes and free energies for the protein–ligand complex.37 Herein, the implicit solvation method with the variable-dielectric generalized Born (VSGB) model, a widely used method for estimating the relative binding free energies was used. The force field utilized OPLS_3e force field, and the free energies were calculated according to the optimized protein–ligand complexes, wherein a total number of 100 frames were extracted from the last 10 ns of MD simulations. The calculation formula is as follows: | ΔGbind = Gcomplex − (Gprotein + Gligand) | (1) |
| ΔGbind = ΔEMM − TΔS + ΔGsol | (2) |
| ΔGbind = ΔEvdw + ΔEele + ΔGGB + ΔGSA − TΔS | (3) |
Herein, ΔGbind represents the total binding energy of the ligand–protein complex; ΔEMM, ΔGsol, and −TΔS indicate the sum of the total gas-phase energy, polar and nonpolar contributions to solvation, and conformational entropy, respectively. ΔEvdw and ΔEele represent the internal van der Waals and electrostatic energies under the molecular mechanics (MM) force field, respectively. The polar solvation energy was calculated using the Poisson Boltzmann equation and the generalized Born equation, while the non-polar solvation energy was calculated using a linear function of the solvent accessible surface area (SASA).
2.6 Pharmacophore modeling
The pharmacophore features were collected using LigandScout 4.338 according to the MD-generated protein–ligand complexes containing the functional groups that can interact with the receptor active centers through hydrogen bonds, electrostatic interactions, van der Waals forces, and hydrophobic bonds. All default parameters were utilized here.
2.7 DFT calculation
The density functional theory (DFT) method was carried out to optimize the ligand structures at the Becke–Lee–Yang–Parr (B3LYP) level of theory within a 6-31G* basis set using Gaussian 09W package.39 The frequency calculation that represents the ability of the molecules to participate in the interactions was performed, wherein the HOMO and LUMO energy gap values reflect the ability of electrons to transfer from the upper orbit to the empty orbit. The energies were calculated with the corresponding molecular orbitals and visualized using Multiwfn software.40
2.8 Alanine scanning mutagenesis
Alanine scanning mutagenesis (ASM) analysis, an appealing method to study the function of specific regions on protein surfaces was performed to further investigate the binding free energy decomposition analysis via Schrödinger.41 In brief, only the side chains outside of the β carbon structure were eliminated to find the functional role of amino acids. The changes of binding free energy ΔΔGbind were calculated according to formula (4) between the variant and wild-type protein while the specific residues were mutated to alanine. | ΔΔGbind = ΔGbindmutant − ΔGbind wide type | (4) |
3 Results and discussion
3.1 Structural comparison between PI3Kα and PI3Kβ
In order to explore the structural and functional relationship between PI3Kα and PI3Kβ, their amino acid sequences were aligned to determine the conserved and nonconservative regions. As there is no crystal structure released for human PI3Kβ, mouse PI3Kβ was used for modeling, which shares 95.86% sequence identity with human PI3Kβ (Fig. S1, ESI†). As revealed in Fig. S2 (ESI†), hPI3Kα and mPI3Kβ exhibit a sequence identity of 41.28%, and a sequence similarity of 72.47% (RMSD = 1.674 Å). Further structural superimposition of PI3Kα/β was performed to distinguish the similarities and differences of the three-dimensional structures. In fact, both PI3K isoforms shared common structural characteristics of kinases, including kinase catalytic domain, regulatory domain, localization domain, and other structurally diverse auxiliary domains. And their catalytic domain was connected by the C-lobe fragment and the N-lobe fragment through a hinge region that was conservative to maintain the catalytic function. As revealed in Fig. 2a, like most ATP-competitive kinase inhibitors, the ATP binding site of the endogenous substrate was located in the catalytic cliff containing important characteristic structures such as the gatekeeper residue, glycine-rich loop (G-loop), and activation loop (A-loop). Of note, the characteristic Asp-Phe-Gly motif, namely the DFG motif, was conserved at the end of the A-loop with its aspartic acid playing an important role in complexing divalent cations (Mg2+/Mn2+) during the catalysis process. In the meanwhile, the adenine loop of ATP underwent a key hydrophobic interaction with PI3K via forming a hydrogen bond with the hinge region. Indeed, there was extensive overlap between the key amino acids of PI3Kα and PI3Kβ. For example, the TRP780, ILE800, LYS802, TYR836, ILE848, SER854, GLU849, VAL851, and MET922 in PI3Kα were counterparts of TRP781, ILE797, LYS799, TYR833, ILE845, SER851, GLU846, VAL848, and MET920 in PI3Kβ (Fig. 2a). In addition, GLN859 in PI3Kα and ASN856 in PI3Kβ were a pair of different but similarly aligned amino acids. According to the sequence aligning result of the active regions between PI3Kα and β, their identity was up to 83.15% (Fig. 2b), revealing a high similarity between the catalytic subunits of PI3Kα and PI3Kβ.
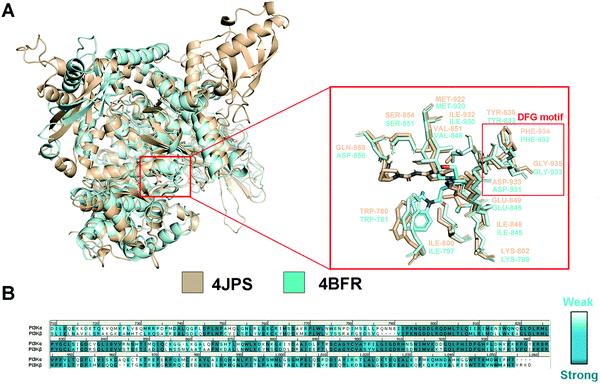 |
| Fig. 2 Structural comparison of PI3Kα and PI3Kβ. (A) 3D superimposition of crystal structures of human PI3Kα (PDB ID: 4JPS) and mouse PI3Kβ (PDB ID: 4BFR). (B) Sequence alignment between PI3Kα and PI3Kβ in the catalytic subunit. PI3Kα structure was displayed in wheat ribbon with its key residues shown in wheat sticks. The PI3Kβ structure is displayed in a pale cyan ribbon with its key residues shown in pale cyan sticks. | |
3.2 Binding patterns revealed from the molecular docking study
Molecular docking was utilized to predict the binding modes and evaluate the binding capacity of PI3K selective ligands. Typically, the co-crystal ligands of PI3Kα (PDB code 4JPS) and PI3Kβ (PDB code 4BFR) were extracted from the crystal complexes and re-docked into the binding pockets using Glide to compare the experimental and docked poses. As shown in Fig. S3 (ESI†), the generated RMSD value was 0.637 Å and 0.260 Å for 4JPS and 4BFR, respectively, suggesting that Glide is reliable to reproduce the binding modes observed in experimental crystal structures. Then, docking analysis was performed on the two PI3K ligands, wherein the docking scores were consistent with their biological activity tendency (Table 1). Moreover, it was observed from the molecular docking obtained binding modes that VAL851 and GLN859 were hydrogen bond donors and acceptors participating in hydrogen bond formation in PI3Kα, whereas only VAL848 participated in hydrogen bond formation in PI3Kβ. In the meanwhile, the hydrophobic effect played an important role in both PI3Kα and β. In PI3Kα, various amino acids took part in the hydrophobic effects, including PRO778, TRP780, ILE800, TYR836, VAL850, VAL851, MET922, PHE930, and ILE932, while in PI3Kβ, PRO779, TRP781, ILE797, TYR833, VAL847, VAL848, MET920, PHE928, and ILE930 contributed to the hydrophobic effects (Fig. 3). In addition to the hydrophobic effects, π–π stacking interactions and hydrogen bonding also contributed to the binding. For example, TYR836 in PI3Kα, and TRP781 in PI3Kβ were involved in the formation of the π–π stacking interactions, and the selective PI3Kα inhibitor Compd1 tended to form strong hydrogen bonds with VAL851 through its heterocyclic nitrogen atoms, while the selective PI3Kβ inhibitor Compd2 formed a strong hydrogen bond with VAL848 via the oxygen atom of its morpholine ring (Fig. 3). The interactions of each compound at the binding pocket are summarized in Table S1 and compared in Fig. S4 (ESI†).
Table 1 Glide docking scores of Compd 1 and 2 against PI3Kα/β
Entry |
IC50 (nM) |
XP (kcal mol−1) |
Glide (kcal mol−1) |
Glide (kcal mol−1) |
PI3Kα/Compd1 |
32 |
−11.44 |
−57.48 |
−90.60 |
PI3Kα/Compd2 |
5000 |
−4.92 |
−38.02 |
−48.07 |
PI3Kβ/Compd1 |
>12 500 |
−5.22 |
−38.40 |
−39.65 |
PI3Kβ/Compd2 |
5 |
−10.04 |
−53.30 |
−77.99 |
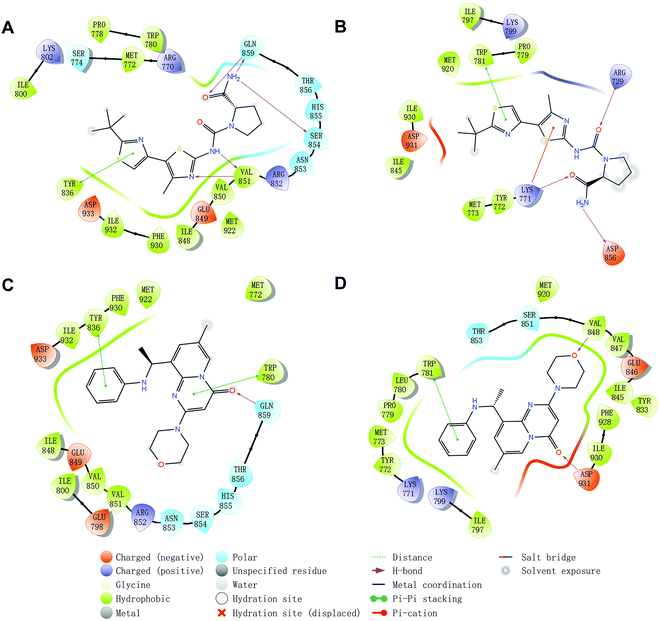 |
| Fig. 3 Predicted binding patterns of PI3Kα/β inhibitors. (A) PI3Kα/Compd1. (B) PI3Kβ/Compd1. (C) PI3Kα/Compd2. (D) PI3Kβ/Compd2. | |
3.3 Molecular dynamics simulations
3.3.1 Stability of dynamics trajectory from RMSD analysis.
Molecular dynamics simulation is an effective method to further verify the interaction between proteins and ligands, thus the docked compound–protein complexes were initiated for 100 ns MD simulation to evaluate the binding stability of the protein–ligand complex. Herein, RMSD, the sum of all atomic deviations of the conformations was employed to detect the relative stability of the PI3K complexes during the simulation process. As an important indicator for evaluating protein stability, an average change of 1 to 3 Å is an acceptable range for RMSD. As shown in Fig. 4A, all systems reached equilibrium after 50 ns with an average RMSD value under 3.5 Å, wherein both apo PI3Kα and PI3Kα/Compd1 complex reached a steady state after a short period of 10 ns, stabilizing at 2.4 Å and 3.5 Å, respectively, and the PI3Kα/Compd2 complex reached a stable state after experiencing a wide range of fluctuations in the first 40 ns of the simulated trajectory, and finally stabilized at 3.1 Å. In terms of the PI3Kβ system, the apo PI3Kβ experienced a wide range of fluctuations in the first 20 ns and eventually stabilized at around 2.5 Å. A similar process was observed in the PI3Kβ/Compd2 complex that eventually stabilized at 2.8 Å. The final RMSD value for PI3Kβ/Compd1 was around 2.5 Å after 30 ns simulation trajectory (Fig. 4B). Together, these data confirmed that the conformations of the complexes all reached a steady state during the dynamic simulations.
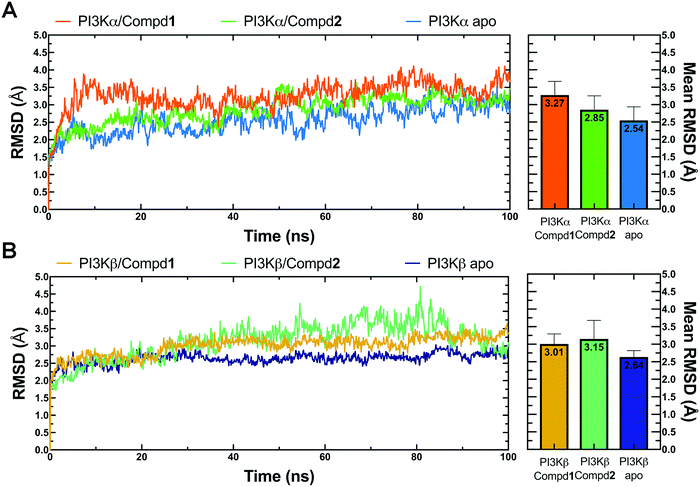 |
| Fig. 4 RMSD plots of MD simulations. (A) RMSD line chart and bar chart of PI3Kα. (B) RMSD line chart and bar chart of PI3Kβ. The average value was labeled on the top of each bar. Error bar indicated the highest value of RMSD during the simulations. | |
3.3.2 Structural flexibility from RMSF analysis.
During the MD simulations, the RMSF value is another useful index that particularly reflects the flexibility of the backbone carbon atoms in PI3K complexes. As reviewed in Fig. 5, all systems exhibited similar RMSF dynamic profiles, indicating that the small molecules exhibited similar binding patterns within PI3Ks pockets. Notably, the RMSF curve in the loop region fluctuated greatly, reflecting the flexibility of each loop region of the protein. Of note, some important amino acids such as VAL851 in PI3Kα, as well as SER854 and VAL848 in PI3Kβ barely represented any RMSF fluctuations, pointing that these residues are located at the binding sites and participated in intermolecular interactions. In terms of PI3Kα/Compd1, the amino acids that exhibited the least fluctuations within the active pocket seemed to be responsible for the formation of stable interactions (Fig. 5A). Moreover, residues in apo PI3Kα fluctuated more sharply than those in the complex, suggesting that the antagonists formed strong interactions with PI3Kα and thus stabilized the protein conformation (Fig. 5A). Similarly, Compd2 displayed a potent effect on stabilizing PI3Kβ as revealed by the observation that residues in PI3Kβ/Compd2 fluctuated more smoothly compared to that of apo PI3Kβ (Fig. 5B). Together, these data supported the previous molecular docking results.
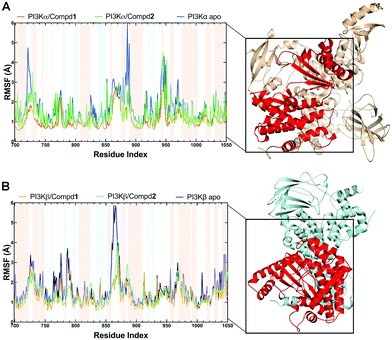 |
| Fig. 5 RMSF plots of MD simulations. (A) RMSF chart of the PI3Kα structure during the MD simulation. (B) RMSF chart of PI3Kβ structure during the MD simulation. Backgrounds in blue indicated residues in the β-sheet secondary structure and red ones indicated residues in the α-helix secondary structure. | |
3.4 Intermolecular interaction analysis by MD simulation
In order to compare and elucidate the specific interaction mode differences between the specific inhibitor and its target, the intermolecular interaction analysis of Compd1 (the specific inhibitor of PI3Kα) towards PI3Kα and PI3Kβ was first performed. As shown in Fig. 6A and 7A, ILE932 and ILE800 in the PI3Kα/Compd1 complex kept forming a water bridge for 58% and 57% of the whole MD simulation period, respectively. Of note, with regard to the PI3Kα/Compd1 complex, VAL851 of PI3Kα not only formed a hydrogen bond with the nitrogen atom of the thiazole ring in Compd1 for up to 90% of the whole monitoring period, but also formed a hydrogen bond with the amide nitrogen atom for about 87% of the period. Moreover, the primary amino group of Compd1 acted as a hydrogen bond donor for SER854 (for 81% of the whole period), though GLN859 seemed to participate less in hydrogen bond formation compared with the docked pose. Similarly, it was observed that ILE930 of the PI3Kβ exerted a strong hydrophobic effect on Compd1 (Fig. 6B and 7B), and ASP856 (for 78% of the whole period) acted as a hydrogen bond acceptor to form a hydrogen bond with Compd1. Impressively, extensive water bridges were observed in the PI3Kβ/Compd1 complex involving ASP856 (34%), ASP774 (38%), GLU852 (37%), and THR853 (33%).
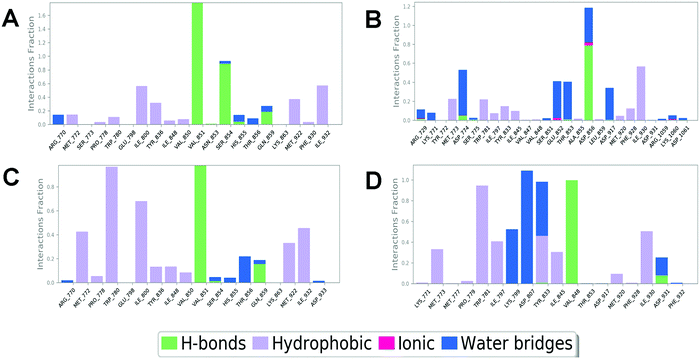 |
| Fig. 6 Interaction fractions of protein–ligand interactions obtained through the MD trajectory for complexes of PI3Kα/Compd1 (A), PI3Kβ/Compd1 (B), PI3Kα/Compd2 (C), and PI3Kβ/Compd2 (D). | |
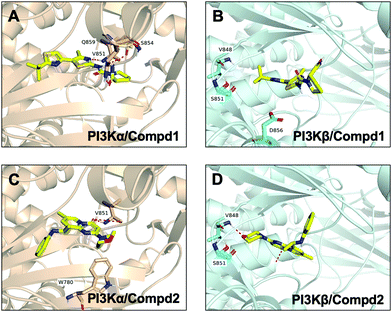 |
| Fig. 7 Snapshot of molecular interactions captured in the last frames from the 100 ns MD simulations for complexes PI3Kα/Compd1 (A), PI3Kβ/Compd1 (B), PI3Kα/Compd2 (C), and PI3Kβ/Compd2 (D). Herein, 4JPS was shown in wheat and 4BFR was shown in pale cyan. Images that depicted the proposed binding modes were generated using PyMOL software. | |
In terms of the PI3Kβ/Compd2 complex, various residues were observed to form hydrophobic interactions with Compd2 including MET773 (36%), ILE797 (41%), ILE845 (34%), TRP781 (96%), TYR833 (44%), and ILE930 (53%) (Fig. 6D and 7D). Moreover, the benzene ring of Compd2 formed a π–π stacking effect with TRP781 (91%), in the meanwhile, the morpholine ring formed a hydrogen bond with VAL848 (99%). In addition, ASP807 (108%), LYS799 (52%), and TYR833 (51%) were found to form water bridges with the oxygen atom connected to the pyrimidine ring in the PI3Kβ/Compd2 complex. In contrast, not only TRP780 (98%), ILE800 (79%), MET772 (42%), MET922 (34%), and ILE932 (46%) participated in hydrophobic interaction in the PI3Kα/Compd2 complex, but TRP780 (77%) also formed a π–π stacking effect with the pyrimidine ring of Compd2 (Fig. 6C and 7C). In addition, the carbonyl oxygen atom of Compd2 formed the hydrogen bond with VAL851 (97%) in PI3Kα (Fig. 6D). Moreover, the detailed MD results of each complex are appended in Fig. S5–S8 (ESI†), including the schematic of detailed ligand–atom interactions as well as the timeline representation of the interactions and contacts. Clearly, both VAL851 and SER854 of PI3Kα mainly participated in the intermolecular interaction throughout the 100 ns MD simulation process in the PI3Kα/Compd1 complex, while both TRP781, ASP807, and VAL848 of PI3Kβ mainly formed the intermolecular interaction during the 100ns MD simulation process in the PI3Kβ/Compd2 complex. Furthermore, the ligand torsion profile of four complexes is shown in Fig. S9 (ESI†), wherein Compd1 has more rotatable bonds, indicating that Compd1 was more flexible than Compd2. Besides, various ligand properties were envisaged in the MD process, including ligand RMSD, radius of gyration (rGyr), intramolecular hydrogen bonds (intraHB), molecular surface area (MolSA), SASA, and polar surface area (PSA) (Fig. S10, ESI†). Indeed, all these parameters of the PI3Kα/Compd1 complex fluctuated less than those of the PI3Kα/Compd2 complex, supporting that Compd1 formed more stable intermolecular interactions with PI3Kα, which sets a stage for elucidating the mechanism of selectivity between PI3Kα and β. As revealed in Fig. 7, different molecular scaffolds formed hydrogen bonds with VAL851 of PI3Kα but VAL848 of PI3Kβ which greatly affected the binding selectivity of the substrate. In addition, SER854 of PI3Kα formed stable hydrogen bonds with its inhibitor (Fig. 6A and C). In contrast, TRP781 of PI3Kβ formed π–π stacking effects (Fig. 6B and D). In particular, PI3Kβ formed more water bridges, indicating that selective PI3Kβ inhibitors may appeal to the hydrogen bonding interactions mediated by water molecules. Collectively, in line with the molecular docking results, MD simulation data confirmed that various important amino acids, such as VAL851 and SER854 of PI3Kα, and VAL848 of PI3Kβ served to maintain stable interactions with small molecules during the MD simulation.
3.5 Binding free energy calculation
In order to evaluate the binding affinity of the ligands towards PI3K isoforms, the \MM-GBSA method was employed to calculate the binding free energy, such as ΔG_Bind, ΔG_Bind_Coulomb, ΔG_Bind_Hbond, ΔG_Bind_Lipo, and ΔG_Bind_vdW. Herein, the 100 frames extracted from the last 10ns during the total 100 ns MD process were selected for calculation. As shown in Fig. 8, the ΔG_Bind values of PI3Kα/Compd1 (−70.77 kcal mol−1) and PI3Kβ/Compd1 (−30.87 kcal mol−1) were consistent with their corresponding experimentally determined affinity in the qualitative analysis. Consistent with the MD data in Fig. 8, Compd1 displayed a relatively tighter binding affinity for PI3Kα compared to PI3Kβ (Table S2, ESI†), with Coulomb potential (ΔG_Bind_Coulomb = −26.16 kcal mol−1) and van der Waals force (ΔG_Bind_vdW = −53.52 kcal mol−1) being the main contributors. By contrast, in line with the experimental data, Compd2 exhibited a better ΔG_Bind value of −39.46 kcal mol−1 towards PI3Kα compared to that of −52.45 kcal mol−1 towards PI3Kβ, which further supported that Compd2 selectively inhibited PI3Kβ. Therefore, the binding free energy data confirmed that the junctions ΔG_Bind_Coulomb and ΔG_Bind_vdW were the main contributors to the entire binding free energy.
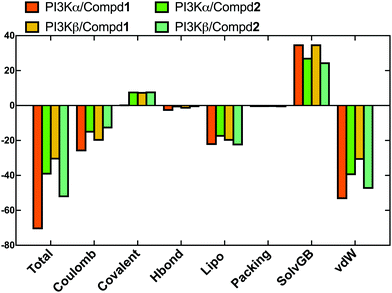 |
| Fig. 8 The sum of the contributions to the binding energy between protein–ligand complexes determined by MM/GBSA calculations. | |
3.6 Pharmacophore features for selective inhibition of PI3Kα/β
In order to elucidate the pharmacophore features between PI3Kα and PI3Kβ inhibitors, pharmacophore analysis was performed. As shown in Fig. 9, TYR836, PHE930, and ILE932 constituted a hydrophobic environment in the PI3Kα/Compd1 complex (Fig. 9A). Consistently, the nitrogen atom in the thiazole ring of Compd1 formed a hydrogen bond with VAL851 serving as a hydrogen bond acceptor. In the meanwhile, VAL851 was also observed to form a hydrogen bond with Compd1 as a hydrogen bond acceptor, wherein the primary amino group of Compd1 acted as a hydrogen bond donor to SER854 and GLN859 of PI3Kα. With regard to the PI3Kβ/Compd2 complex, a hydrophobic system was observed consisting of MET773, ILE797, THR853, TRP781, and ILE930. Besides, VAL848 contributed to the hydrogen bonding with the morpholine ring, and TYR833 participated in the hydrogen bonding between the double-bonded oxygen atoms (Fig. 9D). These results suggested that regarding the PI3Kα selective inhibitor, the amino group was the essential hydrogen donor to form the hydrogen bond with VAL851, SER854, and GLN859 of PI3Kα, while for PI3Kβ selective inhibitor, both the morpholine ring and the carbonyl group were pivotal hydrogen bond acceptor for VAL848 and TYR833 of PI3Kβ.
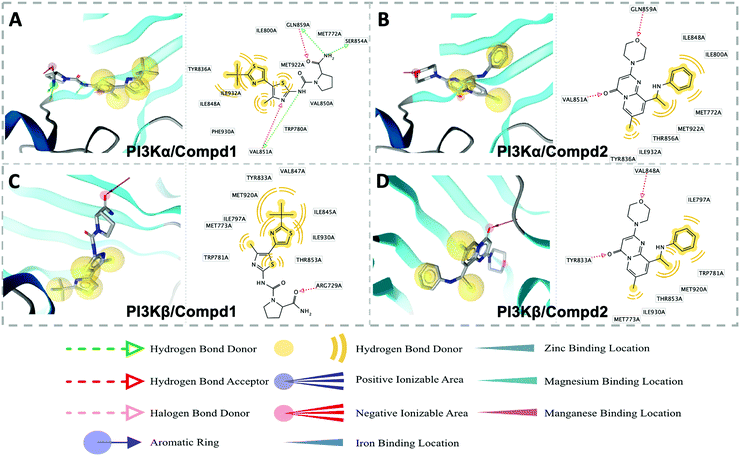 |
| Fig. 9 Structure-based pharmacophore models for PI3Kα/Compd1 (A), PI3Kα/Compd2 (B), PI3Kβ/Compd1 (C), and PI3Kβ/Compd2 (D). Hydrophobic and hydrogen bond acceptor interactions are depicted as yellow spheres and red arrows, respectively. | |
3.7 DFT calculations
To further elucidate the potential π–π interactions involved in the system, both the highest occupied molecular orbital (HOMO) and lowest unoccupied molecular orbital (LUMO), two important indicators of chemical reactivity and stability of receptor–ligand complexes were further calculated. As revealed in Fig. 10, the LUMO and HOMO orbitals of Compd1 are mainly located at the largest aromatic ring system, however, the LUMO and HOMO orbitals of Compd2 located at the [1,2-a]pyrimidin-5-ium area. Consistently, the primary amino group of Compd1 and the carbonyl group of Compd2 were observed to affect the reactivity, which provided valuable theoretical references for the future design of tailor-made selective PI3K α/β inhibitors.
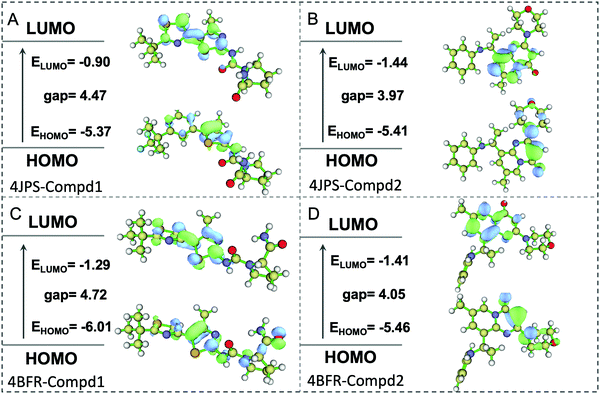 |
| Fig. 10 The HOMO and LUMO analysis via DFT calculations. (A) The HOMO and LUMO analysis of PI3Kα/Compd1. (B) The HOMO and LUMO analysis of PI3Kα/Compd2. (C) The HOMO and LUMO analysis of PI3Kβ/Compd1. (D) The HOMO and LUMO analysis of PI3Kβ/Compd2. The corresponding energy values and energy gap were represented in eV mol−1. | |
3.8 Alanine scanning mutagenesis
Alanine scanning mutagenesis technology was further employed to verify the explored binding patterns. As reflected in Fig. 11A, the PI3Kα/Compd1 system became unstable when ILE932, TRP780, GLN859, and VAL851 of PI3Kα were mutated to alanine, confirming that these amino acid side chains were crucial for protein–ligand binding. Alternatively, the mutation of SER854 showed less influence on the system, suggesting that it mainly worked on the backbone in affecting the selectivity. The mutation of GLN859 in PI3Kα exhibited more influence than the mutation of ASP856 in PI3Kβ because glutamine has a longer side chain than asparagine, which has a greater impact on the system.
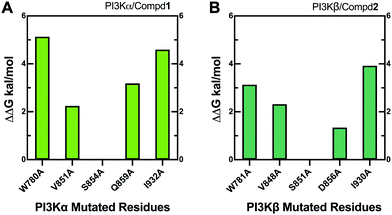 |
| Fig. 11 Alanine scanning mutagenesis analysis of PI3Kα/Compd1 (A) and PI3Kβ/Compd2 (B) complexes. | |
4 Conclusion
Although PI3Kα/β isoforms show only tiny structural differences, they exhibit obvious clinical distinctions due to their different pharmacological profiles. The key characteristic binding patterns towards their specific inhibitor were comprehensively explored through combinational applications of molecular docking, molecular dynamics simulations, mutagenesis, and DFT technologies. It was found that the VAL851 of PI3Kα and VAL848 of PI3Kβ remarkably affected their selectivity by forming hydrogen bonds with different molecular scaffolds. Moreover, a diverse amino acid, GLN859 of PI3K α and ASP856 of PI3Kβ greatly contributed to distinguishing the inhibitory selectivity between PI3K α and β. Of note, PI3Kβ seemed to form more water bridges with the surrounding water molecules. Collectively, these data shed promising light in depicting the PI3K α/β selective mechanisms, which guided the future strategy for a rational design of selective inhibitors towards PI3Kα/β.
Abbreviations
A-loop | Activation loop |
ASM | Alanine scanning mutagenesis |
B3LYP | Becke–Lee–Yang–Parr |
DFG motif | Asp-Phe-Gly motif |
DFT | Density functional theory |
G-loop | Gycine-rich loop |
HOMO | Highest occupied molecular orbital |
intraHB) | Intramolecular hydrogen bonds |
LUMO | Lowest unoccupied molecular orbital |
MD | Molecular dynamics |
MM | Molecular mechanics |
MM-GBSA | Molecular mechanics/generalized Born surface area |
MolSA | Molecular surface area |
PI3K | Phosphatidylinositol 3-kinase |
PME | Particle mesh ewald |
PSA | polar surface area |
rGyr | Radius of gyration |
RMSD | Root-mean-square deviation |
RMSF | Root-mean-square fluctuation |
SASA | Solvent accessible surface area |
XP | Extra-precision |
Conflicts of interest
There are no conflicts of interest to declare.
Acknowledgements
This work was financially supported by the Natural Science Foundation of Liaoning Province (2019-ZD-0455), the Program for Innovative Talents of Higher Education of Liaoning (2012520005), and the Virtual educational center of Liaoning Province.
Notes and references
- L. C. Cantley, Science, 2002, 296, 1655–1657 CrossRef CAS
.
- D. A. Fruman, H. Chiu, B. D. Hopkins, S. Bagrodia, L. C. Cantley and R. T. Abraham, Cell, 2017, 170, 605–635 CrossRef CAS
.
- T. Aoyagi and T. Matsui, Curr. Pharm. Des., 2011, 17, 1818–1824 CrossRef CAS
.
- S. J. Leevers, B. Vanhaesebroeck and M. D. Waterfield, Curr. Opin. Cell Biol., 1999, 11, 219–225 CrossRef CAS
.
- J. A. Fresno Vara, E. Casado, J. de Castro, P. Cejas, C. Belda-Iniesta and M. Gonzalez-Baron, Cancer Treat. Rev., 2004, 30, 193–204 CrossRef
.
- T. L. Yuan and L. C. Cantley, Oncogene, 2008, 27, 5497–5510 CrossRef CAS
.
- I. Vivanco and C. L. Sawyers, Nat. Rev. Cancer, 2002, 2, 489–501 CrossRef CAS
.
- M. K. Rathinaswamy and J. E. Burke, Adv. Biol. Regul., 2020, 75, 100657 CrossRef CAS
.
- J. M. Backer, Curr. Top. Microbiol. Immunol., 2010, 346, 87–114 CAS
.
- I. B. Greenwell, C. R. Flowers, K. A. Blum and J. B. Cohen, Expert Rev. Anticancer Ther., 2017, 17, 271–279 CrossRef CAS
.
- E. Ciraolo, F. Morello and E. Hirsch, Curr. Med. Chem., 2011, 18, 2674–2685 CrossRef CAS
.
- T. J. Sundstrom, A. C. Anderson and D. L. Wright, Org. Biomol. Chem., 2009, 7, 840–850 RSC
.
- S. P. Jackson and S. M. Schoenwaelder, J. Thromb. Haemostasis, 2012, 10, 2123–2126 CrossRef CAS
.
- F. M. Ferguson and N. S. Gray, Nat. Rev. Drug Discovery, 2018, 17, 353–377 CrossRef CAS
.
- S. Muller, A. Chaikuad, N. S. Gray and S. Knapp, Nat. Chem. Biol., 2015, 11, 818–821 CrossRef CAS
.
- S. Kang, A. Denley, B. Vanhaesebroeck and P. K. Vogt, Proc. Natl. Acad. Sci. U. S. A., 2006, 103, 1289–1294 CrossRef CAS
.
- C. Costa and E. Hirsch, Curr. Top. Microbiol. Immunol., 2010, 346, 171–181 CAS
.
- J. Y. Lee, Y. H. Chiu, J. Asara and L. C. Cantley, Proc. Natl. Acad. Sci. U. S. A., 2011, 108, 14157–14162 CrossRef CAS
.
- L. M. Thorpe, H. Yuzugullu and J. J. Zhao, Nat. Rev. Cancer, 2015, 15, 7–24 CrossRef CAS
.
- Z. A. Knight, B. Gonzalez, M. E. Feldman, E. R. Zunder, D. D. Goldenberg, O. Williams, R. Loewith, D. Stokoe, A. Balla, B. Toth, T. Balla, W. A. Weiss, R. L. Williams and K. M. Shokat, Cell, 2006, 125, 733–747 CrossRef CAS
.
- F. M. Elmenier, D. S. Lasheen and K. A. M. Abouzid, Eur. J. Med. Chem., 2019, 183, 111718 CrossRef CAS
.
- V. R. Sopasakis, P. Liu, R. Suzuki, T. Kondo, J. Winnay, T. T. Tran, T. Asano, G. Smyth, M. P. Sajan, R. V. Farese, C. R. Kahn and J. J. Zhao, Cell Metab., 2010, 11, 220–230 CrossRef CAS
.
- S. P. Jackson, S. M. Schoenwaelder, I. Goncalves, W. S. Nesbitt, C. L. Yap, C. E. Wright, V. Kenche, K. E. Anderson, S. M. Dopheide, Y. Yuan, S. A. Sturgeon, H. Prabaharan, P. E. Thompson, G. D. Smith, P. R. Shepherd, N. Daniele, S. Kulkarni, B. Abbott, D. Saylik, C. Jones, L. Lu, S. Giuliano, S. C. Hughan, J. A. Angus, A. D. Robertson and H. H. Salem, Nat. Med., 2005, 11, 507–514 CrossRef CAS
.
- S. Nylander, B. Kull, J. A. Bjorkman, J. C. Ulvinge, N. Oakes, B. M. Emanuelsson, M. Andersson, T. Skarby, T. Inghardt, O. Fjellstrom and D. Gustafsson, J. Thromb. Haemostasis, 2012, 10, 2127–2136 CrossRef CAS
.
- S. M. Schoenwaelder, A. Ono, W. S. Nesbitt, J. Lim, K. Jarman and S. P. Jackson, J. Biol. Chem., 2010, 285, 2886–2896 CrossRef CAS
.
- N. Ilic and T. M. Roberts, Curr. Top. Microbiol. Immunol., 2010, 347, 55–77 CAS
.
- P. Singh, M. S. Dar and M. J. Dar, FEBS Lett., 2016, 590, 3071–3082 CrossRef CAS
.
- F. Fratev, T. Steinbrecher and S. O. Jonsdottir, ACS Omega, 2018, 3, 4357–4371 CrossRef CAS
.
- E. M. Zayed, M. A. Zayed, H. A. Abd El Salam and M. A. Noamaan, Comput. Biol. Chem., 2019, 78, 260–272 CrossRef CAS
.
- M. D. Donato, B. Righino, F. Filippetti, A. Battaglia, M. Petrillo, D. Pirolli, G. Scambia, M. C. D. Rosa and D. Gallo, Sci. Rep., 2018, 8, 16047 CrossRef
.
- S. Karunagaran, R. Kavitha, M. Vadivelu, K. W. Lee and C. Meganathan, Curr. Comput. – Aided Drug Des., 2017, 13, 275–293 CAS
.
- O. A. Pemberton, X. Zhang and Y. Chen, J. Med. Chem., 2017, 60, 3525–3530 CrossRef CAS
.
- E. Harder, W. Damm, J. Maple, C. Wu, M. Reboul, J. Y. Xiang, L. Wang, D. Lupyan, M. K. Dahlgren, J. L. Knight, J. W. Kaus, D. S. Cerutti, G. Krilov, W. L. Jorgensen, R. Abel and R. A. Friesner, J. Chem. Theory Comput., 2016, 12, 281–296 CrossRef CAS
.
- V. Wagner, L. Jantz, H. Briem, K. Sommer, M. Rarey and C. D. Christ, ChemMedChem, 2017, 12, 1866–1872 CrossRef CAS
.
- H. Wang, Z. Gao, P. Song, B. Hu, J. Wang and M. Cheng, Phys. Chem. Chem. Phys., 2019, 21, 24147–24164 RSC
.
- Y. Wang, X. He, C. Li, Y. Ma, W. Xue, B. Hu, J. Wang, T. Zhang and F. Zhang, Eur. J. Med. Chem., 2020, 193, 112235 CrossRef CAS
.
- F. Fogolari, A. Corazza and G. Esposito, Front. Mol. Biosci., 2018, 5, 11 CrossRef
.
- M. A. Alamri and M. A. Alamri, Bioinformation, 2019, 15, 586–595 CrossRef
.
-
G. W. T. M. J. Frisch, H. B. Schlegel, G. E. Scuseria, M. A. Robb, J. R. Cheeseman, G. Scalmani, V. Barone, G. A. Petersson, H. Nakatsuji, X. Li, M. Caricato, A. Marenich, J. Bloino, B. G. Janesko, R. Gomperts, B. Mennucci, H. P. Hratchian, J. V. Ortiz, A. F. Izmaylov, J. L. Sonnenberg, D. Williams-Young, F. Ding, F. Lipparini, F. Egidi, J. Goings, B. Peng, A. Petrone, T. Henderson, D. Ranasinghe, V. G. Zakrzewski, J. Gao, N. Rega, G. Zheng, W. Liang, M. Hada, M. Ehara, K. Toyota, R. Fukuda, J. Hasegawa, M. Ishida, T. Nakajima, Y. Honda, O. Kitao, H. Nakai, T. Vreven, K. Throssell, J. A. Montgomery, Jr, J. E. Peralta, F. Ogliaro, M. Bearpark, J. J. Heyd, E. Brothers, K. N. Kudin, V. N. Staroverov, T. Keith, R. Kobayashi, J. Normand, K. Raghavachari, A. Rendell, J. C. Burant, S. S. Iyengar, J. Tomasi, M. Cossi, J. M. Millam, M. Klene, C. Adamo, R. Cammi, J. W. Ochterski, R. L. Martin, K. Morokuma, O. Farkas, J. B. Foresman and D. J. Fox, Gaussian Manual, Gaussian, Inc., Wallingford CT, USA, 2009 Search PubMed
.
- T. Lu and F. Chen, J. Comput. Chem., 2012, 33, 580–592 CrossRef CAS
.
- L. Boukharta, H. Gutierrez-de-Teran and J. Aqvist, PLoS Comput. Biol., 2014, 10, e1003585 CrossRef
.
Footnotes |
† Electronic supplementary information (ESI) available. See DOI: 10.1039/d0nj04216a |
‡ The authors contributed equally to this work. |
|
This journal is © The Royal Society of Chemistry and the Centre National de la Recherche Scientifique 2021 |
Click here to see how this site uses Cookies. View our privacy policy here.