DOI:
10.1039/D1CY00922B
(Minireview)
Catal. Sci. Technol., 2021,
11, 6601-6629
Mechanistic and multiscale aspects of thermo-catalytic CO2 conversion to C1 products
Received
23rd May 2021
, Accepted 26th August 2021
First published on 30th September 2021
Abstract
The increasing environmental concerns due to anthropogenic CO2 emissions have called for an alternate sustainable source to fulfill rising chemical and energy demands and reduce environmental problems. The thermo-catalytic activation and conversion of abundantly available CO2, a thermodynamically stable and kinetically inert molecule, can significantly pave the way to sustainably produce chemicals and fuels and mitigate the additional CO2 load. This can be done through comprehensive knowledge and understanding of catalyst behavior, reaction kinetics, and reactor design. This review aims to catalog and summarize the advances in the experimental and theoretical approaches for CO2 activation and conversion to C1 products via heterogeneous catalytic routes. To this aim, we analyze the current literature works describing experimental analyses (e.g., catalyst characterization and kinetics measurement) as well as computational studies (e.g., microkinetic modeling and first-principles calculations). The catalytic reactions of CO2 activation and conversion reviewed in detail are: (i) reverse water-gas shift (RWGS), (ii) CO2 methanation, (iii) CO2 hydrogenation to methanol, and (iv) dry reforming of methane (DRM). This review is divided into six sections. The first section provides an overview of the energy and environmental problems of our society, in which promising strategies and possible pathways to utilize anthropogenic CO2 are highlighted. In the second section, the discussion follows with the description of materials and mechanisms of the available thermo-catalytic processes for CO2 utilization. In the third section, the process of catalyst deactivation by coking is presented, and possible solutions to the problem are recommended based on experimental and theoretical literature works. In the fourth section, kinetic models are reviewed. In the fifth section, reaction technologies associated with the conversion of CO2 are described, and, finally, in the sixth section, concluding remarks and future directions are provided.
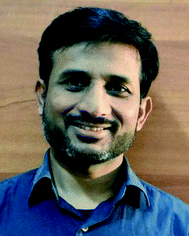 Md. Imteyaz Alam | Md. Imteyaz Alam obtained his master's and Ph. D. degree in Chemistry from the University of Delhi, India in 2009 and 2015, respectively. After a brief research experience at SC Johnson Products Pvt. Ltd. (Delhi), Indian Agricultural Research Institute (Delhi) and Indian Institute of technology Delhi (2014–2019), he joined Prof. Matteo Maestri's group in 2019 as a Marie Sklodowska-Curie (MSCA) Postdoctoral fellow at Politecnico di Milano (Italy). His research interests are focused on heterogeneous catalysis and sustainable chemistry to key energy conversion technologies including biomass transformation and CO2 conversion for fuels, chemicals and materials. |
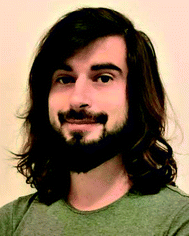 Raffaele Cheula | Raffaele Cheula is a postdoctoral fellow at Politecnico di Milano. He has been a member of Prof. Matteo Maestri's group since 2016. He obtained his PhD in Chemical Engineering and Industrial Chemistry from Politecnico di Milano in 2020. His work focuses on the development of a multiscale and multidisciplinary approach in the analysis and simulation of heterogeneous catalytic processes, focusing especially on microkinetic modeling based on density functional theory calculations. He developed and applied a framework for the concerted simulation of surface reactions and morphological modifications of catalyst materials inside chemical reactors. His primary interests are related to the modeling of catalytic processes for the interpretation of the experimental evidence and the discovery and engineering of catalytic materials. |
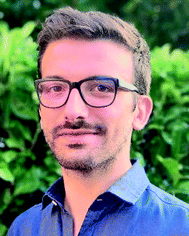 Gianluca Moroni | Gianluca Moroni has been a Ph.D. student in Chemical Engineering and Industrial Chemistry at Politecnico di Milano since 2017. He received his Master's degree in Chemical Engineering in 2015 from Politecnico di Milano with a thesis on the study of the support structure effect on the DeNOx activity of SCR Cu-based catalysts. He is mainly interested in heterogeneous catalysis and structure-dependent microkinetic analysis of catalytic processes. As a Ph.D. student, he has been a member of Prof. Matteo Maestri's group since November 2017. His main work focuses on the investigation of metal-based catalyst deactivation in methane steam and dry reforming reactions with operando spectroscopic techniques. |
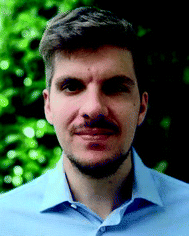 Luca Nardi | Luca Nardi has been a Ph.D. student in Chemical Engineering and Industrial Chemistry at Politecnico di Milano since November 2019 in Prof. Matteo Maestri's group. He received his bachelor's degree and master's degree in Chemical Engineering from Politecnico di Milano in 2017 and 2019, respectively. His research is focused on heterogeneous catalysis and structure-dependent microkinetic analysis of CO2 transformation into valuable products on metal-based catalysts. |
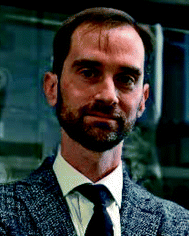 Matteo Maestri | Matteo Maestri is a full professor of Chemical Engineering at Politecnico di Milano, Italy. He received his Ph.D. in Chemical Engineering from Politecnico di Milano in 2008. He was a visiting scholar at the Center for Catalytic Science and Technology of the Department of Chemical Engineering of the University of Delaware, USA (2006–2007); Alexander von Humboldt Fellow at the Fritz-Haber-Institut der Max-Planck-Gesellschaft in Berlin, Germany (2009–2010); and at the Catalysis Research Center (CRC)–Department of Chemistry of the Technische Universität München, Germany (2011). He has received several awards, including the ERC Starting Grant from the European Research Council. His main research interests are fundamental analysis of catalytic kinetics and multiscale modeling of catalytic processes, by applying and developing methods that span from atomistic (DFT) calculations to CFD, and from kinetic analysis to operando-spectroscopy. |
1. Introduction
Energy and materials are key requirements of our society and economy. 84% of the primary energy consumed in 2020 was provided by fossil fuels.1 Long geological processes (106–108 years) brought about the formation of coal and hydrocarbons from organic materials that originally stored solar energy in their chemical bonds via the photosynthesis process from water and CO2. Today, while burning hydrocarbons, we make available the energy stored in their chemical bonds with the concomitant re-emission into the atmosphere of the CO2 originally consumed in the photosynthesis process. For instance, approximately 22 million tons of coal, 12 million tons of oil (85 million barrels), and 10 billion m3 of natural gas are consumed per day to fulfill ∼82% of the total energy demand, resulting in about 30 billion tons of CO2 emission every year.2 Moreover, today the average power consumption on Earth is expected to increase to 27.6 terawatts by 2050 with an increased population of ∼9.1 billion.3 Also, industrial sectors such as steel, iron, and cement industries emit tens to hundreds of tons of CO2 annually in a localized and concentrated form for the production of materials essential to our economy. For instance, in 2018 in the European Union, CO2 accounted for 81% of total green-house gas emissions, which was 3970 million tons of CO2 equivalent, 22.4% of which was from industrial processes, agriculture, and waste management sectors.4 As such, all these anthropogenic activities are causing an accumulation of carbon dioxide in the atmosphere.5 The average CO2 concentration in the atmosphere has been stable for hundred-thousand years in the range of 200–300 ppm, while in the last two centuries it has started to steeply increase, exceeding nowadays 400 ppm.6,7 On the one hand, the presence of CO2 in the atmosphere is crucial for life on Earth, being involved in the C-cycle and in the regulation of the temperature of the Earth. Without CO2, life on Earth would not be possible.8 On the other hand, the actual value of the CO2 concentration in the atmosphere matters in maintaining the ecosystem compatible with our life. For instance, the increase of the average temperature on Earth causes a wide variety of problems such as more frequent droughts, more intense hurricanes, and the rising sea level.9–11 The Intergovernmental Panel on Climate Change (IPCC) has shown a strict link between the rise of CO2 concentration in the atmosphere due to anthropogenic activities and the increase of the average temperature on Earth.6 Hence, the IPCC stressed that more than 1000 Gt of CO2 should not be emitted from 2000 to 2050 to limit the temperature rise to 2 °C.12 Moreover, the Paris Agreement in 2015 suggested holding the increasing global temperature below 2 °C compared to preindustrial levels and pursuing efforts to limit the temperature increase to 1.5 °C above the preindustrial level.12 Therefore, policymakers are taking action to achieve net-zero CO2 emissions. The European Union and other countries such as New Zealand and South Korea are taking action to achieve CO2 neutrality by 2050, while China is moving towards the idea of achieving carbon neutrality by 2060. Research and Development (R&D) efforts are required not only to circumvent the CO2 problem but to turn it into opportunities by developing resource and energy-efficient processes towards net-zero CO2 emissions without compromising the nature, economics, and environment.13–17
Solving the problem by eliminating the need for fossil fuels as an energy source is not straightforward since fossil resources are the basis of our current chemical industry.18 From a technological point of view, fossil-carbon fuels are also very hard to replace as the main energy vector in our society for three main reasons: (i) they are characterized by huge energy densities; (ii) they are chemically stable under normal conditions; (iii) they are easy to store and transport. A promising concept would be replacing the fossil feedstocks currently used in the chemical and energy industry with sustainably produced chemicals and fuels by reducing CO2 using renewable energy. In this view, renewable H2 (e.g., water electrolysis with electricity from wind or solar energy) or renewable electricity can be combined with the undesired and highly available CO2 for the production of commercially important fuels and chemicals, resulting in a carbon-neutral technology of energy transformation and storage.
Such transformation can be done via electrocatalysis, photocatalysis, and thermal catalysis that are essential components in any sustainable energy and chemical production.19,20 With the highest oxidized state (+4) and extreme heat of formation (
= −393.5 kJ mol−1 at 25 °C), CO2 is thermodynamically a highly stable molecule (
= −394.4 kJ mol−1 at 25 °C), with a very strong C
O bond energy (799 kJ mol−1). As a result, the activation and transformation of CO2 need very high temperatures or a catalytic process working under suitable operating conditions. For the process of CO2 activation and conversion, different technologies have been proposed and developed,21–38 including bioconversion (e.g. enzyme catalysis),21,22,28 electrocatalysis,31–36 photochemical reduction,23–27,37–39 thermochemical processes,40–42 and their combination. This review is focused on thermo-catalytic CO2 activation43–46 to provide clean, affordable, and secure energy and chemicals by substituting conventional feedstocks.47 Furthermore, major attention is paid to the importance of using earth-abundant catalytic materials for CO2 activation, which has been widely studied and highlighted in several literature works.48–54
State-of-the-art experiments have shown the potential of CO2 in making chemical intermediates such as syngas,55,56 carbon monoxide,57 formic acid,41 methanol,58 methane,59 dimethyl ether,60 olefins,61 carbamates, carbonates, hydrocarbons (alkanes and aromatics)62 and higher alcohols,63 which can subsequently be transformed into a myriad of high-value products including chemical process intermediates and fuels. Despite the multitude of fundamental research studies on CO2 conversion and its potential to yield important chemical compounds, very few processes have been demonstrated so far with their commercial viability.64 The main technological challenges associated with the catalytic conversion of CO2 are (i) requirement of significant energy input from carbon-neutral sources to prevent further CO2 emission, (ii) need for high temperature and/or pressure processes to achieve good process performances, (iii) requirement of stable and active catalysts to prevent continuous waste of the catalytic material, (iv) poisoning of catalysts by residual water,65 (v) sintering of catalyst particles,66–74 (vi) coke formation during the reaction,75,76 (vii) overoxidation to toxic metal carbonyl (e.g., Ni(CO)4),77–84 and (viii) waste generation by undesired side reactions.
In this review, we present an overview of the state-of-the-art thermally-catalyzed approaches to utilize captured CO2 directly by making fuels and chemicals. In particular, we focus on the analysis of four main chemical reactions: (i) reverse water-gas shift (RWGS), (ii) CO2 methanation, (iii) CO2 hydrogenation to methanol, and (iv) dry reforming of methane (DRM). These reactions are illustrated in Fig. 1. In the analysis and description of the current literature on the selected reaction systems, major attention is paid to (i) catalyst materials employed, (ii) information on the reaction mechanisms gained from experiments and first-principles calculations, (iii) kinetic experiments and corresponding rate equations, (iv) most important challenges and possible solutions, and (v) available reactor technologies.
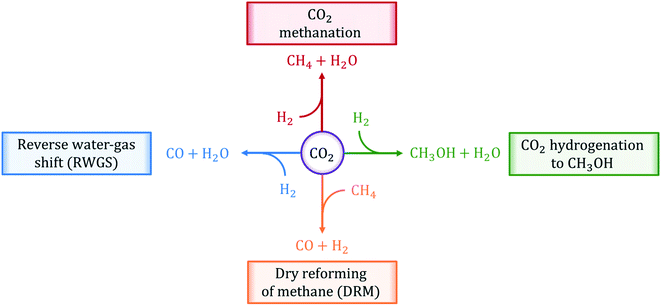 |
| Fig. 1 Chemical reactions of thermo-catalytic activation and conversion of CO2 to C1 products reviewed in this work. | |
2. Materials and mechanisms
The reaction mechanism of CO2 activation and conversion can vary over different catalysts and reaction conditions (e.g., temperature, pressure), and it involves the formation of several different reaction intermediates. The key steps of the reaction scheme are (i) chemical adsorption of CO2 and co-reactants (e.g., H2, CH4), which dissociate over the catalyst surface into reaction intermediates, (ii) surface diffusion and reaction of the intermediates at the catalyst active sites, and (iii) desorption of product species (e.g., CO, CH4, CH3OH, H2O) from the catalyst surface. The information on the reaction kinetics and mechanisms is crucial for the understanding of the pathways of CO2 activation and conversion, the identification of the rate-determining steps (RDSs), and the elucidation of the active sites of the catalyst. Such information is fundamental for the optimization of existing processes and the discovery of new catalytic materials, by guiding new experiments or by in silico catalyst design. An important concept to take into account when analyzing catalytic systems is that the reaction mechanism and the distribution of the active sites of a catalyst evolve under reaction conditions.85,86 Indeed, the local reaction environment that forms during the reaction highly affects the reaction rates,87 and catalyst materials can also undergo morphological transformation such as surface reconstruction, phase transition, and deactivation by sintering or coking. These are multiscale phenomena, which need a proper description based on the combination of experimental and theoretical analyses representing the different time and length scales (i.e., from the atomic scale to the reactor scale).
2.1 Mechanism of CO2 activation and conversion to C1 products
The reactions of transformation of CO2 into C1 products (CO, CH4, and CH3OH) proceed through a complex reaction scheme.88–107 A simplified version of the scheme is represented in Fig. 2. For illustrative purposes, four main reaction paths98,99 are identified in the reaction mechanism and their reaction intermediates are highlighted with different colours. The paths are (i) RWGS redox path, in orange, (ii) CO* methanation path, in grey, (iii) carboxyl path, in yellow, and (iv) formate path, in light blue. In the RWGS redox path, adsorbed
dissociates into CO* and O*. Then, CO* can desorb as gaseous CO, or it can further react. This path is called “redox” because in RWGS it is accompanied by the oxidation of H* to H2O. The dissociation of CO* to C* and O* is the first step of the CO* methanation mechanism, and it is followed by the successive reduction of C* to CH*,
,
, and gaseous CH4, by the addition of H* species. The carboxyl (COOH*) path involves the formation of a COOH* molecule by the addition of an H* to the O atom of
. In the successive steps, COOH* then loses an O* atom, producing COH*, which is successively hydrogenated to HCOH*, and H2COH*. The further reduction of H2COH* produces CH3OH in the gas phase. Similarly, the formate (HCOO*) path involves the reaction of
with an H* atom. However, an HCOO* molecule is formed when H* binds to the C atom of
. The formate path continues with the removal of an O* from HCOO* to give HCO*, which is hydrogenated to H2CO*, H3CO*, and eventually to CH3OH. The
dissociation, methanation, carboxyl, and formate reaction paths are interconnected by several elementary reactions; indeed, CO and CH4 can be formed also from intermediates of COOH* and HCOO* paths. The elementary reactions in the kinetic mechanism involve the addition or the removal of H*, O*, and OH* species. Those adsorbed species are provided by either other elementary steps depicted in Fig. 2 or by the dissociative adsorption of H2, and they are precursors for the formation of H2O. In DRM, the reaction paths in Fig. 2 which link CH4 to CO are followed backwards. Indeed, the adsorption and oxidation of CH4 produce CO* and H* species, which are the precursors of syngas (mixture of CO and H2). During DRM, carboxyl and formate pathways can also produce CH3OH as a side product. Besides the main intermediates and pathways reported in Fig. 2, other reaction intermediates can participate in the overall reaction mechanism. For example, in the carboxyl path, COH* can be formed from COOH* through a
intermediate. In the formate path, the formation of H2CO* from HCOO* can proceed with H2COO* or HCOOH* as an intermediate instead of HCO*. Other possible reaction intermediates include
and species adsorbed onto the catalyst support. Moreover, CO2 can also react directly from the gas phase to give HCOO*, COOH*, or CO* and O* species, without being first adsorbed.
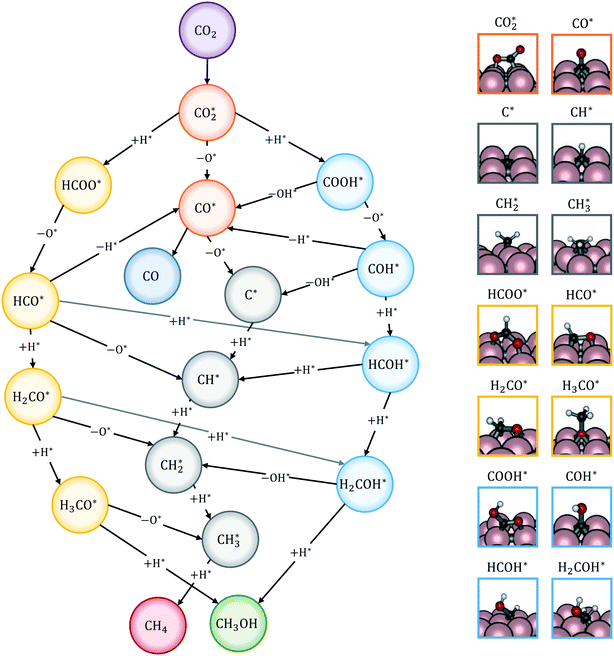 |
| Fig. 2 Simplified reaction mechanism of CO2 reduction to CO, CH4, and CH3OH, in which four main reaction paths are highlighted: RWGS redox path (orange), CO* methanation path (grey), formate path (yellow), and carboxyl path (light blue). On the right hand side, the structures of the reaction intermediates99 are highlighted with the colors of the corresponding reaction path. | |
In heterogeneous catalysis, CO2 activation consists of the interaction of the molecule with a catalytic surface, which usually consists of the chemical adsorption of CO2 (the first step of Fig. 2) to give the activated reaction intermediate
. Usually, the adsorption on the active sites of a surface implies the bending of the CO2 molecule. This bending lowers the energy level of the in-plane contribution of the 2π orbital (the lowest unoccupied molecular orbital, LUMO), which makes the carbon atom electrophilic. On metallic surfaces, CO2 activation usually consists of electronic charge transfer from the metal to CO2.108–110 On metal oxides, on the other hand, the adsorption exploits the amphoteric nature of CO2, which has a partial positive charge on the C atom (+0.37 e from Mulliken's population analysis) and negative charges on the two O atoms (−0.18 e).40 During the adsorption, the electron-deficient C atom and the more electron-rich O atoms can be attacked by either electron-rich or electron-deficient active sites, respectively.40 Typically, the carbon atom of CO2 interacts through O2− lattice atoms, and the oxygen atoms of CO2 interact with the metal cations (e.g., Mn2+).
The group of Mpourmpakis studied with DFT the CO2 activation on monometallic, core-shell, and decorated icosahedral CuNi (ref. 108) and CuZr (ref. 109 and 110) nanoparticles. For the case of CuNi, it is reported that the presence of surface Ni is key in strongly adsorbing the CO2 molecule, which occurs through a charge transfer from the nanoparticles to the CO2 molecule, where the local metal d-orbital density localization on surface Ni plays a pivotal role. Additionally, they found a linear relationship between the local-site d-band center and the CO2 adsorption energy and observed that the active sites of strong adsorption localize the HOMO (highest occupied molecular orbital) orbitals with increased d-character.108 For the case of CuZr nanoparticles, they observed that CO2 activation is endothermic on metallic Cu, whereas it becomes barrierless and exothermic on the Zr-decorated Cu nanoparticles, and that the rate of CO2 dissociation to CO* and O* is much higher on CuZr than Cu.109 Moreover, they showed that Zr sites can be oxidized because of their high oxophilicity; however, they are still able to adsorb and activate CO2 easily.110 Dixit et al.111 investigated CO2 adsorption on molybdenum carbide (Mo2C), showing an important influence of the O* coverage on the CO2 adsorption energy and the CO2 dissociation barrier. They explained this by showing an electronic modification on the catalyst surface (e.g., d-band shift on Mo atoms) with increasing oxygen coverage.
The adsorption configuration of
changes with the structure and composition of the catalyst surface.99,108–114 Usually, the C atom and one O atom of
bind to one or two metal atoms108–110,112 (on the “top” or “bridge” adsorption sites). The other O atom of
may interact with the surface.99,111 Particularly relevant is the interaction of
with the (100) facets of metal catalysts. Indeed, the square geometry of such facets allows for a
adsorption configuration in which C and O interact with two metal atoms each, which promotes the breaking of the C
O bond of
. This yields low activation barriers for
dissociation to CO* and O* on (100) facets, representing an example of a structure-sensitive elementary step.112,115 Regarding the elementary steps needed in the production of hydrocarbons (e.g., CH4) from
(Fig. 2), other structure-sensitive effects are reported in the literature.86,99,116–118 In those processes, the slow steps are related to the breaking of the triple bond in the CO* molecule (C
O),118 which can occur by direct CO* dissociation86,116,117 or by hydrogen-assisted dissociation (via a HCO* intermediate).99,118 For those reactions, the highest rates are usually provided by the stepped sites available on, e.g., (211),116,117 (311),86,112 and (110)99 facets.
2.2 Reverse water-gas shift (RWGS)
RWGS is a reversible and endothermic reaction (ΔH0R = +41.2 kJ mol−1 at 25 °C), in which CO2 reacts with H2 to give CO and H2O.93,119,120 It is an equimolar reaction, so its chemical equilibrium is independent of the pressure. At low temperatures, it is usually accompanied by side production of CH4via CO2 methanation.121,122 This is because the production of CH4 is favored by thermodynamics below ∼400 °C. As a result, high CO selectivity can be achieved by flowing CO2 and H2 at elevated temperatures.
Several supported metals including Pd,123,124 Pt,100,125–127 Rh,128–130 Au,122,131,132 Fe,133,134 Ni,47,94,135 and Cu,136,137 have been reported as active catalysts for the RWGS reaction. In addition, supported metal alloys, such as Pd–In/SiO2,138 Co–Fe/Al2O3,139 Fe–Cu/Al2O3,140 and Fe–Cu–Cs/Al2O3,140 were employed as catalyst materials, showing high CO selectivity (90–100%) and CO2 conversion ranging from 20 to 55%. Among supported metals, high catalytic performances were shown by Cu/CeO2 (ref. 137) (25 gCO gcat−1 h−1), Pt/TiO2 (ref. 100) (51 gCO gcat−1 h−1), and Rh supported on silicalite-1 (ref. 130) (76 gCO gcat−1 h−1) at 400–450 °C. At the same reaction temperatures, K–Mo2C/γ-Al2O3 (ref. 141) demonstrated also a good catalytic activity (50 gCO gcat−1 h−1), with a catalyst cost much lower than that of noble metals. At a higher temperature (600 °C), Cu/β-Mo2C (ref. 136) showed high catalytic activity (40% CO2 conversion, 99% CO selectivity) and very good catalyst stability provided by the support that prevents the sintering of Cu nanoparticles.
Based on experimental and theoretical observations, two kinds of mechanisms have been proposed,125 namely the redox mechanism142–144 and carboxyl (COOH*) mechanism.145–147 Formate species, which can form under reaction conditions,148–150 are usually reported as spectator molecules that do not participate actively in the reaction. In the redox mechanism,
dissociates to CO* and O*, and the latter reacts with H* (produced by H2 adsorption) to give OH* and then H2O*.114 In the carboxyl mechanism,
reacts with H* to give COOH*, which dissociates into CO* and OH*.151,152 Maestri and co-workers153 studied with DFT calculations the two mechanisms on the (111) surfaces of Pt, Rh, Ni, Cu, Ag, and Pd, showing different reaction paths on the different metals. Indeed, they showed that the redox mechanism is preferred on Rh(111), Ni(111), and Cu(111), whereas the COOH* mechanism is favored on Pt(111), Pd(111), and Ag(111). Brønsted–Evans–Polanyi (BEP) relations for
dissociation and hydrogenation were derived, and the occurrence of different reaction mechanisms was correlated with the oxophilicity of the metals, thus explaining that the stronger the interaction of O* with the metal, the lower the activation energy of
dissociation, which results in a preferred redox mechanism over the competing COOH* path (Fig. 3).
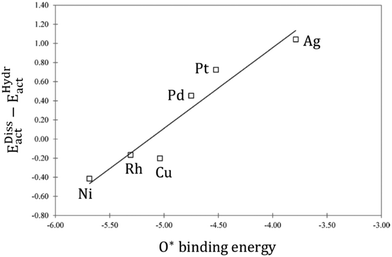 |
| Fig. 3 Linear correlation between the O* binding energy and the difference in the activation energies of dissociation (EDissact) and hydrogenation (EHydract). Adapted with permission from ref. 153. Copyright 2015 American Chemical Society. | |
Several theoretical and experimental observations reported a strong structure-sensitive character of the reactions of CO2 conversion,99,113,154 including RWGS.114,155 For example, Cai et al.113 investigated the interaction of CO2 (and H2O) on Ni(111) and Ni(100) surfaces using ambient pressure X-ray photoelectron spectroscopy and theoretical DFT calculations and observed a very different distribution of dissociation products on the two Ni facets in the presence of 0.2 Torr of CO2. On Ni(111), more than 90% of the adsorbed surface species are carbonate
, whereas Ni(100) is mainly covered by adsorbed CO* and graphitic C*. Moreover, they observed with DFT a very high difference in the activation energy of the CO2 dissociation reaction on the two facets: 0.33 eV on Ni(100) and 1.34 eV on Ni(111). This is explained by the geometry of Ni(100), which stabilizes the transition state of CO2 dissociation by interaction with four Ni atoms interacting with the π orbitals of CO2.115 Along with this, they showed that the conversion of CO2 on Ni(111) and Ni(100) tends to follow different reaction paths, in agreement with their experimental observations. Zhang et al.114 investigated the RWGS redox mechanism on Ni(111) and Ni(311), showing that the stepped (311) surface has higher catalytic activity than the flat (111) surface. Lin et al.155 investigated redox and carboxyl mechanisms on Ni(110) in the presence of a high density of subsurface hydrogen, showing that subsurface H atoms can play an important role in RWGS, by both lowering the energy barriers of the kinetic mechanism and participating actively in the hydrogenation elementary steps. They also concluded that, in their system, the redox mechanism is the most favorable RWGS pathway. Wang et al.156 investigated with transient quantitative temporal analysis of products (TAP) the ability of CO2 to re-oxidize a pre-reduced Au/CeO2 catalyst. They observed that the reduced catalyst is partially oxidized by CO2, suggesting that the redox path is possible on Au/CeO2. Wang and Nakamura157 performed DFT calculations on different Cu surfaces and revealed a strong structure-sensitivity of
and H2O* dissociation reactions. They calculated activation energies in the following order Cu(110) < Cu(100) < Cu(111) for both elementary dissociation reactions. They reported a “late” nature of the transition states, which resemble the geometry of dissociation products, and they concluded that the activation energies of
and H2O* dissociation are influenced significantly by the binding energies of OH* and O*, respectively. Liu et al.158 investigated the redox mechanism on the (100) surfaces of Fe, Ni, Co, and Cu. They reported that Fe(100) shows the highest CO2 adsorption energy, which, however, does not facilitate the reaction, but causes a thermodynamic sink on the reaction coordinate. Co(100) and Ni(100), on the other hand, are more favorable in terms of a smaller fluctuation in reaction energies and barriers. They also studied CO2 adsorption on Fe bcc(100) and Co hcp(1010) surfaces and suggested that that not only metals but also the surface structures significantly affect the reaction kinetics.
A widely accepted concept is that in the redox mechanism, the RDS is
dissociation.153,155–158 In the COOH* mechanism, on the other hand, COOH* formation is usually the limiting step.153 The reactions of water formation, i.e., OH* + H* → H2O* (and also O* + H* → OH* in the redox mechanism), can also be relevant for the kinetics of the RWGS reaction. Experimental159,160 and theoretical112,161,162 observations on an Rh/Al2O3 catalyst suggested that direct WGS and RWGS can follow different reaction mechanisms, where the H2O* formation steps are pseudo-equilibrated for RWGS, but they are the slow steps for direct WGS. Maestri and co-workers161,162 suggested that the RWGS reaction proceeds through
dissociation over Rh/Al2O3, whereas direct WGS proceeds with a COOH* intermediate. They calculated that H2O* dissociation and
dissociation are the RDSs for direct and reverse WGS, respectively, in agreement with the experimental reaction orders of Donazzi et al.159,160 Cheula and Maestri112 derived a structure-dependent microkinetic model of direct and reverse WGS on Rh/Al2O3, which describes the morphological evolution of the catalyst along with the surface reactions on five different Rh facets: (100), (110), (111), (311), and (331). Their study allowed to rationalize that far from equilibrium the two different reacting systems not only follow different reaction pathways but also show that the active sites are different for WGS and RWGS. Indeed, the WGS reaction occurs mainly on the Rh(111) facet, whereas RWGS proceeds on the active sites of Rh(100), which promotes the
dissociation elementary step thanks to its structural arrangement.
2.3 CO2 methanation
CO2 methanation, called also the Sabatier reaction,163 is an exothermic reaction (ΔH0r = −165.0 kJ mol−1 at 25 °C) favored at low temperatures, in which CO2 reacts with 4 molecules of H2 to give CH4 and 2 molecules of H2O. The production of CH4 from CO2 and H2 is advantageous due to the high energy density of CH4 and its easiness to be fed into conventional natural gas infrastructure.164 Moreover, because of the possibility to convert the Martian CO2 atmosphere into methane and water for the fuels and life support of astronauts,165 the National Aeronautics and Space Administration (NASA) is spending its efforts to apply this reaction for space colonization on Mars.165 The catalysts are typically based on Ni, Ru, Rh, and Co as active phases, with Ni being the most used due to its activity, selectivity, and low cost.166–172 The temperature is usually between 100 and 350 °C, as at higher temperatures, the production of CO by RWGS is favored by thermodynamics.173
Ni has been the most studied material for industrial CO2 methanation applications.174–176 High CO2 conversion (55–60%) and selectivity to methane (80–90%) were obtained on supported Ni catalysts.135,177 However, Ni presents severe deactivation and loss of activity due to carbon formation,178 metal sintering,179,180 and formation of Ni carbonyls.181,182 Alternatively, Rh, Ru, Pd, Pt, and Re on different oxide supports were studied.130,183–186 Among them, Ru and Rh showed very high catalytic performances (up to 95% selectivity to CH4 and 65–80% CO2 conversion).130,135,187 Cheaper materials, such as Co,188,189 Fe,190–194 and Mn,166,189 and bimetallic particles containing noble metals, Ni and Fe (ref. 194 and 195), were also investigated.
CO2 methanation was reported to be highly dependent on metal–support interaction.98,196–200 This phenomenon was related to the ability of CO2 to adsorb on the support materials. For instance, Pandey and Deo194 studied the reaction on Ni–Fe supported on different materials (i.e., Al2O3, ZrO2, TiO2, SiO2, and Nb2O5) and observed that the support yielding the highest activity, Al2O3, is the one that more favorably adsorbs CO2. Martin et al.197 studied CO2 methanation over Rh/CeO2 and Ni/CeO2 using spectroscopic analyses and demonstrated that Rh/CeO2 exhibits higher methane selectivity than the Ni/CeO2 catalyst with the same loading. This was explained by the lower Rh particle sizes resulting from the strong metal–support interaction. Moreover, they argued that the CeO2 support can influence the reaction rates by getting partially reduced during the reaction and producing Ce3+, which can facilitate the activation of CO2. Ocampo et al.170 investigated the Ni/Ce0.72Zr0.28O2 system and reported excellent activity and selectivity of CO2 methanation as well as good catalyst stability. The good performances of their catalyst were attributed to the good ability to store active oxygen and to the high Ni dispersibility.201–203
The proposed reaction mechanisms of CO2 methanation are mainly three: (i) carbide pathway,204–206 (ii) COOH* pathway,207,208 and (iii) HCOO* pathway,209,210 as illustrated in Fig. 4.b. Several authors proposed that a part of the reaction mechanisms can occur on the support207,211–213 or at the interfacial sites between the metal and support,103 while H2 is always supposed to dissociate on the metal. Particularly on the support, the formation of
by the oxidation of CO2 is also observed, which can be reduced to bicarbonate
and participate in the reaction mechanism211,212 by producing HCOO* species. Qin et al.213 achieved high conversion and methane selectivity at 280 °C and over bimetallic catalysts containing Ni, and they suggested bicarbonate, carbonate, and formate as intermediates based on their in situ FTIR analyses. Falbo et al.214 investigated CO2 methanation on Rh/Al2O3 at low and high temperatures and observed higher catalyst stability when CO was added to the inlet. Their spectroscopic analyses were consistent with a path where CO2 is adsorbed as bicarbonate on Al2O3 and it is successively hydrogenated to CH4 on Ru, passing through formate and carbonyl intermediates. Aldana et al.207 studied the reaction on strong and weak basic sites of Ni/Ce0.5Zr0.5O2 and Ni/γ-Al2O3 and showed that the weak basic sites result in monodentate carbonate, which is more prone to hydrogenation, while the strong basic sites form bidentate carbonates, which do not participate in the reaction mechanism. Yang et al.103 studied with DFT the CO2 methanation over a cluster of Rh supported on TiO2, highlighting the role of the metal–support interface, which is a significant charge accumulation region and can provide electrons for CO2 reduction. In their work, they showed that the interface active sites are more feasible for CO2 activation than the Rh nanoparticle sites. Moreover, they calculated that the COOH* mechanism involving the formation of a COH* intermediate is the favorite reaction pathway of CO2 methanation over the Rh/TiO2 catalyst, while the redox WGS and the formate pathways show higher energy barriers. Such preferred pathways proceed with the intermediates
, COOH*, CO*, COH*, HCOH*, H2COH*,
, and
, and show COOH* dissociation into CO* as the RDS.
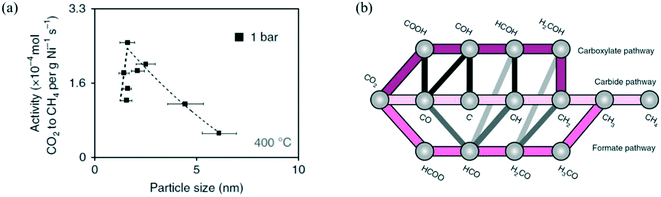 |
| Fig. 4 (a) The influence of Ni particle size on activity normalized to the Ni loading for CO2 methanation on Ni/SiO2, at 400 °C, 1 bar, and H2/CO2 = 4. Reproduced with permission from ref. 154. (b) Proposed reaction mechanism of CO2 methanation on Ni. Reproduced with permission from ref. 99. | |
Vogt et al.99,154 highlighted the strong structure-sensitive character of CO2 methanation over Ni catalysts. They studied the effect of a Ni/SiO2 nanocluster with particle size 1–7 nm and observed an optimum in the CO2 methanation activity at a particle size of 2–3 nm (Fig. 4.a).154 To understand such a particle size effect, they also studied CO2 methanation on Ni catalysts supported on different metal oxide supports (Al2O3, CeO2, ZrO2, TiO2), and they developed a microkinetic model based on DFT calculations on the (100), (110), (111), and (211) facets of Ni (reaction mechanism shown in Fig. 4.b). The integration of their microkinetic model allowed them to select the most active Ni facet, i.e., Ni(110), and identify the dissociation of HCO* (HCO* → CH* + O*) on such a facet as the RDS of the reaction mechanism. The maximum in the activity vs. size plot was explained by two phenomena: (i) the catalytic activity decreases with the particle size because big particles have a lower dispersion (i.e., the fraction of metal atoms at the surface) and therefore a lower number of active sites per gram of catalyst, and (ii) particles with size lower than 2–3 nm have low turnover frequency (TOF), (i.e., the catalytic activity per active site) because they do not show the most active sites for the reaction.215
The main phenomena that determine catalyst deactivation during CO2 methanation are sintering and coking. To identify the nature of carbon formed during methanation reactions over Ni/Al2O3, Olesen et al.216 performed temperature-programmed hydrogenation experiments and observed three major carbon peaks, attributed to carbide (∼650 K) and polymeric carbon (∼650 and ∼775 K). Loss of activity with an increase in the carbon-to-nickel ratio was noticed, primarily due to polymeric carbon formation that resulted in catalyst deactivation. A linear correlation was observed between the amount of carbon formed and the degree of deactivation of the catalyst.216 Barrientos et al.217 investigated various Ni/Al2O3 catalysts promoted with MgO, CaO, BaO, and ZrO2 under low-temperature conditions (300 °C), and they showed that the presence of Zn lowers the formation of polymeric carbon. Galhardo et al.,92 with in situ spectroscopic analyses, observed that the accumulation of carbon species on the surface of a Ni/SiO2 catalyst at high temperatures leads to a Ni3C-like phase, which changes the process selectivity towards the formation of CO. After carbon depletion from the surface of the Ni particles by oxidation, the catalyst regains its high selectivity to CH4 production. However, the selectivity readily shifts back toward CO formation after exposing the catalysts to a new temperature-programmed CO2 hydrogenation cycle. The fraction of weakly adsorbed CO* increases on the Ni3C-like surface when compared to a clean nickel surface, explaining the higher selectivity to CO.
2.4 Dry reforming of methane (DRM)
Syngas (a mixture of CO and H2) is an important intermediate used as a building block molecule to manufacture high-value chemicals (e.g., methanol, olefins, and hydrocarbons), and it can be produced from CO2via DRM,218,219 a reaction in which CO2 combines with CH4 to give 2 molecules of CO and 2 molecules of H2. As DRM is an endothermic reaction (ΔH0R = +247.0 kJ mol−1 at 25 °C), it is thermodynamically favorable at high temperature, so the reaction temperature is usually between 550 and 800 °C. On the surface of a catalyst, CO2 and CH4 dissociate into reaction intermediates (e.g., CO*, O*, H*, OH*,
,
), which combine to give the final reaction products (CO and H2). The most relevant unwanted side-product is coke, which can accumulate and deactivate the catalyst.
Nickel is the most important and utilized material for DRM, because of its high activity, high abundance, and low cost. High conversion of both CO2 and CH4 (80–95% at 700–800 °C) was obtained with Ni catalysts supported on different materials such as Al2O3,220 SiO2,221 and BN.222 However, rapid coke deposition on the catalyst surfaces during the reaction limits the practical use of Ni-based catalysts.217,223 To cope with such challenges, noble metals like Rh Ru, Pt, Ir, and Pd were also investigated, because of their higher coking resistance.224–226 Good catalyst performances and resistance to deactivation by coking were showed by supported bimetallic catalysts such as Ni–Rh/LaAlO3,227 Ni–Cu/MgO,228 and Ni–Mn/Al2O3.229 Such hybrid materials have a lower cost than noble metal catalysts and show high catalytic performances (85–97% CO2 and CH4 conversion at 750–800 °C).227–229
Several researchers have attempted to understand the mechanism of DRM on supported metal catalysts.230–235 Bradford et al.235 performed kinetic studies on Ni supported on different materials (SiO2, TiO2, MgO, and C) and suggested a reaction mechanism consistent with their experimental data. In their mechanism, CO2 dissociates to CO* and O*, and CH4 dissociates into
and H*. Then, OH* species are formed and act as an oxidant. Indeed,
gets oxidized by OH* to form CHxOH* species, which produce CO and H2 upon subsequent reactions. Both CH4 dissociation and CHxOH decomposition were supposed as the slow steps of the mechanism. Múnera et al.236 have concluded in their kinetic studies of DRM on Rh/La2O3–SiO2 that CH4 decomposition takes place on the metal, whereas CO2 activation occurs on the support. Maestri et al.237 proposed CH4 dissociation (CH4 →
+ H*) as the RDS on an Rh/Al2O3 catalyst, from hierarchical microkinetic modeling. With their analysis, they show that CH4 dehydrogenates into atomic carbon (C*), which gets oxidized subsequently by OH* over Rh surfaces. Mark et al.238 concluded that CH4 dissociation is the RDS for DRM also over Ir/Al2O3 at 700–850 °C and 1 atm. However, they reported that adsorbed carbon C* reacts via the reverse Boudouard reaction (BR) (C* +
⇌ CO*) to give the final products.239 CH4 dissociation was further confirmed as the RDS in DRM by Iglesia and co-workers through conducting experiments over supported Ni, Pd, and Rh catalysts at temperatures lower than 600 °C using the CH4/CD4 isotope tracer method.239–241 Fan et al.230 derived a comprehensive microkinetic model based on DFT calculations on Ni(111), Ni(211), and Ni(100) facets, and showed that at low CH4 and CO2 partial pressures, both CH4 dissociative adsorption and C* oxidation affect the overall reaction rate, whereas, at high pressures, C* oxidation is suggested as the only RDS for the DRM reaction, in agreement with previous experimental observations. Manavi and Liu242 investigated the reaction mechanism of DRM on the (111) facet of Co3Mo3N, a catalyst that can activate easily both C
O and C–H bonds, and proposed a reaction network for DRM, as shown in Fig. 5.a. Moreover, they derived a 2D volcano plot illustrating the catalytic activity vs. the binding energy of C* and O* for different materials (shown in Fig. 5.b), highlighting the importance of mild adsorption energies for the highest catalytic activity.
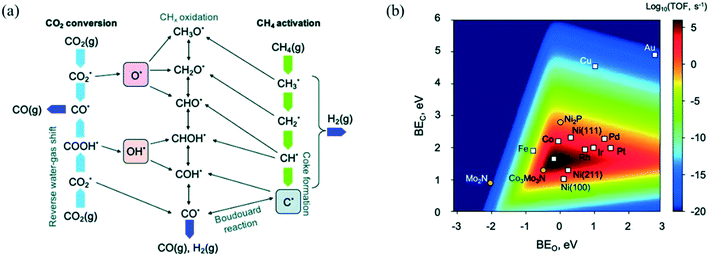 |
| Fig. 5 (a) DRM reaction network displaying the conversion routes of CH4 (green arrows) and CO2 (light blue arrows), including relevant reaction intermediates and elementary steps. (b) 2D volcano plot indicating the DRM catalytic activity (TOF) as a function of the binding energies of C* and O*. Adapted with permission from ref. 242. | |
Since carbon is miscible in Ni surfaces, it can diffuse through the surface and form carbide-like phases such as Ni3C.243,244 To understand the role of Ni3C in syngas production and catalyst deactivation, Wang et al.245 investigated DRM over flat and stepped Ni and Ni3C surfaces with DFT calculations. They reported that, due to the high CH oxidation rate and low CO dissociation, the flat Ni(111) surface shows high catalytic activity for DRM and low coke formation. The flat Ni3C(001) shows good DRM catalytic activity, but it also produces coke. The stepped surfaces of both nickel – Ni(211) – and nickel carbide – Ni3C(111) – show instead poor DRM performances and high coke formation.
The support material is another important factor that can modify the reactivity and stability of the catalyst under reaction conditions by changing its dispersion and its electronic properties.246 The role of different supports and additives in coke deposition and the durability of Ni-based catalysts has been extensively studied.194,247–249 Sokolov et al.250 investigated the role of different supports in the activity of Ni at 400 °C. Their Ni/La2O3ZrO2 catalyst was reported to be the most active and most stable. They also studied different structures of La2O3ZrO2, i.e., nonstructured, mesoporous, and microporous. The mesoporous support showed no change in activity over 180 h on-stream, whereas the others deactivated, by the formation of graphene-like coke layers and NiO shells over Ni particles. The enhanced stability of Ni on the mesoporous La2O3ZrO2 was attributed to a pore confinement effect. Yavuz and co-workers218 demonstrated very high stability of the Ni–Mo/MgO catalyst (up to 850 h) without coke formation at 800 °C under DRM conditions. This high stability was presumed to be due to the small particle size (2.9 nm) of the Ni–Mo/MgO nano-catalyst that may lead to better dispersibility and prevent coke formation.
2.5 CO2 hydrogenation to methanol
Methanol is an important chemical compound that can be used as an additive for fuels or as a precursor of, e.g., dimethyl ether, gasoline, and diesel. CO2 hydrogenation to methanol is an exothermic reaction (ΔH0r = −49.5 kJ mol−1 at 25 °C), favored at low temperatures and high pressures. A good catalyst for the production of methanol from CO2 must have active sites for the activation of CO2, dissociate H2, and suppress competitive side reactions (e.g., RWGS, methanation).251–254 Usually, this implies the use of active metal-oxide materials,52,253 which can also be doped to change their surface electronic properties.255–258
Industrially, methanol is produced from synthesis gas mixtures (CO/CO2/H2) at 200–300 °C and 50–100 bar (ref. 259) over Cu/ZnO/Al2O3 catalysts, selected because of the low cost of Cu and the good synergism between Cu and ZnO. For CO2 conversion, the Cu/ZnO/Al2O3 catalyst displays lower activity towards methanol formation due to the competitive RWGS reaction260 and water-induced deactivation.261,262 The production of H2O is higher when CO2 is present in the feed because the CO2 to methanol reaction stoichiometry (CO2 + 3H2 ⇌ CH3OH + H2O) implies the formation of an H2O molecule for every CO2 molecule.261–263 The production of H2O enhances also the sintering of the catalyst.70–74,263 To overcome these challenges, different support materials, which can promote structural and electronic properties and stabilize smaller Cu particles,264 were investigated, including ZrO2,265,266 La2O3,267 MoC2,267 CeO2,268 and La2O2CO3 (ref. 267) and ZnO–ZrO2.98,259,269–275 In particular, Cu/ZnO/ZrO2 showed very high activity and selectivity at low temperatures (180–240 °C).276–279 This is likely due to the weak hydrophilic nature of ZrO2, which may inhibit the poisoning effect of water on the active sites272,273 and promote the activity by increasing Cu dispersion, which can strongly affect CO2 adsorption and methanol selectivity.280,281 Other materials investigated for CO2 hydrogenation to methanol are Au/CeOx/TiO2,282 In2O3,283–287 Ir–In2O3,288 Ni–In2O3,289 Mn–Co,290 Ni–Ga,291 ZnO–ZrO2,292 GaPd2,293 and Co/SiO2.91 In particular, In2O3 is attracting great interest in the scientific community283–287 because of its high catalyst stability265,286 and very high methanol selectivity.294–298 However, there is still a debate on the currently available best catalyst of methanol synthesis from CO2, mainly because the performances of the catalysts strongly depend on the experimental conditions under which they are tested. An In2O3/ZrO2 catalyst287 provided high methanol selectivity (99.8%), low CO2 conversion (5.2%) and a productivity of 0.30 gMeOH gcat−1 h−1 at 300 °C, 50 bar, with a gas hourly space velocity (GHSV) of 16
000 h−1. Under similar experimental conditions (240 °C and 50 bar), a Cu/ZnO/ZrO2 catalyst showed higher methanol productivity (1.2 gMeOH gcat−1 h−1), a CO2 conversion of 9.7% and a methanol selectivity of 62.0%.299 At 280 °C, 46 bar, and GHSW = 10
000 h−1, Cu/ZnO/Al2O3 (ref. 300) showed higher CO2 conversion (23.8%) but lower methanol selectivity (22.8%) and a productivity of 0.15 gMeOH gcat−1 h−1. The same Cu/ZnO/Al2O3 catalyst at extreme pressure (442 bars) showed much better performances, i.e., a CO2 conversion of 84.7%, a methanol selectivity 93.1% and a productivity of 2.18 gMeOH gcat−1 h−1,300 highlighting the strong positive effect of the pressure on the thermodynamics and kinetics of the reaction.
Three main pathways301 have been proposed for CO2 hydrogenation to methanol (Fig. 6), with a mechanism slightly different from the one represented in Fig. 2. In the formate (HCOO*) mechanism,259,302,303
is hydrogenated to HCOO*, H2COO* (or HCOOH*), and to H2COOH*. Then, an OH* is removed, yielding H2CO*, which is hydrogenated to H3CO* and CH3OH. In the RWGS mechanism, CO* is formed from
through a COOH* intermediate, then CO* is hydrogenated to HCO*, H2CO*, H3CO*, and CH3OH. In the hydroxycarbonyl mechanism, COH* is formed through a
intermediate, and then it is hydrogenated to HCOH*, H2CO*, H3CO*, and CH3OH.
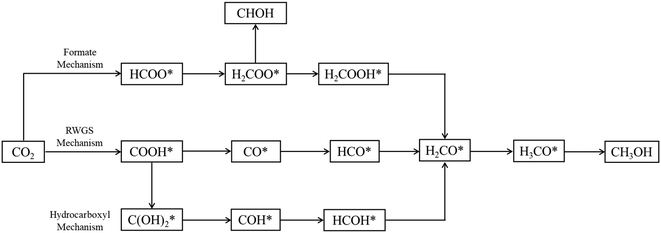 |
| Fig. 6 Proposed reaction paths301 of methanol synthesis via CO2 hydrogenation over Cu-based catalysts. | |
Regarding the Cu/ZnO system, many hypotheses on the nature of the active sites were proposed.259,304,305 Fujita et al.306 investigated the system with diffuse reflectance FT-IR spectroscopy and temperature-programmed desorption, showing that two types of formate species and zinc methoxide – Zn(CH3O)2 – form during the reaction. Zinc methoxide was readily hydrolyzed to methanol, whereas H2O formed through RWGS was suggested to be involved in the hydrolysis of zinc methoxide. In a recent communication, Muhler and co-workers307 investigated CO2 hydrogenation to methanol on Cu/ZnO/Al2O3 with a surface-sensitive operando method using a high-pressure pulse experiment (HPPE) and proposed a mechanism for long-term catalyst deactivation, as illustrated in Fig. 7. In the beginning, reduced ZnOx species migrate onto the metallic Cu0 nanoparticles and form Cu0–Zn0 alloys.305 Then, under CO2 hydrogenation, different oxygen-containing adsorbates form, which partially oxidize Zn0 to Znδ+ at the defective Cu0 site.259 The subsequent migration of Zn species leads to a graphitic-like ZnOx layer on Cu0 surfaces,304 which ultimately turns into a stable and crystalline layer of ZnO,304 covering the Cu0 surface completely or partially.269 During the process, the highly active Cu0–Znδ+ sites were found embedded in a constantly changing matrix provided by the Cu/ZnO/Al2O3 catalyst.
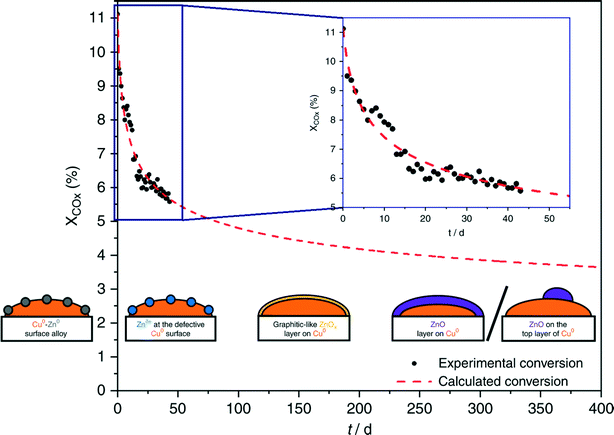 |
| Fig. 7 Long-term methanol production over the industrial Cu/ZnO/Al2O3 catalyst at 210 °C and 60 bar, under different controlled conditions. Black points refer to the recorded degrees of conversion of the reaction; the dashed red line describes the intra- and extrapolation of the experimental data. Illustrations from right to left: Cu0–Zn0 surface alloy, Znδ+ species at the defective Cu0 surface, and graphitic-like ZnOx layer on Cu0. ZnO layer on Cu0 and ZnO on the top layer of Cu0. Reproduced with permission from ref. 307. | |
The reaction mechanism and RDS for methanol production over Cu are still under debate.308–312 Bowker et al.310 suggested the hydrogenation of H2CO* to H3CO* as the rate-limiting step. Other authors308,309 reported instead the hydrogenation of HCOO* to H2COO* as the RDS over Cu(111). Zhao et al.311 showed that the HCOO* path is kinetically unfavorable compared to the COOH* route, especially in the presence of water. The isotope labeling experiment by Chinchen et al.313 suggested that CO may not be an essential intermediate for methanol formation from CO2. Mavrikakis and co-workers312 produced a microkinetic model based on DFT calculations which describes WGS and methanol synthesis from CO and CO2 on Cu(111) and highlighted the role of HCOOH* as a reaction intermediate. They showed different sequences of intermediates in methanol production from CO (HCO*, CH2O*, and CH3O*) and CO2 (HCOO*, HCOOH*,
, CH2O*, CH3O*). They show that methanol synthesis rates are limited by methoxy (CH3O*) formation in CO-rich environments and by CH3O* hydrogenation in CO2-rich feeds. Higham et al.314 have concluded in their DFT studies that Cu(110) and Cu(100) are more active facets for CO2 dissociation and hydrogenation than the more abundant Cu(111) surface. Moreover, they showed that the synthesis of methanol on those Cu surfaces can follow also reaction paths with COOH* as an intermediate. Eventually, some experimental observations suggest that the formate mechanism should not be the preferred one, because (i) direct hydrogenation of formate on the Cu surface did not result in methanol in the absence of water;309 (ii) the experimentally observed formate hydrogenation kinetics was inconsistent with that of methanol formation,308 and (iii) during methanol synthesis, formaldehyde, easily produced from H2CO*, has not been detected as a side product.315,316 Therefore, further theoretical studies, especially on the study of the active sites at the catalyst–support interface, are required.
Recent experimental studies demonstrated high performances of In2O3 catalysts for methanol synthesis from CO2.283–287 Martin et al.287 produced an In2O3/ZrO2 catalyst showing 100% methanol selectivity at 200–300 °C and 1–5 MPa and high stability (1000 h on stream). The excellent selectivity of the catalyst was attributed to the formation of oxygen vacancies, promoted by both the ZrO2 support and a co-feeding of CO. The group of Nørskov317 investigated with DFT the methanol synthesis on In2O3(111) and In2O3(110). They produced a theoretical volcano plot illustrating a clear relationship between the number of reduced surface In layers and the catalytic activity of In2O3(111). Moreover, they explained the positive effect on the catalytic activity of the ZrO2 support, which influences the number of reduced In layers.
Following these findings, Zhou et al.318 investigated with DFT analyses the reaction mechanism on In2O3(111) and In2O3(110). They reported that CO production from CO2via both redox and COOH* routes is kinetically slower than methanol formation under typical steady-state conditions, in agreement with the experimental observations showing high methanol selectivity of In2O3. They reported a formate mechanism of methanol synthesis in which H2COO* is produced from HCOO* and then dissociates to H2CO* and O*. The O* fills the oxygen vacancy site of In2O3, and H2CO* is then hydrogenated to H3CO* and CH3OH. Their calculated RDS is the homolytic H2 dissociation and suggested that the reaction rate can be enhanced by introducing transition metal dopants which speed up H2 dissociation. Dang et al.319 investigated with DFT the cubic and hexagonal surfaces of In2O3, showing that the hexagonal In2O3(104) shows far superior catalytic performance. Moreover, they synthesized a novel hexagonal In2O3 nanomaterial that exhibits very high activity, methanol selectivity, and catalytic stability. On In2O3, the formate mechanism is suggested as the most probable pathway, entailing cyclic creation and annihilation of oxygen vacancy active sites, and involving the reaction intermediates HCOO*, H2COO*, H2CO*, and H3CO*.318
A major shortcoming of In2O3 catalysts is the long-term stability. To investigate morphological changes of the In2O3 catalyst during CO2 hydrogenation to CH3OH, Tsoukalou et al.285 performed combined time-resolved operando XAS–XRD and in situ TEM experiments. Their results show the reduction of In2O3 during the reaction by the formation of oxygen vacancy sites (In2O3−x), followed by the amorphization of In2O3 nanoparticles into a dynamic mixture of In0/In2O3−x, in which crystalline and amorphous phases coexist and continuously interconvert. The formation of metallic In0 was reported to cause the deactivation of the catalyst.
3. Carbon formation and design for coke-tolerant catalysts
Catalyst deactivation due to carbon formation is one of the critical technological problems for CO2 conversion processes.66,223 It is an inevitable process that can lead to a temporary or permanent catalyst deactivation via pore blockage,320,321 metal particle encapsulation,322 or breakage of the catalyst pellets.217 In the processes reviewed in this work, carbon is primarily produced by CO disproportionation (2CO → C* + CO2) and CH4 dehydrogenation (CH4 → C* + 2H2). The latter reaction is favored at high temperature, while CO disproportionation is exothermic and thus thermodynamically favored at a temperature lower than 500 °C.
The carbon formed at the catalyst surface can assume various forms depending on the operating conditions,323–325 each form exhibiting unique features and different reactivities.244,326
As shown in Fig. 8, CO and methane dissociate on the metal surface to form a highly reactive carbon species, Cα (probably an adsorbed atomic carbon), which can be gasified (to CH4) or turned into the less reactive Cβ and assume the form of polymeric carbon films or filaments.327 On exposure to high temperature (T > 500–550 °C), amorphous carbon (Cβ) is transformed into an even less reactive graphitic form (Cc).327 Some of these carbon structures such as polymeric (Cβ) and graphitic (Cc) films are responsible for deactivation due to encapsulation of the metal particles at the catalyst surface.328 Meanwhile, other forms such as filamentous carbon, generally do not deactivate metal surfaces but may plug catalyst pores and break up catalyst pellets.328 The growth of such carbon species depends on the possibility and the ease of the carbon Cα to dissolve through the metal particles.329 The dissolved carbon can diffuse through the metallic crystallite to nucleate and precipitate at the rear of the particles.244 This process leads to the formation of carbon whiskers, which lift the crystallite from the support surface and eventually result in the fragmentation of the catalyst.328 The driving force for intra-particle carbon diffusion and whisker growth is a gradient in carbon activity (i.e., concentration) across the metal particle.244 Noble metals, except for palladium at 650 °C, do not form this carbon structure; the structure of the carbon formed on noble metals is difficult to distinguish from the catalyst structure, with few atomic layers of carbon covering almost completely the surface.330 Leung et al.331 proposed that carbon formation rates and morphologies on Ni (i.e., filamentous or encapsulating) are solely determined by the pressure ratio PCH4PCO/PCO2, which sets the thermodynamic carbon activity at the metal surface. Low values of this ratio lead to the formation of carbon filaments with a rate proportional to the ratio itself. Meanwhile, high values of carbon activity lead to the simultaneous nucleation of multiple carbon patches, with the consequent formation of carbon adlayers that brings about the encapsulation of Ni nanoparticles. In this sense, carbon formation rates decrease with decreasing metal particle size because of the lower stability and concomitant increase in activity of the smaller diameter carbon filaments formed on smaller metal nanoparticles. This phenomenon was reported also by other authors.332,333
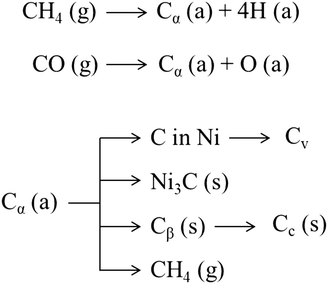 |
| Fig. 8 Various forms of carbon327 generated from CO over the Ni surface. (a), (g), and (s) refer to adsorbed, gaseous, and solid states, respectively. | |
Under common industrial operative conditions (T > 800 °C), CH4 decomposition is the main cause of carbon formation during DRM.333 For a given CO2/CH4 ratio, the temperature below which carbon deposits are formed decreases as the pressure decreases, while at constant pressure, this temperature limit increases as the CO2/CH4 ratio decreases.334,335 Thus, working with an excess of CO2 in the feed may reduce carbon formation at lower temperatures. With stoichiometric feeds, a temperature higher than 800 °C should suppress carbon deposits where there is thermodynamic potential.224 Catalyst deactivation due to carbon formation was observed during RWGS only when the reaction is conducted at low temperatures. Goguet et al.336 observed a slow but continued catalyst deactivation due to carbon formation over 2% Pt/CeO2 under RWGS conditions at 300 °C mainly due to CO disproportionation. Witte et al.337 experienced a loss in activity of a Ni catalyst during an 1100 h test at 350 °C under CO2 methanation conditions. They proved the presence of carbon deposits through TPO with oxygen conducted over the spent Ni-based catalyst and suggested the addition of steam into the reactor feed to prevent coking. Carbon deposits were also proved to form under the same conditions over noble metals catalysts by Solymosi et al.171,338,339
Once formed, carbon may be removed from the catalyst surface to restore the initial activity. The alternatives are gasification with H2 or H2O or controlled oxidation with oxygen or oxygen-containing compounds (e.g., CO2 itself).341 Gasification with H2O and H2 occurs at significant rates from 500 to 700 °C once the encapsulated material has been removed, making the metallic particles available for the catalyzed reaction.342 Moreover, the gasification of carbon with water was found to be faster than the one with hydrogen.342 Indeed, the removal of carbonaceous deposits with H2, like the one with CO2, involves dissociative adsorption and a surface reaction with carbon. In this sense, it has been successfully demonstrated that the addition of different promoters such as alkali and alkaline-earth metals enhances the gasification of carbon species and increases the stability with time. These elements enhance the adsorption and dissociation of water and CO2, increase the rate of gasification by H2 and lower the carbon solubility in the active metal. ZrO2, commonly used as a metal support in heterogeneous catalysts, can be used as an additive to favor CO2 activated adsorption, thus promoting higher levels of activity for the DRM reaction and the gasification of intermediate precursors in the carbon generation.340 In particular, the combination of Al2O3 and ZrO2 yields a reduction of catalyst deactivation by coking (Fig. 9). Although oxygen is the most effective in removing carbon deposits, a loss of activity following the regeneration process has been observed due to the oxidation of the active phase and to the loss of metallic atoms.327
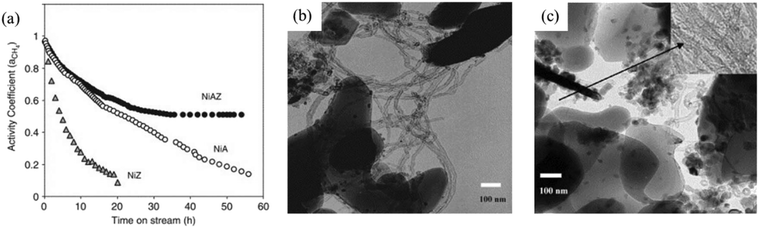 |
| Fig. 9 (a) Catalyst deactivation in terms of the CH4 activity coefficient (ratio between the CH4 consumption rate and the initial consumption rate) during DRM over Ni-αAl2O3 (NiA), Ni–ZrO2 (NiZ), and Ni–ZrO2–Al2O3 (NiAZ) catalysts at 700 °C. (b) TEM image of the Ni–αAl2O3 catalyst after DRM deactivation at 700 °C. (c) TEM image of the Ni–ZrO2–Al2O3 catalyst after the DRM deactivation test at 700 °C. Adapted with permission from ref. 340. | |
For industrial applications, it is important to significantly improve the stability of the catalyst and make it resistant to coke. In this view, both active phase and support optimization is needed. Particle size, support defects, particle composition, and temperature-induced aggregation are the principal causes of catalyst instability. Precious metals (e.g., Ru, Rh) exhibit superior activity but are limited and expensive, and thus R&D efforts have been shifted towards abundant and active metals like Ni.92,94,96,222,343,344 To increase the catalyst performance while retaining its stability and resistance to coke formation, several approaches such as carbon gasification221,345–347 through introducing redox or basic materials, alloying active metals (e.g., Ni) with other metals,228,348–351 and enhancing the thermal stability of active metal particles by strong interaction with the support or confinement inside stable structures352–355 have been attempted. In order to reduce coke formation and improve catalyst stability/activity, numerous strategies involving modification in the catalyst surface composition and metal–support interaction have been attempted.219,220,222,356–368 To develop a coke-resistant catalyst, approaches based on selective blockage of the defect sites of the active metal (e.g., Ni) nanoparticles by the use of inert elements such as S,330 Sn,369 Au,370 and K (ref. 234 and 371) were attempted. Atoms of these elements are supposed to be favorably localized on the defect sites of the Ni surface. For instance, Juan-Juan et al.371 have summarized the role of K in improving the stability of Ni metal with the following assumptions: (i) introduction of a small portion of K can increase the reducibility of Ni species as it enhances the interaction of NiO with the alumina support; (ii) K migrates from the support to the Ni metal surface and neutralizes a portion of active sites; (iii) coke formed during the reaction gets gasified by K without any change in its structure; (iv) K has no role in the modification of particle size or the structure of the catalyst. In an analogous study, elements such as K, S, and Au have shown improved catalytic activity by selectively blocking the active sites of the Ni stepped facet such as Ni (211),332 which are responsible for coke formation.372 Similarly, Chen et al.373 observed a promotional role of a B-based catalyst with improved coking resistance during the experiment for partial oxidation of methane (POM). Moreover, boron was suggested for effectively blocking the subsurface sites and avoiding carbon diffusion into the bulk, which compels carbon atoms to remain on the surface and react.374,375 Most recently, Song et al.218 developed a new technique for the preparation of Ni–Mo/MgO catalysts, wherein Mo doped Ni nano-crystallites move towards the edges of the MgO support, unite, and then stabilize at the step edges. Their catalyst exhibited high conversion and strong catalyst stability (850 hours) under DRM reaction conditions with anti-sintering and anti-coke properties.
4. Kinetic models
The data obtained from the reaction kinetics are applied in designing reactors, studying reaction mechanisms, and elucidating reaction–property relationships of catalyst materials. In general, the power law model is the simplest and can provide a satisfactory description of the process through a rough estimation of the required parameters.239,376 However, it does not account for the underlying reaction mechanisms. The Eley–Rideal (ER) model377 assumes that one reactant (e.g., CH4 or CO2 in DRM) adsorbs onto the catalyst surface, whereas the other one reacts from the gas phase. Then, the Langmuir Hinshelwood–Hougen–Watson (LHHW) model is the result of a reaction mechanism that implies (i) the adsorption of the reactants on the active sites, (ii) their reaction at the catalyst surface, and (iii) the desorption of the reaction products, and it is based on the hypothesis that there is one (or more) reaction step slow enough to be rate-limiting (i.e., the RDS), while the other ones are pseudo-equilibrated. More detailed modeling comes from microkinetic modeling (MKM), a powerful tool for the description of catalytic processes with an atomistic level of detail. A microkinetic model is a detailed kinetic model in which the single elementary steps of the reaction mechanism are characterized by their thermodynamic (e.g., binding energies of reaction intermediates) and kinetic properties (e.g., activation energies). The integration in time of a microkinetic model allows for the rational understanding of the reaction mechanisms occurring at the catalyst surfaces, the identification of preferred reaction paths, and corresponding active sites and RDSs. A great breakthrough was achieved with the possibility to combine DFT calculation with MKM.230,378–380 This powerful combination can elucidate different aspects of the reaction mechanism and the reaction kinetics without a priori assumptions compared with the traditional experimental kinetic methods.372,381
4.1 Reverse water-gas shift (RWGS)
The kinetics of the RWGS reaction has been widely investigated in the literature as a stand-alone process for the conversion of CO2 to syngas151,382–384 and as an inevitable side reaction in reforming processes.144,160,385–389 The RWGS kinetics over CuO/ZnO/Al2O3 was studied by the use of both differential and integral plug flow reactors by Ginés et al.390 A good agreement between the experimental and calculated data was obtained under different conditions by considering a Langmuir–Hinshelwood redox mechanism on Cu. However, in agreement with other authors who studied Cu-based catalysts, they noticed that the activity and the order of reaction of CO2 and H2 depend strongly on the reactants' partial pressures. This is due to a recontraction process that affected the catalyst surface caused by H2-rich working conditions, as proposed by Campbell and Ernst391 and then confirmed by many studies over Cu single-atom catalysts. Chen et al.392 performed a kinetic study of the RWGS reaction over silica-supported Cu-nanoparticles and proposed a Langmuir–Hinshelwood model starting from a formate mechanism. Kim et al.,248 on the other hand, derived two different reaction rates for the conversion of CO2 to CO according to associative and redox mechanisms. The initial reaction rate of the two mechanisms was consistent with the experimental data under low and high H2 partial pressures. However, only the redox-derived rate was able to fit the experimental data under moderate hydrogen partial pressures. Wolf et al.393 used a commercial Ni catalyst to study the RWGS reaction and determine its intrinsic kinetics to design an industrial plant. The intrinsic kinetics was examined at low residence time in a differential packed bed reactor and then modified to consider pore and external diffusion limitations. Then, the kinetic model was used in the simulation of a 1D fixed-bed reactor and validated with experimental data. Several authors used microkinetic models already developed for other processes (i.e. steam reforming, CO2 methanation, and CO2 hydrogenation to methanol) to gain new insights into the RWGS reaction system.376,394 A detailed multi-step heterogeneous reaction mechanism developed for SRM, partial and total oxidation of methane, and RWGS, for Ni-based catalysts was used by Benzinger et al.395 to interpret the experimental data obtained from the RWGS reaction in a monolith reactor. The microkinetic model, which included 42 reactions, 7 gas-phase species, and 12 surface species, was adjusted for thermodynamic consistency in the temperature range used for the study and validated with data derived from isothermal experiments in a fixed bed reactor over a commercial Ni/Al2O3 catalyst. Maestri et al.161,162,237 refined their first microkinetic model on Rh guided by the DFT-based analysis of the WGS/RWGS pathways and based on a comprehensive set of isothermal experimental data. Their semiempirical microkinetic model refined with DFT calculations was used to quantitatively describe the roles of WGS and its reverse in catalytic partial oxidation of methane on a Rh-based catalyst and it was extensively validated with experiments.
The structure-dependent microkinetic model of Cheula and Maestri112 describing WGS and RWGS on Rh/Al2O3 represents a novel methodology for the simulation of structure and activity of catalyst materials, allowing for the identification of the “nature” and “identity” of the active site in a self-consistent manner. In their model, the morphology of heterogeneous catalyst nanoparticles – that represents the “nature” of the active sites – is calculated using ab initio thermodynamics and Wulff–Kaishew construction methods. The reaction rates – that determine the “identity” of the dominant active sites – are calculated by integrating a DFT-based microkinetic model describing the catalytic activity of the crystal facets exposed by the catalyst under reaction conditions. Their microkinetic model well reproduced experimental kinetic data and reaction orders and allowed for a concomitant description of the nature of the catalyst material under reaction conditions and of its catalytic consequences in terms of reactivity.
4.2 CO2 methanation
CO2 methanation is thermodynamically favorable at low temperatures. However, it is limited by kinetics that strongly depends on the selected catalyst. Over the past few years, many kinetic expressions of CO2 methanation over different catalysts have been published.394,396–401 Some of them were based on simple power law models,402,403 while others followed more complex kinetic models.404–407 Most of these models are empirical and do not take into account the approach to equilibrium. Moreover, their parameters were estimated at low CO2 conversions and atmospheric pressure, thus far from the conditions of industrial interest. One of the first detailed mechanistic models was proposed by Weatherbee and Bartholomew408 for the kinetics of a 3% Ni/SiO2 catalyst. However, in their experiments, the gas composition was highly diluted and far away from the one required for direct injection in the gas grid without further purification or separation steps. An important breakthrough was achieved by Kai et al.409 They performed kinetic studies at atmospheric pressure over a La2O3 promoted Ni on alumina catalyst by using both a differential and an integral reactor. With an integral reactor operating at high CO2 conversion, they develop a Langmuir–Hinshelwood rate equation based on the mechanism proposed by Weatherbee and Bartholomew,408 resulting in a model able to describe in detail the influence of the reaction's products on the kinetics. Lunde and Kester165 proposed an empirical model over a Ru-based catalyst potentially able to predict the catalyst activity from differential to thermodynamically limited CO2 conversion. The original expression was derived using a fitting procedure made by the authors to data collected under differential conditions at atmospheric pressure. The same model had been used by other authors to fit experimental data collected in a larger range of CO2 conversions and higher pressures by modifying the kinetic parameters. Falbo et al.410 derived a novel kinetic rate equation from the one proposed by Lunde and Kester to account for the negative dependence on the partial pressure of water, improving the model capability to simulate the catalyst performance in a wide range of process conditions. The kinetics of CO2 methanation over a 10% Ru/γ-Al2O3 catalyst were investigated by Duyar et al.411 using a differential reactor at atmospheric pressure to obtain an empirical rate equation consistent with an Eley–Rideal mechanism where gas phase H2 reacts with surface species resulting from the adsorption of CO2. This implies that excess H2 would be required to boost the reaction rate. Avanesian et al.412 developed a mean-field microkinetic model for the Sabatier reaction based on DFT calculation on Ru(0001) which consisted of 18 elementary steps and was able to successfully predict the experimental data at different temperatures and reactants' partial pressures. However, this model was not able to predict the thermodynamic equilibrium conversion at different temperatures. Raghu and Kaisare413 proposed a mean-field microkinetic model for CO2 methanation at atmospheric pressure over a Ru-based catalyst by using a bottom-up modeling strategy. According to the authors, the model provided a reasonably good prediction of CO2 conversion within the temperature range of interest for the methanation reaction as well as under equilibrium conditions.
4.3 CO2 hydrogenation to methanol
The kinetic modeling of methanol synthesis from CO2/H2 mixtures using industrial Cu-based catalysts had been widely studied in the literature.268,311,414–418 Although it is generally accepted that methanol is primarily formed via CO2 hydrogenation (formate route), the role of the active sites and the effect of different catalyst components are still under debate. A variety of global kinetic models were published in the past years.317,419–422 Power laws and LHHW models were widely used to describe methanol synthesis, first from CO and then from CO2 as the main carbon source. Graaf et al.423 and Vanden Bussche and Froment424,425 were among the first authors to propose an LHHW kinetic model for CO2 hydrogenation to methanol over a commercial Cu/ZnO/Al2O3 catalyst. The main difference between the two models was the active sites involved in the activation of reactants. According to Vanden Bussche and Froment, both H2 and CO2 adsorb on the same type of active site (Cu). The first microkinetic model was proposed by Askgaard et al.426 from results obtained in surface science studies over a Cu(100) single-crystal catalyst and then successfully extrapolated for industrial conditions. Ovesen et al.427 proposed a detailed microkinetic model for methanol synthesis based on the experimental evidence collected by in situ EXAFS. This “dynamic” model was able to describe the change in particle morphology with the change in reaction environment as well as the reaction rate over the three basal Cu surface planes of a Cu/ZnO catalyst, both crucial features to describe the kinetic data measured under industrial conditions. Grabow and Mavrikakis312 conducted an extensive set of periodic, self-consistent DFT calculations over the Cu(111) facet to fit a mean-field microkinetic model to published experimental methanol synthesis rate data under realistic conditions over a commercial ternary Cu catalyst. This model included novel reaction intermediates which allowed for the formation of formic acid, formaldehyde, and methyl formate as by-products. A statistical kinetic model was derived from first-principles density functional theory (DFT) calculations and kinetic Monte Carlo simulations by Tang et al.121 over a Cu/ZrO2 catalyst. Despite the complexity of the reaction system, they were able to model the metal/oxide interface and demonstrate that methanol was produced through both RWGS and formate routes, assessing the contribution of each reaction channel in terms of the reaction rate. In agreement with Tang et al.,121 Ye et al.428 identified in the metal/oxide interface the most active site for CO2 adsorption and hydrogenation. By combining DFT calculations and microkinetic modeling to study CO2 to methanol conversion over a Pd4/In2O3 model catalyst, they demonstrated the dynamical nature of the structure of the supported Pd4 cluster, which transforms in response to the presence of OH in the reaction environment, modifying the main reaction pathway. Chiavassa et al.429 modeled the synthesis of methanol from a CO2/H2 mixture on a Ga2O3–Pd/SiO2 catalyst by combining kinetic information with relevant spectroscopic FT-IR data. They proposed a detailed reaction scheme for the CO2 hydrogenation bifunctional mechanism where reactants' activation occurred on two different active sites, serving as a basis for the development of an LHHW type kinetic model, able to predict the negative influence of CO due to the competitive adsorption with H2 on Pd sites.
4.4 Dry reforming of methane (DRM)
Different kinetic mechanisms for the dry reforming reaction have been published in the last few years.224,430–435 Only a few reports are present in the literature employing the Eley–Rideal (ER) model for DRM on Ni-based catalysts.377 The Langmuir Hinshelwood–Hougen–Watson model (LHHW) has more extensive applications and reports in the literature.436,437 Mhadeshwar et al. and Maestri et al. developed a C1 semiempirical microkinetic model for the conversion of CH4 to syngas on a Rh-based catalyst applying a hierarchical multiscale approach and first-principles calculations.162,438 Both were able to quantitatively predict the behaviors of several C1 reaction systems, including SR, DR, and CPO under different reaction conditions. Delgado et al.439 proposed a different multistep surface kinetic model on a Ni-based catalyst, consisting of 52 elementary steps with 14 surface and 6 gas-phase species. The mechanism was implemented into a 1D model of a fixed bed reactor that is able to describe the conversion of methane with oxygen, steam, and CO2 as well as methanation, WGS, and carbon formation via the Boudouard reaction. Their model has been derived by comparison of numerical simulations with data derived from isothermal experiments in a packed bed reactor, using different inlet gas compositions and operating temperatures (up to 900 °C). Aparicio440 derived a microkinetic model for methane reforming over Ni catalysts with many parameters obtained either from the surface science literature or from fitting the results of transient kinetic experiments. However, the model's predictions were not quantitative, as predicted rates can deviate from the experimental ones, but it could correctly predict activation energies, reaction orders, and the major trends for several reactions, such as steam and dry reforming, CO and CO2 methanation, WGS and RWGS. Foppa et al.225 presented an ab initio mechanistic study of DRM over the flat (111) surfaces of Ni, Pd, and Pt for the development of a microkinetic model made of 16 elementary steps. They provided new insights into intrinsic catalytic activity over the metallic surfaces and evaluated the interplay among all the competitive reactions that can occur under DRM experimental conditions (i.e., SRM, RWGS, methane cracking, and Boudouard reaction). Based on experiments conducted in an oscillating microbalance reactor, Chen et al.441 were able to study the catalyst deactivation due to carbon formation during DRM and proposed an advanced microkinetic model for methane reforming, carbon formation, and deactivation over a Ni-based catalyst. A good agreement with the experimental kinetic data during dry reforming over fresh catalyst and with time on stream was obtained. However, the quantitative description of the deactivation process that affects reforming catalysts due to carbon formation is still an issue. The formulation of a structure-dependent microkinetic model able to simulate carbon formation under DRM conditions and its effect on the overall catalyst activity represents an intriguing challenge for heterogeneous catalysis and scientific research.
5. Reactor technologies
In CO2 conversion technologies, the design of chemical reactors is very important for its key involvement in controlling both the thermodynamic and kinetic aspects of the processes. Significant efforts have been undertaken to enhance the catalytic activity on various reactor systems starting from fixed to fluidized beds and then to multistage reactors.57,442
The reactions considered in this work are both endothermic (e.g., DRM, RWGS) and exothermic (e.g., CO2 methanation, CO2 hydrogenation to methanol). Thus, the reactor design faces different problems depending on the reaction considered but presents similarities when dealing with heat management. Exothermic reactions present heat dissipation and possible hotspot problems. The classic design of fixed bed reactors utilized in industry for several years, such as multistage adiabatic reactors and multi-tubular fixed bed reactors, presents a convenient mechanical design, low maintenance cost, high productivity per unit of volume, and limited residence time distribution of reactants and products in the reactor, in addition to the secular know-how in the construction and management. Nevertheless, such reactors present large axial and radial temperature gradients with possible hotspots, which are important issues.443 Recently, multi-tubular shell cooled reactors typically used in industry have been studied for the methanation reaction, with interesting results concerning the optimization of the operating conditions.444 The interest in structured reactors is growing due to good heat management performances, high surface-to-volume ratio, and more efficient use of the catalyst. The use of microchannel reactors provides an interesting solution for the management of heat transfer and also for improved gas/solid contact compared to traditional reactor design.445,446 Packed bed foams show a promising role in intensifying processes and improve the heat management of strongly exothermic/endothermic reactions, with fundamental studies that are paving the way towards the improvement in the design of such reactors.447,448 The use of highly conductive Ru/Cex/Ni foams has been investigated for the methanation reaction, demonstrating promise for the management of highly exothermic catalytic reactions.449 Depending on the materials used for the structure and/or for the catalysts, different techniques exist and should be approached to identify the best one, with possible clogging and material coating feasibility that must be taken into account.450 Fluidized bed reactors are another technology already used in some industrial processes, due to the almost perfect heat management, with small or even negligible temperature gradients, thanks to the fluidization of the bed. Nevertheless, such technology suffers from the intrinsic complexity of the management of fluidization and of the broad residence time distribution. In this view, several efforts are made to increase the know-how of such technology employing advanced CFD simulations451 and improve their design in CO2 hydrogenation processes.452,453 Recently, plasma reactors have attracted attention in the hydrogenation of CO2, mainly because of their capability to run the reaction at low temperatures.187 This technology can involve electrically-based plasma454 or the addition of plasma in the gas-phase.455,456
Endothermic reactions present heat supply problems, which can result in the formation of cold spots in the reactors and decrease the process performance. Such problems are usually addressed by technologies similar to the ones for exothermic reactions, where the heat is usually supplied by boilers. Recently, new solutions to provide heat to the chemical reactions have been proposed, including the use of microwaves457 and electrified reactors.458,459 The electrification of reactors is a very promising technology, and it has been investigated both for the steam and dry reforming of methane,458,459 showing good energetic efficiency, increasing catalyst utilization, and reducing the thermal gradient in the reactor. Steady and consistent power supply, thermo-mechanical and physical properties of materials, and energy efficiency represent the main challenges when considering electrified reactors.458–460
Besides heat management, thermodynamics is an intrinsic limit of some of these processes. To increase thermodynamic equilibrium yields, suitable pressure and temperature should be chosen, but convenient designs help to achieve good yields with less severe operating conditions (e.g., lower pressure). Product removal is an increasingly feasible opportunity with several solutions proposed. The separation of CO production and subsequent methanol production has proven to be a feasible technology, with the intermediate separation of water with a separating unit (Fig. 10.a).88,462 Selective membranes187,463 are a promising technology to separate water to push the yield beyond thermodynamic limitations (Fig. 10.b). Polymeric membranes can be employed but suffer from problems at high temperatures and pressures.464 Zeolite membranes seem to offer a valid alternative, overcoming such problems, being stable at relatively high pressures and temperatures, and showing a relevant yield increase compared to traditional reactors.465–467 Stable and efficient materials for membranes at process temperatures, pressures, and compositions represent a challenge for membrane reactors.58 Moreover, separating water is challenging due to the similar kinetic diameters of H2O, CH3OH, CO2, and H2 (0.30 nm, 0.38 nm, 0.33 nm, and 0.29 nm, respectively).468 Sorption enhanced processes (Fig. 10.c) are another breakthrough technology, allowing thermodynamic limitations to be overcome by the use of an adsorbent material that removes water from the reaction environment, and have applications either in methanol production or in higher alcohols production.469,470 The adsorption of water simulating a complete process proved to increase the methanol productivity by 15% under practically relevant operating conditions.471 Recently, sorption enhanced methanol production from CO2 hydrogenation has provided outlet methanol concentrations up to 290% of the one without the sorption enhancement effect at 60 bar.472 To improve methanol production from carbon dioxide at low temperatures, magnetic field-assisted reactors are being investigated, showing positive effects on CO2 conversion and selectivity towards methanol.187,473 The presence of carbon-producing reactants and/or intermediates and/or products is another trivial challenge for some of these reactions (e.g., DRM) as mentioned above. Even if catalyst design is the key to overcome such a problem, the operating conditions must be properly chosen to find the optimum between yield and catalyst deactivation, to maximize the profitability of the process.
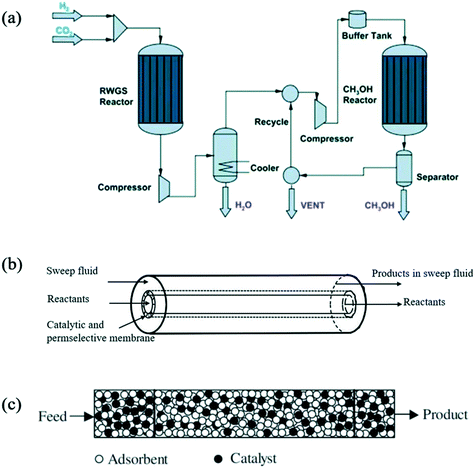 |
| Fig. 10 Possible solutions to suppress thermodynamic equilibrium limitations in CO2 conversion reactions. (a) CAMERE process (carbon dioxide hydrogenation to form methanol via a reverse-water-gas-shift reaction). Reproduced with permission from ref. 88. (b) Membrane reactor. (c) Sorption enhanced reactor. Reproduced with permission from ref. 461. | |
6. Summary and outlook
CO2 is an essential molecule for our life on Earth. It plays a crucial role in the carbon-cycle which is at the basis of the mechanism of storing solar energy in chemical bonds. Moreover, thanks to its green-house properties, it affects the thermoregulation of the Earth. Without the presence of CO2 in the atmosphere, the average temperature of the planet would be considerably lower than the values required for life and the environment as we know them. The intensive use of fossil fuels made in the last two centuries has allowed a dramatic improvement in the quality of life thanks to the availability of enormous quantities of energy at high energy and power densities. On the flip side of the same coin, the exploitation of the energy “trapped” in the chemical bonds of the fossil fuels has released the CO2 originally used in the photosynthesis process to store the solar energy in the chemical bonds. As a result of these processes and together with the rapid growth of production of materials such as cement, iron, and steel (CO2 intensive processes), a rapid increase of the concentration of the CO2 in the atmosphere from 300 pm (in the pre-industrial period) to values higher than 400 ppm has been observed. The IPCC demonstrated that this higher concentration of CO2 can be explained with 95% confidence only by accounting for the anthropogenic activities of the last two centuries. As such, the increase of the temperature of the Earth with all the consequent effects on our life and the environment is directly linked with human activities.
Therefore, policymakers are taking action to achieve net-zero CO2 emissions. Solving the problem by simply eliminating the need for fossil fuels as an energy source is not straightforward since fossil resources are the basis of our current chemical and energy industry. Moreover, they are difficult to replace due to their huge energy densities. A promising concept is to replace the fossil feedstocks currently used in the chemical and energy industry with sustainably produced base chemicals and fuels by reducing CO2 using renewable energy (e.g., H2 from water electrolysis with electricity from wind or solar energy – “green hydrogen”) for the production of commercially important fuels and chemicals by means of electrocatalysis, photocatalysis, or thermal catalysis routes. This would result in a carbon-neutral technology of energy transformation and storage, since CO2 consumption and production would occur at comparable characteristic times.
In this review, we have reported an in-depth survey of the state-of-the-art mechanistic and multiscale aspects of CO2 conversion to C1 products via thermo-catalysis. Though there are many chemicals in the domain of CO2 activation, hydrogenation products such as CO (RWGS and dry reforming routes), methane (methanation reaction) and methanol are industrially and economically highly relevant.
Catalyst design requires in-depth understanding of the reaction mechanism and surface characterization techniques. However, due to the formation of multiple products and lack in development of effective in situ probing techniques for thermocatalytic CO2 hydrogenation, elucidating the reaction mechanism and separation of product mixtures are difficult. Insights from different techniques have been reported, spanning from theoretical calculations to spectroscopy studies and kinetic investigations. We reviewed the main materials used to thermo-catalytically activate CO2 (a very thermodynamically stable molecule) and the main conclusions and hypothesis on the activation mechanisms and elementary pathways. The systematic understanding of the reaction mechanism with key intermediate formation and identification of surface-active sites of the catalysts are of great help in establishing the CO2 activation process and obtained desired conversion. A significant role of the catalyst's shape was also reported. This finding could lead to rational design of catalysts and judicious plans of new nano-catalysts for potential use in carbon dioxide hydrogenation. Particular attention has been paid to catalyst deactivation due to coke formation that is a major technological and scientific challenge, requiring innovations in materials and process design to combat. Deactivation is mainly caused by filamentous carbon as it has high mechanical strength that leads to mechanical deformation of the catalyst and blocks metal active sites. We then reviewed the main kinetic schemes reported in the literature (both detailed microkinetic models based on first-principles calculations and rate equations): the main open area of progress for kinetic modeling in this context is to couple the description of the kinetic events with coke formation and its kinetic consequences. Design, innovations, and engineering of catalytic materials including reactors and process technologies are vital solutions, but such approaches are yet to be established for commercial success. Integrating reactor design and developing more effective and selective catalysts may solve scientific and technological challenges by reducing the activation barrier in an energy efficient process.
Conflicts of interest
There are no conflicts to declare.
Acknowledgements
The authors acknowledge funding under the European Union's Horizon 2020 research and innovation program from the European Research Council, ERC (Grant agreement No. 677423/SHAPE: “Structure Dependent Microkinetic Modelling of Heterogeneous Catalytic Processes”) and from the MSCA-IF (Grant agreement No. 846255/Biogas2Syngas: “Rational Design for Coke-resistant Dry Reforming Catalyst using Combined Theory and Operando Raman Experiments”).
References
- Statistical Review of World Energy, 2020.
- International Energy Outlook 2013, 2013.
- M. I. Hoffert, K. Caldeira, A. K. J. Jain, E. F. Haites, L. D. D. D. Harvey, S. D. Potter, S. H. Schlesinger, R. G. Watts, T. M. L. Wigley and D. J. Wuebbles, Nature, 1998, 395, 881–884 CrossRef CAS
.
- Annual European Union greenhouse gas inventory 1990–2018 and inventory report 2020 Submission to the UNFCCC Secretariat 27 May 2020.
- J. A. Martens, A. Bogaerts, N. De Kimpe and P. A. Jacobs, ChemSusChem, 2017, 10, 1039–1055 CrossRef CAS PubMed
.
-
T. F. Stocker, D. Qin, G.-K. Plattner, M. M. B. Tignor, S. K. Allen, J. Boschung, A. Nauels, Y. Xia, V. Bex and P. M. Midgley, IPCC 2013. Climate Change 2013: The Physical Science Basis. Contribution of Working Group I to the Fifth Assessment Report of the Intergovernmental Panel on Climate Change, Cambridge University Press, 2014, DOI:10.1017/CBO9781107415324.
- A. W. Kleij, M. North and A. Urakawa, ChemSusChem, 2017, 10, 1036–1038 CrossRef CAS PubMed
.
-
G. Pacchioni, W la CO2. Possiamo trasformare il piombo in oro?, Il Mulino, 2021.
- U.S. Global Change Research Program, Third Climate Assessment, 2014.
- S. A. Kulp and B. H. Strauss, Nat. Commun., 2019, 10, 4844 CrossRef CAS PubMed
.
-
D. J. Wuebbles, D. W. Fahey, K. A. Hibbard, D. J. Dokken, B. C. Stewart and T. K. Maycock, USGCRP, 2017: Climate Science Special Report: Fourth National Climate Assessment, Volume I, U.S. Global Change Research Program, Washington, DC, USA, DOI:10.7930/J0J964J6.
- C. McGlade and P. Ekins, Nature, 2015, 517, 187–190 CrossRef CAS PubMed
.
- M. Höök and X. Tang, Energy Policy, 2013, 52, 797–809 CrossRef
.
-
U. Riebesell, in Encyclopedia of Earth Sciences Series, 2016, vol. Part 2, pp. 541–542 Search PubMed
.
- E. R. H. Moore, M. R. Smith, D. Humphries, R. Dubrow and S. S. Myers, Challenges, 2020, 11, 4 CrossRef
.
- P. Akhter, M. A. Farkhondehfal, S. Hernández, M. Hussain, A. Fina, G. Saracco, A. U. Khan and N. Russo, J. Environ. Chem. Eng., 2016, 4, 3934–3953 CrossRef CAS
.
- A. R. Brandt, A. Millard-Ball, M. Ganser and S. M. Gorelick, Environ. Sci. Technol., 2013, 47, 8031–8041 CrossRef CAS PubMed
.
- S. Perathoner, K. M. Van Geem, G. B. Marin and G. Centi, Chem. Commun., 2021 10.1039/d1cc03154f
.
-
J. K. Nørskov, B. Weckhuysen, G. Centi, S. R. ChorkendorffI, G. Marin, A. Grimaud, J. Rossmeisl, P. Strasser, M. Koper and B. Roldan, Energy X.
- G. Centi and S. Perathoner, Catal. Today, 2009, 148, 191–205 CrossRef CAS
.
- H. Y. Yang, B. L. Bao, J. Liu, Y. Qin, Y. R. Wang, K. Z. Su, J. C. Han and Y. Mu, Bioelectrochemistry, 2018, 119, 180–188 CrossRef CAS PubMed
.
- S. K. M. R. Rittmann, A. H. Seifert and S. Bernacchi, Appl. Energy, 2018, 216, 751–760 CrossRef CAS
.
- J. J. Leung, J. Warnan, K. H. Ly, N. Heidary, D. H. Nam, M. F. Kuehnel and E. Reisner, Nat. Catal., 2019, 2, 354–365 CrossRef CAS
.
- D. Yang, H. Yu, T. He, S. Zuo, X. Liu, H. Yang, B. Ni, H. Li, L. Gu, D. Wang and X. Wang, Nat. Commun., 2019, 10, 1–10 CrossRef PubMed
.
- J. Chen, J. Yin, X. Zheng, H. Ait Ahsaine, Y. Zhou, C. Dong, O. F. Mohammed, K. Takanabe and O. M. Bakr, ACS Energy Lett., 2019, 4, 1279–1286 CrossRef CAS
.
- X. Deng, R. Li, S. Wu, L. Wang, J. Hu, J. Ma, W. Jiang, N. Zhang, X. Zheng, C. Gao, L. Wang, Q. Zhang, J. Zhu and Y. Xiong, J. Am. Chem. Soc., 2019, 141, 10924–10929 CrossRef CAS PubMed
.
- H. Y. Kang, D. H. Nam, K. D. Yang, W. Joo, H. Kwak, H. H. Kim, S. H. Hong, K. T. Nam and Y. C. Joo, ACS Nano, 2018, 12, 8187–8196 CrossRef CAS PubMed
.
- B. B. Cardias, M. G. de Morais and J. A. V. Costa, Bioresour. Technol., 2018, 267, 77–83 CrossRef CAS PubMed
.
- M. Aresta and A. Dibenedetto, Dalton Trans., 2007, 2975–2992 RSC
.
- M. Aresta, A. Dibenedetto and E. Quaranta, J. Catal., 2016, 343, 2–45 CrossRef CAS
.
- S. Zhang, Q. Fan, R. Xia and T. J. Meyer, Acc. Chem. Res., 2020, 53, 255–264 CrossRef CAS PubMed
.
- A. Hasani, M. A. Teklagne, H. H. Do, S. H. Hong, Q. Van Le, S. H. Ahn and S. Y. Kim, Carbon Energy, 2020, 2, 158–175 CrossRef CAS
.
- L. Fan, C. Xia, P. Zhu, Y. Lu and H. Wang, Nat. Commun., 2020, 11, 1–9 Search PubMed
.
- M. Wang, K. Torbensen, D. Salvatore, S. Ren, D. Joulié, F. Dumoulin, D. Mendoza, B. Lassalle-Kaiser, U. Işci, C. P. Berlinguette and M. Robert, Nat. Commun., 2019, 10, 1–8 CrossRef PubMed
.
- M. D. Hossain, Y. Huang, T. H. Yu, W. A. Goddard and Z. Luo, Nat. Commun., 2020, 11, 1–14 Search PubMed
.
- F. P. García de Arquer, C. T. Dinh, A. Ozden, J. Wicks, C. McCallum, A. R. Kirmani, D. H. Nam, C. Gabardo, A. Seifitokaldani, X. Wang, Y. C. Li, F. Li, J. Edwards, L. J. Richter, S. J. Thorpe, D. Sinton and E. H. Sargent, Science, 2020, 367, 661–666 CrossRef PubMed
.
- J. L. White, M. F. Baruch, J. E. Pander, Y. Hu, I. C. Fortmeyer, J. E. Park, T. Zhang, K. Liao, J. Gu, Y. Yan, T. W. Shaw, E. Abelev and A. B. Bocarsly, Chem. Rev., 2015, 115, 12888–12935 CrossRef CAS PubMed
.
- S. Cao, Y. Wang, B. Zhu, G. Xie, J. Yu and J. R. Gong, J. Mater. Chem. A, 2020, 8, 7671–7676 RSC
.
- T. Baran, S. Wojtyła, A. Dibenedetto, M. Aresta and W. Macyk, ChemSusChem, 2016, 9, 2933–2938 CrossRef CAS PubMed
.
- A. Álvarez, M. Borges, J. J. Corral-Pérez, J. G. Olcina, L. Hu, D. Cornu, R. Huang, D. Stoian and A. Urakawa, ChemPhysChem, 2017, 18, 3135–3141 CrossRef PubMed
.
- X. Dong, X. Liu, Y. Chen and M. Zhang, J. CO2 Util., 2018, 24, 64–72 CrossRef CAS
.
- W. Tu, M. Ghoussoub, C. V. Singh and Y. H. C. Chin, J. Am. Chem. Soc., 2017, 139, 6928–6945 CrossRef CAS PubMed
.
- D. L. Tomasko, H. Li, D. Liu, X. Han, M. J. Wingert, L. J. Lee and K. W. Koelling, Ind. Eng. Chem. Res., 2003, 42, 6431–6456 CrossRef CAS
.
-
D. Roberts, These uses of CO2 could cut emissions—and make trillions of dollars.
- D. Y. C. Leung, G. Caramanna and M. M. Maroto-Valer, Renewable Sustainable Energy Rev., 2014, 39, 426–443 CrossRef CAS
.
-
G. C. Nelson, Agric. Trade, Environ. Discov. Meas. Crit. Linkages, 2019, pp. 295–299 Search PubMed.
- S. G. Wang, X. Y. Liao, J. Hu, D. B. Cao, Y. W. Li, J. Wang and H. Jiao, Surf. Sci., 2007, 601, 1271–1284 CrossRef CAS
.
- L. Wang, W. Chen, D. Zhang, Y. Du, R. Amal, S. Qiao, J. Wu and Z. Yin, Chem. Soc. Rev., 2019, 48, 5310–5349 RSC
.
- R. W. Dorner, D. R. Hardy, F. W. Williams and H. D. Willauer, Energy Environ. Sci., 2010, 3, 884–890 RSC
.
- K. Damen, M. Van Troost, A. Faaij and W. Turkenburg, Prog. Energy Combust. Sci., 2006, 32, 215–246 CrossRef CAS
.
- X. M. Liu, G. Q. Lu, Z. F. Yan and J. Beltramini, Ind. Eng. Chem. Res., 2003, 42, 6518–6530 CrossRef CAS
.
- J. A. Rodriguez, P. Liu, D. J. Stacchiola, S. D. Senanayake, M. G. White and J. G. Chen, ACS Catal., 2015, 5, 6696–6706 CrossRef CAS
.
- E. V. Kondratenko, G. Mul, J. Baltrusaitis, G. O. Larrazábal and J. Pérez-Ramírez, Energy Environ. Sci., 2013, 6, 3112–3135 RSC
.
- A. Goeppert, M. Czaun, J. P. Jones, G. K. Surya Prakash and G. A. Olah, Chem. Soc. Rev., 2014, 43, 7995–8048 RSC
.
- D. Qin and J. Lapszewicz, Catal. Today, 1994, 21, 551–560 CrossRef CAS
.
- W. Zhou, K. Cheng, J. Kang, C. Zhou, V. Subramanian, Q. Zhang and Y. Wang, Chem. Soc. Rev., 2019, 48, 3193–3228 RSC
.
- S. Roy, A. Cherevotan and S. C. Peter, ACS Energy Lett., 2018, 3, 1938–1966 CrossRef CAS
.
- X. Jiang, X. Nie, X. Guo, C. Song and J. G. Chen, Chem. Rev., 2020, 120, 7984–8034 CrossRef CAS PubMed
.
- W. Wang and J. Gong, Front. Chem. Sci. Eng., 2011, 5, 2–10 CrossRef CAS
.
- K. Saravanan, H. Ham, N. Tsubaki and J. W. Bae, Appl. Catal., B, 2017, 217, 494–522 CrossRef CAS
.
- Z. Li, J. Wang, Y. Qu, H. Liu, C. Tang, S. Miao, Z. Feng, H. An and C. Li, ACS Catal., 2017, 7, 8544–8548 CrossRef CAS
.
- J. Wei, Q. Ge, R. Yao, Z. Wen, C. Fang, L. Guo, H. Xu and J. Sun, Nat. Commun., 2017, 8, 1–8 CrossRef PubMed
.
- C. Yang, R. Mu, G. Wang, J. Song, H. Tian, Z. J. Zhao and J. Gong, Chem. Sci., 2019, 10, 3161–3167 RSC
.
- E. Alper and O. Yuksel Orhan, Petroleum, 2017, 3, 109–126 CrossRef
.
- O. Martin and J. Pérez-Ramírez, Catal. Sci. Technol., 2013, 3, 3343–3352 RSC
.
- J. T. Sun, I. S. Metcalfe and M. Sahibzada, Ind. Eng. Chem. Res., 1999, 38, 3868–3872 CrossRef CAS
.
- E. D. Goodman, J. A. Schwalbe and M. Cargnello, ACS Catal., 2017, 7, 7156–7173 CrossRef CAS
.
- Y. Dai, P. Lu, Z. Cao, C. T. Campbell and Y. Xia, Chem. Soc. Rev., 2018, 47, 4314–4331 RSC
.
- J. Sehested, J. A. P. Gelten and S. Helveg, Appl. Catal., A, 2006, 309, 237–246 CrossRef CAS
.
- B. Dargatz, J. Gonzalez-Julian, M. Bram, P. Jakes, A. Besmehn, L. Schade, R. Röder, C. Ronning and O. Guillon, J. Eur. Ceram. Soc., 2016, 36, 1207–1220 CrossRef CAS
.
- T. W. Hansen, A. T. Delariva, S. R. Challa and A. K. Datye, Acc. Chem. Res., 2013, 46, 1720–1730 CrossRef CAS PubMed
.
- M. V. Twigg and M. S. Spencer, Top. Catal., 2003, 22, 191–203 CrossRef CAS
.
- M. B. Fichtl, D. Schlereth, N. Jacobsen, I. Kasatkin, J. Schumann, M. Behrens, R. Schlögl and O. Hinrichsen, Appl. Catal., A, 2015, 502, 262–270 CrossRef CAS
.
- S. R. Challa, A. T. Delariva, T. W. Hansen, S. Helveg, J. Sehested, P. L. Hansen, F. Garzon and A. K. Datye, J. Am. Chem. Soc., 2011, 133, 20672–20675 CrossRef CAS PubMed
.
- J. H. Bitter, W. Hally, K. Seshan, J. G. Van Ommen and J. A. Lercher, Catal. Today, 1996, 29, 349–353 CrossRef CAS
.
- J. H. Bitter, K. Seshan and J. A. Lercher, J. Catal., 1999, 183, 336–343 CrossRef CAS
.
- M. Jacquemin, A. Beuls and P. Ruiz, Catal. Today, 2010, 157, 462–466 CrossRef CAS
.
- W. M. Shen, J. A. Dumesic and C. G. Hill, J. Catal., 1981, 68, 152–165 CrossRef CAS
.
- M. Agnelli, M. Kolb and C. Mirodatos, J. Catal., 1994, 148, 9–21 CrossRef CAS
.
- M. Agnelli, H. M. Swaan, C. Marquez-Alvarez, G. A. Martin and C. Mirodatos, J. Catal., 1998, 175, 117–128 CrossRef CAS
.
- M. Mihaylov, K. Hadjiivanov and H. Knözinger, Catal. Lett., 2001, 76, 59–63 CrossRef CAS
.
- P. Munnik, M. E. Z. Velthoen, P. E. de Jongh, K. P. de Jong and C. J. Gommes, Angew. Chem., 2014, 126, 9647–9651 CrossRef
.
- K. S. Park, K. Saravanan, S. J. Park, Y. J. Lee, K. W. Jeon and J. W. Bae, Catal. Sci. Technol., 2017, 7, 4079–4091 RSC
.
- S. M. Kim, J. W. Bae, Y. J. Lee and K. W. Jun, Catal. Commun., 2008, 9, 2269–2273 CrossRef CAS
.
- R. Cheula, A. Soon and M. Maestri, Catal. Sci. Technol., 2018, 8, 3493–3503 RSC
.
- R. Cheula, M. Maestri and G. Mpourmpakis, ACS Catal., 2020, 10, 6149–6158 CrossRef CAS
.
- M. Vyshnepolsky, Z.-B. Ding, P. Srivastava, P. Tesarik, H. Mazhar, M. Maestri and K. Morgenstern, Angew. Chem., Int. Ed., 2021, 133, 18365–18370 CrossRef
.
- G. Centi, E. A. Quadrelli and S. Perathoner, Energy Environ. Sci., 2013, 6, 1711–1731 RSC
.
- E. S. Gutterød, A. Lazzarini, T. Fjermestad, G. Kaur, M. Manzoli, S. Bordiga, S. Svelle, K. P. Lillerud, E. Skúlason, S. Øien-ØDegaard, A. Nova and U. Olsbye, J. Am. Chem. Soc., 2020, 142, 999–1009 CrossRef PubMed
.
- F. Jiang, B. Liu, S. Geng, Y. Xu and X. Liu, Catal. Sci. Technol., 2018, 8, 4097–4107 RSC
.
- L. Wang, E. Guan, Y. Wang, L. Wang, Z. Gong, Y. Cui, X. Meng, B. C. Gates and F. S. Xiao, Nat. Commun., 2020, 11, 1–9 Search PubMed
.
- T. S. Galhardo, A. H. Braga, B. H. Arpini, J. Szanyi, R. V. Gonc, B. F. Zornio, C. R. Miranda and L. M. Rossi, J. Am. Chem. Soc., 2021, 143, 4268–4280 CrossRef CAS PubMed
.
- R. V. Gonçalves, L. L. R. Vono, R. Wojcieszak, C. S. B. Dias, H. Wender, E. Teixeira-Neto and L. M. Rossi, Appl. Catal., B, 2017, 209, 240–246 CrossRef
.
- M. M. Millet, G. Algara-Siller, S. Wrabetz, A. Mazheika, F. Girgsdies, D. Teschner, F. Seitz, A. Tarasov, S. V. Levchenko, R. Schlögl and E. Frei, J. Am. Chem. Soc., 2019, 141, 2451–2461 CrossRef CAS PubMed
.
- M. C. Silaghi, A. Comas-Vives and C. Copéret, ACS Catal., 2016, 6, 4501–4505 CrossRef CAS
.
- M. Roiaz, E. Monachino, C. Dri, M. Greiner, A. Knop-Gericke, R. Schlögl, G. Comelli and E. Vesselli, J. Am. Chem. Soc., 2016, 138, 4146–4154 CrossRef CAS PubMed
.
- J. R. Rostrup-Nielsen, J. Sehested and J. K. Nørskov, Adv. Catal., 2002, 47, 65–139 CAS
.
- S. Kattel, P. Liu and J. G. Chen, J. Am. Chem. Soc., 2017, 139, 9739–9754 CrossRef CAS PubMed
.
- C. Vogt, M. Monai, E. B. Sterk, J. Palle, A. E. M. Melcherts, B. Zijlstra, E. Groeneveld, P. H. Berben, J. M. Boereboom, E. J. M. Hensen, F. Meirer, I. A. W. Filot and B. M. Weckhuysen, Nat. Commun., 2019, 10, 1–10 CrossRef PubMed
.
- X. Chen, X. Su, H. Duan, B. Liang, Y. Huang and T. Zhang, Catal. Today, 2017, 281, 312–318 CrossRef CAS
.
- E. S. Gutterød, S. Øien-ØDegaard, K. Bossers, A. E. Nieuwelink, M. Manzoli, L. Braglia, A. Lazzarini, E. Borfecchia, S. Ahmadigoltapeh, B. Bouchevreau, B. T. Lønstad-Bleken, R. Henry, C. Lamberti, S. Bordiga, B. M. Weckhuysen, K. P. Lillerud and U. Olsbye, Ind. Eng. Chem. Res., 2017, 56, 13206–13218 CrossRef
.
- H. Kusama, K. K. Bando, K. Okabe and H. Arakawa, Appl. Catal., A, 2001, 205, 285–294 CrossRef CAS
.
- Y. Yang, J. Liu, F. Liu and D. Wu, Fuel, 2020, 276, 118093 CrossRef CAS
.
- J. H. Kwak, L. Kovarik and J. Szanyi, ACS Catal., 2013, 3, 2449–2455 CrossRef CAS
.
- A. Aitbekova, L. Wu, C. J. Wrasman, A. Boubnov, A. S. Hoffman, E. D. Goodman, S. R. Bare and M. Cargnello, J. Am. Chem. Soc., 2018, 140, 13736–13745 CrossRef CAS PubMed
.
- S. Tada, O. J. Ochieng, R. Kikuchi, T. Haneda and H. Kameyama, Int. J. Hydrogen Energy, 2014, 39, 10090–10100 CrossRef CAS
.
- X. Shao, X. Yang, J. Xu, S. Liu, S. Miao, X. Liu, X. Su, H. Duan, Y. Huang and T. Zhang, Chem, 2019, 5, 693–705 CAS
.
- N. Austin, B. Butina and G. Mpourmpakis, Prog. Nat. Sci.: Mater. Int., 2016, 26, 487–492 CrossRef CAS
.
- N. Austin, J. Ye and G. Mpourmpakis, Catal. Sci. Technol., 2017, 7, 2245–2251 RSC
.
- J. Dean, Y. Yang, N. Austin, G. Veser and G. Mpourmpakis, ChemSusChem, 2018, 11, 1169–1178 CrossRef CAS PubMed
.
- M. Dixit, X. Peng, M. D. Porosoff, H. D. Willauer and G. Mpourmpakis, Catal. Sci. Technol., 2017, 7, 5521–5529 RSC
.
- R. Cheula and M. Maestri, Catal. Today, 2021 DOI:10.1016/j.cattod.2021.05.016
.
- J. Cai, Y. Han, S. Chen, E. J. Crumlin, B. Yang, Y. Li and Z. Liu, J. Phys. Chem. C, 2019, 123, 12176–12182 CrossRef CAS
.
- M. Zhang, B. Zijlstra, I. A. W. Filot, F. Li, H. Wang, J. Li and E. J. M. Hensen, Can. J. Chem. Eng., 2020, 98, 740–748 CrossRef CAS
.
- R. A. Van Santen, Acc. Chem. Res., 2009, 42, 57–66 CrossRef CAS PubMed
.
- I. A. W. Filot, S. G. Shetty, E. J. M. Hensen and R. A. Van Santen, J. Phys. Chem. C, 2011, 115, 14204–14212 CrossRef CAS
.
- M. Mavrikakis, M. Bäumer, H. J. Freund and J. K. Nørskov, Catal. Lett., 2002, 81, 153–156 CrossRef CAS
.
- H. Mansour and E. Iglesia, J. Am. Chem. Soc., 2021, 143, 11582–11594 CrossRef CAS PubMed
.
- M. Zhu, Q. Ge and X. Zhu, Trans. Tianjin Univ., 2020, 26, 172–187 CrossRef CAS
.
- M. González-Castaño, B. Dorneanu and H. Arellano-García, React. Chem. Eng., 2021, 6, 954–976 RSC
.
- Q. L. Tang, Q. J. Hong and Z. P. Liu, J. Catal., 2009, 263, 114–122 CrossRef CAS
.
- N. Ishito, K. Hara, K. Nakajima and A. Fukuoka, J. Energy Chem., 2016, 25, 306–310 CrossRef
.
- D. J. Pettigrew and N. W. Cant, Catal. Lett., 1994, 28, 313–319 CrossRef CAS
.
- N. C. Nelson, L. Chen, D. Meira, L. Kovarik and J. Szanyi, Angew. Chem., Int. Ed., 2020, 59, 17657–17663 CrossRef CAS PubMed
.
- A. Goguet, S. O. Shekhtman, R. Burch, C. Hardacre, F. C. Meunier and G. S. Yablonsky, J. Catal., 2006, 237, 102–110 CrossRef CAS
.
- S. S. Kim, K. H. Park and S. C. Hong, Fuel Process. Technol., 2013, 108, 47–54 CrossRef CAS
.
- Z. Zhao, M. Wang, P. Ma, Y. Zheng, J. Chen, H. Li, X. Zhang, K. Zheng, Q. Kuang and Z. X. Xie, Appl. Catal., B, 2021, 291, 120101 CrossRef CAS
.
- X. Chen, Y. Chen, C. Song, P. Ji, N. Wang, W. Wang and L. Cui, Front. Chem., 2020, 8, 1–21 CrossRef PubMed
.
- M. M. Kauppinen, M. M. Melander, A. S. Bazhenov and K. Honkala, ACS Catal., 2018, 8, 11633–11647 CrossRef CAS
.
- C. Wang, E. Guan, L. Wang, X. Chu, Z. Wu, J. Zhang, Z. Yang, Y. Jiang, L. Zhang, X. Meng, B. C. Gates and F. S. Xiao, J. Am. Chem. Soc., 2019, 141, 8482–8488 CrossRef CAS PubMed
.
- Z. Zheng, C. Sun, R. Dai, S. Wang, X. Wu, X. An and X. Xie, Catal. Sci. Technol., 2015, 5, 2590–2601 RSC
.
- L. F. Bobadilla, J. L. Santos, S. Ivanova, J. A. Odriozola and A. Urakawa, ACS Catal., 2018, 8, 7455–7467 CrossRef CAS
.
- M. Wenzel, N. V. R. Aditya Dharanipragada, V. V. Galvita, H. Poelman, G. B. Marin, L. Rihko-Struckmann and K. Sundmacher, J. CO2 Util., 2017, 17, 60–68 CrossRef CAS
.
- D. H. Kim, S. W. Han, H. S. Yoon and Y. D. Kim, J. Ind. Eng. Chem., 2015, 23, 67–71 CrossRef CAS
.
- R. Mutschler, E. Moioli, W. Luo, N. Gallandat and A. Züttel, J. Catal., 2018, 366, 139–149 CrossRef CAS
.
- X. Zhang, X. Zhu, L. Lin, S. Yao, M. Zhang, X. Liu, X. Wang, Y. W. Li, C. Shi and D. Ma, ACS Catal., 2017, 7, 912–918 CrossRef CAS
.
- S. C. Yang, S. H. Pang, T. P. Sulmonetti, W. N. Su, J. F. Lee, B. J. Hwang and C. W. Jones, ACS Catal., 2018, 8, 12056–12066 CrossRef CAS
.
- J. Ye, Q. Ge and C. J. Liu, Chem. Eng. Sci., 2015, 135, 193–201 CrossRef CAS
.
- S. Sengupta, A. Jha, P. Shende, R. Maskara and A. K. Das, J. Environ. Chem. Eng., 2019, 7, 102911 CrossRef CAS
.
- L. Pastor-Pérez, F. Baibars, E. Le Sache, H. Arellano-García, S. Gu and T. R. Reina, J. CO2 Util., 2017, 21, 423–428 CrossRef
.
- M. Juneau, M. Vonglis, J. Hartvigsen, L. Frost, D. Bayerl, M. Dixit, G. Mpourmpakis, J. R. Morse, J. W. Baldwin, H. D. Willauer and M. D. Porosoff, Energy Environ. Sci., 2020, 13, 2524–2539 RSC
.
- J. Nakamura, J. M. Campbell and C. T. Campbell, J. Chem. Soc., Faraday Trans., 1990, 86, 2725–2734 RSC
.
- C. V. Ovesen, P. Stoltze, J. K. Nørskov and C. T. Campbell, J. Catal., 1992, 134, 445–468 CrossRef CAS
.
- J. Yoshihara and C. T. Campbell, J. Catal., 1996, 161, 776–782 CrossRef CAS
.
- P. Liu and J. A. Rodriguez, J. Chem. Phys., 2007, 126, 164705 CrossRef PubMed
.
- Q. L. Tang, Z. X. Chen and X. He, Surf. Sci., 2009, 603, 2138–2144 CrossRef CAS
.
- A. A. Gokhale, J. A. Dumesic and M. Mavrikakis, J. Am. Chem. Soc., 2008, 130, 1402–1414 CrossRef CAS PubMed
.
- G. Peng, S. J. Sibener, G. C. Schatz and M. Mavrikakis, Surf. Sci., 2012, 606, 1050–1055 CrossRef CAS
.
- C.-S. Chen, W.-H. Cheng and S. Lin, Catal. Lett., 2000, 68, 45–48 CrossRef CAS
.
- E. Vesselli, M. Rizzi, L. de Rogatis, X. Ding, A. Baraldi, G. Comelli, L. Savio, L. Vattuone, M. Rocca, P. Fornasiero, A. Baldereschi and M. Peressi, J. Phys. Chem. Lett., 2010, 1, 402–406 CrossRef CAS
.
- A. Goguet, F. C. Meunier, D. Tibiletti, J. P. Breen and R. Burch, J. Phys. Chem. B, 2004, 108, 20240–20246 CrossRef CAS
.
- X. Su, X. Yang, B. Zhao and Y. Huang, J. Energy Chem., 2017, 26, 854–867 CrossRef
.
- L. Dietz, S. Piccinin and M. Maestri, J. Phys. Chem. C, 2015, 119, 4959–4966 CrossRef CAS
.
- C. Vogt, E. Groeneveld, G. Kamsma, M. Nachtegaal, L. Lu, C. J. Kiely, P. H. Berben, F. Meirer and B. M. Weckhuysen, Nat. Catal., 2018, 1, 127–134 CrossRef CAS
.
- W. Lin, K. M. Stocker and G. C. Schatz, J. Phys. Chem. C, 2016, 120, 23061–23068 CrossRef CAS
.
- L. C. Wang, M. Tahvildar Khazaneh, D. Widmann and R. J. Behm, J. Catal., 2013, 302, 20–30 CrossRef CAS
.
- G. C. Wang and J. Nakamura, J. Phys. Chem. Lett., 2010, 1, 3053–3057 CrossRef CAS
.
- C. Liu, T. R. Cundari and A. K. Wilson, J. Phys. Chem. C, 2012, 116, 5681–5688 CrossRef CAS
.
- A. Donazzi, A. Beretta, G. Groppi and P. Forzatti, J. Catal., 2008, 255, 241–258 CrossRef CAS
.
- A. Donazzi, A. Beretta, G. Groppi and P. Forzatti, J. Catal., 2008, 255, 259–268 CrossRef CAS
.
- M. Maestri and K. Reuter, Chem. Eng. Sci., 2012, 74, 296–299 CrossRef CAS
.
- M. Maestri, D. Livio, A. Beretta and G. Groppi, Ind. Eng. Chem. Res., 2014, 53, 10914–10928 CrossRef CAS
.
- P. Sabatier and J. B. Senderens, J. Chem. Soc., 1902, 82, 333 Search PubMed
.
- W. Wang, S. Wang, X. Ma, J. Gong and W. Wang, Chem. Soc. Rev., 2011, 40, 3703–3727 RSC
.
- P. J. Lunde and F. L. Kester, Ind. Eng. Chem. Process Des. Dev., 1974, 13, 27–33 CrossRef CAS
.
- Y. Wu, J. Lin, Y. Xu, G. Ma, J. Wang and M. Ding, ChemCatChem, 2020, 12, 3553–3559 CrossRef CAS
.
- K. Ravindranathan Thampi, L. Lucarelli and J. Kiwi, Langmuir, 1991, 7, 2642–2648 CrossRef
.
- A. Karelovic and P. Ruiz, ACS Catal., 2013, 3, 2799–2812 CrossRef CAS
.
- H. Y. Kim, H. M. Lee and J. N. Park, J. Phys. Chem. C, 2010, 114, 7128–7131 CrossRef CAS
.
- F. Ocampo, B. Louis and A. C. Roger, Appl. Catal., A, 2009, 369, 90–96 CrossRef CAS
.
- F. Solymosi and A. Erdöhelyi, Stud. Surf. Sci. Catal., 1981, 7, 1448–1449 CrossRef CAS
.
- X. Dong, B. Jin, S. Cao, F. Meng, T. Chen, Q. Ding and C. Tong, Waste Manage., 2020, 107, 244–251 CrossRef CAS PubMed
.
- J. Gao, Y. Wang, Y. Ping, D. Hu, G. Xu, F. Gu and F. Su, RSC Adv., 2012, 2, 2358–2368 RSC
.
- S. Rönsch, J. Schneider, S. Matthischke, M. Schlüter, M. Götz, J. Lefebvre, P. Prabhakaran and S. Bajohr, Fuel, 2016, 166, 276–296 CrossRef
.
- G. Xu, K. Shi, Y. Gao, H. Xu and Y. Wei, J. Mol. Catal. A: Chem., 1999, 147, 47–54 CrossRef CAS
.
- S. Wang and G. Q. M. Lu, Appl. Catal., B, 1998, 16, 269–277 CrossRef CAS
.
- M. González-Castaño, J. C. N. de Miguel, A. Penkova, M. A. Centeno, J. A. Odriozola and H. Arellano-Garcia, Appl. Mater. Today, 2021, 23, 27–30 Search PubMed
.
- Y. Yan, Y. Dai, Y. Yang and A. A. Lapkin, Appl. Catal., B, 2018, 237, 504–512 CrossRef CAS
.
- X. Bai, S. Wang, T. Sun and S. Wang, React. Kinet., Mech. Catal., 2014, 112, 437–451 CrossRef CAS
.
- J. R. Rostrup-Nielsen, K. Pedersen and J. Sehested, Appl. Catal., A, 2007, 330, 134–138 CrossRef CAS
.
- W. Gac, W. Zawadzki, M. Rotko, G. Słowik and M. Greluk, Top. Catal., 2019, 62, 524–534 CrossRef CAS
.
- C. Mirodatos, H. Praliaud and M. Primet, J. Catal., 1987, 107, 275–287 CrossRef CAS
.
- J. Cored, A. García-Ortiz, S. Iborra, M. J. Climent, L. Liu, C. H. Chuang, T. S. Chan, C. Escudero, P. Concepción and A. Corma, J. Am. Chem. Soc., 2019, 141, 19304–19311 CrossRef CAS PubMed
.
- J. Ashok, S. Pati, P. Hongmanorom, Z. Tianxi, C. Junmei and S. Kawi, Catal. Today, 2020, 356, 471–489 CrossRef CAS
.
- Y. Chen, J. Long and Z. Li, Trends Chem., 2019, 1, 459–460 CrossRef CAS
.
- S. Navarro-Jaén, J. C. Navarro, L. F. Bobadilla, M. A. Centeno, O. H. Laguna and J. A. Odriozola, Appl. Surf. Sci., 2019, 483, 750–761 CrossRef
.
- S. Saeidi, S. Najari, V. Hessel, K. Wilson, F. J. Keil, P. Concepción, S. L. Suib and A. E. Rodrigues, Prog. Energy Combust. Sci., 2021, 85, 100905 CrossRef
.
- B. Alrafei, I. Polaert, A. Ledoux and F. Azzolina-Jury, Catal. Today, 2020, 346, 23–33 CrossRef CAS
.
- T. A. Le, J. Kim, J. K. Kang and E. D. Park, Catal. Today, 2020, 348, 80–88 CrossRef CAS
.
- C. Mebrahtu, F. Krebs, S. Perathoner, S. Abate, G. Centi and R. Palkovits, Catal. Sci. Technol., 2018, 8, 1016–1027 RSC
.
- M. A. Serrer, A. Gaur, J. Jelic, S. Weber, C. Fritsch, A. H. Clark, E. Saraçi, F. Studt and J. D. Grunwaldt, Catal. Sci. Technol., 2020, 10, 7542–7554 RSC
.
- T. Franken and A. Heel, J. CO2 Util., 2020, 39, 101175 CrossRef CAS
.
- A. I. Tsiotsias, N. D. Charisiou and I. V. Yentekakis, Nanomaterials, 2021, 21, 1–34 Search PubMed
.
- D. Pandey and G. Deo, J. Ind. Eng. Chem., 2016, 33, 99–107 CrossRef CAS
.
- H. H. Shin, L. Lu, Z. Yang, C. J. Kiely and S. McIntosh, ACS Catal., 2016, 6, 2811–2818 CrossRef CAS
.
- A. Jangam, S. Das, N. Dewangan, P. Hongmanorom, W. M. Hui and S. Kawi, Catal. Today, 2020, 358, 3–29 CrossRef CAS
.
- N. M. Martin, F. Hemmingsson, A. Schaefer, M. Ek, L. R. Merte, U. Hejral, J. Gustafson, M. Skoglundh, A. C. Dippel, O. Gutowski, M. Bauer and P. A. Carlsson, Catal. Sci. Technol., 2019, 9, 1644–1653 RSC
.
- A. Trovarelli, C. de Leitenburg, G. Dolcetti and J. L. Lorca, J. Catal., 1995, 151, 111–124 CrossRef CAS
.
- C. De Leitenburg, A. Trovarelli and J. Kašpar, J. Catal., 1997, 166, 98–107 CrossRef CAS
.
- C. De Leitenburg and A. Trovarelli, J. Catal., 1995, 156, 171–174 CrossRef CAS
.
- Q. Pan, J. Peng, T. Sun, S. Wang and S. Wang, Catal. Commun., 2014, 45, 74–78 CrossRef CAS
.
- F. Ocampo, B. Louis, A. Kiennemann and A. C. Roger, IOP Conf. Ser.: Mater. Sci. Eng., 2011, 19, 012007 Search PubMed
.
- F. Ocampo, B. Louis, L. Kiwi-Minsker and A. C. Roger, Appl. Catal., A, 2011, 392, 36–44 CrossRef CAS
.
- S. Eckle, H. G. Anfang and R. J. Behm, J. Phys. Chem. C, 2011, 115, 1361–1367 CrossRef CAS
.
- A. Beuls, C. Swalus, M. Jacquemin, G. Heyen, A. Karelovic and P. Ruiz, Appl. Catal., B, 2012, 113–114, 2–10 CrossRef CAS
.
- A. Karelovic and P. Ruiz, J. Catal., 2013, 301, 141–153 CrossRef CAS
.
- P. A. U. Aldana, F. Ocampo, K. Kobl, B. Louis, F. Thibault-Starzyk, M. Daturi, P. Bazin, S. Thomas and A. C. Roger, Catal. Today, 2013, 215, 201–207 CrossRef CAS
.
- D. C. Upham, A. R. Derk, S. Sharma, H. Metiu and E. W. McFarland, Catal. Sci. Technol., 2015, 5, 1783–1791 RSC
.
- W. Li, H. Wang, X. Jiang, J. Zhu, Z. Liu, X. Guo and C. Song, RSC Adv., 2018, 8, 7651–7669 RSC
.
- E. Baraj, S. Vagaský, T. Hlinčik, K. Ciahotný and V. Tekáč, Chem. Pap., 2016, 70, 395–403 CAS
.
- J. A. H. Dreyer, P. Li, L. Zhang, G. Khai, R. Zhang, P. H. Sit and W. Yang, Appl. Catal., B, 2017, 219, 715–726 CrossRef CAS
.
- A. Quindimil, U. De-la-torre, B. Pereda-ayo and A. Davó-quiñonero, Catal. Today, 2020, 356, 419–432 CrossRef CAS
.
- D. Qin, D. Xie, H. Zheng, Z. Li, J. Tang and Z. Wei, Catal. Lett., 2021, 151, 2894–2905 CrossRef CAS
.
- L. Falbo, C. G. Visconti, L. Lietti and J. Szanyi, Appl. Catal., B, 2019, 256, 117791 CrossRef CAS
.
- C. Vogt, M. Monai, G. J. Kramer and B. M. Weckhuysen, Nat. Catal., 2019, 2, 188–197 CrossRef CAS
.
- S. E. Olesen, K. J. Andersson, C. D. Damsgaard and I. Chorkendorff, J. Phys. Chem. C, 2017, 121, 15556–15564 CrossRef CAS
.
- J. Barrientos, N. Gonzalez, M. Boutonnet and S. Järås, Top. Catal., 2017, 60, 1276–1284 CrossRef CAS
.
- Y. Song, E. Ozdemir, S. Ramesh, A. Adishev, S. Subramanian, A. Harale, M. Albuali, B. A. Fadhel, A. Jamal, D. Moon, S. H. Choi and C. T. Yavuz, Science, 2020, 781, 777–781 CrossRef PubMed
.
- M. Akri, S. Zhao, X. Li, K. Zang, A. F. Lee, M. A. Isaacs, W. Xi, Y. Gangarajula, J. Luo, Y. Ren, Y. T. Cui, L. Li, Y. Su, X. Pan, W. Wen, Y. Pan, K. Wilson, L. Li, B. Qiao, H. Ishii, Y. F. Liao, A. Wang, X. Wang and T. Zhang, Nat. Commun., 2019, 10, 1–10 CrossRef CAS PubMed
.
- W. Y. Kim, Y. H. Lee, H. Park, Y. H. Choi, M. H. Lee and J. S. Lee, Catal. Sci.
Technol., 2016, 6, 2060–2064 RSC
.
- S. Das, A. Jangam, S. Xi, A. Borgna, K. Hidajat and S. Kawi, ACS Appl. Energy Mater., 2020, 3, 7719–7735 CrossRef CAS
.
- K. Bu, J. Deng, X. Zhang, S. Kuboon, T. Yan, H. Li, L. Shi and D. Zhang, Appl. Catal., B, 2020, 267, 118692 CrossRef CAS
.
- Z. Yao, J. Jiang, Y. Zhao, F. Luan, J. Zhu, Y. Shi, H. Gao and H. Wang, RSC Adv., 2016, 6, 19944–19951 RSC
.
- M. C. J. Bradford and M. A. Vannice, Catal. Rev.: Sci. Eng., 1999, 41, 1–42 CrossRef CAS
.
- L. Foppa, M. C. Silaghi, K. Larmier and A. Comas-Vives, J. Catal., 2016, 343, 196–207 CrossRef CAS
.
- R. K. Singha, A. Yadav, A. Shukla, M. Kumar and R. Bal, Catal. Commun., 2017, 92, 19–22 CrossRef CAS
.
- H. Arandiyan, Y. Wang, J. Scott, S. Mesgari, H. Dai and R. Amal, ACS Appl. Mater. Interfaces, 2018, 10, 16352–16357 CrossRef CAS PubMed
.
- K. Song, M. Lu, S. Xu, C. Chen, Y. Zhan, D. Li, C. Au, L. Jiang and K. Tomishige, Appl. Catal., B, 2018, 239, 324–333 CrossRef CAS
.
- W. L. Vrijburg, G. Garbarino, W. Chen, A. Parastaev, A. Longo, E. A. Pidko and E. J. M. Hensen, J. Catal., 2020, 382, 358–371 CrossRef CAS
.
- C. Fan, Y. A. Zhu, M. L. Yang, Z. J. Sui, X. G. Zhou and D. Chen, Ind. Eng. Chem. Res., 2015, 54, 5901–5913 CrossRef CAS
.
- S. G. Wang, D. B. Cao, Y. W. Li, J. Wang and H. Jiao, J. Phys. Chem. B, 2006, 110, 9976–9983 CrossRef CAS PubMed
.
- J. Niu, X. Du, J. Ran and R. Wang, Appl. Surf. Sci., 2016, 376, 79–90 CrossRef CAS
.
- A. Nandini, K. K. Pant and S. C. Dhingra, Appl. Catal., A, 2006, 308, 119–127 CrossRef CAS
.
- T. Osaki and T. Mori, J. Catal., 2001, 204, 89–97 CrossRef CAS
.
- M. C. J. Bradford and M. A. Vannice, Appl. Catal., A, 1996, 142, 97–122 CrossRef CAS
.
- J. F. Múnera, L. M. Cornaglia, D. V. Cesar, M. Schmal and E. A. Lombardo, Ind. Eng. Chem. Res., 2007, 46, 7543–7549 CrossRef
.
- M. Maestri, D. G. Vlachos, A. Beretta, G. Groppi and E. Tronconi, J. Catal., 2008, 259, 211–222 CrossRef CAS
.
- M. F. Mark, F. Mark and W. F. Maier, Chem. Eng. Technol., 1997, 20, 361–370 CrossRef CAS
.
- J. Wei and E. Iglesia, J. Catal., 2004, 224, 370–383 CrossRef CAS
.
- A. Yamaguchi and E. Iglesia, J. Catal., 2010, 274, 52–63 CrossRef CAS
.
- J. Wei and E. Iglesia, J. Catal., 2004, 225, 116–127 CrossRef CAS
.
- N. Manavi and B. Liu, Catal. Sci. Technol., 2021, 11, 3724–3736 RSC
.
- D. L. Trimm, Catal. Today, 1999, 49, 3–10 CrossRef CAS
.
- J. Rostrup-Nielsen and D. L. Trimm, J. Catal., 1977, 48, 155–165 CrossRef CAS
.
- Z. Wang, X. M. Cao, J. Zhu and P. Hu, J. Catal., 2014, 311, 469–480 CrossRef CAS
.
- M. A. A. Aziz, A. A. Jalil, S. Wongsakulphasatch and D. V. N. Vo, Catal. Sci. Technol., 2020, 10, 35–45 RSC
.
- R. J. Zhang, G. F. Xia, M. F. Li, Y. Wu, H. Nie and D. D. Li, Ranliao Huaxue Xuebao, 2015, 43, 1359–1365 CAS
.
- S. S. Kim, H. H. Lee and S. C. Hong, Appl. Catal., A, 2012, 423–424, 100–107 CAS
.
- M. Ferrandon and T. Krause, Appl. Catal., A, 2006, 311, 135–145 CrossRef CAS
.
- S. Sokolov, E. V. Kondratenko, M. Pohl, A. Barkschat and U. Rodemerck, Appl. Catal., B, 2012, 113–114, 19–30 CrossRef CAS
.
- J. Zhong, X. Yang, Z. Wu, B. Liang, Y. Huang and T. Zhang, Chem. Soc. Rev., 2020, 49, 1385–1413 RSC
.
- F. Calaza, C. Stiehler, Y. Fujimori, M. Sterrer, S. Beeg, M. Ruiz-oses, N. Nilius, M. Heyde, T. Parviainen, K. Honkala, H. Häkkinen and H. Freund, Angew. Chem., Int. Ed., 2015, 54, 12484–12487 CrossRef CAS PubMed
.
- J. Graciani, K. Mudiyanselage, F. Xu, A. E. Baber, J. Evans, S. D. Senanayake, D. J. Stacchiola, P. Liu, J. Hrbek, J. Fernández Sanz and J. A. Rodriguez, Science, 2014, 345, 546–550 CrossRef CAS PubMed
.
- J. C. Frost, Nature, 1988, 334, 577–580 CrossRef CAS
.
- F. Abild-pedersen, F. Studt and T. Bligaard, Proc. Natl. Acad. Sci. U. S. A., 2011, 108, 937–943 CrossRef PubMed
.
- J. Xiao and T. Frauenheim, J. Phys. Chem. C, 2013, 117, 1804–1808 CrossRef CAS
.
- N. Acerbi, S. C. E. Tsang, G. Jones, S. Golunski and P. Collier, Angew. Chem., Int. Ed., 2013, 52, 7737–7741 CrossRef CAS PubMed
.
- M. U. Khan, L. Wang, Z. Liu, Z. Gao, S. Wang, H. Li, W. Zhang, M. Wang, Z. Wang, C. Ma and J. Zeng, Angew. Chem., Int. Ed., 2016, 55, 9548–9552 CrossRef PubMed
.
- M. Behrens, F. Studt, I. Kasatkin, S. Kühl, M. Hävecker, F. Abild-pedersen, S. Zander, F. Girgsdies, P. Kurr, B. Kniep, M. Tovar, R. W. Fischer, J. K. Nørskov and R. Schlögl, Science, 2012, 759, 893–898 CrossRef PubMed
.
- M. Behrens, Angew. Chem., Int. Ed., 2016, 55, 14906–14908 CrossRef CAS PubMed
.
- B. Liang, J. Ma, X. Su, C. Yang, H. Duan, H. Zhou, S. Deng, L. Li and Y. Huang, Ind. Eng. Chem. Res., 2019, 58, 9030–9037 CrossRef CAS
.
- J. Wu, M. Saito, M. Takeuchi and T. Watanabe, Appl. Catal., A, 2001, 218, 235–240 CrossRef CAS
.
- A. Prašnikar, A. Pavlišič, F. Ruiz-Zepeda, J. Kovač and B. Likozar, Ind. Eng. Chem. Res., 2019, 58, 13021–13029 CrossRef
.
- A. Parastaev, V. Muravev, E. Huertas Osta, A. J. F. van Hoof, T. F. Kimpel, N. Kosinov and E. J. M. Hensen, Nat. Catal., 2020, 3, 526–533 CrossRef CAS
.
- E. Lam, J. J. Corral-Pérez, K. Larmier, G. Noh, P. Wolf, A. Comas-Vives, A. Urakawa and C. Copéret, Angew. Chem., 2019, 131, 14127–14134 CrossRef
.
- I. A. Fisher, H. C. Woo and A. T. Bell, Catal. Lett., 1997, 44, 11–17 CrossRef CAS
.
- Z. Shi, Q. Tan, C. Tian, Y. Pan, X. Sun, J. Zhang and D. Wu, J. Catal., 2019, 379, 78–89 CrossRef CAS
.
- K. Larmier, W. C. Liao, S. Tada, E. Lam, R. Verel, A. Bansode, A. Urakawa, A. Comas-Vives and C. Copéret, Angew. Chem., Int. Ed., 2017, 56, 2318–2323 CrossRef CAS PubMed
.
- J. Nakamura, T. Fujitani, S. Kuld, S. Helveg, I. Chorkendorff and J. Sehested, Science, 2017, 357, 1296–1299 CrossRef PubMed
.
- R. Van Den Berg, G. Prieto, G. Korpershoek, L. I. Van Der Wal, A. J. Van Bunningen, S. Lægsgaard-Jørgensen, P. E. De Jongh and K. P. De Jong, Nat. Commun., 2016, 7 DOI:10.1038/ncomms13057
.
- Y. Wang, S. Kattel, W. Gao, K. Li, P. Liu, J. G. Chen and H. Wang, Nat. Commun., 2019, 1166 CrossRef PubMed
.
- Y. Wang, W. Gao, K. Li, W. Na, J. G. Chen, H. Wang, Y. Wang, W. Gao, K. Li, Y. Zheng, Z. Xie and W. Na, Chem, 2019, 10, 1–12 CrossRef
.
- F. Arena, K. Barbera, G. Italiano, G. Bonura, L. Spadaro and F. Frusteri, J. Catal., 2007, 249, 185–194 CrossRef CAS
.
- X. Guo, D. Mao, G. Lu, S. Wang and G. Wu, J. Catal., 2010, 271, 178–185 CrossRef CAS
.
- T. Phongamwong, U. Chantaprasertporn, T. Witoon, T. Numpilai, Y. Poo-arporn, W. Limphirat, W. Donphai, P. Dittanet, M. Chareonpanich and J. Limtrakul, Chem. Eng. J., 2017, 316, 692–703 CrossRef CAS
.
- L. Angelo, K. Kobl, L. M. M. Tejada, Y. Zimmermann, K. Parkhomenko and A. C. Roger, C. R. Chim., 2015, 18, 250–260 CrossRef CAS
.
- F. Arena, G. Italiano, K. Barbera, S. Bordiga, G. Bonura, L. Spadaro and F. Frusteri, Appl. Catal., A, 2008, 350, 16–23 CrossRef CAS
.
- T. Witoon, N. Kachaban, W. Donphai, P. Kidkhunthod, K. Faungnawakij, M. Chareonpanich and J. Limtrakul, Energy Convers. Manage., 2016, 118, 21–31 CrossRef CAS
.
- C. Yang, Z. Ma, N. Zhao, W. Wei, T. Hu and Y. Sun, Catal. Today, 2006, 115, 222–227 CrossRef CAS
.
- P. Gao, F. Li, H. Zhan, N. Zhao, F. Xiao, W. Wei, L. Zhong, H. Wang and Y. Sun, J. Catal., 2013, 298, 51–60 CrossRef CAS
.
- G. Bonura, M. Cordaro, C. Cannilla, F. Arena and F. Frusteri, Appl. Catal., B, 2014, 152–153, 152–161 CrossRef CAS
.
- X. Yang, S. Kattel, S. D. Senanayake, J. A. Boscoboinik, X. Nie, J. Graciani, J. A. Rodriguez, P. Liu, D. J. Stacchiola and J. G. Chen, J. Am. Chem. Soc., 2015, 137, 10104–10107 CrossRef CAS PubMed
.
- A. Cao, Z. Wang, H. Li and J. K. Nørskov, ChemSusChem, 2020, 13, 6160–6181 CrossRef PubMed
.
- M. Dou, M. Zhang and Y. Chen, New J. Chem., 2018, 42, 3293–3300 RSC
.
- A. Tsoukalou, P. M. Abdala, D. Stoian, X. Huang, M. Willinger, A. Fedorov and C. R. Mu, J. Am. Chem. Soc., 2019, 141, 13497–13505 CrossRef CAS PubMed
.
- J. Ye, C. Liu, D. Mei and Q. Ge, ACS Catal., 2013, 3, 1296–1306 CrossRef CAS
.
- O. Martin, A. J. Martín, C. Mondelli, S. Mitchell, T. F. Segawa, R. Hauert, C. Drouilly, D. Curulla-Ferré and J. Pérez-Ramírez, Angew. Chem., Int. Ed., 2016, 55, 6261–6265 CrossRef CAS PubMed
.
- C. Shen, K. Sun, Z. Zhang, N. Rui, X. Jia, D. Mei and C. Liu, ACS Catal., 2021, 11, 4036–4046 CrossRef CAS
.
- M. S. Frei, C. Mondelli, O. V. Safonova, N. López, R. García-muelas, J. Morales-vidal, M. Philipp, J. A. Stewart, D. C. Ferré and J. Pérez-ramírez, Nat. Commun., 2021, 1–9 Search PubMed
.
- C. S. Li, G. Melaet, W. T. Ralston, K. An, C. Brooks, Y. Ye, Y. S. Liu, J. Zhu, J. Guo, S. Alayoglu and G. A. Somorjai, Nat. Commun., 2015, 6, 1–5 CAS
.
- F. Studt, I. Sharafutdinov, F. Abild-Pedersen, C. F. Elkjær, J. S. Hummelshøj, S. Dahl, I. Chorkendorff and J. K. Nørskov, Nat. Chem., 2014, 6, 320–324 CrossRef CAS PubMed
.
- J. Wang, G. Li, Z. Li, C. Tang, Z. Feng, H. An, H. Liu, T. Liu and C. Li, Sci. Adv., 2017, 3, 1–11 Search PubMed
.
- E. M. Fiordaliso, I. Sharafutdinov, H. W. P. Carvalho, J. D. Grunwaldt, T. W. Hansen, I. Chorkendorff, J. B. Wagner and C. D. Damsgaard, ACS Catal., 2015, 5, 5827–5836 CrossRef CAS
.
- J. Ye, Q. Ge and C. Liu, J. Phys. Chem. C, 2012, 116, 7817–7825 CrossRef CAS
.
- G. Korotcenkov, I. Boris, V. Brinzari, V. Golovanov, Y. Lychkovsky, G. Karkotsky, A. Cornet, E. Rossinyol, J. Rodrigue and A. Cirera, Sens. Actuators, B, 2004, 103, 13–22 CrossRef CAS
.
- K. Soulantica, L. Erades, M. Sauvan, F. Senocq, A. Maisonnat and B. Chaudret, Adv. Funct. Mater., 2003, 13, 553–557 CrossRef CAS
.
- K. Sun, Z. Fan, J. Ye, J. Yan, Q. Ge, Y. Li, W. He, W. Yang and C. J. Liu, J. CO2 Util., 2015, 12, 1–6 CrossRef CAS
.
- M. Zhang, M. Dou and Y. Yu, Appl. Surf. Sci., 2018, 433, 780–789 CrossRef CAS
.
- F. Arena, G. Mezzatesta, G. Zafarana, G. Trunfio, F. Frusteri and L. Spadaro, J. Catal., 2013, 300, 141–151 CrossRef CAS
.
- R. Gaikwad, A. Bansode and A. Urakawa, J. Catal., 2016, 343, 127–132 CrossRef CAS
.
- Z. Wu, J. Cole, H. L. Fang, M. Qin and Z. He, Journal of Advances in Nanomaterials, 2017, 2, 1–10 Search PubMed
.
- Y. Yang, J. Evans, J. A. Rodriguez, M. G. White and P. Liu, Phys. Chem. Chem. Phys., 2010, 12, 9909–9917 RSC
.
- J. Zhu, Y. Su, J. Chai, V. Muravev, N. Kosinov and E. J. M. Hensen, ACS Catal., 2020, 10, 11532–11544 CrossRef CAS
.
- T. Lunkenbein, J. Schumann, M. Behrens, R. Schlögl and M. G. Willinger, Angew. Chem., 2015, 127, 4627–4631 CrossRef
.
- J. Nakamura, Y. Choi and T. Fujitani, Top. Catal., 2003, 22, 277–285 CrossRef CAS
.
- S. Fujita, M. Usui, E. Ohara and N. Takezawa, Catal. Lett., 1992, 13, 349–358 CrossRef CAS
.
- D. Laudenschleger, H. Ruland and M. Muhler, Nat. Commun., 2020, 11, 3898 CrossRef PubMed
.
- P. A. Taylor, P. B. Rasmussen and I. Chorkendorff, J. Chem. Soc., Faraday Trans., 1995, 91, 1267–1269 RSC
.
- Y. Yang, C. A. Mims, R. S. Disselkamp, J. H. Kwak, C. H. F. Peden and C. T. Campbell, J. Phys. Chem. C, 2010, 114, 17205–17211 CrossRef CAS
.
- M. Bowker, R. A. Hadden, H. Houghton, J. N. K. Hyland and K. C. Waugh, J. Catal., 1988, 109, 263–273 CrossRef CAS
.
- Y. F. Zhao, Y. Yang, C. Mims, C. H. F. Peden, J. Li and D. Mei, J. Catal., 2011, 281, 199–211 CrossRef CAS
.
- L. C. Grabow and M. Mavrikakis, ACS Catal., 2011, 1, 365–384 CrossRef CAS
.
- G. C. Chinchen, C. Plant, M. S. Spencer and D. A. Whan, Surf. Sci., 1987, 184, L370–L374 CrossRef CAS
.
- M. D. Higham, M. D. Higham, M. G. Quesne, M. G. Quesne, C. R. A. Catlow, C. R. A. Catlow and C. R. A. Catlow, Dalton Trans., 2020, 49, 8478–8497 RSC
.
- P. B. Rasmussen, P. M. Holmblad, T. Askgaard, C. V. Ovesen, P. Stoltze, J. K. Nørskov and I. Chorkendorff, Catal. Lett., 1994, 26, 373–381 CrossRef CAS
.
- P. B. Rasmussen, M. Kazuta and I. Chorkendorff, Surf. Sci., 1994, 318, 267–280 CrossRef CAS
.
- A. Cao, Z. Wang, H. Li and J. K. Nørskov, ACS Catal., 2021, 11, 1780–1786 CrossRef CAS
.
- Z. Zhou, B. Qin, S. Li and Y. Sun, Phys. Chem. Chem. Phys., 2021, 23, 1888–1895 RSC
.
- S. Dang, B. Qin, Y. Yang, H. Wang, J. Cai, Y. Han, S. Li, P. Gao and Y. Sun, Sci. Adv., 2020, 6 DOI:10.1126/sciadv.aaz2060
.
- M. Yang, Z. Lingjun, Z. Xiaonan, R. Prasert and W. Shurong, J. CO2 Util., 2020, 42, 101304 CrossRef CAS
.
- W. A. Wan Abu Bakar, R. Ali and N. S. Mohammad, Arabian J. Chem., 2015, 8, 632–643 CrossRef CAS
.
- B. R. Powell and S. E. Whittington, J. Catal., 1983, 81, 382–393 CrossRef CAS
.
- S. De Llobet, J. L. Pinilla, M. J. Lazaro, R. Moliner and I. Suelves, Fuel, 2013, 111, 778–783 CrossRef CAS
.
- V. Pawar, D. Ray, C. Subrahmanyam and V. M. Janardhanan, Energy Fuels, 2015, 29, 8047–8052 CrossRef CAS
.
- P. G. Menon, J. Mol. Catal., 1990, 59, 207–220 CrossRef CAS
.
- J. G. McCarty, P. Y. Hou, D. Sheridan and H. Wise, ACS Symp. Ser., 1982, 253–282 CAS
.
- C. H. Bartholomew, Catal. Rev.: Sci. Eng., 1982, 24, 67–112 CrossRef CAS
.
- D. L. Trimm, Catal. Today, 1997, 37, 233–238 CrossRef CAS
.
- J. R. Rostrup-Nielsen, Catal. Today, 1993, 18, 305–324 CrossRef
.
- J. R. Rostrup-Nielsen and J. H. Bak Hansen, J. Catal., 1993, 144, 38–49 CrossRef CAS
.
- S. L. Leung, J. Wei, W. L. Holstein, M. Avalos-Borja and E. Iglesia, J. Phys. Chem. C, 2020, 124, 20143–20160 CrossRef CAS
.
- H. S. Bengaard, J. K. Nørskov, J. Sehested, B. S. Clausen, L. P. Nielsen, A. M. Molenbroek and J. R. Rostrup-Nielsen, J. Catal., 2002, 209, 365–384 CrossRef CAS
.
-
L. Guczi and A. Erdöhelyi, Catalysis for alternative energy generation, 2011, vol. 9781461403 Search PubMed
.
- S. Wang, G. Q. Lu and G. J. Millar, Energy Fuels, 1996, 10, 896–904 CrossRef CAS
.
- A. M. Gadalla and M. E. Sommer, Chem. Eng. Sci., 1989, 44, 2825–2829 CrossRef CAS
.
- A. Goguet, F. Meunier, J. P. Breen, R. Burch, M. I. Petch and A. Faur Ghenciu, J. Catal., 2004, 226, 382–392 CrossRef CAS
.
- J. Witte, A. Calbry-Muzyka, T. Wieseler, P. Hottinger, S. M. A. Biollaz and T. J. Schildhauer, Appl. Energy, 2019, 240, 359–371 CrossRef CAS
.
- A. Erdöhelyi, M. Pásztor and F. Solymosi, J. Catal., 1986, 98, 166–177 CrossRef
.
- F. Solymosi, A. Erdöhelyi and M. Kocsis, J. Chem. Soc., Faraday Trans. 1, 1981, 77, 1003–1012 RSC
.
- F. Pompeo, N. N. Nichio, O. A. Ferretti and D. Resasco, Int. J. Hydrogen Energy, 2005, 30, 1399–1405 CrossRef CAS
.
- J. L. Figueiredo and D. L. Trimm, J. Catal., 1975, 40, 154–159 CrossRef CAS
.
- D. L. Trimm, Catal. Rev.: Sci. Eng., 1977, 16, 155–189 CrossRef CAS
.
- M. Zhu, P. Tian, M. E. Ford, J. Chen, J. Xu, Y.-F. Han and I. E. Wachs, ACS Catal., 2020, 10, 7857–7863 CrossRef CAS
.
- J. Gödde, M. Merko, W. Xia and M. Muhler, J. Energy Chem., 2021, 54, 323–331 CrossRef
.
- X. Yan, T. Hu, P. Liu, S. Li, B. Zhao, Q. Zhang, W. Jiao, S. Chen, P. Wang, J. Lu, L. Fan, X. Deng and Y. X. Pan, Appl. Catal., B, 2019, 246, 221–231 CrossRef CAS
.
- G. H. Lai, J. H. Lak and D. H. Tsai, ACS Appl. Energy Mater., 2019, 2, 7963–7971 CrossRef CAS
.
- S. Kawi, Y. Kathiraser, J. Ni, U. Oemar and Z. Li, ChemSusChem, 2015, 8, 3556–3575 CrossRef CAS PubMed
.
- K. Tomishige, D. Li, M. Tamura and Y. Nakagawa, Catal. Sci. Technol., 2017, 7, 3952–3979 RSC
.
- Z. Bian, S. Das, M. H. Wai, P. Hongmanorom and S. Kawi, ChemPhysChem, 2017, 18, 3117–3134 CrossRef CAS PubMed
.
- P. Djinović and A. Pintar, Appl. Catal., B, 2017, 206, 675–682 CrossRef
.
- D. Li, Y. Nakagawa and K. Tomishige, Appl. Catal., A, 2011, 408, 1–24 CrossRef CAS
.
- J. Dou, R. Zhang, X. Hao, Z. Bao, T. Wu, B. Wang and F. Yu, Appl. Catal., B, 2019, 254, 612–623 CrossRef CAS
.
- A. P. Tathod, N. Hayek, D. Shpasser, D. S. A. Simakov and O. M. Gazit, Appl. Catal., B, 2019, 249, 106–115 CrossRef CAS
.
- Z. Li, S. Das, P. Hongmanorom, N. Dewangan, M. H. Wai and S. Kawi, Catal. Sci. Technol., 2018, 8, 2763–2778 RSC
.
- H. Peng, X. Zhang, X. Han, X. You, S. Lin, H. Chen, W. Liu, X. Wang, N. Zhang, Z. Wang, P. Wu, H. Zhu and S. Dai, ACS Catal., 2019, 9, 9072–9080 CrossRef CAS
.
- Z. Li, Z. Wang and S. Kawi, ChemCatChem, 2019, 11, 202–224 CrossRef CAS
.
- Z. Bian, I. Y. Suryawinata and S. Kawi, Appl. Catal., B, 2016, 195, 1–8 CrossRef CAS
.
- R. K. Singha, A. Yadav, A. Agrawal, A. Shukla, S. Adak, T. Sasaki and R. Bal, Appl. Catal., B, 2016, 191, 165–178 CrossRef CAS
.
- L. Li, L. Zhou, S. Ould-Chikh, D. H. Anjum, M. B. Kanoun, J. Scaranto, M. N. Hedhili, S. Khalid, P. V. Laveille, L. D'Souza, A. Clo and J. M. Basset, ChemCatChem, 2015, 7, 819–829 CrossRef CAS
.
- X. Du, D. Zhang, L. Shi, R. Gao and J. Zhang, Nanoscale, 2013, 5, 2659–2663 RSC
.
- C. Zhang, W. Zhu, S. Li, G. Wu, X. Ma, X. Wang and J. Gong, Chem. Commun., 2013, 49, 9383–9385 RSC
.
- J. Wang, Y. Fu, W. Kong, F. Jin, J. Bai, J. Zhang and Y. Sun, Appl. Catal., B, 2021, 282, 119546 CrossRef CAS
.
- M. Lu, X. Zhang, J. Deng, S. Kuboon, K. Faungnawakij, S. Xiao and D. Zhang, Catal. Sci. Technol., 2020, 10, 4237–4244 RSC
.
- X. Wang, Y. Liu, K. Liu, J. Zhang and J. Wei, Int. J. Hydrogen Energy, 2020, 45, 28325–28336 CrossRef CAS
.
- X. Chen, L. Yin, K. Long, H. Sun, M. Sun, H. Wang, Q. Zhang and P. Ning, J. Energy Inst., 2020, 93, 2255–2263 CrossRef CAS
.
- W. Y. Kim, B. J. Lee, H. Park, Y. H. Choi, J. H. Kim and J. S. Lee, ChemCatChem, 2018, 10, 2214–2218 CrossRef CAS
.
- T. Zhang, Z. Liu, Y. A. Zhu, Z. Liu, Z. Sui, K. Zhu and X. Zhou, Appl. Catal., B, 2020, 264, 118497 CrossRef CAS
.
- R. Thakur, A. Vahidmohammadi, J. Smith, M. Hoffman, J. Moncada, M. Beidaghi and C. A. Carrero, ACS Catal., 2020, 10, 5124–5134 CrossRef CAS
.
- E. Nikolla, A. Holewinski, J. Schwank and S. Linic, J. Am. Chem. Soc., 2006, 128, 11354–11355 CrossRef CAS PubMed
.
- F. Besenbacher, I. Chorkendorff, B. S. Clausen, B. Hammer, A. M. Molenbroek, J. K. Nørskov and I. Stensgaard, Science, 1998, 279, 1913–1915 CrossRef CAS PubMed
.
- J. Juan-Juan, M. C. Román-Martínez and M. J. Illán-Gómez, Appl. Catal., A, 2006, 301, 9–15 CrossRef CAS
.
- F. Jalid, M. A. Haider, M. I. Alam and T. S. Khan, Catal. Sci. Technol., 2021, 11, 2130–2143 RSC
.
- L. Chen, Y. Lu, Q. Hong, J. Lin and F. M. Dautzenberg, Appl. Catal., A, 2005, 292, 295–304 CrossRef CAS
.
- J. Xu and M. Saeys, J. Catal., 2006, 242, 217–226 CrossRef CAS
.
- J. Xu and M. Saeys, Chem. Eng. Sci., 2007, 62, 5039–5041 CrossRef CAS
.
- L. Maier, B. Schädel, K. H. Delgado, S. Tischer and O. Deutschmann, Top. Catal., 2011, 54, 845–858 CrossRef CAS
.
- E. Akpan, Y. Sun, P. Kumar, H. Ibrahim, A. Aboudheir and R. Idem, Chem. Eng. Sci., 2007, 62, 4012–4024 CrossRef CAS
.
- Y. A. Zhu, D. Chen, X. G. Zhou and W. K. Yuan, Catal. Today, 2009, 148, 260–267 CrossRef CAS
.
- S. Chen, J. Zaffran and B. Yang, Appl. Catal., B, 2020, 270, 118859 CrossRef CAS
.
- W.-Y. Wang and G.-C. Wang, Catal. Sci. Technol., 2021, 1395–1406 RSC
.
- Z. Xie, Q. Liao, M. Liu, Z. Yang and L. Zhang, Energy Convers. Manage., 2017, 153, 526–537 CrossRef CAS
.
- K. H. Ernst, C. T. Campbell and G. Moretti, J. Catal., 1992, 134, 66–74 CrossRef CAS
.
- C. S. Chen, W. H. Cheng and S. S. Lin, Appl. Catal., A, 2004, 257, 97–106 CrossRef CAS
.
- L. Wang, S. Zhang and Y. Liu, J. Rare Earths, 2008, 26, 66–70 CrossRef
.
- M. C. J. Bradford and M. A. Vannice, J. Catal., 1998, 173, 157–171 CrossRef CAS
.
- H. Purnama, T. Ressler, R. E. Jentoft, H. Soerijanto, R. Schlögl and R. Schomäcker, Appl. Catal., A, 2004, 259, 83–94 CrossRef CAS
.
- J. K. Lee, J. B. Ko and D. H. Kim, Appl. Catal., A, 2004, 278, 25–35 CrossRef CAS
.
- K. Hou and R. Hughes, Chem. Eng. J., 2001, 82, 311–328 CrossRef CAS
.
- H. C. Wu, Y. C. Chang, J. H. Wu, J. H. Lin, I. K. Lin and C. S. Chen, Catal. Sci. Technol., 2015, 5, 4154–4163 RSC
.
- M. J. L. Ginés, A. J. Marchi and C. R. Apesteguía, Appl. Catal., A, 1997, 154, 155–171 CrossRef
.
-
C. T. Campbell and K.-H. Ernst, Surface Science of Catalysis, American Chemical Society, 1992, vol. 482, pp. 130–142, DOI:10.1021/bk-1992-0482.ch008
.
- C. S. Chen, J. H. Wu and T. W. Lai, J. Phys. Chem. C, 2010, 114, 15021–15028 CrossRef CAS
.
- A. Wolf, A. Jess and K. Christoph, Chem. Eng. Technol., 2016, 298, 1040–1048 CrossRef
.
- J. A. Hernandez Lalinde, P. Roongruangsree, J. Ilsemann, M. Bäumer and J. Kopyscinski, Chem. Eng. J., 2020, 390, 124629 CrossRef CAS
.
- W. Benzinger, E. Daymo, M. Hettel, L. Maier, C. Antinori, P. Pfeifer and O. Deutschmann, Chem. Eng. J., 2019, 362, 430–441 CrossRef CAS
.
- P. Marocco, E. A. Morosanu, E. Giglio, D. Ferrero, C. Mebrahtu, A. Lanzini, S. Abate, S. Bensaid, S. Perathoner, M. Santarelli, R. Pirone and G. Centi, Fuel, 2018, 225, 230–242 CrossRef CAS
.
- J. Lefebvre, S. Bajohr and T. Kolb, Fuel, 2019, 239, 896–904 CrossRef CAS
.
- Z. Bian, Y. M. Chan, Y. Yu and S. Kawi, Catal. Today, 2020, 347, 31–38 CrossRef CAS
.
- G. Garbarino, C. Wang, T. Cavattoni, E. Finocchio, P. Riani, M. Flytzani-Stephanopoulos and G. Busca, Appl. Catal., B, 2019, 248, 286–297 CrossRef CAS
.
- S. Rönsch, J. Köchermann, J. Schneider and S. Matthischke, Chem. Eng. Technol., 2016, 39, 208–218 CrossRef
.
- F. Koschany, D. Schlereth and O. Hinrichsen, Appl. Catal., B, 2016, 181, 504–516 CrossRef CAS
.
- J. H. Chiang and J. R. Hopper, Ind. Eng. Chem. Prod. Res. Dev., 1983, 22, 225–228 CrossRef CAS
.
- J. K. Kesavan, I. Luisetto, S. Tuti, C. Meneghini, G. Iucci, C. Battocchio, S. Mobilio, S. Casciardi and R. Sisto, J. CO2 Util., 2018, 23, 200–211 CrossRef CAS
.
- J. Yang Lim, J. McGregor, A. J. Sederman and J. S. Dennis, Chem. Eng. Sci., 2016, 141, 28–45 CrossRef
.
- O. Mohan, S. Shambhawi, X. Rong, A. A. Lapkin and S. H. Mushrif, ChemCatChem, 2021, 13, 2420–2433 CrossRef CAS
.
- A. Loder, M. Siebenhofer and S. Lux, J. Ind. Eng. Chem., 2020, 85, 196–207 CrossRef CAS
.
- X. Wang, Y. Hong, H. Shi and J. Szanyi, J. Catal., 2016, 343, 185–195 CrossRef CAS
.
- G. D. Weatherbee and C. H. Bartholomew, J. Catal., 1984, 87, 352–362 CrossRef CAS
.
- T. Kai, T. Takahashi and S. Furusaki, Can. J. Chem. Eng., 1988, 66, 343–347 CrossRef CAS
.
- L. Falbo, M. Martinelli, C. G. Visconti, L. Lietti, C. Bassano and P. Deiana, Appl. Catal., B, 2018, 225, 354–363 CrossRef CAS
.
- M. S. Duyar, A. Ramachandran, C. Wang and R. J. Farrauto, J. CO2 Util., 2015, 12, 27–33 CrossRef CAS
.
- T. Avanesian, G. S. Gusmão and P. Christopher, J. Catal., 2016, 343, 86–96 CrossRef CAS
.
- A. K. Raghu and N. S. Kaisare, Ind. Eng. Chem. Res., 2020, 59, 16161–16169 CrossRef CAS
.
- C. Seidel, A. Jörke, B. Vollbrecht, A. Seidel-Morgenstern and A. Kienle, Chem. Eng. Sci., 2018, 175, 130–138 CrossRef CAS
.
- T. Fujitani, I. Nakamura, T. Uchijima and J. Nakamura, Surf. Sci., 1997, 383, 285–298 CrossRef CAS
.
- M. Peter, M. B. Fichtl, H. Ruland, S. Kaluza, M. Muhler and O. Hinrichsen, Chem. Eng. J., 2012, 203, 480–491 CrossRef CAS
.
- A. Karelovic, G. Galdames, J. C. Medina, C. Yévenes, Y. Barra and R. Jiménez, J. Catal., 2019, 369, 415–426 CrossRef CAS
.
- J. F. Portha, K. Parkhomenko, K. Kobl, A. C. Roger, S. Arab, J. M. Commenge and L. Falk, Ind. Eng. Chem. Res., 2017, 56, 13133–13145 CrossRef CAS
.
- K. Ahmad and S. Upadhyayula, Fuel, 2020, 278, 118296 CrossRef CAS
.
- X. Jiang, X. Wang, X. Nie, N. Koizumi, X. Guo and C. Song, Catal. Today, 2018, 316, 62–70 CrossRef CAS
.
- M. S. Frei, M. Capdevila-Cortada, R. García-Muelas, C. Mondelli, N. López, J. A. Stewart, D. Curulla Ferré and J. Pérez-Ramírez, J. Catal., 2018, 361, 313–321 CrossRef CAS
.
- S. Ghosh, J. Sebastian, L. Olsson and D. Creaser, Chem. Eng. J., 2021, 416, 129120 CrossRef CAS
.
- G. H. Graaf, E. J. Stamhuis and A. A. C. M. Beenackers, Chem. Eng. Sci., 1988, 43, 3185–3195 CrossRef CAS
.
- K. M. V. Bussche and G. F. Froment, Appl. Catal., A, 1994, 112, 37–55 CrossRef
.
- K. M. Vanden Bussche and G. F. Froment, J. Catal., 1996, 161, 1–10 CrossRef CAS
.
- T. S. Askgaard, J. K. Norskov, C. V. Ovesen and P. Stoltz, J. Catal., 1995, 156, 229–242 CrossRef CAS
.
- C. V. Ovesen, B. S. Clausen, J. Schiøtz, P. Stoltze, H. Topsøe and J. K. Nørskov, J. Catal., 1997, 168, 133–142 CrossRef CAS
.
- J. Ye, C. J. Liu, D. Mei and Q. Ge, J. Catal., 2014, 317, 44–53 CrossRef CAS
.
- D. L. Chiavassa, S. E. Collins, A. L. Bonivardi and M. A. Baltanás, Chem. Eng. J., 2009, 150, 204–212 CrossRef CAS
.
- D. Zambrano, J. Soler, J. Herguido and M. Menéndez, Top. Catal., 2019, 62, 456–466 CrossRef CAS
.
- H. Zhou, T. Zhang, Z. Sui, Y. A. Zhu, C. Han, K. Zhu and X. Zhou, Appl. Catal., B, 2018, 233, 143–159 CrossRef CAS
.
- G. D. Wehinger, T. Eppinger and M. Kraume, Chem. Eng. Sci., 2015, 122, 197–209 CrossRef CAS
.
- D. Pakhare, V. Schwartz, V. Abdelsayed, D. Haynes, D. Shekhawat, J. Poston and J. Spivey, J. Catal., 2014, 316, 78–92 CrossRef CAS
.
- U. Olsbye, T. Wurzel and L. Mleczko, Ind. Eng. Chem. Res., 1997, 36, 5180–5188 CrossRef
.
- J. Wei and E. Iglesia, J. Phys. Chem. B, 2004, 108, 4094–4103 CrossRef CAS
.
- X. E. Verykios, Chem. Ind. Chem. Eng. Q., 2002, 8, 238–255 Search PubMed
.
- M. M. B. Quiroga and A. E. C. Luna, Ind. Eng. Chem. Res., 2007, 46, 5265–5270 CrossRef
.
- A. B. Mhadeshwar and D. G. Vlachos, J. Phys. Chem. B, 2005, 109, 16819–16835 CrossRef CAS PubMed
.
- K. H. Delgado, L. Maier, S. Tischer, A. Zellner, H. Stotz and O. Deutschmann, Catalysts, 2015, 5, 871–904 CrossRef CAS
.
- L. M. Aparicio, J. Catal., 1997, 165, 262–274 CrossRef CAS
.
- D. Chen, R. Lødeng, A. Anundskås, O. Olsvik and A. Holmen, Chem. Eng. Sci., 2001, 56, 1371–1379 CrossRef CAS
.
- M. Younas, L. L. Kong, M. J. K. Bashir, H. Nadeem, A. Shehzad and S. Sethupathi, Energy Fuels, 2016, 30, 8815–8831 CrossRef CAS
.
-
H.-H. Greev, Ullmann's Encyclopedia of Industrial Chemistry, Wiley-VCH Verlag GmbH & Co. KGaA, 2000 Search PubMed
.
- E. Giglio, R. Pirone and S. Bensaid, Renewable Energy, 2021, 170, 1040–1051 CrossRef CAS
.
- S. Pérez, J. J. Aragón, I. Peciña and E. J. Garcia-Suarez, Top. Catal., 2019, 62, 518–523 CrossRef
.
- K. P. Brooks, J. Hu, H. Zhu and R. J. Kee, Chem. Eng. Sci., 2007, 62, 1161–1170 CrossRef CAS
.
- M. Bracconi, M. Ambrosetti, M. Maestri, G. Groppi and E. Tronconi, Chem. Eng. Process., 2018, 129, 181–189 CrossRef CAS
.
- R. Balzarotti, M. Ambrosetti, A. Beretta, G. Groppi and E. Tronconi, Chem. Eng. J., 2020, 391, 123494 CrossRef CAS
.
- S. Cimino, E. M. Cepollaro, L. Lisi, S. Fasolin, M. Musiani and L. Vázquez-Gómez, Catalysts, 2021, 11, 1–15 Search PubMed
.
- V. Meille, Appl. Catal., A, 2006, 315, 1–17 CrossRef CAS
.
- R. Uglietti, M. Bracconi and M. Maestri, React. Chem. Eng., 2020, 5, 278–288 RSC
.
- C. Jia, Y. Dai, Y. Yang and J. W. Chew, Particuology, 2020, 49, 55–64 CrossRef CAS
.
- H. Nam, J. H. Kim, H. Kim, M. J. Kim, S. G. Jeon, G. T. Jin, Y. Won, B. W. Hwang, S. Y. Lee, J. I. Baek, D. Lee, M. W. Seo and H. J. Ryu, Energy, 2021, 214, 118895 CrossRef CAS
.
- E. Jwa, S. B. Lee, H. W. Lee and Y. S. Mok, Fuel Process. Technol., 2013, 108, 89–93 CrossRef CAS
.
- M. Nizio, A. Albarazi, S. Cavadias, J. Amouroux, M. E. Galvez and P. Da Costa, Int. J. Hydrogen Energy, 2016, 41, 11584–11592 CrossRef CAS
.
- F. Azzolina-Jury, D. Bento, C. Henriques and F. Thibault-Starzyk, J. CO2 Util., 2017, 22, 97–109 CrossRef CAS
.
- E. Meloni, M. Martino, A. Ricca and V. Palma, Int. J. Hydrogen Energy, 2021, 46, 13729–13747 CrossRef CAS
.
- S. Renda, M. Cortese, G. Iervolino, M. Martino, E. Meloni and V. Palma, Catal. Today, 2020 DOI:10.1016/j.cattod.2020.11.020
.
- K. De Ras, R. Van de Vijver, V. V. Galvita, G. B. Marin and K. M. Van Geem, Curr. Opin. Chem. Eng., 2019, 26, 81–87 CrossRef
.
- A. I. Stankiewicz and H. Nigar, React. Chem. Eng., 2020, 5, 1005–1016 RSC
.
- G. H. Xiu, P. Li and A. E. Rodrigues, Chem. Eng. Sci., 2003, 58, 3425–3437 CrossRef CAS
.
- O. S. Joo, K. D. Jung, I. Moon, A. Y. Rozovskii, G. I. Lin, S. H. Han and S. J. Uhm, Ind. Eng. Chem. Res., 1999, 38, 1808–1812 CrossRef CAS
.
- S. Saeidi, N. A. S. Amin and M. R. Rahimpour, J. CO2 Util., 2014, 5, 66–81 CrossRef CAS
.
- R. P. W. J. Struis, M. Quintilii and S. Stucki, J. Membr. Sci., 2000, 177, 215–223 CrossRef CAS
.
- F. Samimi, D. Karimipourfard and M. R. Rahimpour, Chem. Eng. Res. Des., 2018, 140, 44–67 CrossRef
.
- F. Gallucci and A. Basile, Int. J. Hydrogen Energy, 2007, 32, 5050–5058 CrossRef CAS
.
- P. Rodriguez-Vega, A. Ateka, I. Kumakiri, H. Vicente, J. Ereña, A. T. Aguayo and J. Bilbao, Chem. Eng. Sci., 2021, 234, 116396 CrossRef CAS
.
- K. I. Sawamura, T. Shirai, M. Takada, Y. Sekine, E. Kikuchi and M. Matsukata, Catal. Today, 2008, 132, 182–187 CrossRef CAS
.
- S. Guffanti, C. G. Visconti, J. van Kampen, J. Boon and G. Groppi, Chem. Eng. J., 2021, 404, 126573 CrossRef CAS
.
- S. Guffanti, C. G. Visconti and G. Groppi, Ind. Eng. Chem. Res., 2021, 60, 6767–6783 CrossRef CAS PubMed
.
- A. Zachopoulos and E. Heracleous, J. CO2 Util., 2017, 21, 360–367 CrossRef CAS
.
- P. Maksimov, A. Laari, V. Ruuskanen, T. Koiranen and J. Ahola, Chem. Eng. J., 2021, 418, 129290 CrossRef CAS
.
- C. Sriakkarin, W. Umchoo, W. Donphai, Y. Poo-arporn and M. Chareonpanich, Catal. Today, 2018, 314, 114–121 CrossRef CAS
.
Footnote |
† All authors contributed equally to this work. |
|
This journal is © The Royal Society of Chemistry 2021 |