DOI:
10.1039/D0SC02175J
(Edge Article)
Chem. Sci., 2020,
11, 5637-5649
Twisting and tilting of a mechanosensitive molecular probe detects order in membranes†
Received
16th April 2020
, Accepted 29th April 2020
First published on 21st May 2020
Abstract
Lateral forces in biological membranes affect a variety of dynamic cellular processes. Recent synthetic efforts have introduced fluorescent “flippers” as environment-sensitive planarizable push–pull probes that can detect lipid packing and membrane tension, and respond to lipid-induced mechanical forces by a shift in their spectroscopic properties. Herein, we investigate the molecular origin of the mechanosensitivity of the best known flipper, Flipper-TR, by an extended set of molecular dynamics (MD) simulations in membranes of increasing complexity and under different physicochemical conditions, revealing unprecedented details of the sensing process. Simulations enabled by accurate refinement of Flipper-TR force field using quantum mechanical calculations allowed us to unambiguously correlate the planarization of the two fluorescent flippers to spectroscopic response. In particular, Flipper-TR conformation exhibits bimodal distribution in disordered membranes and a unimodal distribution in highly ordered membranes. Such dramatic change was associated with a shift in Flipper-TR excitation spectra, as supported both by our simulated and experimentally-measured spectra. Flipper-TR sensitivity to phase-transition is confirmed by a temperature-jump protocol that alters the lipid phase of an ordered membrane, triggering an instantaneous mechanical twisting of the probe. Simulations show that the probe is also sensitive to surface tension, since even in a naturally disordered membrane, the unimodal distribution of coplanar flippers can be achieved if a sufficiently negative surface tension is applied to the membrane. MD simulations in ternary mixtures containing raft-like nanodomains show that the probe can discriminate lipid domains in phase-separated complex bilayers. A histogram-based approach, called DOB-phase classification, is introduced that can differentiate regions of disordered and ordered lipid phases by comparing dihedral distributions of Flipper-TR. Moreover, a new sensing mechanism involving the orientation of Flipper-TR is elucidated, corroborating experimental evidence that the probe tilt angle is strongly dependent on lipid ordering. The obtained atomic-resolution description of Flipper-TR mechanosensitivity is key to the interpretation of experimental data and to the design of novel mechanosensors with improved spectroscopic properties.
1 Introduction
Biological membranes are complex, heterogeneous structures not only defining the boundaries that protect the cellular machinery from external stress, but also acting as a major platform for a variety of key processes in living cells.1,2 Lipid constituents of membranes can compartmentalize into domains of various sizes and properties, whose formation and dynamics affect the behavior of the entire cell.3 Lipid bilayers are resistant to stretching or shearing and can therefore bear substantial changes in lateral tension.4 Despite the importance of lipid domains and membrane tension, lateral forces within microscopic domains of a membrane are still challenging to determine experimentally. There has been a lack of experimental techniques that can reliably measure these forces in a dynamic environment such as a living cell.5
In the last few years, a new class of environment-sensitive dyes have been introduced to non-invasively track lateral forces and lipid domains in membranes.6–15 Dithienothiophene (DTT)-based push–pull mechanosensors termed “flippers” are characterized by an excitation band that shifts to lower energy when partitioned in ordered lipid environments. These probes appear to respond to lateral forces in lipid bilayers, thereby discriminating between ordered and disordered lipid phases, as shown experimentally by fluorescence imaging of unilamellar vesicles.7,13 The currently best performing and spectroscopically-efficient flipper mechanosensor is Flipper-TR (Fig. 1a). The liquid-disordered (Ld) to solid-ordered (So) transition causes a remarkable red shift in Flipper-TR excitation of Δλex = 50–90 nm, a strong increase of fluorescence intensity and lifetime (Fig. 1b), and the appearance of some vibrational finestructure.16 Flipper-TR is composed of (i) a triazole-carboxylate hydrophilic head for positioning into the membrane and stability, and (ii) a DTT-based chromophore responsible for the spectroscopic behavior. It has been hypothesized that the two DTT flippers may respond to lateral forces by switching between planar and nonplanar conformations as a result of twisting around the “mechanosensitive” dihedral Θ (Fig. 1a and Movie 1†). The mechanosensing mechanism does not rely on rearrangement of the geometry in the excited state but uniquely on ground-state conformational changes. However, despite the experimentally demonstrated effectiveness of flipper probes, the mechanism needs yet to be clarified and characterized at the molecular level.
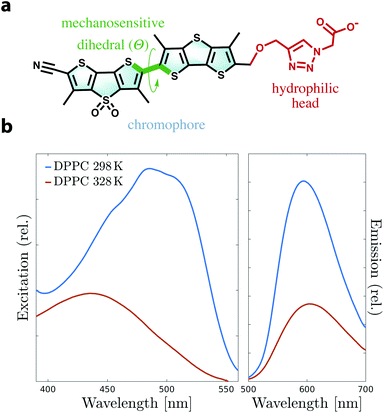 |
| Fig. 1 (a) Molecular structure of Flipper-TR highlighting the twisting of the two flippers along the mechanosensitive dihedral (Θ, green). The chromophore (cyan) and the hydrophilic head (red) are also highlighted. (b) Experimental excitation and emission spectra of Flipper-TR in DPPC large unilamellar vesicles (LUVs) at 298 K and 328 K. The generation of an ordered membrane at low temperature causes a remarkable red shift in excitation and an increase in fluorescence. | |
Molecular dynamics simulation continues to play a pivotal role in studying microscopic aspects of the organization of biological membranes and interactions among their various components.17–21 The microscopic view supplied by simulations can grant access to multiple facets of membranes, such as domain or pore formation,22–29 curvature sensing30–38 and self-assembly of lipids.39–42 A major key factor for the success of such simulations is the quality of the force field, i.e. the parameters describing the physical interaction among the atoms, which depends on an accurate refinement of these parameters while comparing the outcomes of simulations with experimental or quantum mechanical (QM) data.43,44 The exponential increase in computer resources and more efficient implementations of algorithms have also contributed to the dramatic development and proliferation of computer simulations.18
The aim of this study is to dissect the mechanosensitivity of flippers by combining atomistic molecular dynamics (MD) simulations with experimental measurements. Refinement of Flipper-TR force field using QM calculations, a critical step for accurate description of the process, is here carefully validated by comparing the free energy of membrane intercalation with experimental partition coefficients. MD simulations are then performed for the flipper in unary, binary and ternary lipid mixtures to cover all Ld, So, Lo lipid phases and phase-separated membranes. Correlation of probe planarization with shifts in excitation is investigated by a combination of MD and QM to calculate the excitation spectra. Additional simulations featuring temperature jumps or surface tension transitions are also performed to demonstrate how Flipper-TR rapidly responds to lateral force changes in membranes. We introduce a generalized method to discriminate between lipid phases in MD simulations based on conformational dynamics of exogenous probes such as flippers, using a histogram-based approach that, here, we call DOB-phase classification. Using this approach, the effect of membrane fluidity on biological activity can be studied by embedding Flipper-TR into a membrane and simply following the change in the twisted state of the flippers. Finally, Flipper-TR orientation is corroborated as a reporter of lateral forces in membranes, as previously proposed in an experimental work.10 The broadening of orientation distribution in more disordered membranes predicted by the simulations is in contrast with previous assumptions and is critical for the interpretation of experimental results.
2 Material and methods
2.1 Molecular dynamics simulations
2.1.1 General protocols.
All classical MD simulations were performed using NAMD2.45 The fully atomistic CHARMM36 force field46,47 was employed for lipids whereas the standard TIP3P model48 was used for water. Initial topology files for Flipper-TR were retrieved from the CHARMM General Force Field (CGenFF).49 Force field parameters were further refined using Force Field Toolkit (ffTK)50 implemented in VMD51(details in ESI, Fig. S1 and S2†). Membrane systems of various lipid composition (DOPC: 1,2-dioleoyl-sn-glycero-3-phosphocholine, DPPC: 1,2-dipalmitoyl-sn-glycero-3-phosphocholine, SM: N-palmitoyl-D-erythro-sphingosylphosphorylcholine, CL: cholesterol) were generated using Membrane Builder in CHARMM-GUI52 (Table S1†).
Nonbonded interactions were evaluated with a cutoff of 12 Å, with a switching function starting at 10 Å. Long-range electrostatic interactions were accounted for by the particle mesh Ewald (PME) method53 with a grid density of 1 Å−3 and PME interpolation order of 6. All bonds involving hydrogen atoms were kept rigid using the SHAKE algorithm.54
All simulations were performed in a flexible cell, which allows the dimensions of the periodic cell to fluctuate independently. The aspect ratio of the unit cell in the x–y plane was kept constant. The NPT ensemble was maintained by a Nosé–Hoover Langevin piston and a Langevin thermostat (1 atm, period: 50 fs, decay: 25 fs, damping coefficient: 1.0 ps−1).55,56 The simulation timestep was set to 2 fs, and both Lennard-Jones and PME forces were updated at every timestep. All membranes were equilibrated for at least 40 ns before starting any production run. The simulations used for analysis ran for 250–300 ns, unless specified otherwise. The equilibration of the simulation systems was examined by monitoring the total energy, area per lipid, mass interdigitation, membrane thickness, lipid tilt angle, deuterium order parameter (SCD) of lipids, and mass density. Averages of time-dependent properties in blocks of 100 ns show a deviation of ≤2%. Membrane physicochemical properties were generally in agreement with values found in the literature47,57–59 (details in ESI, Fig. S3–S9†). The lowest temperature used for binary and ternary mixtures was 310 K in order to accelerate equilibration of these more complex membranes.
MD trajectories were visualized by VMD and data were analyzed using MEMBPLUGIN60 and in-house scripts (details on analyses are reported in ESI†). Since the chromophore of Flipper-TR possesses a plane of symmetry, the mechanosensitive dihedral was reported between 0 and 180° by flipping the sign of all negative dihedrals.
2.1.2 Generation of ternary mixtures.
For ternary mixtures, square-shaped equilibrated SM/CL domains were embedded into an equilibrated DOPC bilayer, eliminating overlapping lipids. The resulting ternary systems were first minimized while restraining water molecules and then relaxed under NPT condition. No significant water penetration between the SM/CL domain and DOPC was observed. For these simulations, Langevin piston period and decay were both set to 200 fs. Grid density and interpolation for PME were set to 2.1 Å−3 and 8, respectively, in order to speed up the simulations. PME forces were updated every other time step.
2.1.3 Umbrella sampling simulations.
Free energy profiles for membrane-water partitioning of Flipper-TR in different lipid bilayers at 330 K were calculated using the umbrella sampling (US) technique.61,62 Initial structures for US simulations were obtained from steered MD trajectories.63,64 In each bilayer, Flipper-TR was pulled out from its equilibrium position inside the membrane by subjecting the center of mass of its carboxylate group to a harmonic potential with force constant of 5 kcal mol−1 Å−2 and moving at a velocity of 75 Å ns−1. To avoid artificial translation of the bilayer or individual lipids, the center of mass of phosphorus atoms as well as the position of few atoms of closest lipids were restrained along the membrane normal. US simulations included 36 (for DOPC) or 41 (for DPPC and SM/CL) windows spaced by 1 Å in the z direction, and each window was simulated for 30 ns, with an applied harmonic potential of k = 2.5 kcal mol−1 Å−2. The weighted histogram analysis method (WHAM)65,66 was used to generate the final free energy profiles with a 0.5 Å histogram bin. Free energies of membrane partitioning are estimated as the difference between minimum (membrane) and maximum (water) in the free energy profiles.
2.2 Electronic structure calculations
All density functional theory (DFT) calculations were performed using Gaussian 09 (Rev. B or D)67 in the gas phase. The long-range-corrected CAM-B3LYP functional68 was employed with Pople 6-31G(d) and 6-311+G(d,p) basis sets for geometry optimization and vertical transition calculations, respectively. Electronic transition energies were obtained from time-dependent (TD) DFT69,70 and reported as simulated excitation spectra where each vertical transition was convolved with a Lorentzian lineshape of 0.15 eV width. In particular, each spectrum was generated by extracting 250 random probe conformations from each classical MD trajectory and by calculating the lowest 10 vertical excitation energies for each conformation.71–73 The entire set of Lorentzian-broadened excitations were combined to build the final excitation profile. Simulated spectra were shifted by 0.07 eV to lower energy for a better comparison with the experimental data, since CAM-B3LYP is known to systematically blue-shift vertical transitions with respect to experiments.74
2.3 Experimental materials and methods
Flipper-TR was synthesized and purified according to procedures described in ref. 9. CL was purchased from Sigma-Aldrich, egg sphingomyelin, DOPC, DPPC and Mini-extruder were purchased from Avanti Polar Lipids. Fluorescence measurements were performed at 298 K or 328 K using a FluoroMax-4 spectrofluorometer (Horiba Scientific) equipped with a stirrer and a temperature controller. All fluorescence spectra were background subtracted and corrected using correction factors supplied by the manufacturer. Excitation and emission spectra were smoothed in GraphPad Prism 8.0.1 using the Savitzky and Golay75 method with second order polynomial and 15 neighboring points to average. Preparation of vesicles and determination of partition coefficients are described in detail in ESI.†
3 Results and discussion
3.1 Partitioning of Flipper-TR in membranes
Flipper-TR is a chemically stable probe that localizes exclusively in the membrane when added to cellular samples.9 We characterized its partitioning into bilayers by measuring partition coefficients in large unilamellar vesicles (LUVs) of different compositions (Tables 1 and S2, Fig. S11 and S12†). A rigorous refinement of Flipper-TR force field parameters was initially performed using higher level QM calculations. Several partial charges, bond, angle and dihedral parameters were taken into consideration and adjusted to reproduce QM results. Exploratory simulations in DOPC or DPPC membranes showed that Flipper-TR spontaneously intercalates into the bilayers within few tens of nanoseconds (Movie 2†). In order to quantitatively compare the partitioning of the probe in experiments and simulations, free energy profiles were obtained by means of umbrella sampling simulations62 (Fig. 2). Profiles for pure DOPC or DPPC and binary SM/CL (7
:
3 molar ratio) membranes results in excellent agreement with experimental partition coefficients (Table 1). The membrane positioning of the probe reflects the amphiphilic nature of its chemical structure, with the hydrophilic head pointing towards the water phase and the two flippers sitting in between the lipid tails. Both computed and measured free energies suggest a small preference for Flipper-TR partitioning towards the highly-disordered DOPC bilayer. DPPC generates disordered membranes at temperatures higher than its phase transition and therefore afforded a similar partition coefficient. On the other hand, SM/CL mixture provides a more ordered environment even at 330 K.59 Such behavior manifested in a slightly smaller free energy of intercalation. The close agreement between experimental and calculated free energies for Flipper-TR partitioning in different membranes provides strong support for the quality of the developed force field parameters.
Table 1 Experimental membrane phase transition temperatures (Tm)59,76,77 and Flipper-TR partition coefficients (Kp) measured in LUVs at 328 K using the same composition as in simulated membranes. Experimental free energies of partitioning were obtained from ΔG = −RT
ln
Kp, whereas the free energies of partitioning from simulations are estimated as the difference between minimum and bulk water in the free energy profiles in Fig. 2
|
DOPC |
DPPC |
SM/CL (7 : 3) |
T
m [K] |
Exp. |
256 |
314 |
N.A. |
K
p × 10−6 |
|
8.0 ± 0.8 |
3.2 ± 0.3 |
2.2 ± 0.3 |
ΔG [kcal mol−1] |
Exp. |
−10.4 ± 0.1 |
−9.8 ± 0.1 |
−9.6 ± 0.1 |
Sim. |
−11.0 ± 0.8 |
−10.9 ± 0.9 |
−10.3 ± 0.9 |
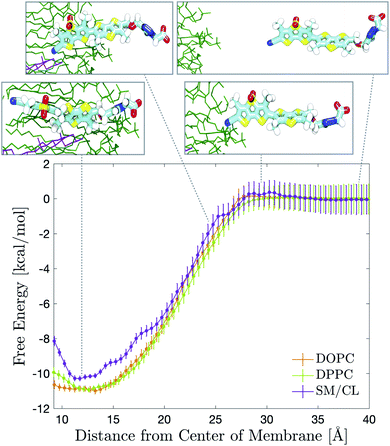 |
| Fig. 2 Simulated free energy of partitioning of Flipper-TR in DOPC (orange), DPPC (light green) and SM/CL (7 : 3 molar ratio, violet) bilayers at 330 K. Positioning of the probe in the SM (green)/CL (violet) membrane at different stage of the partitioning process is shown in the insets from MD simulation snapshots. | |
3.2 Characterization of mechanosensitivity in unary and binary phases
Mechanosensitivity of Flipper-TR was first studied in membranes composed of either pure DOPC or pure DPPC lipids at 298 K and 330 K. In agreement with a fluid-like Ld state, DOPC membranes at both temperatures display high values of area per lipid and tail interdigitation, and low values of SCD (Fig. S3 and S4†). The analysis of DPPC bilayer at high temperature points also to a disordered membrane (Fig. S5†). However, lowering the temperature to 298 K causes a drastic reduction of area per lipid and an increase of SCD in the lipid alkyl chain indicating the formation of a gel-like phase (Fig. S6†). Under these conditions, acyl chains arrange in local hexagonal substructures due to dense packing, as reported in the literature78,79 (Fig. 3a). The twist angle between the two flippers (Θ, Fig. 1a), which is hypothesized to be responsible for the mechanosensitivity, was extracted from these simulations as a function of time and the histograms were fit to skewed Gaussians (Fig. S13, S14, and Table S4†).80 The histograms in DOPC consist of a double peak (Θc ≃ 70 ± 2° and 117 ± 1°) and do not show changes at the two temperatures (Fig. 3b). The bimodal distribution and the peak intensity asymmetry, which favors dihedrals close to 117°, are in agreement with QM calculations.10 A bimodal distribution with similar fitting parameters is also observed for DPPC at 330 K (Fig. 3b and Table S4†). However, Flipper-TR twisting in the ordered DPPC membrane at 298 K results in a single-peaked histogram (Θc = 143 ± 1°). Such drastic change in Θ can be attributed to the lateral forces in the ordered bilayer that favor planarization of the two Flipper-TR flippers (Movie 3†). The two central methyl groups of Flipper-TR however do not allow full planarization (Θc ≃ 180°) because of steric and electrostatic repulsion. Moreover, the average twist angle in DPPC membranes coincides with the minimum in the potential energy surface for the first excited state calculated by torsion scan of Θ using TD-DFT10 and suggests that in ordered membranes Flipper-TR does not undergo significant structural rearrangements after excitation, a phenomenon instead observed for unplanarized flippers.8,10
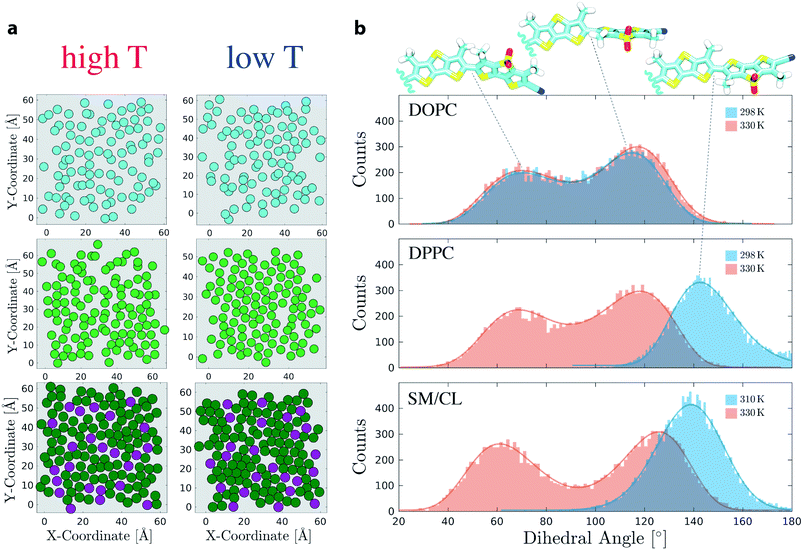 |
| Fig. 3 (a) Center of mass (com) of acyl chains in DOPC (cyan), DPPC (light green) and SM (dark green) or com of whole CL (purple) rendered as filled circles. The plots were extracted from the last frame of DOPC, DPPC and SM/CL (7 : 3 molar ratio) simulations at high and low temperatures (shown on right panel). Only one leaflet is shown. (b) Histograms of mechanosensitive dihedral Θ for the simulations in DOPC, DPPC and SM/CL (7 : 3). Fitting curves are shown as solid lines. Each simulation was 300 ns long. Snapshots show Flipper-TR with twist angles corresponding to main peaks in the histograms. | |
Simulations in SM/CL (7
:
3) at 310 K and 330 K were performed to compare Flipper-TR behavior in Lo phases (physicochemical analysis in Fig. S7 and S8†). As observed for So DPPC, hexagonal substructures of SM saturated chains appeared also in SM/CL bilayers with cholesterol occupying interstitial regions between these substructures (Fig. 3a). At 330 K, the higher temperature reduced the hexagonally-ordered regions. However, packed substructures are still observed suggesting the existence of a partially-ordered environment even at this temperature.59 Histograms of flippers twist angle in SM/CL (7
:
3) are reported in Fig. 3b (fitting parameters and residuals in Table S4 and Fig. S14†). As for DPPC membranes, bimodal and unimodal distributions are retrieved at high and low temperatures, respectively. However, DPPC undergoes a phase transition in the temperature interval considered here, whereas SM/CL (7
:
3) exhibits always a Lo membrane in the same range of temperature (Fig. S7 and S8†). Whereas twist angles in SM/CL and in DPPC at low temperatures share a very similar average value (Θc = 139 ± 1° and 143 ± 1°, respectively) indicating that lipids are in a highly ordered state, the bimodal distribution at 330 K in SM/CL with respect to DPPC shows a shift towards more planar flipper conformations (+8° and −6° for higher and lower peaks, respectively, with an estimated uncertainty of ±2). The depletion of dihedrals around 90° (Fig. 3c) clearly suggests that SM/CL at high temperature presents still a partially-ordered environment, contrarily to DPPC. Flipper twist angles in the 130–150° range are most favored in ordered lipid environments because such a conformation minimizes the intramolecular steric hindrance between the innermost methyl groups and reduces the electrostatic repulsion between sigma-holes in sulfur atoms (modeled here as small, positive partial charges). To support this conclusion, 50 different snapshots from the trajectories obtained for ordered DPPC (298 K) and SM/CL (310 K) membranes were selected and each simulated independently for 10 ns, initially setting all the mechanosensitive dihedrals to 50°. Flipper twist angles for the last nanosecond of each simulation were then collected in the same histogram (Fig. S16†), which shows that the initial dihedral population at 50° almost entirely migrates towards the 130–150° range for SM/CL. Similarly, about half of the Flipper-TR population in DPPC (298 K) also adopts a more planar conformation over the timescale of these short simulations; based on these results, it appears that the remaining simulations of the DPPC (298 K) would also migrate to the 130–150° range if the timescale of the simulation were to be extended over 10 ns. Taken together, these simulations show that the dihedrals around 50–70° are not favorable in ordered membranes and constitute a metastable conformational state. Moreover, the simulations predict that the distribution of the mechanosensitive dihedral does not significantly depend on the relative position of the probe with respect to the membrane. The bimodal distribution of mechanosensitive dihedrals in disordered phases collected at different distances from the midplane of the membranes shows a linear trend with slopes of at most ∼0.2, the latter observed for the Lo SM/CL bilayer (Fig. S15 and Table S5†).
The local dynamics of Flipper-TR twisting was quantitatively investigated by torsion time autocorrelation functions (TACFs)81 for systems showing bimodal twisting distribution (Fig. S18 and Table S7†). A stretched exponential function was fit to each TACF and the resulting lifetimes clearly show a fast dynamics of twisting in disordered membranes and at high temperatures (τ = 0.25 and 0.17 ns for DOPC and DPPC at 330 K, respectively). TACF decays one order of magnitude slower in DOPC at 298 K (τ = 1.67 ns), suggesting that Flipper-TR twisting is also sensitive to temperature changes. An even slower decay is observed in SM/CL at 330 K (τ = 2.90 ns), which can be attributed to increased lateral forces in the Lo phase. The trend in the dynamics of Flipper-TR conformational change is confirmed by estimating the twisting frequency (Tf), i.e. the number of switching between the two most stable states (Θ = 50–70° and Θ = 130–150°, Table S7†). Flipper-TR twists about 2 times slower in Lo phase (Tf = 0.7 ns−1, cycles per nanosecond, in SM/CL) than in all other disordered membranes. The high fluidity of both DOPC and DPPC bilayers at high temperature promotes a fast twisting (Tf = ∼1.8 ns−1), while at a lower temperature (DOPC at 298 K), the twisting frequency has an intermediate value between Lo phase and high-temperature fluid membranes (Tf = 1.2 ns−1).
3.3 Excitation spectra link twisting to mechanosensitivity
In order to investigate the direct relation between the twist angle and the spectroscopic behavior of Flipper-TR, excitation spectra were computed using TD-DFT calculations of the Flipper-TR conformational ensembles generated from each of the MD simulations. For each membrane system, 250 Flipper-TR conformers were randomly selected from the trajectory and used to generate vertical transitions by TD-DFT. The final spectra were generated by broadening each transition with a Lorentzian function and combining all obtained lineshapes. We also measured experimentally the excitation spectra of Flipper-TR in LUVs using the same composition as in the simulations and at both low and high temperatures (298 K and 328 K, Fig. 4). The agreement between experimental and computational spectra is excellent for all systems and temperatures. In particular, whereas the spectra of Flipper-TR in DOPC at both temperatures or in DPPC at high temperature are all centered at ∼430 nm (Fig. 4a and b), the spectrum of the probe in DPPC at low temperature shows a clear red-shift with a maximum at around 485 nm. Such a remarkable shift (Δλex ∼ 55 nm) towards a lower excitation energy is also observed for Flipper-TR in SM/CL at low temperature (Fig. 4c). At the higher temperature, the spectroscopic shift of the probe in SM/CL is less dramatic but the spectra can still be discriminated from those recorded in disordered membranes, confirming that SM/CL (7
:
3) generates partially-ordered phases even at temperatures close to 330 K. Combining experiments and simulations, we can clearly conclude that the change in the flippers twist angle is correlated with the spectroscopic response of Flipper-TR. The quantitative agreement between the spectra provides another validation of the force field developed and used for the probe to sample its conformational space in classical simulations.
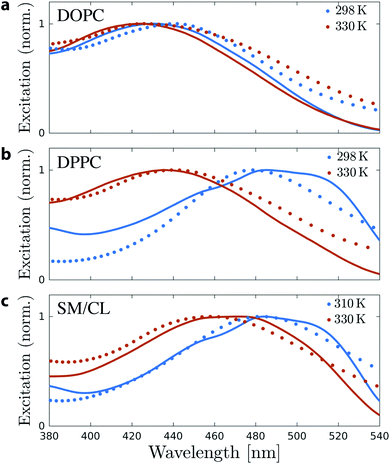 |
| Fig. 4 Normalized experimental (solid) and simulated (dot) excitation spectra of Flipper-TR in (a) DOPC, (b) DPPC and (c) SM/CL membranes and at low (blue) and high (red) temperatures. Computed spectra were red-shifted by 0.07 eV for a better comparison with the experimental spectra (recorded at 298 K and 328 K). | |
3.4 Flipper-TR responds instantaneously to rapid temperature changes
Mechanosensitive probes belonging to the flipper class were hypothesized to respond instantaneously to changes in bilayer lateral forces. In order to test this hypothesis for Flipper-TR, a temperature-jump protocol was designed and executed in three independent simulations in which the temperature of a So DPPC membrane in equilibrium at 298 K was instantaneously shifted to 330 K. After running the system for 60 ns, the temperature was instantaneously switched back to 298 K, and the membrane simulated for additional 60 ns (Fig. 5 and Movie 4,† two other replicas in Fig. S10†). The increase in temperature results in the melting of DPPC membrane within few tens of nanoseconds, as highlighted by dramatic variations in area per lipid, interdigitation, and SCD (Fig. 5 and S10†). The molecular probe responds to this change by shifting the average distribution of the flippers twist angle from the equilibrium value at 298 K to the typical bimodal distribution at 330 K. This distribution persists until the temperature is switched back to the initial low value (298 K). However, the rapid cooling of DPPC causes the formation of a ripple-like phase (Pβ)57 contrarily to the initial So phase. In fact, although the area per lipid decreases to around the initial value, lipids still show a high degree of interdigitation. However, the mechanosensitive dihedral recovers a unimodal distribution as obtained in DPPC at 298 K, though the average value is now <140°, consistent with a slightly less ordered membrane.
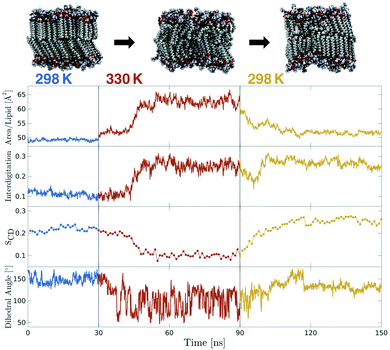 |
| Fig. 5 Temperature-jump simulation of a DPPC membrane containing Flipper-TR (two additional replicas in Fig. S10†). Initial equilibrium simulation at 298 K (blue) was heated instantaneously to 330 K for 60 ns (red) and then cooled down back to 298 K (orange). Area per lipid and interdigitation were extracted at 100 ps intervals. SCD of only one carbon is plotted (carbon number 15 of sn-2) and each point is the average over 1 ns. The dihedral angle plot shows the twist angle moving average over 1 ns windows. | |
3.5 Flipper-TR responds to membrane surface tension variations
In a recent experimental work, Flipper-TR was used to monitor membrane tension in live cells and artificial membranes by introducing osmotic shocks.82 We devised a set of simulations to examine directly and at the microscopic level the response of Flipper-TR as a tension reporter to different surface tensions83,84 applied to a DOPC bilayer at 298 K.
Applying a negative surface tension to a simulated bilayer leads generally to a decrease in surface area with a subsequent rise of lipid ordering. Until −100 dyn cm−1, the histograms of the twist angle do not show significant changes, with the higher peak shifting only marginally (Θc shifts from 116° to 120°), though the bimodal distribution becomes slightly more asymmetric (Fig. 6 and S17, and Table S6†). At the same time, the area per lipid decreases from 68 to 54 Å2 and SCD increases for most of the carbons in the acyl tails (Fig. S9†). Such appreciable changes in membrane properties do not seem to cause an increase in lateral forces and therefore no remarkable change in flipper twisting is observed. Experimentally, DOPC GUVs subjected to hypo- or hyperosmotic shocks show no changes in Flipper-TR lifetime,82 in agreement with our simulations. A lateral pressure of −200 dyn cm−1, much higher than experimentally applied, results in the emergence of a unimodal distribution in the twist angle (Θc = 134°), suggesting a more ordered environment. At this surface tension, the area per lipid shows a substantial decrease to 48 Å2 (without causing lysis or deformation of the membrane). Therefore, Flipper-TR was sensitive to such variations even in membranes at extreme physicochemical states.
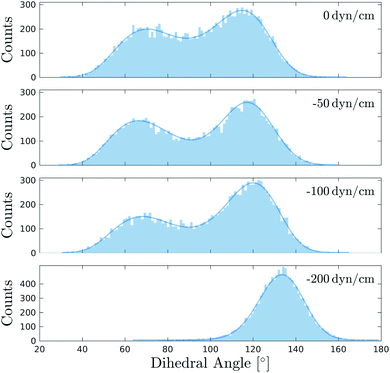 |
| Fig. 6 Distributions of the mechanosensitive dihedral Θ of Flipper-TR in DOPC bilayers simulated at 298 K and under different surface tensions. Fitting curves are shown as solid line. The distribution at 0 dyn cm−1 is retrieved from the first 250 ns of the simulation in DOPC at 298 K (Fig. 3). All other systems were simulated for 250 ns after an initial equilibration of 40 ns at the target surface tension. | |
3.6 Characterization of mechanosensitivity in ternary lipid mixtures
The sensitivity to lipid packing and surface tension makes Flipper-TR an attractive probe to track domain formation and dynamics in membranes. Lipid nanodomains, often known as rafts, are defined as small and highly dynamic domains rich in sterols and sphingolipids that can segregate cellular processes.3,85 The tight packing of saturated lipids in nanodomains provides a natural testing platform for Flipper-TR and its ability to discriminate between disordered and ordered environments within the same membrane. Therefore, we designed simulations in ternary mixtures to investigate the behavior of Flipper-TR in more complex membranes.
Due to the slow diffusion of lipids and the microsecond timescale required to form lipid domains, most of MD studies involving simulations of lipid nanodomains employ coarse-grained models,20,86–91 although few studies using all-atom simulations can be found in the literature.78,79,92–95 Here, we retain the atomic-level resolution of Flipper-TR as its working mechanism may not be accurately represented by a coarse-grained model. In order to avoid the long timescale needed to form domains, we created a preformed nanodomain by inserting a pre-equilibrated SM/CL membrane inside a larger Ld DOPC matrix.18,96 Two DOPC/SM/CL mixtures with the following molar ratios were thus generated: 50
:
39
:
11 (Membrane 1) and 26
:
57
:
17 (Membrane 2, Fig. 7a and Movie 5†). The latter composition is the same as phase-separated GUVs used to test flipper mechanosensitivity in a previous experimental study,7 whereas the former composition was chosen to investigate the effect of decreasing SM and CL fractions. The two ternary systems contained 8 and 6 Flipper-TRs, respectively, distributed equally between the two leaflets. Two replicas per system were simulated, which differed in the initial positioning of the probes (Fig. 7, S20 and S21†). Each membrane was simulated at 310 K for 1 μs, during which some lateral mixing of lipids was clearly observed (Fig. 7b). Although the timescale of these simulations may be too short to achieve an equilibrium lateral distribution of lipids, the aim here was to determine the sensitivity and response of Flipper-TR to the presence of different lipid phases in the same membrane.
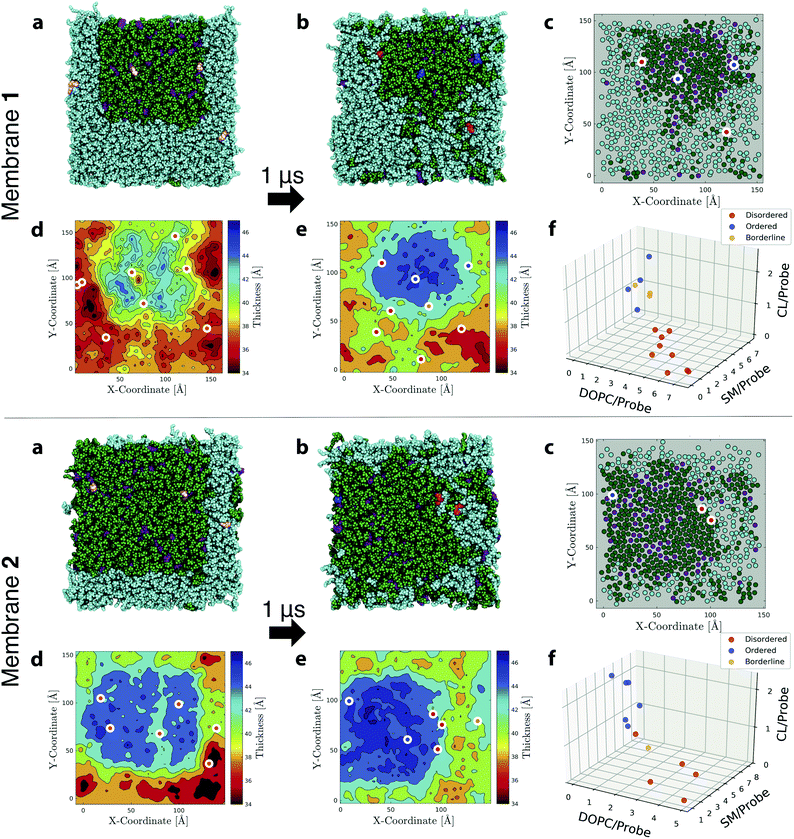 |
| Fig. 7 Simulations and analysis of ternary lipid mixture membranes. (a) First and (b) last snapshots from MD simulations of Flipper-TR in ternary mixtures with DOPC (cyan)/SM (green)/CL (purple) of molar-ratio composition 50 : 39 : 11 (Membrane 1, upper panel) and 26 : 57 : 17 (Membrane 2, lower panel). Several Flipper-TRs (pink in initial snapshots) were inserted into the membranes (8 and 6 for Membranes 1 and 2, respectively; only one leaflet shown). In (b), the probes were colored according to the disordered, ordered, borderline (DOB)-phase classification as reported in Table S9.† Membranes were simulated for 1 μs and additional replicas can be found in Fig. S21.† DOB analysis was performed on the last 500 ns. (c) Same snapshot as in (b) with the center of mass of CL, or acyl chains of SM and DOPC rendered as filled circles. White-bordered discs indicate center of mass of Flipper-TRs in the leaflet, with the inner color following the DOB-phase classification. Thickness map of (d) first and (e) last snapshots with the white-bordered discs indicating center of mass of Flipper-TRs of both leaflets and the inner color following the DOB-phase classification. (f) Average number of lipids within 3 Å of each Flipper-TR in the membrane (data from 2 replicas are merged in the same plot). Circles are colored according to the DOB-phase classification. | |
After 1 μs of simulation, the initial square-shaped SM/CL nanodomain in each system assumed a more circular shape (observed consistently in all replicas). This change is dictated by the minimization of line tension, i.e., the excess free energy per unit length of the edge separating membrane nanodomains, which reaches a minimum for a circular shape.97 Line tension is induced by the mismatch between the thicknesses of nanodomains and their surrounding lipids (Fig. 7d and f). Nanodomains at the end of simulations were about 5–6 Å thicker than the encircling lipid matrix.96,98 Coexistence of Ld and Lo phases with hexagonal substructure formed inside SM/CL nanodomains is also observed (Fig. 7c).78,79 Membrane 2 generates larger and thicker raft-like domains due to the higher percentage of SM/CL lipids.
To better quantify the behavior of Flipper-TR in such phase-separated systems, a statistical classification protocol was developed for the analysis of ternary mixtures. This method, which was inspired by the histogram intersection algorithm proposed by Swain et al. to recognize colors,99 is composed of the following steps: (1) obtain area-normalized histograms of flippers twist angle in the region of interest of the investigated membrane and in single-phase reference membranes displaying purely disordered or ordered phases; (2) calculate the intersection between the histogram for the region of interest and all reference histograms; and, (3) assign a disordered (D) or an ordered (O) label to Flipper-TR according to the maximum intersection; a borderline (B) label is assigned if the percent difference between the two highest intersections is less than a certain threshold (3% in this work), which indicates that a decision on the lipid order surrounding that particular Flipper-TR cannot be made. Intersections between distributions, therefore, serve as an indicator for discriminating lateral forces sensed by Flipper-TRs in their surrounding. Here, we will call this approach DOB-phase classification, as it provides a D, O or B label for each mechanosensitive probe in the membrane.
References histograms of flippers twist angles in DOPC at 298 K and in SM/CL at both 310 K and 330 K were selected (Fig. 3b), since they contain the same lipids as individual domains within the ternary mixtures and thus are expected to provide comparable lateral forces. The DOB-phase classification was then applied to the simulated ternary mixture membranes, using the last 500 ns of each simulation (intersections and classification for all membranes and probes are reported in Table S9†). The method was able to correctly assign an ordered label to all Flipper-TRs inside the nanodomains, whereas all outer probes were labeled as disordered (Fig. 7c–f, S20 and S21†). Furthermore, the few probes identified as borderlines are all located at the boundaries of the nanodomains, suggesting that lateral forces at the edge of the domain are intermediate between ordered and disordered states. Plots of Flipper-TRs as a function of average number of lipids surrounding each probe allow the visualization of clusters formed by the DOB-phase classification (Fig. 7f and S22†). High concentrations of DOPC around each flipper correlate with D-labeled classifications (red circles), whereas, on the contrary, high concentrations of SM/CL give rise to O-labeled classifications (blue circles). Borderline cases (orange circles) are found at intermediate average number of lipids between the two above-mentioned cases. D- and O-labeled Flipper-TRs can be therefore used to locate bulky disordered and ordered regions in membranes. On the other end, B-labeled Flipper-TRs can be used to define the boundary of domains or may be used to track smaller and fast-forming domains, which might not experience well-defined disordered or ordered lipid phases.
3.7 Tilting of Flipper-TR as an alternative sensing proxy
Flipper-TR orientation can be tracked down by experimental techniques such as Surface Second Harmonic Generation (SSHG)10,71,100,101 and, recently, it was found to be a reporter of lateral pressure and lipid order.10 However, in those experiments, a very narrow distribution of orientation needed to be assumed to retrieve the absolute orientation of the probe. Besides using chemical intuition, there are no other ways to experimentally determine such a property from steady-state SSHG. Therefore, we carried out a thorough analysis of Flipper-TR orientation in different membranes and surface tensions in order to provide a more solid basis for the interpretation of experimental data.
The angle between Flipper-TR transition dipole moment (TDM) and the normal at the surface, defined here as tilt angle or θ, was extracted from all simulated systems (Fig. 8a and Table S10, details on the analysis in ESI†). The mean of θ distribution varies substantially between disordered (θc = ∼22–38°) and ordered (θc = 14°) membranes. The decrease of tilt angle in more ordered environments was already reported in the literature by SSHG measurements.10 Flipper-TR orientation in DPPC at low temperature, however, represents a special case. The mean is centered at a higher value than in Lo phase (θc = 32.3° vs. 14°) due to the additional tilting of lipids in gel phase membranes. The most striking outcome from this analysis is that the distribution of tilt angles varies dramatically with lipid order. While a reasonably narrow distribution is predicted in DPPC and SM/CL at low temperature (full width at half maximum, FWHM = 10–11°), the broadening increases in SM/CL at higher temperature (FWHM = 24°) and in disordered membranes (FWHM = ∼34–40°), with Flipper-TR spanning a broad range of orientations in DOPC at 330 K (FWHM = 89°). Experimental evidences of such orientational broadening are provided by the strong and weak fluorescence anisotropies observed in imaging of Lo and Ld GUVs, respectively.102 This result is in contrast with the assumption of a very narrow distribution in the analysis of SSHG data.
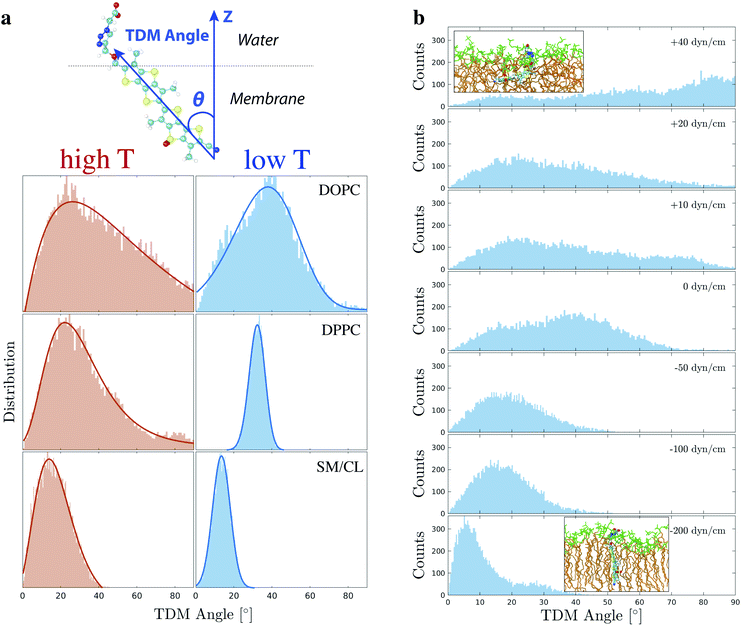 |
| Fig. 8 (a) The orientation of Flipper-TR was described by the transition dipole moment angle (θ), i.e. the angle between the probe transition dipole moment (TDM, blue vector along the molecule long axis) and the normal at the membrane (Z vector). A skewed Gaussian (solid line) was fit to the θ distribution in DOPC, DPPC (298 and 330 K), and SM (310 and 330 K). Optimized parameters are reported in Table S10.† (b) TDM angle was also extracted from simulation in DOPC (298 K) at different positive and negative surface tensions, corresponding to stretching and compression of the membrane respectively. The distribution at 0 dyn cm−1 is retrieved from the first 250 ns of the simulation in DOPC at 298 K (Fig. 3). All other systems were simulated for 250 ns after an initial equilibration of 40 ns at the target surface tension. Two representative snapshots with Flipper-TR orientation are shown for membranes at the highest and lowest surface tension (green: lipid head group, brown: lipid acyl chain). | |
Flipper-TR tilt angle absolute orientation and distribution also vary under different surface tensions (DOPC at 298 K, Fig. 8b). At a very negative surface tension, the tilt angle is mostly below 10° while at positive surface tensions it adopts a wide range of orientations. At the highest applied surface tension (+40 dyn cm−1, area per lipid = ∼85 Å2), Flipper-TR lies almost perpendicular to the interface due to the looseness of lipids in the membrane.
4 Conclusions
The challenge of measuring lateral forces in membranes has reached a turning point with the recent introduction of dithienothiophene-based mechanosensor flippers, which can discriminate between ordered and disordered lipid phases and are sensitive to surface tension. In this report, a combination of MD and QM simulations using a variety of membranes and under varying physicochemical conditions shows conclusive evidence that the mechanosensitivity of Flipper-TR, the presently best performing flipper, is associated with the degree of twisting between its two DTT flippers, switching between non-planar and planar conformations. In disordered environments, the twisting of the flippers generates a bimodal distribution, whereas in ordered environments, it collapses to a unimodal distribution closer to a planar conformation. Band shifts in simulated and experimental excitation spectra linked unequivocally the change in the mechanosensitive dihedral to the spectroscopic response of the probe, confirming the essential role of flippers twisting in the sensing process. The force field of Flipper-TR, which was accurately refined in order to reproduce quantum mechanical data, was further validated by an excellent agreement between simulated and experimental partition coefficients and excitation spectra.
The DOB-phase classification introduced for the analysis of ternary mixture membranes was used to distinguish lipid order in different regions of complex membranes by using the twist angle histograms as a differentiation criterion. In most MD simulations, the determination of lipid phases and the detection of nanoscopic domains rely on involved calculations and analyses, such as the calculation of deuterium order parameters, estimation of cumulative radial distribution functions86 or the use of sophisticated hidden Markov models.78 Besides being computationally demanding, these approaches may be sensitive to undulations and distortions of membranes, which can affect the accuracy of the calculation and introduce errors. Flipper-TR provides a general probe to directly sense lateral forces in complex membranes regardless of their shape or composition, specially in very large systems and mixed lipid bilayers where more traditional techniques might be either too expensive or inaccurate. Explicit inclusion of Flipper-TR might of course introduce undesired perturbation to the system, particularly in small patches of lipid bilayers. However, its main advantage is to provide a very easy and fast metric to measure membrane order, as the mechanosensitivity relies on a single internal degree of freedom. Thus, specific lateral forces are always converted into the same twist angle distribution, and consequently into the same spectroscopic response, as shifts in excitation depend solely on the degree of planarization of the flipper. The use of Flipper-TR in simulations may become especially beneficial for very large membranes with complex lipid compositions and for on the fly lipid order detection, since no a priori knowledge of the lipid nature is required.
Of particular interest is tension-induced lipid membrane reorganization. Domain formation and disassembly have been identified to dominate the response of Flipper-TR in living cells. These processes promise to have high biological significance. Mechanical control over signal transduction, for example, has already been demonstrated using Flipper-TR.12 The identified responsiveness to surface tension indicates that computational simulations might provide access to a deeper understanding of these important yet complex processes.
Here, our simulations show that the assumption of a very narrow tilt angle distribution for Flipper-TR is too simplistic and can result in inaccurate absolute orientations by SSHG measurements in very fluid membranes. A narrow distribution is observed only in highly ordered bilayers, and a broad one in systems with higher lipid disorder. Such critical information predicted by our atomistic simulations is crucial to accurately obtain the true orientation of the probe.
Flippers present new tools to investigate lipid order and nanodomains in biological membranes using both experimental techniques and MD simulations, as shown by results in this study. In future computational works, Flipper-TR can allow the investigation of domain formation in other physicochemical systems and in various biological environments, such as in proximity of membrane proteins that induce the formation of functional rafts.3,103 The DOB-phase classification introduced here can be extended to other mechanosensitive probes where other degree of freedoms may be responsible for the sensitivity.
Conflicts of interest
Flipper-TR® is commercially available from Spirochrome, through the NCCR Store (https://nccr-chembio.ch/technologies/nccr-store/). The NCCR receives 15% of the revenues.
Acknowledgements
The authors thank the National Institutes of Health (NIH P41-GM104601 and R01-GM123455 to E. T.), the University of Geneva, the Swiss National Centre of Competence in Research (NCCR) Chemical Biology, the NCCR Molecular System Engineering and the Swiss NSF (to S. M.) for financial support. All the simulations were performed using resources provided by NCSA Blue Waters and XSEDE allocations to E. T. (Grant MCA06N060).
Notes and references
- D. Casares, P. V. Escribá and C. A. Rosselló, Int. J. Mol. Sci., 2019, 20, 2167 CrossRef CAS PubMed.
- K. Jacobson, P. Liu and B. C. Lagerholm, Cell, 2019, 177, 806–819 CrossRef CAS PubMed.
- D. Lingwood and K. Simons, Science, 2010, 327, 46–50 CrossRef CAS PubMed.
- W. Rawicz, B. A. Smith, T. J. McIntosh, S. A. Simon and E. Evans, Biophys. J., 2008, 94, 4725–4736 CrossRef CAS PubMed.
- A. S. Klymchenko and R. Kreder, Chem. Biol., 2014, 21, 97–113 CrossRef CAS PubMed.
- A. Fin, A. Vargas Jentzsch, N. Sakai and S. Matile, Angew. Chem., Int. Ed. Engl., 2012, 51, 12736–12739 CrossRef CAS PubMed.
- M. Dal Molin, Q. Verolet, A. Colom, R. Letrun, E. Derivery, M. Gonzalez-Gaitan, E. Vauthey, A. Roux, N. Sakai and S. Matile, J. Am. Chem. Soc., 2015, 137, 568–571 CrossRef CAS PubMed.
- Q. Verolet, A. Rosspeintner, S. Soleimanpour, N. Sakai, E. Vauthey and S. Matile, J. Am. Chem. Soc., 2015, 137, 15644–15647 CrossRef CAS PubMed.
- S. Soleimanpour, A. Colom, E. Derivery, M. Gonzalez-Gaitan, A. Roux, N. Sakai and S. Matile, Chem. Commun., 2016, 52, 14450–14453 RSC.
- G. Licari, J. S. Beckwith, S. Soleimanpour, S. Matile and E. Vauthey, Phys. Chem. Chem. Phys., 2018, 20, 9328–9336 RSC.
- K. Strakova, S. Soleimanpour, M. Diez-Castellnou, N. Sakai and S. Matile, Helv. Chim. Acta, 2018, 101, e1800019 CrossRef.
- M. Riggi, K. Niewola-Staszkowska, N. Chiaruttini, A. Colom, B. Kusmider, V. Mercier, S. Soleimanpour, M. Stahl, S. Matile, A. Roux and R. Loewith, Nat. Cell Biol., 2018, 20, 1043–1051 CrossRef CAS PubMed.
- A. Goujon, A. Colom, K. Strakova, V. Mercier, D. Mahecic, S. Manley, N. Sakai, A. Roux and S. Matile, J. Am. Chem. Soc., 2019, 141, 3380–3384 CrossRef CAS PubMed.
- A. Goujon, K. Strakova, N. Sakai and S. Matile, Chem. Sci., 2019, 10, 310–319 RSC.
- A. S. Klymchenko, Acc. Chem. Res., 2017, 50, 366–375 CrossRef CAS PubMed.
- K. Strakova, A. I. Poblador-Bahamonde, N. Sakai and S. Matile, Chem.–Eur. J., 2019, 25, 14935–14942 CrossRef CAS PubMed.
- M. P. Muller, T. Jiang, C. Sun, M. Lihan, S. Pant, P. Mahinthichaichan, A. Trifan and E. Tajkhorshid, Chem. Rev., 2019, 119, 6086–6161 CrossRef CAS PubMed.
- G. Enkavi, M. Javanainen, W. Kulig, T. Róg and I. Vattulainen, Chem. Rev., 2019, 119, 5607–5774 CrossRef CAS PubMed.
- S. J. Marrink, V. Corradi, P. C. Souza, H. I. Ingólfsson, D. P. Tieleman and M. S. Sansom, Chem. Rev., 2019, 119, 6184–6226 CrossRef CAS PubMed.
- M. Cebecauer, M. Amaro, P. Jurkiewicz, M. J. Sarmento, R. Šachl, L. Cwiklik and M. Hof, Chem. Rev., 2018, 118, 11259–11297 CrossRef CAS PubMed.
- L. Zhu, Q. Cui, H. Xiao, X. Liao and X. Chen, J. Mech. Behav. Biomed. Mater., 2019, 90, 502–514 CrossRef CAS PubMed.
- Y. Miyazaki, S. Okazaki and W. Shinoda, Biochim. Biophys. Acta, Biomembr., 2019, 1861, 1409–1419 CrossRef CAS PubMed.
- M. Vögele, R. M. Bhaskara, E. Mulvihill, K. van Pee, Ö. Yildiz, W. Kühlbrandt, D. J. Müller and G. Hummer, Proc. Natl. Acad. Sci. U. S. A., 2019, 116, 13352–13357 CrossRef PubMed.
- C. Allolio, A. Magarkar, P. Jurkiewicz, K. Baxová, M. Javanainen, P. E. Mason, R. Šachl, M. Cebecauer, M. Hof, D. Horinek, V. Heinz, R. Rachel, C. M. Ziegler, A. Schröfel and P. Jungwirth, Proc. Natl. Acad. Sci. U. S. A., 2018, 115, 11923–11928 CrossRef CAS PubMed.
- Y. Shi, M. Wan, L. Fu, S. Zhang, S. Wang, L. Gao and W. Fang, Biophys. J., 2018, 115, 1518–1529 CrossRef CAS PubMed.
- V. H. Man, P. M. Truong, M. S. Li, J. Wang, N.-T. Van-Oanh, P. Derreumaux and P. H. Nguyen, J. Phys. Chem. B, 2018, 123, 71–78 CrossRef PubMed.
- W. F. D. Bennett, C. K. Hong, Y. Wang and D. P. Tieleman, J. Chem. Theory Comput., 2016, 12, 4524–4533 CrossRef CAS PubMed.
- L. Cwiklik and P. Jungwirth, Chem. Phys. Lett., 2010, 486, 99–103 CrossRef CAS.
- L. Thøgersen, B. Schiøtt, T. Vosegaard, N. C. Nielsen and E. Tajkhorshid, Biophys. J., 2008, 95, 4337–4347 CrossRef PubMed.
- F. Elías-Wolff, M. Lindén, A. P. Lyubartsev and E. G. Brandt, Soft Matter, 2019, 15, 792–802 RSC.
- A. Belessiotis-Richards, S. G. Higgins, B. Butterworth, M. M. Stevens and A. Alexander-Katz, Nano Lett., 2019, 19, 4770–4778 CrossRef CAS PubMed.
- P.-C. Hsu, F. Samsudin, J. Shearer and S. Khalid, J. Phys. Chem. Lett., 2017, 8, 5513–5518 CrossRef CAS PubMed.
- G. C. A. da Hora, N. L. Archilha, J. L. S. Lopes, D. M. Müller, K. Coutinho, R. Itri and T. A. Soares, Soft Matter, 2016, 12, 8884–8898 RSC.
- D. S. Patel, S. Park, E. L. Wu, M. S. Yeom, G. Widmalm, J. B. Klauda and W. Im, Biophys. J., 2016, 111, 1987–1999 CrossRef CAS PubMed.
- A. Sodt, R. Venable, E. Lyman and R. Pastor, Phys. Rev. Lett., 2016, 117, 138104 CrossRef CAS PubMed.
- H. Cui, E. Lyman and G. A. Voth, Biophys. J., 2011, 100, 1271–1279 CrossRef CAS PubMed.
- H. T. McMahon and J. L. Gallop, Nature, 2005, 438, 590–596 CrossRef CAS PubMed.
- Q. Cui, J. Gen. Physiol., 2018, 150, 777–780 CrossRef CAS PubMed.
- E. J. Spangler, P. B. S. Kumar and M. Laradji, Soft Matter, 2018, 14, 5019–5030 RSC.
- S. Sharma, B. N. Kim, P. J. Stansfeld, M. S. Sansom and M. Lindau, PLoS One, 2015, 10, e0144814 CrossRef PubMed.
- A. Debnath and L. V. Schäfer, J. Phys. Chem. B, 2015, 119, 6991–7002 CrossRef CAS PubMed.
- Å. A. Skjevik, B. D. Madej, C. J. Dickson, K. Teigen, R. C. Walker and I. R. Gould, Chem. Commun., 2015, 51, 4402–4405 RSC.
- A. N. Leonard, E. Wang, V. Monje-Galvan and J. B. Klauda, Chem. Rev., 2019, 119, 6227–6269 CrossRef CAS PubMed.
- J. S. Smith, O. Isayev and A. E. Roitberg, Chem. Sci., 2017, 8, 3192–3203 RSC.
- J. C. Phillips, R. Braun, W. Wang, J. Gumbart, E. Tajkhorshid, E. Villa, C. Chipot, R. D. Skeel, L. Kale and K. Schulten, J. Comput. Chem., 2005, 26, 1781–1802 CrossRef CAS PubMed.
- K. Hart, N. Foloppe, C. M. Baker, E. J. Denning, L. Nilsson and A. D. Mackerell Jr, J. Chem. Theory Comput., 2012, 8, 348–362 CrossRef CAS PubMed.
- J. B. Klauda, R. M. Venable, J. A. Freites, J. W. O'Connor, D. J. Tobias, C. Mondragon-Ramirez, I. Vorobyov, A. D. MacKerell Jr and R. W. Pastor, J. Phys. Chem. B, 2010, 114, 7830–7843 CrossRef CAS PubMed.
- W. Jorgensen, J. Chandrasekhar, J. D. Maudura, R. W. Impey and M. L. Klein, J. Chem. Phys., 1983, 79, 926–935 CrossRef CAS.
- K. Vanommeslaeghe, E. Hatcher, C. Acharya, S. Kundu, S. Zhong, J. Shim, E. Darian, O. Guvench, P. Lopes, I. Vorobyov and A. D. MacKerell Jr, J. Comput. Chem., 2010, 31, 671–690 CAS.
- C. G. Mayne, J. Saam, K. Schulten, E. Tajkhorshid and J. C. Gumbart, J. Comput. Chem., 2013, 34, 2757–2770 CrossRef CAS PubMed.
- W. Humphrey, A. Dalke and K. Schulten, J. Mol. Graphics, 1996, 14, 33–38 CrossRef CAS PubMed.
- J. Lee, X. Cheng, J. M. Swails, M. S. Yeom, P. K. Eastman, J. A. Lemkul, S. Wei, J. Buckner, J. C. Jeong, Y. Qi, S. Jo, V. S. Pande, D. A. Case, C. L. Brooks III, A. D. MacKerell Jr, J. B. Klauda and W. Im, J. Chem. Theory Comput., 2016, 12, 405–413 CrossRef CAS PubMed.
- T. Darden, D. York and L. Pedersen, J. Chem. Phys., 1993, 98, 10089–10092 CrossRef CAS.
- J.-P. Ryckaert, G. Ciccotti and H. J. C. Berendsen, J. Comput. Phys., 1977, 23, 327–341 CrossRef CAS.
- G. J. Martyna, D. J. Tobias and M. L. Klein, J. Chem. Phys., 1994, 101, 4177–4189 CrossRef CAS.
- S. E. Feller, Y. Zhang and R. W. Pastor, J. Chem. Phys., 1995, 103, 4613–4621 CrossRef CAS.
- P. Khakbaz and J. B. Klauda, Biochim. Biophys. Acta, Biomembr., 2018, 1860, 1489–1501 CrossRef CAS PubMed.
- E. Wang and J. B. Klauda, J. Phys. Chem. B, 2017, 121, 4833–4844 CrossRef CAS PubMed.
- T. Bartels, R. S. Lankalapalli, R. Bittman, K. Beyer and M. F. Brown, J. Am. Chem. Soc., 2008, 130, 14521–14532 CrossRef CAS PubMed.
- R. Guixa-Gonzalez, I. Rodriguez-Espigares, J. M. Ramirez-Anguita, P. Carrio-Gaspar, H. Martinez-Seara, T. Giorgino and J. Selent, Bioinformatics, 2014, 30, 1478–1480 CrossRef CAS PubMed.
- B. Roux, Comput. Phys. Commun., 1995, 91, 275–282 CrossRef CAS.
- B. Roux, Biophys. J., 1999, 77, 139–153 CrossRef CAS PubMed.
- H. Grubmüller, B. Heymann and P. Tavan, Science, 1996, 271, 997–999 CrossRef PubMed.
- S. Izrailev, S. Stepaniants, M. Balsera, Y. Oono and K. Schulten, Biophys. J., 1997, 72, 1568–1581 CrossRef CAS PubMed.
- S. Kumar, J. M. Rosenberg, D. Bouzida, R. H. Swendsen and P. A. Kollman, J. Comput. Chem., 1992, 13, 1011–1021 CrossRef CAS.
-
A. Grossfield, WHAM: the weighted histogram analysis method, version 2.0.9, 2013, http://membrane.urmc.rochester.edu/wordpress/?page_id=126 Search PubMed.
-
M. J. Frisch, G. W. Trucks, H. B. Schlegel, G. E. Scuseria, M. A. Robb, J. R. Cheeseman, G. Scalmani, V. Barone, G. A. Petersson, H. Nakatsuji, X. Li, M. Caricato, A. Marenich, J. Bloino, B. G. Janesko, R. Gomperts, B. Mennucci, H. P. Hratchian, J. V. Ortiz, A. F. Izmaylov, J. L. Sonnenberg, D. Williams-Young, F. Ding, F. Lipparini, F. Egidi, J. Goings, B. Peng, A. Petrone, T. Henderson, D. Ranasinghe, V. G. Zakrzewski, J. Gao, N. Rega, G. Zheng, W. Liang, M. Hada, M. Ehara, K. Toyota, R. Fukuda, J. Hasegawa, M. Ishida, T. Nakajima, Y. Honda, O. Kitao, H. Nakai, T. Vreven, K. Throssell, J. A. Montgomery Jr, J. E. Peralta, F. Ogliaro, M. Bearpark, J. J. Heyd, E. Brothers, K. N. Kudin, V. N. Staroverov, T. Keith, R. Kobayashi, J. Normand, K. Raghavachari, A. Rendell, J. C. Burant, S. S. Iyengar, J. Tomasi, M. Cossi, J. M. Millam, M. Klene, C. Adamo, R. Cammi, J. W. Ochterski, R. L. Martin, K. Morokuma, O. Farkas, J. B. Foresman, and D. J. Fox, Gaussian 09, Revision B and D, Gaussian, Inc., Wallingford CT, 2016 Search PubMed.
- T. Yanai, D. P. Tew and N. C. Handy, Chem. Phys. Lett., 2004, 393, 51–57 CrossRef CAS.
- R. Bauernschmitt and R. Ahlrichs, Chem. Phys. Lett., 1996, 256, 454–464 CrossRef CAS.
- R. E. Stratmann, G. E. Scuseria and M. J. Frisch, J. Chem. Phys., 1998, 109, 8218 CrossRef CAS.
- G. Licari, L. Cwiklik, P. Jungwirth and E. Vauthey, Langmuir, 2017, 33, 3373–3383 CrossRef CAS PubMed.
- M. W. Baig, M. Pederzoli, P. Jurkiewicz, L. Cwiklik and J. Pittner, Molecules, 2018, 23, 1707 CrossRef PubMed.
- J. L. Palma, E. Atas, L. Hardison, T. B. Marder, J. C. Collings, A. Beeby, J. S. Melinger, J. L. Krause, V. D. Kleiman and A. E. Roitberg, J. Phys. Chem. C, 2010, 114, 20702–20712 CrossRef CAS.
- Z. L. Cai, M. J. Crossley, J. R. Reimers, R. Kobayashi and R. D. Amos, J. Phys. Chem. B, 2006, 110, 15624–15632 CrossRef CAS PubMed.
- A. Savitzky and M. J. E. Golay, Anal. Chem., 1964, 36, 1627–1639 CrossRef CAS.
- R. L. Biltonen and D. Lichtenberg, Chem. Phys. Lipids, 1993, 64, 129–142 CrossRef CAS.
- A. S. Ulrich, M. Sami and A. Watts, Biochim. Biophys. Acta, Biomembr., 1994, 1191, 225–230 CrossRef CAS.
- A. J. Sodt, M. L. Sander, K. Gawrisch, R. W. Pastor and E. Lyman, J. Am. Chem. Soc., 2014, 136, 725–732 CrossRef CAS PubMed.
- A. J. Sodt, R. W. Pastor and E. Lyman, Biophys. J., 2015, 109, 948–955 CrossRef CAS PubMed.
- P. F. Rusch and J. P. Lelieur, Anal. Chem., 1973, 45, 1541–1543 CrossRef CAS.
- A. Bahramian, Thin Solid Films, 2015, 592, 39–53 CrossRef CAS.
- A. Colom, E. Derivery, S. Soleimanpour, C. Tomba, M. D. Molin, N. Sakai, M. Gonzalez-Gaitan, S. Matile and A. Roux, Nat. Chem., 2018, 10, 1118–1125 CrossRef CAS PubMed.
- J. Gullingsrud, D. Kosztin and K. Schulten, Biophys. J., 2001, 80, 2074–2081 CrossRef CAS PubMed.
- J. Gullingsrud and K. Schulten, Biophys. J., 2003, 85, 2087–2099 CrossRef CAS PubMed.
- G. M'Baye, Y. Mély, G. Duportail and A. S. Klymchenko, Biophys. J., 2008, 95, 1217–1225 CrossRef PubMed.
- T. S. Carpenter, C. A. López, C. Neale, C. Montour, H. I. Ingólfsson, F. Di Natale, F. C. Lightstone and S. Gnanakaran, J. Chem. Theory Comput., 2018, 14, 6050–6062 CrossRef CAS PubMed.
- M. Podewitz, Y. Wang, P. Gkeka, S. Von Grafenstein, K. R. Liedl and Z. Cournia, J. Phys. Chem. B, 2018, 122, 10505–10521 CrossRef CAS PubMed.
- H. I. Ingólfsson, M. N. Melo, F. J. van Eerden, C. Arnarez, C. A. López, T. A. Wassenaar, X. Periole, A. H. de Vries, D. P. Tieleman and S. J. Marrink, J. Am. Chem. Soc., 2014, 136, 14554–14559 CrossRef.
- H. J. Risselada and S. J. Marrink, Proc. Natl. Acad. Sci. U. S. A., 2008, 105, 17367–17372 CrossRef CAS PubMed.
- M. J. Stevens, J. Am. Chem. Soc., 2005, 127, 15330–15331 CrossRef CAS PubMed.
- G. A. Pantelopulos, T. Nagai, A. Bandara, A. Panahi and J. E. Straub, J. Chem. Phys., 2017, 147, 095101 CrossRef PubMed.
- P. S. Niemelä, S. Ollila, M. T. Hyvönen and I. Vattulainen, PLoS Comput. Biol., 2007, 3, e34 CrossRef PubMed.
- M. Adams, E. Wang, X. Zhuang and J. B. Klauda, Biochim. Biophys. Acta, Biomembr., 2018, 1860, 2134–2144 CrossRef CAS PubMed.
- S. A. Pandit, E. Jakobsson and H. L. Scott, Biophys. J., 2004, 87, 3312–3322 CrossRef CAS PubMed.
- M. Javanainen, H. Martinez-Seara and I. Vattulainen, Sci. Rep., 2017, 7, 1143 CrossRef PubMed.
- S. A. Pandit, S. Vasudevan, S. W. Chiu, R. J. Mashl, E. Jakobsson and H. L. Scott, Biophys. J., 2004, 87, 1092–1100 CrossRef CAS PubMed.
- P. I. Kuzmin, S. A. Akimov, Y. A. Chizmadzhev, J. Zimmerberg and F. S. Cohen, Biophys. J., 2005, 88, 1120–1133 CrossRef CAS PubMed.
- H. A. Rinia, M. M. Snel, J. P. Van Der Eerden and B. De Kruijff, FEBS Lett., 2001, 501, 92–96 CrossRef CAS PubMed.
- M. J. Swain and D. H. Ballard, Int. J. Comput. Vis., 1991, 7, 11–32 CrossRef.
- Q. Wei, D. Zhou and H. Bian, Phys. Chem. Chem. Phys., 2018, 20, 11758–11767 RSC.
- D. Headley, R. S. Young, M. Reece and M. Subir, J. Phys. Chem. C, 2018, 122, 4945–4954 CrossRef CAS.
- M. Macchione, A. Goujon, K. Strakova, H. V. Humeniuk, G. Licari, E. Tajkhorshid, N. Sakai and S. Matile, Angew. Chem., Int. Ed. Engl., 2019, 58, 15752–15756 CrossRef CAS PubMed.
- E. Sezgin, I. Levental, S. Mayor and C. Eggeling, Nat. Rev. Mol. Cell Biol., 2017, 18, 361–374 CrossRef CAS PubMed.
Footnote |
† Electronic supplementary information (ESI) available: Details on the refinement of Flipper-TR force field and relative files, analysis of membranes, fitting procedures of dihedral distributions, analysis of ternary mixtures, additional experimental materials and methods, movie captions. See DOI: 10.1039/d0sc02175j |
|
This journal is © The Royal Society of Chemistry 2020 |